- 1Discipline of Geography, School of Agricultural, Earth and Environmental Sciences, University of KwaZulu-Natal, Pietermaritzburg, South Africa
- 2Department of Space Science and Applied Physics, Faculty of Science, University of Zimbabwe, Harare, Zimbabwe
Assessment of the responses of the urban thermal environment to climate is important, especially because of their possible influence on low- and high-temperature extreme events. This study assessed the combination of remotely sensed land surface temperature (LST) and local climate zones (LCZs) with in situ air temperature-retrieved extreme temperature indices. It aimed to assess the effect of urban growth on the three-dimensional thermal environment in the Bulawayo metropolitan area, Zimbabwe. LST and LCZ were derived from the Landsat data for 1990, 2005, and 2020, while extreme temperature indices and trends were derived from daily minimum and maximum temperature data from a local weather station. Results showed that the built LCZ expanded at the expense of vegetation-based LCZ. Average LST for each LCZ increased from 1990 to 2020, which was attributed to background warming, while the expansion of high LST areas was associated with LCZ transitions. Although average minimum temperature decreased, cool nights increased, warmest nights remained unchanged, and the lowest minimum increased, the highest minimum temperatures decreased, but the trends were not statistically significant (p > 0.05). Indices of daytime warming showed significant changes, which includes an increase in average maximum temperature (p = 0.002), increase in lowest maximum temperature (p = 0), increase in the number of very warm days (p = 0.004), and decrease in the number of cool days (p = 0). The significant increase in daytime extremes was attributed to an increase in highly absorbing LCZ and daytime pollution due to industrial activities. The study also concluded that development in water areas or siltation of water bodies has a greater warming effect than other LCZ changes. The findings show that development needs to consider potential effects on the thermal environment and temperature extremes.
Introduction
Urbanization and related anthropogenic activities are causing myriad environmental changes (Grimmond, 2007; Imhoff et al., 2010; Gusso et al., 2014; Nyamekye et al., 2020). Among the changes are land use and land cover (LULC) transformations that alter the near-surface thermal characteristics (e.g., Mbithi et al., 2010; Amiri et al., 2009; Uddin et al., 2010; Nurwanda and Honjo, 2020). Such alterations in thermal characteristics have caused warming-related challenges that include increased heat-related health risks and mortalities and compromised air quality, thereby further worsening health woes (D’Ambrosio Alfano et al., 2013; Harlan and Ruddell, 2011; McMichael et al., 2006). Furthermore, surface warming has been linked with increased energy and water demand for surface, body, and room cooling (Haghighat, 2002; Pérez-Andreu et al., 2018). On the other hand, development-induced activities, such as the growth of industries, produce pollutants that add to the atmospheric composition of greenhouse gases, which in turn increase warming and related effects (Satterthwaite, 2008; Harlan and Ruddell, 2011). Hence, urbanization has the potential to cause a rise in land surface and air temperatures, whose combined effects significantly compromise urban socioeconomic activities and the environment.
Over the years, urban landscape and thermal analysis has benefited from multispectral space-borne remotely sensed datasets, such as the Landsat series, at various spatial and temporal scales (Weng et al., 2007; Jawak and Luis, 2013; Sithole and Odindi, 2015; Odindi et al., 2017). Generally, city-scale analysis is influenced by the heterogeneous nature of urban LULC types that influence thermal characteristics and distinguish them among nearby locations. Fortunately, sensor developments and improved data handling capabilities have enabled a spatially explicit analysis of these changes, even in complex environments (Dousset and Gourmelon, 2003; Meng et al., 2010; Zhou et al., 2012; Vlassova et al., 2014). For instance, sensors, such as the Landsat series, have provided data at 30 m resolution from 1972 to the present (US Geological Survey, 2019), which is capable of revealing the interaction between the land surface and the thermal environment at fine scales. Furthermore, thermal analysis has also been enhanced by the development of the local climate zones (LCZs) approach, which maps cities into categories related to surface and air temperatures (Bechtel et al., 2012; Stewart et al., 2014; Cai et al., 2016, 2017). The LCZs provide a complete overview of human effects on the climate system as they include both effects of land cover and land use (such as industrialization) on the thermal environment (Stewart and Oke, 2012). Understanding the responses of the thermal environment to long-term changes is important not only for monitoring a city’s climate but also for assessing the sustainability of its development trajectories.
Among the known impacts of climate change is the intensification of extreme events, such as heat waves, strong winds, heavy precipitation, and prolonged dry spells (Hallegatte and Corfee-Morlot, 2011; Parnell and Walawege, 2011; Goddard and Gershunov, 2020). Small changes in the mean can cause a large change in the likelihood of extreme climate events (Sensoy et al., 2013). For instance, rising temperatures will influence human societies and natural ecosystems with potentially severe effects globally, while the increased heat load in urban areas will harm public health (Sensoy et al., 2013; Geleti et al., 2019). To aid and standardize the analysis, 27 extreme climate indices (precipitation and temperature) have been developed and improved over time (Stenseth et al., 2003; Zubler et al., 2014; Sajjad and Ghaffar, 2019). In line with this, the RClimdex software was developed by the National Climate Data Centre of NOAA to compute the 27 indices of extreme climate using R software. The program provides an easy-to-use software package for the calculation of indices of climate extremes as well as their long-term trends for monitoring and detecting climate change impacts. The indices describe special characteristics of extremes, including amplitude, frequency, and persistence (Toure et al., 2017). They cover a large range of climates and have a large signal-to-noise ratio. Most importantly, they involve the calculation of the number of days in a year exceeding specific thresholds. The indices were found to reveal details hidden in the trends of annual average values. For instance, Kruger (2006) and Kruger and Sekele (2012) observed insignificant trends in annual precipitation and absolute temperatures (maximum and minimum), while extreme precipitation and temperature indices showed significant trends in South Africa. Similarly, a number of studies have indicated strong trends in extreme climate events, especially those related to temperature due to warming (e.g., Kruger, 2006; Mónica and Santos, 2011; Vincent et al., 2011; Athar, 2014; Sein et al., 2018). The indices are of great value, thereby needing application to different types of environments to understand climate trends and their impact.
Although indices have been applied in previous studies, the focus has mainly been on large-scale analysis, such as regional and national (e.g., Kruger, 2006; Vincent et al., 2011; Kruger and Sekele, 2012; Zubler et al., 2014; Rahimi and Hejabi, 2017; Popov et al., 2018; Ogunjo et al., 2021). For instance, Kruger (2006) and Kruger and Sekele (2012) focused on South Africa and observed a rising trend in temperature and precipitation extremes, respectively. On a similar scale, (Vincent et al., 2011) observed significant trends in warming-related extremes and weak trends in precipitation extremes in western India. Recently, Ogunjo et al. (2021) observed rising trends in heat-related extremes and falling trends in cold extremes, such as cold days over Nigeria. While large-scale analysis provides a broader picture, localized analyses are important for area-specific and dynamic adaptation and mitigation strategies and activities. This is important, especially in urban areas of developing countries, where rapid development activities and population growth are influencing climate, thereby worsening the potential for harsh extreme events, especially those related to warming. However, to the best of our knowledge, studies that focus on extreme climate events, especially those related to temperature in urban areas, are scarce. Furthermore, there is a paucity of studies that relate urban growth to trends in extreme climate events, especially in developing countries, despite the projected growth and climate patterns. The growth of cities, which has occurred over the years, has changed the spatial structure of LCZs, and the responses of the thermal environment to these long-term changes need to be monitored and understood. The impact of climate change-related extreme events is greater where large populations and high-value properties are at great risk (Brown et al., 2010), which are characteristic of urban areas. A variety of socioeconomic and development activities can be adversely affected by harsh climatic extremes. For instance, high-temperature extremes can cause the melting of tarred roads and affect the density of air, thereby causing aircrafts to lose lift as well as causing mechanical problems in machinery and human health issues (Abatan et al., 2016). Evidently, the effects of long-term historical urban growth patterns on the thermal environment help in understanding the cumulative effects of slow and unmitigated anthropogenic activities that are important for planning future development trajectories.
Urban growth causes LCZ transitions that alter the spatial distribution of the LST, which perpetually influences the air temperature above. Therefore, the analysis of LST patterns provides two-dimensional information on the extent to which land surfaces are heating the surrounding air and influencing extreme temperature events. Similarly, temporal changes in the LCZ and the LST provide an overview of the effect of changes in surface properties and in the amount of heat received and emitted by constant surfaces over time. On the other hand, a third dimension that expresses the consequence of anthropogenic and natural influences can be offered by extreme temperature indices. To date, studies have kept surface and air temperature analysis separate, thereby limiting understanding of the interaction between these interfacing components (e.g., Pielke et al., 2011; Wu et al., 2012; Ahmed et al., 2013). For instance, replacing a natural LCZ with an industrial area will not only change LST due to the construction of buildings and impervious surfaces but also change the air temperature and associated extremes due to increased pollution and the greenhouse effect. It is important to quantify changes in LST and extreme temperature indices and isolate the influence of LCZ changes from other causes of LST changes. Air and surface temperature complement each other as the former represents all-sky conditions while the other represents clear-sky conditions only. Air temperature measurements can add value by providing useful information for decision support, such as using indices to highlight trends in extreme temperatures. Evidently, combining LCZ and LST information with extreme temperature indices provides insights into the interface between development and long-term climate extremes in dynamic urban environments. In view of observed and projected changes in temperatures of cities and increased anthropogenic activities, it is important to assess the corresponding changes in indicators of extremes related to low and high temperatures. Therefore, understanding the patterns of low and high-temperature extremes is required for the formulation of adaptation and mitigation strategies as well as for the implementation of sustainable urban development strategies.
Similar to other cities in the developing world, Zimbabwean cities are experiencing exponential growth, thereby resulting in expansive LCZ transitions. A previous study that looked at climate extremes in Zimbabwe focused on a large area by including countries in western central Africa and Guinea Canakry (Aguilar et al., 2009). The study focused on general trends in extreme rainfall and temperature, not a localized link between growth patterns and extreme climate events, especially in the context of LCZ. It is necessary to monitor the long-term changes in the urban thermal environment and understand them in the context of both LCZ dynamics and background (global warming). Hence, this study seeks to quantify 1) the contribution of LCZ changes to long-term changes in LST and extreme temperature events in Bulawayo and 2) the long-term influence of background warming on LST in different LCZs. The study also assesses the combined effect of LCZ changes and background warming on extreme temperature events using extreme temperature indices.
Datasets and Methods
The Study Area
Bulawayo is located in the southeast of Zimbabwe and is the country’s second-largest city. The city is at an elevation of approximately 1358 m above sea level. Rainfall is prevalent between October and March, and the area is characterized as hot and wet, with an average temperature of 25°C. The rest of the months has an average temperature of 15°C under cool and dry conditions (Mutengu et al., 2007). The city is located in a semi-arid climate, where rainfall is erratic rainfall (annual average precipitation of 600 mm and rainfall ranging from 199.3 to 1258.8 mm). Proximity to the Kalahari makes the area vulnerable to droughts (Gumbo et al., 2003; Muchingami et al., 2012). While a network of weather stations is ideal for depicting spatial patterns in temperature extremes, only two weather stations, operated by the Meteorological Services Department, are operational in the city. One at Goertz Observatory is for public weather and climatological services and the other at Joshua Nqabukho Airport (Bulawayo Airport Meteorological Office - BAMO) is for aviation purposes, with its data inaccessible. Hence, due to the limited number of weather stations, combining space-borne LST with in situ air temperature observations enhances spatial and temporal analysis of the city’s thermal environment. Table 1 indicates the main sources of data in this study.
Retrieval of Responses of LCZ to Growth of Bulawayo
Multispectral optical data for 1990 (Landsat 5), 2005 (Landsat 7), and 2020 (Landsat 8) were resampled to ensure the same orientation of pixels for all periods (Table 2). The data were also corrected for atmospheric effects using the dark subtraction methods following solar zenith normalization. For each year, imageries were collected in the cool dry, hot dry, and wet (post-rain) seasons. The rainy season was not used due to difficulty in obtaining cloud-free imageries, thereby making it difficult to have data for the same seasons in all considered periods.
LCZs identified in the study area are described in Table 3. LCZ maps were produced for each year using the World Urban Database and Access Portal Tools (WUDAPT) Level 0 procedure (Bechtel et al., 2012; Mitraka et al., 2015; Cai et al., 2016). The technique involves digitizing training areas in Google Earth, mapping LCZ using the Random Forest classifier, and assessing accuracy in the SAGA GIS software. Sample locations (coordinates) of representative points were collected for each of the LCZ categories at evenly distributed places across the study area between 18 and 27 October. This experience also guided the selection of training areas for historical periods using Google Earth in the absence of field measurements. Field observations increased the validity of the analysis instead of exclusive reliance on Google Earth retrievals. Data were collected in a manner to capture not only interclass but also intraclass variabilities, especially those due to seasonality.
Approximation of Mean Spatial Distribution of Average Air Temperature Across LCZs
The city has only two weather stations (Goertz Observatory and Bulawayo Airport Meteorological Office). The two could not provide a spatial depiction of air temperature caused by LCZ variations in the city. As such, a 21 km2 resolution bias corrected the Worldclim maximum and minimum temperature data (https://www.worldclim.org/data/monthlywth.html). The data for 1990–2018 were used to depict the spatial variations of average air temperature in the city. The 1 km2 resolution data were not used because they did not cover periods after 2000. Although the study period continued to 2020, analysis of Worldclim data was only confined to up to 2018 due to the data available on the online platform. The gridded data were chosen due to the lack of weather stations to map the spatial structure of air temperature in the study area. The link between average air temperature and LST was qualitatively investigated. The Mann–Kendall test at a 95% confidence interval was performed to detect the effect of LCZ changes on annual average maximum and minimum air temperatures derived from the monthly Worldclim data.
Derivation of Extreme Temperature Indices and Their Trends
Brown et al. (2010) provided a definition of 27 extreme climate indices. In this study, we focused on 12 defined in Table 4 which characterizes temperature extremes. In this study, we used indices that compute based on the percentage of days below or above the thresholds (cool nights, warm nights, cool days, and warm days) and the number of days below or above the thresholds (warm spell duration indicator, cool spell duration indicator, frost days, summer days, and tropical nights) as well as intensity-based thresholds (highest maximum temperature, lowest minimum temperature, highest minimum temperature, and daily temperature range). Due to years of improvements, the computation of indices and their temporal trends have been incorporated into the freely accessible RClimdex software used in this study. The computation of extreme temperature indices makes use of continuous records of daily minimum and maximum temperature data for a given area. As such, daily maximum (TX) and minimum (TN) temperature data for the period 1990 to 2020 were used. Although low-temperature extremes, such as ground frost, are experienced in the study area, the ice days (ID0) extreme temperature index was not investigated because there are no records of snow in this semi-arid location. The selection of area-relevant climate extreme indices was in line with Almazroui et al. (2014), as they excluded analysis of extreme precipitation indices between 1981 and 2010 in Saudi Arabia because the area is dry, thereby recording very few days of rainfall in any year. Sein et al. (2018) also selected 19 extreme climate indices in Myanmar based on their applicability in the study area. Although Bulawayo is semi-arid, it receives rainfall on a countable number of days in a year. In this study, rainfall extremes were not included because the focus was on responses of temperatures and related extremes to growth and anthropogenic activities in the area. The data were initially quality controlled and put through a homogeneity test using the RH test, which is also provided as a component for the retrieval of indices (Zhang et al., 2004; Almazroui et al., 2014). Since the procedure included analysis of trends in extreme temperatures, homogeneity test was performed to identify nonclimatic changes, such as changes in location of stations. Fortunately, in the period under study, there was assurance that the instruments and location of stations did not change, given that very recent and known 30 years were analyzed. Previous studies (Sensoy et al., 2013; Almazroui et al., 2014; Sein et al., 2018; Geleti et al., 2019) also used at least 30 years and 30-year intervals to investigate trends in climate extremes. Trends in the indices were computed in RClimdex using ordinary least squares fit, and the Mann–Kendal test was used to test the statistical significance (Abatan et al., 2016). RClimdex manual (Zhang et al., 2004) provides detailed steps on the derivation of extreme climate indices and analysis of trends.
Computation of Long-Term Changes in LST
Landsat 5, Landsat 7, and Landsat 8 thermal data were used to compute LST for 1990, 2005, and 2020. In each season, images were collected around the same dates [anniversary images–(Mouat et al., 1993)], with similar meteorological conditions (mainly on calm days with clear skies) on different days. Mouat et al. (1993) indicated that the influences in seasonal conditions are eliminated using anniversary images in change detection. Feng et al. (2014) used imageries of January to show the influences of land surface changes on LST from 1987 to 2007 in Xiamen City in China. Amorim (2018) showed that LSTs are influenced by rainfall activity prior to satellite overpass and data acquisition. This was achieved by ensuring rainfall activities 10 days prior to each scene were similar for imageries of the same season in different years. Since cloud cover also affects LST, cloud-free imageries were used. Therefore, LST changes in this study resemble changes for days with similar weather conditions preceding and during the satellite overpass. This eliminates randomness due to differences in weather conditions around the image acquisition time. Randomness was further reduced by computing an average LST layer for each year using retrievals from different seasons. Initially, the data were corrected for differences in solar zenith angles. While Landsat 8 has two thermal infrared bands, a single-channel technique was applied for all periods to minimize the effects of differences in computation algorithms on LST variations between times. Digital numbers of thermal data were converted to radiances using the reflectance toolbox in ArcGIS version 10.2, which were then used to determine the brightness and surface temperature using Equation 1 (Stathopoulos et al., 2004; Chen et al., 2006; Srivanit et al., 2012).
K1 takes a value of 607.76, 666.09, and 774.89 W/(m2 srμm), while K2 has values of 1260.56, 1282.71, and 1321.08 W/(m2 srμm) using Landsat 5, Landsat 7, and Landsat 8 data, respectively. A method based on spectral and blackbody radiance of the thermal infrared band was used to obtain a pixel-based land surface emissivity map (
Where
Linking Long-Term Changes in LCZ to LST Dynamics
Using spatial overlays, the average LST per LCZ category was obtained for each period. LST variations in each LCZ were analyzed between 1990 and 2020. Furthermore, data for 1990 and 2020 were used to derive normalized temperatures as well as to determine the effect of LCZ transitions on LST intensities. The normalized temperature approach (Zhou and Wang, 2011) enables the separation of intraclass LST changes from those due to LCZ transitions between any two intervals. In this study, intra-LCZ LST changes were attributed to other external factors, such as background warming, which were removed from interclass LST changes to derive the effects of LCZ transitions. Changes in LST without transition in LCZ were computed using Equation 3.
Composite differences in LST between LCZ j and LCZ i was computed by Equation 4.
The normalized temperature was then used to represent the change in LST due to the transition from LCZ i to LCZ j using Equation 5.
Where dTn is the change in temperature caused by the replacement of LCZ i by LCZ j, ∆Ti is the change due to other factors than LCZ change, and dTij is the change in temperature before normalization.
Results and Discussions
LCZ Mapping and Transitions
The LCZs were mapped with overall accuracies of 98, 98.2, and 95% for 1990, 2005, and 2020, respectively (Figure 1). Built-up LCZs, namely compact low rise, lightweight low rise, and open low rise, expanded between 1990 and 2020. Densely built-up LCZs expanded in the western direction, while open settlement arrangements expanded in the eastern direction. Visual inspection of Figure 1A–C suggests that the expansion of lightweight low rise mainly intruded the low plants LCZ. There is also evidence of change from dense vegetation to low plants, which indicates deforestation as the city grows. Throughout the study period, the Goertz Observatory weather station remained in the open low-rise LCZ category, although changes were experienced in other areas, including its surroundings. Compact low rise, which occupied 3% in 1990, increased coverage to 4.5% of the entire study area in 2020. Marked quantitative expansions were observed in lightweight low rise and open low rise, which increased from 9.1 to 17.3% and 10.4–20.4% of the study area between 1990 and 2020, respectively. Vegetation and water-based LCZ declined during the same period with a marked change in low plants LCZ, which reduced from 68.2 to 51.4% of the study area.
Spatial Distribution of Air Temperature and Link With LCZ
Average minimum and maximum temperatures decreased westwards in the city over the different study periods (Figure 2A–H). When comparing the built LCZs, high average minimum and maximum temperatures generally coincide with areas occupied by densely built LCZ, such as the compact low rise and lightweight low rise. High average temperatures also occurred in low plant areas, especially in the northern half of the city. Low plants LCZ included agricultural land, bare areas, and grasslands. The heat mitigation value of grasslands was overshadowed by the heat absorption effect of bare and dry vegetation of cropland areas in post-planting periods, thereby resulting in high temperatures in the low plants LCZ. On the contrary, low average air temperatures occurred in areas in the west occupied by open low rise, which are characterized by well-spaced buildings within a high fraction of healthy vegetation. Figure 2D shows that the northern half of the city experienced a drop in minimum air temperatures, while the southern half experienced increases. However, the rates of change in minimum air temperature over the 30-year period were almost insignificant (below 0.0001 °C/year). On the other hand, Figure 2G shows a pronounced rate of change in maximum air temperature in all areas of the city. Larger rates of change in maximum air temperature were recorded mostly in the southern half of the city, which corresponded to areas where massive growth of built LCZ occurred between 1990 and 2018. A comparison of temperatures in 1990, 2005, and 2018 showed that the year 2005 was warmer than the others (Figure 2B and Figure 2G). Since the overall trends based on continuous data from 1990 to 2018 showed warming, larger temperatures in 2005 than the other years could be attributed to climate variability with the broad changes. Additionally, before the intensification of the economic crisis in 2008, Bulawayo was the industrial hub of Zimbabwe. The marked warming from 1990 to 2005 could be due to increased industrial pollutants, which slowed down post-2008, thereby causing a drop in temperatures from 2005 to 2018. The temperatures remained higher toward 2018 than around 1990 due to contributions from some of the industrial activities, which managed to continue and other effects, such as LULC changes due to city growth.
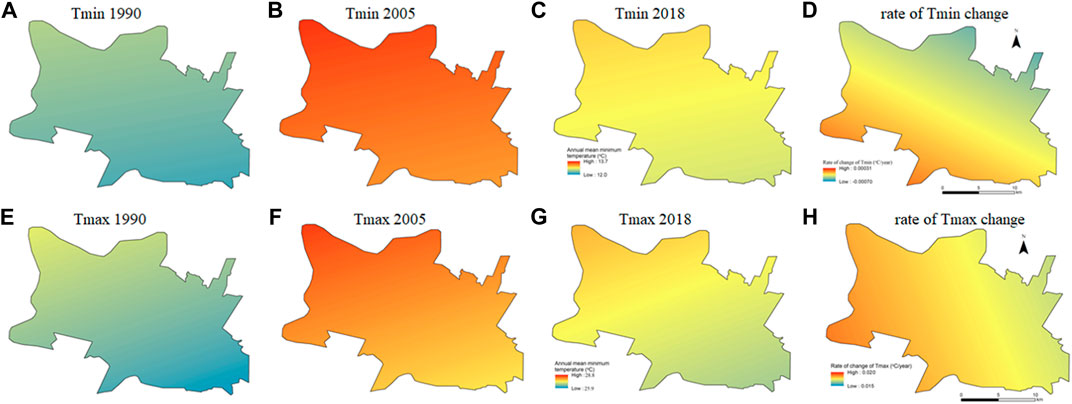
FIGURE 2. Annual mean minimum temperatures (A–C) and their rate of change (D) as well as annual mean maximum temperatures (E–G) and their rate of change (H) from 1990 to 2018.
Responses of Air Temperature Extremes to Urban Growth and LCZ Changes
The changes in minimum temperature extremes were mostly characterized as insignificant trends (p > 0.05) and slope errors, which were greater than the slope at the Goertz Observatory station (Figure 3). As such, the average minimum temperature showed a decreasing trend with a slope of −0.008°C/year, a slope error of absolute value of 0.008°C/year, and an insignificant trend (p = 0.323)—(Figure 3A–H). Similarly, insignificant trends with slope error exceeding slope were observed on warm nights (Figure 3D), cold spell duration indicator (Figure 3E), and the highest minimum temperature (Figure 3F).
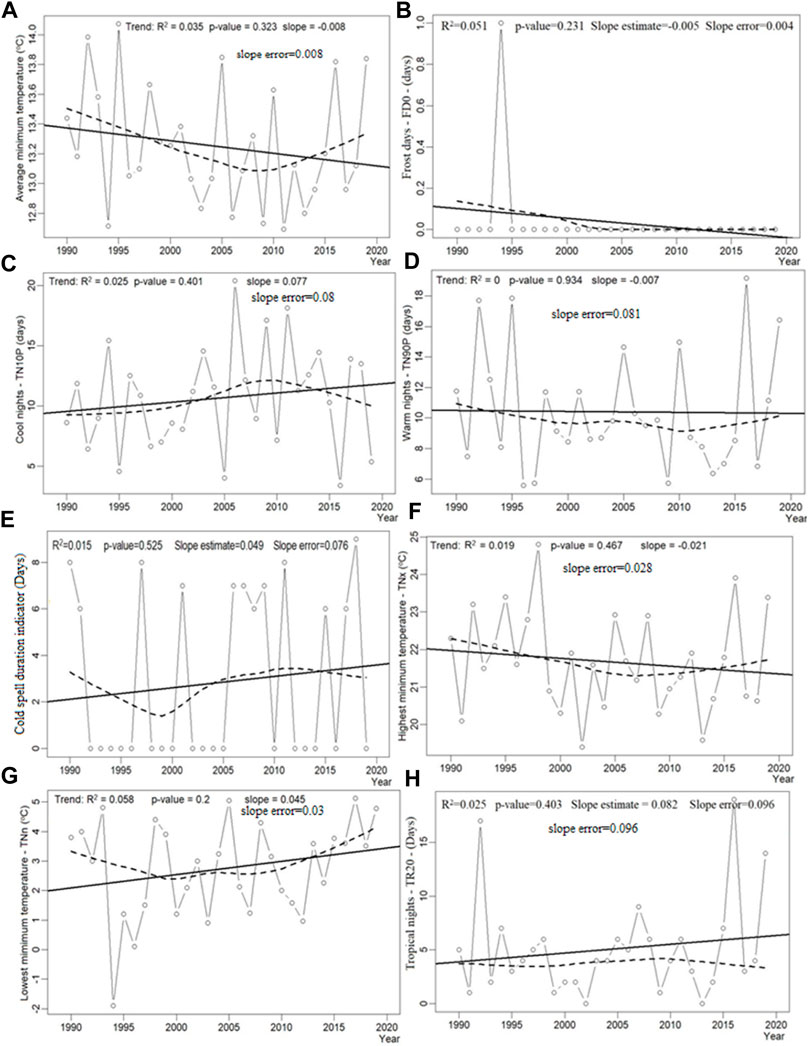
FIGURE 3. Trends in (A) Average minimum, (B) Frost days, (C) Cool nights, (D) Warm nights, (E) Cold spell duration indicator, (F) Highest minimum, (G) Lowest minimum, and (H) Tropical nights at the Goertz Observatory in Bulawayo.
Maximum temperature–related extremes showed meaningfully (slope greater than slope error) and significant trends (p < 0.05) between 1990 and 2020 (Figure 4). From 1990 to 2020, the mean maximum temperature for Bulawayo increased significantly (slope of 0.06°C/year, slope error of 0.017°C/year, and p-value of 0.002) as observed at Goertz Observatory (Figure 4A). Significant trends were also observed in the increase in warm days (Figure 4B), rising in the daily temperature range (Figure 4C), and increase in the number of summer days (Figure 4D), as well as in decrease in cold days (Figure 4E). Significant rising trends were also recorded in lowest and highest maximum temperatures (Figure 4G and Figure 4H), all indicating shifts to tormentingly hot daytimes. Although the trend was insignificant, events of successive warm days are also increasing, as indicated by a rising trend in the warm spell duration indicator (Figure 4F).
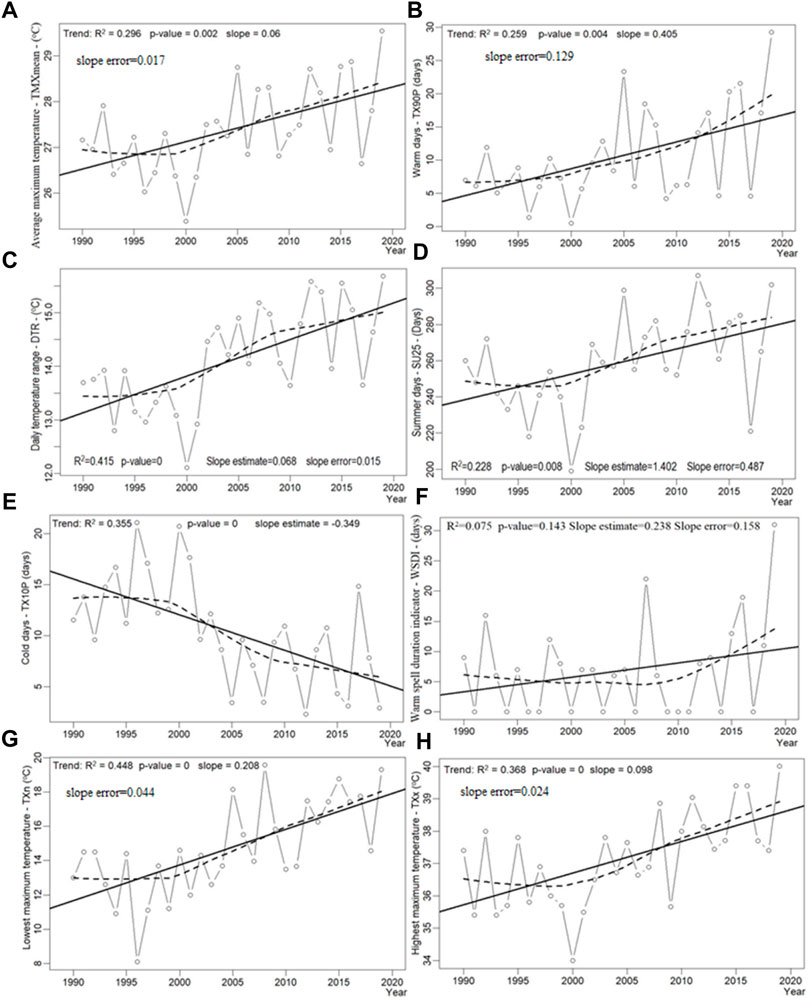
FIGURE 4. Trends in (A) Mean maximum temperature, (B) Warm days, (C) Daily temperature range, (D) Summer days, (E) Cool days, (F) Warm spell duration indicator, (G) Lowest maximum temperature, and (H) Highest maximum temperature at the Goertz Observatory in Bulawayo.
Long-Term Changes in the Two-Dimensional LST in Response to LCZ Changes
Figure 5 shows the expansion of high-temperature surfaces between 1990 and 2015. In 1990, LSTs in the 37.8–43.8°C range (Figure 5A) dominated the city, while LSTs below 43.8°C became uncommon in 2005 (Figure 5B). Visual inspection shows that in 2020, LSTs became even higher, with most areas recording values above 46.8°C (Figure 5C). The water areas were the most stable, with LSTs in the 16.8 to 37.8°C range in 1990, 2005, and 2020.
Lower LSTs were observed in residential rather than commercial built LCZs, with a marked decline as vegetation abundance increased (Figure 6). In all LCZs, LST changes were larger in 1990 to 2005 than in the 2005 to 2020 interval. For instance, the average LST for compact low rise increased from 40.8 to 47.7°C between 1990 and 2005, while it increased from 47.7°C between 2005 and 2020. LST in dense forest areas was almost similar to those in open low-rise regions and cooler than those in other LCZs, except in water areas.
Background Warming Corrected Effects of LCZ Transitions on LST Dynamics
The change from other LCZ types to compact low rise resulted in warming except for such change from low plants’ LCZ, which could lead to a 0.4°C drop in LST (Table 5). Replacing water with any other LCZ would cause warming by at least 9.6°C. Increased vegetation density in lightweight low-rise LCZ areas would cause an LST decrease of approximately 1.2°C.
Discussion of Findings
Although all built LCZs expanded between 1990 and 2020, the residential areas (open low rise and lightweight low rise) expanded more than the central business district (CBD) and industrial areas (compact low rise). The expansion of residential areas provides evidence that the population of urban dwellers is increasing with time in developing countries. People develop the tendency to prefer advanced areas of the country, where improved amenities are available and there is ease of access to basic needs. Generally, in Zimbabwe, ownership of a home in an urban area is a symbol of wealth, which drives citizens to work hard to purchase land and develop it. Furthermore, as incomes improve, people also purchase land in spacious areas, as evidenced by the expansion of open low-rise LCZ, which coincides with medium- to low-density residential areas, where medium- to high-income strata reside. While the built LCZ expanded, vegetation and water-based LCZs shrunk during the same time interval. This is because of spacious housing developments within vegetation LCZs (open low rise) and, in other areas, massive replacement of vegetation with densely built compact low rise and lightweight low rise LCZs. The slower expansion of compact low rise than other built LCZs could be due to the cost of land areas due to commercialization in the CBD and industrial areas.
Analysis of Worldclim air temperature data showed low-temperature values in vegetation than built LCZs. High vegetation fraction areas benefit from the cooling effect of vegetation due to latent heat of vaporization transfer (Ca et al., 1998; Thatcher and Hurley, 2012; Tao et al., 2013; Chun and Guldmann, 2014). Since air temperatures are measured at 1 m, trees provide a shading effect at that height, thereby resulting in low temperatures in occupied LCZ (Toudert, 2005; Hwang et al., 2011; Puliafito et al., 2013; Zhao et al., 2015). A generalization of air temperatures caused by the coarse spatial resolution of the Worldclim data was inadequate for depicting minute temperature structures caused by fragmentation and LCZ heterogeneity in the study area. The details were not as spatially explicit as LST counterparts mapped when using Landsat data resampled to 30 m resolution. However, although the resolution was coarse, the air temperature map successfully showed the general effect of the LCZ structure on the near-surface thermal environment of Bulawayo.
Meaningful, though statistically insignificant, trends were recorded in frost days, lowest minimum temperature, and tropical nights extreme climate indices, which were decreasing, increasing, and increasing, respectively. Based on these trends, we deduced that there is an insignificant increase in night temperature, thereby causing slight warming, which has reduced the occurrence of low-temperature extremes and increased warm/tropical nights in Bulawayo. The night warming could be insignificant, given that Bulawayo is semi-arid with high chances of cloud-free nights, which allow radiation to escape to outer space, thereby moderating nighttime warming extremes. The expansion of the urban fabric could also provide the capacity to lose heat at slow rates, thereby reducing the chances of low-temperature extremes. However, this study did not involve an in-depth analysis of the materials used in urban construction beyond partitioning the city based on the LCZ scheme.
Observed significant rise in daytime temperature concurs with general trends of rising air temperature recorded in other studies in Zimbabwe (Unganai, 1996; Zvigadza et al., 2010; Brazier, 2015). For instance, Zvigadza et al. (2010) observed that temperatures in the country rose by over 1°C over 40 years up to 1998. However, these previous studies used low temporal resolution analysis and focused on average temperatures rather than extreme events. The study also showed that the lowest maximum temperature, highest maximum temperature, and the number of successive hot days significantly increased between 1990 and 2020. In recent years, record-breaking high temperatures and heat waves have occurred in different parts of Zimbabwe, especially during the hot season. Although the LCZ did not change at the Goertz Observatory between 1990 and 2020, maximum temperature extremes significantly changed during the period. This is similar to the findings that the values of extreme climate indices in all LCZs in Brno, Czech Republic, increased from the sixth decade of the 20th century toward the first decade of the 21st century (Geleti et al., 2019). Although this study was on a much-localized scale, significant trends in warming-related climate extremes are also in tandem with observations from other parts of the world. For instance, Rahimi and Hejabi (2017) observed significant warming trends in extreme temperature indices between 1960 and 2014 in Iran. Similar to this study, Popov et al. (2018) observed decreasing/increasing trends in cold/warm extremes in Bosnia and Herzegovina. A decrease in cool days, an increase in warm days, and an increase in mean maximum temperature were also observed over Lagos, Nigeria (Ogunjo et al., 2021). The warming could be attributed to an increase in anthropogenic activities, such as changes in LCZ and industrial activities, which add pollutants to the lower atmosphere.
The city surface was warming as the spatial structure of LCZs changed between 1990 and 2005. According to Nayak and Mandal (2019), urbanization causes temperature changes due to both alterations of LULC and greenhouse gas concentrations. Similarly, in this study, we attributed surface warming to LCZ transitions and background caused by anthropogenic activities, such as industrial emissions. Blake et al. (2011) also reported that Harare was warming, despite cooling in the decade from 1900 to 2002. The heat mitigation value of vegetation was not evident in low plants LCZ during the study period. The low plants LCZ is composed of a mixture of bare, agricultural, and grassland areas, thereby resulting in varied effects on the thermal environment. For instance, dry, bare areas and dry vegetation eliminate the heat mitigation value of healthy low plants. Furthermore, the study was conducted during the hot, dry season when most of the trees had shed leaves during the cool season. Most low plant areas will be bare or covered with dry grass, while agriculture areas will mostly also be bare or covered with dry crop residues with no heat mitigation value due to the absence of moisture. The warming effects of LCZ transitions concur with the assertion by Feng et al. (2014) and Gallo et al. (1993) that urbanization-induced changes can impact temperature trends similar to those expected under an enhanced greenhouse warming scenario.
Vegetation moderated LSTs even in built-up LCZs, such as lightweight low rise. Field surveys and Google Earth images show that in Bulawayo, even in lightweight low rise areas, residents prefer trees and other vegetation covers in available spaces. Similar to patterns observed in Harare (Mushore et al., 2018), open low-rise areas, which correspond to spacious settlements of middle to high-income strata, have abundant and well-maintained vegetation cover. According to Sithole and Odindi (2015), green spaces act as heat sinks as they tend to be porous and assimilate local heat, which explains low temperatures in areas of abundant vegetation. In recent years, although not measured in this study, lifestyle changes noticed in Zimbabwe’s cities, such as an increased volume of private vehicles, could explain larger increases in temperature after than before 2005. The smallest changes in LST were observed in the water LCZ, while similar surface warming patterns appeared in compact low rise and low plants LCZ areas. Even within an LCZ category, LST changes were recorded, thereby implying that the warming was not only due to LCZ transitions but also due to background warming. The warming effect of greenhouse gases within a city is not only confined to industrial areas as the pollutant spread around an open atmosphere. However, the impact is reduced by heat and carbon assimilation effects in areas where vegetation is abundant.
The study showed a three-dimensional warming pattern as the city of Bulawayo was growing. The LST was warming while air temperatures and associated extreme events rose between 1990 and 2020. The replacement of natural covers, especially vegetation and water with built LCZs, increases heat absorption capacity of the city, which warms the air as it moves over these areas, thereby resulting in increased air temperatures. The observed rates of change in LST were consistent with those observed in other studies and were larger than those in air temperatures. This could be due to differences in heat absorption rates and capacities between air and the LCZs. Additionally, air temperature measurements include variability due to clouds and are observed at higher temporal resolution (before averaging) than LST observations, which were only computed for cloud-free days and anniversary imagery. The findings concur with previous studies, which showed that urbanization causes warming in formerly natural environments (Weng et al., 2007; Grossman-Clarke et al., 2010; Jalan and Sharma, 2014). As such, Jalan and Sharma (2014) and Grossman-Clarke et al. (2010) indicated that replacing vegetation with urban fabric can increase LST by 2–4°C. Overall, the study showed that growth-induced LCZ transitions altered the spatial structure of LST while influencing air temperatures and temperature extremes.
Conclusion
This study investigated long-term changes in the thermal environment of Bulawayo metropolitan city by combining both surface and air temperature measurements. Air temperature measurements were used to derive indices for low- and high-temperature extremes. Both surface and air temperature measurements indicated that the city is warming. Although extreme low temperature–related indicators showed rising trends, the patterns were not statistically significant. On the other hand, warming is significantly increasing high temperature–related extremes. This concludes that comfortable hours are reducing during daytimes, while the nighttime relief has been maintained over the years. Besides warming due to LCZ transitions, the temperature rises were also observed within all LCZs. The study concluded that the background temperature rise due to global warming and LCZ dynamics combined effects to cause temperature elevation in the city. Replacing the water with other LCZ categories has the strongest implications on the thermal environment, thereby causing warming by at least 9°C in the altered areas. Construction of buildings in formerly vegetation LCZ areas and densification of buildings also have significant temperature elevation effects. Conversely, the densification of forests, maintenance of vegetation covers, such as grasslands, and conservation of wetlands have strong heat mitigation effects. LCZs and extreme climate indices combine to adequately explain the responses of the climate system to anthropogenic activities.
Data Availability Statement
Publicly available datasets were analyzed in this study. This data can be found here: https://earthexplorer.usgs.gov/.
Author Contributions
TM was involved in all steps from conceptualization of the study to finalization of the manuscript. OM and JO played critical supervisory roles in all phases of the study. They were also involved in data collection and analysis and iterative preparation of the manuscript.
Funding
The research of this article was supported by DAAD within the framework of the climapAfrica program with funds from the Federal Ministry of Education and Research. The publisher is fully responsible for the content. This work was funded by the National Research Foundation of South Africa (NRF) Research Chair in Land Use Planning and Management (Grant Number: 84157).
Conflict of Interest
The authors declare that the research was conducted in the absence of any commercial or financial relationships that could be construed as a potential conflict of interest.
Publisher’s Note
All claims expressed in this article are solely those of the authors and do not necessarily represent those of their affiliated organizations or those of the publisher, the editors, and the reviewers. Any product that may be evaluated in this article or claim that may be made by its manufacturer is not guaranteed or endorsed by the publisher.
References
Abatan, A. A., Abiodun, B. J., Lawal, K. A., and Gutowski, W. J. (2016). Trends in Extreme Temperature over Nigeria from Percentile-Based Threshold Indices. Int. J. Climatol. 36 (6), 2527–2540. doi:10.1002/joc.4510
Aguilar, E., Aziz Barry, A., Brunet, M., Ekang, L., Fernandes, A., Massoukina, M., et al. (2009). Changes in Temperature and Precipitation Extremes in Western Central Africa, Guinea Conakry, and Zimbabwe, 1955-2006. J. Geophys. Res. 114, 1–11. doi:10.1029/2008JD011010
Ahmed, B., Kamruzzaman, M., Zhu, X., Rahman, M., and Choi, K. (2013). Simulating Land Cover Changes and Their Impacts on Land Surface Temperature in Dhaka, bangladesh. Remote Sens. 5 (11), 5969–5998. doi:10.3390/rs5115969
Almazroui, M., Islam, M. N., Dambul, R., and Jones, P. D. (2014). Trends of Temperature Extremes in Saudi Arabia. Int. J. Climatol. 34 (3), 808–826. doi:10.1002/joc.3722
Amiri, R., Weng, Q., Alimohammadi, A., and Alavipanah, S. K. (2009). Spatial-temporal Dynamics of Land Surface Temperature in Relation to Fractional Vegetation Cover and Land Use/cover in the Tabriz Urban Area, Iran. Remote Sens. Environ. 113 (12), 2606–2617. doi:10.1016/j.rse.2009.07.021
Amorim, M. C. d. C. T. (2018). Spatial Variability and Intensity Frequency of Surface Heat Island in a Brazilian City with Continental Tropical Climate through Remote Sensing. Remote Sens. Appl. Soc. Environ. 9, 10–16. doi:10.1016/j.rsase.2017.11.001
Athar, H. (2014). Trends in Observed Extreme Climate Indices in Saudi Arabia during 1979-2008. Int. J. Climatol. 34 (July 2013), 1561–1574. doi:10.1002/joc.3783
Bechtel, B., Langkamp, T., Böhner, J., Daneke, C., Oßenbrügge, J., and Schempp, S. (2012). Classification and Modelling of Urban Micro-climates Using Multisensoral and Multitemporal Remote Sensing Data. Int. Arch. Photogramm. Remote Sens. Spat. Inf. Sci. XXXIX-B8 (September), 463–468. doi:10.5194/isprsarchives-xxxix-b8-463-2012
Blake, R., Grimm, A., Ichinose, T., Horton, R., Gaffin, S., and Jiong, S. (2011). “Urban Climate: Processes, Trends and Projections,” in First Assessment Report of the Urban Climate Change Research Network, 43–81.
Brazier, A. (2015). CLimate Change in Zimbabwe. Facts for Planners and Decision Makers. Alexandra Park, Harare: Konrad-Adenauer-Stiftung 26 Sandringham Drive.
Brown, P. J., Bradley, R. S., and Keimig, F. T. (20102006). Changes in Extreme Climate Indices for the Northeastern United States, 1870-2005. J. Clim. 23, 6555–6572. doi:10.1175/2010JCLI3363.1
Ca, V. T., Asaeda, T., and Abu, E. M. (1998). Reductions in Air Conditioning Energy Caused by a Nearby Park. Energy Build. 29 (1), 83–92. doi:10.1016/s0378-7788(98)00032-2
Cai, M., Ren, C., Xu, Y., Dai, W., and Wang, X. M. (2016). Local Climate Zone Study for Sustainable Megacities Development by Using Improved WUDAPT Methodology - A Case Study in Guangzhou. Procedia Environ. Sci. 36, 82–89. doi:10.1016/j.proenv.2016.09.017
Cai, M., Ren, C., and Xu, Y. (2017). “Investigating the Relationship between Local Climate Zone and Land Surface Temperature,” in 2017 Joint Urban Remote Sensing Event (JURSE), Dubai, United Arab Emirates, 06-08 March 2017, 1–4. doi:10.1109/JURSE.2017.7924622
Chen, X.-L., Zhao, H.-M., Li, P.-X., and Yin, Z.-Y. (2006). Remote Sensing Image-Based Analysis of the Relationship between Urban Heat Island and Land Use/cover Changes. Remote Sens. Environ. 104 (2), 133–146. doi:10.1016/j.rse.2005.11.016
Chun, B., and Guldmann, J.-M. (2014). Spatial Statistical Analysis and Simulation of the Urban Heat Island in High-Density Central Cities. Landsc. Urban Plan. 125, 76–88. doi:10.1016/j.landurbplan.2014.01.016
D’ambrosio Alfano, F. R., Palella, B. I., and Riccio, G. (2013). On the Transition Thermal Discomfort to Heat Stress as a Function of the PMV Value. Ind. Health 51 (3), 285–296. doi:10.2486/indhealth.2012-0163
Dousset, B., and Gourmelon, F. (2003). Satellite Multi-Sensor Data Analysis of Urban Surface Temperatures and Landcover. ISPRS J. Photogrammetry Remote Sens. 58 (1–2), 43–54. doi:10.1016/S0924-2716(03)00016-9
Feng, H., Zhao, X., Chen, F., and Wu, L. (2014). Using Land Use Change Trajectories to Quantify the Effects of Urbanization on Urban Heat Island. Adv. Space Res. 53 (3), 463–473. doi:10.1016/j.asr.2013.11.028
Gallo, K. P., McNab, A. L., Karl, T. R., Brown, J. F., Hood, J. J., and Tarpley, J. D. (1993). The Use of NOAA AVHRR Data for Assessment of the Urban Heat Island Effect. J. Appl. Meteor. 32 (5), 899–908. doi:10.1175/1520-0450(1993)032<0899:TUONAD>2.0.CO;2
Geletič, J., Lehnert, M., Dobrovolný, P., and Žuvela-Aloise, M. (2019). Spatial Modelling of Summer Climate Indices Based on Local Climate Zones: Expected Changes in the Future Climate of Brno, Czech Republic. Clim. Change 152, 487–502. doi:10.1007/s10584-018-2353-5
Goddard, L., and Gershunov, A. (2020). Impact of El Niño on Weather and Climate Extremes. Clim. Data Monit. WCDMP-No. 72, 361–375. doi:10.1002/9781119548164.ch16
Grimmond, S. (2007). Urbanization and Global Environmental Change: Local Effects of Urban Warming. Geogr. J. 173 (1), 83–88. doi:10.1111/j.1475-4959.2007.232_3.x
Grossman-Clarke, S., Zehnder, J. A., Loridan, T., and Grimmond, C. S. B. (2010). Contribution of Land Use Changes to Near-Surface Air Temperatures during Recent Summer Extreme Heat Events in the Phoenix Metropolitan Area. J. Appl. Meteorology Climatol. 49 (8), 1649–1664. doi:10.1175/2010JAMC2362.1
Gumbo, B., Mlilo, S., Broome, J., and Lumbroso, D. (2003). Industrial Water Demand Management and Cleaner Production Potential: A Case of Three Industries in Bulawayo, Zimbabwe. Phys. Chem. Earth, Parts A/B/C 28 (20–27), 797–804. doi:10.1016/j.pce.2003.08.026
Gusso, A., Bordin, F., Veronez, M., Cafruni, C., Lenz, L., and Crija, S. (2014). “Multitemporal Analysis of Thermal Distribution Characteristics for Urban Heat Islands Management,” in The 4th World Sustainability Forum, f009. doi:10.3390/wsf-4-f009
Haghighat, F. (2002). Thermal Confort in Housing and Thermal Environments, Sustainable Built Environment 1
Hallegatte, S., and Corfee-Morlot, J. (2011). Understanding Climate Change Impacts, Vulnerability and Adaptation at City Scale: An Introduction. Clim. Change 104 (1), 1–12. doi:10.1007/s10584-010-9981-8
Harlan, S. L., and Ruddell, D. M. (2011). Climate Change and Health in Cities: Impacts of Heat and Air Pollution and Potential Co-benefits from Mitigation and Adaptation. Curr. Opin. Environ. Sustain. 3 (3), 126–134. doi:10.1016/j.cosust.2011.01.001
Hwang, R.-L., Lin, T.-P., and Matzarakis, A. (2011). Seasonal Effects of Urban Street Shading on Long-Term Outdoor Thermal Comfort. Build. Environ. 46 (4), 863–870. doi:10.1016/j.buildenv.2010.10.017
Imhoff, M. L., Zhang, P., Wolfe, R. E., and Bounoua, L. (2010). Remote Sensing of the Urban Heat Island Effect across Biomes in the Continental USA. Remote Sens. Environ. 114 (3), 504–513. doi:10.1016/j.rse.2009.10.008
Jalan, S., and Sharma, K. (2014). Spatio-temporal Assessment of Land Use/ Land Cover Dynamics and Urban Heat Island of Jaipur City Using Satellite Data. Int. Arch. Photogramm. Remote Sens. Spat. Inf. Sci. XL-8 (1), 767–772. ISPRS Archives. doi:10.5194/isprsarchives-XL-8-767-2014
Jawak, S. D., and Luis, A. J. (2013). Improved Land Cover Mapping Using High Resolution Multiangle 8-band WorldView-2 Satellite Remote Sensing Data. J. Appl. Remote Sens. 7 (1), 073573. doi:10.1117/1.jrs.7.073573
Kruger, A. C. (2006). Observed Trends in Daily Precipitation Indices in South Africa: 1910 – 2004. Int. J. Climatol. 2285 (June), 2275–2285. doi:10.1002/joc10.1002/joc.1368
Kruger, A. C., and Sekele, S. S. (2012). Trends in Extreme Temperature Indices in South Africa: 1962-2009. Int. J. Climatol. 33, 661–676. doi:10.1002/joc.3455
Mbithi, D. M., Demessie, E. T., and Kashiri, T. (2010). The Impact of Land Use Land Cover (LULC) Changes on Land Surface Temperature (LST); a Case Study of Addis Ababa City, Ethiopia Kenya Metrological Services 192, 10400.
McMichael, A. J., Woodruff, R. E., and Hales, S. (2006). Climate Change and Human Health: Present and Future Risks. Lancet 367 (9513), 859–869. doi:10.1016/S0140-6736(06)68079-3
Meng, X., Currit, N., Wang, L., and Yang, X. (2010). “Object-oriented Residential Building Land-Use Mapping Using Lidar and Aerial Photographs,” in American Society for Photogrammetry and Remote Sensing 2010 Annual Conference, 2, 26–30. San Diego
Mitraka, Z., Del Frate, F., Chrysoulakis, N., and Gastellu-Etchegorry, J.-P. (2015). “Exploiting Earth Observation Data Products for Mapping Local Climate Zones,” in 2015 Joint Urban Remote Sensing Event (JURSE), Lausanne, Switzerland, 30 March 2015 - 01 April 2015, 1–5. doi:10.1109/JURSE.2015.7120456June 2013
Mónica, S., and Santos, F. (2011). Trends in Extreme Daily Precipitation Indices in Northern of Portugal. Geophys. Res. Abstr. 13, 11285.
Mouat, D. A., Mahin, G. G., Lancaster, J., Mouat, D. A., Mahin, G. G., and Lancaster, J. (1993). Remote Sensing Techniques in the Analysis of Change Detection. Geocarto Int. 8 (2), 39–50. doi:10.1080/10106049309354407
Muchingami, I., Hlatywayo, D. J., Nel, J. M., and Chuma, C. (2012). Electrical Resistivity Survey for Groundwater Investigations and Shallow Subsurface Evaluation of the Basaltic-Greenstone Formation of the Urban Bulawayo Aquifer. Phys. Chem. Earth, Parts A/B/C 50-52, 44–51. doi:10.1016/j.pce.2012.08.014
Mushore, T. D., Mutanga, O., Odindi, J., and Dube, T. (2018). Determining Extreme Heat Vulnerability of Harare Metropolitan City Using Multispectral Remote Sensing and Socio-Economic Data. J. Spatial Sci. 63 (1), 173–191. doi:10.1080/14498596.2017.1290558
Mutengu, S., Hoko, Z., and Makoni, F. S. (2007). An Assessment of the Public Health Hazard Potential of Wastewater Reuse for Crop Production. A Case of Bulawayo City, Zimbabwe. Phys. Chem. Earth, Parts A/B/C 32 (15–18), 1195–1203. doi:10.1016/j.pce.2007.07.019
Nayak, S., and Mandal, M. (2019). Impact of Land Use and Land Cover Changes on Temperature Trends over India. Land Use Policy 89 (8), 104238. doi:10.1016/j.landusepol.2019.104238
Nurwanda, A., and Honjo, T. (2020). The Prediction of City Expansion and Land Surface Temperature in Bogor City, Indonesia. Sustain. Cities Soc. 52 (December 2018), 101772. doi:10.1016/j.scs.2019.101772
Nyamekye, C., Kwofie, S., Ghansah, B., Agyapong, E., and Boamah, L. A. (2020). Assessing Urban Growth in Ghana Using Machine Learning and Intensity Analysis: A Case Study of the New Juaben Municipality. Land Use Policy 99 (July), 105057. doi:10.1016/j.landusepol.2020.105057
Odindi, J., Mutanga, O., Abdel-Rahman, E. M., Adam, E., and Bangamwabo, V. (2017). Determination of Urban Land-Cover Types and Their Implication on Thermal Characteristics in Three South African Coastal Metropolitans Using Remotely Sensed Data. South Afr. Geogr. J. 99 (1), 52–67. doi:10.1080/03736245.2015.1117015
Ogunjo, S. T., Akinsusi, J. O., and Fuwape, I. A. (2021). “Trends in Extreme Temperature Indices over Lagos, Nigeria,” in 4th International Conference on Science and Sustainable Development (ICSSD 2020), Ota, Nigeria, 3-5 August 2020, 012003. doi:10.1088/1755-1315/655/1/012003IOP Conf. Ser. Earth Environ. Sci.655
Parnell, S., and Walawege, R. (2011). Sub-Saharan African Urbanisation and Global Environmental Change. Glob. Environ. Change 21 (Suppl. 1), S12–S20. doi:10.1016/j.gloenvcha.2011.09.014
Pérez-Andreu, V., Aparicio-Fernández, C., Martínez-Ibernón, A., and Vivancos, J.-L. (2018). Impact of Climate Change on Heating and Cooling Energy Demand in a Residential Building in a Mediterranean Climate. Energy 165, 63–74. doi:10.1016/j.energy.2018.09.015
Pielke, R. A., Pitman, A., Niyogi, D., Mahmood, R., McAlpine, C., Hossain, F., et al. (2011). Land Use/land Cover Changes and Climate: Modeling Analysis and Observational Evidence. WIREs Clim. Change 2 (6), 828–850. doi:10.1002/wcc.144
Popov, T., Gnjato, S., Trbić, G., and Ivanišević, M. (2018). Recent Trends in Extreme Temperature Indices in Bosnia and Herzegovina. Carpath. J. Earth Environ. Sci. 13 (1), 211–224. doi:10.26471/cjees/2018/013/019
Puliafito, S. E., Bochaca, F. R., Allende, D. G., and Fernandez, R. (2013). Green Areas and Microscale Thermal Comfort in Arid Environments: A Case Study in Mendoza, Argentina. Acs 03 (03), 372–384. doi:10.4236/acs.2013.33039
Rahimi, M., and Hejabi, S. (2017). Spatial and Temporal Analysis of Trends in Extreme Temperature Indices in Iran over the Period 1960-2014. Int. J. Climatol. 38, 272–282. doi:10.1002/joc.5175
Sajjad, H., and Ghaffar, A. (2019). Observed, Simulated and Projected Extreme Climate Indices over Pakistan in Changing Climate. Theor. Appl. Climatol. 137, 255–281. doi:10.1007/s00704-018-2573-7
Satterthwaite, D. (2008). “Climate Change and Urbanization: Effects and Impliactions for Urban Governance,” in United Nations Expert Group Meeting on Population Distribution, Urbanization, Internatl Migration and Development, New York, 21-23 January 2008. http://www.un.org/esa/population/meetings/EGM_PopDist/P16_Satterthwaite.pdf.
Sein, K. K., Chidthaisong, A., and Oo, a. K. L. (2018). Observed Trends and Changes in Temperature and Precipitation Extreme Indices over Myanmar. Atmosphere 9, 477. doi:10.3390/atmos9120477
Sensoy, S., Türkoğlu, N., Akçakaya, A., Ekici, M., and Demİrcan, M. (2013). “Trends in Turkey Climate Indices from 1960 to 2010,” in 6th Atmospheric Science Symposium, Istanbul, Turkey, April 24–26, 2013, 1–8. ATMOS 2013 3 - 5 Haziran 2013, İstanbul TRENDS.
Sithole, K., and Odindi, J. (2015). Determination of Urban Thermal Characteristics on an Urban/Rural Land Cover Gradient Using Remotely Sensed Data. SA J Geomatics 4 (4), 384. doi:10.4314/sajg.v4i4.3
Srivanit, M., Hokao, K., and Phonekeo, V. (2012). Assessing the Impact of Urbanization on Urban Thermal Environment: A Case Study of Bangkok Metropolitan. Int. J. Appl. Sci. Technol. 2 (7), 243–256. http://www.ijastnet.com/journals/Vol_2_No_7_August_2012/26.pdf.
Stathopoulos, T., Wu, H., and Zacharias, J. (2004). Outdoor Human Comfort in an Urban Climate. Build. Environ. 39 (3), 297–305. doi:10.1016/j.buildenv.2003.09.001
Stenseth, N. C., Ottersen, G., Hurrell, J. W., Mysterud, A., Lima, M., Chan, K. S., et al. (2003). Review Article. Studying Climate Effects on Ecology through the Use of Climate Indices: the North Atlantic Oscillation, El Niño Southern Oscillation and beyond. Proc. R. Soc. Lond. B 270 (1529), 2087–2096. doi:10.1098/rspb.2003.2415
Stewart, I. D., Oke, T. R., and Krayenhoff, E. S. (2014). Evaluation of the 'local Climate Zone' Scheme Using Temperature Observations and Model Simulations. Int. J. Climatol. 34 (4), 1062–1080. doi:10.1002/joc.3746
Stewart, I. D., and Oke, T. R. (2012). Local Climate Zones for Urban Temperature Studies. BAMS 93 (12), 1879–1900. doi:10.1175/BAMS-D-11-00019.1
Tao, Z., Santanello, J. A., Chin, M., Zhou, S., Tan, Q., Kemp, E. M., et al. (2013). Effect of Land Cover on Atmospheric Processes and Air Quality over the Continental United States - a NASA Unified WRF (NU-WRF) Model Study. Atmos. Chem. Phys. 13 (13), 6207–6226. doi:10.5194/acp-13-6207-2013
Thatcher, M., and Hurley, P. (2012). Simulating Australian Urban Climate in a Mesoscale Atmospheric Numerical Model. Boundary-Layer Meteorol. 142 (1), 149–175. doi:10.1007/s10546-011-9663-8
Toudert, F. A. (2005). Dependence of Outdoor Thermal Comfort on Street Design in Hot and Dry Climate. Berichte des Meteorologischen Institutes der Universität Freiburg Fazia Ali Toudert. Nr. 15(15). Available at: http://www.freidok.uni-freiburg.de/volltexte/2005/2078/.
Touré, H. A., Kalifa, T., and Kyei-Baffour, N. (2017). Assessment of Changing Trends of Daily Precipitation and Temperature Extremes in Bamako and Ségou in Mali from 1961- 2014. Weather Clim. Extrem. 18, 8–16. doi:10.1016/j.wace.2017.09.002
Uddin, S., Al Ghadban, A. N., Al Dousari, A., Al Murad, M., and Al Shamroukh, D. (2010). A Remote Sensing Classification for Land-Cover Changes and Micro-climate in Kuwait. Int. J. SDP 5 (4), 367–377. doi:10.2495/SDP-V5-N4-367-377
Unganai, L. (1996). Historic and Future Climatic Change in Zimbabwe. Clim. Res. 6 (2), 137–145. doi:10.3354/cr006137
U.S. Geological Survey (2019). Landsat 8 Data Users Handbook, 8, 97. Nasa. https://landsat.usgs.gov/documents/Landsat8DataUsersHandbook.pdf.
Vincent, L. A., Aguilar, E., Saindou, M., Hassane, A. F., Jumaux, G., Roy, D., et al. (2011). Observed Trends in Indices of Daily and Extreme Temperature and Precipitation for the Countries of the Western Indian Ocean, 1961-2008. J. Geophys. Res. 116, 1–12. doi:10.1029/2010JD015303
Vlassova, L., Perez-Cabello, F., Nieto, H., Martín, P., Riaño, D., and de la Riva, J. (2014). Assessment of Methods for Land Surface Temperature Retrieval from Landsat-5 TM Images Applicable to Multiscale Tree-Grass Ecosystem Modeling. Remote Sens. 6 (5), 4345–4368. doi:10.3390/rs6054345
Weng, Q., Liu, H., and Lu, D. (2007). Assessing the Effects of Land Use and Land Cover Patterns on Thermal Conditions Using Landscape Metrics in City of Indianapolis, United States. Urban Ecosyst. 10 (2), 203–219. doi:10.1007/s11252-007-0020-0
Wu, S., Mickley, L. J., Kaplan, J. O., and Jacob, D. J. (2012). Impacts of Changes in Land Use and Land Cover on Atmospheric Chemistry and Air Quality over the 21st Century. Atmos. Chem. Phys. 12 (3), 1597–1609. doi:10.5194/acp-12-1597-2012
Yang, J. S. (2004). Estimation of Land Surface Temperature Using Spatial Interpolation and Satellite-Derived Surface Emissivity. J. Env. Inf. 4 (1), 40–47. doi:10.3808/jei.200400035
Zhang, X., and Yang, F. (2004). RClimDex (1.0) User Manual. Canada: Climate Research Branch Environment.
Zhao, Q., Myint, S., Wentz, E., and Fan, C. (2015). Rooftop Surface Temperature Analysis in an Urban Residential Environment. Remote Sens. 7 (9), 12135–12159. doi:10.3390/rs70912135
Zhou, X., and Wang, Y.-C. (2011). Dynamics of Land Surface Temperature in Response to Land-Use/Cover Change. Geogr. Res. 49 (1), 23–36. doi:10.1111/j.1745-5871.2010.00686.x
Zhou, Y., Weng, Q., Gurney, K. R., Shuai, Y., and Hu, X. (2012). Estimation of the Relationship between Remotely Sensed Anthropogenic Heat Discharge and Building Energy Use. ISPRS J. Photogrammetry Remote Sens. 67 (1), 65–72. doi:10.1016/j.isprsjprs.2011.10.007
Zubler, E. M., Scherrer, S. C., Croci-Maspoli, M., Liniger, M. A., and Appenzeller, C. (2014). Key Climate Indices in Switzerland; Expected Changes in a Future Climate. Clim. Change 123, 255–271. doi:10.1007/s10584-013-1041-8
Zvigadza, S., Mharadze, G., and Ngena, S. (2010). “Communitie and Climate Change: Building Local Capacity for Adaptation in Goromonzi District, Munyawiri Ward, Zimbabwe,” in African Centre for Technology Studies, June, 1–16. Available at: https://www.africaportal.org/documents/13932/CBAA_Zimbabwe.pdf
Keywords: LCZ, climate change, temperature extremes, hot days, cold nights, extreme climate indices
Citation: Mushore TD, Mutanga O and Odindi J (2022) Understanding Growth-Induced Trends in Local Climate Zones, Land Surface Temperature, and Extreme Temperature Events in a Rapidly Growing City: A Case of Bulawayo Metropolitan City in Zimbabwe. Front. Environ. Sci. 10:910816. doi: 10.3389/fenvs.2022.910816
Received: 01 April 2022; Accepted: 01 June 2022;
Published: 07 July 2022.
Edited by:
Zhaowu Yu, Fudan University, ChinaReviewed by:
Yongzhu Xiong, Jiaying University, ChinaYuanhui Zhu, Arizona State University, United States
Copyright © 2022 Mushore, Mutanga and Odindi. This is an open-access article distributed under the terms of the Creative Commons Attribution License (CC BY). The use, distribution or reproduction in other forums is permitted, provided the original author(s) and the copyright owner(s) are credited and that the original publication in this journal is cited, in accordance with accepted academic practice. No use, distribution or reproduction is permitted which does not comply with these terms.
*Correspondence: Terence Darlington Mushore, dGRtdXNob3JlQGdtYWlsLmNvbQ==