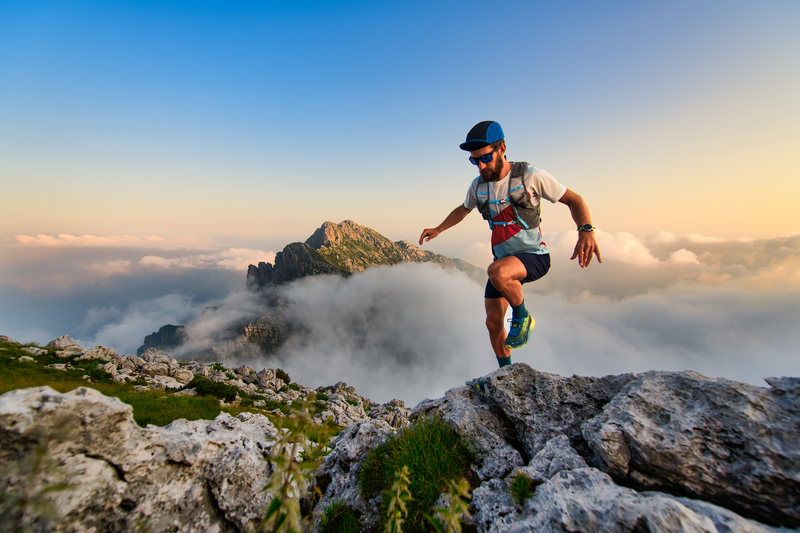
95% of researchers rate our articles as excellent or good
Learn more about the work of our research integrity team to safeguard the quality of each article we publish.
Find out more
ORIGINAL RESEARCH article
Front. Environ. Sci. , 04 July 2022
Sec. Environmental Economics and Management
Volume 10 - 2022 | https://doi.org/10.3389/fenvs.2022.920879
Environmental information disclosure is one of the important ways to realize urban sustainable development. This study explores the transmission mechanism between environmental information disclosure and urban green economic efficiency (UGEE) and solves the “black box” system between them. In this method, first, the urban green economic efficiency is calculated using the data envelopment analysis model with undesirable output. Second, the panel data model and the mediating effects model are employed to test the impact of environmental information disclosure on urban green economic efficiency and the possible mediating variables. The main conclusions of this study are as follows: 1) Environmental information disclosure significantly improves urban green economic efficiency, and reducing industrial pollutant emission and improving technological innovation are the significant transmission mechanisms. (2) There is a U-shaped curve relationship between environmental information disclosure and urban green economic efficiency and environmental information disclosure and technological innovation. On the contrary, there is an inverted U-shaped curve relationship between environmental information disclosure and industrial pollutant emission. 3) Environmental information disclosure has a greater sustainability effect in industrial cities. The conclusion shows that environmental information disclosure can improve the efficiency of urban green economy by improving the ability of urban technological innovation and reducing the emission of industrial pollutants. Environmental information disclosure plays a role of “restraining first and then promoting” to sustainable development. This research provides a reference for how to lessen information asymmetry and reduce negative externalities in the formulation of environmental regulatory policies.
In the past stage, China’s economic growth has achieved remarkable results. According to the data from the China Statistical Yearbook, the total GDP increased from 6,087.1 (unit: billion dollars) in 2010 to 14,722.7 (billion dollars) in 2020, with an average annual growth rate of more than 8.3%. However, the cost of rapid economic growth is the huge consumption of energy and the deterioration of the ecological environment. Since 2010, China has become the largest country in energy consumption. According to the China Energy Development Report 2020, from 2015 to 2020, China’s energy consumption increased from 4.34 to 4.98 (unit: billion tons of standard coal), of which coal and oil still dominate. Achieving sustainable development and coping with global climate change is an important research theme of urban development (Jiang et al., 2022a). The 19th Congress of the Communist Party of China pointed out that China’s economic growth has shifted from a high-speed growth stage to a high-quality development stage. The core of high-quality development is green economic growth, that is, maintaining the balance between economic growth and environmental sustainability (Li et al., 2022). As being the most important administrative unit of economic growth, the city is the carrier of enterprise operation, residents’ life, and economic and social development. In addition to the tertiary industry and service industry, the building industry also plays a non-negligible role in urban carbon neutralization and sustainable development (Sun et al., 2022). The total carbon emission of China’s commercial building industry reached 793 (MtCO2) in 2018, 3.74 times higher than that in 2000 (Xiang et al., 2022a). Therefore, the green total factor productivity with cities as samples is more in line with the needs of realizing the sustainable development of the whole society. Exploring how to achieve urban green economic efficiency under the restriction of environmental protection has important theoretical significance and practical value. The main purpose of this study was to measure the efficiency of urban green economy and analyze its influencing factors.
The existing research has conducted a wealth of research on the methods of measuring the efficiency of green economy. As a nonparametric method, data envelopment analysis (DEA) has greater advantages in dealing with multi-input and multi-output data (Wang et al., 2018b). Chung et al. (1997) incorporated unexpected output into the Malmquist model for the first time and named it as the Malmquist–Luenberger Productivity Index (MLPI). Further, Fare et al. (2010) combined the global frontier method with the MLPI to obtain the Global Malmquist–Luenberger Productivity Index (GMLPI). This method can compare and analyze the productivity of multiple periods; therefore, it is widely used in the productivity analysis of regions and enterprises. For example, Liu et al. (2021), Emrouznejad and Yang (2016), and Gao et al. (2021) measured the green total factor productivity of the transportation industry, the manufacturing industry, and the whole province with 30 provinces in China, respectively. Zhang et al. (2019) measured the green total factor productivity of 220,000 enterprises in China. In addition, Xiang et al. (2022b) applied the Python method to the measurement of input–output system, which greatly expanded the empirical method in this field. However, the existing literature lacks the measurement of total factor green productivity with Chinese cities as samples. This study tries to fill this gap and calculate the GMLPI of 113 cities in China under the constraints of energy and environment.
Among the many influencing factors of urban green economy, emission trading (Jiang et al., 2022b), technological innovation (Du et al., 2021), education (Zafar et al., 2021), infrastructure (Mohmand et al., 2020), industrial upgrading (Shao et al., 2021), and energy market reform (Jiang et al., 2020) have been proved to play an important role. In addition to the above factors, environmental regulation is considered to be the most important means of governance (Liu Y. et al., 2020). The government intervention theory holds that the formal environmental regulation led by the government can significantly promote regional sustainable development by internalizing the negative externalities of pollution, which is the Porter hypothesis (Porter and Linde, 1995). Porter and Linde (1995) hold that strict environmental regulation will induce technological innovation of enterprises and finally achieve a win–win situation of environmental protection and economic development. Zhang et al. (2019) took China as a sample and concluded that environmental regulation is conducive to industrial green growth. Wu et al. (2020) also confirmed the positive effect of environmental regulation on carbon emission reduction. On the contrary, neoclassical economic theory holds that due to the information asymmetry, the government cannot accurately grasp the external cost of each industry or enterprise (Ambec et al., 2013). Environmental regulation policies may cause enterprises to face problems such as additional loss of environmental protection equipment and mismatch between production resources and original equipment, namely “compliance cost,” which leads to the decline in enterprise production efficiency (Hancevic, 2016). Fu et al. (2020) also denied the sustainable effect of environmental regulation on enterprises. The main conflict between the above two views is whether the government can have complete information. Therefore, identifying the information asymmetry between the government and enterprises in pollution will help in the means of environmental regulation to give play to greater economic and social benefits (Jolink and Niesten, 2020). Environmental information disclosure will also promote enterprises to improve their production behavior and promote the efficiency of green economy (Fan et al., 2020). However, the existing literature lacks attention to the problem of information asymmetry in environmental regulation.
The Institute of Public and Environmental Affairs (IPE) and Nature Resources Defense Council (NRDC) have published the Pollution Information Transparency Index (PITI) for 113 cities in China since 2009. It provides new data for exploring the role of information asymmetry in sustainability. The PITI measures the level of information disclosure of urban environment and pollution sources, including daily monitoring of enterprise pollutant emissions, government environmental protection, government affairs disclosure, enterprise violations, and other aspects. Scholars have made many valuable researches using PITI: Tu et al. (2019) put forward that the pollutant emission reduction effect of environmental information disclosure only exists in the eastern region; Feng et al. (2021b) empirically analyzed the impact of the PITI on economic growth and haze pollution; and Zhong et al. (2021) used the spatial Durbin model to analyze the emission reduction effect of the PITI on major industrial pollutants. However, the above researches have the following two defects: Firstly, the impact of EID on sustainable development is regarded as a black box, and there is a lack of discussion on the possible intermediary variable. Secondly, there is a lack of discussion on the possible nonlinear relationship between them. Therefore, this study will make an in-depth analysis of the above defects.
The main innovations of this study are as follows: 1) This study calculates the Global Malmquist–Luenberger Productivity Index of 113 cities in China from 2008 to 2018 for the first time based on the DEA method containing undesirable output. (2) From both theoretical and empirical aspects, this study first analyzes the impact of EID on urban green economic efficiency and its transmission path and uses the mediating effects model to test whether industrial pollution emissions and technological innovation are the possible mediating variables, and it also discusses the possible “U-shaped” or “inverted U-shaped” curve relationship between EID and each of the variables. 3) It conducts heterogeneity analysis of different types of cities, which is helpful for the government to carry out differentiated environmental regulation policies according to urban characteristics. The robustness test and the instrumental variable method show that the conclusion of the research is credible.
The rest of this study is arranged as follows: The second part is the theoretical basis and research hypothesis; the third part introduces the model setting and data sources; the fourth part is the empirical results and further analysis; the fifth part discusses the main conclusions of this research; and the sixth part is the conclusion and policy recommendations.
Environment, as a nonexclusive and compulsory public product with consumption, is facing the dilemma of “more consumers and fewer investors” in economic development (Ajayi et al., 2012). When the means of environmental supervision are missing, the excessive discharge of pollutants forms the negative externality of economic growth (Chen et al., 2015). In order to make up the gap between private cost and social cost, the government usually uses environmental control, allocation of emission rights, and other policies to internalize external costs (Wang and Shen, 2016). However, the policies are constrained by information asymmetry, which makes the policy effect often lower-than-expected (Nie et al., 2021). The root cause of information asymmetry is the low efficiency of updating pollutant emission information of industrial enterprises. The supervision of pollution behavior of industrial enterprises by the government is often quarterly or annual static detection, rather than real-time dynamic monitoring. In addition, the low degree of informatization of environmental supervision and the low degree of public participation make it difficult for environmental policies to achieve the desired results (Elnaboulsi et al., 2018). The main purpose of EID measures is to standardize and promote the environmental protection administrative departments and enterprises to disclose environmental information, ensure the rights of citizens and society to obtain environmental information, and promote public participation and environmental protection. EID will regulate or restrict the behavior of administrative departments and enterprises:
Figure 1 shows the impact of EID on the efficiency of urban green economy and its transmission mechanism. Firstly, EID makes up for the information asymmetry; real-time monitoring of enterprise sewage strengthens the supervision of local governments to enterprises. Under the real-time monitoring of pollutant discharge, the phenomenon of enterprises’ disorderly discharge of pollutants is restricted, and local governments can accurately identify specific pollutant discharge enterprises and then take precise disciplinary measures (Jiang et al., 2021). The supervision of public opinion on enterprises with high pollutant discharge will also force enterprises and competent departments to optimize cleaner production (Qu et al., 2021). At all levels of government, information disclosure promotes the transparency of environmental protection achievements and government affairs and makes up for the shortcomings of “environmental protection supervision” and other systems that cannot continuously supervise local governments. To sum up, EID reduces information asymmetry, strengthens the identification of negative externalities of the environment, and corrects the speculative behavior of government and enterprises, which generally shows the role of promoting sustainable development.
This research puts forward Hypothesis 1: Environmental information disclosure (EID) can promote urban green economic efficiency (UGEE).
However, the above analysis regards the impact process of “EID——UGEE” as black box; it is necessary to discuss its transmission mechanism to provide support for deepening policy implementation. EID will first affect the sewage behavior of the enterprise; transparent sewage information and real-time sewage supervision in the long time may significantly reduce enterprise pollutant emissions (Zhong et al., 2021). The reduction of pollution level will further alleviate haze pollution and ultimately improve the efficiency of urban green economy. In addition, how to reduce pollutant emissions while ensuring economic benefits has become the core of green development of enterprises, and the pursuit of clean production technology and sustainable development technology has also become the common pursuit of enterprises and the government (Xing et al., 2021). Therefore, information disclosure will force enterprises and the government to spend more on scientific and technological innovation, forcing enterprises to adopt more green production technology. Further, technological innovation will promote urban green development and sustainability.
This research puts forward Hypothesis 2: Industrial pollution emission and technological innovation may be the mediating variables of EID affecting UGEE.
Environmental policy shocks may exist diversity in the short and long term. In the early stage of implementation, policies may even show reverse results due to reasons such as guillotine cutting problem and difficult to grasp the scale (Liu J. et al., 2020). This study argues that EID in different periods will also produce heterogeneity, resulting in a nonlinear relationship with UGEE. In the early stage, the low levels of information disclosure may inhibit the green economic growth: On the one hand, information disclosure forces enterprises to reduce pollution by reducing production, which leads to the decline in economic benefits. On the other hand, the government may have some faults at early stage, such as unclear division of responsibilities and inadequate implementation, which lead to the low level of information disclosure and unsatisfactory effect. With the improvement in EID, cleaner production has gradually become a consensus of the government, enterprises, and the public. Moreover, with the improvement in technology innovation, infrastructure construction, and supporting environmental regulation means, the environmental information disclosure gradually forms scale effect and finally shows the promotion effect on sustainable development.
This study puts forward Hypothesis 3: There is a nonlinear relationship between environmental information disclosure and urban green economic efficiency, industrial pollution emissions, and technological innovation.
This study takes 113 cities in China as independent decision-making units (DMU), three variables as input indicators, named as
In Eq. 1,
In Eq. 2,
In order to empirically test whether EID can significantly contribute to UGEE, this study proposes to use mixed OLS regression models, random-effects models, and fixed-effects models for conducting benchmark panel regression models. The basic assumption of the mixed OLS regression model is that there are no individual effects, and based on the research in this study, the OLS model is set as follows:
where GMLPI represents urban green economic efficiency, PITI represents the Environmental Information Disclosure Index, X represents the control variable, i and t represent the individual and period of the sample, respectively, and
Equation 4 represents random-effects and fixed-effects models. The meaning of the characters is the same as in Eq. 1, but the disturbance term is represented by
The above panel data model treats the impact of environmental information disclosure on urban green economic efficiency as a “black box” and lacks a discussion of the transmission mechanisms involved. This study sets up a mediating model as follows:
POLLUTION represents industrial pollution emissions, which is one of the assumed mediating variables in this study. The effect of environmental information disclosure on industrial pollution emissions is measured by
As with the panel data model, the F test, LM test, and Hausman test need to be introduced in the mediating effects model for mixed OLS, random-effects, and fixed-effects model identification.
In addition, in the mediating effects model, it needs to focus on the size of the mediating effect and the weight of the mediating effect in the total effect, which measures the extent to which the mediating variable plays a role in the effect of environmental information disclosure on urban green economic efficiency. According to Mackinnon et al. (1995) and Mackinnon et al. (2002), the mediating effects model is assumed to be
The mediating effect of the mediating variable (M) is ab (either c - c*), and the proportion of the mediating effect in the total effect is ab/(c + ab).
Firstly, this study measured the green economic efficiency of cities using a DEA model that includes undesired outputs to obtain the Global Malmquist–Luenberger Productivity Index. Input and output indicators were selected as follows.
Input indicators: 1) capital—capital represents the sum of all capital that can be invested in the production in the current year, including new fixed and current assets and depreciated fixed assets invested in the previous period. According to Wu et al. (2021), this study uses the perpetual inventory method to calculate the combined assets of each city in the current year; 2) labor force—this study selects the sum of primary, secondary, and tertiary workers in each city in the current year to represent the labor force level; and 3) energy consumption—electricity consumption represents the city’s energy consumption and reflects the level of green economic development of the city under the energy constraint. Drawing on the study by Liu et al. (2019), this study selects the total electricity consumption of each city in the current year to represent the level of energy consumption.
Output indicators: 1) GDP—According to Yang et al. (2021), this study takes the regional GDP of each city for the year to represent the desired output. This indicator measures the economic benefits that can be generated with a certain input of capital, labor, and energy; and 2) PM2.5—PM2.5 concentration measures environmental pollution in cities and reflects the extent of environmental damage caused by economic production in cities (Cheng et al., 2020). The inclusion of PM2.5 concentration as undesirable output in the model indicates the efficiency of a green economy under environmental constraints.
Secondly, this study uses the panel data model and the mediating effects model to empirically test the impact of environmental information disclosure on urban green economic efficiency and the transmission mechanism. The following variables were selected for empirical analysis in this study.
Explained variables: The Global Malmquist–Luenberger Productivity Index (GMLPI) for each city was measured based on the DEA model with undesired output. According to Fare et al. (2010), the GMLPIt, t-1 indicates the combined productivity in period t when the base period is t-1, and since the GMLPI uses the same production frontier for all years, comparisons can be made between multiple years. In this study, the GMLPI is calculated to represent UGEE for each subsequent year using 2008 as the base period. The GMLPI for each year measures the level of urban green economic efficiency compared to 2008.
Core explanatory variables: The Pollution Information Transparency Index (LNPITI), published by IPE and NRDC since 2009, covers the number of subindicators, including environmental supervisory information, interaction and response, enterprise emission data, and environmental impact assessment information, each of which is scored in terms of systematicity, timeliness, friendliness, and completeness. It can effectively represent the level of environmental information disclosure in cities (Feng et al., 2021a; Feng et al., 2021b; Zhong et al., 2021).
Intermediate variables: 1) Industrial pollution emissions (POLLUTION)—industrial pollution emissions measure the damage caused to the environment by enterprises in the production process. Reducing industrial pollutant emissions is an important manifestation for enterprises to achieve clean production and sustainable development. According to Zhang et al. (2018), this study uses the sum of industrial wastewater emissions, industrial sulfur dioxide emissions, and industrial soot emissions to represent urban industrial pollutant emissions; and 2) technological innovation (TECH)—in this study, the total number of patents granted in a city in a year represents the level of technological innovation. The number of patents granted reflects the new basic inventions, utility model inventions, and appearance patents of the city in that year. The higher the number of patents granted, the more likely an enterprise is to achieve product innovation and process innovation.
Control variables: 1) foreign direct investment (FDI)—foreign direct investment has both positive and negative effects on the green economy of a city; foreign direct investment may bring advanced production and management technologies to the city, that is, the “pull effect”, while foreign direct investment may also bring more “energy-intensive and high-polluting” enterprises, which are harmful to the local ecological environment, that is, the “suppression effect.” The “pull effect” is that foreign direct investment may bring advanced production and management technologies to the city, while foreign direct investment may also bring more “energy-intensive and polluting” enterprises, which may harm the local ecological environment. This indicator is measured by the actual amount of foreign investment utilized by each city in the current year; 2) education level (LAOBR)—according to Peng et al. (2020), this study uses (number of students graduating from tertiary education in the year*12 + number of students graduating from secondary school*9 + number of primary school graduates*6) to measure the level of education in a city. The higher the level of education, the higher the quality of the urban labor force and the greater the potential for developing high-end manufacturing or high-tech industries. The expected coefficient of this indicator is positive; 3) industrial structure (OIS)—this study measures the city’s industrial structure by the proportion of tertiary industry output in GDP (Cheng et al., 2018). The higher the proportion of tertiary industry indicates that the city is less dependent on industrial industry and faces fewer obstacles in developing a green economy. The expected coefficient of this indicator is positive; and 4) transportation infrastructure (ROAD)—a well-developed transport infrastructure facilitates the efficient flow of energy, raw materials, and products, and is the basic guarantee for the development of a green economy in a city. Referring to the study of Wang et al. (2020), this study selects the road area per square kilometer to represent the transport infrastructure.
The core explanatory variables, mediating variables, and control variables are treated by taking the natural logarithm in this study in order to ensure data smoothness. Data on the core explanatory variables (PITI) are obtained from the annual Environmental Information Disclosure Index report published by IPE and NRDC (www.ipe.org.cn/). Data for the mediating and control variables are obtained from the China Urban Statistical Yearbook 2009–2019 and the China Urban Construction Statistical Yearbook 2008–2018. Table 1 reports the descriptive statistical results of each variable.
Based on the DEA model with undesirable output, this study calculates the Global Malmquist–Luenberger Productivity Index (GMLPI) of 113 cities from 2008 to 2018, and decomposes it into technology change (TC) and technology efficiency change (EC), which meet GMLPI = EC * TC. The TC in each year indicates that the level of technological innovation changes compared with the previous year, that is, the movement of production frontier. If the TC value is greater than 1, it means that there has been technological progress compared with the previous year. The index of technological efficiency change in each year indicates the utilization and development of the existing technology level when the technology level (i.e., the production frontier) is fixed. If the EC value is greater than 1, it means that the technical efficiency has been improved compared with the previous year; that is, the existing technology has been fully utilized through management level, production proficiency, and other ways. The GMLPI value indicates the change in comprehensive efficiency compared with the previous year, and if GMLPI is greater than 1, it indicates the progress of comprehensive efficiency. Figure 2 illustrates the GMLPI/EC/TC trend over the period 2008–2018, and it can be seen the GMLPI was greater than 1 in 2008–2009, 2010–2012, and 2015–2017, indicating that there was an improvement in the green economic efficiency of cities. The product of the GMLPI values for each year is 1.0994, indicating that the average value of green economic efficiency for the 113 cities rose by approximately 9.94% in 2018 compared with that in 2008.
Table 2 reports the regression results and test results based on mixed OLS regression, random-effects, and fixed-effects models. The results of the LM test and the F test show that the mixed OLS regression is biased, and the possible individual effects should be considered; the Hausman test shows that the fixed-effects model is optimum. The results in the third column of Table 2 show that the coefficient of the LNPITI is positive and significant (0.0235, p-value: 0.066); that is, EID has promoted UGEE obviously. The results in the fourth column of Table 3 show that the quadratic coefficient of the LNPITI is significantly positive and the primary coefficient is significantly negative; there is a “U-shaped” curve relationship between EID and UGEE. According to the coefficient, the value of the LNPITI at the inflection point of the curve is about 3.274; that is, when the PITI is lower than 26.41, it will inhibit UGEE. When the PITI crosses the inflection point, environmental information disclosure, as one of the means of environmental regulation, will promote the development of urban economy to a more sustainable and green direction. In 2008, the PITI of 55 cities was lower than 26.41, and all cities crossed the inflection point in 2018 except Mudanjiang. This warns the government that EID may inhibit industrial development in the short time, and even lead to a crowding-out effect on enterprises with high pollution. However, in the long run, it is conducive to the sustainable development of cities and regions. For the government, the exchange of the cost of environment and energy consumption for economic growth is not a long-time solution; it is obvious that the implementation and strengthening of various environmental policies, promoting industrial green transformation, and upgrading are the fundamental ways of sustainable economic development. In addition, the control variables also provide valuable information. Foreign direct investment, human capital level, and transportation infrastructure construction all play an obvious role in promoting urban green economy.
The above benchmark regression results show that the EID can promote the UGEE. Furthermore, based on the mediating effects model, this section analyzes the mediating effect of industrial pollution emissions and technological innovation.
Table 4 reports the regression results with industrial pollution emissions as the mediating variable: Model 5 and Model 6 describe the impact of information disclosure on industrial pollution; Model 7 describes the impact of industrial pollution on urban green economic efficiency; and Model 8 tests the validity of the mediating variables. The results of Model 5 show that an increase of 1% in the LNPITI can significantly reduce POLLUTION by 0.3831%. The coefficient of POLLUTION in Model 7 is negative and significant (−0.0367, p-value: 0.000), indicating that the reduction in industrial pollution emissions will improve the efficiency of urban green economy. Model 8 shows that when industrial pollution emissions are included in the regression model, the regression coefficient and significance of information disclosure are significantly reduced; that is, the mediating variable is effective. The mediating effect of industrial pollution emissions is about 0.0136, accounting for about 0.367% of the total effect. Through the “supervision effect” and “warning effect,” EID promotes enterprises to improve their cleaner production capacity and reduces industrial pollution emissions. The reduction in industrial pollutant emissions also alleviates haze pollution and ultimately shows the promotion of UGEE. Further, Model 6 shows that the primary coefficient of the LNPITI is significantly positive and the secondary coefficient is significantly negative. It shows there is an “inverted U-shaped” curve relationship between them. According to the coefficient, the value of the LNPITI at the inflection point of the curve is about 3.093; that is, when the PITI exceeds 22.04, the impact on industrial pollution emissions will change. This shows that the “warning effect” and the “supervision effect” need to be accumulated to a certain extent in order to restrict the emission behavior of enterprises. In 2008, a total of 39 cities were on the left side of the curve; that is, the level of information disclosure increased industrial pollution emissions. In 2018, all cities are on the right side of the curve; that is, all sample cities have realized the weakening effect of information disclosure on industrial pollution emissions.
Table 3 reports the regression results with technological innovation as the mediating variable. Model 9 and Model 10 describe the impact of EID on technological innovation. Model 11 describes the impact of technological innovation on UGEE. Model 12 tests the validity of the mediating variables. Model 9 shows that an increase of 1% in the LNPITI will promote technological innovation by about 0.7213%; the coefficient of technological innovation in Model 11 is positive and significant (0.0430, p-value:0.000), which indicates that EID promotes the technological innovation of government and enterprises, and technological innovation further promotes the green economic efficiency of cities. The regression results in Tables 3, 4 support Hypothesis 3; that is, reducing industrial pollution emissions and improving technological innovation are important ways for environmental information disclosure to affect the urban green economic efficiency. The mediating effect of technological innovation is about 0.032, and the ratio of mediating effect to total effect is about 0.579. In Model 10, the primary coefficient of the LNPITI is negative and the secondary coefficient is positive, which indicates there is a “U-shaped” curve relationship between EID and technological innovation. According to the regression coefficient, the value of the LNPITI at the inflection point is about 2.258; that is, when the PITI exceeds 9.57, it will promote technological innovation.
In the previous section, the UGEE is calculated using the Global Malmquist model. In the robustness test, this study uses the following two models to re-evaluate the urban green economic efficiency: 1) Andersen and Petersen (1993) proposed the radial superefficiency DEA model, and Tone (2002) proposed the SBM superefficiency model by combining the SBM distance function with the superefficiency method; and 2) the direction distance function (DDF) is the general expression of the radial DEA model, and it is also the most widely used DEA method. According to Chung et al. (1997), DDF is used to measure the efficiency of urban green economy. The results of the robustness test are reported in Table 5. Model 13, Model 14, and Model 15 report the regression results of GUEE based on the SBM superefficiency model. Model 16, Model 17, and Model 18 are regression results of GUEE based on the DDF model. The conclusion of Table 6 shows that the coefficient of EID is still significantly positive after replacing the measurement method of GUEE, while industrial pollution emission and technological innovation are still important transmission channels.
The previous analysis has empirically tested whether information disclosure can significantly improve the efficiency of urban green economy. However, for the pursuit of sustainable development, cities with higher efficiency of green economy may pursue to improve the level of information disclosure. Therefore, the improvement in urban green economic efficiency may have an impact on environmental information disclosure; that is, there may exist some endogenous problems caused by reverse causality. This section uses the instrumental variable method to solve the problem. The instrumental variable (IV) must satisfy the two conditions of “related to explanatory variables” and “independent of disturbance terms” (Acemoglu and James, 2001). Effective IV should have geographical or historical attributes. Referring to Zhong et al. (2021), this research selects the number of fixed phones per 100 people (PHONE) as the IV of EID. Firstly, fixed phone undertakes the function of Internet lines in the early stage of Internet popularization in China, and families who want to connect to the Internet must have fixed phone at first. The popularity of Internet will also affect the participation of society and residents in Internet information. Therefore, the number of fixed phones per 100 people will significantly affect the EID, which conforms to the requirements of IV and endogenous variable. Secondly, the IV has historical attributes and are not related to the disturbance items in the sample period; that is, the urban green economic efficiency cannot affect the IV. According to Acemoglu and James (2001), the two-stage instrumental variable model was set as follows:
Formula (8) is the first-stage and second-stage model setting of the instrumental variable method, in which the first-stage regression tests the explanatory effect of IV on endogenous variable,
The previous empirical results have verified that EID can promote UGEE. However, cities have various industrial structure, and the direction and coefficient of EID may be unequal. Therefore, it is necessary to discuss the classification of cities.
According to the industrial structure, EID mainly restricts the production behavior of the government and industrial enterprises. Cities with the secondary industry as the pillar face more environmental dilemmas in the process of sustainable development. In the process of sustainable development, eliminating backward production capacity and improving energy efficiency are important ways for industry to change from “high energy consumption and high pollution” to “clean production,” and environmental information disclosure will accelerate the development of the industry to a more sustainable direction. Therefore, this study holds that the impact of environmental information disclosure on industrial cities will be higher than on nonindustrial cities. In this study, cities with the second industry that accounts for more than 50% of GDP are divided into industrial cities, and the rest of the city is divided into nonindustrial cities. Table 5 reports the regression results under the fixed-effects model. For industrial cities, every 1% increase in information disclosure will significantly promote the growth of urban green economic efficiency by about 0.0329%. The coefficient is higher than the regression result of Model 3 in Table 3, which shows that EID plays a more significant role in the green economy of industrial cities. In nonindustrial cities, EID has a positive effect on UGEE, but it is not statistically significant. The results of the mediating effects model show that industrial pollutant emissions and technological innovation are significant transmission pathways in both industrial and nonindustrial cities.
The pervious conclusions show that EID has a significant positive impact on UGEE, and industrial pollution emissions and technological innovation are effective mediating variables. The robustness test confirms that the empirical results are credible. This section will discuss the main conclusions of this study and their possible reasons.
The role of EID in promoting industrial pollutant emission reduction—the environmental information disclosure evaluates the daily pollution emission data and environmental behavior of enterprises. By correcting the information asymmetry between the central government and the local government, and the local government and enterprises in pollutant discharge, EID may restrict the emission of pollutants through the following three ways: 1) The government can formulate more sophisticated environmental policies based on environmental information and adopt more targeted environmental regulation measures for enterprises that cause serious pollution according to the characteristics of the city’s industrial sector; 2) environmental information disclosure makes enterprise pollution behavior exposed to the supervision of society and public, and residents will force enterprises to reduce pollutant emissions through public opinion and “vote with their feet”; and 3) as the environmental restraint of the central government to local governments and enterprises, the central environmental protection supervision has strong mandatory and binding force, and it can produce significant emission reduction and environmental improvement in a short period. The above paths will eliminate the illegal sewage discharge of industrial enterprises and establish a more standardized pollutant discharge supervision system. For example, Qingdao, Shandong Province, has achieved full coverage of online monitoring of pollution emissions from all key industrial enterprises since 2014, which ensures that the industrial pollutants (including wastewater and waste gas) discharged by enterprises every day are under the supervision of the government. Online monitoring avoids enterprises from falsely reporting and concealing pollution data. The online monitoring system has also directly improved the ecological environment of Qingdao, with an average annual PM2.5 from 2008 to 2014. The concentration of PM2.5 has always exceeded 50, showing a significant downward trend after 2014 and 34.8 in 2018. The case of Qingdao also reminds the governments of all cities that online monitoring plays a significant and effective role in pollutant emission reduction. To sum up, EID has a significant regulatory effect on industrial pollutant emissions, and pollutant emission reduction will improve haze pollution and improve the ability of urban cleaner production.
The role of EID in promoting technological innovation—the technological innovation effect of EID is reflected in the fact that the government and enterprises will drive the “competition to the bottom line.” Under the pollutant discharge standards formulated by the government, enterprises need to meet the emission standards by introducing advanced cleaner production technology and environmental protection technology. The more stringent the means of environmental regulation, the higher the investment of enterprises in technological innovation. The empirical results support the Porter hypothesis. Environmental information disclosure may have an impact on enterprises’ innovation behavior through “backward force effect” and “pull effect.” On the one hand, EID sets the bottom line for cleaner production of enterprises; enterprises that exceed the bottom will be fined or stopped production. Therefore, enterprises are bound to consider updating environmental protection equipment and innovating production technology, namely “backward force effect.” On the other hand, technological innovation will impel environmentally friendly products more popular in the market while helping enterprises to reduce emissions, and achieve a win–win situation of cleaner production and enterprise benefits, namely the “pull effect.” For example, in 2015, the Ministry of Industry and Information Technology issued the Action Plan for Clean and Efficient Utilization of Coal in Industrial Field (2015–2020), which puts forward macro constraints on coal utilization, including saving 80 million tons of coal consumption, reducing 500,000 tons of smoke and dust emission, 600,000 tons of sulfur dioxide emission, etc. Under the constraints of sustainable development indicators, the number of invention patents for coal mining and washing industry in China from 2015 to 2017 was 630, 680, and 821, respectively, realizing the rapid development of the cleaner production technology. Under the two effects, EID will change the investment behavior and technological innovation ability of enterprises. Technological innovation will lead to the reduction in pollutant emissions and the improvement in economic benefits, so as to improve the efficiency of urban green economy.
The empirical results show that there is a “U-shaped” curve relationship between EID and UGEE and technological innovation, and an “inverted U-shaped” curve relationship between EID and industrial pollution emissions. When the level of EID is low, it can inhibit sustainable development; when the EID crosses the inflection point, it will promote the green economy. Firstly, there is a time-lag effect in technological innovation and sustainable transformation of enterprises; and secondly, EID needs a long time of exploration in order to achieve “precise policy implementation,” and to formulate targeted environmental regulation policies according to the characteristics of the city’s industries and enterprises. When the scale effect of environmental information disclosure is formed, it can restrict the whole industry and all enterprises. At this time, the construction of environmental information disclosure system will improve the green economic efficiency of enterprises and industries by reducing pollutant emissions and promoting technological innovation.
This study empirically analyses the impact of EID on UGEE using the panel data model based on panel data from 113 cities in China from 2008 to 2018. The mediating effects model is used to explore the “black-box” system between EID and UGEE. Robustness tests are carried out using instrumental variables and replacement variables. The main conclusions of this study are as follows:
1. The urban green economic efficiency of 113 cities showed an upward trend from 2008 to 2018. Compared with 2008, the Global Malmquist–Luenberger Productivity Index in 2018 increased by about 9.94%.
2. Environmental information disclosure has promoted the urban green economic efficiency by improving technological innovation and reducing the emission of industrial pollutants.
3. Environmental information disclosure shows a U-shaped curve relationship of “inhibition before promotion” to urban green economic efficiency, which is mainly due to the U-shaped curve relationship between environmental information disclosure and technological innovation, and the inverted U-shaped curve relationship between environmental information disclosure and industrial pollutant emission.
4. Compared with nonindustrial cities, environmental information disclosure plays a more significant role in sustainable development in industrial cities.
Based on the main findings and discussions, this study makes the following policy recommendations: 1) The government should accelerate the construction of the environmental information disclosure system to optimize the problems of poor information flow and “one-size-fits-all” policies in the current system. Environmental information disclosure can significantly optimize environmental information asymmetries between the central government and local governments, and between local governments and enterprises, which can help to formulate more sophisticated environmental policies. In addition, the findings of the mediating effect suggest that governments need to use policy, economic, and administrative instruments to promote a greater role for environmental information disclosure systems in the reduction of industrial pollutants and improvement in technological innovation. 2) The government should accelerate the initial construction of the environmental information disclosure system so that it can cross the curve inflection point as soon as possible. The curvilinear relationship between environmental information disclosure and the green economy shows that the means of environmental regulation may exist a lag, and the low level of the information disclosure system may even lead to more industrial pollutants. Therefore, the government needs to use environmental monitoring and sewage charges to eliminate the negative environmental externalities that may arise in the early stages of the information disclosure system, and guide enterprises to shift toward cleaner production and green growth. 3) Environmental information disclosure can produce a more effective sustainable development effect in industrial cities, and this warns that industrial city governments should pay more attention to the role of environmental information disclosure. In addition, the role of emission tax, environmental protection inspectors, and technological innovation in a green economy should also be fully exploited. In order to develop green industrial economy, cleaner production should promote by eliminating outdated production capacity, standardizing emission practices, and improving energy efficiency.
There are still some spaces for in-depth discussion in this study. This study does not pay attention to the impact of environmental information disclosure on different types of enterprises, mainly because it is difficult to obtain enterprise-level microdata. In addition, in the measurement of urban green efficiency in this study, PM2.5 is used to represent environmental constraints, without considering other environmental factors such as water environment. With more accurate data and more cutting-edge methods, we can get a deeper understanding of the impact of environmental information disclosure on sustainable development.
The original contributions presented in the study are included in the article/Supplementary Material; further inquiries can be directed to the corresponding author.
SL is the independent author of this manuscript and responsible for all the work of this manuscript, including basic analysis, data collection, empirical analysis, and manuscript writing.
The author declares that the research was conducted in the absence of any commercial or financial relationships that could be construed as a potential conflict of interest.
All claims expressed in this article are solely those of the authors and do not necessarily represent those of their affiliated organizations, or those of the publisher, the editors, and the reviewers. Any product that may be evaluated in this article, or claim that may be made by its manufacturer, is not guaranteed or endorsed by the publisher.
Acemoglu, D., Johnson, S., and Robinson, J. A. (2001). The Colonial Origins of Comparative Development: An Empirical Investigation. Am. Econ. Rev. 91 (5), 1369–1401. doi:10.1257/aer.91.5.1369
Ajayi, O. C., Jack, B. K., and Leimona, B. (2012). Auction Design for the Private Provision of Public Goods in Developing Countries: Lessons from Payments for Environmental Services in Malawi and Indonesia. World Dev. 40 (6), 1213–1223. doi:10.1016/j.worlddev.2011.12.007
Ambec, S., Cohen, M. A., Elgie, S., and Lanoie, P. (2013). The Porter Hypothesis at 20: Can Environmental Regulation Enhance Innovation and Competitiveness? Rev. Environ. Econ. Policy 7 (1), 2–22. doi:10.1093/reep/res016
Andersen, P., and Petersen, N. C. (1993). A Procedure for Ranking Efficient Units in Data Envelopment Analysis. Manag. Sci. 39, 1261–1264. doi:10.1287/mnsc.39.10.1261
Chen, Z.-M., Liu, Y., Qin, P., Zhang, B., Lester, L., Chen, G., et al. (2015). Environmental Externality of Coal Use in China: Welfare Effect and Tax Regulation. Appl. Energy 156, 16–31. doi:10.1016/j.apenergy.2015.06.066
Cheng, Z. H., Li, L. S., and Liu, J. (2018). Industrial Structure, Technical Progress and Carbon Intensity in China’s Province. Renew. Sustain. Energy Rev. 81 (2), 2935–2946. doi:10.1016/j.rser.2017.06.103
Cheng, Z., Li, L., and Liu, J. (2020). The Impact of Foreign Direct Investment on Urban PM2.5 Pollution in China. J. Environ. Manag. 265, 110532. doi:10.1016/j.jenvman.2020.110532
Chung, Y. H., Färe, R., and Grosskopf, S. (1997). Productivity and Undesirable Outputs: A Directional Distance Function Approach. J. Environ. Manag. 51, 229–240. doi:10.1006/jema.1997.0146
Du, K., Cheng, Y., and Yao, X. (2021). Environmental Regulation, Green Technology Innovation, and Industrial Structure Upgrading: The Road to the Green Transformation of Chinese Cities. Energy Econ. 98, 105247. doi:10.1016/j.eneco.2021.105247
Elnaboulsi, J. C., Daher, W., and Sağlam, Y. (2018). On the Social Value of Publicly Disclosed Information and Environmental Regulation. Resour. Energy Econ. 54, 1–22. doi:10.1016/j.reseneeco.2018.05.003
Emrouznejad, A., and Yang, G.-L. (2016). A Framework for Measuring Global Malmquist-Luenberger Productivity Index with CO2 Emissions on Chinese Manufacturing Industries. Energy 115 (1), 840–856. doi:10.1016/j.energy.2016.09.032
Fan, L., Yang, K., and Liu, L. (2020). New Media Environment, Environmental Information Disclosure and Firm Valuation: Evidence from High-Polluting Enterprises in China. J. Clean. Prod. 277, 123253. doi:10.1016/j.jclepro.2020.123253
Fare, R., Grosskopf, S., and Margaritis, D. (2010). Time Substitution With Application to Data Envelopment Analysis. J. Oper. Res. 206 (3), 686–690. doi:10.1016/j.ejor.2010.03.021
Feng, Y., Chen, H., Chen, Z., Wang, Y., and Wei, W. (2021a). Has Environmental Information Disclosure Eased the Economic Inhibition of Air Pollution? J. Clean. Prod. 284, 125412. doi:10.1016/j.jclepro.2020.125412
Feng, Y., Wang, X., and Liang, Z. (2021b). How Does Environmental Information Disclosure Affect Economic Development and Haze Pollution in Chinese Cities? The Mediating Role of Green Technology Innovation. Sci. Total Environ. 775, 145811. doi:10.1016/j.scitotenv.2021.145811
Fu, T., Cai, C., and Jian, Z. (2020). The Illusion of "Win-Win" Solution: Why Environmental Regulation in china Promotes Firm Performance? Struct. Change Econ. Dyn. 52, 366–373. doi:10.1016/j.strueco.2019.12.007
Gao, Y., Zhang, M., and Zheng, J. (2021). Accounting and Determinants Analysis of China's Provincial Total Factor Productivity Considering Carbon Emissions. China Econ. Rev. 65, 101576. doi:10.1016/j.chieco.2020.101576
Hancevic, P. I. (2016). Environmental Regulation and Productivity: the Case of Electricity Generation under the CAAA-1990. Energy Econ. 60, 131–143. doi:10.1016/j.eneco.2016.09.022
Jiang, C. L., Zhang, F. Y., and Chong, W. (2021). Environmental Information Disclosure, Political Connections and Innovation in High-Polluting Enterprises. Sci. Total Environ. 764, 144248. doi:10.1016/j.scitotenv.2020.144248
Jiang, H.-D., Liu, L.-J., Dong, K., and Fu, Y.-W. (2022a). How Will Sectoral Coverage in the Carbon Trading System Affect the Total Oil Consumption in China? A CGE-Based Analysis. Energy Econ. 110, 105996. doi:10.1016/j.eneco.2022.105996
Jiang, H.-D., Purohit, P., Liang, Q.-M., Dong, K., and Liu, L.-J. (2022b). The Cost-Benefit Comparisons of China's and India's NDCs Based on Carbon Marginal Abatement Cost Curves. Energy Econ. 109, 105946. doi:10.1016/j.eneco.2022.105946
Jiang, H. D., Xue, M. M., Dong, K. Y., and Liang, Q. M. (2020). How Will Natural Gas Market Reforms Affect Carbon Marginal Abatement Costs? Evidence from China. Econ. Syst. Res. 34 (2), 1868410. doi:10.1080/09535314.2020.1868410
Jolink, A., and Niesten, E. (2020). Credibly Reducing Information Asymmetry: Signaling on Economic or Environmental Value by Environmental Alliances. Long. Range Plan. 54, 101996. doi:10.1016/j.lrp.2020.101996
Li, K., Ma, M., Xiang, X., Feng, W., Ma, Z., Cai, W., et al. (2022). Carbon Reduction in Commercial Building Operations: A Provincial Retrospection in China. Appl. Energy 306, 118098. doi:10.1016/j.apenergy.2021.118098
Liu, H., Yang, R., Wu, D., and Zhou, Z. (2021). Green Productivity Growth and Competition Analysis of Road Transportation at the Provincial Level Employing Global Malmquist-Luenberger Index Approach. J. Clean. Prod. 279, 123677. doi:10.1016/j.jclepro.2020.123677
Liu, J., Zhao, M., and Wang, Y. (2020b). Impacts of Government Subsidies and Environmental Regulations on Green Process Innovation: A Nonlinear Approach. Technol. Soc. 63, 101417. doi:10.1016/j.techsoc.2020.101417
Liu, X., Ji, X., Zhang, D., Yang, J., and Wang, Y. (2019). How Public Environmental Concern Affects the Sustainable Development of Chinese Cities: An Empirical Study Using Extended DEA Models. J. Environ. Manag. 251, 109619. doi:10.1016/j.jenvman.2019.109619
Liu, Y., Zhu, J., Li, E. Y., Meng, Z., and Song, Y. (2020a). Environmental Regulation, Green Technological Innovation, and Eco-Efficiency: The Case of Yangtze River Economic Belt in China. Technol. Forecast. Soc. Change 155, 119993. doi:10.1016/j.techfore.2020.119993
Mackinnon, D. P., Lockwood, C. M., Hoffman, J. M., West, S. G., and Sheets, V. (2002). A Comparison of Methods to Test Mediation and Other Intervening Variable Effects. Psychol. Methods 7 (1), 83–104. doi:10.1037/1082-989x.7.1.83
Mackinnon, D. P., Warsi, G., and Dwyer, J. H. (1995). A Simulation Study of Mediated Effect Measures. Multivar. Behav. Res. 30 (1), 41–62. doi:10.1207/s15327906mbr3001_3
Mohmand, Y. T., Mehmood, F., Mughal, K. S., and Aslam, F. (2020). Investigating the Causal Relationship between Transport Infrastructure, Economic Growth and Transport Emissions in Pakistan. Res. Transp. Econ. 88, 100972. doi:10.1016/j.retrec.2020.100972
Nie, X., Wu, J., Chen, Z., Zhang, A., and Wang, H. (2021). Can Environmental Regulation Stimulate the Regional Porter Effect? Double Test from Quasi-Experiment and Dynamic Panel Data Models. J. Clean. Prod. 314, 128027. doi:10.1016/j.jclepro.2021.128027
Peng, H., Qi, L., Wan, G., Li, B., and Hu, B. (2020). Child Population, Economic Development and Regional Inequality of Education Resources in China. Child. Youth Serv. Rev. 110, 104819. doi:10.1016/j.childyouth.2020.104819
Porter, M. E., and Linde, C. V. d. (1995). Toward a New Conception of the Environment-Competitiveness Relationship. J. Econ. Perspect. 9 (4), 97–118. doi:10.1257/jep.9.4.97
Qu, W., Qu, G., Zhang, X., and Robert, D. (2021). The Impact of Public Participation in Environmental Behavior on Haze Pollution and Public Health in China. Econ. Model. 98, 319–335. doi:10.1016/j.econmod.2020.11.009
Shao, Q., Chen, L., Zhong, R., and Weng, H. (2021). Marine Economic Growth, Technological Innovation, and Industrial Upgrading: A Vector Error Correction Model for China. Ocean Coast. Manag. 200, 105481. doi:10.1016/j.ocecoaman.2020.105481
Sun, Z., Ma, Z., Ma, M., Cai, W., Xiang, X., Zhang, S., et al. (2022). Carbon Peak and Carbon Neutrality in the Building Sector: A Bibliometric Review. Buildings 12, 128. doi:10.3390/buildings12020128
Tone, K. (2002). A Slacks-Based Measure of Super-Efficiency in Data Envelopment Analysis. Eur. J. Operational Res. 143, 32–41. doi:10.1016/s0377-2217(01)00324-1
Tu, Z., Hu, T., and Shen, R. (2019). Evaluating Public Participation Impact on Environmental Protection and Ecological Efficiency in China: Evidence from PITI Disclosure. China Econ. Rev. 55, 111–123. doi:10.1016/j.chieco.2019.03.010
Wang, C., Lim, M. K., Zhang, X., Zhao, L., and Lee, P. T.-W. (2020). Railway and Road Infrastructure in the Belt and Road Initiative Countries: Estimating the Impact of Transport Infrastructure on Economic Growth. Transp. Res. Part A Policy Pract. 134, 288–307. doi:10.1016/j.tra.2020.02.009
Wang, N., Chen, J., Yao, S., and Chang, Y.-C. (2018b). A Meta-Frontier DEA Approach to Efficiency Comparison of Carbon Reduction Technologies on Project Level. Renew. Sustain. Energy Rev. 82 (3), 2606–2612. doi:10.1016/j.rser.2017.09.088
Wang, Y., and Shen, N. (2016). Environmental Regulation and Environmental Productivity: The Case of China. Renew. Sustain. Energy Rev. 62, 758–766. doi:10.1016/j.rser.2016.05.048
Wu, G., Hong, J., Tian, Z., Zeng, Z., and Sun, C. (2021). Assessing the Total Factor Performance of Wastewater Treatment in China: A City-Level Analysis. Sci. Total Environ. 758, 143324. doi:10.1016/j.scitotenv.2020.143324
Wu, H., Xu, L., Ren, S., Hao, Y., and Yan, G. (2020). How Do Energy Consumption and Environmental Regulation Affect Carbon Emissions in China? New Evidence from a Dynamic Threshold Panel Model. Resour. Policy 67, 101678. doi:10.1016/j.resourpol.2020.101678
Xiang, X., Ma, X., Ma, Z., Ma, M., and Cai, W. (2022b). Python-LMDI: A Tool for Index Decomposition Analysis of Building Carbon Emissions. Buildings 12, 83. doi:10.3390/buildings12010083
Xiang, X., Ma, X., Ma, Z., and Ma, M. (2022a). Operational Carbon Change in Commercial Buildings under the Carbon Neutral Goal: A LASSO-WOA Approach. Buildings 12, 54. doi:10.3390/buildings12010054
Xing, C., Zhang, Y., and Tripe, D. (2021). Green Credit Policy and Corporate Access to Bank Loans in China: The Role of Environmental Disclosure and Green Innovation. Int. Rev. Financial Analysis 77, 101838. doi:10.1016/j.irfa.2021.101838
Yang, Y., Guo, H., Wang, D., Ke, X., Li, S., and Huang, S. (2021). Flood Vulnerability and Resilience Assessment in China Based on Super-Efficiency DEA and SBM-DEA Methods. J. Hydrol. 600, 126470. doi:10.1016/j.jhydrol.2021.126470
Zafar, M. W., Sinha, A., Ahmed, Z., Qin, Q., and Zaidi, S. A. H. (2021). Effects of Biomass Energy Consumption on Environmental Quality: The Role of Education and Technology in Asia-Pacific Economic Cooperation Countries. Renew. Sustain. Energy Rev. 142, 110868. doi:10.1016/j.rser.2021.110868
Zhang, L., Adom, P. K., and An, Y. (2018). Regulation-Induced Structural Break and the Long-Run Drivers of Industrial Pollution Intensity in China. J. Clean. Prod. 198, 121–132. doi:10.1016/j.jclepro.2018.07.008
Zhang, M., Liu, X., Ding, Y., and Wang, W. (2019). How Does Environmental Regulation Affect Haze Pollution Governance?-An Empirical Test Based on Chinese Provincial Panel Data. Sci. Total Environ. 695, 133905. doi:10.1016/j.scitotenv.2019.133905
Keywords: environmental information disclosure, green economy efficiency, mediating effect, nonlinear relationship, sustainability
Citation: Lin S (2022) Can Environmental Information Disclosure Improve Urban Green Economic Efficiency? New Evidence From the Mediating Effects Model. Front. Environ. Sci. 10:920879. doi: 10.3389/fenvs.2022.920879
Received: 15 April 2022; Accepted: 12 May 2022;
Published: 04 July 2022.
Edited by:
Kangyin Dong, University of International Business and Economics, ChinaReviewed by:
Minda Ma, Tsinghua University, ChinaCopyright © 2022 Lin. This is an open-access article distributed under the terms of the Creative Commons Attribution License (CC BY). The use, distribution or reproduction in other forums is permitted, provided the original author(s) and the copyright owner(s) are credited and that the original publication in this journal is cited, in accordance with accepted academic practice. No use, distribution or reproduction is permitted which does not comply with these terms.
*Correspondence: Shihai Lin, bGluc2hpaGFpX2h2Y3N0QDE2My5jb20=
Disclaimer: All claims expressed in this article are solely those of the authors and do not necessarily represent those of their affiliated organizations, or those of the publisher, the editors and the reviewers. Any product that may be evaluated in this article or claim that may be made by its manufacturer is not guaranteed or endorsed by the publisher.
Research integrity at Frontiers
Learn more about the work of our research integrity team to safeguard the quality of each article we publish.