- 1School of Economics, Qingdao University, Qingdao, China
- 2School of Finance and Economics, Jiangsu University, Jiangsu, China
- 3School of Economics and Management, Xinjiang University, Urumqi, China
Achieving high-quality economic development is a crucial feature of China’s new development stage. As an environmental regulation tool by implementing a differentiated credit policy, green credit is an inevitable choice to achieve high-quality economic development. In this paper, ecological welfare performance is used to measure the level of high-quality economic development. And based on the panel data of 30 provinces during 2008–2019, the spatial Durbin model and mediating effect model are used to empirically study the direct and indirect effect of green credit on ecological welfare performance in China. The results show that: (1) China’s ecological welfare performance shows the positive global spatial correlation in the overall spatial scope, with local characteristics of high–high aggregation and low–low aggregation. (2) Green credit can significantly promote the ecological welfare performance of local regions, but has a negative spatial spillover effect on the ecological welfare performance of adjacent regions. (3) Green credit can boost ecological welfare performance by improving technological innovation, industrial structure upgrading, and energy consumption structure. Based on these conclusions, the policy recommendations are put forward.
1 Introduction
Since entering the 21st century, China’s economic output has grown rapidly. China’s overall GDP expanded from 1.21 trillion dollars to 17.72 trillion dollars between 2000 and 2021, propelling it from sixth to second place in the globe (Han et al., 2018). The extensive development model generated from rapid economic expansion has resulted in negative development difficulties, such as low resource utilization, environmental degradation, low welfare output, and so on (Liu and Lin, 2019). For instance, in comparison to 2000, total energy consumption in 2020 increased by 256.55%. And sulfur dioxide emissions, chemical oxygen demand discharge, and industrial solid waste production totaled 3.18 million tons, 25.65 million tons, and 3.68 billion tons, respectively in 2020. China’s economy is currently transitioning from a high-speed growth stage to a high-quality development stage (Liu et al., 2021). The Sixth Plenary Session of the 19th Central Committee of the Communist Party of China (CPC) stated that high-quality economic development, in which green development has become a common form, should be realized. However, achieving high-quality economic development that considers social welfare output, resource conservation, and environmental protection is a difficult task for Chinese society (Zhao X. et al., 2022).
The Chinese government has released a number of measures aimed at achieving high-quality economic growth. The 19th National Congress of the CPC clearly stated that the growth of green finance is one road to achieving high-quality economic development (An et al., 2021), which is compatible with the worldwide financial community’s practical experience (Zhang S. et al., 2021). Moreover, it is estimated that the balance of green credit accounts for more than 90% of the total balance of green financing in China (Scholtens and Dam, 2007), as a result of the bank-dominated character of China’s financial system. Green credit is a new form of environmental regulation tool that can realize environmental protection by implementing differentiated credit policies for different enterprises (Zhang, 2021). Usually, it offers lower loan interest rates and credit support for clean and environmentally friendly enterprises. While on the contrary, it imposes tighter credit exposure for high-energy consumption and heavy pollution enterprises (Song et al., 2021). As the starting point to assist the construction of ecological civilization, green credit plays an important role in fostering the high-quality economic development in China.
At present, the urgent problem to be solved on the road to achieving high-quality economic development is to realize the balanced and coordinated development of resources, environment and welfare output. As the comprehensive performance indicator that can simultaneously characterize resource input, environmental pollution, and social welfare, ecological welfare performance (EWP) cannot only provide the quantitative standard for the level of high-quality economic development, but also provide a reference for policy formulation (Feng et al., 2019). Therefore, revealing the specific impact of green credit on EWP from both theoretical and empirical aspects, and further analyzing its transmission mechanism, is of considerable value for advancing the green transformation of the economy and achieving high-quality economic development.
This paper uses 30 provinces during 2008–2019 in China as research samples to explore the impact and the specific transmission mechanism of green credit on EWP. First, this paper theoretically analyzes the effect and transmission mechanism of green credit on EWP based on relevant theories, and makes hypotheses. Second, on the basis of utilizing Super-SBM DEA to measure EWP and conducting Moran’s I tests, the spatial Durbin model is selected to analyze the local impact and spatial spillover effect of green credit on EWP. Third, the mediating effect model is constructed to test the mediation effect of technological innovation, industrial structure upgrading, and energy consumption structure. Finally, this paper conducts robustness testing, and proposes policy recommendations based on conclusions.
The paper consists of 6 sections. Section 1 is the introduction. In Section 2, the literature review of the impact of green credit on EWP is provided shortly. Section 3 theoretically analyzes the impact mechanism of green credit on EWP, and puts forward hypotheses. In Section 4, the data and empirical methods are introduced. The empirical results of regression models are represented in Section 5. The conclusion and policy recommendations are discussed in Section 6.
2 Literature review
This paper adopts the EWP to evaluate the level of high-quality economic development, and then studies the impact and specific transmission path of green credit on EWP. Based on the research content, the literature review is conducted from two aspects of EWP and green credit.
2.1 Research on ecological welfare performance
The proposal of EWP can be traced back to Daly’s evaluation method of measuring the level of regional sustainable development by the welfare output brought by unit resource consumption (Daly, 1974). On this basis, in order to measure the efficiency level of converting unit resource consumption into social welfare output, Zhu (2008) proposed the concept of EWP for the first time, and used the ratio of human development index to ecological footprint for computation. Starting from this, scholars have conducted in-depth research on the measurement methods, regional differences, and influencing factors of EWP. And considering EWP is a comprehensive indicator of social welfare that takes resource consumption and the ecological environment into account, many scholars used EWP to measure the level of green development (Hu M. et al., 2021; Song and Mei, 2022) and high-quality economic development (Wang and Feng, 2020).
First, in terms of the choice of measurement methods, there are two main methods for measuring EWP in the existing literature. The one is Data Envelopment Analysis (DEA) model and Stochastic Frontier Production model. For example, based on the EWP evaluation index system, some scholars used the Super-SBM DEA model (Bian et al., 2020; Wang et al., 2021), and Super-NSBM model that considers undesired output to measure the EWP (Hou et al., 2020). And Xiao and Zhang (2019) used Stochastic Frontier Production model to estimate the level of EWP in China. Another one is to use the definition ratio method to measure the EWP by the ratio of resource consumption to social welfare output (Knight and Rosa, 2011; Dietz et al., 2012; Zhang et al., 2018). For instance, some literature utilized per capita ecological footprint to measure resource consumption, and chose life expectancy, average happiness index, or human development index to measure welfare output. Second, on the basis of measuring EWP, some scholars have explored the different distribution pattern of regional level of EWP (Long et al., 2020; Yao et al., 2020; Hu M. et al., 2021). For example, Deng et al. (2021) found that the spatial distribution pattern of EWP in China was the strongest in the eastern region and weak in the central and western regions. Third, in the study of influencing factors of EWP, some scholars have pointed out that economic contribution rate (Jorgenson and Dietz, 2015), industrial structure upgrading (Li et al., 2019), technological innovation (Cheng and wang, 2022), resource recycling (Geng, 2020), etc. all significantly impact on EWP.
2.2 Research on green credit
In 2007, the concept of green credit was proposed in the “Opinions on Implementing Environmental Protection Policies and Regulations to Prevent Credit Risks” jointly issued by the State Environmental Protection Administration of China, the People’s Bank of China and the China Banking Regulatory Commission (Cao et al., 2021; Liu and He, 2021). In 2012, the “Green Credit Guidelines” formulated by the China Banking Regulatory Commission further strengthened the implementation of green credit policies (Hu G. et al., 2021). At now, the study on green credit largely focuses on two aspects: theoretical research and influencing effect.
First, in terms of theoretical study, several scholars have undertaken in-depth research on the operation mechanism, institutional innovation and risk management of green credit (Zhang et al., 2011; Xing et al., 2020; Xu, 2020; Zhu et al., 2021). Then based on the calculation and evaluation of its cost benefit and efficiency (Lin et al., 2020; Wen et al., 2021), some papers have taken the reform pilot area as an example to further explore the appropriate development path of green credit (Wang and Lin, 2021), and have proposed suggestions for promoting the development of green credit in China. Second, the research on the influencing effect of green credit largely focuses on micro and macro issues. On the one hand, research from the microscopic perspective mainly focuses on the impacts of green credit on the operational performance of commercial banks (Yin et al., 2021; Zhou et al., 2021), corporate technological innovation (Hong et al., 2021), and investment efficiency (Wang et al., 2020; Yao et al., 2021). On the other hand, most studies from the macro perspective focus on the impact of green credit policy on regional Total Factor Productivity (TFP) (Hu et al., 2022), technological innovation (Tan et al., 2022), industrial structure upgrading (Hu et al., 2020), energy consumption structure (Ma X. et al., 2021), etc.
Essentially, the green credit policy is a means of environmental regulation. At present, the literature on the impact of environmental regulation on high-quality economic development is abundant, but has different conclusions. For instance, some studies have found that environmental regulation policies may greatly promote the level of green and high-quality economic development (Chen et al., 2020). Some papers suggest that environmental regulation has a nonlinear influence on green innovation (Liu et al., 2022) and the development of green economy (Shuai and Fan, 2020; Wang, 2020; Ma and Xu, 2022). And some scholars pointed out that the implementation of environmental regulations will bring spatial spillover effects to high-quality economic development in adjacent areas (Feng and Chen, 2018). However, little literature has deeply explored the effect of environmental regulation on the level of high-quality economic development from the perspective of green credit policy.
Combing the above-related literature, it is found that the existing research mainly focuses on the following three aspects. First, the current analysis of the impact of green credit mainly focuses on micro levels, such as banks and firms. And most of the literature mainly discusses the effect of green credit on the development of local areas, without considering its spatial spillover effect. Second, existing studies mostly use indicators, such as green economic growth and green total factor productivity to measure the level of high-quality economic development (Hu Z. et al., 2021; Sun et al., 2022), and rarely use EWP for analysis. Third, most of the literature only analyzes the direct effect of green credit on high-quality economic development, but does not deeply analyze its specific transmission path.
In contrast, this article may contain the following three contributions. First, this article evaluates, from a macro viewpoint, the impact of green credit policies on the high-quality economic development of the local regions and the spatial spillover impact on adjacent regions using the spatial Durbin model. Second, this research analyzes the level of high-quality economic development from the standpoint of EWP by constructing an EWP evaluation index system that takes into account resource consumption, environmental pollution, and welfare output comprehensively. Third, the article employs the mediating effect model to investigate the transmission channel of the influence of green credits on EWP in terms of technological innovation, industrial structure upgrading, and energy consumption structure.
3 Theoretical analysis and hypotheses
This part theoretically discusses the direct effect of green credit on EWP, and also analyzes the indirect transmission effects of technological innovation, industrial structure upgrading, and energy consumption structure. The specific effect mechanism is shown in Figure 1.
3.1 The direct effect of green credit on ecological welfare performance
Green credit influences the external financing channels of firms by applying differentiated credit rules for environmental protection enterprises and two-high enterprises (high-energy-consuming enterprises, high-pollution enterprises) (Zhang et al., 2021a). This can bring both macro and micro economic effects. From the macro perspective, green credit can change the flow of funds. It enables financial institutions to provide preferential loan interest rates and increase loan quotas for energy-saving enterprises and environmental protection enterprises, but truncate part credit funds of two-high enterprises (Chai et al., 2022; Zhang and Kong, 2022). Therefore, energy-saving enterprises and environmental protection enterprises can obtain sufficient funds to continuously improve the implementation of environmental protection projects (Cui et al., 2022). Furthermore, in order to prevent being eliminated by the market, the two-high enterprises are forced to enter the environmental protection industry through internal technology upgrading (Zhang et al., 2022b). This is helpful in optimizing the macroeconomic structure, fostering the growth of energy-efficient industries, and further enhancing EWP. From the micro perspective, on the one hand, green credit provides financial support for the operation, technical research and market application of environmental protection enterprises, which can ease external financing limitations and improve operational efficiency (Feng and Shen, 2021). On the other hand, to protect the security and profitability of funds, commercial banks and other financial institutions would increase their supervision of lending firms or projects, which can enhance the efficacy of capital allocation (Wen et al., 2021), boost welfare output, and ultimately improve EWP.
In addition, according to the pollution shelter effect (Ouyang et al., 2020), to meet business demands or get loan financing, two-high firms would transfer across areas, which might exacerbate environmental degradation and diminish the welfare output of adjacent regions. Based on the above analysis, Hypothesis 1 is proposed.
Hypothesis 1. Green credit can promote the level of local EWP, and have a negative spatial spillover effect on EWP of adjacent regions.
3.2 The indirect impact of green credit on ecological welfare performance
3.2.1 The mediation effect of technological innovation
First, in response to green credit rules, financial institutions such as banks may enhance credit assistance for environmental protection enterprises, which might alleviate the lack of funds (Wang F. et al., 2019). The growth of financing scale can allow environmental protection firms to expand more in equipment replacement and environmental protection technology research and development. The increase in R&D expenditure may further improve the efficiency of resource utilization, stimulate the generation of new knowledge and technologies, and promote regional technological innovation (Pan et al., 2021). Second, in order to fulfill market demand, polluting firms would moderately raise R&D expenditure to enhance the efficiency of green technology innovation (Ma Q. et al., 2021). Finally, when enterprises are confronted with the innovation compensation effect, in which the cost of pollution treatment and credit exceeds the investment in technology, they will improve or update technical equipment to achieve clean process development, which is advantageous for fostering technological innovation.
On the one hand, by enhancing the manufacturing process, technological innovation may accomplish resource recycling and pollution control (Velenturf and Jopson, 2019), which can minimize resource consumption and pollutant discharge. On the other hand, with the constant transformation of environmental protection technology, high-polluting firms are gradually eliminated from the market. But environmental protection firms with low pollution and high added value are growing their share in the market (Tian et al., 2022). This is favorable to the green transformation of the industrial structure and the development of social welfare. To sum up, technological innovation may enhance resource utilization efficiency and welfare output, and minimize environmental pollution, which is beneficial to the improvement of EWP. Based on the above analysis, Hypothesis 2 is proposed.
Hypothesis 2. Green credit can promote the EWP through technological innovation.
3.2.2 The mediation effect of industrial structure upgrading
Capital is a necessary factor for the establishment, survival, and development of enterprises. In order to alleviate internal financial limitations during the phases of manufacturing, operation, and scaling up, businesses must utilize bank credit for external funding. First, green credit enables environmental protection enterprises to obtain sufficient funds to maintain the development and upgrading of existing projects, then expand their scale continuously (Chen et al., 2022). On the contrary, due to the absence of availability of loan funds, the normal operation and scale expansion of two-high enterprises are constrained (Wang E. et al., 2019). Two-high enterprises would alter their internal structures in order to get loans, which would be beneficial to industry transformation and upgrading (Cheng et al., 2021). Second, the propensity loan policy of commercial banks also sends a signal to the market that the government supports the development of the green economy, which can not only indicate the direction of the development of emerging enterprises, but also can reduce the market share of the high-polluting industries. This signal may boost the growth and prosperity of the energy-saving and environmental protection sector, as well as the upgrading of industrial structures (Li and Chen, 2022).
With the upgrading of the industrial structure, the allocation efficiency of production factors has steadily increased, which is favorable to increasing the TFP and the social welfare output level. And due to the associated technology spillover effect (Huang et al., 2019), environmental protection enterprises may transmit knowledge and technology to other polluting firms through labor mobility and technology sharing, which can effectively increase resource utilization, reduce environmental pollution, and boost EWP. Therefore, Hypothesis 3 is proposed.
Hypothesis 3. Green credit can promote the EWP through industrial structure upgrading.
3.2.3 The mediation effect of energy consumption structure
The green credit policy favors low energy-consumption and low-pollution enterprises. In order to acquire loans, economic organizations will prefer to employ clean and renewable energy to replace fossil energy in production (Zhang et al., 2021b), which can minimize production pollution and optimize energy consumption structure. In addition, the implementation of green credit forces enterprises to carry out technological innovation and implement traditional energy transformation (Guo et al., 2019), which can promote the transformation of social energy consumption to a clean consumption reduction model. The clean transformation of the energy consumption structure may enhance the efficiency of energy utilization and minimize the production pollution output, which can assist in improving the EWP of the society. Therefore, Hypothesis 4 is proposed.
Hypothesis 4. Green credit can promote the EWP through optimizing energy consumption structure.
4 Methods and data
This article chooses the spatial Durbin model and the mediating effect model to empirically test the proposed hypotheses. The specific model selection, indicator measurement methods, and data sources are as follows.
4.1 Spatial autocorrelation analysis
4.1.1 Global spatial autocorrelation
In order to measure the global spatial distribution characteristics of China’s EWP, global spatial autocorrelation index (Moran’s I) is chosen (Li and Li, 2020). And the specific calculation method is shown in Eq. 1.
where n is the number of space observation units;
4.1.2 Local spatial autocorrelation
In this article, the local spatial autocorrelation features of China’s EWP are analyzed using Moran’s scatter plot. The Moran scatter plot divides the plane area into four quadrants corresponding to the four spatial distribution characteristics. The first and third quadrants stand for high–high aggregation and low–low aggregation, respectively, indicating that areas with high (low) observed values are surrounded by areas with high (low) observed values. The second and fourth quadrants represent low–high aggregation and high–low aggregation, which indicates that areas with low (high) values are surrounded by areas with high (low) observed values. The first and third quadrants represent a positive spatial correlation between observation units, implying that provinces with similar EWP values are clustered. Conversely, the second and fourth quadrants indicate a negative spatial correlation.
4.2 Econometric models construction
4.2.1 Spatial weight matrices
Four types of spatial weight matrices, including the spatial adjacency weight matrix, the geographic distance weight matrix, the economic distance weight matrix, and the nested weight matrix, are constructed for empirical analysis based on the research content and existing literature.
4.2.1.1 Spatial adjacency weight matrix
Based on the Queen adjacency rule (Yan et al., 2017), the spatial units with common boundaries or common vertices are defined as adjoining units. The specific calculation method is shown in Eq. 2:
4.2.1.2 Geographic distance weight matrix
In light of the fact that the influence strength of spatial effect is negatively correlated with the distance between spatial units, and in order to mitigate the decay speed of spatial effect with increasing distance, the square of reciprocal geographical distance (
4.2.1.3 Economic distance weight matrix
Due to the fact that geographical factors are not the only factors that produce spatial effects, spatial units with similar levels of economic development have greater opportunities for cooperation and exchange. Consequently, their spatial effects become more potent. Therefore, referring to the method of Pingfang et al. (2011), the economic variable X of different spatial units is selected to construct the economic distance weight matrix. The specific calculation method is shown in Eq. 4:
where
4.2.1.4 Nested weight matrix
In order to accurately describe the complexity of spatial effects brought by both geographical and economic factors, the nested matrix that organically combines the economic weight matrix and the geographical weight matrix is constructed. The specific calculation method is shown in Eq. 5:
where wd represents the geographic distance weight matrix; diag (…) stands for the diagonal matrix;
4.2.2 Spatial econometric model
In consideration of the spatial autocorrelation characteristics of EWP, this article employs the spatial econometric model to assess the effect of green credit on EWP (Zhao P. et al., 2022). Before conducting the empirical analysis, this study firstly conducts LM test, LR test, and Hausman test based on panel data (Xie et al., 2021). The results are shown in Table 1.
In Table 1, the result of LM test shows that the models accept both the spatial lag model and the spatial error model at the 1% significance level under the geographic distance weight matrix and the nested matrix. Therefore, the spatial Durbin model combining the spatial lag model and the spatial error model should be considered. And according to the LR test, the spatial Durbin model cannot be degenerated into the spatial error model and the spatial lag model. The result of Hausman test suggests that the model with fixed effects should be chosen. To sum up, based on the test results in Table 1, it is reasonable to choose the spatial Durbin model with fixed effects for empirical analysis. The specific regression model is shown in Eq. 6.
where
4.2.3 Mediating effect model
In addition, in order to analyze the specific indirect transmission mechanism of green credit on EWP, referring to the research of Wen and Ye (2014), the mediating effect model is constructed for empirical analysis. The specific regression models are shown in Eqs. 7, 8.
where M represents the mediating variables, including technological innovation (Tec), industrial structure upgrading (Isa), and energy consumption structure (Es);
4.3 Index introduction and data sources
4.3.1 Explained variable
Ecological welfare performance (EWP): Based on the principles of systematic and operability, with reference to the method of Long (2019), the EWP evaluation index system is constructed from three dimensions: resource consumption, environmental pollution, and welfare output. On the basis of using the entropy weight method to reduce the dimensionality of the three-level indicators, the Super-SBM DEA model considering undesirable output is chosen for calculation. The evaluation index system of EWP is shown in Table 2.
4.3.2 Explanatory variable
Green credit (Gcl): The existing measurement methods of green credit are as follows. The first is the proportion of energy conservation and environmental protection project loans; the second is the proportion of green credit; the third is the proportion of bank loans in pollution control investment; the fourth is the proportion of interest expenses in six high-energy-consuming industries to the total interest expenditure of the industry. Among them, the data on energy conservation and environmental protection loans, green credits come from the Social Responsibility Report of the Commercial Bank of China, which is lacking for province data. After 2010, bank loans for pollution control investments were no longer counted. Therefore, the first, second, and third methods cannot be used. Referring to the research of Xie and Liu (2019), the fourth method is selected to measure green credit. However, the result of the fourth method is a negative indicator, that is, the higher the proportion of interest expenses in the six energy-intensive industries, the lower the level of green credit. In order to analyze the effect of the data directly, the difference method is employed to turn it into a positive indicator.
4.3.3 Mediating variables
Technological innovation (Tec): Since patents are an important activity output of technological innovation, the number of patent authorizations is used to estimate the level of technological innovation.
Industrial structure upgrading (Isa): This article measures the level of Isa referring to the research method of Fu (2010). First, the GDP is divided into three parts according to three kinds of industries. The proportion of each part of the added value to GDP is used as a component in the space vector. These three components form a set of three-dimensional vectors X0: (x1,0, x2,0, x3,0). Second, according to Eq. 9, the angles
Energy consumption structure (Es): Referring to the research method of Shaohua et al. (2015), this paper chooses the ratio of energy consumption other than coal to total energy consumption to measure the level of Energy consumption structure. And the amount of energy consumption is calculated according to the conversion factor of standard coal. The larger the ES value is, the more optimized the energy consumption structure is.
4.3.4 Control variables
Existing studies have pointed out that factors, such as urbanization, economic scale, fiscal expenditure, industrial structure, and environmental regulation all have an impact on ecological welfare performance (Naigang and Lin, 2021; Yuanjian et al., 2021). Therefore, in order to alleviate the endogeneity problem of empirical analysis, this article selects these variables as control variables. The specific calculation method is as follows.
Urbanization level (Urb): measured by the ratio of the urban population to the total population. Economic scale (Eco): estimated by the ratio of regional real GDP to national GDP. Fiscal expenditure (Fe): represented by the proportion of government fiscal expenditure in GDP. Industrial structure (Is): expressed by the proportion of the added value of the secondary industry to GDP. Environmental regulation (Er): measured by the proportion of investment in pollution control to GDP.
4.3.5 Data sources
In order to assure the integrity and consistency of data, the data during 2008–2019 of 30 provinces in China except Hong Kong, Macao, Taiwan and Tibet is chosen for research. The descriptive statistics of the data are shown in Table 3. Among them, the data of green credit are collected from the National Bureau of Statistics and the China Industrial Statistical Yearbook (2009–2020); the data of EWP evaluation system come from the China Statistical Yearbook (2009–2020), China Environmental Yearbook (2009–2020) and China Environmental Statistical Yearbook (2009–2020); the data of technological innovation are collected from Chinese Research Data Services database; the data of energy consumption and standard coal conversion coefficient are collected from Economy Prediction System database and China Energy Statistical Yearbook (2009–2020).
5 Empirical results
5.1 The results of Moran’s I index of ecological welfare performance
In order to verify the spatial correlation of EWP, the STATA software is used to calculate the Moran’s I index of EWP in China from 2008 to 2019 based on four types of spatial weight matrices. The results are shown in Table 4.
From Table 4, it can be seen that the global Moran’s I statistics of China’s EWP are positive during the study period based on spatial weight matrices. This indicates that there is a positive global spatial correlation between EWP in the overall spatial scope, which means that provinces with higher (lower) EWP are adjacent to provinces with higher (lower). The EWP of each province is not only affected by its own economic development, but also by the surrounding provinces’ EWP, economic development and other factors. Therefore, this also verifies that it is reasonable to consider the spatial correlation of EWP in the empirical analysis.
5.2 The results of Moran scatter plot
In order to further characterize the local spatial aggregation characteristics of EWP, this part depicts the Moran scatter plots. Figure 2A, Figure 2B and Figure 2C show the Moran scatter plots of provinces in 2008, 2014, and 2019 (limited to paper length, only the results based on the spatial adjacency matrix are reported). Where the horizontal axis is the standardized value of EWP, and the vertical axis is the spatial hysteresis, that is, the average value of EWP in the surrounding area.
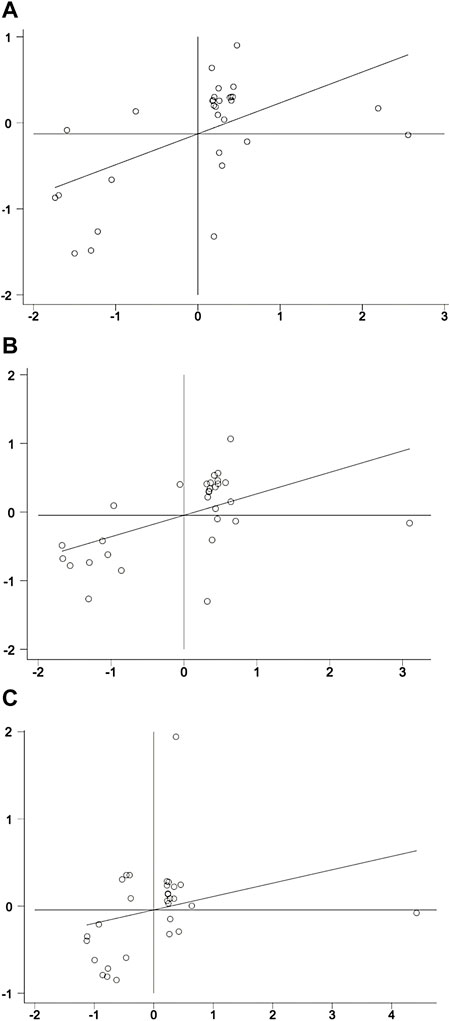
FIGURE 2. Moran scatter plots of EWP of China. (A) Moran scatter plot in 2008. (B) Moran scatter plot in 2014. (C) Moran scatter plot in 2019.
The Moran scatter plot has four quadrants, representing different distribution characteristics. It can be seen from Figure 2A, Figure 2B, and Figure 2C that most provinces are concentrated in the first quadrant and third quadrant, showing the characteristics of high–high aggregation and low–low aggregation. This shows that China’s EWP is not only spatially dependent, but also spatially different.
5.3 Analysis of the effect of green credit on ecological welfare performance
The sample data are regressed according to the established spatial Durbin model (Eq. 6). To reflect the robustness of the result, the regression results of the spatial Durbin model under geographic distance weight matrix and nested matrix are both provided in Table 5.
In Table 5, first, the spatial autocorrelation coefficient
Given that the spatial Durbin model contains explanatory variables with spatial correlation and their spatial lags, the regression coefficients of the model cannot fully explain the spatial spillover relationship between the dependent variable and the independent variable. In order to analyze the spatial spillover effects of green credit on EWP in detail, the spillover effects are decomposed into direct effects and indirect effects based on the partial differentiation method. The direct effect and indirect effect respectively represent the effect of the explanatory variable on the explained variable in the local or adjacent areas. The results are reported in Table 5. It shows that under the geographic distance weight matrix and nested matrix, the coefficients of direct effect are significantly 0.4140 and 0.4490. And the coefficients of indirect effect are significantly −3.2671 and −2.7983. Consistent with the previous regression coefficient’s conclusion, it implies that green credit can improve EWP, but has negative spatial spillovers to adjacent regions.
5.4 Analysis of the mediation effect of green credit on ecological welfare performance
The empirical regression results of spatial Durbin model show that green credit can promote the EWP. In order to analyze the mediating transmission mechanism of the impact of green credit on EWP in local regions, The mediating effect model is used to validate the transmission roles of technological innovation, industrial structure upgrading, and energy consumption structure in the relationship between green credit and EWP. The results under the nested matrix are shown in Table 6.
In Table 6, column 1) represents the result of the impact of green credit on EWP. Columns 2), 4), and 6) present the spatial impact of green credit on technological innovation, industrial structure upgrading, and energy consumption structure, respectively. Columns 3), 5), and 7) show the impact of technological innovation, industrial structure upgrading, energy consumption structure on EWP and the direct impact of green credit on EWP. It can be seen from Table 6 that the spatial autocorrelation coefficient ρ of technological innovation, industrial structure upgrading, and energy consumption structure is significantly positive at the 1% significance level. It indicates that all three mediation variables have positive spatial spillover effects. The results also verify the rationality of using the spatial econometric model for the mediating test model.
First, the results of columns 2), 4) and 6) show that the regression coefficients of green credit on technological innovation, industrial structure upgrading and energy consumption structure in local regions are 1.9920, 0.9097, and 0.2304, indicating that green credit can promote technological innovation, industrial structure upgrading and energy consumption structure. In addition, it also can be seen from columns 3), 5) and 7) that the regression coefficients of technological innovation, industrial structure upgrading and energy consumption structure on EWP in local regions are 0.1261, 0.2026, and 1.0874, representing that technological innovation, industrial structure upgrading, and energy consumption structure all play a positive role in boosting EWP. To sum up, according to the testing results of the mediating effect model, green credit can enhance EWP by boosting technological innovation, industrial structure upgrading, and energy consumption structure. Therefore, Hypotheses 2, 3, and 4 hold.
In addition, the results indicate that the spillover effects of green credits on mediating variables varied. The results of columns 2) represent that the regression coefficient of green credit on technological innovation of adjacent areas is significantly positive, indicating that green credit has a positive spillover effect on technological innovation of adjacent regions. However, it can be seen from columns 4) and 6) that the regression coefficient of green credit on industrial structure upgrading and energy consumption structure in adjacent areas are negative, representing that green credit retrains industrial structure upgrading and energy consumption structure of adjacent regions. Meanwhile, there are differences in the adjacent spillover effects of mediation variables on EWP. It can be seen from columns 3), 4), and 7) that technological innovation can promote EWP of adjacent areas, while industrial structure upgrading and energy consumption structure negatively impact EWP of adjacent areas.
Furthermore, in order to further verify the reliability of the test results of the mediating model, the bootstrap test which was randomly sampled 500 times is chosen for verification. The results are shown in Table 7.
The results of Table 7 indicate that the indirect and direct effects of the mediating variables all do not contain 0 within the 95% confidence interval based on the percentile method and the bias-corrected percentile method, meaning that mediation effect of variables are significant. Therefore, technological innovation, industrial structure upgrading, and energy consumption structure all have a mediation effect on the impact of green credit on EWP, which is the same as the result of Table 6.
5.5 Robustness test
To test the robustness of the empirical results, two methods are chosen for re-estimation of models. First, referring to the method of Qi and Yang (2018), the geographic distance weight matrix is used to re-estimate models. The results are shown in Table 8. Second, different measurement methods of variables are selected for re-estimation of model on the basis of the nested weight matrix. The results are shown in Table 9. Among them, the number of patent applications is used as a substitute variable for scientific and technological innovation; the proportion of output value of tertiary industry in GDP is utilized as substitute variable for industrial structure upgrading; the ratio of natural gas consumption to total energy consumption is selected as a substitute variable for energy consumption structure.
From Table 8 and Table 9, it can be seen that the impact of green credit on EWP and the mediation role of technological innovation, industrial structure upgrading, and energy consumption structure is basically the same as the results of Table 5 and Table 6. To sum up, the results of this paper are robust.
6 Conclusion, discussion and policy recommendations
Exploring the link between environmental regulation and high-quality economic development is the primary theme of sustainable development economics and green economics. This study utilizes panel data from 30 provinces in China during the period 2008–2019 to explore the association between China’s green credit and EWP. First, on the basis of using Moran’s I test and Moran scatter plot to analyze the spatial distribution characteristics of EWP, the spatial Durbin model is used to explore the spatial impact of green credit on EWP. Second, the mediating effect model is used to specifically analyze the mediation role of technological innovation, industrial structure upgrading, and energy consumption structure. In the light of the empirical results, this part draws and discusses the major conclusions, and then proposes policy recommendations.
6.1 Conclusion and discussion
First, China’s EWP shows significantly positive spatial correlation in both temporal and spatial dimensions, which is basically consistent with existing research results (Feng et al., 2019). Therefore, future studies on EWP must take its spatial autocorrelation into account. In addition, the value of EWP is characterized by high–high aggregation and low–low aggregation, which indicates that regions with high (low) EWP levels are surrounded by regions with high (low) EWP in China. The positive spatial correlation of EWP may be due to the following reasons. On the one hand, knowledge, technology diffusion and industrial transfer in provinces with high EWP can improve the supply of capital elements such as manpower and technology in areas with lagging EWP, which will help improve the EWP of adjacent areas. On the other hand, the effect of learning, demonstration and cooperation between adjacent regions is also conducive to the improvement of EWP in underdeveloped regions.
Second, the results of spatial Durbin model show that green credit can significantly promote the EWP, but has a negative spatial spillover effect on the EWP of adjacent areas. According to the existing research findings, it can be explained as follows. The differentiated lending policy of green credit may not only offer environmental protection firms adequate finances to promote the execution of environmental protection projects, but also push the two-high enterprises to enter the clean industry. This facilitates industrial structure upgrading and the reduction of environmental pollution (Peng et al., 2022). Furthermore, under the supervision of financial institutions, the efficiency of enterprise operation, technology research, development and application has been gradually optimized. In the process, more welfare outputs are obtained and EWP is improved in local regions. However, according to the “pollution shelter effect” (Zhang et al., 2021a), two-high enterprises will relocate to regions with looser environmental rules in order to escape the high expenses brought by green credit. This will increase the environmental pollution and limit the enhancement of EWP in transferred areas.
Third, the results of the mediating effect model show that green credit can improve EWP by promoting technological innovation, industrial structure upgrading, and energy consumption structure. Through the differentiated loan terms implemented by banks, environmental protection enterprises can obtain sufficient loan funds to increase investment in research and development, hence, fostering ultimately technological innovation. Then, as a result of “the innovation compensation effect,” the two-high enterprises would adjust their internal structures, develop clean processes, and raise the level of technological innovation even further. In this process, traditional energy consumption can be transformed into a mode of clean consumption reduction, which is conducive to optimizing the structure of energy consumption and achieving industrial structure upgrading. Through technological innovation, industrial structure upgrading, and the optimization of energy consumption structure, on the one hand, through technological innovation, industrial structure upgrading, and the optimization of energy consumption structure, resource recycling, energy utilization, and pollution control may be made more effective, which is possible to limit resource use and environmental pollution per unit of welfare production. On the other hand, production factors might migrate to higher-level industries, therefore, optimizing allocation efficiency and increasing total factor productivity (TFP). This can allow the society to gain higher welfare output and to realize the improvement of EWP.
Although there are research contributions in this study, there are still shortcomings that need to be improved. First, due to the limits of environmental pollution data, this study only used provincial-level data. In the follow-up research, industry data, and microenterprise data can be collected for targeted analysis of the impact of green credit (He et al., 2019; Zhang et al., 2022a). Second, due to data availability limits, the control variables in the regression model are all socioeconomic factors. The influence of regional natural resource endowment should be further considered in the next study. Third, the threshold effect and heterogeneity effect of green credit are not considered in this study. In the subsequent studies, it is advisable to refer to the existing research methods (Sun et al., 2020) to further analyze the nonlinear impact of green credit policy. In addition, the heterogeneity effect of green credit can be analyzed according to regional or development level differences (Hu et al., 2022).
6.2 Policy recommendations
(1) Improve the order of interprovincial cooperation and competition, and utilize the spatial positive autocorrelation effect of EWP. The government and other relevant functional departments should strengthen relevant laws and regulations, actualize the cross-regional connection mechanism of environmental governance. In this process, enterprises can drive the common growth of China’s EWP by making full use of the positive spatial correlation of EWP and realizing healthy competition in the field of environmental protection.
(2) Complete the policy system of green credit and build green channel for credit funds. The government, financial institutions, and microenterprises fully play the synergy, establish credit tightening mechanism for two-high enterprises and loan incentive mechanism for environmental protection enterprises. In this process, safeguard measures, such as green credit approval and risk assessment should be improved. Credit data connection platform among government departments, financial institutions, and microeconomic individuals should be built to enhance the circulation of green credit funds.
(3) Play the supervision role of financial institutions, alleviate the risks of enterprise innovation and transformation, and adjust the propensity of energy consumption. Green credit needs to implement differentiated loan policies to encourage more credit funds to invest in green and environmental protection industries, which is conducive to the realization of technological innovation. At the same time, the supply of loans to high-pollution enterprises should be tightened, forcing them to adjust their internal structures and promote the green transformation and upgrading of their industrial structures. Furthermore, the People’s Bank of China should adjust the credit rationing system, which can increase the proportion of clean and renewable energy consumption and improve the energy consumption structure.
Data availability statement
The original contributions presented in the study are included in the article/Supplementary Material, further inquiries can be directed to the corresponding author.
Author contributions
RZ: conceptualization; validation; data curation; writing—review and editing; project administration; funding acquisition. YC: methodology; software; data curation; visualization; writing—review and editing. KW: Conceptualization; visualization; data curation. HS: validation; writing—review and editing; supervision. All authors have read and agreed to the published version of the manuscript.
Funding
This research was funded by the National Social Science Fund of China (grant number 21BGL269).
Acknowledgments
The authors sincerely thank the editor and reviewers for valuable comments and suggestions.
Conflict of interest
The authors declare that the research was conducted in the absence of any commercial or financial relationships that could be construed as a potential conflict of interest.
Publisher’s note
All claims expressed in this article are solely those of the authors and do not necessarily represent those of their affiliated organizations, or those of the publisher, the editors, and the reviewers. Any product that may be evaluated in this article, or claim that may be made by its manufacturer, is not guaranteed or endorsed by the publisher.
Supplementary Material
The Supplementary Material for this article can be found online at: https://www.frontiersin.org/articles/10.3389/fenvs.2022.950341/full#supplementary-material
References
An, S., Li, B., Song, D., and Chen, X. (2021). Green credit financing versus trade credit financing in a supply chain with carbon emission limits. Eur. J. Oper. Res. 292, 125–142. doi:10.1016/j.ejor.2020.10.025
Bian, J., Ren, H., and Liu, P. (2020). Evaluation of urban ecological well-being performance in China: A case study of 30 provincial capital cities. J. Clean. Prod. 254, 120109. doi:10.1016/j.jclepro.2020.120109
Cao, Y., Zhang, Y., Yang, L., Li, R. Y. M., and Crabbe, M. J. C. (2021). Green credit policy and maturity mismatch risk in polluting and non-polluting companies. Sustainability 13 (7), 3615. doi:10.3390/su13073615
Chai, S., Zhang, K., Wei, W., Ma, W., and Abedin, M. Z. (2022). The impact of green credit policy on enterprises' financing behavior: evidence from Chinese heavily-polluting listed companies. J. Clean. Prod. 363, 132458. doi:10.1016/j.jclepro.2022.132458
Chen, L., Ye, W., Huo, C., and James, K. (2020). Environmental regulations, the industrial structure, and high-quality regional economic development: evidence from China. Land 9 (12), 517. doi:10.3390/land9120517
Chen, Z., Zhang, Y., Wang, H., Ouyang, X., and Xie, Y. (2022). Can green credit policy promote low-carbon technology innovation? J. Clean. Prod. 359, 132061. doi:10.1016/j.jclepro.2022.132061
Cheng, G., and wang, Z. (2022). Technology innovation and regional ecological welfare performance under environmental decentralization. East. Chin. Econ. Manage 36, 44–54. doi:10.19629/j.cnki.34-1014/f.210518003
Cheng, Q., Lai, X., Liu, Y., Yang, Z., and Liu, J. (2021). The influence of green credit on China’s industrial structure upgrade: evidence from industrial sector panel data exploration. Environ. Sci. Pollut. Res. 29, 22439–22453. doi:10.1007/s11356-021-17399-1
Cui, X., Wang, P., Sensoy, A., Nguyen, D. K., and Pan, Y. (2022). Green credit policy and corporate productivity: evidence from a quasi-natural experiment in China. Technol. Forecast. Soc. Change 177, 121516. doi:10.1016/j.techfore.2022.121516
Deng, Y., Yang, X., Ma, Q., and Wang, K. (2021). Regional disparity and convergence of China’s ecological welfare performance level. Chin. J. Popul. Resour. Environ. 31, 132–143. doi:10.12062/cpre.20200637
Dietz, T., Rosa, E. A., and York, R. (2012). Environmentally efficient well-being: is there a Kuznets curve? Appl. Geogr. 32 (1), 21–28. doi:10.1016/j.apgeog.2010.10.011
Feng, Y., and Shen, Q. (2021). How does green credit policy affect total factor productivity at the corporate level in China: the mediating role of debt financing and the moderating role of financial mismatch. Environ. Sci. Pollut. Res. 29, 23237–23248. doi:10.1007/s11356-021-17521-3
Feng, Y., Zhong, S., Li, Q., Zhao, X., and Dong, X. (2019). Ecological well-being performance growth in China (1994–2014): from perspectives of industrial structure green adjustment and green total factor productivity. J. Clean. Prod. 236, 117556. doi:10.1016/j.jclepro.2019.07.031
Feng, Z., and Chen, W. (2018). Environmental regulation, green innovation, and industrial green development: an empirical analysis based on the Spatial Durbin model. Sustainability 10 (1), 223. doi:10.3390/su10010223
Fu, L. (2010). An empirical research on industry structure and economic growth. Stat. Res. 27, 79–81. doi:10.19343/j.cnki.11-1302/c.2010.08.011
Geng, X. (2020). Perception of ecological welfare from the recycling of agricultural resources and its influencing factors. Planning 15 (4), 531–536. doi:10.18280/ijsdp.150413
Guo, Q., Zhou, M., Liu, N., and Wang, Y. (2019). Spatial effects of environmental regulation and green credits on green technology innovation under low-carbon economy background conditions. Int. J. Environ. Res. Public Health 16 (17), 3027. doi:10.3390/ijerph16173027
Han, F., Xie, R., and Fang, J. (2018). Urban agglomeration economies and industrial energy efficiency. Energy 162, 45–59. doi:10.1016/j.energy.2018.07.163
He, L., Zhang, L., Zhong, Z., Wang, D., and Wang, F. (2019). Green credit, renewable energy investment and green economy development: empirical analysis based on 150 listed companies of China. J. Clean. Prod. 208, 363–372. doi:10.1016/j.jclepro.2018.10.119
Hong, M., Li, Z., and Drakeford, B. (2021). Do the green credit guidelines affect corporate green technology innovation? empirical research from China. Int. J. Environ. Res. Public Health 18 (4), 1682. doi:10.3390/ijerph18041682
Hou, J., Ruan, X., Lv, J., and Guo, H. (2020). Two-stage super-efficiency slacks-based model to assess China’s ecological wellbeing. Int. J. Environ. Res. Public Health 17 (19), 7045. doi:10.3390/ijerph17197045
Hu, G., Wang, X., and Wang, Y. (2021a). Can the green credit policy stimulate green innovation in heavily polluting enterprises? evidence from a quasi-natural experiment in China. Energy Econ. 98, 105134. doi:10.1016/j.eneco.2021.105134
Hu, M., Sarwar, S., and Li, Z. (2021b). Spatio-temporal differentiation mode and threshold effect of yangtze river Delta urban ecological well-being performance based on network DEA. Sustainability 13 (8), 4550. doi:10.3390/su13084550
Hu, Q., Li, X., and Feng, Y. (2022). Do green credit affect green total factor productivity? empirical evidence from China. Front. Energy Res. 9. doi:10.3389/fenrg.2021.821242
Hu, Y., Jiang, H., and Zhong, Z. (2020). Impact of green credit on industrial structure in China: theoretical mechanism and empirical analysis. Environ. Sci. Pollut. Res. 27 (10), 10506–10519. doi:10.1007/s11356-020-07717-4
Hu, Z., Lan, F., and Xu, H. (2021c). Green TFP heterogeneity in the ports of China’s pilot free trade zone under environmental constraints. Int. J. Environ. Res. Public Health 18 (24), 12910. doi:10.3390/ijerph182412910
Huang, J., Cai, X., Huang, S., Tian, S., and Lei, H. (2019). Technological factors and total factor productivity in China: evidence based on a panel threshold model. China Econ. Rev. 54, 271–285. doi:10.1016/j.chieco.2018.12.001
Jorgenson, A. K., and Dietz, T. (2015). Economic growth does not reduce the ecological intensity of human well-being. Sustain. Sci. 10 (1), 149–156. doi:10.1007/s11625-014-0264-6
Knight, K. W., and Rosa, E. A. (2011). The environmental efficiency of well-being: a cross-national analysis. Soc. Sci. Res. 40 (3), 931–949. doi:10.1016/j.ssresearch.2010.11.002
Li, C., Zhang, S., Zhang, W., and Liao, X. (2019). Measurement and influencing factors of inter-provincial ecological well-being performance in China. Sci. Geogr. Sin. 39, 1875–1883. doi:10.13249/j.cnki.sgs.2019.12.005
Li, J., and Li, S. (2020). Energy investment, economic growth and carbon emissions in China—empirical analysis based on spatial durbin model. Energy Policy 140, 111425. doi:10.1016/j.enpol.2020.111425
Li, R., and Chen, Y. (2022). The influence of a green credit policy on the transformation and upgrading of heavily polluting enterprises: a diversification perspective. Econ. Anal. Policy 74, 539–552. doi:10.1016/j.eap.2022.03.009
Lin, L., Meng, C., Liu, H., Liu, M., and Zhang, Q. (2020). Cost and benefit accounting of green credit in China. Chin. J. Environ. Manag. 12, 115–124. doi:10.16868/j.cnki.1674-6252.2020.05.115
Liu, B., Wang, J., Li, R. Y. M., Peng, L., and Mi, L. (2022). Achieving carbon neutrality – the role of heterogeneous environmental regulations on urban green innovation. Front. Ecol. Evol. 10, 923354. doi:10.3389/fevo.2022.923354
Liu, K., and Lin, B. (2019). Research on influencing factors of environmental pollution in China: a spatial econometric analysis. J. Clean. Prod. 206, 356–364. doi:10.1016/j.jclepro.2018.09.194
Liu, L., and He, L.-Y. (2021). Output and welfare effect of green credit in China: evidence from an estimated DSGE model. J. Clean. Prod. 294, 126326. doi:10.1016/j.jclepro.2021.126326
Liu, P., Zhu, B., and Yang, M. (2021). Has marine technology innovation promoted the high-quality development of the marine economy?——evidence from coastal regions in China. Ocean. Coast. Manag. 209, 105695. doi:10.1016/j.ocecoaman.2021.105695
Long, L. (2019). Evaluation of urban ecological well-being performance of Chinese major cities based on two-stage super-efficiency network SBM Model. Chin. J. Popul. Resour. Environ. 29, 1–10. doi:10.12062/cpre.20190102
Long, X., Yu, H., Sun, M., Wang, X.-C., Klemeš, J. J., Xie, W., et al. (2020). Sustainability evaluation based on the three-dimensional ecological footprint and human development index: a case study on the four island regions in China. J. Environ. Manage. 265, 110509. doi:10.1016/j.jenvman.2020.110509
Ma, Q., Murshed, M., and Khan, Z. (2021a). The nexuses between energy investments, technological innovations, emission taxes, and carbon emissions in China. Energy Policy 155, 112345. doi:10.1016/j.enpol.2021.112345
Ma, X., Ma, W., Zhang, L., Shi, Y., Shang, Y., Chen, H., et al. (2021b). The impact of green credit policy on energy efficient utilization in China. Environ. Sci. Pollut. Res. 28 (37), 52514–52528. doi:10.1007/s11356-021-14405-4
Ma, X., and Xu, J. (2022). Impact of environmental regulation on high-quality economic development. Front. Environ. Sci. 10, 896892. doi:10.3389/fenvs.2022.896892
Naigang, C., and Lin, Z. (2021). Analysis of the spatial-temporal pattern and influencing factors of ecological welfare performance in shandong province. Resour. Dev. Mark. 7, 787–792. doi:10.3969/j.issn.1005-8141.2021.07.004
Ouyang, X., Li, Q., and Du, K. (2020). How does environmental regulation promote technological innovations in the industrial sector? evidence from Chinese provincial panel data. Energy Policy 139, 111310. doi:10.1016/j.enpol.2020.111310
Pan, X., Guo, S., Li, M., and Song, J. (2021). The effect of technology infrastructure investment on technological innovation——a study based on spatial durbin model. Technovation 107, 102315. doi:10.1016/j.technovation.2021.102315
Peng, B., Yan, W., Elahi, E., and Wan, A. (2022). Does the green credit policy affect the scale of corporate debt financing? evidence from listed companies in heavy pollution industries in China. Environ. Sci. Pollut. Res. 29 (1), 755–767. doi:10.1007/s11356-021-15587-7
Pingfang, Z., Zhengyu, Z., and Guolin, J. (2011). Empirical study of the relationship between FDI and environmental regulation: an intergovernmental competition perspective. Econ. Res. J. 6, 133–145.
Qi, Y., and Yang, F. (2018). Research on regional innovation ability based on optimal financial structure theory. Sci. Technol. Prog. Policy. 35, 52–58. doi:10.6049/kjjbydc.L201808635
Scholtens, B., and Dam, L. (2007). Banking on the equator. Are banks that adopted the equator principles different from non-adopters? World Dev. 35 (8), 1307–1328. doi:10.1016/j.worlddev.2006.10.013
Shaohua, W., Weiyang, Y., and Wei, Z. (2015). Influence mechanism of energy structure on low-carbon economy in China. Forum Sci. Technol. China 1, 119–124. doi:10.13580/j.cnki.fstc.2015.01.022
Shuai, S., and Fan, Z. (2020). Modeling the role of environmental regulations in regional green economy efficiency of China: empirical evidence from super efficiency DEA-Tobit model. J. Environ. Manage. 261, 110227. doi:10.1016/j.jenvman.2020.110227
Song, M., Xie, Q., and Shen, Z. (2021). Impact of green credit on high-efficiency utilization of energy in China considering environmental constraints. Energy Policy 153, 112267. doi:10.1016/j.enpol.2021.112267
Song, Y., and Mei, D. (2022). Sustainable development of China's regions from the perspective of ecological welfare performance: analysis based on GM (1, 1) and the malmquist index. Environ. Dev. Sustain. 24 (1), 1086–1115. doi:10.1007/s10668-021-01485-y
Sun, H., Edziah, B. K., Sun, C., and Kporsu, A. K. (2021). Institutional quality and its spatial spillover effects on energy efficiency. Socioecon. Plann. Sci. 101023. doi:10.1016/j.seps.2021.101023
Sun, H., Samuel, C. A., Amissah, J. C. K., Taghizadeh-Hesary, F., and Mensah, I. A. (2020). Non-linear nexus between CO2 emissions and economic growth: a comparison of OECD and B&R countries. Energy 212, 118637. doi:10.1016/j.energy.2020.118637
Sun, J., Miao, J., Mu, H., Xu, J., and Zhai, N. (2022). Sustainable development in marine economy: assessing carrying capacity of shandong province in China. Ocean. Coast. Manag. 216, 105981. doi:10.1016/j.ocecoaman.2021.105981
Tan, X., Yan, Y., and Dong, Y. (2022). Peer effect in green credit induced green innovation: an empirical study from China's green credit guidelines. Resour. Policy 76, 102619. doi:10.1016/j.resourpol.2022.102619
Tian, C., Li, X., Xiao, L., and Zhu, B. (2022). Exploring the impact of green credit policy on green transformation of heavy polluting industries. J. Clean. Prod. 335, 130257. doi:10.1016/j.jclepro.2021.130257
Velenturf, A. P., and Jopson, J. S. (2019). Making the business case for resource recovery. Sci. Total Environ. 648, 1031–1041. doi:10.1016/j.scitotenv.2018.08.224
Wang, E., Liu, X., Wu, J., and Cai, D. (2019a). Green credit, debt maturity, and corporate investment—evidence from China. Sustainability 11 (3), 583. doi:10.3390/su11030583
Wang, F., Yang, S., Reisner, A., and Liu, N. (2019b). Does green credit policy work in China? the correlation between green credit and corporate environmental information disclosure quality. Sustainability 11 (3), 733. doi:10.3390/su11030733
Wang, R., and Feng, Y. (20202020). Research on China’s ecological welfare performance evaluation and improvement path from the perspective of high-quality development. Math. Probl. Eng., 1–14. doi:10.1155/2020/5476089
Wang, R. (2020). The influence of environmental regulation on the efficiency of China’s regional green economy based on the gMM model. Pol. J. Environ. Stud. 29 (3), 2395–2402. doi:10.15244/pjoes/111234
Wang, S., Zhang, Y., and Yao, X. (2021). Research on spatial unbalance and influencing factors of ecological well-being performance in China. Int. J. Environ. Res. Public Health 18 (17), 9299. doi:10.3390/ijerph18179299
Wang, Y., Lei, X., Long, R., and Zhao, J. (2020). Green credit, financial constraint, and capital investment: evidence from China’s energy-intensive enterprises. Environ. Manage. 66 (6), 1059–1071. doi:10.1007/s00267-020-01346-w
Wang, Z., and Lin, X. (2021). Research on local green finance practice and development path in China—based on the reform of pilot zones. Environ. Prot. 49, 61–64. doi:10.14026/j.cnki.0253-9705.2021.05.013
Wen, H., Lee, C.-C., and Zhou, F. (2021). Green credit policy, credit allocation efficiency and upgrade of energy-intensive enterprises. Energy Econ. 94, 105099. doi:10.1016/j.eneco.2021.105099
Wen, Z., and Ye, B. (2014). Analyses of mediating effects: the development of methods and models. Adv. Psychol. Sci. 22 (5), 731. doi:10.3724/SP.J.1042.2014.00731
Xiao, L., and Zhang, X. (2019). Spatio-temporal characteristics of coupling coordination between green innovation efficiency and ecological welfare performance under the concept of strong sustainability. J. Nat. Resour. 34 (2), 312. doi:10.31497/zrzyxb.20190208
Xie, R., Fu, W., Yao, S., and Zhang, Q. (2021). Effects of financial agglomeration on green total factor productivity in Chinese cities: insights from an empirical spatial durbin model. Energy Econ. 101, 105449. doi:10.1016/j.eneco.2021.105449
Xie, T., and Liu, J. (2019). How does green credit affect China’s green economy growth? Chin. J. Popul. Resour. Environ. 29, 89–90. doi:10.12062/cpre.20190501
Xing, C., Zhang, Y., and Wang, Y. (2020). Do banks value green management in China? the perspective of the green credit policy. Financ. Res. Lett. 35, 101601. doi:10.1016/j.frl.2020.101601
Xu, S.College of Economics and Management, Hunan Institute of Technology, Henghua Road 18, Zhuhui, Hengyang City, Hunan Province, China (2020). International comparison of green credit and its enlightenment to china. Green Financ. 2, 75–99. doi:10.3934/GF.2020005
Yan, D., Lei, Y., Li, L., and Song, W. (2017). Carbon emission efficiency and spatial clustering analyses in China’s thermal power industry: evidence from the provincial level. J. Clean. Prod. 156, 518–527. doi:10.1016/j.jclepro.2017.04.063
Yao, L., Yu, Z., Wu, M., Ning, J., and Lv, T. (2020). The spatiotemporal evolution and trend prediction of ecological wellbeing performance in china. Land 10 (1), 12. doi:10.3390/land10010012
Yao, S., Pan, Y., Sensoy, A., Uddin, G. S., and Cheng, F. (2021). Green credit policy and firm performance: what we learn from china. Energy Econ. 101, 105415. doi:10.1016/j.eneco.2021.105415
Yin, W., Zhu, Z., Kirkulak-Uludag, B., and Zhu, Y. (2021). The determinants of green credit and its impact on the performance of chinese banks. J. Clean. Prod. 286, 124991. doi:10.1016/j.jclepro.2020.124991
Yuanjian, D., Xu, Y., Qiangwen, M., and Kaida, W. (2021). Regional disparity and convergence of china’s ecological welfare performance level. China Popul. Resour. Environ. 4, 132–143.
Zhang, A., Deng, R., and Wu, Y. (2022a). Does the green credit policy reduce the carbon emission intensity of heavily polluting industries?-evidence from China's industrial sectors. J. Environ. Manage. 311, 114815. doi:10.1016/j.jenvman.2022.114815
Zhang, B., Yang, Y., and Bi, J. (2011). Tracking the implementation of green credit policy in china: top-down perspective and bottom-up reform. J. Environ. Manage. 92 (4), 1321–1327. doi:10.1016/j.jenvman.2010.12.019
Zhang, D. (2021). Green credit regulation, induced R&D and green productivity: revisiting the porter hypothesis. Int. Rev. Financial Analysis 75, 101723. doi:10.1016/j.irfa.2021.101723
Zhang, D., and Kong, Q. (2022). Credit policy, uncertainty, and firm R&D investment: A quasi-natural experiment based on the green credit guidelines. Pacific-Basin Finance J. 73, 101751. doi:10.1016/j.pacfin.2022.101751
Zhang, K., Li, Y., Qi, Y., and Shao, S. (2021a). Can green credit policy improve environmental quality? evidence from China. J. Environ. Manage. 298, 113445. doi:10.1016/j.jenvman.2021.113445
Zhang, K., Wang, Y., and Huang, Z. (2021b). Do the green credit guidelines affect renewable energy investment? empirical research from china. Sustainability 13 (16), 9331. doi:10.3390/su13169331
Zhang, S., Wu, Z., Wang, Y., and Hao, Y. (2021c). Fostering green development with green finance: an empirical study on the environmental effect of green credit policy in china. J. Environ. Manage. 296, 113159. doi:10.1016/j.jenvman.2021.113159
Zhang, S., Zhu, D., Shi, Q., and Cheng, M. (2018). Which countries are more ecologically efficient in improving human well-being? an application of the index of ecological well-being performance. Resour. Conserv. Recycl. 129, 112–119. doi:10.1016/j.resconrec.2017.10.015
Zhang, Y., Li, X., and Xing, C. (2022b13038). How does China's green credit policy affect the green innovation of high polluting enterprises? the perspective of radical and incremental innovations. J. Clean. Prod. 336, 130387. doi:10.1016/j.jclepro.2022.130387
Zhao, P., Zeng, L., Li, P., Lu, H., Hu, H., Li, C., et al. (2022a). China's transportation sector carbon dioxide emissions efficiency and its influencing factors based on the EBM DEA model with undesirable outputs and spatial durbin model. Energy 238, 121934. doi:10.1016/j.energy.2021.121934
Zhao, X., Ma, X., Shang, Y., Yang, Z., and Shahzad, U. (2022b). Green economic growth and its inherent driving factors in chinese cities: based on the metafrontier-global-SBM super-efficiency DEA model. Gondwana Res. 106, 315–328. doi:10.1016/j.gr.2022.01.013
Zhou, G., Sun, Y., Luo, S., and Liao, J. (2021). Corporate social responsibility and bank financial performance in China: the moderating role of green credit. Energy Econ. 97, 105190. doi:10.1016/j.eneco.2021.105190
Zhu, D. (2008). Ecological economics: economics and management of sustainable development. Bull. Chin. Acad. Sci. 6, 520–530. doi:10.16418/j.issn.1000-3045.2008.06.010
Keywords: green credit, ecological welfare performance, mechanism analysis, spatial Durbin model, mediating effect model
Citation: Zhang R, Cao Y, Wu K and Sun H (2022) Research on the effect of green credit on ecological welfare performance: Evidence from China. Front. Environ. Sci. 10:950341. doi: 10.3389/fenvs.2022.950341
Received: 22 May 2022; Accepted: 28 June 2022;
Published: 23 August 2022.
Edited by:
Muntasir Murshed, North South University, BangladeshReviewed by:
Ruzi Li, Nanchang University, ChinaRita Yi Man Li, Hong Kong Shue Yan University, Hong Kong SAR, China
Anlu Zhang, Huazhong Agricultural University, China
Copyright © 2022 Zhang, Cao, Wu and Sun. This is an open-access article distributed under the terms of the Creative Commons Attribution License (CC BY). The use, distribution or reproduction in other forums is permitted, provided the original author(s) and the copyright owner(s) are credited and that the original publication in this journal is cited, in accordance with accepted academic practice. No use, distribution or reproduction is permitted which does not comply with these terms.
*Correspondence: Yahui Cao, MjAyMDAyMDAzMUBxZHUuZWR1LmNu