- 1School of Arts and Design, Yanshan University, Qinhuangdao, China
- 2School of Geographical Sciences, Northeast Normal University, Changchun, China
- 3Key Laboratory of Geographical Processes and Ecological Security of Changbai Mountains, Ministry of Education, Changchun, China
- 4School of Fine Arts, Northeast Normal University, Changchun, China
The spatial distribution pattern of brownfields can help governments at all levels, and investors have more detailed information on land resources, prioritize brownfield redevelopment, and guide urban spatial and strategic planning. Despite increasing global concern, yet knowledge of brownfield distribution patterns at the macro-scale remains limited, especially in China. Derived from the China National Knowledge Infrastructure (CNKI), Web of Science (WOS), and Chinese Industrial Heritage List published between 2001 and 2019, we present the first comprehensive dataset of known brownfield sites and their distributions in China. The results revealed that the dataset contains 816 georeferenced brownfield records from 255 cities. Brownfields were mainly distributed southeast of the “Heihe-Tengchong Line,” with an overall spatial distribution pattern of “East-dense-West-sparse.” In terms of brownfield type, industrial brownfields were the most numerous, followed by mining brownfields. Nearest neighbor indicator analysis suggested that brownfields in China present significant spatial agglomeration characteristics, and that the six types of brownfields manifest different scales of spatial agglomeration. The hot spots were mainly concentrated in the Yangtze River Delta, Beijing–Tianjin–Hebei, and Pearl River Delta urban agglomerations. Factors influencing brownfield formation were related to industrial structure adjustments, resource depletion, accelerated urbanization, and the orientation of national policies, with industrial structure adjustments being the leading cause. Mastering the spatial distribution of brownfields can coordinate land use transformation planning and guide brownfield redevelopment.
1 Introduction
The issue of brownfields remains one of the greatest challenges faced by urban planners and developers. In particular, in shrinking cities and old industrial areas, sites previously used for industrial, commercial, or mining purposes have been underutilized or abandoned (Hayek et al., 2010; Rall and Haase, 2011; Zhang et al., 2021). The existence of brownfields can lead to soil pollution and degraded quality of life, which adversely affects a city’s economy, society, and the environment. Brownfield redevelopment can promote the creation of walkable neighborhoods, improve public transportation, control green field encroachment and urban sprawl, and revive local markets, in addition to the direct benefits of returning sites to productive use and increasing the tax base. The rewards of brownfield redevelopment are vast and have the capability to last well into the future (Amekudzi et al., 2003; Chrysochoou et al., 2012).
Brownfields are common in both developed and developing countries (Howard and Olszewska, 2011; Loures and Vaz, 2018; Mahzouni, 2018). To better manage and update brownfield data, various countries have established national brownfield databases. For instance, in the United States, there are 500,000–1,000,000 registered brownfield sites (Hipel et al., 2010), while 21,000 contaminated sites have been identified in England (Longo and Campbell, 2017). In the Czech Republic, there are an estimated 8,500–11,700 brownfield sites (CzechInvest, 2021). The US Environmental Protection Agency (USEPA) established the first brownfield database with relatively complete information. The database is available to the public free of charge and shares the location of brownfield sites, addresses of units releasing toxic substances around them, and information on actions taken against contaminated plots for redevelopment and policy decisions (Leigh and Coffin, 2000; Coffin, 2003). The UK National Environment Agency, Transport, and Land Departments jointly established a National Land Use Database. The database was developed based on the need to monitor the supply of brownfields to provide adequate and strategic supply of land and buildings for housing and other economic activities. In the Czech Republic, an extensive database of brownfields is administered by the Ministry of Environment, the primary purpose of which is the protection of the environment (Osman et al., 2015).
The literature on brownfield spatial distributions and formation factors has grown considerably in the past few years, for example, the city of Minneapolis in the United States (Vaidya, 2015), Brno (Kunc et al., 2014; Frantál et al., 2015), Ostrava (Novosák et al., 2013), and Karvina in the Czech Republic (Martinat et al., 2016; Škrabal et al., 2021) and Changchun in China (Song et al., 2022). The research content of spatial distributions is mainly industrial brownfields (Smoļakova, 2017; Modica, 2019), which mostly guide local soil management (Bambra et al., 2014; Boente et al., 2018), land use policies (Page and Berger, 2006), and brownfield redevelopment (Filip and Cocean, 2012; Kramářová and Juhásová Šenitková, 2018; Bardos et al., 2020). Accurately determining macro-scale brownfield spatial distributions can provide a reference for formulating differentiated and regionalized redevelopment policies according to local conditions (Newell and McGreal, 2017). Previous studies have mainly analyzed the spatial characteristics, restoration methods, and redevelopment of brownfields from a micro perspective. Although they are highly targeted, the overall role of the macro-level has been ignored and has hindered the government from formulating reconstruction strategies. The research scale focuses on the spatial distribution of brownfields in a city or region. Moreover, the study of a single province and city cannot provide an overall grasp of the spatial distribution of macro-scale brownfields, which is limited by data collection. From the research content, they have most often been based on statistical classification of basic information, such as brownfield location, quantity, and area. The characteristics of such distributions have rarely been explored. The factors of brownfield formation are affected by the changes in the economic system, industrial structure adjustment, and accelerated urbanization. Some scholars have attributed the main causes of brownfield formation to deindustrialization and urbanization (Liu et al., 2014). The factors influencing the formation of brownfield sites in China contain both similarities and differences in relation to other countries. Deindustrialization and urbanization are the main factors in the production of global brownfields. Due to China’s late industrialization process and its unique institutional environment, such as the dual land system, land ownership, spatial distribution, the mechanisms by which the sites are created, and the stakeholders involved, certain differences can be perceived compared to other countries.
As the largest developing country and second largest economy in the world, China plays an important role in the world economic system. It is also one of the countries with the largest brownfield stock. According to statistical data for 2015, more than 100,000 factories have closed since 2001, and over two million hectares of brownfield sites that had been seriously polluted have been left untreated in major cities (Liu et al., 2017). At present, China has not established a database of brownfields, and it is unclear how many exist, where they are concentrated, and to what types they belong. Second, due to the lack of open data on soil pollution across the country and the low degree of data availability, scientific research methods to identify brownfields have not been established, which poses a huge obstacle to mastering brownfield spatial distributions and redevelopment planning in China.
The contributions of this study are threefold. First, it established the first comprehensive dataset of known brownfields in China. Second, we analyzed the spatial distribution characteristics of these brownfields and identified the major factors in their formation. Third, this research can help governments at all levels and investors have more detailed information on land resources, prioritize brownfield redevelopment, and guide urban spatial and strategic planning.
The remainder of this article is organized as follows. An overview of the meta-analysis and spatial analysis utilized, with explanations for the main associated procedures is presented in Section 2. The overall spatial distributions of brownfields in China and of different types of brownfields based on the dataset are analyzed in Section 3 and the main factors of brownfield formation are discussed. The results of and comparison with previous studies, as well as suggested policy responses are provided in Section 4. Finally, concluding remarks and discussion of future research directions are presented in Section 5.
2 Materials and Methods
2.1 Meta-Analysis
As an important research method, meta-analysis refers to the systematic and comprehensive statistical analysis of the results of multiple experiments with the same research purpose and independent of each other (Brander et al., 2012; Chaikumbung et al., 2016). It was originally proposed as a research synthesis method by Glass (1976). Existing meta-analyses mainly use the induction, measurements, and conclusions of a research field, cases (Chen et al., 2019), and indicators to identify scientific consensus. Meta-analysis has been used in various fields, such as medicine, pedagogy, and psychology, and has gradually been applied to geography (van Zanten et al., 2014; Eötvös et al., 2018). Preferred Reporting Items for Systematic Reviews and Meta-Analyses (PRISMA) is an evidence-based minimum set of items for reporting in systematic reviews and meta-analyses (Moher et al., 2009). As a basic data source for China’s brownfield spatial distributions, this study applies the PRISMA approach to collect brownfield case information from published literature and industrial heritage directories.
2.2 Data Collection
The literature review procedures are outlined in Figure 1. A literature search was performed following guidelines from PRISMA. Publications in both Chinese and English were collected by searching two major scientific citation indexing services, the China National Knowledge Infrastructure (CNKI) and Web of Science (WOS), respectively. Chinese brownfield-related literature is mainly published on CNKI. WOS is the most authoritative scientific and technological literature retrieval platform. The earliest Chinese literature on the theme of brownfields appeared in 2001 (Niu, 2001). Therefore, publication dates were limited to “2001.1.1–2019.12.31.” We used the topics “brownfield” AND “China” with WOS. Since China has no official definition of brownfields, the following search terms were use in the CNKI: “brownfield,” “industrial heritage AND transformation,” “industrial heritage AND renovate,” “industrial heritage AND regeneration,” “abandoned land AND transformation,” “abandoned land AND renovate,” “abandoned land AND regeneration” in Chinese. We searched publications ranging from journal articles to conference proceedings or degree theses. We also searched the official list of China’s industrial heritage sites and obtained 307 records.
A total of 1924 abstracts were retrieved for screening, 1,638 of which were from CNKI (in Chinese) and 286 from WOS (in English). 1) Abstracts that were not relevant to the brownfield transformation were excluded. This led to 1,217 Chinese and 32 English papers being selected for full-text review and further geo-information extraction. 2) Of the available full texts, publications that did not refer to China’s brownfield cases, lacked brownfield data, or duplicate brownfield sites were deleted, leaving 455 Chinese and 16 English publications eligible for extraction. 3) A total of 196 industrial heritage records were obtained by deleting records that did not meet brownfield requirements from 307 industrial heritage lists.
Location information was extracted from the records of the relevant primary papers. Following Zhang et al. (2019), the longitude and latitude of a location were determined using a combination of geospatial tools, including the Baidu Map, and Baidu Coordinate Picking System. We updated the place names to match the historical administrative names. We further classified all locations into four different levels according to their geographic scales and administrative levels (i.e., provincial, prefectural, county, and township and finer level). This helps potential users of this dataset extract appropriate sections for use. The locations of brownfields were then visualized using geographic information systems (GIS) software. In total, 608 Chinese, 18 English, and 196 industrial heritage records were identified.
After the data were entered, a second person thoroughly checked the dataset for validity, to avoid errors and duplication. A total of 816 records were identified, 605 of which were from CNKI, 17 from WOS, and 194 from China’s industrial heritage list. Brownfield records include 23 provinces, 255 prefecture-level counties/cities, three autonomous regions, four municipalities directly under the central government, and two special administrative regions. The Xizang Autonomous Region and Ningxia Hui Autonomous Region did not have any brownfield sites.
In the dataset of distribution of brownfields in China, each row represents a single record. The columns in the dataset are as follows:
1) Brownfield location: the geographic scale of location (provincial, prefectural, county, township, or finer level)
2) Latitude and longitude coordinates: latitudinal and longitudinal coordinates (WGS1984 Datum)
3) Brownfield type: identifying brownfield types using brownfield definitions and “Code for Classification of Urban Land Use and Planning Standards of Development Land GB50137-2011”
4) Original use of brownfield information: CNKI, WOS, and list of China’s industrial heritage
5) Brownfield formation factors: based on the literature
2.3 Spatial Distribution Analysis Method
2.3.1 Kernel Density Estimation
Kernel density estimation (KDE) is a non-parametric method used to estimate the probability density function of a random variable. When applied to geospatial analysis, the distribution of spatial variables is fitted as a smooth cone-shaped surface using the kernel function to illustrate its spatial distribution characteristics (Li W et al., 2019). We adopted the method to visually analyze brownfield spatial distributions in China. The formula is as follows:
where f(x) is the density value of the estimated point, h is the bandwidth, which indicates the spatial distance in the text. n is the number of known points in the bandwidth, and the number of brownfields within a given spatial distance in the text. d is the dimension of the data, in the text d = 2. K(x) is the kernel function, and the quartic kernel function is used in ArcGIS software; it is the distance from the estimated point to the point i in the bandwidth range.
2.3.2 Nearest Neighbor Indicator
Proposed by Clark and Evans (1954), the nearest neighbor indicator (NNI) is an index that indicates the proximity of the spatial distribution of point-like geographic elements. It can also determine the type of spatial distribution of point-like elements. The NNI calculation formula is as follows:
where d (NN) is the nearest neighbor distance, d (ran) is the expected average nearest neighbor distance, and its value can generally be expressed as
where N is the number of samples and A is the area of the study region. When NNI is less than 1, the sample points are distributed in an agglomeration. When NNI is greater than 1, the sample points are uniformly distributed, and when NNI is equal to 1, the sample points are randomly distributed. The Z test is generally used to test the reliability of the results.
3 Results
3.1 Spatial Distribution Characteristics of Brownfields
Based on the results, brownfields are mainly concentrated in economically developed areas and near central urban areas. As shown in Figure 2A, the overall brownfield spatial distribution in China was basically consistent with the “Heihe-Tengchong Line” as the boundary, exhibiting a spatial pattern of “East-dense-West-sparse.” There were 791 brownfield sites in the Southeast region of the “Heihe-Tengchong Line,” accounting for 96.94% of the total, and 25 in the northwest region, accounting for 3.06% of the total. The “Heihe-Tengchong Line” is an important geographical boundary that reflects China’s urbanization and economic development levels (Qi et al., 2015; Li et al., 2017). As shown in Figure 2B, the economically developed provinces of Jiangsu, Shandong, Guangdong, and Zhejiang have the largest number of brownfields, followed by resource-dependent regions such as Liaoning, Henan, Hebei, Hubei, and Hunan. The southern provinces have more brownfields than the North, followed by the northeastern provinces, with the lowest number in the Midwest. Brownfields have emerged as a result of the ongoing interaction between industrialization and urbanization, and their distribution is closely related to China’s urbanization and economic development. We used the NNI module in ArcGIS to analyze the average nearest neighbor distance of each brownfield site. According to Table 1, the NNI for the overall spatial distribution of the 816 brownfield sites identified in the study was 0.4, and the Z score was −32.90, which passed the 1% significance level test, indicating that the spatial agglomeration characteristics of brownfields in China are highly significant. As shown in Figure 2C, the KDE method was used to analyze the spatial distribution. According to the natural break method, the kernel density values were divided into seven levels, from high to low. Regions with the highest kernel density values “7.27–18.35” were determined to be high-density areas, values from “1.58–7.27” represented medium-density areas, and those from “0–1.58” represented low-density areas. China’s brownfields were characterized by multi-center agglomerations, and high-density areas were found in the Yangtze River Delta, Beijing–Tianjin–Hebei, Pearl River Delta, and Central Plains urban agglomerations, as well as the urban agglomeration in the middle reaches of the Changjiang River with a strong industrial foundation and developed economy. Shanghai, Tianjin, Beijing, Guangzhou, Nanjing, and Wuhan had brownfield density clusters.
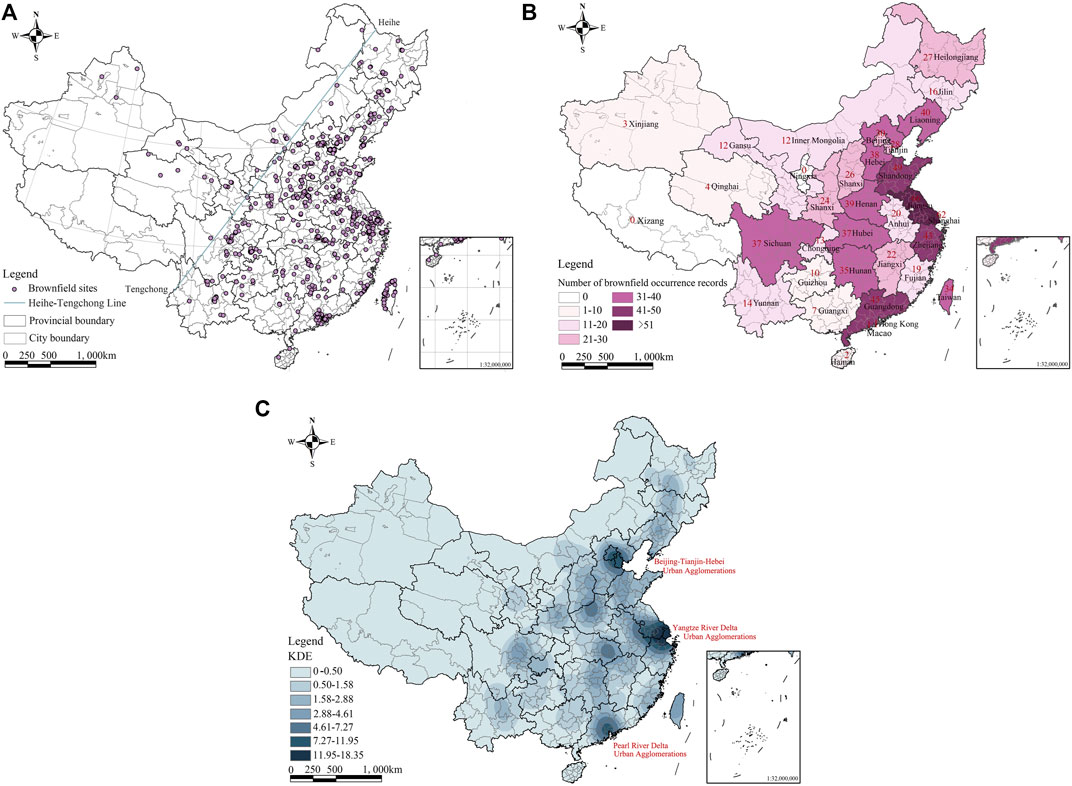
FIGURE 2. Spatial distribution of brownfield occurrence records in China. (A) Locations of brownfield occurrence records in China. (B) Number of brownfield occurrence records by province-level divisions of China. (C) Kernel density estimation of brownfield occurrence records in China.
3.2 Spatial Distribution Characteristics of Different Brownfield Types
According to China’s Code for Classification of Urban Land Use and Planning Standards of Development Land GB50137-2011, brownfield land use functions are classified as industrial, mining, military, commercial activities, storage, public facilities, and transportation facilities land. The brownfield information dataset divided brownfields into six types: industrial, mining, transportation facilities, public facilities, military, and logistics and warehouse (Alker et al., 2000; Franz et al., 2006). Industrial brownfields accounted for the highest number, followed by mining brownfields. Transportation facilities, public facilities, military, and logistics and warehouse brownfields were less common.
NNI was used to test the agglomeration characteristics of the six types of brownfield spatial distribution (Table 1). The NNI of the six types of brownfields was less than 1 in all cases, which passed the 1% significance test and formed a part of a significant agglomeration model. The degree of agglomeration is in the following order: industrial brownfields > transportation facilities brownfields > logistics and warehouse brownfields > public facilities brownfields > military brownfields > mining brownfields.
Although the NNI reflects the overall distribution of brownfields in China, it cannot intuitively reflect the degree of agglomeration. Considering this, KDE was used to further measure six types of brownfield spatial agglomeration characteristics. As shown in Figure 3A, industrial brownfields were mainly concentrated in city clusters with strong industrial foundations, such as the Yangtze River Delta, Pearl River Delta, and Beijing-Tianjin-Hebei urban agglomerations, as well as other industrially developed provinces like Taiwan, Jiangsu, Guangdong, and Zhejiang. Industrial brownfields are widely distributed, regionally concentrated, and are characterized by a large abandoned area. Because deindustrialization and urbanization were higher than in other regions, they were found to be concentrated in the Eastern region. As shown in Figure 3B, high-density concentration areas of mining brownfields appeared in the Central Plains urban agglomerations, the Shandong Peninsula’s urban agglomerations, the urban agglomeration in the middle reaches of the Changjiang River, and the Southern industrial zone. Cities with a large number of mining brownfields included Datong, Jiaozuo, Huangshi, Tongchuan, Xuzhou, Fushun, and other resource-based cities. As shown in Figure 3C, transportation facilities brownfields were concentrated in coastal cities along the Yellow River, Yangtze River, Bohai Bay, and transportation hub cities, such as Zhengzhou, Shanghai, Wuhan, and Tianjin. They mainly include the Shanghai Railway Station, Shanghai-Nanjing Railway, Shanghai Whampoa Dock, Lanzhou-Lianyungang Railway extension, and the Beijing-Guangzhou Railway. As shown in Figure 3D and Figure 3E, public facilities and logistics and warehouse brownfields belong to basic service land with a low degree of abandonment, so the quantity is relatively small. Jiangsu and Shanghai have more public facilities brownfields, and Taiwan and Shanghai have more logistics and warehouse brownfields. As shown in Figure 3F, military brownfields accounted for a small proportion and had a scattered distribution in Sichuan, Chongqing, Shanxi, Gansu, and other remote mountainous areas that are far from cities.
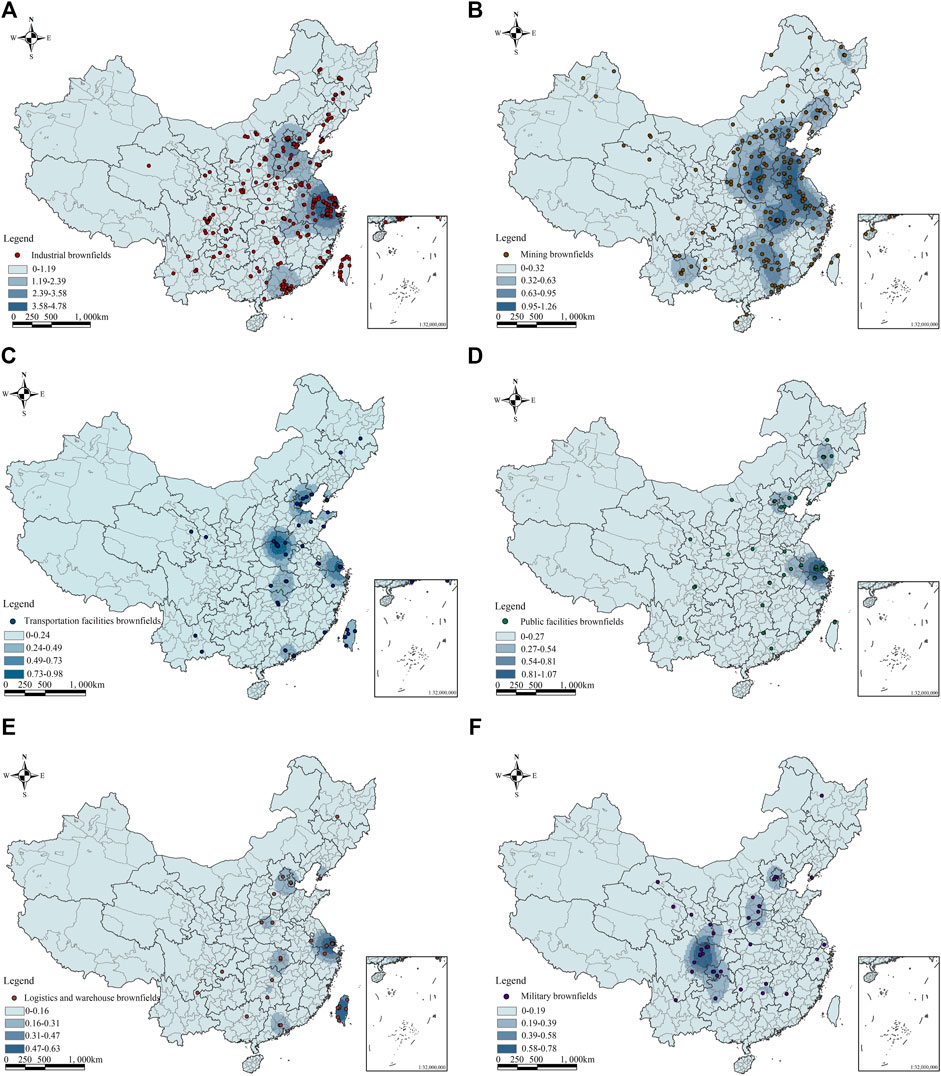
FIGURE 3. Kernel density estimation of six brownfield types in China. (A) Industrial brownfields. (B) Mining brownfields. (C) Transportation facilities brownfields. (D) Public facilities brownfields. (E) Logistics and warehouse brownfields. (F) Military brownfields.
Industrial brownfields are more concentrated in industrially developed cities, mining brownfields are concentrated in areas with rich mineral resources, transportation facilities brownfields are concentrated in cities along the river and coastal and transportation hubs, public facilities brownfields and logistics and warehouse brownfields have no obvious agglomeration rule, and military brownfields are concentrated far from central cities.
3.3 Formation Factors of Brownfields in China
Among the 471 basic documents searched, 252 records from 219 articles summarized the formation factors of brownfields. The frequency counting method was used to count the formation factors mentioned in the articles’ records. The factors can be classified into four categories: industrial structure adjustment, resource depletion, accelerated urbanization, and national policy orientation. Among the four types, industrial structure adjustment exhibits the highest frequency, followed by resource depletion, accelerated urbanization, and national policy orientation (see Table 2). Therefore, these four factors collaborate to produce brownfields, and their effects can be summarized as follows: industrial structure adjustment > resource depletion > urbanization process accelerated > national policy guidance.
To further demonstrate the correlation between brownfield spatial distributions and the factors that influence their formation, the distribution results were compared with the proportion of tertiary industries in China’s provinces (Long et al., 2012), 262 resource-dependent cities (GOSCPRC. General Office of the State Council of the People’s Republic of China, 2013; Feng et al., 2019), the level of urbanization in each province (Song et al., 2019), and the “Third-Front Movement” regions (Liu et al., 2014; Li H. C et al., 2019). Figure 4A compares brownfield locations with the proportion of tertiary industries in each province in 2018. Jiangsu, Guangdong, Zhejiang, Shandong, and Taiwan with a high proportion of tertiary industry also have a large number of brownfields. Figure 4B compares the brownfield locations of 262 resource-dependent cities. Mining brownfields were mostly located in resource-dependent cities, such as Huangshi, Tangshan, Jiaozuo, Fushun, Xuzhou, and Datong. Figure 4C compares those with provincial urbanization levels in 2018. Provinces with higher urbanization levels also had more brownfields. Figure 4D compares brownfield locations with the “Third-Front Movement” regions. Most military brownfields were concentrated in provinces with the “Third-Front Movement.”
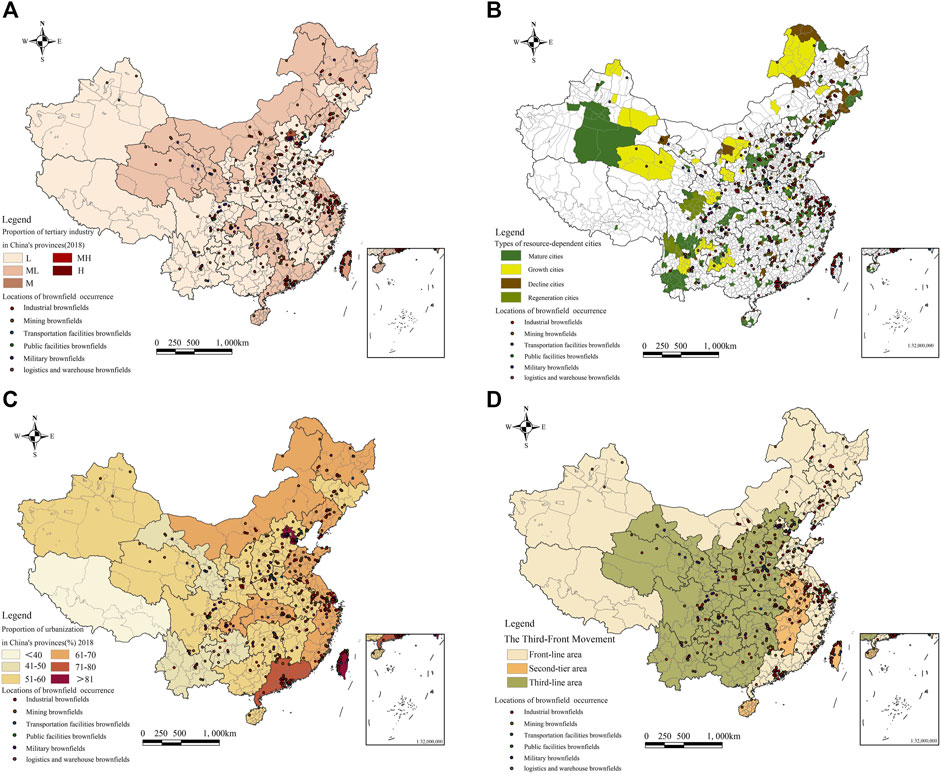
FIGURE 4. Brownfield records in different zones. (A) Geo-locations of brownfield records on the industrial structure map of China. (B) Geo-locations of brownfield records on the resource-dependent cities map of China. (C) Geo-locations of brownfield records on the degree of urbanization map of China. (D) Geo-locations of brownfield records on the Third-Front Movement map of China.
The production of brownfields in China is the result of a combination of many factors. A further comparison of formation factors and types revealed that the adjustment of the industrial structure and the acceleration of urbanization are common factors, namely, those of industrial and mining, public facilities, transportation facilities, and logistics and storage land. The depletion of resources has tended to aggravate the formation of mining brownfields, while the “Third-Front Movement” policy has mainly promoted the formation of military brownfields.
The adjustment of China’s industrial structure has constituted the dominant force in its economic growth over the past 40 years of reform and opening-up. With the acceleration of industrialization and the promotion of the policy of suppressing secondary industry and developing the tertiary sector, the latter occupies a dominant position in the industrial structure. The original urban land structure and industrial patterns are becoming increasingly less adaptable to the needs of China’s economic development (Lai et al., 2020). To adapt to this change, enterprises have closed down or relocated, and leftover industrial and mining industries, logistics and storage industries, and transportation facilities have been abandoned, forming brownfields.
With the acceleration of urbanization and the expansion of urban areas, industrial, mining, public facilities, transportation facilities, and logistics and warehouse enterprises that originally belonged to the suburbs have become part of the city, forcing original industrial enterprises to relocate. This led to the formation of brownfields in China.
Resource depletion factors are key drivers behind the formation of mining brownfields. Due to the exhaustion of resources, the single industrial structure, and serious environmental pollution, the resource industry has shrunk, and industrial efficiency has declined. Resource-dependent industries face transformations and closures, resulting in more mining brownfields.
The “Third-Front Movement” was a major strategic decision made by President Mao Zedong in the mid-1960s. It was intended to strengthen wartime preparations under an increasingly tense international situation as a strategic shift from East to West to gradually change the distribution of China’s productive forces. The emphasis was on construction in the Southwest and Northwest of the country (Naughton, 1988). Because most of the “Third-Front Movement” projects were “backed by the mountain, scattered, hidden,” with the economic recession, they had no advantageous location and difficulty in terms of access led to bankruptcy or relocation, forming military brownfields.
4 Discussion
4.1 Current Research Results
The results of this study revealed that there were more brownfields in the South than North, Northeast, and Midwest. Possible reasons for this are as follows. First, the highest percentage of brownfields in southern provinces was related to the high level of local economic development. There was a greater degree of openness and rapid economic development in the South, coupled with high urbanization, dense population, urban land expansion, and high demand for land, which caused more attention to be paid to abandoned brownfield sites. Second, despite the gap in economic development between the South and North, the transformation of industrial structure and resource-dependent cities also produced a large number of brownfields, especially old industrial and resource-dependent cities in the North (Xie et al., 2022). Third, as the earliest developed old industrial base in the country, the Northeast should have more brownfields, but there were only 83 records in this region. This may be because the regional economy’s relatively slow growth, transformation of its industrial structure, and large stock land resulted in a low demand for abandoned brownfield redevelopment. In addition, local scholars have done relatively little research on brownfields and the limited published documents may have led to a slight discrepancy between the recorded results and the actual situation in the Northeast. Fourth, there were fewer brownfield records in the Midwest provinces, mainly due to the small local population, relatively weak industrial foundation, and insufficient attention to brownfield research.
4.2 Comparison With Other Related Research Results
Compared to previous studies, brownfields in China are mainly concentrated in economically developed areas, near central urban areas, and along transportation routes such as railway hubs. This result is consistent with those of Lange and McNeil (2004), Longo and Campbell (2017), and Frantál et al. (2015), who analyzed the spatial distribution pattern of brownfields in the United States, the United Kingdom, and the Czech Republic, respectively. The brownfield spatial distributions of China were consistent with the results of the Survey Bulletin on Soil Pollution in China released by the State Council in 2014. The report highlighted that soil pollution is more serious in the South than in the North, and soil pollution problems in some regions, such as the Yangtze River Delta and the Pearl River Delta, are more prominent (MEP and MLR, 2014). The spatial distribution of soil pollution is consistent with the results of the current study. Aoki et al. (2018) established a database of known Chinese industrial heritage, and found that its distribution decreased from the Southeast coast to the West, mainly concentrated in the Yangtze River Delta Urban Agglomeration, Beijing-Tianjin-Hebei Urban Agglomeration, and Pearl River Delta Urban Agglomeration. In China, most industrial brownfields are rated industrial heritage due to their own value. The agglomeration results of industrial heritage were consistent with the concentrated industrial brownfield distribution pattern in this study. This study is not only consistent with the spatial distribution of soil pollution and industrial heritage; it is the first to describe the spatial distributions of brownfields in China. The methods and results lend credibility and practical significance to data and spatial distributions of brownfields in China and offer an effective empirical meta-analysis for brownfield studies.
4.3 Suggestions for the Government
China’s urban development is gradually entering the adjustment and transformation period from the stage of rapid expansion, and traditional urban development based on incremental planning is gradually shifting toward inventory planning. Urban land optimization and improvement are important issues. This study proposes the following policy recommendations:
First, a database for China’s brownfields should be established to enhance the openness and transparency of brownfield information. The availability of data is a major issue in China. It is necessary to construct a comprehensive database that includes the size, complexity, original use, ownership relations, and planned/allowed use of a site. To assist various levels of government, developers, research scholars, the public, and other groups of people in obtaining brownfield information, the government should establish a dedicated website for open brownfield databases.
Second, at the macro-level, it is necessary to formulate a targeted regional brownfield development direction strategy based on regional characteristics and brownfield types. The Eastern coastal areas, especially the Yangtze River Delta, Pearl River Delta, and Beijing-Tianjin-Hebei urban agglomerations, have more industrial brownfields, and there have been more successful transformation cases. In the future, the government should increase policies and preferential measures for brownfield redevelopment in these areas, and more attention should be paid to brownfield transformation models and later project supervision. Northern areas, especially Liaoning, Shanxi, Shandong, Henan, and other resource-dependent provinces, have more mining brownfields and are relatively far from urban areas. Brownfield reconstruction projects should be based on ecological restoration and prioritize the development of an ecological park model. A considerable amount of brownfield land remains in transition as an old industrial base in Northeast China. In the revitalization of the Northeast, emphasis should be placed on identifying and investigating existing industrial wastelands, encouraging investment in brownfield redevelopment, and academic research. There were relatively few brownfields in the Midwest. Attention should therefore be paid to their identification and restoration in this area. The Midwest is both densely and sparsely populated, and there is less demand for brownfield redevelopment. Restored brownfields can be used as reserve lands and will play a role in the new pattern of western development in the future. As different types of brownfields vary in terms of their location, area, nature, pollution degree, heritage value, and redevelopment mode, the government should formulate guidelines for the redevelopment of different types of brownfields to guide practice.
Third, local governments should coordinate and prioritize brownfield redevelopment. The Chinese government controls the primary market for land and should be the first promoter of brownfield reuse. Brownfield locations, pollution degree, and stock land should be combined to evaluate the reuse potential and availability of brownfields, redevelopment priorities, and transformation directions, and identify brownfield resources to return them to the market.
5 Conclusion
The spatial distributions and formation factors of brownfields can reveal the characteristics of the times behind China’s industrial development and the micro reconstruction process of industrial spaces within cities. It also helps coordinate land use conversion and guide redevelopment at the national level. We established a dataset of published brownfield records in China using a meta-analysis, which offered interesting conclusions despite their limited scope. Our results indicated that brownfield spatial distribution in China was basically consistent with the “Heihe-Tengchong Line” as the boundary, exhibiting a spatial pattern of “East-dense-West-sparse.” Industrial structure adjustments were the main and direct causes of brownfield production, while resource depletion and accelerated urbanization were secondary and indirect factors. National policy orientation played a background role. This can supplement research on brownfield redevelopment and land renovation, as well as provide a reference for decision-making related to urban vitality enhancements. This is in line with important demands for urban renewal and sustainable development.
As China’s central government attaches greater importance to brownfields, nationwide surveys of polluted land are gradually increasing. The state should increase investment in basic surveys, scientific research, funding, and development policies. Furthermore, brownfield sites should be identified using land contamination data, and land vacancy metrics and methods. In addition, the use of web crawler tools to supplement brownfield data retrieval from the internet, as well as an in-depth analysis of spatio-temporal dynamic features and landscape methods (Yang et al., 2016; Yang et al., 2019), will be the focus of further research. Although this study was conducted in the unique context of China, it is hoped that our research will also benefit other countries. For instance, in countries that have not established a brownfield database, existing brownfield-related data can be used to analyze spatial distributions to assist in the redevelopment of their brownfields.
Data Availability Statement
The raw data supporting the conclusions of this article will be made available by the authors, without undue reservation.
Author Contributions
Conceptualization, methodology, formal analysis, and writing—original draft preparation: XZ; software: XZ and SQ; validation: SQ and YS; investigation and visualization: SQ and DW; resources: SW and DW; data curation: SQ; writing—review and editing, and supervision: YS; and project administration and funding acquisition: YS and SW. All authors have read and agreed to the published version of the manuscript.
Funding
This research was funded by the National Social Science Fund of China (No. 17BJL051); the National Natural Science Foundation of China (No. 42171198); the Starting Research Fund of Yanshan University (No. 8190452); and the Innovation Development Strategy Research Project of Science and Technology Development Plan of Jilin Province.
Conflict of Interest
The authors declare that the research was conducted in the absence of any commercial or financial relationships that could be construed as a potential conflict of interest.
Publisher’s Note
All claims expressed in this article are solely those of the authors and do not necessarily represent those of their affiliated organizations, or those of the publisher, the editors, and the reviewers. Any product that may be evaluated in this article, or claim that may be made by its manufacturer, is not guaranteed or endorsed by the publisher.
Acknowledgments
The authors sincerely thank the reviewers for their valuable comments and suggestions to improve the quality of this paper.
References
Alker, S., Joy, V., Roberts, P., and Smith, N. (2000). The Definition of Brownfield. J. Environ. Plan. Manag. 43 (1), 49–69. doi:10.1080/09640560010766
Amekudzi, A., McNeil, S., and Koutsopoulos, H. N. (2003). Assessing Extrajurisdictional and Areawide Impacts of Clustered Brownfield Developments. J. Urban Plann. Dev. 129, 27–44. doi:10.1061/(asce)0733-9488(2003)129:1(27)
Aoki, N., Zhang, J. H., and Xu, S. (2018). Study on Construction and Application of Chinese Know Industrial Heritage Database. Archit. 4, 76–81. [in Chinese].
Bambra, C., Robertson, S., Kasim, A., Smith, J., Cairns-Nagi, J. M., Copeland, A., et al. (2014). Healthy Land? an Examination of the Area-Level Association between Brownfield Land and Morbidity and Mortality in England. Environ. Plan. A 46, 433–454. doi:10.1068/a46105
Bardos, P., Spencer, K. L., Ward, R. D., Maco, B. H., and Cundy, A. B. (2020). Integrated and Sustainable Management of Post-industrial Coasts. Front. Environ. Sci. 8, 1-14. doi:10.3389/fenvs.2020.00086
Boente, C., Albuquerque, M. T. D., Fernández-Braña, A., Gerassis, S., Sierra, C., and Gallego, J. R. (2018). Combining Raw and Compositional Data to Determine the Spatial Patterns of Potentially Toxic Elements in Soils. Sci. Total Environ. 631-632, 1117–1126. doi:10.1016/j.scitotenv.2018.03.048
Chaikumbung, M., Doucouliagos, H., and Scarborough, H. (2016). The Economic Value of Wetlands in Developing Countries: A Meta-Regression Analysis. Ecol. Econ. 124, 164–174. doi:10.1016/j.ecolecon.2016.01.022
Chen, W. Y., Li, X., and Hua, J. (2019). Environmental Amenities of Urban Rivers and Residential Property Values: A Global Meta-Analysis. Sci. Total Environ. 693, 133628. doi:10.1016/j.scitotenv.2019.133628
Chrysochoou, M., Brown, K., Dahal, G., Granda-Carvajal, C., Segerson, K., Garrick, N., et al. (2012). A GIS and Indexing Scheme to Screen Brownfields for Area-wide Redevelopment Planning. Landsc. Urban Plan. 105, 187–198. doi:10.1016/j.landurbplan.2011.12.010
Clark, P. J., and Evans, F. C. (1954). Distance to Nearest Neighbor as a Measure of Spatial Relationships in Populations. Ecology 35, 445–453. doi:10.2307/1931034
Coffin, S. L. (2003). Closing the Brownfield Information Gap: Some Practical Methods for Identifying Brownfields. Environ. Pract. 5, 34–39. doi:10.1017/s1466046603030126
CzechInvest (2021). Investment and Business Development Agency. Available at: (Accessed 20 April, 2021).
Eötvös, C. B., Magura, T., and Lövei, G. L. (2018). A Meta-Analysis Indicates Reduced Predation Pressure with Increasing Urbanization. Landsc. Urban Plan. 180, 54–59. doi:10.1016/j.landurbplan.2018.08.010
Feng, S., Hou, W., and Chang, J. (2019). Changing Coal Mining Brownfields into Green Infrastructure Based on Ecological Potential Assessment in Xuzhou, Eastern China. Sustainability 11, 2252. doi:10.3390/su11082252
Filip, S., and Cocean, P. (2012). Urban Industrial Brownfields: Constraints and Opportunities in Romania. Carpath. J. Earth. Env. 7, 155–164.
Frantál, B., Greer-Wootten, B., Klusáček, P., Krejčí, T., Kunc, J., and Martinát, S. (2015). Exploring Spatial Patterns of Urban Brownfields Regeneration: The Case of Brno, Czech Republic. Cities 44, 9–18. doi:10.1016/j.cities.2014.12.007
Franz, M., Pahlen, G., Nathanail, P., Okuniek, N., and Koj, A. (2006). Sustainable Development and Brownfield Regeneration.what Defines the Quality of Derelict Land Recycling? Environ. Sci. 3, 135–151. doi:10.1080/15693430600800873
Glass, G. V. (1976). Primary, Secondary, and Meta-Analysis of Research. Educ. Res. 5, 3–8. doi:10.3102/0013189x005010003
GOSCPRC. General Office of the State Council of the People’s Republic of China (2013-2020). The Sustainable Development Plan of Resource-dependent Cities Nationwide. Available at: http://www.gov.cn/zwgk/2013-12/03/content_2540070.htm (Accessed 13 March, 2021).
Hayek, M., Novak, M., Arku, G., and Gilliland, J. (2010). Mapping Industrial Legacies: Building a Comprehensive Brownfield Database in Geographic Information Systems. Plan. Pract. Res. 25, 461–475. doi:10.1080/02697459.2010.511018
Hipel, K. W., Hegazy, T., and Yousefi, S. (2010). Combined Strategic and Tactical Negotiation Methodology for Resolving Complex Brownfield Conflicts. Pesqui. Oper. 30, 281–304. doi:10.1590/S0101-74382010000200003
Howard, J. L., and Olszewska, D. (2011). Pedogenesis, Geochemical Forms of Heavy Metals, and Artifact Weathering in an Urban Soil Chronosequence, Detroit, Michigan. Environ. Pollut. 159, 754–761. doi:10.1016/j.envpol.2010.11.028
Kramářová, Z., and Juhásová Šenitková, I. (2018). Comparison of Informations from the Brownfield Catalog and the Data Needed to Evaluate Real Estate. EDP Sciences. MATEC Web of Confer. 146, 03016. doi:10.1051/matecconf/201814603016
Kunc, J., Martinát, S., Tonev, P., and Bohumil, F. (2014). Destiny of Urban Brownfields: Spatial Patterns Andperceived Consequences of Post-socialistic Deindustrialization. Transylv. Rev. Adm. Sci. 10, 109–128.
Lai, Y., Chen, K., Zhang, J., and Liu, F. (2020). Transformation of Industrial Land in Urban Renewal in Shenzhen, China. Land 9, 371. doi:10.3390/land9100371
Lange, D., and McNeil, S. (2004). Clean it and They Will Come? Defining Successful Brownfield Development. J. Urban Plann. Dev. 130, 101–108. doi:10.1061/(asce)0733-9488(2004)130:2(101)
Leigh, N. G., and Coffin, S. L. (2000). How Many Brownfields Are There? Building an Industrial Legacy Database. J. Urban Technol. 7, 1–18. doi:10.1080/10630730020021690
Li, H. C., Bai, W. L., Xiong, X., and Zhang, F. (2019). Exploration on “Ecological Restoration and Urban Renovation” in “Third-Front Movement” Industrial Cities—Take Jingmen of Hubei Province as an Example. Chin. Landsc. Archit. 35, 78–83. [in Chinese].
Li, J. M., Lu, D., Xu, C. D., Li, Y., and Chen, M. X. (2017). Spatial Heterogeneity and its Changes of Population on the Two Sides of Hu Line. Acta Geogr. Sin. 72, 148–160. [in Chinese]. doi:10.11821/dlxb201701012
Li, W., Wang, D., Li, H., Wang, J., Zhu, Y., and Yang, Y. (2019). Quantifying the Spatial Arrangement of Underutilized Land in a Rapidly Urbanized Rust Belt City: The Case of Changchun City-ScienceDirect. Land Use Policy 83, 113–123. doi:10.1016/j.landusepol.2019.01.032
Liu, C., Song, W., and Zhou, C. (2017). Unsuccessful Urban Governance of Brownfield Land Redevelopment: A Lesson from the Toxic Soil Event in Changzhou, China. Sustainability 9, 824. doi:10.3390/su9050824
Liu, Y., Van Oort, F., Geertman, S., and Lin, Y. (2014). Institutional Determinants of Brownfield Formation in Chinese Cities and Urban Villages. Habitat Int. 44, 72–78. doi:10.1016/j.habitatint.2014.05.005
Long, H., Li, Y., Liu, Y., Woods, M., and Zou, J. (2012). Accelerated Restructuring in Rural China Fueled by 'increasing vs. Decreasing Balance' Land-Use Policy for Dealing with Hollowed Villages. Land Use Policy 29, 11–22. doi:10.1016/j.landusepol.2011.04.003
Longo, A., and Campbell, D. (2017). The Determinants of Brownfields Redevelopment in England. Environ. Resour. Econ. 67, 261–283. doi:10.1007/s10640-015-9985-y
Loures, L., and Vaz, E. (2018). Exploring Expert Perception towards Brownfield Redevelopment Benefits According to Their Typology. Habitat Int. 72, 66–76. doi:10.1016/j.habitatint.2016.11.003
Mahzouni, A. (2018). Urban Brownfield Redevelopment and Energy Transition Pathways: A Review of Planning Policies and Practices in Freiburg. J. Clean. Prod. 195, 1476–1486. doi:10.1016/j.jclepro.2017.11.116
Martinat, S., Dvorak, P., Frantál, B., Klusacek, P., Kunc, J., Navratil, J., et al. (2016). Sustainable Urban Development in a City Affected by Heavy Industry and Mining? Case Study of Brownfields in Karvina, Czech Republic. J. Clean. Prod. 118, 78–87. doi:10.1016/j.jclepro.2016.01.029
M. Brander, L., J. Wagtendonk, A., S. Hussain, S., McVittie, A., Verburg, P. H., de Groot, R. S., et al. (2012). Ecosystem Service Values for Mangroves in Southeast Asia: A Meta-Analysis and Value Transfer Application. Ecosyst. Serv. 1, 62–69. doi:10.1016/j.ecoser.2012.06.003
MEP and MLR (2014). China Ministry of Environmental Protection, Ministry of Land and Resources.Survey Bulletin on Soil Pollution in China. Available at: http://www.gov.cn/foot/2014-04/17/content_2661768.htm (Accessed 21 December, 2021).
Modica, M. (2019). Industrial Brownfield Sites in the Alps. A First Quantitative Overview and Potential Implications for Regional Development. Rev. Geogr. Alp. 107, 1-17. doi:10.4000/rga.5274
Moher, D., Liberati, A., Tetzlaff, J., and Altman, D. G. (2009). Preferred Reporting Items for Systematic Reviews and Meta-Analyses: the PRISMA Statement. BMJ 339, b2535. doi:10.1136/bmj.b2535
Naughton, B. (1988). The Third Front: Defence Industrialization in the Chinese Interior. China Q. 115, 351–386. doi:10.1017/S030574100002748X
Newell, G., and McGreal, S. (2017). The Significance of Development Sites in Global Real Estate Transactions. Habitat Int. 66, 117–124. doi:10.1016/j.habitatint.2017.06.006
Niu, H. (2001). The Renovation and Redevelopment of ‘Brownfield’in USA. Urban Plan. Int. 30, 26–48. [in Chinese].
Novosák, J., Hájek, O., Nekolová, J., and Bednář, P. (2013). The Spatial Pattern of Brownfields and Characteristics of Redeveloped Sites in the Ostrava Metropolitan Area (Czech Republic). Morav. Geogr. Rep. 21, 36–45. doi:10.2478/mgr-2013-0009
Osman, R., Frantál, B., Klusáček, P., Kunc, J., and Martinát, S. (2015). Factors Affecting Brownfield Regeneration in Post-socialist Space: The Case of the Czech Republic. Land Use Policy 48, 309–316. doi:10.1016/j.landusepol.2015.06.003
Page, G. W., and Berger, R. S. (2006). Characteristics and Land Use of Contaminated Brownfield Properties in Voluntary Cleanup Agreement Programs. Land Use Policy 23, 551–559. doi:10.1016/j.landusepol.2005.08.003
Qi, W., Liu, S. H., and Zhao, M. F. (2015). Study on the Stability of Hu Line and Different Spatial Patterns of Population Growth on its Both Sides. Acta Geogr. Sin. 70, 551–566. [in Chinese]. doi:10.11821/dlxb201504004
Rall, E. L., and Haase, D. (2011). Creative Intervention in a Dynamic City: A Sustainability Assessment of an Interim Use Strategy for Brownfields in Leipzig, Germany. Landsc. Urban Plan. 100, 189–201. doi:10.1016/j.landurbplan.2010.12.004
Škrabal, J., Turečková, K., and Nevima, J. (2021). Analysis of Brownfields Sites in the Villages and Towns in the Czech Republic. Tras 64E, 151–169. doi:10.24193/tras.64E.8
Smoļakova, A. (2017). “Exploring Spatial Patterns of Urban Brownfields: the Case of Daugavpils City,” in Environment Technology Resources Proceedings of the 11th International Scientific and Practical Conference, Tomsk, Russia, 21-27 March 2005 1, 265–270. doi:10.17770/etr2017vol1.2589
Song, Y., Lyu, Y., Qian, S., Zhang, X., Lin, H., and Wang, S. (2022). Identifying Urban Candidate Brownfield Sites Using Multi-Source Data: The Case of Changchun City, China. Land Use Policy 117, 106084. doi:10.1016/j.landusepol.2022.106084
Song, Y., Zhang, X. J., Lyu, Y., Wang, S. J., and Lin, H. Y. (2019). Review and Prospects on Urban Brownfield Research from a Geographical Perspective. Sci. Geogr. Sin. 39, 886–897. [in Chinese]. doi:10.13249/j.cnki.sgs.2019.06.003
Vaidya, S. (2015). GIS Analysis of Brownfield Sites in the City of Minneapolis. Master’s Thesis. Mankato,USA: The Minnesota State University.
van Zanten, B. T., Verburg, P. H., Koetse, M. J., and van Beukering, P. J. H. (2014). Preferences for European Agrarian Landscapes: A Meta-Analysis of Case Studies. Landsc. Urban Plan. 132, 89–101. doi:10.1016/j.landurbplan.2014.08.012
Xie, D., Bai, C., and Xiao, W. (2022). Institutional Environment, Development Model Transformation and North-South Economic Disparity in China. Growth Change. doi:10.1111/grow.12629
Yang, J., Guo, A., Li, Y., Zhang, Y., and Li, X. (2019). Simulation of Landscape Spatial Layout Evolution in Rural-Urban Fringe Areas: a Case Study of Ganjingzi District. GIScience Remote Sens. 56, 388–405. doi:10.1080/15481603.2018.1533680
Yang, J., Su, J., Chen, F., Xie, P., and Ge, Q. (2016). A Local Land Use Competition Cellular Automata Model and its Application. Ijgi 5, 106. doi:10.3390/ijgi5070106
Zhang, G., Zheng, D., Tian, Y. Q., and Li, S. (2019). A Dataset of Distribution and Diversity of Ticks in China. Sci. Data 6, 105. doi:10.1038/s41597-019-0115-5
Keywords: brownfield, spatial distributions, formation factors, China, meta-analysis
Citation: Zhang X, Song Y, Qian S, Wang S and Wu D (2022) Exploring Spatial Distributions and Formation Factors of Brownfields in China: From Macro-Scales. Front. Environ. Sci. 10:918621. doi: 10.3389/fenvs.2022.918621
Received: 13 April 2022; Accepted: 24 May 2022;
Published: 04 July 2022.
Edited by:
Bing Xue, Institute of Applied Ecology (CAS), ChinaCopyright © 2022 Zhang, Song, Qian, Wang and Wu. This is an open-access article distributed under the terms of the Creative Commons Attribution License (CC BY). The use, distribution or reproduction in other forums is permitted, provided the original author(s) and the copyright owner(s) are credited and that the original publication in this journal is cited, in accordance with accepted academic practice. No use, distribution or reproduction is permitted which does not comply with these terms.
*Correspondence: Yang Song, song0317@nenu.edu.cn