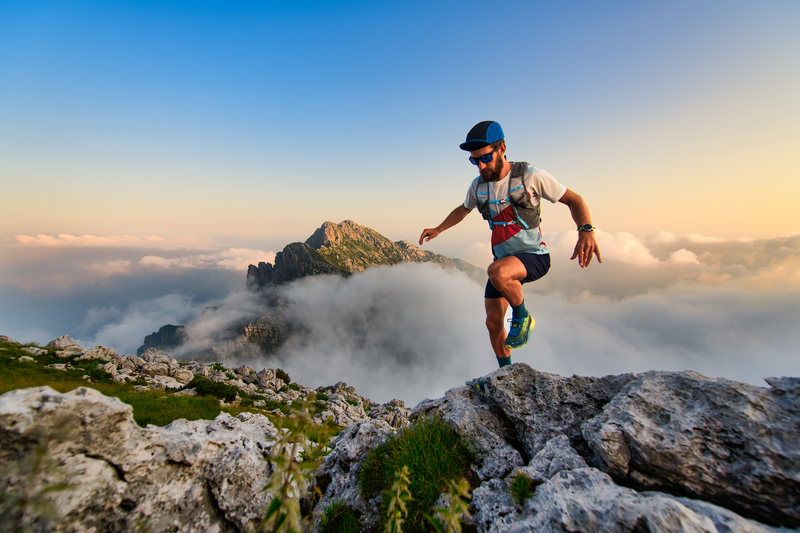
95% of researchers rate our articles as excellent or good
Learn more about the work of our research integrity team to safeguard the quality of each article we publish.
Find out more
ORIGINAL RESEARCH article
Front. Environ. Sci. , 31 May 2022
Sec. Environmental Economics and Management
Volume 10 - 2022 | https://doi.org/10.3389/fenvs.2022.909082
This article is part of the Research Topic Economic Development, Social Consequences, and Technological Innovation Under Climate Change COVID-19 Pandemic Conditions View all 48 articles
Low-carbon energy transformation increases energy efficiency, and environmental regulation is necessary for carbon neutrality. Energy efficiency is widely accepted As a cost-effective method of reducing air pollution and improving the economic performance of manufacturing industries. In order to make their manufacturing sectors more energy-efficient, a large number of countries have implemented legislation. It is possible to tailor these interventions to a specific company or industry by using measures to control and direct interventions in the marketplace. These policies might be beneficial, but the experiential study of the impacts of these interferences on energy-saving technology acceptance has not been conclusive. Many environmental policy measures have an effect on the level of the asset in energy efficiency machinery. The years 2010–2019 are covered in this research, which compiles a database of Chinese industries from various sources. As a result, we have access to a wide range of factors and strategy devices that could influence the adoption of energy efficiency measures. Regulation, taxes, subsidies, and exemptions are among the instruments we will examine. These findings support the development of environmental regulations in China that will further improve energy efficiency and carbon neutrality.
Since its inception as a hidden fuel, energy efficiency has been elevated to the first fuel in a maintainable worldwide energy system, says the International Energy Agency (Chandio et al., 2021; Hao et al., 2021; Tanveer et al., 2021). Energy efficacy is at the topmost of both international and national policy and science schedules because it provides significant and growing benefits to all sectors of the economy (Irfan et al., 2019, 2020; Madurai Elavarasan et al., 2021). There are numerous economic and social advantages to strategies meant to increase energy efficacy and the more conventional approaches to decrease utilization, save money, and reduce airborne pollution (Elavarasan et al., 2021; Irfan and Ahmad 2021; Irfan et al., 2021). In energy supply, a reduction in imported energy dependence can significantly influence energy security (Rehman et al., 2020; Işık et al., 2021; Yang et al., 2021). Additionally, the rise in international energy raw material prices, exacerbated by the decrease in energy consumption, contributes to lower inflationary tensions (Dagar et al., 2021; Jinru et al., 2021; Fang et al., 2022). In order to summarize: Increased efficiency and reliability in the sector can positively impact the macroeconomic environment, from increasing economic activity to creating more jobs to improving the health and well-being of employees, all of which directly influence the economy (Li L. et al., 2021).
The Chinese management has set aspiring goals for energy competence reduction for 2020 and 2030. (at least 20.0% and 32.50% developments in energy efficacy likened to a baseline, correspondingly) (Quan et al., 2021; Yu et al., 2022). As the industrial sector accounts for 25% of final energy preservation and 15% of worldwide air secretions, meeting these targets will require contributions from all energy-consuming sectors (Shao et al., 2021), even though businesses might be enticed to finance energy-competent technologies because of government incentives. Research has shown a space amid the publicly ideal level of an asset in power-saving skills and the quantities invested in reducing costs and becoming more environmentally friendly (Dorsey-Palmateer and Niu 2020). The gap in energy efficiency is known as the efficacy gap. Based on their evaluation of the experiential fiction on the drivers of energy efficacy in industrial organizations (Wang and Luo 2022), conclude that the most crucial reason for organizations to finance energy efficacy is still unclear. According to the authors, researchers are unable to agree on the primary drivers of energy efficiency because of their multidisciplinary approach (Pan and Chen 2021).
Additionally, environmental regulations (ERs) also offer external inducements for the industry to modify its manufacturing procedures in accordance with some restrictions imposed on firms by governments. Scientists have found some advantages to environmental regulations, but theyhave also found some drawbacks. Ai et al. (2021) found that while environmental regulations effectively encourage green technology innovation in trade, such a revolution will move to arise in the prices associated with new product development and, thus, a reduction in the inclination of the industry to innovate. According to (Liu L. et al., 2021), strict environmental regulations directly influence the willingness of international stockholders to enter the local market place in the first place because they raise the environmental prices of innovation. There is a consensus that the government should implement appropriate environmental regulations (ERs) to increase the efficacy of green technology innovation in the industry, but this is not universally agreed upon (Qudrat-Ullah and Nevo 2021).
Green innovation efficacy (GIE) is a metric used to assess how effectively the industry utilizes resources when developing green technologies. Resources invested vs. benefits received are commonly referred to as a return on investment. In order for the industry to better assign funds, gain more profits with a small number of assets, and lower the costs of innovation while maintaining or improving productivity, innovation efficiency must be improved (Xing et al., 2021). Since ERs and GIE have been linked in previous studies e.g., (Andrei et al., 2021), it is clear that more research is needed to grasp how ERs and GIE are interrelated fully. The real-world effects of various ER types and the variety of ER characteristics make it difficult to generalize about ERs.
Since the modification and inaugural strategy, China’s budget has increased and reached significant fall outs. On the other hand, this rapid expansion was fueled by pollution and energy consumption that were out of control. China will accelerate the construction of a resource-saving and environmentally friendly society, according to the Central Committee’s Fifth Plenary Session of the 18th CPC, which met in Beijing. In affirmation of previous decisions, this proposal was a part of implementing a strategy for sustainable development and promoting synchronization between humans and nature (Li et al., 2018; Hu et al., 2022). In order to build a society that is both resource-efficient and environmentally friendly, solid environmental regulation is essential. While improving the environment, energy consumption can be controlled using analytical and active regulatory tools (Andersson et al., 2020). However, it remains to be seen whether China’s energy efficiency will improve due to stricter environmental regulations or as a result of technological advancements. It is essential to understand the relationship between technological innovation and environmental regulation and energy to formulate effective environmental regulation policies. Previous studies have examined environmental regulation, technological innovation, and energy competence separately. Understanding the interrelation between environmental regulation, technological innovation, and energy efficacy has proven difficult due to the scarcity of studies. For this reason, to attain our energy-saving goals through the advancement of technological innovation and energy efficacy, we will investigate the association between environmental regulation, innovation, and effectiveness and their intersection.
A significant contribution is made in two ways. Incentives available in the marketplace environmental regulation and command and control over the environment are subcategories. Once we’ve built a connection amid environmental regulation based on command and market incentives, along with technological advancements and energy efficiency within the same framework, we used direct acyclic graph (DAG) methods to extract three conduct pathways for each of the four variables. Another approach is to utilize a Tobit regression model to track the long-term trends in the command control has a significant impact on the environment regulations, technological innovation, market incentives for environmental regulation, and energy efficacy are four regulated variables.
The remaining article is arranged as follows: For the construction industry and emergency response systems, Section 2 examines the literature on GIE and its impact on these industries. Before moving on to Section 4, Section 3 provides an overview of datasets, indicators/variables, and the data analysis process. Outcomes of the GIE study and regression are written in Section 5 in great detail. Section 6 explains the implications of these findings. Conclusions and upcoming study directions are debated in Section 7 of the paper.
As far as assessing energy efficiency, single-factor and total-factor methods are the most commonly used. Single-factor energy efficacy has two subcategories: intensity and productivity (see Figure 1). The ratio of energy input to GDP multiplied by the term energy intensity is known as energy productivity (Desvallées 2022). Because of its simplicity, this approach has been used by a large number of scientists in their investigations into energy efficacy and the factors that affect it. By applying this method, the energy intensity trends in the number of major OECD countries since 1973 were estimated by Zheng et al. (2021). For their study, they focused on implementing energy efficacy strategies in the United States, Japan, and Western Europe. The findings are parallel to (M. Wang and Feng 2020), who used panel data spanning 2000 to 2009 to examine how different economic structures, consumption patterns, and technological advancements impacted energy intensity in other parts of Eastern Asia. The impact of government spending on energy intensity was examined by (D. Q. Zhou et al., 2016) using regional panel information. According to the researchers, in spite of regional economic discrepancies, the effect remained significant. Conclusions drawn from these findings include the significance of analyzing single-factor efficiencies for economic growth and the extent to which it is energy-dependent (Martelli et al., 2020). Aside from energy, other important factors such as labor and capital are overlooked when this method is examined from the perspective of the entire production process. Since it doesn’t give a complete picture of energy efficiency, structural changes may result in lower energy intensity, but the energy efficiency may not change. A region’s or an industry’s increase in energy efficiency cannot be estimated using this method because it is also affected by the energy value (Khalfaoui et al., 2019, 2021; Sarwar 2019; Sarwar and Alsaggaf 2020; Waheed et al., 2020).
Using (Mehta et al., 2019) energy efficiency concept, which incorporates facets aside from energy consumption like labor and investment into the single-factor efficacy, the concept of total-factor energy efficacy is proposed. This idea came about due to the problems with traditional single-factor energy efficacy. The entire feature energy efficacy of member countries of the Asia-Pacific Economic Cooperation (APEC) countries amid 1991 and 2000 (Su and Urban 2021) found that meeting the energy-saving target without decreasing GDP could be discussed. To find out why China’s energy efficacy is so low, (Jebali et al., 2017), calculated the efficacy of energy from China’s petroleum, char, and sources of clean, renewable power from 1998 to 2010. Due to a low GIE, they concluded that this was the main problem. According to this table, using total-factor energy efficacy improved the exactness of energy efficacy assessments, but the studies above only measured earnings, such as GDP, when determining efficiency levels. As more people become concerned about climate change and environmental issues like carbon dioxide emissions, scientists are beginning to consider the negative consequences. A growing number of academics believe that when evaluating output factors, it is necessary to consider both predictable earnings and unpredicted outputs (toxins like carbon dioxide and sulfur dioxide). As part of their investigation into China’s energy efficiency, (Ren et al., 2020), measured and then planned the total factor energy of 29 Chinese shires between 1997 and 2011. According to (Wu et al., 2021), this study examined the ability to utilize all of a system’s energy sources and latent of China’s provincially based industries from 2000 to 2014, focusing on technological differences and management practices, and scale. Using data from 2008 to 2012, Adua (2021) discovered that the vast majority of Chinese cities are inefficient energy consumers. Almost all academics agree that carbon dioxide emissions are primarily responsible for the adverse effects of human activity. As a result of the manufacturing process, pollutants such as Sulphur dioxide and nitrogen dioxide will be out. Despite the widespread belief that these gases are the primary contributors to warming the planet, acid rain, and diminishing the ozone layer, only a few researchers (Li et al., 2021b) have found their harmful emissions.
In order to examine the relative efficacy of multiple inputs and outputs, the DEA is the most widely used method for calculating total-factor energy efficacy. Total-factor energy efficacy is most commonly calculated using the DEA method. Because the traditional DEA model results differ depending on whether the starting point is a constant or variable scale earnings model. It cannot be used to compare multiple effective units at once. Instead, it employs the CCR and BCC models, both of which have varying scale earnings. In order to improve the model, researchers constantly tweak it. Sedlmeir et al. (2020) devised a super-efficiency DEA model to overcome the drawbacks of traditional DEA models, allowing them to determine the efficiency difference between efficient units as well as the effective order in which decision-making units should be placed. Calculate environmental efficiency in 31 Chinese provinces between 2000 and 2010. Fan et al. used this model to analyze the energy competence of carbon dioxide utilization skills in China (Sun et al., 2020).
Scholars assessing China’s energy competence in specific industries or at the microscopic area level have paid little attention to the country’s energy efficiency. (Gondal et al., 2017) calculated the scale incomes and indemnities of energy efficacy in different regions of China between 2006 and 2010 and provided China’s government with strategies and policies to implement. Researchers in 29 Chinese provinces used the three-dimensional panel econometric model between 1997 and 2011 to examine cluster and influence factors and total-factor energy competence. Between 2002 and 2010, city trade in Beijing, Chongqing, Shanghai, and Tianjin increased emission loads and geological sources, according to a study by (Kiara 2013). Environmental total-factor energy efficiency in China’s industries that use a lot of energy from 2000 to 2013 was significantly lower in four sub-departments than the national average (Zhuo and Qamruzzaman 2022). Li et al. (2021c) used state-owned coal-fired power plants as a case study and calculated how much energy could be saved and how much CO2 could be avoided. Similar studies were carried out by (Huang et al., 2022; Liu et al., 2022; Wen et al., 2022). China’s coal consumption was examined at the regional and industrial levels using the DEA-Malmquist index. Based on an industrial utilization perspective, the DEA-Malmquist model was used in this study. Furthermore, it explains why China’s coal consumption efficiency has fluctuated over time from 2006 to 2015.
Significant research is being done into improving GIE for use in industry (Luo Q. et al., 2019), nology innovation (Wang et al., 2021). In other words, the GIE is a measure of the industry’s ability to utilize available innovation resources and resources. To see if an industry can get the most out of a given level of investment, it uses this formula (Abdul-Rahaman et al., 2021).
The GIE, on the other hand, needs to be improved in order to promote long-term industry growth (Hodson et al., 2018). The performance of the GIE has so far been assessed using a variety of schemes based on indicators developed by researchers. For example (Dias et al., 2022), examined the practical green technology innovation process and developed a system based on administration, process, technological, and product innovation indicators. Q. Wang et al. (2019) discussed China’s green technology innovation environmental factors and projected a system that included non-expected productions (CO2, SO2, etc.).
In addition, prior studies intensive on the variables that affect the GIE score. To study GIE in the high-end industrial industry (Horbach et al., 2012; Saguy 2022), developed an SFA (Stochastic Frontier Analysis) model, which they then applied to GIE in the automotive industry. According to their findings, all of these variables had a substantial influence on GIE: government funding, company size; maturity of the market; and industrial agglomeration. Technology spillover in reverse effects on GIE is primarily determined by the institutional environment, as (Deng et al., 2021) noted in their study.
While previous research on GIE has failed to account for the staggered nature of GTI fully, it becomes clear when reviewing previous literature on the subject. GTI is typically thought of as a one-step process. This simplification may lead to inaccuracies in the estimation of efficiency. Technology innovation resources are transformed into technological R&D accomplishments through the multi-stage process known as the Global Technology Innovation Process (GTI). The company can then reap the benefits of these technological innovations and R&D accomplishments in the form of financial gains (Khan et al., 2021). It necessitates the division of green technology innovation into various phases in order to assess GIE fairly.
Industrial growth and economic growth could be stifled by environmental pollution, a global problem (Isaksen and Trippl 2017). In order to encourage long-term fiscal growth, nationwide administrations have devised a number of strategy devices known as economic development instruments (ERs) (Xie et al., 2019).
The term environmental regulations (ERs) are used to describe a broad range of legislation to protect the environment. Green innovation in the United States is also being driven by environmental regulations, according to (Khalil et al., 2021). To address industrial pollution, environmental remediation (ER) is a highly effective method (K. Du et al., 2021). Environmental regulatory agencies (ERs) can use mandatory measures like environmental taxes to limit pollutant emissions. It is expected that businesses will voluntarily reduce their pollution emissions in order to avoid paying the extra tax imposed by the environmental contamination. Companies must pay a tax on the environment for polluting the environment.
In the industrial sector, ERs can also recover or avert GIE (Dias et al., 2022). On the one hand, manufacturing will typically bear the bulk of the costs associated with innovation; on the other side, it will not always reap the benefits of innovation (H. Khan et al., 2022). This marvel is referred to as the positive externality of innovation. Governments frequently make efforts to rouse manufacturing via subsidies and other resources in order to reduce the adverse effect of positive outwardness on GTI (Dong et al., 2022). As an alternative argument, the industry must continuously recover its GIE and make the most of the profits of innovation in order to decrease the high price of innovation (Anser et al., 2020).
Only a few studies have been done to confirm the consequence of ERs on GIE. An investigation into the United Kingdom by (Luo S. et al., 2019) found that ERs could only improve GIE in the industry under certain circumstances. There is no clear linear relationship between ERs and GIE, as (J. Wang 2011; Zhu et al., 2021) stated. Although ERs initially inhibit GIE, the intensity of these ER increases after an inflection point, according to (Lemieux et al., 2021). As a whole, previous studies have not yielded a definitive answer, and there appear to be discrepancies between the findings of different studies.
There are numerous ways to distinguish ERs from other types of cells. There is no doubt that the industrial Environment is affected differently by different ERs (García-Pérez-de-Lema et al., 2021; Zheng et al., 2021). According to the government’s mandates, three kinds of environmental regulations (ERs) fall into three categories: volunteer regulation, market-based environmental regulation, and command and control environmental regulations (Bayarçelik et al., 2014; Liu Y. et al., 2021). As a result, rather than combining the effects of different ER types, it is necessary to examine each one separately to assess the influence of ERs on GIE accurately.
Unlike traditional economic methods such as ratio analysis or regression, data envelopment analysis (DEA) is considered a more active tool for measuring efficiency in business operations. Efficiency has been defined by a number of academics in this particular context. In this research, we consider (Tang et al., 2019) definition of productivity, which was derived from the research (Curtis and Lee 2019) and is used to define the measure of efficacy comprised of several inputs. According to (Xu and Lin 2020), an organization’s efficiency comprises two gears: technical efficacy and a locative efficacy (or allocation efficiency). Practical efficacy is defined as the ratio of the optimal input to the actual input in input-oriented efficiency measurement. It is defined as the % of the actual output to the optimal output in output-oriented efficiency measurement.
On the other hand, a locative efficiency demonstrates an organization’s ability to utilize its inputs to the greatest extent possible in light of its prices and technological capabilities. The production Frontier or cost Frontier is used to determine the optimal output and input for Decision-Making Units (DMUs) based on the objectives of Decision-Making Units (DMU). In this regard, two approaches are recommended in the literature: a parametric approach and a non-parametric approach. The Frontier of the parametric approach is assigned an efficient method. In contrast, the Frontier of the non-parametric method is not assigned a functional method and instead is defined by the preceding specification. To develop the DEA model for measuring the efficiency of a single DMU for the first time, Lin and Xu (2020) used a non-parametric approach, which the authors of the paper followed. They devised an input-oriented DEA model that assumed a constant return to scale as a starting point. Following studies of the DEA model, on the other hand, take into account a variety of assumptions. The variable returns to scale (VRS) concept was projected by (Harrison et al., 2017), who used mathematical programming in his DEA model to generate a linear best practice Frontier based solely on experimental input-output data. This new approach of the DEA has gained a wider acceptance for measuring the efficiency of different DMUs across industries or countries than the previous approach (Zhang et al., 2020).
A relational and non-rational decrease in contribution was used in two applied DEA variants. In order to calculate hospital efficacy from both DEA variants, an input-oriented TE model with inconstant revenues to scale is quantified. A vector of inputs I = 1, 2, … … m inputs) is formed by n numbers of firms (j = 1, 2, n) using y j = y 1, y 2, … , y J as outputs. x_j = x_1,x_2, … ,x_J; This is the input bundle used by the jth hospital (where j is a number). There is a production relation between the set of inputs and outputs.
where P comprises all possible output and inputs package, the efficacy of jth DMU with the input-output pack
Subject to
The linear programming model described above is used to reduce all inputs proportionally to a given output level. The technical efficiency of a facility is measured by a proportionate reduction in all its inputs based on scaled-down inputs to get to a certain point (where represents the scalar magnitude). The efficacy notch of the hospital under assessment can be planned as the proportion of the negligible input with mention of the built border to the actual input in the hospital under evaluation after resolving the optimization problem. The minimal input requirement includes the proportionally reduced input and some types of denim related to input as a result of the only relative decrease (Ngo 2022). To indicate radial efficiency, the score is denoted by the symbol *. Efficiency equals TE (* = *) because there is no slack in the system with respect to the input. On the other hand, radial efficiency is limited in its ability to provide a comprehensive measure because it only considers the reduction in inputs proportional to the reduction in output.
Researchers are moving toward the submission of a DEA with a non-circular variation, which is founded on non-relational alteration, to increase greater precision and biased power. In order to circumvent the difficulties associated with the radical approach, the slack-based measure (SBM) of efficacy approximation has been projected by (Xiang et al., 2022) in order to achieve greater biased power and wanted scientific properties. Achieving the maximum amount of loss linked with the outputs and inputs used in the manufacturing process to account for the various features of non-proportional lessening is at the heart of this method. One way to look at it is as a by-product of incompetence caused by equally the inputs and outputting factors combined. You can find the derivation of the SBM of competence, along with its measured properties, in (Ye and Wang 2019). The following is a description of the model, which is the SBM of technical efficiency.
Here, the output vector Y and input matrix X have slacks associated with them. The Charnels-Cooper conversion, used in the (Guo and Yuan 2020) CCR model, can quickly transform the fractional form of the above SBM model into a simple linear programming optimization.
Energy use can be alienated into two broad classes: energy input and energy output. There are two subcategories within each of these categories: total and single-factor energy inputs. Each of these categories can be broken down into desirable and undesirable outcomes. The GIE, which includes coal input and output, is the primary focus of this investigation (Khoshnevisan et al., 2013). There are two types of models: one considers only the country’s economic output and the other looks at both the greenhouse gas emissions and the pollution caused by coal consumption in terms of air emitted. The following is an illustration of an input-output indicator:
This system is composed of three distinct components. Begin by looking at the coal standby input index, which measures the amount of standard coal used per 1,000 metric tones of coal consumed. The second index measures the output of coal resources. It is equivalent to one hundred million dollars in Chinese currency; the capital input index is another index that is commonly used. In the end, the output metric is the only metric. According to Yijun Zhang and Song (2021), a fixed asset’s current value is equal to its original value minus its accumulated depreciation value, calculated using the 2005 Fixed Investment Price Index. In other words, 1,000,000 people are equal to 10,000 employees and are expressed as the whole number employed in a specified year. Another way to look at it is to compare all employees’ beginning and ending mean values.
System for showing the results of a task. There are two parts to this system. As a starting point, we can look at the industry’s revenue in 100-million-yuan units, which is known as the financial output index. (Pardo-Bosch et al., 2019) use the 2010 ex-factory price index of manufacturing makers to deflate the impressive statistics from the China Industry Statistical Yearbook to arrive at the final value. Index of environmental and climate change impact also among undesirable outputs (also referred to as the unwanted output). NOX, SO2, and CO2emissions are added together to calculate this index, which is expressed in tones per ten thousand tones of CO2. There are three main factors that led to the selection of these three outputs, according to (Martínez-Moya et al., 2019). The three most concerning aspects of air pollution are acid rain, ozone exhaustion, and global warming. According to popular belief, these problems are caused by too much CO2, SO2, and NOX being emitted into the atmosphere. China’s government has proposed emission reduction targets for the country, which has been the world’s main transmitter of NOX, SO2, and CO2.
The China Industry Statistical Yearbook (2010–2019) was used to gather industry-related data, including data on the industry’s capital stock, workforce, and sales value. 2010 IPCC guidelines for national greenhouse gas inventories were printed in 2010 and used to calculate the CO2 emissions. Following (X. Yu and Li 2020), we calculate carbon dioxide emissions by multiplying each type of energy consumption by its carbon emission coefficient and then totaling up the results to get the full carbon dioxide discharges. The China Energy Statistical Yearbook (2010–2019) was obtained from the Chinese government and used to calculate CO2 emissions. The China Statistical Yearbook on Atmosphere (2010–2019) was used to calculate SO2 emissions for the past 14 years. c) The China Statistical Yearbook on Environmental Statistics has published data on NOX emissions since 2011. This study follows Zeyun Li et al. (2022) and uses a variety of energy consumption factors and NOX emission factors to estimate NOX emissions for unobserved data. The original data are depicted in Table 1 with the descriptive statistics.
A panel of random effects Tobit’s regression is considered for the second stage of the analysis. In this step, the efficiency scores of the DEA gained in the first stage are generated as reliant on variables with the limited (0, 1) series. The Tobit regression model employed in this work is well-defined as follows:
Where
The maximum likelihood method is applied to estimate the Tobit regression. This model is used as a substitute for ordinary least squares regression. This model gives an effective result if the dependent variable is a limit or cut value. Numerous writers used this model by considering the DEA not chasing adequate to the interval (0, 1). The Tobit regression model fixes the issues with asymmetry that make the usage of ordinary least squares. However, academic arguments exist in relation to the functional appropriateness of this model in carrying out second stage examination. For example (Xiang et al., 2022), declares that Tobit regression is not the only method for modelling DEA scores, but in most cases, it generates valuable results. But (Pardo-Bosch et al., 2019), argues that as the DEA scores generated through Tobit contain fractions, it is not a suitable measure. He suggests that the ordinary least squares estimator is instead a reliable estimator. However, this study applies the Tobit model and a linear random-effects model to test the results’ statistical robustness.
In order to protect the environment, CERs must be applied by government sectors or environmental safety firms. CERs, or climate-related emissions reductions, are a standard tool in Chinese environmental regulation. According to previous research, the intensity of CER was previously measured by the number of new regulations enacted. Despite their best efforts, governments often fail to put into effect the legislation they pass, according to (Singhai and Sushil 2021). It was thus decided to use the annual number of environmental administrative penalty cases as an indicator to gauge government CER intensity in accordance with Xu and Lin (2016) recommendation. In the wake of the MER, governments and environmental agencies are able to use market-based mechanisms to switch industrial contamination. Before this study, the strength of MER in the Environment was estimated using contaminant expulsion charges. Since 2003, China’s pollutant expulsion charges system has been broadly applied all over the country’s various regions, and the system’s results have been thoroughly documented in the country’s official statistical yearbooks. China’s regional pollution expulsion payments are used as an indicator of the MER to calculate the MER. Vlontzos et al. (2014) says that by keeping an eye on the industry’s production practices, the public can help protect the environment. VER (China’s Environmental Responsibility) was measured by the number of letters of complaint received by the government regarding environmental pollution and related issues. People are more susceptible to changes in public opinion when they are in their immediate surroundings. Supporters of VER believe that the number of petitions received from the Ministry of Environmental science and Environment’s petitions office is a good indicator of the organization’s strength (China).
As an additional safeguard, the following control variables are employed: As a percentage of provincial GDP, GDP growth is measured by GDP (Gross Domestic Product), industrial growth is measured by IG (Industrial Development Potential), and technology innovation is measured by TI (Scale of Technological Innovation).
Figure 1 presents the average green innovation efficiency of carbon-intensive industries. China’s industrial GIE was estimated from 2010 to 2019 using the super-efficacy DEA model, and the outcomes are exposed in Table 2 of this research. It was clear that the GIE had changed significantly among the sample industries, and the overall trend was upward, from 1.00 to 1.16. According to (Rasoulinezhad and Taghizadeh-Hesary 2022) and the results of this study, which are summarized in Table 3, this was the case. As can be seen from this development, China’s coal consumption is shifting from a high to a low level. GIE growth in the coal industry has lagged behind China’s industrial sector, which has seen rapid increases in energy efficiency.
Because despite China’s significant efforts to promote discharges reduction and energy preservation over the last decade to confirm the consolidation of energy technology innovation and structure of a coal united energy structure, the Chinese government needs to emphasize char manufacturing. The total amount of energy consumed could be reduced, while air pollution and greenhouse gas emissions could be reduced if the coal industry’s energy efficiency improved. It would also have a positive impact on the environment.
The stationary test of the data is required in order to guarantee that the estimated consequences are valid and that pseudo-regression problems are avoided to the greatest extent possible. The ADF method is used for the root-cause analysis in this study, and the fallouts of the test are presented in Table 4. The root-cause analysis outcomes for GIE, TI, and CER are essential at the 1% confidence level, indicating that there is no unit root under the condition of the first alteration, which is the case in this study. However, while the unit roots of the variables in the first variance are significant at 1% after the second difference, the null hypothesis is rejected for all of the variables in this difference. Because all of the models’ orders are fixed, it is possible to observe that the difference between them is stationary. Furthermore, the second-order variance exam does not comprise any unit roots, indicating that it has a high level of stationary.
Performing a co-integration test on each variable is required in order to regulate whether or not a co-integration association exists among them. This is based on the results of the root-cause analysis, which indicates that the order of variable quantity in the replica has integration in the second order; therefore, the co-integration test is required. The Johansen and EG-ADF tests are used in this study to conduct the co-integration test, and both of these tests have been validated. EG-ADF testing yielded the following results, which are presented in the following table: Table 5 shows the results of the survey. This results in an ADF number for the remaining order being statistically meaningful at the 1% confidence level. This indicates the presence of statistically influential co-integration relationships between the variables in the data.
Suppose one is used as the lag order. In that case, the co-integration rank calculation can be performed after a lag order for the variable has been determined (Kordej-De Villa and Slijepcevic 2019), which is consistent with the information criterion. Table 6 presents the findings of the investigation in more detail. When the maximum rank is two, each variable in the model has two co integration relations. There are two co integration relations for each variable if the maximum rank of 2 is exceeded, as indicated by the trace statistic, less than the 5% threshold. To sum it up, data tested for co-integration and found to be significant can mean that the variables have a strong relationship.
There was a dependent variable for the construction industry, an independent variable for each of the three types of ERs, and control variables for the level of fiscal growth, manufacturing growth, and technical and industrial innovation were selected. Between 2000 and 2017, the GIE in the construction industry was examined in relation to three different types of ERs using the Tobit regression model. Table 7 shows the results of the regression analysis which was used in this study. Because all models had LR2 values that were statistically significant with a 95% confidence level, the mockups passed the general worth test (Yuan et al., 2017; Zhang et al., 2021).
CER and GIE have a negative but non-significant linear relationship, as evidenced by the current-period regression model. CER’s current-period effects on GIE construction are not statistically significant, as evidenced by the negative and positive coefficients of the first and second terms. There is a mathematically significant 90% correlation between MER and GIE in the present period; The initial term’s coefficient is negative, while the subsequent term’s coefficient is positive; these coefficients are statistically significant at 95% assurance. Non-linearity amid GIE and MER in the present period is depicted as a U-shape in the association between the two variables.
The rectilinear association between the present GIE and VER was negative and statistically significant, according to a 95 %assurance interval (CFI). The first term’s coefficient is positive, while the second term’s coefficient is negative, but these differences are not significant statistically. A direct association exists between the present GIE and VER in energy-intensive industries. According to a regression model used for the lagged phase, the CER of the lagged stage and the GIE of the intensive energy industries have an optimistic but non-statistically significant linear correlation (Cheng et al., 2021). When the coefficient of the non-linear relationship’s primary term is negative, the coefficient of the quadratic term is positive, and the coefficient of the quadratic term is negative, the coefficient is statistically significant. Furthermore, the regression model demonstrates a statistically important inconsistent association between the energy-intensive industries commerce GIE and the lag phase CER.
At the 99 %assurance level, there is no mathematically important association amid MER in the GIE and the lag phase in the energy-intensive industries. Still, there is a negative correlation between MER and GIE. The primary term of the coefficient of a non-linear relationship is positive, but there is no statistically significant connection between MER and GIE. The inverted U-shaped relationship between the mean lag phase error rate and the construction industry GIE is found to be a significant non-linear relationship (Brent and Ward 2018). Although the VER and the GIE for the energy-intensive industries show a negative linear relationship, this relationship is not statistically significant. Despite the fact that a non-linear relationship’s primary term has a positive coefficient and the quadratic polynomial term has a negative one, neither is statistically significant, suggesting that the lag phase’s VER has little impact on the construction industry’s GIE.
This study employs a reversion model to examine the heftiness of the approximation outcomes in order to assess their heftiness in the Tobit model approximation. Fallouts are said to be robust if they still have statistical significance for the test’s core variables. If the most important variables are statistically insignificant, the results will not be taken seriously. Rather than using the Tobit model, we used the GLM model and the gathering robust standard error technique to estimate the standard deviation. Table 8 displays the test results. It may be understood that the GLM model’s analysis results are robust, as both the core variable coefficient and significance are unaffected following the regression.
This study found a significant U-shape association between the construction industry’s green technology innovation efficiency (GIE) and the environment’s control and lag phase regulation. Given the time it takes for government administrative rights to take effect, CER exhibits a significant temporal lag, which is understandable (Abbas et al., 2021). According to the graph below, the impact of CER on GIE in China’s construction industry has reached a clearly defined inflection point. CER implementation intensity appears to have an effect on GIE, which first declines and then rises as the intensity of CER implementation is gradually increased. Because of this, the Chinese government should continue to encourage the adoption of the CER, better environmental laws and regulations, and increased administrative penalties for environmental offenses.
The construction industry’s global trade in environmental goods has a significant inverted U-shape association with market-based environmental regulations (MERs) in the lag phase (GIE). Market-based environmental regulations (MER) are more significant in the lag phase than now because of the market’s openness and dynamic nature. In the construction industry, it could be seen that MER are the most common ERs and have the most significant influence on the GIE of the industry at this time. The GIE of the energy-intensive sectors first increases and then decreases as a result of an increase in the intensity of MER implementation. According to Y. Zhou et al. (2013), reasonable implementation strength of MER improves the GIE in the energy-intensive industries. The market has a moderate incentive. The construction industry GIE can be enhanced by increasing the MER intensity (Hou et al., 2019). The construction industry is expected to actively pursue green technology innovation in order to evade high ecological safety prices while ensuring that it remains competitive (Tan and Lin 2020). High-strength MER, on the other hand, may force the energy-intensive industries to rise the costs of ecological contamination switch and investment contribution, which might result in the asset being diverted from other features of the manufacturing and have a bad influence on its overall operations, according to the MER report (Z. Cheng et al., 2020).
In the current period, there is a mathematically substantial negative correlation between GDP in the construction industry and voluntary environmental regulations (VER) (GIE). There are a number of environmental protection initiatives included in the VER program, which is carried out by a variety of stakeholders such as local residents, construction companies, and non-governmental organizations. GTI efficiency in the construction industry, on the other hand, is negatively impacted by VER, contrary to previous research. The higher the VER, the more expensive the energy-intensive industries (W. Du et al., 2020; Liu et al., 2018). According to previous research, it is clear that excessive VER strength in the construction industry is likely to stifle the advancements of GTI.
A summary of this study’s findings can be summarized: The Chinese construction industry’s GIE is affected by environmental regulations to varying degrees. However, it is possible to encourage a more environmentally friendly development of this industry by combining various environmental regulations (Luo Q. et al., 2019). Undoubtedly, the Multilateral Environmental Agreement (MER) has the most significant influence on the three kinds of environmental regulations. In order to promote green construction, it is essential to recover MER presentation by encouraging the broader operation of discharges transactions and enhancing the use of market mechanisms to address outwardness issues in the construction industry as a result of this. According to this report, the government should tool MER and CER in their entirety to strengthen green technological innovation in the energy-intensive industries.
It was determined that the phases of green technology development and research (GTRD) and commercialization (GTC) in the construction industry are distinct from one another in terms of their timing. A network SBM model has assessed the effectiveness of green technology innovation in the Chinese energy-intensive sector. For the final step, a Tobit regression model was applied to investigate the influence of three distinct types of environmental regulations (ERs) on the efficiency of green technology innovation (GIE). The following are the most significant findings of the study:
There is a misalignment between research and commercialization in the energy-intensive industries of green technology innovation. Since 2001, with the exception of 2000, the efficiency of green technology study and growth has consistently outperformed the efficiency of commercialization in the construction industry. Taking new technologies and turning them into a profit center is something that the construction industry is adept at. There is a lot of duplication in green technology’s research and growth stage, which means that a lot of money is spent on research that does not result in any breakthroughs. Greater R&D efficiency in green technology can be achieved by increasing the number of R&D accomplishments delivered with the resources allocated to green technology during the R&D stage.
The efficacy of green technology innovation can vary depending on the regulation. Implementing all three kinds of environmental regulations simultaneously has the potential to improve the GIE score significantly. In the first place, environmental regulations that are easy to understand and implement have a U-designed association with the GIE, with a significant time delay between the two events. In other words, the rule’s command-and-control approach to environmental regulations wills almost certainly reduce GIE. When the inflection point is crossed, environmental regulations systems for command and control can have a helpful influence on GIE systems. Considering that environmental regulations based on command and control will not be implemented directly, it is essential to be patient. However, they will not have an immediate impact until they have been in place for a period of time. Second, the connection between market place environmental regulations and GIE is shaped like are versed U. Market place environmental regulation can be beneficial to GIE in the beginning, but after the inflection point, market environmental regulations will become a hindrance to GIE’s development and growth. Even though voluntary environmental regulations are linked to GIE, this is only true for the time being. One of the primary reasons is that current volunteer environmental regulation is not flawless and, therefore, cannot have an optimistic impact on improving green technology innovation in the short term. As a result, the general public must be educated on the importance of environmental regulation and the necessity of voluntary regulations. Environmental regulations, on the whole, have been found to have no significant impact on GIE in general. Environmental regulations should be combined as a means of increasing GIE’s effectiveness.
There are two ways in which this research subsidizes the body of information about the efficacy of green technology invention: first, it increases the amount of information available about green technology innovation competence; second, it increases the amount of information available about green technology innovation efficiency. As a first and foremost result of the investigation, a new and more effective method of evaluating the efficacy of green technology innovation in energy-intensive industries has been proposed. Until recently, the innovation process for green technology was regarded as a black box with no way to see inside. Therefore, we were able to divide the process into two stages (green technology study and growth) and commercialize the results of these researches so that we could better understand the impact of green technology study and growth on manufacturing firms in the energy-intensive industries. In addition, this adds to the body of previous theoretical research on the effectiveness of green technology innovation, which has been conducted.
Moreover, it provides governments with specific guidance on developing more active environmental regulations. As demonstrated in this study, various environmental regulations have dissimilar impacts on the effectiveness of green technology innovation. They also have an effect on the development of green technology at different stages throughout history. Because of this, when developing environmental regulations for energy-intensive industries, governments should take into consideration the varying effects and time lags that may be present.
The scope of this investigation has also been limited in some ways. As a result, because the market mechanism is limited, the study begins by examining how environmental regulations affect GIE and then moves on to other topics. The government is increasingly being called upon to play a role in encouraging industry to improve its ability to innovate efficiently. Despite this, it is important to recognize that the market place machinery is not without significance. In the upcoming study, it will be necessary to examine the pouring mechanisms of GIE in the context of the double role played by government strategies and marketplace mechanisms. To carry out this research, the Chinese energy-intensive industries will be used as a case study to demonstrate how environmental regulations can improve GIE in other countries throughout the world. Even though the findings of this study can be applied in other frameworks, the operation process must take into consideration the conditions of other countries. Regarding these topics, there is still a lot to discover.
The raw data supporting the conclusion of this article will be made available by the authors, without undue reservation.
All authors listed have made a substantial, direct, and intellectual contribution to the work and approved it for publication.
This research was funded by research on driving mechanism and performance benefit of green innovation of industrial enterprises in Guizhou province under the background of strong industry (No.2017SWBZD05).
The authors declare that the research was conducted in the absence of any commercial or financial relationships that could be construed as a potential conflict of interest.
All claims expressed in this article are solely those of the authors and do not necessarily represent those of their affiliated organizations, or those of the publisher, the editors and the reviewers. Any product that may be evaluated in this article, or claim that may be made by its manufacturer, is not guaranteed or endorsed by the publisher.
Abbas, Q., Hanif, I., Taghizadeh-Hesary, F., Iqbal, W., and Iqbal, N. (2021). Improving the Energy and Environmental Efficiency for Energy Poverty Reduction. Econ. Law, Institutions Asia Pac., 231–248. doi:10.1007/978-981-16-1107-0_11
Abdul-Rahaman, A., Issahaku, G., and Zereyesus, Y. A. (2021). Improved Rice Variety Adoption and Farm Production Efficiency: Accounting for Unobservable Selection Bias and Technology Gaps Among Smallholder Farmers in Ghana. Technol. Soc. 64, 101471. doi:10.1016/j.techsoc.2020.101471
Adua, L. (2021). U.S. States Initiation of Energy Efficiency Policies in the Era of Climate Change: Throwing a Searchlight on the Influence of Political Partisanship. Environ. Sci. Policy, 115, 133–142. doi:10.1016/j.envsci.2020.09.021
Ai, H., Hu, Y., and Li, K. (2021). Impacts of Environmental Regulation on Firm Productivity: Evidence from China's Top 1000 Energy-Consuming Enterprises Program. Appl. Econ. 53 (7), 830–844. doi:10.1080/00036846.2020.1815642
Andersson, M., Moen, O., and Brett, P. O. (2020). The Organizational Climate for Psychological Safety: Associations with SMEs' Innovation Capabilities and Innovation Performance. J. Eng. Technol. Manag., 55, 101554. doi:10.1016/j.jengtecman.2020.101554
Andrei, M., Thollander, P., Pierre, I., Gindroz, B., and Rohdin, P. (2021). Decarbonization of Industry: Guidelines towards a Harmonized Energy Efficiency Policy Program Impact Evaluation Methodology. Energy Rep., 7, 1385–1395. doi:10.1016/j.egyr.2021.02.067
Anser, M. K., Iqbal, W., Ahmad, U. S., Fatima, A., and Chaudhry, I. S. (2020). Environmental Efficiency and the Role of Energy Innovation in Emissions Reduction. Environ. Sci. Pollut. Res. 27 (23), 29451–29463. doi:10.1007/s11356-020-09129-w
Bayarçelik, E. B., Taşel, F., and Apak, S. (2014). A Research on Determining Innovation Factors for SMEs. Procedia - Soc. Behav. Sci. 150, 202–211. doi:10.1016/j.sbspro.2014.09.032
Brent, D. A., and Ward, M. B. (2018). Energy Efficiency and Financial Literacy. J. Environ. Econ. Manag. 90, 181–216. doi:10.1016/j.jeem.2018.05.004
Chandio, A. A., Jiang, Y., Akram, W., Adeel, S., Irfan, M., and Jan, I. (2021). Addressing the Effect of Climate Change in the Framework of Financial and Technological Development on Cereal Production in Pakistan. J. Clean. Prod. 288, 125637. doi:10.1016/j.jclepro.2020.125637
Cheng, G., Zhao, C., Iqbal, N., Gülmez, Ö., Işik, H., and Kirikkaleli, D. (2021). Does Energy Productivity and Public-Private Investment in Energy Achieve Carbon Neutrality Target of China? J. Environ. Manag. 298, 113464. doi:10.1016/j.jenvman.2021.113464
Cheng, Z., Liu, J., Li, L., and Gu, X. (2020). Research on Meta-Frontier Total-Factor Energy Efficiency and its Spatial Convergence in Chinese Provinces. Energy Econ. 86, 104702. doi:10.1016/j.eneco.2020.104702
Curtis, E. M., and Lee, J. M. (2019). When Do Environmental Regulations Backfire? Onsite Industrial Electricity Generation, Energy Efficiency and Policy Instruments. J. Environ. Econ. Manag. 96, 174–194. doi:10.1016/j.jeem.2019.04.004
Dagar, V., Khan, M. K., Alvarado, R., Rehman, A., Irfan, M., Adekoya, O. B., et al. (2021). Impact of Renewable Energy Consumption, Financial Development and Natural Resources on Environmental Degradation in OECD Countries with Dynamic Panel Data. Environ. Sci. Pollut. Res. 29, 18202–18212. doi:10.1007/s11356-021-16861-4
Deng, Y., You, D., and Zhang, Y. (2021). Research on Improvement Strategies for Low-Carbon Technology Innovation Based on a Differential Game: The Perspective of Tax Competition. Sustain. Prod. Consum., 26, 1046–1061. doi:10.1016/j.spc.2021.01.007
Desvallées, L. (2022). Low-carbon Retrofits in Social Housing: Energy Efficiency, Multidimensional Energy Poverty, and Domestic Comfort Strategies in Southern Europe. Energy Res. Soc. Sci. 85, 102413. doi:10.1016/j.erss.2021.102413
Dias, Á. L., Cunha, I., Pereira, L., Costa, R. L., and Gonçalves, R. (2022). Revisiting Small- and Medium-Sized Enterprises’ Innovation and Resilience during COVID-19: The Tourism Sector. J. Open Innovation Technol. Mark. Complex. 8, 11. doi:10.3390/JOITMC8010011
Dong, F., Zhu, J., Li, Y., Chen, Y., Gao, Y., Hu, M., et al. (2022). How Green Technology Innovation Affects Carbon Emission Efficiency: Evidence from Developed Countries Proposing Carbon Neutrality Targets. Environ. Sci. Pollut. Res. 1, 20. doi:10.1007/S11356-022-18581-9/TABLES/13
Dorsey-Palmateer, R., and Niu, B. (2020). The Effect of Carbon Taxation on Cross-Border Competition and Energy Efficiency Investments. Energy Econ. 85, 104602. doi:10.1016/j.eneco.2019.104602
Du, K., Cheng, Y., and Yao, X. (2021). Environmental Regulation, Green Technology Innovation, and Industrial Structure Upgrading: The Road to the Green Transformation of Chinese Cities. Energy Econ. 98, 105247. doi:10.1016/j.eneco.2021.105247
Du, W., Wang, F., and Li, M. (2020). Effects of Environmental Regulation on Capacity Utilization: Evidence from Energy Enterprises in China. Ecol. Indic. 113, 106217. doi:10.1016/j.ecolind.2020.106217
Elavarasan, R. M., Pugazhendhi, R., Shafiullah, G. M., Irfan, M., and Anvari-Moghaddam, A. (2021). A Hover View over Effectual Approaches on Pandemic Management for Sustainable Cities - the Endowment of Prospective Technologies with Revitalization Strategies. Sustain. Cities Soc. 68, 102789. doi:10.1016/j.scs.2021.102789
Fang, Z., Razzaq, A., Mohsin, M., and Irfan, M. (2022). Spatial Spillovers and Threshold Effects of Internet Development and Entrepreneurship on Green Innovation Efficiency in China. Technol. Soc. 68, 101844. doi:10.1016/j.techsoc.2021.101844
García-Pérez-de-Lema, D., Ruiz-Palomo, D., and Diéguez-Soto, J. (2021). Analysing the Roles of CEO's Financial Literacy and Financial Constraints on Spanish SMEs Technological Innovation. Technol. Soc. 64, 101519. doi:10.1016/j.techsoc.2020.101519
Gondal, I. A., Masood, S. A., and Amjad, M. (2017). Review of Geothermal Energy Development Efforts in Pakistan and Way Forward. Renew. Sustain. Energy Rev. 71, 687–696. doi:10.1016/j.rser.2016.12.097
Guo, R., and Yuan, Y. (2020). Different Types of Environmental Regulations and Heterogeneous Influence on Energy Efficiency in the Industrial Sector: Evidence from Chinese Provincial Data. Energy Policy 145, 111747. doi:10.1016/j.enpol.2020.111747
Hao, Y., Gai, Z., Yan, G., Wu, H., and Irfan, M. (2021). The Spatial Spillover Effect and Nonlinear Relationship Analysis between Environmental Decentralization, Government Corruption and Air Pollution: Evidence from China. Sci. Total Environ. 763, 144183. doi:10.1016/j.scitotenv.2020.144183
Harrison, A., Martin, L. A., and Nataraj, S. (2017). Green Industrial Policy in Emerging Markets. Annu. Rev. Resour. Econ. 9, 253–274. doi:10.1146/annurev-resource-100516-053445
Hodson, E. L., Brown, M., Cohen, S., Showalter, S., Wise, M., Wood, F., et al. (2018). U.S. Energy Sector Impacts of Technology Innovation, Fuel Price, and Electric Sector CO2 Policy: Results from the EMF 32 Model Intercomparison Study. Energy Econ., 73, 352–370. doi:10.1016/j.eneco.2018.03.027
Horbach, J., Rammer, C., and Rennings, K. (2012). Determinants of Eco-Innovations by Type of Environmental Impact - the Role of Regulatory Push/pull, Technology Push and Market Pull. Ecol. Econ. 78, 112–122. doi:10.1016/j.ecolecon.2012.04.005
Hou, Y., Iqbal, W., Muhammad Shaikh, G., Iqbal, N., Ahmad Solangi, Y., and Fatima, A. (2019). Measuring Energy Efficiency and Environmental Performance: A Case of South Asia. Processes 7 (6), 325. doi:10.3390/pr7060325
Hu, S., Wu, H., Liang, X., Xiao, C., Zhao, Q., Cao, Y., et al. (2022). A Preliminary Study on the Eco-Environmental Geological Issue of In-Situ Oil Shale Mining by a Physical Model. Chemosphere 287, 131987. doi:10.1016/j.chemosphere.2021.131987
Huang, W., Saydaliev, H. B., Iqbal, W., and Irfan, M. (2022). Measuring the Impact of Economic Policies on CO2 Emissions: Ways to Achieve Green Economic Recovery in the Post-COVID-19 Era. Clim. Change Econ. doi:10.1142/s2010007822400103
Irfan, M., Ahmad, M., Fareed, Z., Iqbal, N., Sharif, A., and Wu, H. (2021). On the Indirect Environmental Outcomes of COVID-19: Short-Term Revival with Futuristic Long-Term Implications. Int. J. Environ. Health Res. 1, 11. doi:10.1080/09603123.2021.1874888
Irfan, M., and Ahmad, M. (2021). Relating Consumers' Information and Willingness to Buy Electric Vehicles: Does Personality Matter? Transp. Res. Part D Transp. Environ. 100, 103049. doi:10.1016/j.trd.2021.103049
Irfan, M., Zhao, Z.-y., Ahmad, M., Batool, K., Jan, A., and Mukeshimana, M. C. (2019). Competitive Assessment of Indian Wind Power Industry: A Five Forces Model. J. Renew. Sustain. Energy 11 (6), 063301. doi:10.1063/1.5116237
Irfan, M., Zhao, Z.-Y., Ikram, M., Gilal, N. G., Li, H., and Rehman, A. (2020). Assessment of India's Energy Dynamics: Prospects of Solar Energy. J. Renew. Sustain. Energy 12 (5), 053701. doi:10.1063/1.5140236
Isaksen, A., and Trippl, M. (2017). Innovation in Space: The Mosaic of Regional Innovation Patterns. Oxf. Rev. Econ. Policy 33 (1), 122–140. doi:10.1093/oxrep/grw035
Işık, C., Ahmad, M., Ongan, S., Ozdemir, D., Irfan, M., and Alvarado, R. (2021). Convergence Analysis of the Ecological Footprint: Theory and Empirical Evidence from the USMCA Countries. Environ. Sci. Pollut. Res. 28 (25), 32648–32659. doi:10.1007/s11356-021-12993-9
Jebali, E., Essid, H., and Khraief, N. (2017). The Analysis of Energy Efficiency of the Mediterranean Countries: A Two-Stage Double Bootstrap DEA Approach. Energy 134, 991–1000. doi:10.1016/j.energy.2017.06.063
Jinru, L., Changbiao, Z., Ahmad, B., Irfan, M., and Nazir, R. (2021). How Do Green Financing and Green Logistics Affect the Circular Economy in the Pandemic Situation: Key Mediating Role of Sustainable Production. Econ. Research-Ekonomska Istraživanja 1, 21. doi:10.1080/1331677X.2021.2004437
Jinzhou, W. (2011). Discussion on the Relationship between Green Technological Innovation and System Innovation. Energy Procedia 5, 2352–2357. doi:10.1016/j.egypro.2011.03.404
Khalfaoui, R., Baumöhl, E., Sarwar, S., and Výrost, T. (2021). Connectedness between Energy and Nonenergy Commodity Markets: Evidence from Quantile Coherency Networks. Resour. Policy 74 (August), 102318. doi:10.1016/j.resourpol.2021.102318
Khalfaoui, R., Sarwar, S., and Tiwari, A. K. (2019). Analysing Volatility Spillover between the Oil Market and the Stock Market in Oil-Importing and Oil-Exporting Countries: Implications on Portfolio Management. Resour. Policy 62 (March), 22–32. doi:10.1016/j.resourpol.2019.03.004
Khalil, M., Khawaja, K. F., and Sarfraz, M. (2021). The Adoption of Blockchain Technology in the Financial Sector during the Era of Fourth Industrial Revolution: a Moderated Mediated Model. Qual. Quant. 1, 18. doi:10.1007/s11135-021-01229-0
Khan, H., Weili, L., and Khan, I. (2022). Examining the Effect of Information and Communication Technology, Innovations, and Renewable Energy Consumption on CO2 Emission: Evidence from BRICS Countries. Environ. Sci. Pollut. Res. 1, 17. doi:10.1007/S11356-022-19283-Y/TABLES/7
Khan, S. A. R., Ponce, P., Tanveer, M., Aguirre-Padilla, N., Mahmood, H., and Shah, S. A. A. (2021). Technological Innovation and Circular Economy Practices: Business Strategies to Mitigate the Effects of COVID-19. Sustainability 13 (15), 8479. doi:10.3390/SU13158479
Khoshnevisan, B., Rafiee, S., Omid, M., and Mousazadeh, H. (2013). Reduction of CO2 Emission by Improving Energy Use Efficiency of Greenhouse Cucumber Production Using DEA Approach. Energy 55, 676–682. doi:10.1016/J.ENERGY.2013.04.021
Kiara, C. (2013). Determinants that Influence the Implementation of Infrastructure Development Projects in Renewable Energy Sector in Kenya; A Case of Kenya Electricity Generating Company Limited. J. Econ. Res.
Kordej-De Villa, Z., and Slijepcevic, S. (2019). Assessment of Local Councillors' Attitudes towards Energy Efficiency Projects in Croatia. J. Env. Assmt. Pol. Mgmt. 21, 1950012. doi:10.1142/S1464333219500121
Lemieux, V. L., Mashatan, A., Safavi-Naini, R., and Clark, J. (2021). A Cross-Pollination of Ideas about Distributed Ledger Technological Innovation through a Multidisciplinary and Multisectoral Lens: Insights from the Blockchain Technology Symposium '21. TIM Rev. 11 (6), 58–66. doi:10.22215/timreview/1445
Li, A., Zhang, A., Huang, H., and Yao, X. (2018). Measuring Unified Efficiency of Fossil Fuel Power Plants across Provinces in China: An Analysis Based on Non-radial Directional Distance Functions. Energy 152, 549–561. doi:10.1016/j.energy.2018.03.164
Li, L., Zhang, S., Cao, X., and Zhang, Y. (2021a). Assessing Economic and Environmental Performance of Multi-Energy Sharing Communities Considering Different Carbon Emission Responsibilities under Carbon Tax Policy. J. Clean. Prod., 328, 129466. doi:10.1016/j.jclepro.2021.129466
Li, Z., Kuo, T.-H., Siao-Yun, W., and The Vinh, L. (2022). Role of Green Finance, Volatility and Risk in Promoting the Investments in Renewable Energy Resources in the Post-covid-19. Resour. Policy 76, 102563. doi:10.1016/J.RESOURPOL.2022.102563
Li, Z., Sarwar, S., and Jin, T. (2021b). Spatiotemporal Evolution and Improvement Potential of Agricultural Eco-Efficiency in Jiangsu Province. Front. Energy Res. 9 (August), 1–12. doi:10.3389/fenrg.2021.746405
Li, Z., Wang, J., and Che, S. (2021c). Synergistic Effect of Carbon Trading Scheme on Carbon Dioxide and Atmospheric Pollutants. Sustainability 13 (10), 5403. doi:10.3390/su13105403
Lin, B., and Xu, B. (2020). Effective Ways to Reduce CO2 Emissions from China's Heavy Industry? Evidence from Semiparametric Regression Models. Energy Econ. 92, 104974. doi:10.1016/j.eneco.2020.104974
Liu, H., Li, J., Long, H., Li, Z., and Le, C. (2018). Promoting Energy and Environmental Efficiency within a Positive Feedback Loop: Insights from Global Value Chain. Energy Policy 121, 175–184. doi:10.1016/j.enpol.2018.06.024
Liu, H., Tang, Y. M., Iqbal, W., and Raza, H. (2022). Assessing the Role of Energy Finance, Green Policies, and Investment towards Green Economic Recovery. Environ. Sci. Pollut. Res. 29 (15), 21275–21288. doi:10.1007/s11356-021-17160-8
Liu, L., Wang, J., Wang, F., and Yang, X. (2021a). The Impact of the Planting of Forest Biomass Energy Plants under the Embedded Internet of Things Technology on the Biodiversity of the Local Environmental Ecology. Environ. Technol. Innovation, 24, 101894. doi:10.1016/j.eti.2021.101894
Liu, Y., Zhang, Z., Liu, X., Wang, L., and Xia, X. (2021b). Ore Image Classification Based on Small Deep Learning Model: Evaluation and Optimization of Model Depth, Model Structure and Data Size. Miner. Eng. 172, 107020. doi:10.1016/j.mineng.2021.107020
Luo, Q., Miao, C., Sun, L., Meng, X., and Duan, M. (2019a). Efficiency Evaluation of Green Technology Innovation of China's Strategic Emerging Industries: An Empirical Analysis Based on Malmquist-Data Envelopment Analysis Index. J. Clean. Prod. 238, 117782. doi:10.1016/j.jclepro.2019.117782
Luo, S., Zhang, L., and Fan, Y. (2019b). Energy-efficient Scheduling for Multi-Objective Flexible Job Shops with Variable Processing Speeds by Grey Wolf Optimization. J. Clean. Prod. 234, 1365–1384. doi:10.1016/j.jclepro.2019.06.151
Luo, Y., Lu, Z., and Long, X. (2020). Heterogeneous Effects of Endogenous and Foreign Innovation on CO2 Emissions Stochastic Convergence across China. Energy Econ. 91, 104893. doi:10.1016/J.ENECO.2020.104893
Madurai Elavarasan, R., Leoponraj, S., Dheeraj, A., Irfan, M., Gangaram Sundar, G., and Mahesh, G. K. (2021). PV-Diesel-Hydrogen Fuel Cell Based Grid Connected Configurations for an Institutional Building Using BWM Framework and Cost Optimization Algorithm. Sustain. Energy Technol. Assessments 43, 100934. doi:10.1016/j.seta.2020.100934
Martelli, E., Freschini, M., and Zatti, M. (2020). Optimization of Renewable Energy Subsidy and Carbon Tax for Multi Energy Systems Using Bilevel Programming. Appl. Energy, 267, 115089. doi:10.1016/j.apenergy.2020.115089
Martínez-Moya, J., Vazquez-Paja, B., and Gimenez Maldonado, J. A. (2019). Energy Efficiency and CO2 Emissions of Port Container Terminal Equipment: Evidence from the Port of Valencia. Energy Policy 131, 312–319. doi:10.1016/j.enpol.2019.04.044
Mehta, K., Sharma, R., and Vyas, V. (2019). Efficiency and Ranking of Sustainability Index of India Using DEA-TOPSIS. Jibr 11 (2), 179–199. doi:10.1108/JIBR-02-2018-0057
Ngo, T. Q. (2022). How Do Environmental Regulations Affect Carbon Emission and Energy Efficiency Patterns? A Provincial-Level Analysis of Chinese Energy-Intensive Industries. Environ. Sci. Pollut. Res. 29 (3), 3446–3462. doi:10.1007/S11356-021-15843-W/FIGURES/1
Pan, D., and Chen, H. (2021). Border Pollution Reduction in China: The Role of Livestock Environmental Regulations. China Econ. Rev. 69, 101681. doi:10.1016/j.chieco.2021.101681
Pardo-Bosch, F., Cervera, C., and Ysa, T. (2019). Key Aspects of Building Retrofitting: Strategizing Sustainable Cities. J. Environ. Manag. 248, 109247. doi:10.1016/j.jenvman.2019.07.018
Quan, Q., Gao, S., Shang, Y., and Wang, B. (2021). Assessment of the Sustainability of Gymnocypris Eckloni Habitat under River Damming in the Source Region of the Yellow River. Sci. Total Environ. 778, 146312. doi:10.1016/j.scitotenv.2021.146312
Qudrat-Ullah, H., and Nevo, C. M. (2021). The Impact of Renewable Energy Consumption and Environmental Sustainability on Economic Growth in Africa. Energy Rep. 7, 3877–3886. doi:10.1016/j.egyr.2021.05.083
Rasoulinezhad, E., and Taghizadeh-Hesary, F. (2022). Role of Green Finance in Improving Energy Efficiency and Renewable Energy Development. Energy Effic. 15 (2), 1–12. doi:10.1007/S12053-022-10021-4/TABLES/11
Rehman, A., Zhang, D., Chandio, A. A., and Irfan, M. (2020). Does Electricity Production from Different Sources in Pakistan Have Dominant Contribution to Economic Growth? Empirical Evidence from Long-Run and Short-Run Analysis. Electr. J. 33 (3), 106717. doi:10.1016/j.tej.2020.106717
Ren, F.-r., Tian, Z., Liu, J., and Shen, Y.-t. (2020). Analysis of CO2 Emission Reduction Contribution and Efficiency of China's Solar Photovoltaic Industry: Based on Input-Output Perspective. Energy 199, 117493. doi:10.1016/j.energy.2020.117493
Saguy, I. S. (2022). Food SMEs' Open Innovation: Opportunities and Challenges, Innovation Strategies Food Industry (Second Ed. 39, 52. doi:10.1016/B978-0-323-85203-6.00004-9
Sarwar, S., and Alsaggaf, M. I. (2020). The Willingness and Perception of People Regarding Green Roofs Installation. Environ. Sci. Pollut. Res. 27 (20), 25703–25714. doi:10.1007/s11356-020-08511-y
Sarwar, S. (2019). Role of Urban Income, Industrial Carbon Treatment Plants and Forests to Control the Carbon Emission in China. Environ. Sci. Pollut. Res. 26 (16), 16652–16661. doi:10.1007/s11356-019-04854-3
Sedlmeir, J., Buhl, H. U., Fridgen, G., and Keller, R. (2020). The Energy Consumption of Blockchain Technology: Beyond Myth. Bus. Inf. Syst. Eng. 62 (6), 599–608. doi:10.1007/s12599-020-00656-x
Shao, X., Zhong, Y., Liu, W., and Li, R. Y. M. (2021). Modeling the Effect of Green Technology Innovation and Renewable Energy on Carbon Neutrality in N-11 Countries? Evidence from Advance Panel Estimations. J. Environ. Manag. 296, 113189. doi:10.1016/j.jenvman.2021.113189
Singhai, R., and Sushil, R. (2021). An Investigation of Various Security and Privacy Issues in Internet of Things. Mater. Today Proc. doi:10.1016/j.matpr.2021.07.259
Su, C., and Urban, F. (2021). Circular Economy for Clean Energy Transitions: A New Opportunity under the COVID-19 Pandemic. Appl. Energy 289, 116666. doi:10.1016/j.apenergy.2021.116666
Sun, H., Kporsu, A. K., Taghizadeh-Hesary, F., and Edziah, B. K. (2020). Estimating Environmental Efficiency and Convergence: 1980 to 2016. Energy 208, 118224. doi:10.1016/j.energy.2020.118224
Tan, R., and Lin, B. (2020). The Influence of Carbon Tax on the Ecological Efficiency of China's Energy Intensive Industries-A Inter-fuel and Inter-factor Substitution Perspective. J. Environ. Manag., 261, 110252. doi:10.1016/j.jenvman.2020.110252
Tang, J., Zhang, C., and Zhou, W. (2019). Environmental Regulatory Efficiency and its Influencing Factors in China. Energy Effic. 12 (4), 947–962. doi:10.1007/s12053-018-9732-7
Tanveer, A., Zeng, S., Irfan, M., and Peng, R. (2021). Do perceived Risk, Perception of Self-Efficacy, and Openness to Technology Matter for Solar Pv Adoption? an Application of the Extended Theory of Planned Behavior. Energies 14 (16), 5008. doi:10.3390/en14165008
Vlontzos, G., Niavis, S., and Manos, B. (2014). DecemberA DEA Approach for Estimating the Agricultural Energy and Environmental Efficiency of EU Countries. Renew. Sustain. Energy Rev. 40, 91–96. doi:10.1016/j.rser.2014.07.153
Waheed, R., Sarwar, S., and Mighri, Z. (2020). Role of High Technology Exports for Energy Efficiency: Empirical Evidence in the Context of Gulf Cooperation Council Countries. Energy & Environ. 32 (5), 803–819. doi:10.1177/0958305X20954196
Wang, H., Cui, H., and Zhao, Q. (2021). Effect of Green Technology Innovation on Green Total Factor Productivity in China: Evidence from Spatial Durbin Model Analysis. J. Clean. Prod. 288, 125624. doi:10.1016/j.jclepro.2020.125624
Wang, H., and Luo, Q. (2022). Can a Colonial Legacy Explain the Pollution Haven Hypothesis? A City-Level Panel Analysis. Struct. Change Econ. Dyn. 60, 482–495. doi:10.1016/J.STRUECO.2022.01.004
Wang, M., and Feng, C. (2020). Regional Total-Factor Productivity and Environmental Governance Efficiency of China's Industrial Sectors: A Two-Stage Network-Based Super DEA Approach. J. Clean. Prod. 273, 123110. doi:10.1016/j.jclepro.2020.123110
Wang, Q., Qu, J., Wang, B., Wang, P., and Yang, T. (2019). Green Technology Innovation Development in China in 1990-2015. Sci. Total Environ. 696, 134008. doi:10.1016/j.scitotenv.2019.134008
Wen, C., Akram, R., Irfan, M., Iqbal, W., Dagar, V., Acevedo-Duqued, Á., et al. (2022). The Asymmetric Nexus between Air Pollution and COVID-19: Evidence from a Non-linear Panel Autoregressive Distributed Lag Model. Environ. Res. 209, 112848. doi:10.1016/j.envres.2022.112848
Wu, H., Hao, Y., Ren, S., Yang, X., and Xie, G. (2021). Does Internet Development Improve Green Total Factor Energy Efficiency? Evidence from China. Energy Policy 153, 112247. doi:10.1016/j.enpol.2021.112247
Xiang, D., Zhao, T., and Zhang, N. (2022). How Can Government Environmental Policy Affect the Performance of SMEs: Chinese Evidence. J. Clean. Prod. 336, 130308. doi:10.1016/j.jclepro.2021.130308
Xie, X., Huo, J., and Zou, H. (2019). Green Process Innovation, Green Product Innovation, and Corporate Financial Performance: A Content Analysis Method. J. Bus. Res. 101, 697–706. doi:10.1016/j.jbusres.2019.01.010
Xing, G., Duan, Z., Yan, W., and Baykal-Gürsoy, M. (2021). Evaluation of "innovation Chain + Supply Chain" Fusion Driven by Blockchain Technology under Typical Scenario. Int. J. Prod. Econ., 242, 108284. doi:10.1016/j.ijpe.2021.108284
Xu, B., and Lin, B. (2020). Investigating Drivers of CO2 Emission in China's Heavy Industry: A Quantile Regression Analysis. Energy 206, 118159. doi:10.1016/j.energy.2020.118159
Xu, B., and Lin, B. (2016). Reducing Carbon Dioxide Emissions in China's Manufacturing Industry: a Dynamic Vector Autoregression Approach. J. Clean. Prod. 131, 594–606. doi:10.1016/j.jclepro.2016.04.129
Yang, C., Hao, Y., and Irfan, M. (2021). Energy Consumption Structural Adjustment and Carbon Neutrality in the Post-COVID-19 Era. Struct. Change Econ. Dyn. 59, 442–453. doi:10.1016/j.strueco.2021.06.017
Ye, F.-F., and Wang, Y.-M. (2019). The Effects of Two Types of Environmental Regulations on Economic Efficiency: An Analysis of Chinese Industries. Energy & Environ. 30 (5), 898–929. doi:10.1177/0958305X18813690
Yu, J., Tang, Y. M., Chau, K. Y., Nazar, R., Ali, S., and Iqbal, W. (2022). Role of Solar-Based Renewable Energy in Mitigating CO2 Emissions: Evidence from Quantile-On-Quantile Estimation. Renew. Energy 182, 216–226. doi:10.1016/j.renene.2021.10.002
Yu, X., and Li, Y. (2020). Effect of Environmental Regulation Policy Tools on the Quality of Foreign Direct Investment: An Empirical Study of China. J. Clean. Prod. 270, 122346. doi:10.1016/j.jclepro.2020.122346
Yuan, Y., Yu, X., Yang, X., Xiao, Y., Xiang, B., and Wang, Y. (2017). Bionic Building Energy Efficiency and Bionic Green Architecture: A Review. Renew. Sustain. Energy Rev. 74, 771–787. doi:10.1016/j.rser.2017.03.004
Zhang, D., Awawdeh, A. E., Hussain, M. S., Ngo, Q. T., and Hieu, V. M. (2021). Assessing the Nexus Mechanism between Energy Efficiency and Green Finance. Energy Effic. 14 (8), 1–18. doi:10.1007/S12053-021-09987-4/TABLES/6
Zhang, Y., and Song, Y. (2021). Environmental Regulations, Energy and Environment Efficiency of China's Metal Industries: A Provincial Panel Data Analysis. J. Clean. Prod. 280, 124437. doi:10.1016/j.jclepro.2020.124437
Zhang, Y., Xiong, Y., Li, F., Cheng, J., and Yue, X. (2020). Environmental Regulation, Capital Output and Energy Efficiency in China: An Empirical Research Based on Integrated Energy Prices. Energy Policy 146, 111826. doi:10.1016/j.enpol.2020.111826
Zheng, Y., Han, W., and Yang, R. (2021). Does Government Behaviour or Enterprise Investment Improve Regional Innovation Performance - Evidence from China. Ijtm 85 (2–4), 274–296. doi:10.1504/IJTM.2021.115266
Zhou, D. Q., Wu, F., Zhou, X., and Zhou, P. (2016). Output-specific Energy Efficiency Assessment: A Data Envelopment Analysis Approach. Appl. Energy 177, 117–126. doi:10.1016/j.apenergy.2016.05.099
Zhou, Y., Xing, X., Fang, K., Liang, D., and Xu, C. (2013). Environmental Efficiency Analysis of Power Industry in China Based on an Entropy SBM Model. Energy Policy 57, 68–75. doi:10.1016/j.enpol.2012.09.060
Zhu, Z., Bai, Y., Dai, W., Liu, D., and Hu, Y. (2021). Quality of E-Commerce Agricultural Products and the Safety of the Ecological Environment of the Origin Based on 5G Internet of Things Technology. Environ. Technol. Innovation, 22, 101462. doi:10.1016/j.eti.2021.101462
Keywords: environment regulations, green technology innovation, sustainable development, tobit regression model, network DEA model
Citation: Wang P and Liang S (2022) Environmental Regulations and Energy Efficiency: The Mediating Role of Climate Change and Technological Innovation. Front. Environ. Sci. 10:909082. doi: 10.3389/fenvs.2022.909082
Received: 31 March 2022; Accepted: 22 April 2022;
Published: 31 May 2022.
Edited by:
Muhammad Irfan, Beijing Institute of Technology, ChinaCopyright © 2022 Wang and Liang. This is an open-access article distributed under the terms of the Creative Commons Attribution License (CC BY). The use, distribution or reproduction in other forums is permitted, provided the original author(s) and the copyright owner(s) are credited and that the original publication in this journal is cited, in accordance with accepted academic practice. No use, distribution or reproduction is permitted which does not comply with these terms.
*Correspondence: Peng Wang, NTY1ODU2Nzc2QHFxLmNvbQ==; Shuai Liang, MzU0MDAxMzQ2QHFxLmNvbQ==
Disclaimer: All claims expressed in this article are solely those of the authors and do not necessarily represent those of their affiliated organizations, or those of the publisher, the editors and the reviewers. Any product that may be evaluated in this article or claim that may be made by its manufacturer is not guaranteed or endorsed by the publisher.
Research integrity at Frontiers
Learn more about the work of our research integrity team to safeguard the quality of each article we publish.