- 1School of International Trade and Economics, University of International Business and Economics, Beijing, China
- 2UIBE Belt and Road Energy Trade and Development Center, University of International Business and Economics, Beijing, China
- 3University of International Business and Economics, Beijing, China
The original intention of green finance advocacy is to provide financing support for energy conservation and emission-reduction activities. In this context, the carbon dioxide (CO2) emission-reduction effect is worth further discussion. To this end, by gauging the green finance index, we apply the econometric method to evaluate the impact of green finance on CO2 emissions. We also discuss geographical heterogeneity and the impact mechanism. The main findings imply that: 1) China’s implementation of green finance is an effective measure to mitigate greenhouse gas emissions; in other words, green finance in China can effectively reduce CO2 emissions; 2) both green finance and CO2 emissions show significant geographical heterogeneity and asymmetry; only in the eastern and central regions, can green finance help alleviate the greenhouse effect; and 3) besides the total effect, green finance can affect the greenhouse effect by promoting the rapid growth of the provincial economy, restraining the improvement of energy efficiency, and accelerating the optimization of the current industrial structure. Following the above three findings, we propose some policy suggestions related to green finance evolution and CO2 emissions reduction.
Highlights
➢ We assess the impact of China’s green finance on CO2 emissions.
➢ Both geographic heterogeneity and CO2 emission asymmetry are explored.
➢ This study evaluates how green finance affects CO2 emissions.
➢ Green finance is an effective strategy for solving the greenhouse effect.
➢ Green finance in China reduces CO2 mainly by promoting industrial optimization.
1 Introduction
With the rise of global temperatures, the frequent occurrence of natural disasters, and global environmental pollution, the issue of climate change has increasingly attracted the attention of scholars around the world (Hulme et al., 2018; Wang et al., 2021a; Zhao et al., 2022a; Ren et al., 2022c; Yan et al., 2022). If left unchecked, carbon dioxide (CO2) emissions will cause an additional 1–3.7°C increase in global average temperatures by the end of this century, leading to irreversible changes (Dusenge et al., 2019; IPCC, 2022). Accordingly, the United Nations has held several climate change conferences aimed at cooperating with countries around the world to alleviate the problems caused by climate change and CO2 emissions. For example, at the COP 26 UN Climate Change Conference, governments committed to phasing out coal-fired power generation, providing a credible guarantee for mitigating global CO2 emissions (Arora and Mishra, 2021). As the world’s largest emission producer, China is also actively seeking ways to reduce CO2 emissions. China’s 14th Five-Year Plan points out that by 2025, energy intensity should be reduced by 13.5%, and non-fossil energy should account for one fifth of primary energy consumption by 2030 (He, 2015; Pan and Dong, 2022), so as to gradually achieve China’s worldwide commitment to a zero-carbon target by 2060 (Zhao et al., 2022b).
In order to achieve climate goals, the world needs to transition to a greener, more climate-resilient economy (Ren et al., 2022a), in which green finance will accelerate the construction of greener infrastructure and innovation. In 2012, the China Banking Regulatory Commission released the “Green Credit Guidelines,” which established the core framework of the green credit system, and more and more funds are used in terms of low-carbon environmental protection (Yu et al., 2021). In 2015, China became the first country to establish a sound green financial policy system around the world (Lv et al., 2021). The scale of China’s green finance market continues to expand (Ren et al., 2022b). In 2018, China issued more than 280 billion yuan of green bonds, and the stock of green bonds is worth close to 600 billion yuan, ranking among the top in the world (PBC, 2019). Therefore, green finance has become the main way for China to deal with climate change and reduce the greenhouse effect. On the one hand, green finance provides financial services for green innovation industries and offers more advanced environmental-protection technologies (Khan et al., 2021). On the other hand, green finance can help mitigate the greenhouse effect by underwriting environmental regulations and environmental directives (Nouira et al., 2016). Statistics published by the International Energy Agency (IEA, 2017) show that green finance will reduce fossil fuel combustion by 26%. However, large-scale inefficient investments caused by green finance should also be taken into account, with the negative effects of climate change (Nawaz et al., 2021). Figure 1 also presents the average levels of green finance and CO2 emissions in China, both of which show an increasing trend. Therefore, we urgently need to clarify the role of green finance in addressing the problem of climate change and mitigating CO2 emissions.
At the same time, there are significant differences in CO2 emission levels across provinces in China, as shown in Figure 2, which has led to different carbon-mitigation policies and the different influences of green finance. Although a few scholars have (explored the nexus between green finance and CO2 emissions Saeed Meo and Karim, 2022; Xiong and Sun, 2022), there is still insufficient research on the heterogeneity among Chinese provinces. Furthermore, the path through which green finance affects CO2 emissions is still unclear. Therefore, we put propose to address the following questions: 1) Does green finance help mitigate CO2 emissions in China? 2) Is green finance affecting CO2 emissions heterogeneously? 3) What is the impact mechanism between green finance and CO2 emissions? Based on the panel data of 30 provinces in China from 2004 to 2018, we construct a comprehensive indicator of green finance and assess the impact of green finance on CO2 emissions in China. We also explore their heterogeneous and mediating effects.
Accordingly, this paper makes the following research contributions. First, we explore the relationship between China’s green finance development and CO2 emissions, which clarifies the direction of China’s green finance development and will make the government more confident in investing in green industries. Second, we conduct heterogeneity analyses by geographic position and CO2 emissions level, which provide more valuable references for regional governments. Third, we innovatively study the mediating effect between green finance and CO2 emissions, which can help governments formulate clearer emission-reduction paths.
The remainder of this paper is organized as follows. The next section is the literature review; Section 3 represents the econometric methodology and data; Section 4 discusses the nexus between green finance and CO2 emissions; in Section 5, we analyze the heterogeneity and mediating effect, followed by the conclusion and policy implications in Section 6.
2 Literature Review
2.1 An Overview of Green Finance
In 2010, in an attempt to provide financial support for developing countries dealing with climate change, 194 countries established the Green Fund, which is a prototype of the concept of “green finance” (Zhang et al., 2019). Generally speaking, green finance refers to a financial activity that promotes environmental development, improves resource utilization, and responds to climate change (Cui et al., 2020). The green financial market will use various green products as trading platforms, including mainly green credit, green funds, and green derivatives (Taghizadeh-Hesary and Yoshino, 2019). With the severe situation of global climate change in the international community, various governments and scholars have focused on the role of green finance, and carried out large-scale research on this subject.
Constructing and evaluating financial risk indicators accurately are the first concerns of scholars. Some scholars use a single indicator, such as green bonds, as a proxy variable for green finance (Saeed Meo and Karim, 2022). However, a completely green financial system should not only contain a single indicator, but should also be a comprehensive indicator that includes economic reform, economic transformation, and the combination of environmental benefits (Lv et al., 2021). Therefore, Wang et al. (2021b) use the improved fuzzy comprehensive evaluation method combined with the relevant statistical indicators of China’s green credit to evaluate the development level of China’s green finance. Yang et al. (2021) measure green finance by integrating green credit, green investment, green insurance, green securities, and carbon finance.
Based on the establishment of comprehensive indicators, many scholars have conducted in-depth explorations on the influence of green finance on the global economy and environment (Wang and Zhi, 2016; Li and Gan, 2021; Zhang et al., 2021; Dong et al., 2022). For example, Zhou et al. (2020) point out that green finance can significantly balance the relationship between economic development and environmental quality, and achieve a win-win situation between economic development and the environment. Some scholars have also explored the nexus between green finance and energy security (Sachs et al., 2019a; Sachs et al., 2019b), green finance and non-fossil energy use (Ren et al., 2020), and green finance and energy efficiency (Jin et al., 2021). All of the above studies have shown that green finance supports green and sustainable development and contributes to the progress of the energy system towards a cleaner, more efficient trajectory.
2.2 Studies on the Green Finance-CO2 Emissions Nexus
Green finance aims to finance green industries, help improve environmental quality, and promote technological innovation in green industries (La Rovere et al., 2018). Therefore, it is not difficult to guess that green finance will have a significant impact on CO2 emissions. In fact, many scholars have proved the above hypothesis. Globally, Saeed Meo and Karim (2022) study the relationship between green finance and greenhouse gases in major economies that support green finance. Their results show that green finance negatively affects CO2 emissions. Moreover, from the perspective of a specific country, the nexus between green finance and CO2 emissions is also a popular subject of research (Xiong and Sun, 2022). For example, Tran (2021) uses multivariate time series analysis to investigate the relationship between green finance and CO2 emissions in Vietnam. Their results show that there is a one-way causal negative relationship between green investment and CO2 emissions. In addition, some scholars have explored the sensitivity of CO2 emissions in a certain industry to green finance. For example, Guo et al. (2022) measure the relationship between green finance and agricultural CO2 emissions. Their results show that green finance can negatively affect agricultural CO2 emissions. Gholipour et al. (2022) explore the relationship between green property finance and CO2 emissions in the construction industry. Their results indicate an obvious negative correlation between the above two factors.
2.3 Literature Gaps
The discussion on green finance has gradually matured in the international community, and research on the green finance-CO2 emissions nexus has recently emerged. However, we still find knowledge gaps in the existing literature: First, there is no unified standard for measuring green finance, which leads to significant differences in the conclusions of relevant researches on green finance. Second, scholars rarely discuss the nexus between green finance and CO2 emissions in China. Third, the influence mechanism of green finance on CO2 emissions is still unclear, and most scholars have failed to show a clearer path for mechanism analysis.
3 Empirical Model and Data Sources
3.1 Empirical Model
To quantitatively interpret the CO2 emission reduction effect of green finance development in China, we build an econometric model in the following framework:
where i denotes Chinese 30 provinces, and t refers to the time (2004–2018). CO2 means the total amount of CO2 emissions, and GFI indicates green finance. Pgdp, EE, ISU, Tra, Edu, Wage, and Gap represent economic growth, energy efficiency, industrial structure upgrading, trade openness, education level, wage level, and income inequality, respectively.
To effectively address the problems of dimensional inconsistency of the variables and data fluctuation, we apply the natural logarithm of Eq. 1, as follows:
where
To address the impact mechanism between green finance and CO2 emissions, based on the conventional and commonly used theory of three effects of CO2 emissions proposed by Copeland and Taylor (1994), we choose economic effect, technical effect, and structural effect as mediating variables to further explore whether green finance influences CO2 emissions by affecting regional economy, technology, and industrial structure. The specific equations of the mediation effect model are constructed as follows:
where the parameters in front of the variables are estimated coefficients. M in these equations denotes economic effect, technical effect, and structural effect, respectively, which are gauged by economic growth, energy efficiency, and industrial structure upgrading, respectively. In these equations,
Furthermore, to investigate the asymmetric effect between green finance and CO2 emissions, we construct the quantile regression model following the estimation framework of Coad and Rao (2006) and Kang et al. (2021):
where the dependent variable
3.2 Variables Setting
3.2.1 Explained Variable
CO2 emissions (denoted as CO2). China’s official statistics bureau or yearbook currently does not directly publish research data on CO2 emissions. Generally speaking, most scholars use data on CO2 emissions caused by fossil energy consumption, which can more accurately assess the greenhouse effect caused by CO2 emissions (Wang et al., 2021a; Wang et al., 2021b).
3.2.2 Explanatory Variables
Since China put forward the concept of green finance in 2016, many scholars have launched a series of discussions and measures on green finance; however, there is no unified standard for gauging green finance at present. Thus, to effectively assess green finance in China, we refer to the indictor system of Jiang et al. (2020), which consists of three dimensions (i.e., economy, finance, and environment); the specific second-level indicators are listed in Supplementary Appendix Table S1 in the Supplementary Appendix S1. By using the improved entropy method, we gauge the provincial composite index of green finance for the period 2004–2018; the specific calculation steps can refer to the work of Zhao et al. (2021).
On this basis, we further conduct a spatio-temporal analysis on China’s green finance. In Figure 1, we display the time trend chart of average values of green finance from 2004 to 2018; obviously, before 2011, green finance showed a substantial upward trend and reached its peak in 2011. Thereafter, it gradually declined and then rose slowly between 2016 and 2018. In addition, Figure 3 presents the spatial pattern of China’s green finance in 2004, 2009, 2014, and 2018. These charts imply that green finance shows a wide range of heterogeneous distribution. More specifically, the phenomenon of green finance in the Beijing-Tianjin-Hebei region, the Yangtze River Delta, Pearl River Delta, and inland western provinces is obviously better than that in the central region. A possible reason is that the relatively prosperous coastal provinces such as Beijing-Tianjin-Hebei, the Yangtze River Delta, and the Pearl River Delta usually have a sound and complete financial system and relatively frequent financial investment activities. The inner western provinces have a favorable ecological environment despite their relatively backward financial development.
3.2.3 Control Variables
In addition to CO2 emissions and green finance, we also introduce some control variables which influence the CO2 emissions. Referring to Bano et al. (2018), Wang and Zhang (2021), and Zhao et al. (2021), we introduce economic growth, energy efficiency, industrial structure upgrading, trade openness, education level, wage level, and income inequality as the determinants of CO2 emissions.
3.2.4 Mediator Variables
We select three mediator variables, namely economic effect, technical effect, and structural effect. Among them, referring to Zhang et al. (2020), we use the per capita GDP as the proxy variable of economic effect. Referring to Wang et al. (2022), we use the energy efficiency as the proxy variable of technical effect. And referring to Luan et al. (2021), we use the ratio of output value of tertiary industry to GDP as the proxy variable of structural effect. And their definition and units are listed in Table 1.
3.3 Data
The explained variable (CO2 emissions) is obtained from the China Emission Accounts and Datasets (CEADs, 2019). The key explanatory variable (green finance) is calculated by three dimensions—economy, finance, and environment—and the relevant data have been collected from the China Statistical Yearbook (CSY, 2021), the China Regional Financial Operation Report, and the China Environment Statistical Yearbook. Furthermore, CSY (2021) and the China Energy Statistical Yearbook provide the data on the control variables and mediator variables. The specific measures and descriptive statistics of variables used are presented in Table 1.
4 Empirical Findings
4.1 Correlation Check
Before examining the estimated results between green finance and CO2 emissions, we first check the correlations of all the variables, and present their results in Table 2. Except for lnTra, the correlation tests between the other variables and the dependent variable (lnCO2) are all significant at the 5% significance level, which indicates that the variables selected in this paper are reliable. Moreover, lnISU and lnGap are negatively correlated with the dependent variables, which indicates that industrial upgrading and income inequality may negatively affect CO2 emissions, but more accurate results require further estimation. In addition, most of the correlation test results between the variables are less than 0.8, which indicates that there is no serious multicollinearity issue between the variables.
4.2 Benchmark Estimates
Next, we examine the correlation between green finance and CO2 emissions, and show the results in Table 3. We apply the feasible generalized least squares (FGLS) method to estimate. FGLS generates estimates that are dependent on the disturbance covariance matrix estimations as well as any estimated autocorrelation parameters (Gulnaz and Manglani, 2022). When the exact form of data heteroskedasticity is known, FGLS is the most suitable model, which is resistant to any type of heteroscedasticity. Also, we set three tests for the FGLS model—the Wald test, the Wooldridge test, and the CD test. Their results show that the null hypotheses are all rejected at the 1% level, indicating that the panel data have groupwise heteroskedasticity, first-order autocorrelation, and cross-sectional dependence (Shahzad et al., 2018). Therefore, the FGLS method can solve the above problems and obtain unbiased and consistent estimators.
The results of the FGLS method show that the coefficient of green finance is always negative, regardless of whether control variables are added to the model. In other words, green finance negatively affects CO2 emissions in China. Guo et al. (2022) and Saeed Meo and Karim (2022) also draw the same conclusion by examining the agricultural sector and the top ten economies. Green finance focuses on investment in green-related industries and encourages companies to develop innovative technologies that are conducive to low-carbon energy, such as renewable energy technologies (Saeed Meo and Karim, 2022). China has basically established an overall green financial framework such as green bonds, green insurance, green credit, and so on (Guo et al., 2022). This framework will guide consumers and enterprises to establish the concept of green consumption and reduce the generation of carbon footprint by supporting domestic green-friendly projects (Yang et al., 2021). At the same time, local governments have accelerated the construction of the green financial industry, including establishing green financial reform and innovation pilot zones, accelerating the green transformation of local economies, and providing subsidies for the transformation of high-polluting enterprises, thereby reducing fossil energy consumption and CO2 emissions (PBC, 2019).
As for the control variables, economic growth effectively promotes CO2 emissions, which can be attributed to industrial expansion and energy consumption brought about by economic growth. Energy efficiency significantly reduces CO2 emissions, a fact confirmed in Liu et al. (2019) and He et al. (2021). This is because improved energy efficiency represents advanced technology, which reduces the energy consumption per unit of economic output, thus contributing to the reduction of CO2 emissions. Industrial structure upgrading has also significantly reduced CO2 emissions. By guiding the upgrading of strategic emerging industries, China has shifted the country’s industrial focus from secondary industry to tertiary industry. The expansion of service industries and high-tech industries has effectively alleviated the large consumption of energy and reduced CO2 emissions (Wu et al., 2021). Finally, education level and wage level are significant; the former positively promotes CO2 emissions, while the latter negatively affects CO2 emissions.
4.3 Robustness Tests
4.3.1 Alternative Estimated Method
In the case of small sample sizes, an econometric model may produce inconsistent estimates; and due to the high correlation among macroeconomic variables, independent variables are correlated with random error terms. Selecting appropriate instrumental variables (IV) can solve the above issues. In general, the IV needs to satisfy the assumption that it should correlate highly with endogenous dependent variables and not correlate with random error terms. Lewbel (2012) constructs IV using heteroscedasticity, and the estimated results are represented in Table 3. The test of Hansen J rejects the null hypothesis, and the IV selected in this method is suitable. The coefficient of green finance is significantly negative, and the benchmark result is robust.
4.3.2 Alternative Explained Variables
Further, we replace the dependent variables with sulfur dioxide (SO2) emissions and chemical oxygen demand (COD) emissions. Similar to CO2 emissions, these two pollutants are also the targets of key emission reductions in China (Liu and Wang, 2017); their estimated results are listed in Table 4. Green finance negatively affects SO2 and COD emissions, which is consistent with the results of the benchmark regression. This further confirms the robustness of the benchmark regression results.
5 Further Discussion
5.1 Heterogeneous Analysis
5.1.1 Geographic Heterogeneity
In the last section, we conducted the benchmark regression on the CO2 emission reduction effect of China’s green finance. Notably, the spatial patterns of China’s CO2 emissions and green finance imply significant geographic heterogeneity across different provinces. To this end, in this section, we proceed to investigate the regional heterogeneous effects of green finance on CO2 emissions by dividing the whole sample into three subsamples—the eastern, central, and western regions. The specific provinces of these three regions are listed in Supplementary Appendix Table S2.
Also using the FGLS technique, we evaluate the CO2 emission effect of green finance in these three regions, and present the corresponding results in Table 5. More specifically, in the eastern region, each 1% increase in green finance will result in a 0.619% reduction in CO2 emissions. This finding verifies the effective role of green finance popularization in the eastern coastal provinces of China in alleviating the greenhouse effect. The superior geographical location and convenient transportation of our eastern provinces have contributed to the rapid economic growth and basic perfection of financial institutions in this region. In response to the governments’ call for environmental protection and the achievement of the provinces’ own green and sustainable development, financial institutions have begun to invest their capital in low-carbon technological innovation projects, which is conducive to alleviating the deteriorating ecological environment.
Consistent with the eastern region, the evolution of green finance and CO2 emissions also shows a significant negative correlation. Table 5 shows that an increase of green finance by 1% can reduce CO2 emissions by 0.574%. The CO2 emission-reduction effect of green finance in the eastern region is significantly better than that in the central region. On the contrary, the widespread advocacy of green finance policies in the western region is not a powerful weapon for mitigating greenhouse gas emissions. The results in Table 5 illustrate that a 1% increase of green finance can increase CO2 emissions by 0.205%. As we all know, the rugged geographical location and harsh climate in the western provinces limit population agglomeration and economic evolution, and the scattered pollution and backward economic system hinder the extensive distribution of financial institutions and the continuous perfection of the financial system, which is not conducive to alleviating the greenhouse effect.
5.1.2 Heterogeneity of CO2 Emissions
In addition to geographical heterogeneity, we further examine the differential causal linkage between green finance and the greenhouse effect under different quantiles of CO2 emissions. The corresponding results are listed in Table 6. Furthermore, this study displays the variation characteristics of green finance and control variables under different quantiles (Figure 4).
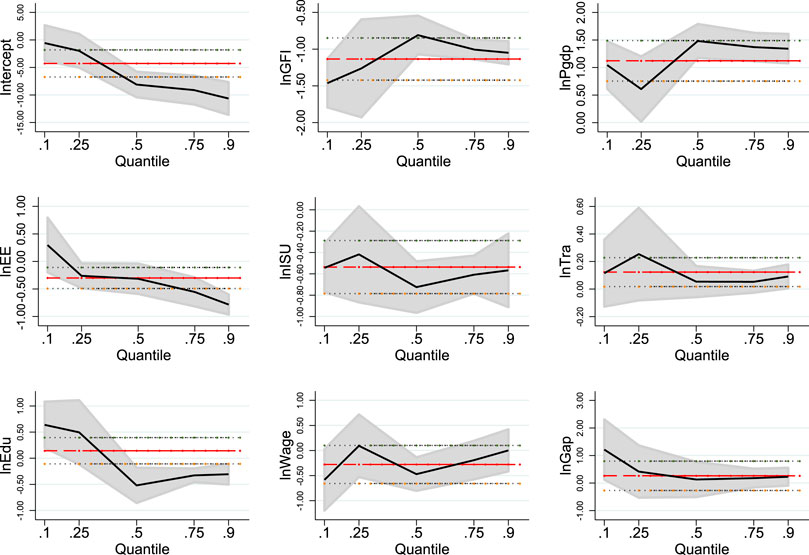
FIGURE 4. Change in panel quantile regression coefficients. Notes: The x-axis represents the conditional quantiles of CO2 emissions and y-axis denotes the coefficient values of various variables. The red line indicates the coefficient values of panel data model with fixed effect.
As this table shows, although under different quantiles, green finance contributes to the mitigation of greenhouse gas emissions, the magnitude of its impact varies. At the 10th quantile of CO2 emissions, green finance plays the largest role in promoting carbon reduction, followed by the effect in the 25th quantile. This suggests that the carbon reduction effect of green finance is particularly pronounced in regions with relatively low CO2 emissions. Furthermore, at the 90th and 75th quantiles, we find that the coefficients of green finance are −1.050 and −1.007, respectively, while at the 50th quantile, an increase of green finance by 1% can reduce CO2 emissions by 0.809%. The above analysis emphasizes that both green finance and CO2 emissions at the 10th, 25th, 50th, 75th, and 90th quantiles exhibit a U-shaped feature. Actively guiding the green investment of financial institutions is an effective measure to manage the increasingly deteriorating ecological environment.
5.2 Mediating Analysis
In Section 4, we perform a systematic analysis on the specific and heterogeneous impacts of green finance on China’s greenhouse effect; thus, an interesting question ignites our consideration—through what channels does green finance reduce CO2 emissions?
By employing the Sobel test and bootstrap sampling method simultaneously, we estimate the above three equations, and present the estimated results in Table 7. In terms of the economic effect, the value of the Sobel test is 0.153, which is significant at the 1% level. This test underscores the effective mediating role of economic growth. Moreover, from the first three columns of Table 7, the coefficients of green finance from column (1) to column (3) are −1.225, 0.095, and −1.377. This implies that while the development of green finance policies in China directly hinders the intensification of the greenhouse effect, it will increase greenhouse gas emissions by facilitating economic growth, and the proportion of total effect that is mediated by economic growth is 12.5%. Green finance policies, while promoting the transfer of investment to environmentally friendly enterprises, also facilitate the corresponding facility construction and productivity improvement, thus boosting economic growth; however, economic growth is highly dependent on fossil fuel burning, thus exacerbating the greenhouse effect. Furthermore, in the bootstrap test, _bs_1 and _bs_2 refer to the indirect and direct effects, respectively. And the confidence intervals do not contain 0, which further verifies the reliability of the mediation effect of economic growth on the green finance-CO2 emissions nexus.
Regarding the technical effect, the value of the Sobel test is 0.157, and is significant at the 1% level; this finding confirms the effectiveness of energy efficiency in affecting the green finance-CO2 emissions nexus. Specifically, the coefficients of green finance in column (4) and energy efficiency in column (5) are −0.335 and −0.467, respectively. This suggests that the development of green finance cannot enhance energy efficiency and thus reduce greenhouse gas emissions, and the proportion of total effect that is mediated is 12.8%, which is contrary to the findings of Rasoulinezhad and Taghizadeh-Hesary (2022) and Yu et al. (2022). This may be because the implementation of green finance aims to apply economic leverage to guide capital to gradually withdraw from high-energy-consuming enterprises and integrate into energy-saving projects, so as to facilitate the low-carbon allocation of financial resources. However, the ambiguity of credit standards among financial institutions in the early stage of implementation of the policy leads to the phenomenon of “bad money driving out good money” in unfair competition, resulting in an unreasonable layout of green finance and producing the opposite effect on energy efficiency. In addition, a check of the bootstrap test confirms the mediating role of energy efficiency.
The Sobel test of the structural effect implies the validity of the mediating role of industrial structure upgrading. To be specific, the coefficients of green finance in column (6) and industrial structure upgrading in column (7) are 0.553 and −0.997, respectively, which suggests that green finance can effectively facilitate the optimization and upgrading of the existing industrial structure, thereby alleviating the greenhouse effect. Moreover, the proportion of total effect that is mediated is 45%. This ratio suggests that industrial structure upgrading has the most obvious mediation effect between green finance and CO2 emissions. In summary, we can conclude that China’s green finance not only directly mitigates CO2 emissions, but also affects the greenhouse effect by promoting economic growth, restraining energy efficiency improvement, and facilitating industrial structure upgrading. We also draw a mechanism diagram to illustrate the above relationships (Figure 5).
6 Conclusion and Policy Implications
6.1 Conclusion
Based on China’s provincial panel dataset from 2004 to 2018, this paper estimates the impact of green finance on CO2 emissions using the FGLS method, and further explores their heterogeneity and mediating effect. Accordingly, we have drawn the following main conclusion:
1) China’s green finance negatively affects CO2 emissions, which indicates that China’s green finance investment is conducive to the country’s carbon mitigation process. Moreover, the benchmark regression results pass the robustness test of the alternative estimation method and dependent variables.
2) The results of the heterogeneous analysis suggest that green finance has a heterogeneity impact on China’s CO2 emissions. Specifically, green finance negatively affects CO2 emissions in eastern and central China; in addition, green finance has a stronger negative impact on CO2 emissions in regions with a lower level of emissions.
3) The estimated results of the Sobel test and bootstrap test indicate that China’s green finance not only helps mitigate the greenhouse effect directly, but also can affect CO2 emissions by promoting economic growth, inhibiting energy efficiency improvement, and accelerating the optimization and transfer of the current industrial structure.
6.2 Policy Implications
Based on the above three findings, we propose the following policy implications. First, given the negative effect of green finance on the greenhouse effect, it is imperative to strengthen the development of green finance. At present, China’s green finance is still in an exploratory stage, so promoting the sustainable and stable development of green finance is crucial. To be more specific, first, local governments should pay attention to comprehensive and specific planning for the overall development of green finance, and establish and improve legal guarantees for green finance. Enterprises that pay attention to environmental protection will be provided with preferential credit or tax policies, and capital will be guided into green financial investment through cooperation between the government and private capital. Second, in addition to government control and guidance, relevant departments should strengthen the supervision of green bonds and improve enterprises’ social responsibility in the field of green finance. In addition, environmental protection departments can use advanced technologies such as cloud computing or big data to disclose corporate environmental information and promote timely access and the effective transmission of relevant information by financial institutions. Credit investigation departments should establish an enterprise credit investigation system, and financial institutions should provide timely feedback on green finance-related information to environmental protection departments. Third, comprehensive financial human capital is key to promoting the effective implementation and stable development of green finance. Thus, China should focus on training financial talents, accelerate the transfer and upgrading of intermediate business models, establish scientific talent-training plans, and help employees in the financial industry to carry out financial knowledge training in colleges and universities.
Second, the empirical results of geographic heterogeneity and CO2 emissions heterogeneity show that provincial governments should introduce or formulate policies and regulations related to green finance or CO2 emission reduction according to actual local conditions. To be more specific, in the prosperous eastern coastal provinces, especially in specific economic agglomeration areas such as the Beijing-Tianjin-Hebei area, the Yangtze River Delta, and the Pearl River Delta, financial institutions and the policy system are relatively sound. On the basis of the continuous promotion of green finance, the eastern provinces should focus on the agglomeration of technology, human capital, material capital, and public resources, establish financial centers, and give play to their spatial diffusion and radiation functions to drive the rapid evolution of financial institutions in the central and western regions. Provinces in the central region should capitalize on the advantages of the regional economy, actively implement green finance policies, and fully tap the supporting role of green finance in reducing CO2 emissions. In the economically backward western provinces, the excellent ecological environment in this area should be used to actively exploit the potential role of green finance to overcome obstacles. On the basis of drawing on the advanced experience of green finance in the eastern and central regions, the green credit process and a scheme suitable for local development are being formulated in combination with the actual features of the local region, and the rapid evolution of green finance in the western region will be actively promoted.
Third, the mediation effects of economic, technical, and structural effects imply that the industrial structure optimization effect of green finance has been significantly verified, but its promotion effect on energy efficiency has not been fully explored. Thus, it is vital to further guide and strengthen green finance for investment in tertiary industries with high added value and low pollution. Furthermore, local government should comprehensively determine credit standards to avoid deviations caused by vague and inconsistent investment standards. In addition, financial institutions should strengthen financial investment in enterprises actively engaged in the research and development of green, low-carbon technologies, and provide financial support for environmental protection projects.
Data Availability Statement
The raw data supporting the conclusion of this article will be made available by the authors, without undue reservation.
Author Contributions
JW: validation, visualization, writing—original draft, investigation, resources, and data curation. YM: conceptualization, methodology, software, writing—review and editing. All authors contributed to the article and approved the submitted version.
Conflict of Interest
The authors declare that the research was conducted in the absence of any commercial or financial relationships that could be construed as a potential conflict of interest.
Publisher’s Note
All claims expressed in this article are solely those of the authors and do not necessarily represent those of their affiliated organizations, or those of the publisher, the editors and the reviewers. Any product that may be evaluated in this article, or claim that may be made by its manufacturer, is not guaranteed or endorsed by the publisher.
Supplementary Material
The Supplementary Material for this article can be found online at: https://www.frontiersin.org/articles/10.3389/fenvs.2022.931086/full#supplementary-material
References
Arora, N. K., and Mishra, I. (2021). COP26: More Challenges Than Achievements. Environ. Sustain. 4, 585–588. doi:10.1007/s42398-021-00212-7
Bano, S., Zhao, Y., Ahmad, A., Wang, S., and Liu, Y. (2018). Identifying the Impacts of Human Capital on Carbon Emissions in Pakistan. J. Clean. Prod. 183, 1082–1092. doi:10.1016/j.jclepro.2018.02.008
CEADs (2019). Province CO2 Emissions Inventory 1997-2019. Avaialable at: https://www.ceads.net/data/province/by_apparent_accounting/.
Coad, A., and Rao, R. (2006). Innovation and Market Value: a Quantile Regression Analysis. Econ. Bull. 15, 1–10. Avaialable at: http://www.accessecon.com/pubs/EB/2006/Volume15/EB-06O10012A.pdf.
Copeland, B. R., and Taylor, M. S. (1994). North-south Trade and the Environment. Q. J. Econ. 109 (3), 755–787. doi:10.2307/2118421
CSY (2021). National Bureau of Statistics, China Statistical Yearbook 2021. Avaialable at: http://www.stats.gov.cn/tjsj/ndsj/.
Cui, H., Wang, R., and Wang, H. (2020). An Evolutionary Analysis of Green Finance Sustainability Based on Multi-Agent Game. J. Clean. Prod. 269, 121799. doi:10.1016/j.jclepro.2020.121799
Dong, K., Taghizadeh-Hesary, F., and Zhao, J. (2022). How Inclusive Financial Development Eradicates Energy Poverty in China? the Role of Technological Innovation. Energy Econ. 109, 106007. doi:10.1016/j.eneco.2022.106007
Dusenge, M. E., Duarte, A. G., and Way, D. A. (2019). Plant Carbon Metabolism and Climate Change: Elevated CO2 and Temperature Impacts on Photosynthesis, Photorespiration and Respiration. New Phytol. 221, 32–49. doi:10.1111/nph.15283
Gholipour, H. F., Arjomandi, A., and Yam, S. (2022). Green Property Finance and CO2 Emissions in the Building Industry. Glob. Finance J. 51, 100696. doi:10.1016/j.gfj.2021.100696
Gulnaz, S., and Manglani, H. (2022). Does Gravity Work in the Context of India and ASEAN Bilateral Trade? an Application of the FGLS Method. Theor. Appl. Econ. 29 (1), 143–160.
Guo, L., Zhao, S., Song, Y., Tang, M., and Li, H. (2022). Green Finance, Chemical Fertilizer Use and Carbon Emissions from Agricultural Production. Agriculture 12, 313. doi:10.3390/agriculture12030313
He, J.-K. (2015). China's INDC and Non-fossil Energy Development. Adv. Clim. Change Res. 6, 210–215. doi:10.1016/j.accre.2015.11.007
He, Y., Fu, F., and Liao, N. (2021). Exploring the Path of Carbon Emissions Reduction in China's Industrial Sector through Energy Efficiency Enhancement Induced by R&D Investment. Energy 225, 120208. doi:10.1016/j.energy.2021.120208
Hulme, M., Obermeister, N., Randalls, S., and Borie, M. (2018). Framing the Challenge of Climate Change in Nature and Science Editorials. Nat. Clim. Change 8, 515–521. doi:10.1038/s41558-018-0174-1
IEA (2017). Data and Statistics. Avaialable at: https://www.iea.org/.
IPCC (2022). in Intergovernmental Panel on Climate Change.Climate Change 2022: Impacts, Adaptation and Vulnerability
Jiang, L., Wang, H., Tong, A., Hu, Z., Duan, H., Zhang, X., et al. (20202020). The Measurement of Green Finance Development Index and its Poverty Reduction Effect: Dynamic Panel Analysis Based on Improved Entropy Method. Discrete Dyn. Nat. Soc. 2020, 1–13. doi:10.1155/2020/8851684
Jin, Y., Gao, X., and Wang, M. (2021). The Financing Efficiency of Listed Energy Conservation and Environmental Protection Firms: Evidence and Implications for Green Finance in China. Energy Policy 153, 112254. doi:10.1016/j.enpol.2021.112254
Kang, Q., Wu, J., Chen, M., and Jeon, B. (2021). Do Macroprudential Policies Affect the Bank Financing of Firms in China? Evidence from a Quantile Regression Approach. J. Int. Money Financ. 115, 102391. doi:10.1016/j.jimonfin.2021.102391
Khan, M. A., Riaz, H., Ahmed, M., and Saeed, A. (2022). Does Green Finance Really Deliver what Is Expected? an Empirical Perspective. Borsa Istanb. Rev. 22, 586–593. doi:10.1016/j.bir.2021.07.006
La Rovere, E. L., Grottera, C., and Wills, W. (2018). Overcoming the Financial Barrier to a Low Emission Development Strategy in Brazil. Int. Econ. 155, 61–68. doi:10.1016/j.inteco.2017.12.004
Lewbel, A. (2012). Using Heteroscedasticity to Identify and Estimate Mismeasured and Endogenous Regressor Models. J. Bus. Econ. Statistics 30, 67–80. doi:10.1080/07350015.2012.643126
Li, C., and Gan, Y. (2021). The Spatial Spillover Effects of Green Finance on Ecological Environment-Empirical Research Based on Spatial Econometric Model. Environ. Sci. Pollut. Res. 28, 5651–5665. doi:10.1007/s11356-020-10961-3
Liu, Q., and Wang, Q. (2017). How China Achieved its 11th Five-Year Plan Emissions Reduction Target: A Structural Decomposition Analysis of Industrial SO2 and Chemical Oxygen Demand. Sci. Total Environ. 574, 1104–1116. doi:10.1016/j.scitotenv.2016.08.176
Liu, X., Shen, B., Price, L., Hasanbeigi, A., Lu, H., Yu, C., et al. (2019). A Review of International Practices for Energy Efficiency and Carbon Emissions Reduction and Lessons Learned for China. WIREs Energy Environ. 8, e342. doi:10.1002/wene.342
Luan, B., Zou, H., Chen, S., and Huang, J. (2021). The Effect of Industrial Structure Adjustment on China's Energy Intensity: Evidence from Linear and Nonlinear Analysis. Energy 218, 119517. doi:10.1016/j.energy.2020.119517
Lv, C., Bian, B., Lee, C.-C., and He, Z. (2021). Regional Gap and the Trend of Green Finance Development in China. Energy Econ. 102, 105476. doi:10.1016/j.eneco.2021.105476
Nawaz, M. A., Seshadri, U., Kumar, P., Aqdas, R., Patwary, A. K., and Riaz, M. (2021). Nexus between Green Finance and Climate Change Mitigation in N-11 and BRICS Countries: Empirical Estimation through Difference in Differences (DID) Approach. Environ. Sci. Pollut. Res. 28, 6504–6519. doi:10.1007/s11356-020-10920-y
Nouira, I., Hammami, R., Frein, Y., and Temponi, C. (2016). Design of Forward Supply Chains: Impact of a Carbon Emissions-Sensitive Demand. Int. J. Prod. Econ. 173, 80–98. doi:10.1016/j.ijpe.2015.11.002
Pan, Y., and Dong, F. (2022). Design of Energy Use Rights Trading Policy from the Perspective of Energy Vulnerability. Energy Policy 160, 112668. doi:10.1016/j.enpol.2021.112668
PBC (2019). People's Bank of China. Avaialable at: http://www.gov.cn/xinwen/2019-11/20/content_5453843.htm.China Green Finance Development Report 2018
Rasoulinezhad, E., and Taghizadeh-Hesary, F. (2022). Role of Green Finance in Improving Energy Efficiency and Renewable Energy Development. Energy Effic. 15 (2), 1–12. doi:10.1007/s12053-022-10021-4
Ren, X., Dou, Y., Dong, K., and Li, Y. (2022a). Information Spillover and Market Connectedness: Multi-Scale Quantile-On-Quantile Analysis of the Crude Oil and Carbon Markets. Appl. Econ., 1–21. doi:10.1080/00036846.2022.2030855
Ren, X., Li, Y., Yan, C., Wen, F., and Lu, Z. (2022b). The Interrelationship between the Carbon Market and the Green Bonds Market: Evidence from Wavelet Quantile-On-Quantile Method. Technol. Forecast. Soc. Change 179, 121611. doi:10.1016/j.techfore.2022.121611
Ren, X., Shao, Q., and Zhong, R. (2020). Nexus between Green Finance, Non-fossil Energy Use, and Carbon Intensity: Empirical Evidence from China Based on a Vector Error Correction Model. J. Clean. Prod. 277, 122844. doi:10.1016/j.jclepro.2020.122844
Ren, X., Tong, Z., Sun, X., and Yan, C. (2022c). Dynamic Impacts of Energy Consumption on Economic Growth in China: Evidence from a Non-parametric Panel Data Model. Energy Econ. 107, 105855. doi:10.1016/j.eneco.2022.105855
Sachs, J. D., Woo, W. T., Yoshino, N., and Taghizadeh-Hesary, F. (2019b). Importance of Green Finance for Achieving Sustainable Development Goals and Energy Security. Handb. green finance Energy Secur. Sustain. Dev. 10, 1–10. doi:10.1007/978-981-10-8710-3_13-1
Sachs, J., Woo, W. T., Yoshino, N., and Taghizadeh-Hesary, F. (2019a). Handbook of Green Finance: Energy Security and Sustainable Development. Springer.
Saeed Meo, M., and Karim, M. Z. A. (2022). The Role of Green Finance in Reducing CO2 Emissions: An Empirical Analysis. Borsa Istanb. Rev. 22, 169–178. doi:10.1016/j.bir.2021.03.002
Shahzad, F., Rehman, I. U., Nawaz, F., and Nawab, N. (2018). Does Family Control Explain Why Corporate Social Responsibility Affects Investment Efficiency? Corp. Soc. Resp. Env. Ma 25, 880–888. doi:10.1002/csr.1504
Taghizadeh-Hesary, F., and Yoshino, N. (2019). The Way to Induce Private Participation in Green Finance and Investment. Finance Res. Lett. 31, 98–103. doi:10.1016/j.frl.2019.04.016
Tran, Q. H. (2021). The Impact of Green Finance, Economic Growth and Energy Usage on CO2 Emission in Vietnam - a Multivariate Time Series Analysis. Cfri 12, 280–296. doi:10.1108/CFRI-03-2021-0049
Wang, B., Zhao, J., Dong, K., and Jiang, Q. (2022). High-quality Energy Development in China: Comprehensive Assessment and its Impact on CO2 Emissions. Energy Econ. 110, 106027. doi:10.1016/j.eneco.2022.106027
Wang, J., Dong, K., Dong, X., and Jiang, Q. (2021a). Research on the Carbon Emission Effect of the Seven Regions along the Belt and Road-Based on the Spillover and Feedback Effects Model. J. Clean. Prod. 319, 128758. doi:10.1016/j.jclepro.2021.128758
Wang, J., Jiang, Q., Dong, X., and Dong, K. (2021b). Decoupling and Decomposition Analysis of Investments and CO2 Emissions in Information and Communication Technology Sector. Appl. Energy 302, 117618. doi:10.1016/j.apenergy.2021.117618
Wang, Q., and Zhang, F. (2021). The Effects of Trade Openness on Decoupling Carbon Emissions from Economic Growth - Evidence from 182 Countries. J. Clean. Prod. 279, 123838. doi:10.1016/j.jclepro.2020.123838
Wang, X., Wang, X., Ren, X., and Wen, F. (2022c). Can Digital Financial Inclusion Affect CO2 Emissions of China at the Prefecture Level? Evidence from a Spatial Econometric Approach. Energy Econ. 109, 105966. doi:10.1016/j.eneco.2022.105966
Wang, X., Zhao, H., and Bi, K. (2021c). The Measurement of Green Finance Index and the Development Forecast of Green Finance in China. Environ. Ecol. Stat. 28, 263–285. doi:10.1007/s10651-021-00483-7
Wang, Y., and Zhi, Q. (2016). The Role of Green Finance in Environmental Protection: Two Aspects of Market Mechanism and Policies. Energy Procedia 104, 311–316. doi:10.1016/j.egypro.2016.12.053
Wu, L., Sun, L., Qi, P., Ren, X., and Sun, X. (2021). Energy Endowment, Industrial Structure Upgrading, and CO2 Emissions in China: Revisiting Resource Curse in the Context of Carbon Emissions. Resour. Policy 74, 102329. doi:10.1016/j.resourpol.2021.102329
Xiong, Q., and Sun, D. (2022). Influence Analysis of Green Finance Development Impact on Carbon Emissions: an Exploratory Study Based on fsQCA. Environ. Sci. Pollut. Res. doi:10.1007/s11356-021-18351-z
Yan, D., Ren, X., Zhang, W., Li, Y., and Miao, Y. (2022). Exploring the Real Contribution of Socioeconomic Variation to Urban PM2.5 Pollution: New Evidence from Spatial Heteroscedasticity. Sci. Total Environ. 806, 150929. doi:10.1016/j.scitotenv.2021.150929
Yang, Y., Su, X., and Yao, S. (2021). Nexus between Green Finance, Fintech, and High-Quality Economic Development: Empirical Evidence from China. Resour. Policy 74, 102445. doi:10.1016/j.resourpol.2021.102445
Yu, C.-H., Wu, X., Zhang, D., Chen, S., and Zhao, J. (2021). Demand for Green Finance: Resolving Financing Constraints on Green Innovation in China. Energy Policy 153, 112255. doi:10.1016/j.enpol.2021.112255
Yu, M., Zhou, Q., Cheok, M. Y., Kubiczek, J., and Iqbal, N. (2022). Does Green Finance Improve Energy Efficiency? New Evidence from Developing and Developed Economies. Econ. Change Restruct. 55 (1), 485–509. doi:10.1007/s10644-021-09355-3
Zhang, D., Mohsin, M., Rasheed, A. K., Chang, Y., and Taghizadeh-Hesary, F. (2021). Public Spending and Green Economic Growth in BRI Region: Mediating Role of Green Finance. Energy Policy 153, 112256. doi:10.1016/j.enpol.2021.112256
Zhang, D., Zhang, Z., and Managi, S. (2019). A Bibliometric Analysis on Green Finance: Current Status, Development, and Future Directions. Finance Res. Lett. 29, 425–430. doi:10.1016/j.frl.2019.02.003
Zhang, J., Fan, Z., Chen, Y., Gao, J., and Liu, W. (2020). Decomposition and Decoupling Analysis of Carbon Dioxide Emissions from Economic Growth in the Context of China and the ASEAN Countries. Sci. Total Environ. 714, 136649. doi:10.1016/j.scitotenv.2020.136649
Zhao, J., Jiang, Q., Dong, X., Dong, K., and Jiang, H. (2022a). How Does Industrial Structure Adjustment Reduce CO2 Emissions? Spatial and Mediation Effects Analysis for China. Energy Econ. 105, 105704. doi:10.1016/j.eneco.2021.105704
Zhao, J., Shahbaz, M., Dong, X., and Dong, K. (2021). How Does Financial Risk Affect Global CO2 Emissions? the Role of Technological Innovation. Technol. Forecast. Soc. Change 168, 120751. doi:10.1016/j.techfore.2021.120751
Zhao, X., Ma, X., Chen, B., Shang, Y., and Song, M. (2022b). Challenges toward Carbon Neutrality in China: Strategies and Countermeasures. Resour. Conservation Recycl. 176, 105959. doi:10.1016/j.resconrec.2021.105959
Keywords: carbon dioxide (CO2) emissions, green finance, heterogeneous analysis, mediation effect analysis, China
Citation: Wang J and Ma Y (2022) How Does Green Finance Affect CO2 Emissions? Heterogeneous and Mediation Effects Analysis. Front. Environ. Sci. 10:931086. doi: 10.3389/fenvs.2022.931086
Received: 28 April 2022; Accepted: 20 May 2022;
Published: 08 June 2022.
Edited by:
Xiaohang Ren, Central South University, ChinaReviewed by:
Y.P. Zhang, China University of Mining and Technology, ChinaMingshun Zheng, Northwest A&F University, China
Copyright © 2022 Wang and Ma. This is an open-access article distributed under the terms of the Creative Commons Attribution License (CC BY). The use, distribution or reproduction in other forums is permitted, provided the original author(s) and the copyright owner(s) are credited and that the original publication in this journal is cited, in accordance with accepted academic practice. No use, distribution or reproduction is permitted which does not comply with these terms.
*Correspondence: Ying Ma, MTM4MTE3MzQ1ODBAMTYzLmNvbQ==