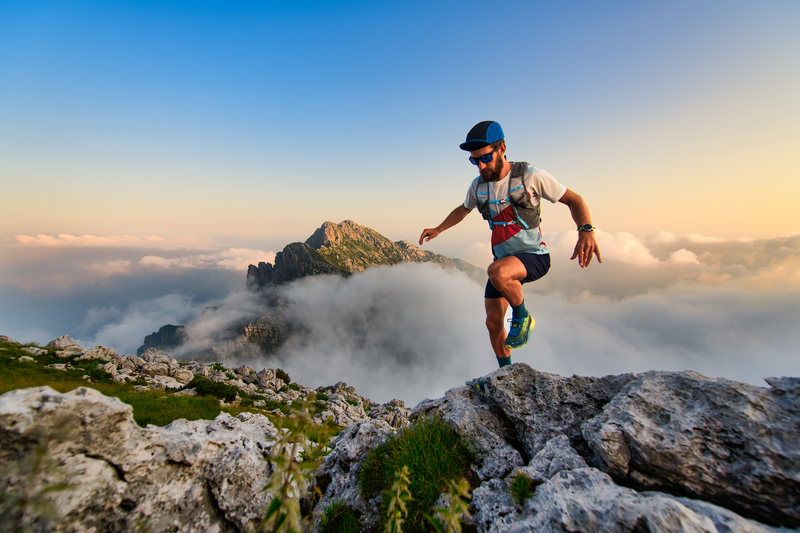
95% of researchers rate our articles as excellent or good
Learn more about the work of our research integrity team to safeguard the quality of each article we publish.
Find out more
ORIGINAL RESEARCH article
Front. Environ. Sci. , 31 August 2022
Sec. Environmental Economics and Management
Volume 10 - 2022 | https://doi.org/10.3389/fenvs.2022.951262
This article is part of the Research Topic Green Energy Technologies for Environmental Sustainability: Drivers, Challenges, and Future Aspects View all 27 articles
Traditional community support, prevention from disasters, and mitigation depend on the top-down mode of management regulated by the government. This study aims to analyze the association between social networks and its structure on the decision of households’ participation in community. A households survey was conducted in Yanliang district of Shaanxi province of China and 393 households were targeted. An ordered multi classification logistic regression model was utilized to empirically analyze the association between the variables. The results showed that social networks have a significant positive impact on the households’ participation in cooperatives/communities. Among other variables, network interaction, network learning, network trust, and households’ participation in green energy technologies and cooperatives/community showed significant and positive correlation, but the variable network reciprocity was found to be negatively correlated to the adoption of green energy technologies. Study findings further revealed that, there is an interaction effect in the participation of households’ behavioral decision-making and joining cooperatives. Furthermore, the social experience of the head of the household and the source of income of the family showed a significant positive association with households’ participation in cooperatives/communities. Based on the study findings, it is suggested that the utilization and cultivation of households’ social network, service level of cooperatives need to be improved.
At present, the contradiction between small farmers and the large market presented by the simple production and operation mode of farmers’ households is increasingly prominent. The problem of farmer cooperation is considered an important way to solve rural problems and is even considered a “modernization” in the process of rural development. The only way to go is having a relatively stable cooperative relationship that can bring the effective continuity of cooperative benefits of farmers. The cooperative economic organization established by the cooperative based on members of the peasant household is an effective carrier connecting small peasant households with the big market and plays an important role in the development of the rural economy (Hakelius and Hansson 2016; Dan and Zimin 2017; Sun et al., 2022a), which improves the degree of organization and welfare of agricultural production and operation level (Hind 1997; Wang and Li 2011; Zhigang et al., 2017), exerted internal and external service functions (Cook 1995), saved transaction costs (Li and Ito 2021; Irfan and Ahmad 2022), realized factor integration and economies of scale (Pokharel and Featherstone 2019), increased farmers’ income (Yang and Liu 2012; Ma and Abdulai 2016), and improved agricultural production. Production efficiency (LeVay 1983) solves the contradiction between “small farmers” and “big market,” conforms to the development trend of the market economy, and meets the development requirements of China’s modern agriculture. At the end of November 2021, there were 2.219 million cooperatives of farmers legally registered in China and almost half of the farmer households were driven by radiation. However, the current level of farmer household cooperation is not deep enough, the scale of cooperation is generally small, the internal structure is relatively simple (Baoyu 2015; Jan et al., 2021), the organization is losing the service-driven ability. Problems such as being weak and limited (Liao et al., 2016; Zhong et al., 2016) have seriously affected the development of cooperatives and their functions, and the low participation and willingness of members in the development of cooperatives is still an important problem that plagues the development of cooperatives (Chen et al., 2019; Javed et al., 2022). Keeping the following under consideration, this paper believes that the existing cooperative mechanism of cooperatives has an extremely limited effect on stimulating farmers’ cooperative behavior, and there is the possibility of “mechanism failure”. It should be considered from the perspective of endogenous demand to explore the formation mechanism of farmers’ participation in cooperative production.
In a typical rural social background, the social network of farmers is formed mainly based on the kinship, blood and geographical relationship between farmers, resulting in the social interaction and lifestyle of farmers, which are deeply affected by their social network (Hong 2016; Sun et al., 2022b; Nureen et al., 2022), especially in farmers’ families as well as production and life decision-making behavior (Warriner and Moul 1992; Watts and Strogatz, 1998; Miao 2014; Su et al., 2022). In recent years, more and more scholars have paid attention and studied the role of social capital of farmers in their behavioral decision making. Judging from the existing literature, most studies focus on social trust, while ignoring the explanatory power of social networks on farmers’ participation in cooperative decision-making; a few scholars have systematically examined its impact on farmers’ participation in cooperative decision-making from the perspective of social capital, but lack of depth.
Keeping above into consideration, this paper draws on existing research, based on the perspective of farmers’ social network, uses micro-farmer survey data in Yanliang District, Shaanxi Province in 2019, and uses the binary logistic regression analysis method to analyze farmers’ social network and its network interaction and network learning. There are four dimensions of network reciprocity and network trust. First, it discusses its influence on farmers’ participation in cooperative behavior decision-making. Second, it analyzes the interaction effect of the social network and income level on farmers’ participation in cooperative behavior decision making. This has important guiding significance for understanding and grasping the influence mechanism of the social network and its structure on farmers’ participation in cooperative behavior decision making and reasonably guiding farmers to participate in cooperatives. It can enrich existing research content and provide more references for future research.
The social network refers to a relationship network formed by members through interaction (Granovetter 1995). The members of the network have a high degree of trust and share various information resources and knowledge through mutual learning and communication. There is a “differential pattern” of social relations in China, and farmers’ production and living decisions are often affected by the social network in which they are located. The social network can help farmers alleviate the constraints caused by information asymmetry through the acquisition of information and other resources, and promote the use of information. Diffusion improves farmers’ cognition level, thereby reducing the uncertainty in farmers’ decision-making. The higher the level of the social network, the more favorable it is for farmers to obtain more corresponding resources, thus enhancing the enthusiasm of farmers to participate in collective activities (Lu et al., 2017; Fahad and Jing 2018). Therefore, with the help of the social network, it is beneficial to change the attitude and behavior of farmers. Based on the theory of social networks, this paper draws on relevant research results (Wang and Lu 2015; Wang et al., 2019; Fahad et al., 2022a) and divides the social network of farmers into four dimensions: network interaction, network learning, network reciprocity, and network trust.
Network learning is the most important function of the social network, which exists in every moment of farmers’ lives (Fahad and Wang, 2018; Liu 2019). Members acquire knowledge and information, accumulate experience through learning, communication and problem solving together and through the network learning effect. The resources flowing in the medium are used to achieve self-improvement and achieve their own goals. Therefore, when farmers learn about cooperatives from other members of the social network, especially from major farmers, technicians and relevant government staff, they will deepen their understanding of cooperatives. When benefiting, it will enhance their willingness to join the society, thereby affecting their own behavior of joining the society.
Network interaction reflects the degree of mutual communication and connection between members of a relationship, emphasizing that members achieve certain goals through language or behavioral contact. Network interaction can promote the communication of network members and the diffusion of information and technology. The interaction between farmers and members of the network is also a learning process (Wang and Lu 2015; Fahad et al., 2022b), which improves the level of cognition of cooperatives and affects their behavior in choosing to join cooperatives.
Network reciprocity reflects the quality of the social network (Liu 2019), which means that network members use network relationships to coordinate and cooperate with each other’s interests. It not only reflects the willingness and degree of reciprocity between individuals, but also reflects the degree of reciprocity of the individual’s status (Wang et al., 2019; Su et al., 2021). Network reciprocity enables members of the network to get the help they need by helping each other, exchanging information etc. and mainly through the information acquisition and transmission mechanism to speed up the spread of cooperative information, and to accelerate farmers’ learning of cooperative-related knowledge, so as to improve farmers’ knowledge. The cognition of cooperatives will ultimately affect the decision-making of farmers’ production and living behaviors.
Network trust refers to the implicit understanding and mutual trust formed by network members in long-term exchanges or potential resource collection (Cai and Han 2012). It reflects the degree of trust between members in mutual exchanges and allows individuals to evaluate the actions of other individuals, thereby affecting their own behavior, specifically the degree of recognition of the behavior and actions of other members within the network, thereby imitating the behavior of its trusted members. Trust is the driving force to maintain sustainable economic development. The higher the level of trust in the network of farmers, the easier it is to achieve cooperation. From the perspective of reducing transaction costs, network trust can reduce transaction costs (Wang et al., 2019) and facilitate the achievement of cooperation. Whether a farmer chooses to participate in a cooperative will be particularly affected by the behavior of the farmer he trusts. The greater the degree of trust, the greater the influence of other members (Hansen et al., 2002). From the perspective of reducing communication costs, mutual trust among farmers can effectively reduce communication costs and reduce barriers of cooperation between farmers. To a certain extent, it determines whether farmers are willing to follow the advice of others to change their actions and promote the realization of collective action (Cai and Zhu 2017). Therefore, this paper proposes the following research hypotheses:
Hypothesis H1. The social network has a significant positive impact on the choice of farmers to participate in cooperatives.
Hypothesis H2. Online learning has a significant positive impact on farmers’ choice to participate in cooperatives.
Hypothesis H3. Network interaction has a significant positive impact on farmers’ choice to participate in cooperatives.
Hypothesis H4. Network reciprocity has a significant positive impact on farmers choosing to participate in cooperatives.
Hypothesis H5. Network trust has a significant positive impact on farmers choosing to participate in cooperatives.
Social networks play an important role in helping farmers improve their income levels. They can alleviate poverty, share risks, and improve income levels. Quantification shows that social networks contribute 12.1–13.4% to rural income gaps (Zhao and Lu 2010; Sun and Razzaq 2022). Qualitative social networks improve the income status of poor farmers more obviously (Campbell et al., 1986; Krackhardt 1995; Lai et al., 1998; Ye and Zhou 2010). From the perspective of social capital, Liang et al. (2014) found that social capital is beneficial for farmers to join cooperatives and has a significant positive impact on the income level of members. From the perspective of influence mechanism, social networks can influence individuals’ human capital accumulation, occupation, and capital lending through mechanisms such as sharing information, sharing risks, and improving collective decision making, thus alleviating poverty and increasing income levels. Research by Ma and Yang (2011) showed that farmers obtain the necessary entrepreneurial capital through social networks, and then promote the development of self-operated industry and commerce, so as to achieve the improvement of income level. However, the formation and expansion of social networks requires not only time and energy, but also financial support, so income levels also play an important role in maintaining and consolidating social networks. (Wallace and Bassuk 1991; D'Angelo and Lilla 2011). study found that income level affects the construction of social networks, high-income families are more able to build and expand social network, on the contrary, low-income families hope to increase income through network construction.
There is a dialectical relationship between the economic base and the superstructure. Joining a cooperative has an impact on the income level of farmers, and the income level of farmers also affects whether they join the cooperative. The family income of farmers has an important influence on their participation in cooperatives. Whether cooperatives can increase the agricultural income of farmers who join cooperatives is the key factor for farmers to choose for joining cooperatives (Zhang and Zhou, 2013; Li et al., 2015). In other words, the income level of farmers not only affects the construction of a social network, but also directly affects the behavior of farmers who join the society. The higher the income level of the farmers, the higher the level of social network, and the more they can play their role in information sharing, risk sharing, and improving collective decision making. Their decision will be joining a cooperative. Therefore, this paper proposes the following research hypotheses:
Hypothesis H6. The income level of farmers has an interactive effect on the impact of social networks on farmers’ participation in cooperative decision-making.
Data used in this article was collected by the research team from July to August 2019, through the analysis of five villages including Wutun town and Guanshan town in Yanling district, Shaanxi Province, two villages in Yabai town and Louguan town in Zhouzhi county, Xianyang, 10 villages in Dazhai town, Nugu town, Wuquan town and Yangcun town in Yanliang district of the city, and 1 village in Wugong town in Wugong county were surveyed through household interviews and questionnaires. Respondents were farmers who had no communication barriers and were willing to cooperate. A total of 420 questionnaires were distributed and 420 volumes were recovered, of which 393 were valid questionnaires, with an effective rate of 93.81%.
In this survey sample, from the perspective of age structure, the age of the head of the household is mainly older than 50 years old; from the perspective of gender distribution, the proportion of men is relatively large, specifically, males account for 62.65%, and females account for 37.35%. In terms of education level, 44.16% of households with junior high school education represented the highest proportion, and 4.82% of households with college education or above were the least; 12.47% were party members, and 87.53% were not party members. Physical condition of the subjects surveyed is mainly healthy, accounting for 51.52%; the average farmland area of the surveyed households is 6.88 mu; the number of household labor is mainly 2-3 people, accounting for 69.72%. The main source of living of the surveyed households 43.78% went out to work, followed by farming accounts for 37.4%; agriculture was the main business type of farmers, accounting for 57.00%, followed by agriculture-based part-time jobs, accounting for 29.50%. In general, the basic characteristics of the sample farmers are more consistent with the rural situation in my country.
The independent variable of this paper is the social network and the assessment of its measurement variable uses SPSS 25.0 software and adopts the factor analysis method. First, Gronbach’s α coefficient was used to test the reliability of the questionnaire, and the KMO test and the Bartlett sphericity test were used to judge the applicability of factor analysis. Among them, the overall Gronbach’s α value is 0.841 and greater than 0.5, indicating that the questionnaire has good reliability; the KMO test value of the social network variables is 0.814 greater than 0.5, indicating that the factor analysis effect is good; the significance level of the Bartlett sphericity test value is 0.00 and less than 0.05, indicating that the characterization indicators and sample data used in this article have passed the KMO and Bartlett tests, which are very suitable for factor analysis. The test results are shown in Table 1, Table 2. Then, using the principal component factor analysis method, according to the principle that the eigenvalue is greater than 1, and rotated by the maximum variance method, the cumulative variance contribution rate is 70.472%, and a total of four common factors are extracted. According to the factor load scores, the four factors obtained by dimensionality reduction are named as network learning (f1), network interaction (f2), network reciprocity (f3), and network trust (f4). Based on the scores of these four common factors and their variance contribution rates, the calculation formula of the value of the social network index is the following.
where, SN represents the social network.
The dependent variable in this paper is the farmers’ behavior of joining the cooperative, which is a binary variable (yes = 1, no = 0). It is measured by the question “whether you join a cooperative”. Among them, 65 households participate in a cooperative, accounting for 16.54%, 328 households did not participate in cooperatives, accounting for 83.46%.
Based on previous studies (Liu J., 2017), this paper divides the selected control variables into three parts:
i. Farmer individual characteristics: Such as age, gender, education level, health status, party membership, social experience, and risk preference of the household head.
ii. Family characteristics: Such as family population, labor force, cultivated land area, whether family members serve as cadres, social status, number of contacts, main source of income, business type and income level.
iii. The characteristics of the village environment: Including the operation status of rules and regulations in the village and the relationship between villagers.
Since there are many independent variables involved in this paper, in order to avoid the problem of multicollinearity between variables, this paper conducts a multicollinearity test on all independent variables before performing regression analysis. The test results show that the inflation factor (VIF) of each variable is less than two and the tolerance value is greater than 0.01, indicating that there is no multicollinearity between the variables. Specific test results are shown in Table 3.
The dependent variable in this paper is a binary variable that is suitable for binary logistic regression analysis. The equation fitted by logistic regression is:
where P represents the probability of an event occurring, 1-p represents the probability that the event does not occur, and the value of p ranges from 0 to 1.
TABLE 4. Regression results of social networks affecting the decision-making model of farmers’ participation in behavior.
This paper constructs three models. Model 1 only introduces control variables, Model two introduces core variables on the basis of Model 1, and Model three introduces four dimensions of core variables on the basis of Model 1. When the core variable is introduced, the log-likelihood value of Model two changes from -148.284 to -143.632, indicating that the social network has an improving effect on the model after processing the control variable. From the results, the social network index passed the significance test at the 0.01 level and the regression coefficient was positive, indicating that the social network had a significant positive impact on the decision of farmers to participate in cooperatives. In Model 3, network interaction, network learning, and network trust all have positive effects on farmers’ choice to participate in cooperatives, and all have passed the 0.05 level significance test, and the research hypotheses H1, H2, H3 and H5 have been verified. Further analysis shows that the social network has a greater influence on the behavior of farmers choosing to participate in cooperatives than the income level, and different dimensions of social networks have different influences on the behavior of farmers choosing to participate in cooperatives. Among them, the influence of network interaction is significant. Next derives the online trust and finally online learning. This indicates that the higher the frequency of farmers’ network interaction, the stronger the network learning ability, and the higher the degree of network trust, the higher the level of social network, and the greater the possibility of farmers making decisions about participating in cooperatives. Specifically, farmers can obtain valuable information from other network members through interactive communication and learning on the Internet and improve their own awareness of cooperatives. The higher the degree of trust among network members, the easier it is to listen to and imitate the production and business behaviors of other members, and it is also easier to obtain support mainly in terms of funds, labor, and technology. The impact of network reciprocity on farmers’ participation in cooperative behavior decision-making is negative, which is contrary to our expectation, that is, the research hypothesis H4 has not passed the verification. The explanation for this is: the higher the degree of network reciprocity, the easier it is for farmers to solve problems with financing needs, production and operation, and living problems with the help of network members such as relatives, friends, neighbors, etc., while the willingness decreases. Therefore, the regression results will show a negative impact on the relationship.
Among the control variables, the age of the head of the household, social experience, income level, main source of livelihood, and cultivated land have a positive impact on the choice of farmers to participate in cooperatives, and passed the 10, 1, 1, 10, and 10% levels of significance, respectively which is also consistent with the research expectations. It shows that the age of the household head, social experience, income level, main source of livelihood, and cultivated land area all have a positive role in promoting the choice of farmers to participate in cooperatives. The reason is that farmers engaged in agricultural production have certain requirements on their physical fitness. As farmers grow older, their labor capacity and physical strength will gradually decline, their income sources will also decrease, and the demand for joining the cooperatives will become stronger. The richer the social experience of the farmers, the wider the network of contacts they have accumulated, the more channels they can obtain from various information sources, and higher the quality of the information, the more conducive the farmers are to realize the benefits brought by the cooperative, and then make the decision to participate in the cooperative. In rural areas, generally speaking, the higher the income level of farmers, the more idle funds they have and the stronger their desire to invest. Cooperatives have the function of sharing risks and a guaranteed investment option. The more farmers rely on agricultural income, the more likely they are to be impoverished. Therefore, the demand for developing specialized production and management, and improving income levels is stronger, and the need to improve the degree of agricultural organization is also more urgent, and the possibility of participating in cooperatives is greater. The larger agricultural land, the greater the demand for human, material and financial resources for farmers, and the greater the risks of agricultural production that farmers face, and cooperatives can provide them with the social services and risk prevention and control they need. However, the influence of whether a family member serves as a cadre is negative, with a significance level of 1%. The reason may be that farmers who serve as cadres have rich knowledge reserves and a broader vision than ordinary farmers. They have certain insight and avoid market risks and grasp the market. The ability to exploit opportunities, they know how to spread risks, and they will not invest all the funds in the cooperative, which has a negative effect on the joining behavior of other farmers who trust him.
For the question of whether there is an interaction between social network and income level in the decision-making of farmers’ participation in cooperative behavior and whether it is a complementary effect or a substitute effect, this paper adds an interaction term to Model 3) and Model 4). Table 5 shows the specific regression results.
TABLE 5. Regression results of social network and income level interactively affecting farmers’ participation in cooperative decision-making model.
From the model estimation results in Table 5, it can be seen that the Prob > chi2 values of the four models are all 0.000, which indicates that the fitting effect of the models is good. In model 1), social network, income level, whether there are family cadres, and social experience passed the 1% significance test. In model 2), network interaction, network learning, and network trust all passed the significance test at the 5% level, but network reciprocity was not significant. Among the control variables, income level, whether family members serve as cadres, social experience, age, number of cultivated land and source of life passed the significance test at the levels of 1, 1, 1, 10, 10, and 10% levels, respectively. In model 3), the influence of social network and income level on farmers’ choice to participate in cooperatives is positive, and both have passed the significance test at the 1% level. The level of significance test further shows that both social networks and income levels are conducive to farmers’ decision to participate in cooperatives, and there is a substitution effect between social networks and income levels. It is assumed that with the increase of income level, the influence of social network on farmers’ decision to participate in cooperatives is weakened. Hence the research hypothesis H6 is verified.
In model 4), the influence coefficients of the four dimensions of social network and income level on farmers’ participation in cooperative decision-making are all positive, and income level, network learning, and network trust all pass the 1% significance test. Furthermore, gender, age, social status, source of life, whether family members served as cadres, and social experience passed the significance tests at the levels of 10, 10, 10, 5, 1 and 1%, respectively. The influence coefficient of whether family members serve as cadres is negative. From the perspective of the sign of the influence coefficient, the interaction coefficients of the four dimensions of the social network and the income level are all negative, which means that the four dimensions of the social network and the income level have a substitution effect in improving the possibility of farmers participating in cooperatives. The effect of the four dimensions of the network on improving the possibility of farmers making decisions to participate in cooperatives will weaken with the increase of income level, or in other words, with the improvement of the four dimensions of the social network, the income level will affect farmers’ decision-making to participate in cooperatives. The impact is waning. Among them, the interaction items of online learning, online trust, and income level all passed the significance test at the 1% level, but the interaction items of online interaction and online reciprocity and income level were not significant. It shows that income level mainly affects farmers’ participation in cooperative behavior decision-making through the interaction between the two dimensions of online learning and online trust.
In this paper, binary probit regression analysis is used to test the robustness of the model in Table 5. The specific test results are shown in Table 6. It can be seen from Model 5) that the influence coefficients of social network and income level on farmers’ choice to participate in cooperatives are both positive and have passed the 1% significance test. In Model 6), income level, online learning, online interaction, and online trust significantly and positively affect farmers’ participation in cooperatives, with significance levels of 1, 5, 5, and 5%, respectively. In Model 7), the social network positively affects farmers’ participation in cooperative behavior decision-making at the 5% level of significance, but the interaction term between the social network and income level has a negative impact on farmers’ choice to participate in cooperatives. The significance test shows that income level has a substitution effect on the social network. In Model ), the income level, online learning, and online trust have a positive impact on the choice of farmers to participate in cooperatives at the 1% significance level. The interaction items of the four dimensions of the social network and the income level have a negative impact on the farmers’ choice to participate in cooperatives. Among them, the interaction items of online learning, online trust, and income level have passed the significance test at the 1% level. The interaction terms of network reciprocity and income level did not pass the significance test. These test results are consistent with the regression results of the original model (Table 5). Additionally, the sign of the regression coefficient of the control variable is consistent with the regression results of the above model (Table 5), and the coefficient value does not change much. Therefore, the above model has passed the robustness test, and the research conclusions are relatively robust.
In this paper, the binary logistic model is used to empirically analyze the relationship between social networks and farmers’ behavior in joining the cooperative. Finally, the following conclusions are drawn: both the social network and income level have a significant positive impact on farmers’ participation in cooperative behavior decision making, and the social network has the greatest effect. The social network has a significant positive impact on farmers’ participation in cooperative behavior decision making, mainly through network interaction, network learning, and network trust, but network reciprocity is negatively and insignificantly correlated with it. Income level has an interaction effect on the influence of social network on farmers’ participation in cooperative behavior decision-making. Among them, network learning and network trust have a significant substitution relationship with income level when increasing farmers’ participation rate. In addition to social network, income level and their interaction terms, which significantly affect the decision-making behavior of farmers participating in cooperatives, factors such as farmers’ social experience, whether family members serve as cadres, and main sources of life also have an important impact on promoting farmers’ participation in cooperatives. Among them, the social experience of the head of the household and the main source of income of the family have a significant positive impact on the behavioral decision-making of farmers participating in the cooperative; whether a family member serves as a cadre has a significant negative impact on it.
Based on the above research conclusions, this paper believes that:
1. For the grass-roots government: while actively advocating farmers’ participation in cooperatives, they should pay attention to the utilization and cultivation of farmers’ social network. First, we carry forward the traditional rural atmosphere of “pro-mutual benefit and trust”. Strengthen the construction of a rural cultural environment and create a good rural cultural environment. By improving rural cultural facilities, leisure facilities, and organizing cultural activities, farmers can improve the cultural life of households and improve interaction and trust among the residents. Second, promote the formation of higher trust between farmers and village cadres. Actively guide and organize farmers to participate in collective activities such as skill training and encourage farmers to learn, interact, and communicate with technicians and village cadres to improve their skills. Create more opportunities for farmers to participate in politics and improve communication and understanding between farmers and village cadres. Finally, strengthen the publicity and promotion of cooperatives and related policy information. Make full use of the social network of farmers, through its information acquisition, transmission, and risk avoidance mechanisms, so that farmers can grasp information, accumulate knowledge and experience through mutual help and learning interaction, and achieve the purpose of improving their own awareness of cooperatives.
2. For cooperatives: First of all, it is necessary to continuously improve its service level. Regular production, sales and agricultural technology training for members; for non-member farmers, the model cooperatives take the lead, and cooperate with village cadres to carry out “online + offline” reporting meetings to report their business status and benefits obtained by members, and collect the production of ordinary farmers. Technical problems in operation, strengthen the publicity and training of farmers who have not joined the cooperative, improve the skills level of farmers and their awareness of cooperatives, so that they have a clear understanding of the benefits of participating in cooperatives as well as to attract more future farmers. Link farmers especially for those whose income level is low and mainly depends on agricultural income, they should be patiently guided and publicized. They have a higher demand for increasing their income and obtaining production, supply, and marketing services, and they are more likely to choose to join cooperatives.
The original contributions presented in the study are included in the article/Supplementary Material, further inquiries can be directed to the corresponding author.
Data collection and methodology were performed by GH, UL and JL; SF and JW did formal analysis and software. GH wrote the original draft. Review and editing were performed by JW.
This research study is supported by “Research on Farmers’ Production Linkage Mechanism and Its Correlation Credit Risk Evolution Mechanism” National Natural Science Foundation of China Grant No. 71873101.
The authors declare that the research was conducted in the absence of any commercial or financial relationships that could be construed as a potential conflict of interest.
All claims expressed in this article are solely those of the authors and do not necessarily represent those of their affiliated organizations, or those of the publisher, the editors and the reviewers. Any product that may be evaluated in this article, or claim that may be made by its manufacturer, is not guaranteed or endorsed by the publisher.
Baoyu, C. (2015). Farmer specialized cooperatives: Mechanism employed and effect value of social capital[J]. China Agric. Univ. J. Soc. Sci. Ed. 32 (04), 101–109.
Cai, Q., and Zhu, Y. (2017). The influence of relational networks on farmers’ participation in village collective action: Based on farmers’ participation in the investment of small irrigation system[J]. J. Nanjing Agri. Univ. Soc. Sci. Ed. 17 (01), 108–118. +147-148.
Cai, R., and Han, H. (2012). Analyzing the determinates of farmers’ choice of participate in cooperatives–based on apple growers from Shandong Province[J]. China Rural. Surv. 2012 (05), 32–40+95.
Campbell, K. E., Marsden, P. V., and Hurlbert, J. S. (1986). Social resources and socioeconomic status. Soc. Netw. 8 (1), 97–117. doi:10.1016/s0378-8733(86)80017-x
Chen, Y., Ren, X. D., and Li, S. Z. (2019). Analysis of members' participation, cooperative behavior and willingness to professional cooperatives under the new situation - a case study of five cooperatives in Guizhou Province[J]. Rural. Econ. (01), 139–144.
Cook, M. L. (1995). The future of U.S. Agricultural cooperatives: a neo-institutional approach. Am. J. Agric. Econ. 77 (5), 1153–1159. doi:10.2307/1243338
D'Angelo, E., and Lilla, M. (2011). Social networking and inequality: The role of clustered networks. Camb. J. regions, Econ. Soc. 4 (1), 63–77. doi:10.1093/cjres/rsq034
Dan, Y., and Zimin, L. (2017). Farmers' specific investment, relationship between farmers and cooperatives and farmers' income growth[J]. Chin. Rural. Econ. (05), 45–57.
Fahad, S., Alnori, F., Su, F., and Deng, J. (2022b). Adoption of green innovation practices in SMEs sector: Evidence from an emerging economy. Economic Research-Ekonomska Istraživanja, 1–16.
Fahad, S., Hossain, M. S., Huong, N. T. L., Nassani, A. A., Haffar, M., and Naeem, M. R. (2022a). An assessment of rural household vulnerability and resilience in natural hazards: evidence from flood prone areas. Environ. Dev. Sustain. doi:10.1007/s10668-022-02280-z
Fahad, S., and Jing, W. (2018). Evaluation of Pakistani farmers’ willingness to pay for crop insurance using contingent valuation method: the case of Khyber Pakhtunkhwa province. Land use policy 72, 570–577. doi:10.1016/j.landusepol.2017.12.024
Fahad, S., and Wang, J. (2018). Farmers’ risk perception, vulnerability, and adaptation to climate change in rural Pakistan. Land Use Policy 79, 301–309. doi:10.1016/j.landusepol.2018.08.018
Granovetter, M. (1995). Getting a job: a study of contacts and careers[M]. University of Chicago Press.
Hakelius, K., and Hansson, H. (2016). Measuring changes in farmers’ attitudes to agricultural cooperatives: Evidence from Swedish agriculture 1993-2013. Agribusiness 32 (4), 531–546. doi:10.1002/agr.21464
Hansen, M. H., Morrow, J. L., and Batista, J. C. (2002). The impact of trust on cooperative membership retention, performance, and satisfaction: an exploratory study. Int. food Agribus. Manag. Rev. 5 (1), 41–59. doi:10.1016/s1096-7508(02)00069-1
Hind, A. M. (1997). The changing values of the cooperative and its business focus. Am. J. Agric. Econ. 79 (4), 1077–1082. doi:10.2307/1244265
Hong, M. A. (2016). The impact of the interaction between migrant workers’ individual social capital and private lending on their income: an empirical study based on the survey of migrant workers in wuhan[J]. Econ. Problems (10), 67–72.
Irfan, M., and Ahmad, M. (2022). Modeling consumers' information acquisition and 5G technology utilization: Is personality relevant? Personality Individ. Differ. 188, 111450. doi:10.1016/j.paid.2021.111450
Jan, A., Xin-Gang, Z., Ahmad, M., Irfan, M., and Ali, S. (2021). Do economic openness and electricity consumption matter for environmental deterioration: silver bullet or a stake? Environ. Sci. Pollut. Res. 28, 54069–54084. doi:10.1007/s11356-021-14562-6
Javed, H., Irfan, M., Shehzad, M., Abdul Muqeet, H., Akhter, J., Dagar, V., et al. (2022). Recent trends, challenges, and future aspects of P2P energy trading platforms in electrical-based networks considering blockchain technology: a roadmap toward environmental sustainability. Front. Energy Res. 10, 810395. doi:10.3389/fenrg.2022.810395
Krackhardt, D., and Burt, R. S. (1995). Wanted: a good network theory of organization. Adm. Sci. Q. 40 (2), 350. doi:10.2307/2393643
Lai, G., Lin, N., and Leung, S. Y. (1998). Network resources, contact resources, and status attainment. Soc. Netw. 20 (2), 159–178. doi:10.1016/s0378-8733(97)00012-9
LeVay, C. (1983). Agricultural co-operative theory: a review[J]. J. Agric. Econ. 34 (1), 1–44. doi:10.1111/j.1477-9552.1983.tb00973.x
Li, M., Wang, L. L., and Guo, H. L. (2015). Analysis on influencing factors of farmers' willingness to participate in cooperatives: Based on the data of yangling demonstration area in Shaanxi province[J]. Yunnan Soc. Sci. (03), 63–67.
Li, X., and Ito, J. (2021). An empirical study of land rental development in rural Gansu, China: The role of agricultural cooperatives and transaction costs. Land Use Policy 109, 105621. doi:10.1016/j.landusepol.2021.105621
Liang, Q., Wu, W., and Liu, M. (2014). Impacts of social capital on member participation and performance of farmer cooperatives[J]. Issues Agric. Econ. 35 (11), 71–79+111.
Liao, X. J., Ying, R. Y., and Deng, H. S. (2016). The income effect and benefit distribution: analysis on the effect of farmers' cooperation[J]. China Soft Sci. (05), 30–42.
Liu, J. (2017a). The impact of farmers' specialized cooperatives on rural poor households' income: Evidences from shandong and guizhou provinces[J]. Chin. Rural. Econ. (02), 44–55.
Liu, Y. (2019). Research on the impact of the management ability of agricultural enterprises by small farmers' social network[J]. Reg. Econ. Rev. (04), 89–96.
Lu, S., Yao, Y., and Wang, H. (2017). How do social capital influence rural households' life after land acquisition: an empirical analysis based on ordered logistic model[J]. China Land Sci. 31 (06), 3–12.
Ma, G., and Yang, E. (2011). Social networks, informal finance and entrepreneurship[J]. Econ. Res. J. 46 (03), 83–94.
Ma, W., and Abdulai, A. (2016). Does cooperative membership improve household welfare? Evidence from apple farmers in China[J]. Food Policy 58, 94–102. doi:10.1016/j.foodpol.2015.12.002
Miao, S. (2014). Farmers’ small-scale irrigation facilities participative behavior under multi-dimensional social capital perspective[J]. China Popul. Resour. Environ. 24 (12), 46–54.
Nureen, N., Liu, D., Ahmad, B., and Irfan, M. (2022). Exploring the technical and behavioral dimensions of green supply chain management: a roadmap toward environmental sustainability. Environ. Sci. Pollut. Res. Int., 1–14. doi:10.1007/s11356-022-20352-5
Pokharel, K. P., and Featherstone, A. M. (2019). Estimating multiproduct and product-specific scale economies for agricultural cooperatives. Agric. Econ. 50 (3), 279–289. doi:10.1111/agec.12483
Su, F., Song, N., Shang, H., and Fahad, S. (2022). The impact of economic policy uncertainty on corporate social responsibility: a new evidence from food industry in China. PLoS ONE 17 (6), e0269165. doi:10.1371/journal.pone.0269165
Su, F., Song, N., Ma, N., Sultanaliev, A., Ma, J., Xue, B., et al. (2021). An assessment of poverty alleviation measures and sustainable livelihood capability of farm households in rural China: a sustainable livelihood approach. Agriculture 11, 1230. doi:10.3390/agriculture11121230
Sun, Y., Guan, W., Razzaq, A., Shahzad, M., and An, N. B. (2022b). Transition towards ecological sustainability through fiscal decentralization, renewable energy and green investment in OECD countries. Renew. Energy 190, 385–395. doi:10.1016/j.renene.2022.03.099
Sun, Y., and Razzaq, A. (2022). Composite fiscal decentralisation and green innovation: Imperative strategy for institutional reforms and sustainable development in OECD countries. Sustainable Development. doi:10.1002/sd.2292
Sun, Y., Razzaq, A., Sun, H., and Irfan, M. (2022a). The asymmetric influence of renewable energy and green innovation on carbon neutrality in China: analysis from non-linear ARDL model. Renew. Energy 193, 334–343. doi:10.1016/j.renene.2022.04.159
Wallace, R., and Bassuk, E. (1991). Housing famine and homelessness: how the low-income housing crisis affects families with inadequate supports. Environ. Plan. A 23 (4), 485–498. doi:10.1068/a230485
Wang, G. L., and Lu, Q. (2015). Inverted u-shaped relationship between social network and farmers' technology adoption: an example of water-saving irrigation technology in minqin county, gansu province[J]. J. Agrotechnical Econ. (10), 92–106.
Wang, L., Yang, X. H., and Jang, M. D. (2019). Study on Farmers' willingness to participate in the supply of small-scale farmland water conservancy facilities from the perspective of social network relationship embedding[J]. Rural. Econ. 2019 (01), 111–117.
Wang, X., and Li, M. (2011). Researches on agricultural cooperative economic organization promoting agricultural insurance development[J]. Asian Agric. Res. 3 (07), 75–79.
Warriner, G. K., and Moul, T. M. (1992). Kinship and personal communication network influences on the adoption of agriculture conservation technology. J. rural Stud. 8 (3), 279–291. doi:10.1016/0743-0167(92)90005-q
Watts, D. J., and Strogatz, S. H. (1998). Collective dynamics of ‘small-world’ networks. nature 393 (6684), 440–442. doi:10.1038/30918
Yang, D., and Liu, Z. (2012). Study on Chinese farmer cooperative economy organization and agricultural specialization. Agric. Econ. 58 (3), 135–146. doi:10.17221/17/2011-agricecon
Ye, jingyi, and Zhou, yexin (2010). Social capital transformation and income of migrant workers: Evidence from beijing data[J]. Manag. World (10), 34–46.
Zhang, Q. W., and Zhou, H. P. (2013). Analysis on influencing factors of farmers' willingness to participate in cooperatives: a case study of liaodian township, acheng city, heilongjiang province[J]. J. Agrotechnical Econ. (03), 98–104.
Zhao, J., and Lu, M. (2010). The contribution of guanxi to income inequality in rural China and a cross-regional comparison : a regression-based decomposition[J]. China Econ. Q. 9 (01), 363–390.
Zhigang, X., Zheyi, Z., and Hengshan, D. (2017). Product premium, industrial risk and sales function of farmers specialized cooperatives: an empirical analysis of the cooperative game between large and small-scale farmers[J]. China Rural. Surv. (05), 102–115.
Keywords: sustainable development, social networks, community participation, households’ behavior, cooperatives
Citation: Hu G, Wang J, Laila U, Fahad S and Li J (2022) Evaluating households’ community participation: Does community trust play any role in sustainable development?. Front. Environ. Sci. 10:951262. doi: 10.3389/fenvs.2022.951262
Received: 23 May 2022; Accepted: 27 June 2022;
Published: 31 August 2022.
Edited by:
Asif Razzaq, Ilma University, PakistanReviewed by:
Farhan Ullah, Northwestern Polytechnical University, ChinaCopyright © 2022 Hu, Wang, Laila, Fahad and Li. This is an open-access article distributed under the terms of the Creative Commons Attribution License (CC BY). The use, distribution or reproduction in other forums is permitted, provided the original author(s) and the copyright owner(s) are credited and that the original publication in this journal is cited, in accordance with accepted academic practice. No use, distribution or reproduction is permitted which does not comply with these terms.
*Correspondence: Jing Wang, d2o2Nnh5eEAxMjYuY29t
Disclaimer: All claims expressed in this article are solely those of the authors and do not necessarily represent those of their affiliated organizations, or those of the publisher, the editors and the reviewers. Any product that may be evaluated in this article or claim that may be made by its manufacturer is not guaranteed or endorsed by the publisher.
Research integrity at Frontiers
Learn more about the work of our research integrity team to safeguard the quality of each article we publish.