- Department of Agricultural and Applied Economics, University of Georgia, Athens, GA, United States
Owing to farmers’ issues on accessibility and affordability of chemical fertilizers in Nepal, organic manure has been advocated as a more environmentally sustainable fertilizer input alternative. Using household survey data from Nepal, we analyze the adoption and productivity effects of improved organic manure practices. Our empirical strategy utilizes a recently developed control function approach in the endogenous switching regression model that allows for substantial heterogeneities accounting for potential self-selection problem due to observables and unobservables. Findings reinforce the crucial role of social capital as farm households’ adoption decisions are significantly influenced by 1) having adopter neighbors or friends, 2) residing in proximity to the market, and 3) gaining easy access to agricultural extension services. Beyond environmental and social considerations, this study establishes economic motivations for the adoption decision. The results show that the adoption of improved organic manure practices increased crop yield by 17 percentage points. These practices did not only result in significantly higher yields among adopting farms, but counterfactual analyses indicate that non-adopting farms would have realized much better yield conditions had they implemented the technology. The study concludes with implications for policies and initiatives to expand the adoption and impacts of improved organic manure practices in Nepal.
Introduction
In both developed and developing economies, new production technologies have been credited for realizing improvements in crop yields and food security conditions (Duan et al., 2016). Achieving higher agricultural productivity requires adopting modern technologies and inputs such as improved seeds and varieties, integrated pests, soil, fertilizer, and water management practices. Among production inputs, chemical fertilizer is the key to raising agricultural productivity and allows producers to generate a higher annualized rate of economic returns (Duflo et al., 2011).
In Nepal, overall chemical fertilizer use has been lower than its peer countries, owing to accessibility and affordability issues (World Bank, 2017). In the face of constant pressure for its agricultural industry to meet the economy’s increasing food and feed demands, the pressing challenge has been to find a viable fertilizer alternative to reconcile its low chemical fertilizer use. Improved organic manure practices such as improved farmyard manure (cattle shed improvement), compost and crop residue, and vermicompost could be alternatives to increase or maintain crop yield. Studies show that adopting these practices for certain growing conditions and management systems might close the yield gap with farms that only use chemical fertilizers (Seufert et al., 2012). Of the several manure alternatives, vermicompost has received significant attention in recent years due to increased awareness of sustainable organic agriculture and its economic and environmental benefits (Bajeli et al., 2016).
Vermicomposting involves feeding earthworms with biodegradable waste to convert them into slow-release organic manure (Yami et al., 2003; Joshi et al., 2015). Like any other organic fertilizer, vermicomposting offers a host of environmental benefits. It serves as a valuable resource to supply plant nutrients and refill the soil’s organic matter content, thereby maintaining soil fertility, soil organic carbon content, and crop yield (Vig et al., 2011; Sangeetha et al., 2012; Bajeli et al., 2016). It helps increase yield by providing better growth and reducing pest infestation (Joshi et al., 2015); it can be a substitute for chemical fertilizers (Bhat et al., 2018). In the light of today’s rapid urbanization and increased rural-urban migration trends, solid waste management issues have become an important concern in large urban areas. Incorporating earthworms in the composting process could somehow solve waste management and reduce environmental loads (Bhat et al., 2018). Realizing the benefits of vermicomposting, Nepal’s government launched its vermicompost production program in 2014. The program was designed to accelerate vermicompost technology diffusion and enhance vermicompost use (Amgai et al., 2017). Under the program, a subsidy is granted to any producer who constructed a vermicomposting bin on their farm. Around 25 organic fertilizer firms with 100600 Mton annual compost production capacity were established by 2016.
Despite the method’s apparent benefits and government support, its adoption rate has been slow. The adoption decision is usually weighed against two considerations: economic viability and environmental sustainability (Wang et al., 2018). Certain studies contend that a shift to organic farming methods usually involves a gestation phase in the adoption when the crop yield effect is initially slow and variable in the shorter-term horizon (Khaliq et al., 2006), and growing methods are usually more labor-intensive (Wang et al., 2016). However, proponents of organic agricultural systems contend that economic incentives cannot solely influence farmers’ adoption behavior as they also consider external environmental benefits accruing to the organic methods (Blackstock et al., 2010). Thus, it is imperative to identify and understand factors that affect the adoption of such a practice and its impacts on crop productivity to formulate more widespread adoption and diffusion policies. Only a few studies modeled yield response to vermicomposting in an experimental trial (for example, Panta and Yami, 2008). However, to the best of our knowledge, no study has empirically investigated the adoption of this practice and its impacts on crop yield using observational data.
This study fills such a research gap by analyzing farm household survey data from two districts of Nepal. This research has a two-fold objective: first, identifying principal determinants of adoption, and second, determining the effect of a household’s adoption decision on crop yield.1 Our analytical framework is designed to address several estimation issues. The adoption status of farmers is endogenous due to self-selection into a treatment, thus causing selection bias. Selection bias may arise because farmers who adopt improved practice may share some common unobserved characteristics, such as management abilities and knowledge and skills, which correlate with yield. On the other hand, adopters are more likely to be systematically different from non-adopters in observed and unobserved characteristics that could affect crop yield. Failure to account for selection bias arising from the endogenous treatment indicator capturing the adoption decision (labeled as “adoption” in our empirical model) may result in inconsistent estimation. Therefore, we explicitly consider the problem of non-random selection into treatment following the control function approach in the endogenous switching regression model (CF-ESR) proposed by Murtazashvili and Wooldridge (2016).
Under normality assumption, we estimate the selection corrected yield function using a two-step control function procedure. The first stage utilizes the probit model for the adoption decision. In the second stage, the selection correction term (generalized residual term) derived from the first stage is used as a control function. Finally, we build a counterfactual analysis and compare the expected crop yield under the actual and counterfactual conditions of adoption and non-adoption. We evaluate heterogeneous treatment effects of adoption decisions using estimates from the second step to identify the crop yield differences between adopters and non-adopters. Our preferred specification indicates that the adoption of improved practice is associated with an approximately 17 percent increase in crop productivity. The improved organic manure practice did not only result in significantly higher yields among adopting farms, but counterfactual analyses indicate that non-adopting farms would have realized much better yield conditions had they implemented the technology.
This study offers important empirical evidence relevant to ongoing discussions of the relative strengths of organic production methods. While the environmental benefits of manures eradicate such risks as soil deterioration, greenhouse emissions, and water contamination commonly associated with chemical fertilizer applications (Makkar et al., 2022), we explain its economic viability through yield potential. Findings offer important implications for Nepal’s and other developing countries’ farmers and policyholders who aspire to realize higher farm productivity and rural farm incomes that coincide with environmentally sustainable and sound growing practices.
Country and Industry Context
Agriculture plays a substantial role in Nepal’s economy. It accounts for a third of the nation’s GDP and two-thirds of its labor force. The sector’s performance is key to overall economic growth, poverty alleviation, and improving national productivity (MoAD, 2018). Notably, Nepal has made considerable progress toward overall poverty reduction and food security in recent years, yet uneven dispersion of economic activities and benefits results in the persistence of poverty and food insecurity conditions in its rural areas. Around 77% of the national population still earns less than $2 a day (World Bank, 2015).
Most Nepalese farmers are smaller holders, with nearly half of the farm households operating less than 0.5 hectares of land under an integrated farming arrangement. Farming is still predominantly for subsistence, and the adoption rate of advanced farm technology is marginal. The agricultural sector’s weak performance and slow growth rates (averaging 2.8% per year) have dragged down overall economic growth (World Bank, 2017), as agriculture is the country’s largest employer.
Chemical Fertilizer Use
Chemical fertilizer applications in Nepal increased substantially from 5.64 kg/ha in 2006 to 74.08 kg/ha in 2016. Despite such an upward trend in average fertilizer use, the country’s chemical fertilizer use is still much lower than the utilization rates in other South Asian countries (estimated around 160 kg/ha) (World Bank, 2017).
Several factors explain the relatively lower levels of chemical fertilizer use in Nepal. First, the Nepalese chemical fertilizer industry is still facing significant supply constraints. Market demand consistently exceeds supply; thus, the appropriate products are usually unavailable to the farmers. For instance, according to Kyle et al. (2017), only 25% of Nepalese households could purchase fertilizer in sufficient quantities. These individuals must walk quite a long distance and then wait in line to avail of even small fertilizer amounts. Second, farmers are not well-informed by technical advisers from the industry and other institutions about the proper choices for fertilizers appropriate to their respective farming situations. Third, low-income smallholder farmers typically face credit constraints as credit delivery systems in the country’s farm sector are often riddled with anomalous lending decisions. Specifically, allegations surface that loans for chemical fertilizers often go to politically connected people. Fourth, although Nepal’s government has set up price subsidies for chemical fertilizers designed to alleviate the farmers’ cost burden, market reality dictates that the resulting subsidized fertilizer price is still much higher than other countries’ prices, such as those in India. The government’s fertilizer price subsidy program has been criticized as “regressive.” Strong allegations contend that only wealthier farmers and those with more land often benefit most from the government’s price subsidies (Donovan, 2004). Besides, Nepal’s agro-climatic zones result in some heterogeneity in patterns of chemical fertilizer use among farmers. For example, the hilly regions representing more than half of total cultivated land only account for a fourth of total fertilizer use.
Methodology
Data Sources
The data set comes from a face-to-face household survey conducted from October to December 2015 in Chitwan and Lalitpur districts in Nepal’s central region. This region grows rice, maize, wheat, potato, and oilseeds, representing more than 90% of the total grain production and cultivated area (MoAD, 2018). Mixed cropping is the most common type of farming, and farmers grow two to three crops annually, depending upon whether the land is irrigated. Rice-wheat-maize or rice-vegetables-maize is the major cropping pattern in irrigated land, while the rice-wheat-fallow or rice-fallow-maize is the primary pattern in the rainfed condition (MoAD, 2018). In selecting survey households, we used a multi-level stratified sampling approach. We first identified the Chitwan and Lalitpur districts as the main improved organic manure practicing areas, given their high intensity of improved manure users. Afterward, we purposely selected four village development committees (VDCs)2 from each district with guidance from the District Agriculture Extension Officer.
The household survey was conducted in two stages. First, we did a reconnaissance survey to better understand the use, production, and crops grown in the survey areas. We also did in-depth key informant interviews with farmers, extension staff, and cooperatives officials. This first stage’s information was used to improve the survey instrument, identify the survey areas, and refine the sampling framework. We then randomly surveyed approximately 25–35 farm households from each VDC. The share of sample size allocated to each VDC was proportional to the number of adopters. Finally, a sample of 480 farm households (240 from each district), comprising of 200 non-adopters and 280 adopters, was chosen for the study. After employing further data cleaning protocols, the final sample size was reduced to 444 households. In this study, the primary variable of interest is a binary indicator of adoption, denoting whether a farm household produced and/or applied any of three improved organic manure practices: improved farmyard manure (FYM), vermicompost, and other composts, in their farms a year before the survey year. Non-adopters did not use any of the three improved organic manure practices to produce any of their crops.
The household survey questionnaires were structured and pre-tested. The survey provides comprehensive information about household demographic characteristics, farm activities, social networks, access to information, crop production details (input use, yield, and revenue), land and livestock ownership, organic manure use, preparation methods, and market information.
Estimation Strategy
A household’s decision to adopt the improved technology and its impacts on crop yield is modeled in a two-stage framework. The first stage captures the adoption decision. We model the adoption decision in a random utility framework under the assumption that a farmer decides whether to adopt this practice based on expected utility considerations. A farm household
where
In the second stage, we model the effect of technology adoption on crop productivity. Let
where
Several approaches have been proposed to address this estimation issue, such as 1) selection models (Heckman and Robb, 1986; Powell, 1994), 2) instrumental variable models (Heckman and Vytlacil, 2005; Heckman et al., 2006), and 3) matching methods (Heckman and Navarro-Lozano, 2004). To account for the endogeneity problem discussed above, we utilize the endogenous switching regression (ESR) model developed by Heckman (1976), where the coefficient on a binary endogenous treatment is allowed to differ across units in both observed and unobserved characteristics. The ESR can be estimated with the two-step method using a control function (CF) or simultaneously via full information maximum likelihood (FIML-ESR). The FIML-ESR implemented in Stata by Lokshin and Sajaia (2004) has increasingly been used in multiple studies to deal with self-selection in Eq. 1 technology adoption (Asfaw et al., 2012; Manda et al., 2019; Kassie et al., 2020), 2) cooperative membership (Ma and Abdulai, 2016), and 3) climate change adaptation (Di Falco et al., 2011; Di Falco and Veronesi, 2014; Dhakal et al., 2022). Maximum likelihood (ML) is a widely used technique with some limitations. The ML produces more efficient estimators and asymptotically correct standard error estimates under the appropriate assumption (Wooldridge, 2010). However, ML estimators tend to be more vulnerable to misspecification (Greene, 2007, p.421). Under certain circumstances, such as dealing with a small sample size, the ML estimation can be computationally complicated and costly to implement, thus limiting its use (Nguimkeu et al., 2019). For instance, correlations between the outcomes equation and selection equation errors might not be strongly identified, resulting in multiple local maxima or, at times, leading to non-convergence problems.
In contrast, the two-step method always results in convergence. The CF approach, combined with instrumental variables, produces consistent estimation in the presence of endogenous regressors under standard identification assumptions (Wooldridge 2015). It considers the non-linear interaction between an endogenous regressor and the error terms. Besides, unlike the FIML-ESR, the CF-ESR approach provides a direct marginal effect of endogenous treatment on outcomes and consistent estimates in the presence of unobserved heterogeneity between adopters and non-adopters (Murtazashvili and Wooldridge, 2016). Considering these issues, we employ the CF-ESR model proposed by Murtazashvili and Wooldridge (2016)3.
Consider the following ESR model
where
where
were
Control Function Approach
The control function approach involves two-step estimation procedures. The first step involves the estimation of the probit model of
where
In order to achieve identification, we should satisfy the exclusion restriction that requires at least one excluded exogenous variable
This approach also requires evidence of
In the second step, generalized residuals are obtained as
where
Subsequently, our preferred estimating equation would be
which is estimated by two-stage least squares using instrumental variables
In the final step, Eq. 10 is estimated separately when
Heterogeneity Analysis
This study investigates the treatment effects of improved organic manure practice adoption on crop productivity. The three most used treatment parameters to explore the impact of adoption in the program evaluation literature are the average treatment effect (ATE), the average treatment effect on treated (ATT), and the average treatment effect on untreated (ATU). The ATE measure would be the average outcome if individuals were randomly assigned to treatment, and ATT measures the average effects on individuals specifically assigned to treatment. ATT then is the appropriate parameter to identify the impact of adoption on adopting households. If, however, the interest focuses on the impact of adoption on households of a certain type as if they were randomly selected, then ATE is the parameter of interest to recover. The ATE, ATT, and ATU measures are defined using conventions introduced by Wooldridge (2015), as follows:
From Eq 11, 12
The
The effect of adoption on the adopting farm household (i.e., ATT) is given by
The
On the other hand, the effect of adoption on the non-adopting households (ATU) is given by
The
Results
We first report summary statistics of variables used in our analysis. We then estimate Eq. 10 for the full sample and Eq. 11 and Eq. 12 for adopting and non-adopting households, respectively, to examine the extent to which the estimated effect is heterogeneous across adoption status.
Summary Statistics
Figure 1 presents the distribution of crop yield by adoption status. As the plots indicate, adopters have higher crop productivity when the distribution shift to the right than non-adopters.
Table 1 presents the summary statistics and statistical significance tests on equality of means and proportions for continuous and dummy variables, respectively, among adopters and non-adopters. This study’s choices of dependent variables are drawn from approaches employed in the existing literature on technology adoption and crop yield (Kassie et al., 2015; Manda et al., 2019; Kassie et al., 2020, for example). This study’s analytical model includes household characteristics (such as sex, age, educational attainment, household size, and social networks), membership in cooperatives and agricultural groups, access to credit and training, wealth indicators (in terms of land holdings and livestock ownership), and production input costs (manure and fertilizers, labor, irrigation and machinery, seeds, and planting) to control for the observed heterogeneity between adopters and non-adopters. We hypothesize that household characteristics and input costs affect farmers’ adoption decisions and crop productivity levels. In this study’s sample, around 60% of the households adopt improved practices to produce crops (Table 1).
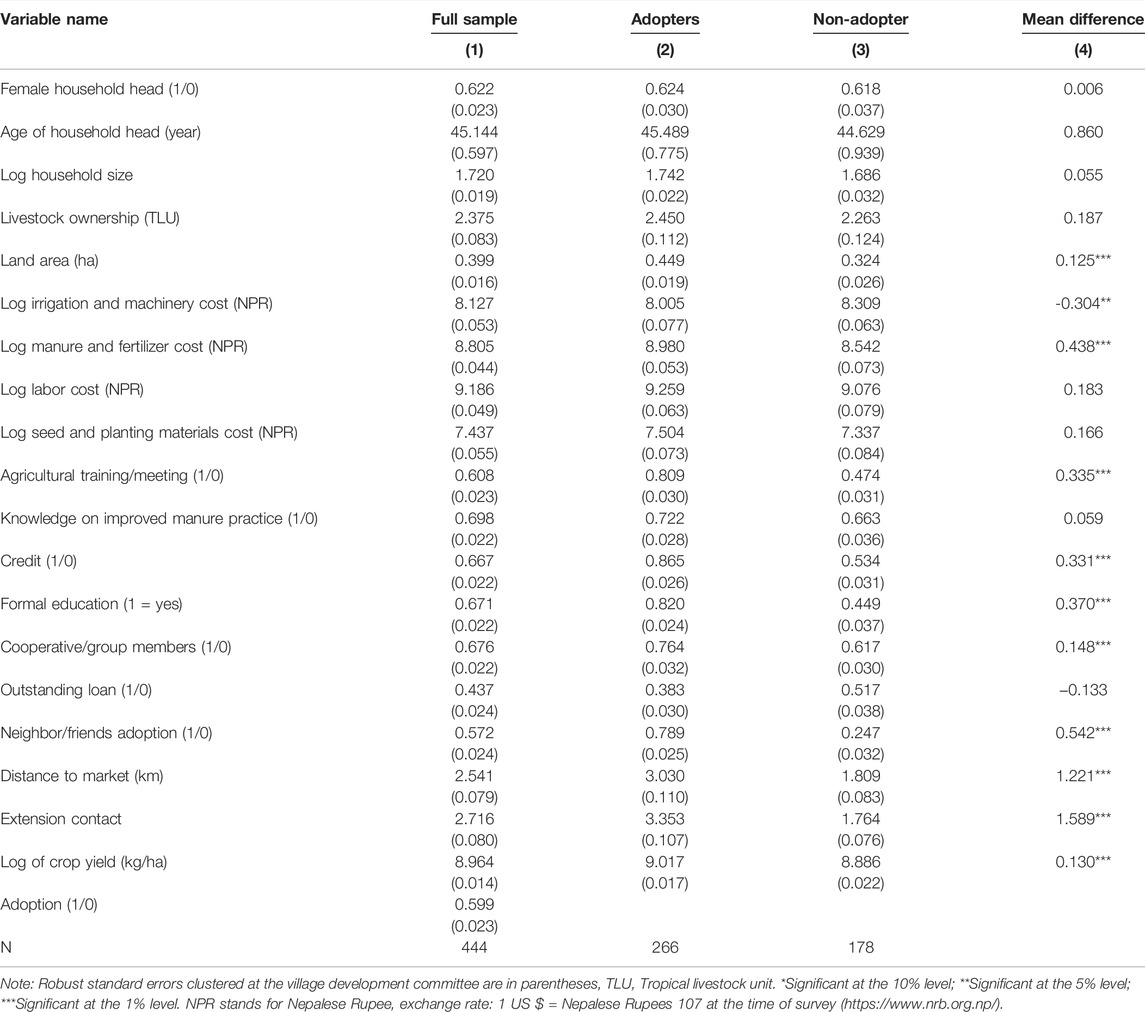
TABLE 1. Descriptive statistics and mean differences in variables between adopters and non-adopters.
The average age of the sample household head is 41 years, whereas 62% are female-headed. There is no significant difference in the observed age and gender of the household head. The average livestock ownership measured in tropical livestock units (LTU4) is 2.38. Household size and livestock numbers between adopting and non-adopting households are not significantly different. The mean cultivated land area (owned) of sample households is 0.399 ha, and the average adopters’ cultivated land area is significantly larger than that for non-adopters. We find statistically significant differences between adopters and non-adopters for most of the other explanatory variables. In general, adopters are more likely to be educated, trained, and cooperative or group members. Moreover, they have better access to credit, extension services, and marketing centers. In terms of input costs (control variables), adopters tend to spend significantly more on manure and fertilizers, while non-adopters are more likely to spend more on irrigation and machinery. Adopting households also register significantly higher crop productivity levels compared to non-adopting households. The summary statistics suggest that adopters are largely positively self-selected, and the impact of improved manure practices on yield seems to be positive.
Determinants of Adoption
Data columns 1 and 2 in Table 2 present the first stage-probit estimates and their marginal effects on adopters and non-adopters. The estimated coefficients help find factors that significantly influence the farm household’s decision to adopt. However, the probit model’s estimated coefficients are not directly interpretable; therefore, marginal effects are evaluated to quantify the marginal change in the probability of adoption with a unit change in each response variable.
Results show that most of the covariates hypothesized to influence adoption decisions register the expected results. Five sources of heterogeneity largely explain the households’ decision to adopt. These include demographics (age and sex), sources of information (access to extension services, improved skill, knowledge, and training), social and human capital (access to credit, cooperative/group membership, and educational attainment), location, and self-reported adoption status of neighbors/friends. The resulting coefficient estimates identify three main factors that determine the farm household’s decision to adopt practices: 1) having adopter neighbors/friends, 2) residing in proximity to the market, and 3) gaining easy access to extension services. These variables appear to pave the way for the wider and more effective dissemination of relevant information for the adopting household’s assimilation of the improved technology. For instance, households residing near the market would have more information on improved organic manure practices and are 20% more likely to adopt the technology. Households also receive information about improved technologies and inputs from university extension services and their neighbors/friends, which altogether play a vital role in influencing decisions on technology adoption. The findings indicate that having access to extension services and being in adopters’ neighborhoods can reduce farm households’ constraints on adopting the technology and increase the likelihood of adoption by 6% and 56%, respectively. This result is consistent with the findings of other studies on technology adoption that document how farmers learn about how innovative technology from their neighbors/friends on social networks (Conley and Udry, 2010). Empirical evidence indicates that most information on input use come from neighbors/friends and extension agents (Asfaw et al., 2012; Krishnan and Patnam, 2014; Wossen et al., 2019). Households may also acquire knowledge and information about improved manure practices by participating in cooperatives or agricultural groups. Results show that membership in cooperatives/groups is positive and significant, indicating that farm households are around 27% more likely to adopt improved technology than non-members. This result conforms with the findings of previous adoption studies (Ma and Abdulai, 2016; Wossen et al., 2017). Similarly, education, access to credit, training, and knowledge about improved manure practices are positively and significantly associated with the adoption. Our findings thus lend support to previously validated positive effects of education (Huffman, 2020; Mukherjee, 2020), access to credit (Croppenstedt et al., 2003; Barrett et al., 2004; Mukherjee, 2020), and training (Nakano et al., 2018; Kumar et al., 2020). However, the estimated coefficient of the female household head dummy is negative and significant, thus suggesting that women are about 33% less likely to adopt than their male counterparts. Some studies attribute this trend to women’s usual lack of access to resources, extension information, infrastructure, education, and credit services (Satyavathi et al., 2010; Rola-Rubzen et al., 2020).
Validity of Instruments
Instrumental variables (IVs) are expected to significantly affect technology adoption. Living in closer proximity to the market, having frequent contact with extension staff, and having neighbors or friend adopters increase the probability of adoption. These instrument variables provide information about the benefit of using compost and increase the likelihood of adoption.
The confirmation of the validity of this study’s instruments is presented in Supplementary Appendix SA1. As noted by Bound et al. (1995), the “cure can be worse than the disease” when the excluded instruments are only weakly correlated with the endogenous variables. Weak IVs can lead to inconsistent and unreliable estimates. To test the relevancy condition (to identify weak instruments), we regress the binary adoption variable on a set of excluded instruments. The result shows that instruments are jointly significant for explaining the adoption decision (χ2 = 235.43; p < 0.001 for the probit model and F-statistics = 113.08; p < 0.001 for the linear probability model). More importantly, Cragg-Donald’s F-test statistics are large enough to reject the hypothesis that the excluded variables are weak instruments (see for details, Stock and Yogo, 2005).
Further, in order for the model to be identified, exclusion restrictions need to be enforced, whereby instruments must be highly correlated with the endogenous binary adoption decisions but not directly correlated with crop productivity. In other words, the IVs’ impact on crop yield should come only through adoption decisions. However, the exclusion restriction can be violated if households learn productivity-improving methods from friends and neighbors. Intuitively, we argue that farm households are less likely to know about friends’ and neighbors’ unobserved characteristics. Moreover, the distance variables are random from the household location after controlling for other household characteristics.
We also carried out the “falsification test” (Di Falco et al., 2011) to test the validity of IVs. In this test, the crop yield among households that did not adopt is regressed (data column 2 of Supplementary Appendix SA1) on all IVs. The results support exclusion restriction as none of these IVs are significant.
Impacts of Improved Manure Practice Adoption on Crop Yield
The OLS estimates of the yield functions, controlling for various observables and inputs, are in column 3 of Table 2. The parameter estimates suggest a positive and significant effect of improved organic manure practice use on crop yield. The OLS results show households adopting improved technology have 11% higher crop yield5 than non-adopting households after controlling for observed characteristics. However, the OLS estimates assume that adoption is exogenously determined while potentially endogenous due to sample selection. This approach does not consider the unobservable, such as innate ability and skills that are likely correlated with the adoption decisions and crop productivity, thus leading to inconsistent estimates.
Column 4 in Table 2 presents the results of the CF-ESR setting. A notable result is the sign and significance of the coefficient of the generalized residual
These findings justify the use of the CF-ESR to account for selection bias to obtain consistent estimates. The two-step control function approach uses the two-step estimation procedure to address observed and unobserved heterogeneities between adopters and non-adopters. Our preferred specification suggests a positive and significant return to improved manure practice; adopting the technology leads to a 17% higher return in crop yield after controlling for confoundings. As such, sample selection results in an attenuation bias as the crop productivity impact of adoption is 6% higher when using a two-step control function approach than when using OLS. In the absence of sample selection, parameter estimates from OLS and the two-step models should be the same. The differential productivity impacts of adoption estimated with two approaches indicate substantial unobserved heterogeneities between adopters and non-adopters. Consistent with economic theory, input costs such as irrigation and machinery, fertilizers, and manures are significantly associated with increased crop yield per hectare.
The land area’s estimated coefficient is negative and statistically significant, suggesting that productivity negatively correlates with cultivated land area. More specifically, the result indicates that a 1% increase in the cultivated land area decreases rice yield by 3%.6 The inverse relationship between farm size and crop productivity is consistent with many existing studies (Huang et al., 2015) that small-sized farms are more productive.
We also report the estimated coefficients of the crop productivity equations for adopters and non-adopters separately (Supplementary Appendix SA2). The results show a substantial difference in yield equation coefficients between adopters and non-adopters. The difference in the estimated coefficients of covariates in the adopters and non-adopters equations supports heterogeneity across adopting and non-adopting households.
Heterogeneous Treatment Effects
Table 3 reports the expected productivity under actual and counterfactual conditions for adopters and non-adopters. The results show that adoption significantly increases crop productivity. The average predicted crop productivity of adopting farm households is about 8,764 kg/ha. In comparison, it is about 7,482 kg/ha for non-adopting farm households indicating an average yield advantage (LATE) of 1,282 kg/ha (around 17%). Specifically, farm households that did not adopt the technology would have increased their crop yield by 1,448 kg/ha (20% higher yield return) had they adopted the improved practice. In another counterfactual case, adopters would have produced about 1,171 kg/ha (14% less) if they had not adopted.
Similarly, yield improvement from adoption would have been significantly higher (277 kg/ha) for non-adopters than adopters had both groups adopted the technology; that is, the effect is bigger for non-adopting households with respect to adopting households. The positive and significant effects of technology adoption on crop productivity support the results of previous studies (Moyo et al., 2007; Kumar et al., 2020). Similarly, significant heterogeneous treatment effects offer other unobservable factors that make adopters systematically better producers than non-adopters, consistent with technology adoption literature (Abdulai and Huffman, 2014; Wossen et al., 2017). These findings indicate many opportunities for farmers to realize higher productivity by simply using these techniques.
Robustness Check
We checked our findings’ validity by comparing the results from treatment effects models and propensity score matching (PSM). We also use the treatment effect model using Stata’s canned routine for the comparison of our findings. The estimated coefficients and treatment effects (both ATT and ATE) are consistent with estimated parameters with CF-ESR (Supplementary Appendix SA3). We used the nearest neighbor matching (NNM) estimator for the propensity score matching. The NMM approach takes each adopter and searches for the non-adopter with the closest propensity score, and all adopters find a match with non-adopters (Caliendo and Kopeinig, 2008). The matched comparison group is understood to be counterfactual. The PSM method’s average treatment effect is positive and significant, indicating higher productivity impacts of adoption. However, the ATE under this approach is slightly lower than that estimated by the CF-ESR (11% vs. 17%). The results suggest that inability to control for unobservable results in underestimating ATEs in the PSM. The PSM balances the observed distribution of covariates between adopters and non-adopters and accounts for only observed heterogeneity, while CF-ESR accounts for both observables and non-observables. These findings align with earlier studies suggesting the PSM’s shortcomings in technology adoption literature (Andam et al., 2008; Shahzad and Abdulai, 2020).
Conclusion and Policy Implications
This article offers empirical support to the growing literature on the adoption behavior of farmers in developing economies. Its focus on improved organic manure practices helps solidify arguments for the greater relevance of organic production methods in general. Under this expanded perspective, this study reinforces the usual environmental, social, and economic motivations that may influence farmers’ adoption decisions.
As the organic manure alternative weans, farm businesses from dependence on chemical production inputs, apparent gains in environmental health and sustainability can strongly influence farmers’ adoption behavior. Although not directly validated in this analysis, this underlying motivation is supplemented by social and economic arguments. In this study, the crucial role of social capital in adoption decisions is evident. More than just a validation of farmers’ “free-riding behavior” where earlier adopters among peer farmers can help induce adoption decisions, the social dimension of adoption can reflect information sharing and collective empirical learning modes that socially cohesive and cooperative rural community systems in developing countries usually subscribe to. This contention is easily bolstered by the success of earlier rural development efforts that capitalized on the communities’ pervading cultural norms and social networks. A case in point is the microfinance paradigm that confirms the sustained effectiveness of the group lending implemented for many decades now in many rural communities, especially in South Asia, that has effectively bolster poverty alleviation goals.
Beyond environmental and social considerations, this study also establishes economic motivations for adopting such alternative technology. Improved organic manure practice has not only resulted in significantly higher yields among adopting farms, but results of counterfactual analyses show that non-adopting farms would have realized much better yield conditions had they implemented the technology.
As Nepal’s government continues to promote improved organic manure practice as a more affordable, practical production input, this study lays out encouraging parameters that prospective adopters may factor into their decisions. The composite effects of an alternative technology that reaps environmental, social, and economic benefits can only translate to a more sustainable farm economy, especially in a country like Nepal.
Data Availability Statement
The raw data supporting the conclusion of this article will be made available by the authors, without undue reservation.
Author Contributions
CD: Conceptualization; Methodology; Project administration; Writing-original draft; Writing-review and editing. CE: Project administration; Resources; Supervision; Writing-original draft; Writing-review and editing.
Conflict of Interest
The authors declare that the research was conducted in the absence of any commercial or financial relationships that could be construed as a potential conflict of interest.
Publisher’s Note
All claims expressed in this article are solely those of the authors and do not necessarily represent those of their affiliated organizations, or those of the publisher, the editors and the reviewers. Any product that may be evaluated in this article, or claim that may be made by its manufacturer, is not guaranteed or endorsed by the publisher.
Supplementary Material
The Supplementary Material for this article can be found online at: https://www.frontiersin.org/articles/10.3389/fenvs.2022.912860/full#supplementary-material
Footnotes
1Our measure of crop yield is productivity, which is defined as the total crop harvested per hectare of cropland. In this article, productivity and yield are used interchangeably.
2VDC were the smallest administrative unit of Nepal during the household survey.
3Unlike Murtazashvili and Wooldridge (2016), we assume all covariates (except a binary treatment status adaptation) in the ESR model to be exogenous.
4TLU = =0.7*(Buffalo + +Cattle)+ 0.1*Goat+0.2*Pig+0.01*Poultry.
5As dependent variable is expressed in logarithm the returns are calculated as
6Since land area is in hectare, we report the elasticity at means as
References
Abadie, A., and Cattaneo, M. D. (2018). Econometric Methods for Program Evaluation. Annu. Rev. Econ. 10, 465–503. doi:10.1146/annurev-economics-080217-053402
Abdulai, A., and Huffman, W. (2014). The Adoption and Impact of Soil and Water Conservation Technology: An Endogenous Switching Regression Application. Land Econ. 90 (1), 26–43. doi:10.3368/le.90.1.26
Amgai, S., Paudel, S. R., Bista, D. R., and Poudel, S. R. (2017). Government Intervention on Organic Fertilizer Promotion: a Key to Enhancing Soil Health and the Environment. J. Agric. Environ. 18, 131–139. doi:10.3126/aej.v18i0.19898
Andam, K. S., Ferraro, P. J., Pfaff, A., Sanchez-Azofeifa, G. A., and Robalino, J. A. (2008). Measuring the Effectiveness of Protected Area Networks in Reducing Deforestation. Proc. Natl. Acad. Sci. U.S.A. 105 (42), 16089–16094. doi:10.1073/pnas.0800437105
Asfaw, S., Shiferaw, B., Simtowe, F., and Lipper, L. (2012). Impact of Modern Agricultural Technologies on Smallholder Welfare: Evidence from Tanzania and Ethiopia. Food policy 37 (3), 283–295. doi:10.1016/j.foodpol.2012.02.013
Bajeli, J., Tripathi, S., Kumar, A., Tripathi, A., and Upadhyay, R. K. (2016). Organic Manures a Convincing Source for Quality Production of Japanese Mint (Mentha Arvensis L.). Industrial Crops Prod. 83, 603–606. doi:10.1016/j.indcrop.2015.12.064
Barrett, C. B., Moser, C. M., McHugh, O. V., and Barison, J. (2004). Better Technology, Better Plots, or Better Farmers? Identifying Changes in Productivity and Risk Among Malagasy Rice Farmers. Am. J. Agric. Econ. 86 (4), 869–888. doi:10.1111/j.0002-9092.2004.00640.x
Bhat, S. A., Singh, S., Singh, J., Kumar, S., Bhawana, A. P., and Vig, A. P. (2018). Bioremediation and Detoxification of Industrial Wastes by Earthworms: Vermicompost as Powerful Crop Nutrient in Sustainable Agriculture. Bioresour. Technol. 252, 172–179. doi:10.1016/j.biortech.2018.01.003
Blackstock, K. L., Ingram, J., Burton, R., Brown, K. M., and Slee, B. (2010). Understanding and Influencing Behaviour Change by Farmers to Improve Water Quality. Sci. total Environ. 408 (23), 5631–5638. doi:10.1016/j.scitotenv.2009.04.029
Blundell, R., and Costa Dias, M. (2009). Alternative Approaches to Evaluation in Empirical Microeconomics. J. Hum. Resour. 44 (3), 565–640. doi:10.1353/jhr.2009.0009
Bound, J., Jaeger, D. A., and Baker, R. M. (1995). Problems with Instrumental Variables Estimation when the Correlation between the Instruments and the Endogenous Explanatory Variable Is Weak. J. Am. Stat. Assoc. 90 (430), 443–450. doi:10.1080/01621459.1995.10476536
Caliendo, M., and Kopeinig, S. (2008). Some Practical Guidance for the Implementation of Propensity Score Matching. J. Econ. Surv. 22 (1), 31–72. doi:10.1111/j.1467-6419.2007.00527.x
Conley, T. G., and Udry, C. R. (2010). Learning about a New Technology: Pineapple in Ghana. Am. Econ. Rev. 100 (1), 35–69. doi:10.1257/aer.100.1.35
Croppenstedt, A., Demeke, M., and Meschi, M. M. (2003). Technology Adoption in the Presence of Constraints: the Case of Fertilizer Demand in Ethiopia. Rev. Dev. Econ. 7 (1), 58–70. doi:10.1111/1467-9361.00175
Dhakal, C., Khadka, S., Park, C., and Escalante, C. L. (2022). Farm Income and Revenue Risk Exposure Effects of Climate Change Adaptation in Nepal: Control Function Approach in the Endogenous Switching Regression Model. Ecol. Econ.
Di Falco, S., and Veronesi, M. (2014). Managing Environmental Risk in Presence of Climate Change: the Role of Adaptation in the Nile Basin of Ethiopia. Environ. Resour. Econ. 57 (4), 553–577. doi:10.1007/s10640-013-9696-1
Di Falco, S., Veronesi, M., and Yesuf, M. (2011). Does Adaptation to Climate Change Provide Food Security? A Micro‐Perspective from Ethiopia. Am. J. Agric. Econ. 93 (3), 829–846. doi:10.1093/ajae/aar006
Duan, Y., Xu, M., Gao, S., Liu, H., Huang, S., and Wang, B. (2016). Long-term Incorporation of Manure with Chemical Fertilizers Reduced Total Nitrogen Loss in Rain-Fed Cropping Systems. Sci. Rep. 6 (1), 1–10. doi:10.1038/srep33611
Duflo, E., Kremer, M., and Robinson, J. (2011). Nudging Farmers to Use Fertilizer: Theory and Experimental Evidence from Kenya. Am. Econ. Rev. 101 (6), 2350–2390. doi:10.1257/aer.101.6.2350
Heckman, J. J., and Robb, R. (1986). “Alternative Methods for Solving the Problem of Selection Bias in Evaluating the Impact of Treatments on Outcomes,” in Drawing Inferences from Self-Selected Samples (New York, NY: Springer), 63–107. doi:10.1007/978-1-4612-4976-4_7
Heckman, J. J. (1976). The Common Structure of Statistical Models of Truncation, Sample Selection and Limited Dependent Variables and a Simple Estimator for Such Models. Ann. Econ. Soc. Meas. 5, 475–492.
Heckman, J. J., Urzua, S., and Vytlacil, E. (2006). Understanding Instrumental Variables in Models with Essential Heterogeneity. Rev. Econ. Statistics 88 (3), 389–432. doi:10.1162/rest.88.3.389
Heckman, J. J., and Vytlacil, E. (2005). Structural Equations, Treatment Effects, and Econometric Policy Evaluation1. Econometrica 73 (3), 669–738. doi:10.1111/j.1468-0262.2005.00594.x
Heckman, J., and Navarro-Lozano, S. (2004). Using Matching, Instrumental Variables, and Control Functions to Estimate Economic Choice Models. Rev. Econ. statistics 86 (1), 30–57. doi:10.1162/003465304323023660
Huang, J., Wang, Y., and Wang, J. (2015). Farmers' Adaptation to Extreme Weather Events through Farm Management and its Impacts on the Mean and Risk of Rice Yield in China. Am. J. Agric. Econ. 97 (2), 602–617. doi:10.1093/ajae/aav005
Huffman, W. E. (2020). Human Capital and Adoption of Innovations: Policy Implications. Appl. Econ. Perspect. Policy 42 (1), 92–99. doi:10.1002/aepp.13010
Joshi, R., Singh, J., and Vig, A. P. (2015). Vermicompost as an Effective Organic Fertilizer and Biocontrol Agent: Effect on Growth, Yield and Quality of Plants. Rev. Environ. Sci. Biotechnol. 14 (1), 137–159. doi:10.1007/s11157-014-9347-1
Kassie, M., Fisher, M., Muricho, G., and Diiro, G. (2020). Women's Empowerment Boosts the Gains in Dietary Diversity from Agricultural Technology Adoption in Rural Kenya. Food Policy 95, 101957. doi:10.1016/j.foodpol.2020.101957
Kassie, M., Teklewold, H., Marenya, P., Jaleta, M., and Erenstein, O. (2015). Production Risks and Food Security under Alternative Technology Choices in Malawi: Application of a Multinomial Endogenous Switching Regression. J. Agric. Econ. 66 (3), 640–659. doi:10.1111/1477-9552.12099
Khaliq, A., Abbasi, M., and Hussain, T. (2006). Effects of Integrated Use of Organic and Inorganic Nutrient Sources with Effective Microorganisms (EM) on Seed Cotton Yield in Pakistan. Bioresour. Technol. 97 (8), 967–972. doi:10.1016/j.biortech.2005.05.002
Krishnan, P., and Patnam, M. (2014). Neighbors and Extension Agents in Ethiopia: Who Matters More for Technology Adoption? Am. J. Agric. Econ. 96 (1), 308–327. doi:10.1093/ajae/aat017
Kumar, A., Takeshima, H., Thapa, G., Adhikari, N., Saroj, S., Karkee, M., et al. (2020). Adoption and Diffusion of Improved Technologies and Production Practices in Agriculture: Insights from a Donor-Led Intervention in Nepal. Land Use Policy 95, 104621. doi:10.1016/j.landusepol.2020.104621
Kyle, J., Resnick, D., and Karkee, M. (2017). Improving the Equity and Effectiveness of Nepal’s Fertilizer Subsidy Program. Washington, DC: Intl Food Policy Res Inst.
Lokshin, M., and Sajaia, Z. (2004). Maximum Likelihood Estimation of Endogenous Switching Regression Models. Stata J. 4 (3), 282–289. doi:10.1177/1536867x0400400306
Ma, W., and Abdulai, A. (2016). Does Cooperative Membership Improve Household Welfare? Evidence from Apple Farmers in China. Food Policy 58, 94–102. doi:10.1016/j.foodpol.2015.12.002
Makkar, C., Singh, J., Parkash, C., Singh, S., Vig, A. P., and Dhaliwal, S. S. (2022). Vermicompost Acts as Bio-Modulator for Plants under Stress and Non-stress Conditions. Environ. Dev. Sustain., 1–52. doi:10.1007/s10668-022-02132-w
Manda, J., Alene, A. D., Tufa, A. H., Abdoulaye, T., Wossen, T., Chikoye, D., et al. (2019). The Poverty Impacts of Improved Cowpea Varieties in Nigeria: A Counterfactual Analysis. World Dev. 122, 261–271. doi:10.1016/j.worlddev.2019.05.027
MoAD, (2018). Statistical Information on Nepalese Agriculture 2017/2018. Agribusiness Promotion and Statistic Division. Hariharbhawan, Lalitpur. Kathmandu: Ministry of Agricultural Development.
Moyo, S., Norton, G. W., Alwang, J., Rhinehart, I., and Deom, C. M. (2007). Peanut Research and Poverty Reduction: Impacts of Variety Improvement to Control Peanut Viruses in Uganda. Am. J. Agric. Econ. 89 (2), 448–460. doi:10.1111/j.1467-8276.2007.00991.x
Mukherjee, S. (2020). Access to Formal Banks and New Technology Adoption: Evidence from India. Am. J. Agric. Econ. 102 (5), 1532–1556. doi:10.1002/ajae.12083
Murtazashvili, I., and Wooldridge, J. M. (2016). A Control Function Approach to Estimating Switching Regression Models with Endogenous Explanatory Variables and Endogenous Switching. J. Econ. 190 (2), 252–266. doi:10.1016/j.jeconom.2015.06.014
Nakano, Y., Tsusaka, T. W., Aida, T., and Pede, V. O. (2018). Is Farmer-To-Farmer Extension Effective? the Impact of Training on Technology Adoption and Rice Farming Productivity in Tanzania. World Dev. 105, 336–351. doi:10.1016/j.worlddev.2017.12.013
Nguimkeu, P., Denteh, A., and Tchernis, R. (2019). On the Estimation of Treatment Effects with Endogenous Misreporting. J. Econ. 208 (2), 487–506. doi:10.1016/j.jeconom.2018.10.005
Pant, S. R., and Yami, K. D. (2008). Selective Utilization of Organic Solid Wastes by Earthworm (Eisenia Foetida). Nepal J. Sci. Technol. 9, 99–104.
Rola‐Rubzen, M. F., Paris, T., Hawkins, J., and Sapkota, B. (2020). Improving Gender Participation in Agricultural Technology Adoption in Asia: From Rhetoric to Practical Action. Appl. Econ. Perspect. Policy 42 (1), 113–125.
Sangeetha, M., Paramasivam, P., and Jegadeeswari, D. (2012). Response of Catharanthus Roseusl to Organic and Inorganic Fertilization. Agric. Sci. Digest-A Res. J. 32 (4), 329–331.
Satyavathi, C. T., Bharadwaj, C., and Brahmanand, P. S. (2010). Role of Farm Women in Agriculture. Gend. Technol. Dev. 14 (3), 441–449. doi:10.1177/097185241001400308
Seufert, V., Ramankutty, N., and Foley, J. A. (2012). Comparing the Yields of Organic and Conventional Agriculture. Nature 485 (7397), 229–232. doi:10.1038/nature11069
Shahzad, M. F., and Abdulai, A. (2020). The Heterogeneous Effects of Adoption of Climate-Smart Agriculture on Household Welfare in Pakistan. Appl. Econ. 53, 1–26. doi:10.1080/00036846.2020.1820445
Stock, J. H., and Yogo, M. (2005). “Testing for Weak Instruments in Linear IV Regression,” in Andrews DWK Identification and Inference for Econometric Models (New York: Cambridge University Press), 80–108. doi:10.1017/cbo9780511614491.006
Suri, T. (2011). Selection and Comparative Advantage in Technology Adoption. Econometrica 79 (1), 159–209.
Vig, A. P., Singh, J., Wani, S. H., and Singh Dhaliwal, S. (2011). Vermicomposting of Tannery Sludge Mixed with Cattle Dung into Valuable Manure Using Earthworm Eisenia fetida (Savigny). Bioresour. Technol. 102 (17), 7941–7945. doi:10.1016/j.biortech.2011.05.056
Wang, L., Yang, F., E, Y., Yuan, J., Raza, W., Huang, Q., et al. (2016). Long-term Application of Bioorganic Fertilizers Improved Soil Biochemical Properties and Microbial Communities of an Apple Orchard Soil. Front. Microbiol. 7, 1893. doi:10.3389/fmicb.2016.01893
Wang, Y., Zhu, Y., Zhang, S., and Wang, Y. (2018). What Could Promote Farmers to Replace Chemical Fertilizers with Organic Fertilizers? J. Clean. Prod. 199, 882–890. doi:10.1016/j.jclepro.2018.07.222
Wooldridge, J. M. (2015). Control Function Methods in Applied Econometrics. J. Hum. Resour. 50 (2), 420–445. doi:10.3368/jhr.50.2.420
World Bank (2017). Climbing Higher: toward a Middle-Income Nepal. Available from: http://documents1.worldbank.org/curated/en/358501495199225866/pdf/115156-CEM-PUBLIC-SAREC-70p-Country-Economic-Memorandum-19-May-2017.pdf.
Wossen, T., Abdoulaye, T., Alene, A., Haile, M. G., Feleke, S., Olanrewaju, A., et al. (2017). Impacts of Extension Access and Cooperative Membership on Technology Adoption and Household Welfare. J. rural Stud. 54, 223–233. doi:10.1016/j.jrurstud.2017.06.022
Wossen, T., Abdoulaye, T., Alene, A., Nguimkeu, P., Feleke, S., Rabbi, I. Y., Haile, M. G., and Manyong, V. (2019). Estimating the Productivity Impacts of Technology Adoption in the Presence of Misclassification. Am. J. Agric. Econ. 101 (1), 1–16. doi:10.1093/ajae/aay017
Keywords: technology adoption, endogenous switching regression, control function, organic manure, crop yield, Nepal
Citation: Dhakal C and Escalante CL (2022) The Productivity Effects of Adopting Improved Organic Manure Practices in Nepal. Front. Environ. Sci. 10:912860. doi: 10.3389/fenvs.2022.912860
Received: 05 April 2022; Accepted: 11 May 2022;
Published: 20 June 2022.
Edited by:
Jaswinder Singh, Khalsa College, IndiaReviewed by:
Rakesh Kumar, Khalsa College, IndiaKeshav Singh, Deen Dayal Upadhyay Gorakhpur University, India
Copyright © 2022 Dhakal and Escalante. This is an open-access article distributed under the terms of the Creative Commons Attribution License (CC BY). The use, distribution or reproduction in other forums is permitted, provided the original author(s) and the copyright owner(s) are credited and that the original publication in this journal is cited, in accordance with accepted academic practice. No use, distribution or reproduction is permitted which does not comply with these terms.
*Correspondence: Chandra Dhakal, Y2hhbmRyYS5kaGFrYWwyNUB1Z2EuZWR1