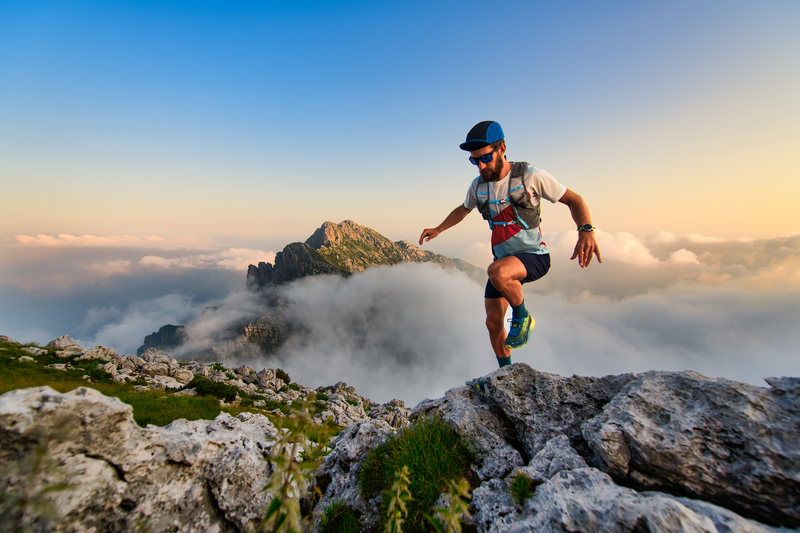
94% of researchers rate our articles as excellent or good
Learn more about the work of our research integrity team to safeguard the quality of each article we publish.
Find out more
ORIGINAL RESEARCH article
Front. Environ. Sci. , 31 August 2022
Sec. Environmental Informatics and Remote Sensing
Volume 10 - 2022 | https://doi.org/10.3389/fenvs.2022.949442
This article is part of the Research Topic Recent Advances in Remote Sensing of Forest Fires View all 5 articles
Wildfires burn heterogeneously across the landscape and create complex forest structures. Quantifying the structural changes in post-fire forests is critical to evaluating wildfire impacts and providing insights into burn severities. To advance the understanding of burn severities at a fine scale, forest structural attributes at the individual tree level need to be examined. The advent of drone laser scanning (DLS) and mobile laser scanning (MLS) has enabled the acquisition of high-density point clouds to resolve fine structures of individual trees. Yet, few studies have used DLS and MLS data jointly to examine their combined capability to describe post-fire forest structures. To assess the impacts of the 2017 Elephant Hill wildfire in British Columbia, Canada, we scanned trees that experienced a range of burn severities 2 years post-fire using both DLS and MLS. After fusing the DLS and MLS data, we reconstructed quantitative structure models to compute 14 post-fire biometric, volumetric, and crown attributes. At the individual tree level, our data suggest that smaller pre-fire trees tend to experience higher levels of crown scorch than larger pre-fire trees. Among trees with similar pre-fire sizes, those within mature stands (age class: > 50 years) had lower levels of crown scorch than those within young stands (age class: 15—50 years). Among pre-fire small- and medium-diameter trees, those experiencing high crown scorch had smaller post-fire crowns with unevenly distributed branches compared to unburned trees. In contrast, pre-fire large-diameter trees were more resistant to crown scorch. At the plot level, low-severity fires had minor effects, moderate-severity fires mostly decreased tree height, and high-severity fires significantly reduced diameter at breast height, height, and biomass. Our exploratory factor analyses further revealed that stands dominated by trees with large crown sizes and relatively wide spacing could burn less severely than stands characterized by regenerating trees with high crown fuel density and continuity. Overall, our results demonstrate that fused DLS-MLS point clouds can be effective in quantifying post-fire tree structures, which facilitates foresters to develop site-specific management plans. The findings imply that the management of crown fuel abundance and configuration could be vital to controlling burn severities.
Wildfires shape the stand structure, species composition, nutrient cycling, as well as many other processes in forest ecosystems (Reilly et al., 2006; Wieder et al., 2009; McGee et al., 2015; Koontz et al., 2020). Extreme wildfire events can result in extensive tree mortality, posing threats to the ecosystem’s biodiversity, resilience, and stability (Crockett and Westerling, 2018; Stevens-Rumann et al., 2018; Steel et al., 2022). With climate warming, rising temperatures and declining precipitation have facilitated the increased incidence of wildfires in many regions across the globe (Liu et al., 2010; Jolly et al., 2015; Davis et al., 2019). Over the past 3 decades, for example, western North America has seen a significant rise in wildfire occurrences, triggering public concerns about environmental, economic, and social impacts (Bartels et al., 2016; Westerling, 2016; Cascio, 2018; Coops et al., 2018; Walker et al., 2019). The historical practice of fire suppression, in conjunction with climate warming, has altered the regime of contemporary wildfires (Prichard et al., 2017; Hanan et al., 2021). Consequently, wildfires are projected to increase in terms of frequency, extent, severity, and duration in the next 50 years, adding barriers to forest management (Flannigan et al., 2013; Prichard et al., 2017; Hanan et al., 2021). Therefore, it is crucial to better understand the impacts of wildfires on fire-prone forests.
To evaluate the magnitude of wildfire impacts on soil and vegetation, post-fire assessments are needed (Robichaud et al., 2014; Klauberg et al., 2019). At an individual tree level, wildfire impacts can be estimated using crown scorch which measures the fire’s consumption of foliage (Wallin et al., 2003). As crown scorch quantifies the areas of discolored foliage following a fire, it is commonly used to indicate the heat damage on individual trees (Hood et al., 2018; Varner et al., 2021). With increasing crown scorch, for example, the foliage color can change from green and yellow (minor damage) to brown and black (complete damage), suggesting an external fire-induced injury (Hood et al., 2018; Varner et al., 2021). Based on crown scorch, the internal injury of individual trees due to fires can also be interpreted since the reduced foliage is associated with many physiological processes, such as water uptake, photosynthesis, and carbon assimilation (Alonso et al., 2002; Wallin et al., 2003; O’Brien et al., 2010).
At a plot level, wildfire impacts can be categorized into different burn-severity classes, such as low, moderate, and high. A low-severity fire (i.e., surface fire) partially consumes the surface fuel, with most of the trees unscorched or lightly scorched (Keeley, 2009). Following a moderate-severity fire, forest structure is altered due to the burning of multiple vegetation strata from ground to canopy (Keeley, 2009; Ager et al., 2013; Kramer et al., 2016). Low- and moderate-severity fires may benefit residual trees with reduced competition and increased seed germination and sprouting (Collins et al., 2018; Jean et al., 2019; Cannon et al., 2021). By contrast, high-severity fires (i.e., crown fires) are characterized by a major loss in the above-ground biomass due to the consumption of surface, ladder, and crown fuels (Chambers et al., 2016; Jones et al., 2016; Garcia et al., 2017). Meanwhile, the prolonged droughts associated with high-severity fires can also cause delayed mortality of remaining trees, further challenging the survival and recovery of trees in forested habitats (Savage et al., 2013; Ruffault et al., 2018; Rodman et al., 2020).
Due to the interacting effects of fuel availability, weather conditions, and topographic features, wildfires burn with a mixture of severities, generating a mosaic pattern of burned and unburned patches within the fire perimeter (Kane et al., 2013; Foster et al., 2017; Crockett and Westerling, 2018; Walker et al., 2020; Churchill et al., 2022). As a result, wildfires can create complex forest structures with high spatial heterogeneity (Bassett et al., 2017; Carlson et al., 2017; Foster et al., 2017). During the post-fire assessment, the evaluation of wildfire impacts on forests can be based on visual estimations (e.g., percentage of ground scorch) and field measurements (e.g., char height) (Robichaud et al., 2007; Chuvieco, 2009). Many structural attributes of forests that are important to examining post-fire biomass, such as crown volume, can be hard to measure in the field (Karna et al., 2019). Therefore, the post-fire assessment may not fully reflect the structural condition of forest ecosystems. Quantifying the post-fire forest structure is, in this case, critical to improving our understanding of wildfire impacts, especially for large and severe fires.
The use of light detection and ranging (LiDAR) or laser scanning technologies allows the acquisition of three-dimensional point clouds to accurately represent the structure of forest stands and individual trees (Goodwin et al., 2006; Wulder et al., 2008). Previous studies conducted across a range of forest types have utilized airborne laser scanning (ALS) data to examine the characteristics of post-fire vegetation (e.g., Botequim et al., 2019; Kane et al., 2019; Gelabert et al., 2020), evaluate the wildfire effects by comparing burned with unburned forest plots (e.g., Alonzo et al., 2017; Hoffman et al., 2018; Hu et al., 2019; Karna et al., 2020), and assess the mortality and recovery of remaining trees with the aid of multispectral satellite imagery (e.g., Kane et al., 2014; Bolton et al., 2015; McCarley et al., 2017; Klauberg et al., 2019). From ALS data, researchers have examined post-fire forest structures across a wide range of forest types globally to improve our local understanding of the impact of wildfires across landscapes (Botequim et al., 2019; Kane et al., 2019; Karna et al., 2019; Karna et al., 2020). Research has shown, for example, that ALS-derived forest canopy cover decreased by ∼ 30% after moderate- and high-severity fires (Karna et al., 2020). High-severity fires also impacted the spacing of dominant vegetation, leading to stands with decreased canopy height, increased canopy gaps, and increased habitat fragmentation (Karna et al., 2020). At the individual-tree level, several structural attributes (e.g., crown dimensions) can also be calculated (Casas et al., 2016; Hu et al., 2019; Klauberg et al., 2019), with researchers finding that, after high-severity fires, trees had significantly smaller crown width, cover, and biomass compared to trees burned by low- or moderate-severity fires (Karna et al., 2019). Tree crowns burned by high-severity fires also became more elongated and conical-shaped instead of round-shaped, implying their reduced recovery and primary productivity (Karna et al., 2019).
The majority of research on the post-fire stand and tree structures, in general, has been undertaken from piloted airborne systems. As laser scanning technologies continue to evolve, it is possible to utilize these devices on an increased variety of platforms, specifically with advances in drone laser scanning (DLS), terrestrial laser scanning (TLS), and mobile laser scanning (MLS). These three platforms, enable researchers to obtain point clouds of ultra-high densities (e.g., 500—20,000 points/m2), which can capture structural information of stands and trees at much finer spatial scales than conventional piloted ALS datasets, thus opening up new possibilities for studies of wildfire effects. Compared to ALS, DLS systems fly much lower to the ground and can be deployed with greater flexibility, allowing the improved detection of post-fire crown structures (Bruggisser et al., 2019; Vandendaele et al., 2021). Using DLS data, studies have estimated various tree structural attributes, such as leaf area density, crown volume, and crown area, to promote the assessment of crown fuels (e.g., Viedma et al., 2020a; Arkin et al., 2021; Hillman et al., 2021). With these attributes, researchers can differentiate crown fires from surface fires by comparing the degree of damage to vegetation structures at different height strata (Viedma et al., 2020a). To gain even greater detail of the sub-canopy vegetation structure, studies have also utilized TLS or MLS data (e.g., Loudermilk et al., 2012; Chen et al., 2016; Hillman et al., 2021). As the sensors are mounted close to the ground and scan upward, dense point clouds can be acquired to resolve the fine structures of trees at the sub-canopy level. These data, thus, support the retrieval of vital attributes, such as crown base height, and allow a refined characterization of fuel loads horizontally and vertically.
Each of these laser scanning platforms offers unique information on the post-fire forest structures. However, to date, there have been a limited number of studies exploring how these datasets can be used together to provide both a ground-up and top-down assessment of post-fire forest structure. To examine the combined ability of these perspectives to predict post-fire tree attributes, we created a fused DLS-MLS dataset to reconstruct individual tree structures after fires. To do so, we sampled trees that experienced a range of crown scorch levels from low-, moderate-, and high-severity sites. We investigated the following questions: 1) At the individual tree level, how is crown scorch related to pre-fire tree size and post-fire tree structures? 2) At the plot level, how does tree size change in response to wildfires with different burn severities? And finally, 3) from the structural attributes of post-fire trees, what can be inferred about the wildfire burn-severity pattern? By examining the differences in post-fire tree structures, this research aims to improve the understanding of relationships between burn severities and tree-level responses, therefore informing fire behavior modelling, fire risk mitigation, and fire-prone forest management.
The Elephant Hill wildfire started on July 6th, 2017, near Ashcroft, British Columbia (BC), Canada, and was not contained until the end of September 2017 (BC Wildfire Services, 2022). This wildfire burned a total area of 191,865 ha, representing one of the largest fires in the 2017 wildfire season (BC Wildfire Services, 2022). It burned across a broad range of elevations and forest types, including naturally regenerating forests dominated by interior Douglas-fir (Pseudotsuga menziesii var. glauca) and young plantations of lodgepole pine (Pinus contorta). Ponderosa pine (Pinus ponderosa) co-dominates at the lowest elevations, while hybrid spruce (Picea engelmannii X Picea glauca) commonly occurs at mid- and high elevations (Meidinger and Pojar, 1991). Trembling aspen (Populus tremuloides) is also distributed across the elevation range (Meidinger and Pojar, 1991). Natural disturbances and forest management, together with the terrain features, have created forests with diverse stand ages and canopy closure conditions (Meidinger and Pojar, 1991). Historically, lower elevations in the study area experienced frequent fires with low-to-moderate severity, while higher-elevation forests burned at longer intervals but with higher severity (Meidinger and Pojar, 1991). However, fire suppression after the 1940s greatly reduced the annual area burned and effectively eliminated lower severity fires, while industrial forest management increased. From 2000 to 2010, lodgepole pine forests were highly impacted by the mountain pine beetle and forest plantations were established after salvage logging.
In BC, the status of forest resources is regularly assessed through three monitoring programs based on permanent sampling plots on a gridded network, including the Change Monitoring Inventory program, the Young Stand Monitoring program, and the National Forest Inventory program (Government of BC, 2022). These plots provide temporal re-measurements of forest stands throughout the province using consistent sampling methods to inform disturbance impacts, timber supply, silviculture practices, and growth and yield modelling (Government of BC, 2022). The Elephant Hill wildfire burned 23 permanent plots, nine of which were located near the center of the fire and could be safely accessed for re-sampling (Figure 1). These nine study sites ranged in elevation from 1014 to 1219 m above sea level (masl) and all were located in the Interior Douglas-fir biogeoclimatic zone (Meidinger and Pojar 1991). Pre-fire forest inventory data provided by the BC Ministry of Forests showed that six sites included mature forests (age class: > 50 years) and three were regenerating plantations (age class: 15–50 years); all were dominated or co-dominated by interior Douglas-fir and lodgepole pine (Table 1). In the summer of 2019, field work was conducted at these nine study sites to evaluate wildfire impacts at individual tree and plot scales, and each study site was scanned using both DLS and MLS, as described below.
FIGURE 1. The location of the study area (A) in the Elephant Hill wildfire that burned in 2017. The distribution of the study sites was highlighted using circles and dots in (B) and (C). The area burned was visualized using a Sentinel-2 image (true color composite) with a 0.5 percent minimum-maximum clip and 1.3 gamma stretch to differentiate burned (magenta) and unburned (green) regions.
TABLE 1. The pre-fire field information of each study site (VRP—variable-radius plot, FRP—fixed-radius plot, masl—meters above sea level, SE—standard error, Fd—Douglas-fir, Pl—lodgepole pine, Sx—hybrid spruce, Py—ponderosa pine, At—trembling aspen)
The most recent field measurements of the study sites (2015–2016) before the Elephant Hill wildfire were used as the pre-fire forest inventory data. Among the nine study sites, 36 trees were measured in fixed-radius plots (r = 11.28m) in 6 mature forests and 172 trees were measured with prisms in 3 regenerating forests (Table 1). For each tree, its key attributes, including species, diameter at breast height (DBH in cm) and height (H in m) were measured in the field; basal area (BA in m2) for each tree was calculated from DBH (BA = Π (DBH/2)2). To assess the pre-fire biomass of stems and branches of individual trees, we used species-specific allometric equations from Ung et al. (2008):
where the dry mass (kg) of tree compartments (i.e., wood, bark, stem, and branches) is represented as y, DBH (cm) and height (m) were manually measured in the field, and the species-specific allometric parameters are denoted as β, and estimation errors are represented as e.
The post-fire conditions of the nine study sites were examined in August and September 2019 by relocating the permanent sampling plots and assessing fire effects at both individual tree and plot scales. To measure the tree-level burn severity, the percentage of crown scorch for each tree that could be matched in the pre-fire inventory data was evaluated. In total, 86 trees were recorded with crown scorch, with 80 coniferous trees (i.e., interior Douglas-fir, lodgepole pine, and hybrid spruce) and 6 trembling aspens.
To estimate the plot-scale burn severity, we calculated the Composite Burn Index (CBI) using the post-fire field data by considering the average impacts of wildfires on five vertical vegetation strata (Key and Benson, 2006; De Santis and Chuvieco, 2009). To do so, we used the CBI concept from Key and Benson (2006) and modified some strata to minimize the assumptions of pre-fire conditions. The five strata were: 1) substrates, 2) understory plants (mosses, bryophytes, herbs and ferns), 3) shrubs, 4) sub-canopy trees, and 5) canopy trees. For this study, our modified CBI is referred to as CBI. On the forest floor, we examined the degree of ground scorch, percentage of exposed soil, and depth of burn classes (Ryan, 1982). We also estimated the percentage of understory plants and shrubs affected by the fire. For sub-canopy and canopy trees, we estimated the percentage of crown scorch and measured the char height on individual trees and averaged them to represent each study site. Table 2 shows the CBI scores for each study site across the five vertical vegetation strata. Finally, we categorized the plot-level burn severity into three classes: 1) low (CBI ≤1.5), 2) moderate (1.5 < CBI ≤2.25), and 3) high (2.25 < CBI ≤3). Examples of study sites from different burn-severity levels are presented in Figure 2.
TABLE 2. The burn severity classification of study sites with CBI scores by vertical vegetation stratum and plot (the dash symbol indicates that there are no sub-canopy trees at the site)
Post-fire laser scanning data were collected using DLS and MLS over 2 days in late July 2019. For the DLS data acquisition, a GreenValley LiAir S200 laser scanning system was used with a ±2 cm horizontal range accuracy and ±5 cm vertical range accuracy. The global navigation satellite systems and the inertial navigation system were integrated into LiAir S200 to georeference the point clouds (relative accuracy = ±5 cm). The LiAir S200 was installed on a DJI Matrice 600 drone to fly at ∼ 80 m above the ground for each study site. The collected DLS point clouds had high densities (>1,000 points/m2) and were clipped for further processing (Figure 3).
FIGURE 3. Examples of DLS, MLS, and fused DLS-MLS point clouds that are classified as different burn-severity levels
MLS data was acquired from a GeoSLAM ZEB Horizon laser scanner which is equipped with 16 sensors that collect >300,000 points/sec with a relative accuracy of ∼6 mm. The scanner uses a simultaneous localization and mapping (SLAM) algorithm to perceive unknown environments while tracking the location and movement of the system (Sammartano and Spanò, 2018). As the forests were consistently scanned, new point clouds were sequentially added to and aligned with previous ones during this process (Bosse et al., 2012; Sammartano and Spanò, 2018). At the end of scanning, the system incorporated a closed-loop algorithm to reduce the accumulated errors from the sequential scanning (Bosse et al., 2012; Sammartano and Spanò, 2018). At each study site, an operator held the laser scanner at the plot center and walked outwards spirally to scan the forests.
The DLS point clouds were used as reference data to register the MLS point clouds with the workflow in Figure 4. After the original DLS and MLS point clouds were clipped (r = 20 m), they were approximately aligned in the CloudCompare software using manual shifting. The features from the DLS point clouds (e.g., the highest tree, trees with large crowns, etc.) guided the process of manual shifting. Once the MLS point clouds roughly overlapped with the DLS point clouds, the iterative closest point (ICP) algorithm from CloudCompare was used to perform the fine registration (Besl and McKay, 1992; CloudCompare, 2015). The ICP algorithm minimized the distance between two paired point clouds, allowing the precise registration of MLS data (Besl and McKay, 1992). To ensure the quality of registration, we calculated the cloud-to-cloud distance in CloudCompare for each pair of MLS and DLS point clouds. If the cloud-to-cloud distance was >5 cm, the steps of approximate alignment and fine registration were repeated. For the final registered MLS point clouds, their distances to the DLS point clouds were ∼3 cm, indicating that they have been precisely aligned. Next, the precisely aligned MLS point clouds were merged with DLS point clouds to create the fused dataset.
FIGURE 4. The workflow of point cloud registration, fusion, and matching with fixed or variable-radius field plots
As the pre-fire plots were sampled using both fixed- and variable-radius methods, we further clipped the laser scanning data to reduce their geometric inconsistencies compared to the sizes and shapes of field plots. For fixed-radius plots, we directly clipped the fused point clouds to circles with a radius of 11.28 m. For variable-radius plots, we identified the horizontal distance between the plot center and each tallied tree from the field data (Figure 4). The largest center-to-tree distance in each field plot was used as the clipping radius for the laser scanning data (Figure 4). The resulting clipping radii ranged from 6.4 to 10.78 m. The average point density of final fused point clouds ranged from 25,498 to 32,743 points/m2 across the burn-severity levels.
The Computree software (Computree, 2021) was used for individual tree segmentation and modelling, as follows. The final fused point clouds were classified into ground and vegetation point clouds (Figure 5). Points tagged as vegetation were then subset into upper (≥1.3 m) and lower canopy sections (<1.3 m). Using the locations of stems identified in the lower canopy section, we applied a Dijkstra-based tree segmentation algorithm, as proposed by Hackenberg et al. (2015), to separate individual trees. This algorithm connects each vegetation point to its closest identified stems using the nearest neighbor search based on the Dijkstra distance (Dijkstra, 1959; Hackenberg et al., 2015).
FIGURE 5. The key processing steps of vegetation point clouds separation, individual tree segmentation, and QSM reconstruction
Quantitative structure models (QSMs) represent the topology, geometry, and volume of objects using a series of hierarchically ordered geometric primitives, such as cylinders (Raumonen et al., 2013; Raumonen et al., 2015). In forestry, QSMs have been recently demonstrated to be an effective methodology to extract detailed information on tree structures in a non-destructive manner. A broad range of forest structural attributes, such as DBH, height, crown size, and volumes, can be accurately retrieved from well-modelled QSMs at an individual tree scale (e.g., Raumonen et al., 2013; Raumonen et al., 2015; Gonzalez de Tanago et al., 2018; Brede et al., 2019). In this study, we reconstructed the structure of individual trees with QSMs based on the methods proposed by Hackenberg et al. (2014) and Hackenberg et al. (2015). The major steps of the QSM reconstruction are outlined in Figure 5.
First, we applied the basic sphere following algorithm to denoised tree clouds (Hackenberg et al., 2015). During this process, a search sphere was created to partition each tree cloud into small segments. Initial cylinders were then generated inside the search sphere to match the surface points of each segment. As the search sphere moved upwards to the treetop, the initial cylinders were linked following a topological order to form the basic QSM. Meanwhile, we ran the QSM-based tree cloud clustering function (Hackenberg et al., 2015) to align the point clouds with their closest cylinders and separate them into two main clusters: 1) the stem and 2) branch clusters. Next, we performed the advanced sphere following function to improve the modelling of the stem and branches separately using the clustered point clouds (Hackenberg et al., 2015). For each cluster, the cylinders were fit with the surface points iteratively to reduce modelling errors. Cylinders with extra-large or small radii compared to their neighbors were filtered and replaced using the median radii of the adjacent cylinders. To further optimize the modelling of QSMs with accurate cylinder sizes, stem and branch cylinders were corrected based on the allometric relationship between cylinder radii and accumulated cylinder volume, as described by Hackenberg et al. (2015). The individual tree QSMs were grouped based on the plot-level burn-severity of their study sites (Figure 6). To pair individual tree QSMs with post-fire field measurements, we compared their spatial coordinates and used a buffer range of the average crown size in each plot to match the corresponding tree datasets.
We extracted post-fire biometric, crown, and volumetric attributes at the individual tree level using QSMs (Table 3). In terms of biometric attributes, we retrieved the diameter of stem cylinders at 1.3 m above ground as an estimate of DBH. The coordinates of the highest and lowest cylinders were used to calculate the tree height. Using the DBH and tree height, we computed the biomass using the aforementioned allometric equations (Ung et al., 2008). Eight crown attributes were examined in this study to quantitatively describe the horizontal and vertical structure of tree crowns. The horizontal attributes include crown diameter, crown projection area (CPA), crown compactness, and crown evenness. The vertical attributes include crown base height (CBH), crown length, crown ratio, and crown elongation. To extract the crown attributes, we isolated the branch cylinders from QSMs. The coordinates of the branch cylinders were used to compute the crown diameter, CBH, and crown length. With these three attributes, we further derived crown ratio and crown elongation. Of all the branch cylinders from a QSM, we identified the outermost ones and connected their locations to delineate the 2D shape of crowns on the horizontal plane. From this 2D shape, we calculated the CPA and crown compactness. The crown evenness index was adapted from Åkerblom et al. (2017) to evaluate the lateral distribution of branches. In addition, we also summarized the stem, branch, and total volumes of individual trees using the volumetric information provided by QSMs.
We performed an initial Kruskal–Wallis test (Kruskal and Wallis, 1952) using the field data and did not find significant differences among interior Douglas-fir, lodgepole pine, and hybrid spruce in terms of pre-fire DBH, height, and biomass (see Supplementary Figures S1–3). These coniferous trees also experienced similar levels of crown scorch regardless of species (see Supplementary Figure S4). Since there were only six trembling aspens recorded in the field data, their impact on the analyses of post-fire tree structure was anticipated to be minor. Overall, this pre-fire analysis suggests that pre-fire tree species should not be a significant confounding factor in the subsequent analyses.
To understand the impacts of wildfires on individual tree structures, we first examined the relationship between crown scorch and trees of different pre-fire sizes (DBH, height, and biomass) in mature (age class: > 50 years) and young (age class: 15—50 years) stands. We quantified the relationship using Spearman’s rank correlation coefficient considering the non-normal distribution of the data (Gauthier, 2001). This coefficient measures both the direction and strength of the correlation between two variables based on the ranks of the data (Gauthier, 2001).
where di denotes the difference between the ranks of each pair of observations and n represents the number of observations. The coefficient (R) ranges between -1 and +1, with R > 0 indicating a positive correlation, R = 0 indicating no correlation, and R < 0 indicating a negative correlation. The stronger the correlation, the greater the absolute value of R.
To compare the post-fire tree structures across a range of crown scorch levels, we used pre-fire DBH to separate individual trees into three groups according to the provincial standards of timber cruising (Government of BC, 2021): 1) small (DBH <12.5 cm), 2) medium (12.5 ≤ DBH <30 cm), and 3) large (DBH ≥30 cm). For each group of trees, we examined the relationship between crown scorch and post-fire tree attributes using Spearman’s rank correlation coefficient (Gauthier, 2001).
To further understand the burn severity at the plot level, we aggregated individual trees based on the CBI score of their study sites. We examined the changes in pre-and post-fire tree attributes using the paired Wilcoxon rank-sum test. This test is a non-parametric technique to compare the ranking of observations of two groups of paired samples (Cuzick, 1985; Wilcoxon, 1992). The three biometric attributes investigated were DBH, tree height, and biomass. DBH and height were manually measured in the field before the fire and were derived from QSMs after the fire. Pre- and post-fire biomass were calculated from species-specific allometric equations based on DBH and height, as described above.
Exploratory factor analysis (EFA) is a multivariate technique that aims to uncover the underlying patterns and relationships among the variables (Taherdoost et al., 2014). In this study, we performed EFA on tree attributes to infer the burn-severity pattern of wildfires at the plot level. Before extracting common factors, we assessed the data adequacy and suitability using Kaiser-Meyer-Olkin (KMO) and Bartlett’s tests. The KMO test measures the correlations among variables in the correlation matrix for EFA, which provides information on the sampling adequacy that is important for the grouping of variables (Kaiser, 1970; Taherdoost et al., 2014). The statistic of KMO ranges from 0 to 1, with values below 0.5 meaning that the data are not adequate for EFA (Taherdoost et al., 2014). Bartlett’s test of Sphericity examines whether the correlation matrix is the same as an identity matrix (Bartlett, 1950). A significant result (p < 0.05) is needed to ensure that the data are suitable for EFA (Taherdoost et al., 2014). After confirming the data adequacy and suitability, we extracted uncorrelated factors using a principal component method and they were further rotated using the equamax rotation technique (Manly, 2005). With this orthogonal rotation technique, the data variance was re-distributed across the common factors to minimize the complexity between them and the variables (Akhtar-Danesh, 2017). Using the common factors, we compared their loadings across burn-severity levels to study their correlations with the variables. The meanings of the common factors were then interpreted as each of them encompassed a unique set of relationships with the post-fire tree attributes. We used 0.80 as the cut-off value for strong correlations when defining the meanings of common factors from their loadings. The meanings of common factors across burn severities may indicate the differences in the burn-severity pattern of wildfires.
All statistical analyses were conducted in RStudio (RStudio Team, 2020).
Figure 7 shows the correlations between crown scorch and trees in mature and young stands with different pre-fire sizes. Overall, we found significant and negative correlations between crown scorch and pre-fire DBH, height, and biomass, indicating that trees with small pre-fire sizes tend to experience higher levels of crown scorch than trees with large pre-fire sizes. In addition, the scatter plots show that young stands (age class: 15—50 years) were mostly occupied by small trees, whereas mature stands (age class: > 50 years) included trees of a range of sizes. Among trees from young stands, the majority of them were severely scorched, while trees from mature stands experienced relatively lower levels of crown scorch (Figures 7A–C).
FIGURE 7. The scatter plots and trend lines between crown scorch (%) and pre-fire (A) DBH, (B) height, and (C) biomass of trees at mature and young stands
The correlations between crown scorch and post-fire biometric, crown, and volumetric attributes are presented in Figure 8 and Table 4. Among small-diameter trees, post-fire volumetric and crown attributes exhibited significant and moderate correlations with crown scorch levels. The negative trends indicate that trees that experienced higher levels of crown scorch had smaller post-fire volumes, especially the branch volumes (Table 4). Their post-fire crown structures were also smaller both horizontally and vertically, as well as more compacted and uneven (Figure 8; Table 4). Among medium-diameter trees, we found similar patterns in the relationships between crown scorch and post-fire attributes compared to small-diameter trees. Specifically, post-fire DBH, biomass, total and branch volumes, and most crown attributes were significantly correlated with crown scorch levels (Table 4). In contrast, we did not find any significant correlations between crown scorch and post-fire attributes among large-diameter trees (Figure 8; Table 4). These non-significant correlations indicate that large-diameter trees were relatively more resistant to different levels of crown scorch than small- and medium-diameter trees.
FIGURE 8. The scatter plots and trend lines between crown scorch (%) and post-fire (A) DBH, (B) height, (C) biomass, (D) crown diameter, (E) crown evenness, and (F) CBH among small-, medium-, and large-diameter trees classified using pre-fire DBH
TABLE 4. Spearman’s rank correlation coefficients (R) between crown scorch (%) and each post-fire tree attribute among small-, medium-, and large-diameter trees (significant correlations (p < 0.05) are highlighted in bold).
Impacts of wildfires with different plot-level burn severities on individual tree DBH, height, and biomass are presented in Figure 9. Overall, low-severity fires had negligible effects on individual tree biometric attributes, moderate-severity fires mostly influenced tree height, while high-severity fires significantly reduced the DBH, height, and biomass of individual trees. At low-severity sites, we found no significant differences between pre- and post-fire biometric attributes. At moderate-severity sites, we observed a significant decline (p < 0.05) between pre- and post-fire tree height, with the median values decreasing from 12.5 m to 9.7 m (Figure 9B). By contrast, at high-severity sites, all three biometric attributes were significantly different post-fire from their corresponding pre-fire values. The median values of individual tree DBH, height, and biomass decreased by 16%, 25%, and 29%, respectively (Figure 9A–C).
FIGURE 9. The comparisons between pre-fire field-measured and post-fire laser scanning-derived (A) DBH, (B) tree height, and (C) biomass across plot-level burn-severity classes. The violin plots show the data range of each attribute, with the curves representing the probability density distribution, the boxes representing the interquartile range, the bars representing the median, and the dots representing outliers. Significant differences among medians (p < 0.05) are indicated with different letters.
The exploratory factor analyses (EFA) (Kaiser-Meyer-Olkin > 0.65; p of Bartlett’s test <0.05) with the equamax rotation revealed a clear factor pattern across the burn-severity levels. Four common factors emerged from the EFA, accounting for ∼80% of the total data variance. Factors 1 and 2 were dominant factors with ∼50% combined contribution to the total data variance, and factors 3 and 4 jointly explained ∼30% of the total data variance. The correlation coefficients between common factors and the post-fire tree attributes (i.e., factor loadings) are summarized in Figure 10.
FIGURE 10. The loadings of common factors for post-fire attributes in (A) low, (B) moderate, and (C) high plot-level burn-severities. The loadings represent the correlation coefficients between each post-fire attribute and each factor. The factors are ordered in terms of their contribution to the data variance from high to low.
At low-severity sites (Figure 10A), factor 1 exhibited strong and positive correlations with two crown attributes (i.e., crown diameter and CPA). This factor, thus, reflected the relationship between horizontal crown size and burn severity. Factor 2 showed strong correlations with total, stem, and branch volumes (loadings = ∼ 0.90), which served as an indicator of post-fire tree volumes. Factor 3 was positively correlated with crown ratio (loading = 0.86) and negatively correlated with crown elongation (loading = - 0.83), indicating the vertical crown size of post-fire trees. The last factor recorded great correlations with DBH and biomass, which provided information on the sub-canopy tree size.
At moderate-severity sites (Figure 10B), factor 1 directly correlated with tree height and crown length (loadings >0.89), suggesting an indicator of vertical crown size. Similar to trees from low-severity sites, factor 2 was also strongly and positively correlated with tree volumes, with loadings ranging from 0.89 to 0.94. Factor 3 indicated the sub-canopy tree size as it exhibited substantial correlations with DBH and biomass, with loadings >0.96. Factor 4 reflected the amount of crown fuel as it was positively correlated with CBH (loading = 0.86) and negatively correlated with crown ratio (loading = - 0.82).
At high-severity sites (Figure 10C), the strongest factor became an indicator of tree volumes, with positive loadings ranging from 0.80 (branch volume) to 0.92 (total volume). The next strongest factor offered information on the sub-canopy tree size with direct correlations with DBH and biomass (loadings >0.93). We found no post-fire tree attribute strongly correlated with factor 3 but moderate correlations with crown compactness and elongation, which can be viewed as a reflection of crown shapes. Similar to trees from moderate-severity sites, the last factor also indicated the amount of crown fuel as it was strongly correlated with CBH (loading = - 0.94) and crown ratio (loading = 0.87).
In general, following low-severity fires, we found that the variations in tree characteristics were mainly driven by large horizontal crown sizes and tree volumes, which reflected the relatively greater size and wider spacing of trees in low-severity sites. Following moderate-severity fires, the dominant factors driving the variations in tree characteristics changed to vertical crown sizes and tree volumes, indicating a more prevalent fire impact along the stem. Following high-severity fires, the dominant factors further changed to reduced tree volumes and sub-canopy tree sizes, emphasizing the remaining tree structures with deeply burned crowns.
In this research, we fused DLS and MLS point clouds to examine the structure of post-fire trees using QSMs. Through QSMs, we retrieved biometric, volumetric, and crown attributes of post-fire trees to compare the effects of the tree- and plot-level burn severities. Our method can, thus, be complementary to studies that investigated wildfire impacts on stand and tree structures via computing metrics (e.g., height percentiles, skewness, kurtosis, etc.) directly from ALS point clouds. The findings of this research could refine our understanding of the variation in wildfire impacts on dry forests at both individual tree and plot scales. As with other studies that investigate the chronological impacts of wildfires, we acknowledge that we cannot completely rule out the potential influence of pre-fire conditions on post-fire forests (Kane et al., 2013; Kane et al., 2014). Therefore, we focused on the general trends when interpreting the burn-severity effects and their patterns.
At the individual tree level, our results suggest that trees with small pre-fire sizes tend to be more susceptible to higher levels of crown scorch than trees with large pre-fire sizes. Among small- and medium-diameter trees (DBH <30 cm) that were severely scorched, they tend to have smaller post-fire volumes and crowns compared to the ones that were slightly scorched or unburned. A potential explanation is that, as crown scorch levels increased, a greater amount of branches and foliage was consumed by fires which may result in the compacted and uneven shape of post-fire crowns. We also observed that many small- and medium-diameter trees had relatively low CBH after experiencing low to moderate crown scorch (<60%). The low CBH indicates that fires may have resulted in a degree of crown kill, which triggered basal and epicormic resprouting of trees (Hood et al., 2018; Woodward et al., 2020; Varner et al., 2021). Yet, as the crown scorch level further increased, small- and medium-diameter trees could have post-fire crowns with high CBH and little to no branch volumes. This implies that a high crown scorch could damage trees with top-kill and reduce the occurrence of epicormic resprouting (Hood et al., 2018; Bär et al., 2019). Thus, the structure of smaller and younger trees could be simplified by high levels of crown scorch, and their post-fire survival and recovery could also be threatened due to the severe injury. Larger and more mature trees, however, could be more resistant to fires, which is likely related to the protection of their thick bark.
At the plot level, low-severity fires had relatively minor impacts on tree structures as they mostly consumed surface fuels. Although younger trees may be charred during the burn, their subsequent growth can mask fire effects (Kane et al., 2014; Hoffman et al., 2018). This finding is similar to multiple studies (e.g., Becker and Lutz, 2016; Kauffman et al., 2019; Klauberg et al., 2019). Yet, it is contrary to the findings of Kane et al. (2013) who found that low-severity fires significantly modified the canopy structure of coniferous forests in comparison with unburned sites in Yosemite National Park, United States. The major difference between the two studies is that we examined the effects of a single fire, whereas they analyzed the combined effects of fires over 2 decades. As Kane et al. (2013) noted, the unburned reference sites that they selected outside fire perimeters may not fully represent the pre-fire conditions of burned forests, which can contribute to the differences in post-fire tree structures. Additionally, the tree species composition, topography, and the classification of burn severities can also contribute to the different interpretations of burn severity effects on tree structures.
Principally, low- and moderate-severity fires burn more surface than crown fuels, and may remove small-diameter trees (Keeley, 2009; Kane et al., 2013). Consequently, the average post-fire DBH and tree height increase due to the larger residual trees. In our study, 2 years post-fire, we did not find changes in DBH but detected a decreasing trend in tree height following moderate-severity fires, consistent with several studies (Kane et al., 2014; Hoffman et al., 2018; Karna et al., 2019). This suggests that tall trees can also be affected by moderate-severity fires. Possible explanations include that, during the stem exclusion phase, trees invested in vertical growth to compete for growing space and resources (Agee and Huff, 1987), yet their bark may not be thick enough to tolerate moderate-severity fires. As a result, in places with aggregated ladder fuels, shorter trees may help fires spread into the canopy, causing the adjacent taller trees to be charred, snapped off, or killed. With moderate burn severity, fires could remove thin-bark trees, which might lead to greater dominance of thick-bark trees (e.g., Douglas-fir) in post-fire forests.
In contrast, following high-severity fires, the study sites were characterized by abundant standing dead trees (i.e., snags) that were completely or near-completely charred. These trees had significantly smaller DBH, height, and biomass after the fire, implying that high-severity fires might have substantially altered their structures, similar to findings from multiple studies that were conducted across different types of forests (Kane et al., 2014; Karna et al., 2019; Kauffman et al., 2019; Karna et al., 2020). The decreased DBH after high-severity fires was likely due to the removal of large-diameter trees, and the damage to roots and boles causing hydraulic dysfunction of xylem (i.e., the inability of water transport) in the remaining trees (Midgley et al., 2011; Bär et al., 2019).
The interactions among vegetation, weather, and topography influence the burn-severity pattern of wildfires (Kane et al., 2013; Foster et al., 2017; Walker et al., 2020). Research has demonstrated that pre-fire vegetation has a vital impact on burn-severity patterns and post-fire vegetation resembles its pre-fire condition (Kane et al., 2014; Viedma et al., 2020a; Walker et al., 2020). Yet, we also acknowledge that both weather and topography could have critical impacts on burn-severity patterns.
Across the nine sites in this study, trees from mature stands (age class: > 50 years) experienced relatively lower levels of crown scorch compared to trees of similar sizes in young stands (age class: 15—50 years). This is broadly consistent with previous work (e.g., Lydersen et al., 2016; Alonzo et al., 2017; Stephens and York, 2017; Bowd et al., 2021). At the plot level, our results of EFA also imply that burn severities were lower in stands dominated by mature trees with low densities and large horizontal crown structures. In contrast, stands that tended to burn more severely were those occupied by regenerating trees with high densities of vertical crown fuels. This finding implies that the differences in fuel abundance and configuration could play a critical role in driving the burn-severity patterns across the study sites.
In general, a primary factor that likely contributed to the different burn-severity patterns between mature and young stands is related to pre-fire tree size and fuel density. In the six mature stands, trees had larger pre-fire sizes and were less densely distributed compared to those in the three young stands. For large-diameter trees, their thick bark can resist heat during the fire, which increases their survival following the fire (Agee, 1993). In addition, as we observed, trees from the six mature stands had experienced stem exclusion and reached a relatively closed canopy. High canopy closure blocks sunlight from directly infiltrating the understory, and limits the growth of sub-canopy trees and ladder fuels (Hoffman et al., 2018; Karna et al., 2019), which did not favor the vertical or horizontal spread of high-severity crown fires. Yet, during the understory re-initiation phase of closed-canopy stands or in open-canopy stands, the increasing abundance of understory vegetation and surface fuels could make them more susceptible to moderate-severity fires due to torching or passive crown fires.
Among the three young stands, we noticed that they were characterized by trees of smaller sizes and higher densities. As these stands also had a low canopy closure, surface fuels might be exposed to sunlight and wind, decreasing their moisture content and increasing their flammability (Lyons-Tinsley and Peterson, 2012). Meanwhile, short tree heights and the closeness of adjacent tree crowns enhanced the fuel continuity vertically and horizontally, allowing fires to spread into and across the canopy. As a result, these trees- and stand-level characteristics could support the incidence and propagation of fires with increasing burn severities.
Overall, the structural attributes derived from individual tree QSMs were effective in quantifying burn-severity effects and patterns across the study sites. Our findings may inform site-specific management decisions regarding fuel load monitoring and treatment. Yet, we acknowledge that a major limitation of this research is related to the measurements of pre-fire forest conditions. Ideally, bi-temporal laser scanning data are required to compare forest structures pre- and post-fire. Since wildfires have legacy effects on coniferous forests that persist over decades (Bolton et al., 2015), multi-temporal laser scanning data are needed to monitor the long-term change in forest structures. To our knowledge, however, only a few studies have obtained bi-temporal or multi-temporal laser scanning data when investigating wildfire impacts (e.g., Alonzo et al., 2017; McCarley et al., 2017; Hu et al., 2019; Karna et al., 2020). This is mainly because of the operational challenge in acquiring high-quality data before wildfires (Viedma et al., 2020a), as they tend to be unpredictable in space and time.
In this research, we relied on field measurements to examine the pre-fire tree and stand structures. Both fixed- and variable-radius sampling strategies were employed due to different site characteristics. As a result, inconsistencies existed between variable-radius plots in the field and the inclusion of the relevant laser scanning data. Although we accounted for this during data processing, it still influenced the comparison between pre- and post-fire tree attributes to some extent. Since variable-radius sampling can be efficient in the field, more research should aim to develop methods that can reduce errors in matching the shape and size of these plots with laser scanning data. To compare pre- and post-fire biomass, we used species-specific allometric equations. As these equations were based on unburned trees, they may not reflect the actual change in biomass due to wildfires. Hence, new allometric equations should be developed in the future to compute post-fire biomass using field measurements or to transform QSM-derived volumes into biomass.
Another limitation is that we were not able to differentiate tree species or their mortality using laser scanning data alone. To consider this, we used pre- and post-fire field data to broadly evaluate the impacts of wildfires on recorded tree species and their mortality status. Since changes in tree species composition and mortality can be crucial to understanding forest recovery and fire-caused secondary succession (Kane et al., 2013; Hood et al., 2018; Steady et al., 2019), we recommend that future studies should combine DLS/MLS data with high-resolution optical images from satellites or drones to distinguish fire effects or other tree mortality drivers (e.g., infestation, drought) on different species. This would, therefore, allow foresters to identify species-specific plans in terms of forest management and restoration.
In this study, we inferred the wildfire burn-severity patterns using post-fire tree attributes. As several studies pointed out, the role of vegetation on burn-severity patterns could be overwhelmed if fires propagated under extreme weather conditions (Schoennagel et al., 2004; Viedma et al., 2020b). Therefore, for future research, detailed weather information during fire propagation should be integrated to investigate the interactions among weather, vegetation, and burn-severity patterns. In addition, as we mainly focused on trees that represent ladder and crown fuels, the role of surface fuels was not fully considered. Research has shown that the abundance and moisture in surface fuels may determine fire behavior and burn severities (Lyons-Tinsley and Peterson, 2012). With highly dense TLS/MLS data, we suggest that future work also extract point clouds of forest floor and understory vegetation to examine the effects of surface fuels on driving burn-severity patterns.
Post-fire tree structures reflect the impacts of wildfires on forest ecosystems. This research compared pre-fire field measurements with QSM-derived post-fire tree attributes to assess the burn severity of the 2017 Elephant Hill wildfire. At the individual tree level, we found significant and negative correlations between crown scorch and pre-fire DBH, height, and biomass, suggesting that smaller pre-fire trees tend to experience higher levels of crown scorch than larger pre-fire trees. Meanwhile, we also noticed that trees at mature stands (age class: > 50 years) experienced relatively lower levels of crown scorch than trees with similar pre-fire sizes at young stands (age class: 15—50 years). Among pre-fire small- and medium-diameter trees, we found that many post-fire volumetric and crown attributes were significantly and negatively correlated with crown scorch, indicating that trees following high crown scorch could have smaller volumes and crowns compared to the ones that were slightly scorched or unburned. In contrast, among pre-fire large-diameter trees, no post-fire attribute exhibited significant correlations with crown scorch, indicating that they can be more resistant to fires. At the plot level, the effects of low-severity fires were probably masked by the subsequent growth of trees since we did not detect structural changes in DBH, height or biomass. At moderate-severity sites, we observed a major reduction in tree height, implying that moderate-severity fires could consume tall trees with thin bark in places where aggregated ladder fuels existed. At high-severity sites, we found a large number of completely charred trees with smaller DBH, height, and biomass compared to their pre-fire condition. Further, our results suggest that stands with trees of low densities and large crown sizes tend to burn less severely than stands with trees of high crown fuel density and continuity.
In this study, the QSMs generated from the fused DLS-MLS point clouds enabled us to effectively quantify post-fire tree structures, which promotes our understanding of burn severities at a fine scale. The findings of this research support foresters and scientists to develop site-specific plans for fuel treatments. Moving forward, we suggest that future work improve the biomass estimation of burned trees to better evaluate the changes in post-fire carbon dynamics. In addition to DLS/MLS data, high-resolution optical images can also be used to assist with the analyses of fire effects on different tree species. Detailed weather data during the fire event should be incorporated to examine the interacting effects of weather and vegetation on controlling burn-severity patterns. Moreover, the role of surface fuels in moderating burn severities needs to be further investigated using the highly-dense point clouds.
The post-fire data presented in this research are available upon appropriate request from the corresponding author. Inquiries regarding the pre-fire forest inventory data from provincial monitoring programs can be directed to the Forest Productivity and Sample Data Team Lead (https://www2.gov.bc.ca/gov/content/industry/forestry/managing-our-forest-resources/forest-inventory/ground-sample-inventories/provincial-monitoring).
YQ: conceptualization, methodology, analysis, software, visualization, writing. NC: conceptualization, funding acquisition, methodology, supervision, writing. LD: conceptualization, methodology, supervision, writing. CB: conceptualization, methodology, supervision, writing.
This study received funding from the British Columbia Ministry of Forests (2019FAIB501) and the Natural Sciences and Engineering Research Council of Canada (NSERC Discovery Grant).
We thank the FYBR Solutions Inc., Lukas Jarron, Jeremy Arkin, and Felix Poulin for the acquisition of laser scanning data. We acknowledge the support from the Forest Inventory in the Forest Analysis and Inventory Branch for conducting fieldwork. We also thank Arial Eatherton and Spencer Bronson for collecting field data, as well as Katlyn Wise for developing CBI calculations and the burn severity classification. We appreciate the support from Integrated Remote Sensing Studio and the Tree Ring Lab.
The authors declare that the research was conducted in the absence of any commercial or financial relationships that could be construed as a potential conflict of interest.
All claims expressed in this article are solely those of the authors and do not necessarily represent those of their affiliated organizations, or those of the publisher, the editors and the reviewers. Any product that may be evaluated in this article, or claim that may be made by its manufacturer, is not guaranteed or endorsed by the publisher.
The Supplementary Material for this article can be found online at: https://www.frontiersin.org/articles/10.3389/fenvs.2022.949442/full#supplementary-material
Agee, J. K., and Huff, M. H. (1987). Fuel succession in a Western hemlock/Douglas-fir forest. Can. J. For. Res. 17, 697–704. doi:10.1139/x87-112
Ager, A. A., Vaillant, N. M., and McMahan, A. (2013). Restoration of fire in managed forests: A model to prioritize landscapes and analyze tradeoffs. Ecosphere 4, 1–19. doi:10.1890/ES13-00007.1
Åkerblom, M., Raumonen, P., Mäkipää, R., and Kaasalainen, M. (2017). Automatic tree species recognition with quantitative structure models. Remote Sens. Environ. 191, 1–12. doi:10.1016/J.RSE.2016.12.002
Akhtar-Danesh, N. (2017). A comparison between major factor extraction and factor rotation techniques in Q-methodology. Open J. Appl. Sci. 7, 147–156. doi:10.4236/OJAPPS.2017.74013
Alonso, M., Rozados, M. J., Vega, J. A., Pérez-Gorostiaga, P., Cuiñas, P., Fontúrbel, M. T., et al. (2002). Biochemical responses of Pinus pinaster trees to fire-induced trunk girdling and crown scorch: Secondary metabolites and pigments as needle chemical indicators. J. Chem. Ecol. 28 (4), 687–700. doi:10.1023/A:1015276423880
Alonzo, M., Morton, D. C., Cook, B. D., Andersen, H. E., Babcock, C., and Pattison, R. (2017). Patterns of canopy and surface layer consumption in a boreal forest fire from repeat airborne lidar. Environ. Res. Lett. 12, 065004. doi:10.1088/1748-9326/AA6ADE
Arkin, J., Coops, N. C., Daniels, L. D., and Plowright, A. (2021). Estimation of vertical fuel layers in tree crowns using high density lidar data. Remote Sens. (Basel). 13, 4598. doi:10.3390/RS13224598
Bär, A., Michaletz, S. T., and Mayr, S. (2019). Fire effects on tree physiology. New Phytol. 223, 1728–1741. doi:10.1111/NPH.15871
Bartels, S. F., Chen, H. Y. H., Wulder, M. A., and White, J. C. (2016). Trends in post-disturbance recovery rates of Canada’s forests following wildfire and harvest. For. Ecol. Manage. 361, 194–207. doi:10.1016/J.FORECO.2015.11.015
Bartlett, M. S. (1950). Tests of significance in factor analysis. Br. J. Stat. Psychol. 3, 77–85. doi:10.1111/j.2044-8317.1950.tb00285.x
Bassett, M., Leonard, S. W. J., Chia, E. K., Clarke, M. F., and Bennett, A. F. (2017). Interacting effects of fire severity, time since fire and topography on vegetation structure after wildfire. For. Ecol. Manage. 396, 26–34. doi:10.1016/J.FORECO.2017.04.006
BC Wildfire Services (2022). Wildfires of note. Available at: http://bcfireinfo.for.gov.bc.ca/hprScripts/WildfireNews/OneFire.asp?ID=620 (Accessed March 12, 2022).
Becker, K. M. L., and Lutz, J. A. (2016). Can low-severity fire reverse compositional change in montane forests of the Sierra Nevada, California, USA? Ecosphere 7, 1–22. doi:10.1002/ECS2.1484
Besl, P. J., and McKay, N. D. (1992). A method for registration of 3-D shapes. IEEE Trans. Pattern Anal. Mach. Intell. 14, 239–256. doi:10.1109/34.121791
Bolton, D. K., Coops, N. C., and Wulder, M. A. (2015). Characterizing residual structure and forest recovery following high-severity fire in the Western boreal of Canada using Landsat time-series and airborne lidar data. Remote Sens. Environ. 163, 48–60. doi:10.1016/J.RSE.2015.03.004
Bosse, M., Zlot, R., and Flick, P. (2012). Zebedee: Design of a spring-mounted 3-D range sensor with application to mobile mapping. IEEE Trans. Robot. 28, 1104–1119. doi:10.1109/TRO.2012.2200990
Botequim, B., Fernandes, P. M., Borges, J. G., González-Ferreiro, E., and Guerra-Hernández, J. (2019). Improving silvicultural practices for Mediterranean forests through fire behaviour modelling using LiDAR-derived canopy fuel characteristics. Int. J. Wildland Fire 28, 823–839. doi:10.1071/WF19001
Bowd, E. J., McBurney, L., and Lindenmayer, D. B. (2021). Temporal patterns of vegetation recovery after wildfire in two obligate seeder ash forests. For. Ecol. Manage. 496, 119409. doi:10.1016/J.FORECO.2021.119409
Brede, B., Calders, K., Lau, A., Raumonen, P., Bartholomeus, H. M., Herold, M., et al. (2019). Non-destructive tree volume estimation through quantitative structure modelling: Comparing UAV laser scanning with terrestrial LIDAR. Remote Sens. Environ. 233, 111355. doi:10.1016/J.RSE.2019.111355
Bruggisser, M., Hollaus, M., Kükenbrink, D., and Pfeifer, N. (2019). Comparison of forest structure metrics derived from UAV lidar and ALS data. ISPRS Ann. Photogramm. Remote Sens. Spat. Inf. Sci. 4, 325–332. doi:10.5194/ISPRS-ANNALS-IV-2-W5-325-2019
Cannon, J. B., Warnick, K. J., Elliott, S., and Briggs, J. S. (2021). Low- and moderate-severity fire offers key insights for landscape restoration in ponderosa pine forests. Ecol. Appl. 32, e2490. doi:10.1002/EAP.2490
Carlson, A. R., Sibold, J. S., Assal, T. J., and Negrón, J. F. (2017). Evidence of compounded disturbance effects on vegetation recovery following high-severity wildfire and spruce beetle outbreak. PLoS One 12, e0181778. doi:10.1371/JOURNAL.PONE.0181778
Casas, Á., García, M., Siegel, R. B., Koltunov, A., Ramírez, C., and Ustin, S. (2016). Burned forest characterization at single-tree level with airborne laser scanning for assessing wildlife habitat. Remote Sens. Environ. 175, 231–241. doi:10.1016/J.RSE.2015.12.044
Cascio, W. E. (2018). Wildland fire smoke and human health. Sci. Total Environ. 624, 586–595. doi:10.1016/J.SCITOTENV.2017.12.086
Chambers, M. E., Fornwalt, P. J., Malone, S. L., and Battaglia, M. A. (2016). Patterns of conifer regeneration following high severity wildfire in ponderosa pine – dominated forests of the Colorado Front Range. For. Ecol. Manage. 378, 57–67. doi:10.1016/J.FORECO.2016.07.001
Chen, Y., Zhu, X., Yebra, M., Harris, S., and Tapper, N. (2016). Strata-based forest fuel classification for wild fire hazard assessment using terrestrial LiDAR. J. Appl. Remote Sens. 10, 046025. doi:10.1117/1.JRS.10.046025
Churchill, D. J., Jeronimo, S. M. A., Hessburg, P. F., Cansler, C. A., Povak, N. A., Kane, V. R., et al. (2022). Post-fire landscape evaluations in Eastern Washington, USA: Assessing the work of contemporary wildfires. For. Ecol. Manage. 504, 119796. doi:10.1016/J.FORECO.2021.119796
Chuvieco, E. (2009). Earth observation of wildland fires in mediterranean ecosystems. Berlin, Heidelberg: Springer. doi:10.1007/978-3-642-01754-4
CloudCompare (2015). CloudCompare version 2.6.1 user manual. Available at: http://www.cloudcompare.org/doc/qCC/CloudCompare v2.6.1 - User manual.pdf (Accessed March 15, 2022).
Collins, B. M., Lydersen, J. M., Everett, R. G., and Stephens, S. L. (2018). How does forest recovery following moderate-severity fire influence effects of subsequent wildfire in mixed-conifer forests? Fire Ecol. 14, 3–9. doi:10.1186/s42408-018-0004-x
Computree (2021). The computree platform. Available at: http://computree.onf.fr/?page_id=31 (Accessed November 18, 2021).
Coops, N. C., Hermosilla, T., Wulder, M. A., White, J. C., and Bolton, D. K. (2018). A thirty year, fine-scale, characterization of area burned in Canadian forests shows evidence of regionally increasing trends in the last decade. PLoS One 13, e0197218. doi:10.1371/JOURNAL.PONE.0197218
Crockett, J. L., and Westerling, A. L. (2018). Greater temperature and precipitation extremes intensify Western U.S. droughts, wildfire severity, and sierra Nevada tree mortality. J. Clim. 31, 341–354. doi:10.1175/JCLI-D-17-0254.1
Davis, K. T., Dobrowski, S. Z., Higuera, P. E., Holden, Z. A., Veblen, T. T., Rother, M. T., et al. (2019). Wildfires and climate change push low-elevation forests across a critical climate threshold for tree regeneration. Proc. Natl. Acad. Sci. U. S. A. 116, 6193–6198. doi:10.1073/pnas.1815107116
De Santis, A., and Chuvieco, E. (2009). GeoCBI: A modified version of the composite burn index for the initial assessment of the short-term burn severity from remotely sensed data. Remote Sens. Environ. 113, 554–562. doi:10.1016/J.RSE.2008.10.011
Dijkstra, E. W. (1959). A note on two problems in connexion with graphs. Numer. Math. 1, 269–271. doi:10.1007/BF01386390
Flannigan, M., Cantin, A. S., De Groot, W. J., Wotton, M., Newbery, A., and Gowman, L. M. (2013). Global wildland fire season severity in the 21st century. For. Ecol. Manage. 294, 54–61. doi:10.1016/J.FORECO.2012.10.022
Foster, C. N., Barton, P. S., Robinson, N. M., MacGregor, C. I., and Lindenmayer, D. B. (2017). Effects of a large wildfire on vegetation structure in a variable fire mosaic. Ecol. Appl. 27, 2369–2381. doi:10.1002/eap.1614
Garcia, M., Saatchi, S., Casas, A., Koltunov, A., Ustin, S., Ramirez, C., et al. (2017). Quantifying biomass consumption and carbon release from the California Rim fire by integrating airborne LiDAR and Landsat OLI data. J. Geophys. Res. Biogeosci. 122, 340–353. doi:10.1002/2015JG003315
Gauthier, T. D. (2001). Detecting trends using Spearman’s rank correlation coefficient. Environ. Forensics 2, 359–362. doi:10.1006/ENFO.2001.0061
Gelabert, P. J., Montealegre, A. L., Lamelas, M. T., and Domingo, D. (2020). Forest structural diversity characterization in Mediterranean landscapes affected by fires using Airborne Laser Scanning data. GIsci. Remote Sens. 57, 497–509. doi:10.1080/15481603.2020.1738060
Gonzalez de Tanago, J., Lau, A., Bartholomeus, H., Herold, M., Avitabile, V., Raumonen, P., et al. (2018). Estimation of above-ground biomass of large tropical trees with terrestrial LiDAR. Methods Ecol. Evol. 9, 223–234. doi:10.1111/2041-210X.12904
Goodwin, N. R., Coops, N. C., and Culvenor, D. S. (2006). Assessment of forest structure with airborne LiDAR and the effects of platform altitude. Remote Sens. Environ. 103, 140–152. doi:10.1016/j.rse.2006.03.003
Government of BC (2022). Provincial monitoring. Available at: https://www2.gov.bc.ca/gov/content/industry/forestry/managing-our-forest-resources/forest-inventory/ground-sample-inventories/provincial-monitoring (Accessed March 11, 2022).
Government of BC (2021). Timber cruising manual - province of British Columbia. Available at: https://www2.gov.bc.ca/gov/content/industry/forestry/competitive-forest-industry/timber-pricing/timber-cruising/timber-cruising-manual (Accessed July 05, 2022).2020
Hackenberg, J., Morhart, C., Sheppard, J., Spiecker, H., and Disney, M. (2014). Highly accurate tree models derived from terrestrial laser scan data: A method description. Forests 5, 1069–1105. doi:10.3390/f5051069
Hackenberg, J., Spiecker, H., Calders, K., Disney, M., Raumonen, P., Hyyppä, J., et al. (2015). SimpleTree-an efficient open source tool to build tree models from TLS clouds. Forests 6, 4245–4294. doi:10.3390/f6114245
Hanan, E. J., Ren, J., Tague, C. L., Kolden, C. A., Abatzoglou, J. T., Bart, R. R., et al. (2021). How climate change and fire exclusion drive wildfire regimes at actionable scales. Environ. Res. Lett. 16, 024051. doi:10.1088/1748-9326/abd78e
Hillman, S., Wallace, L., Reinke, K., and Jones, S. (2021). A comparison between TLS and UAS LiDAR to represent eucalypt crown fuel characteristics. ISPRS J. Photogramm. Remote Sens. 181, 295–307. doi:10.1016/j.isprsjprs.2021.09.008
Hoffman, K. M., Trant, A. J., Nijland, W., and Starzomski, B. M. (2018). Ecological legacies of fire detected using plot-level measurements and LiDAR in an old growth coastal temperate rainforest. For. Ecol. Manage. 424, 11–20. doi:10.1016/j.foreco.2018.04.020
Hood, S. M., Varner, J. M., Van Mantgem, P., and Cansler, C. A. (2018). Fire and tree death: Understanding and improving modeling of fire-induced tree mortality. Environ. Res. Lett. 13, 113004. doi:10.1088/1748-9326/aae934
Hu, T., Ma, Q., Su, Y., Battles, J. J., Collins, B. M., Stephens, S. L., et al. (2019). A simple and integrated approach for fire severity assessment using bi-temporal airborne LiDAR data. Int. J. Appl. Earth Obs. Geoinf. 78, 25–38. doi:10.1016/j.jag.2019.01.007
Jean, S. A., Pinno, B. D., and Nielsen, S. E. (2019). Trembling aspen root suckering and stump sprouting response to above ground disturbance on a reclaimed boreal oil sands site in Alberta, Canada. New50, 771–784. doi:10.1007/s11056-018-09698-2
Jolly, W. M., Cochrane, M. A., Freeborn, P. H., Holden, Z. A., Brown, T. J., Williamson, G. J., et al. (2015). Climate-induced variations in global wildfire danger from 1979 to 2013. Nat. Commun. 6, 7537. doi:10.1038/ncomms8537
Jones, G. M., Gutiérrez, R. J., Tempel, D. J., Whitmore, S. A., Berigan, W. J., and Peery, M. Z. (2016). Megafires: An emerging threat to old-forest species. Front. Ecol. Environ. 14, 300–306. doi:10.1002/fee.1298
Kaiser, H. F. (1970). A second generation little jiffy. Psychometrika 35, 401–415. doi:10.1007/BF02291817
Kane, V. R., Bartl-Geller, B. N., North, M. P., Kane, J. T., Lydersen, J. M., Jeronimo, S. M. A., et al. (2019). First-entry wildfires can create opening and tree clump patterns characteristic of resilient forests. For. Ecol. Manage. 454, 117659. doi:10.1016/j.foreco.2019.117659
Kane, V. R., Lutz, J. A., Roberts, S. L., Smith, D. F., McGaughey, R. J., Povak, N. A., et al. (2013). Landscape-scale effects of fire severity on mixed-conifer and red fir forest structure in Yosemite National Park. For. Ecol. Manage. 287, 17–31. doi:10.1016/j.foreco.2012.08.044
Kane, V. R., North, M. P., Lutz, J. A., Churchill, D. J., Roberts, S. L., Smith, D. F., et al. (2014). Assessing fire effects on forest spatial structure using a fusion of Landsat and airborne LiDAR data in Yosemite national park. Remote Sens. Environ. 151, 89–101. doi:10.1016/j.rse.2013.07.041
Karna, Y. K., Penman, T. D., Aponte, C., and Bennett, L. T. (2019). Assessing legacy effects of wildfires on the crown structure of fire-tolerant eucalypt trees using airborne LiDAR data. Remote Sens. (Basel). 11, 2433. doi:10.3390/rs11202433
Karna, Y. K., Penman, T. D., Aponte, C., Hinko-Najera, N., and Bennett, L. T. (2020). Persistent changes in the horizontal and vertical canopy structure of fire-tolerant forests after severe fire as quantified using multi-temporal airborne lidar data. For. Ecol. Manage. 472, 118255. doi:10.1016/j.foreco.2020.118255
Kauffman, J. B., Ellsworth, L. M., Bell, D. M., Acker, S., and Kertis, J. (2019). Forest structure and biomass reflects the variable effects of fire and land use 15 and 29 years following fire in the Western Cascades, Oregon. For. Ecol. Manage. 453, 117570. doi:10.1016/j.foreco.2019.117570
Keeley, J. E. (2009). Fire intensity, fire severity and burn severity: A brief review and suggested usage. Int. J. Wildland Fire 18, 116–126. doi:10.1071/WF07049
Key, C. H., and Benson, N. C. (2006). Landscape Assessment (LA) sampling and analysis methods. Ogden, UT: USDA For. Serv. - Gen. Tech. Rep. RMRS-GTR.
Klauberg, C., Hudak, A. T., Silva, C. A., Lewis, S. A., Robichaud, P. R., and Jain, T. B. (2019). Characterizing fire effects on conifers at tree level from airborne laser scanning and high-resolution, multispectral satellite data. Ecol. Modell. 412, 108820. doi:10.1016/J.ECOLMODEL.2019.108820
Koontz, M. J., North, M. P., Werner, C. M., Fick, S. E., and Latimer, A. M. (2020). Local forest structure variability increases resilience to wildfire in dry Western U.S. coniferous forests. Ecol. Lett. 23, 483–494. doi:10.1111/ele.13447
Kramer, H., Collins, B., Lake, F., Jakubowski, M., Stephens, S., and Kelly, M. (2016). Estimating ladder fuels: A new approach combining field photography with LiDAR. Remote Sens. (Basel). 8, 766. doi:10.3390/rs8090766
Kruskal, W. H., and Wallis, W. A. (1952). Use of ranks in one-criterion variance analysis. J. Am. Stat. Assoc. 47, 583–621. doi:10.1080/01621459.1952.10483441
Kunz, M., Fichtner, A., Härdtle, W., Raumonen, P., Bruelheide, H., and von Oheimb, G. (2019). Neighbour species richness and local structural variability modulate aboveground allocation patterns and crown morphology of individual trees. Ecol. Lett. 22, 2130–2140. doi:10.1111/ele.13400
Liu, Y., Stanturf, J., and Goodrick, S. (2010). Trends in global wildfire potential in a changing climate. For. Ecol. Manage. 259, 685–697. doi:10.1016/j.foreco.2009.09.002
Loudermilk, E. L., O’Brien, J. J., Mitchell, R. J., Cropper, W. P., Hiers, J. K., Grunwald, S., et al. (2012). Linking complex forest fuel structure and fire behaviour at fine scales. Int. J. Wildland Fire 21 (7), 882–893. doi:10.1071/WF10116
Lydersen, J. M., Collins, B. M., Miller, J. D., Fry, D. L., and Stephens, S. L. (2016). Relating fire-caused change in forest structure to remotely sensed estimates of fire severity. fire Ecol. 12, 99–116. doi:10.4996/fireecology.1203099
Lyons-Tinsley, C., and Peterson, D. L. (2012). Surface fuel treatments in young, regenerating stands affect wildfire severity in a mixed conifer forest, eastside Cascade Range, Washington, USA. For. Ecol. Manage. 270, 117–125. doi:10.1016/j.foreco.2011.04.016
Madsen, C., Kunz, M., von Oheimb, G., Hall, J., Sinacore, K., Turner, B. L., et al. (2021). Influence of neighbourhoods on the extent and compactness of tropical tree crowns and root systems. Trees Berl. West. 35, 1673–1686. doi:10.1007/s00468-021-02146-3
Manly, B. F. J. (2005). Multivariate statistical methods: A primer. Boca Raton, Florida: Chapman & Hall/CRC Press.
McCarley, T. R., Kolden, C. A., Vaillant, N. M., Hudak, A. T., Smith, A. M. S., Wing, B. M., et al. (2017). Multi-temporal LiDAR and Landsat quantification of fire-induced changes to forest structure. Remote Sens. Environ. 191, 419–432. doi:10.1016/j.rse.2016.12.022
McGee, T., McFarlane, B., and Tymstra, C. (2015). “Wildfire: A Canadian perspective,” in Wildfire hazards, risks, and disasters (Amsterdam: Elsevier), 35–58. doi:10.1016/B978-0-12-410434-1.00003-8
Meidinger, D., and Pojar, J. (1991). Ecosystems of British Columbia. Spec. Rep. Ser. - Minist. For. Br. Columbia. Victoria: BC Ministry of Forests.
Midgley, J. J., Kruger, L. M., and Skelton, R. (2011). How do fires kill plants? The hydraulic death hypothesis and cape proteaceae “fire-resisters. S. Afr. J. Bot. 77, 381–386. doi:10.1016/j.sajb.2010.10.001
O’Brien, J. J., Hiers, J. K., Mitchell, R. J., Varner, J. M., and Mordecai, K. (2010). Acute physiological stress and mortality following fire in a long-unburned longleaf pine ecosystem. fire Ecol. 6, 1–12. doi:10.4996/fireecology.0602001
Prichard, S. J., Stevens-Rumann, C. S., and Hessburg, P. F. (2017). Tamm Review: Shifting global fire regimes: Lessons from reburns and research needs. For. Ecol. Manage. 396, 217–233. doi:10.1016/j.foreco.2017.03.035
Raumonen, P., Casella, E., Calders, K., Murphy, S., Åkerblom, M., and Kaasalainen, M. (2015). Massive-scale tree modelling from TLS data. ISPRS Ann. Photogramm. Remote Sens. Spat. Inf. Sci. 2, 189–196. doi:10.5194/ISPRSANNALS-II-3-W4-189-2015
Raumonen, P., Kaasalainen, M., Markku, Å., Kaasalainen, S., Kaartinen, H., Vastaranta, M., et al. (2013). Fast automatic precision tree models from terrestrial laser scanner data. Remote Sens. (Basel). 5, 491–520. doi:10.3390/RS5020491
Reilly, M. J., Wimberly, M. C., and Newell, C. L. (2006). Wildfire effects on β-diversity and species turnover in a forested landscape. J. Veg. Sci. 17, 447. doi:10.1658/1100-9233(2006)17[447:weodas]2.0.co;2
Robichaud, P. R., Lewis, S. A., Laes, D. Y. M., Hudak, A. T., Kokaly, R. F., and Zamudio, J. A. (2007). Postfire soil burn severity mapping with hyperspectral image unmixing. Remote Sens. Environ. 108, 467–480. doi:10.1016/j.rse.2006.11.027
Robichaud, P. R., Rhee, H., and Lewis, S. A. (2014). A synthesis of post-fire Burned Area Reports from 1972 to 2009 for Western US Forest Service lands: Trends in wildfire characteristics and post-fire stabilisation treatments and expenditures. Int. J. Wildland Fire 23, 929–944. doi:10.1071/WF13192
Rodman, K. C., Veblen, T. T., Chapman, T. B., Rother, M. T., Wion, A. P., and Redmond, M. D. (2020). Limitations to recovery following wildfire in dry forests of southern Colorado and northern New Mexico, USA. Ecol. Appl. 30, e02001–20. doi:10.1002/eap.2001
RStudio Team (2020). RStudio: Integrated development for R. Available at: https://www.rstudio.com/ (Accessed June 12, 2020).
Ruffault, J., Curt, T., Martin-Stpaul, N. K., Moron, V., and Trigo, R. M. (2018). Extreme wildfire events are linked to global-change-type droughts in the northern Mediterranean. Nat. Hazards Earth Syst. Sci. 18, 847–856. doi:10.5194/nhess-18-847-2018
Ryan, K. C. (1982). “Techniques for assessing fire damage to trees,” in Proceedings of Symposium of Fire–Its Field Effects (Jackson, Wyoming: Intermountain Fire Council and Rocky Mountain Fire Council).
Sammartano, G., and Spanò, A. (2018). Point clouds by SLAM-based mobile mapping systems: Accuracy and geometric content validation in multisensor survey and stand-alone acquisition. Appl. Geomat. 10, 317–339. doi:10.1007/s12518-018-0221-7
Savage, M., Mast, J. N., and Feddema, J. J. (2013). Double whammy: High-severity fire and drought in ponderosa pine forests of the Southwest. Can. J. For. Res. 43, 570–583. doi:10.1139/cjfr-2012-0404
Schoennagel, T., Veblen, T. T., and Romme, W. H. (2004). The interaction of fire, fuels, and climate across Rocky Mountain forests. Bioscience 54, 661–676. doi:10.1641/0006-3568(2004)054[0661:TIOFFA]2.0.CO;2
Steady, W. D., Feltrin, R. P., Johnson, D. M., Sparks, A. M., Kolden, C. A., Talhelm, A. F., et al. (2019). The survival of pinus ponderosa saplings subjected to increasing levels of fire behavior and impacts on post-fire growth. Fire 2, 23–13. doi:10.3390/fire2020023
Steel, Z. L., Fogg, A. M., Burnett, R., Roberts, L. J., and Safford, H. D. (2022). When bigger isn’t better—implications of large high-severity wildfire patches for avian diversity and community composition. Divers. Distrib. 28, 439–453. doi:10.1111/ddi.13281
Stephens, C. W., and York, R. A. (2017). An evaluation of stand age as a factor of mastication efficiency and effectiveness in the central sierra Nevada, California. Northwest Sci. 91, 389–398. doi:10.3955/046.091.0408
Stevens-Rumann, C. S., Kemp, K. B., Higuera, P. E., Harvey, B. J., Rother, M. T., Donato, D. C., et al. (2018). Evidence for declining forest resilience to wildfires under climate change. Ecol. Lett. 21, 243–252. doi:10.1111/ele.12889
Taherdoost, H., Sahibuddin, S., and Jalaliyoon, N. (2014). “Exploratory factor Analysis: Concepts and theory,” in Advances in applied and pure mathematics (Gdansk, Poland: WSEAS), 375–382.
Ung, C. H., Bernier, P., and Guo, X. J. (2008). Canadian national biomass equations: New parameter estimates that include British Columbia data. Can. J. For. Res. 38, 1123–1132. doi:10.1139/X07-224
Vandendaele, B., Fournier, R. A., Vepakomma, U., Pelletier, G., Lejeune, P., and Martin-ducup, O. (2021). Estimation of northern hardwood forest inventory attributes using uav laser scanning (Uls): Transferability of laser scanning methods and comparison of automated approaches at the tree- and stand-level. Remote Sens. (Basel) 13, 2796–2827. doi:10.3390/RS13142796
Varner, J. M., Hood, S. M., Aubrey, D. P., Yedinak, K., Hiers, J. K., Jolly, W. M., et al. (2021). Tree crown injury from wildland fires: Causes, measurement and ecological and physiological consequences. New Phytol. 231, 1676–1685. doi:10.1111/nph.17539
Viedma, O., Almeida, D. R. A., and Moreno, J. M. (2020a). Postfire tree structure from high-resolution LiDAR and RBR sentinel 2A fire severity metrics in a Pinus halepensis-dominated burned stand. Remote Sens. (Basel). 12, 3554. doi:10.3390/rs12213554
Viedma, O., Chico, F., Fernández, J. J., Madrigal, C., Safford, H. D., and Moreno, J. M. (2020b). Disentangling the role of prefire vegetation vs. burning conditions on fire severity in a large forest fire in SE Spain. Remote Sens. Environ. 247, 1–17. doi:10.1016/j.rse.2020.111891
Walker, X. J., Baltzer, J. L., Cumming, S. G., Day, N. J., Ebert, C., Goetz, S., et al. (2019). Increasing wildfires threaten historic carbon sink of boreal forest soils. Nature 572, 520–523. doi:10.1038/s41586-019-1474-y
Walker, X. J., Rogers, B. M., Veraverbeke, S., Johnstone, J. F., Baltzer, J. L., Barrett, K., et al. (2020). Fuel availability not fire weather controls boreal wildfire severity and carbon emissions. Nat. Clim. Chang. 10, 1130–1136. doi:10.1038/s41558-020-00920-8
Wallin, K. F., Kolb, T. E., Skov, K. R., and Wagner, M. R. (2003). Effects of crown scorch on ponderosa pine resistance to bark beetles in northern Arizona. Environ. Entomol. 32, 652–661. doi:10.1603/0046-225X-32.3.652
Westerling, A. L. R. (2016). Increasing Western US forest wildfire activity: Sensitivity to changes in the timing of spring. Phil. Trans. R. Soc. B 371, 20150178. doi:10.1098/rstb.2015.0178
Wieder, R. K., Scott, K. D., Kamminga, K., Vile, M. A., Vitt, D. H., Bone, T., et al. (2009). Postfire carbon balance in boreal bogs of Alberta, Canada. Glob. Chang. Biol. 15, 63–81. doi:10.1111/j.1365-2486.2008.01756.x
Wilcoxon, F. (1992). “Individual comparisons by ranking methods,” in Breakthroughs in statistics (New York, NY: Springer), 196–202. doi:10.1007/978-1-4612-4380-9_16
Woodward, B. D., Romme, W. H., and Evangelista, P. H. (2020). Early postfire response of a northern range margin coast redwood forest community. For. Ecol. Manage. 462, 117966. doi:10.1016/j.foreco.2020.117966
Wulder, M. A., Bater, C. W., Coops, N. C., Hilker, T., and White, J. C. (2008). The role of LiDAR in sustainable forest management. For. Chron. 84, 807–826. doi:10.5558/TFC84807-6
Keywords: wildfire, burn severity, tree structure, lidar point clouds, drone, SLAM, quantitative structure model
Citation: Qi Y, Coops NC, Daniels LD and Butson CR (2022) Assessing the effects of burn severity on post-fire tree structures using the fused drone and mobile laser scanning point clouds. Front. Environ. Sci. 10:949442. doi: 10.3389/fenvs.2022.949442
Received: 20 May 2022; Accepted: 22 July 2022;
Published: 31 August 2022.
Edited by:
Olga Viedma Sillero, University of Castilla La Mancha, SpainReviewed by:
Elizabeth (Liz) Tasker, NSW Government, AustraliaCopyright © 2022 Qi, Coops, Daniels and Butson. This is an open-access article distributed under the terms of the Creative Commons Attribution License (CC BY). The use, distribution or reproduction in other forums is permitted, provided the original author(s) and the copyright owner(s) are credited and that the original publication in this journal is cited, in accordance with accepted academic practice. No use, distribution or reproduction is permitted which does not comply with these terms.
*Correspondence: Yangqian Qi, eWFuZ3FpYW4ucWlAYWx1bW5pLnViYy5jYQ==
Disclaimer: All claims expressed in this article are solely those of the authors and do not necessarily represent those of their affiliated organizations, or those of the publisher, the editors and the reviewers. Any product that may be evaluated in this article or claim that may be made by its manufacturer is not guaranteed or endorsed by the publisher.
Research integrity at Frontiers
Learn more about the work of our research integrity team to safeguard the quality of each article we publish.