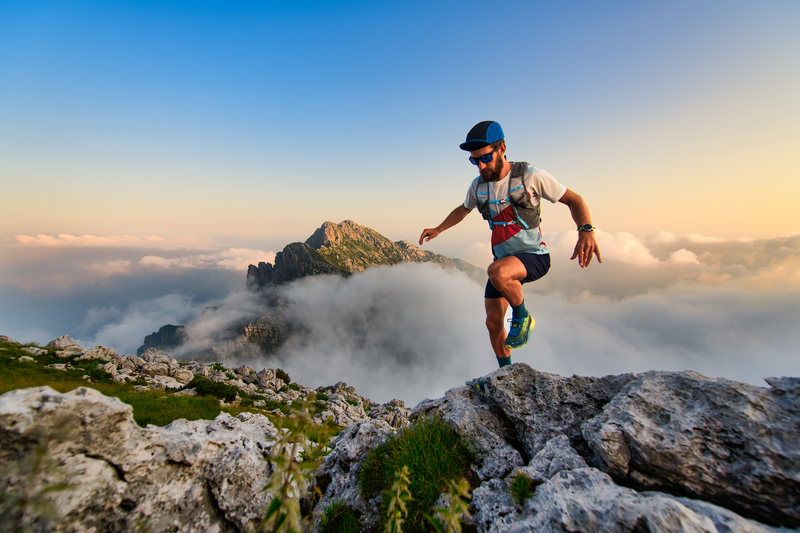
95% of researchers rate our articles as excellent or good
Learn more about the work of our research integrity team to safeguard the quality of each article we publish.
Find out more
ORIGINAL RESEARCH article
Front. Environ. Sci. , 16 June 2022
Sec. Land Use Dynamics
Volume 10 - 2022 | https://doi.org/10.3389/fenvs.2022.904779
This article is part of the Research Topic Sustainable Cultivated Land Use and Management View all 18 articles
China has achieved sustained growth in grain production and significant changes in grain patterns since the early 21st century. Meanwhile, the contradiction between the shortage of water resources and the development of agriculture is becoming more and more severe. This study introduced Gravity Recovery and Climate Experiment (GRACE) gravity satellite Total Water Storage (TWS) Product to indicate total water storage and calculated the Cumulated Normalized Difference Vegetation Index (CNDVI) of cropland as an indicator for grain growth. Based on the continuous satellite data, this paper revealed the spatial mismatch between water resources supply and grain growth pattern in China. The center of gravity of the CNDVI tends to move northwest, while the GRACE TWS data’s center of gravity is in the opposite direction. There were different relationships between GRACE-TWS and CNDVI changes in different zones. We calculated the pixel-wise spatial Pearson Correlation coefficients of TWS and CNDVI. The TWS data and CNDVI data show negative correlation trends in the water-limited areas such as the northern arid-semiarid region and northern China plain, while they show a positive correlation in relatively sufficient water resources in southeast China. According to the results, the changing pattern of grain production in China is likely to cause the depletion of grain production potential in the water-limited regions, while the southeastern regions with higher potential still have more capacity for agricultural production.
In parallel to the importance of other energy resources for production systems (Elahi et al., 2022a; Elahi et al., 2022b), water is also one of the imperative resources for plant production. Water, which is one of the most fundamental inputs for agricultural production. Irrigation, fertilizer applications or pesticide, water takes part in almost every section of agricultural activities (Elahi et al., 2021a; Elahi et al., 2021b). Accompanied by the rapidly increasing demands on water, the development of China’s agriculture leads to severe water stress in some regions where water is the limiting factor in the local ecosystem. Nationwide, agriculture accounts for 62% of water consumption, industry consumes 22%, domestic water takes 14%, and other use 2% (National Bureau of Statistics of China, 2020). With the demand for water resources continuing to grow, the drought afterward brings about serious damages to agricultural production. In China, the average annual crop area affected by drought was 62 million acres at the turn of the century, compared to 28.7 million acres in the 1950’s (Ministry of Water Resources, 2010). From January 2009 to April 2010, China endured three severe droughts. The intensity of meteorological droughts in all three disasters reached the level of a 100-years event. Millions of people and livestock had difficulty accessing potable water, the survival of tens of millions of hectares of crops was threatened, and there were far-reaching long-term economic, social and ecological impacts. Subsequently, from 2010 to 2011, drought struck the North China and Huang-Huai-Hai regions again (Ye et al., 2012).
Water resources have a significant impact on whole ecosystems and socioeconomic systems. Many scientists have begun to look across traditional disciplines to explore the interactions between water, vegetation, and nutrients using an ecohydrology perspective (Bonell, 2009). Researchers have emphasized the importance of tracking the movement of water in those water-limited areas. However, cost and logistics impede the availability of monitoring the water resource on a large scale (Rodell and Famiglietti, 2001). In China, hydrological monitoring sites and satellite observations were widely used to monitor water track in a large area. Although researchers can estimate water resources by these methods, the shortcomings of these methods are also evident. These drawbacks include, the monitoring of hydrological condition at a large scale needs a lot of ground observation sites; the integration and preprocessing of such a large amount of data are time-consuming and labor-intensive. When building hydrological models, the total amount of water resources in an area is determined by point sources such as ground-based observations, introducing huge errors. Gravity Recovery and Climate Experiment project, launched on 17 March 2002 by NASA and German Aerospace Center (GLR), is able to measure the changes of Earth’s gravity field (Adam, 2002). Scientists are subsequently capable of interpreting these changes in gravity as water movement, whether on the surface or under the ground. Many studies have used representative regions as study areas (northern India, Australia, northern China, etc.) and compared the output of traditional hydrological models to conclude that GRACE data are reliable indicators of local water storage changes (Landerer and Swenson, 2012; Feng et al., 2013; Yang et al., 2014; Cao et al., 2015; Iqbal et al., 2016). Famiglietti et al. (2011) took the central California valley as the study area, finding that GRACE data precisely captured the water storage changes in this area. Wahr et al. (2004) find that both on land and ocean Total Water Storage changes from GRACE data displayed a high consistency with outputs from the hydrological model.
In recent decades, China’s grain yield has continued to grow and the pattern of grain production has changed significantly. The center of grain production in China is gradually shifting to the north. Wang et al. (2005) observed that during the entire period of 1990–2005, the grain output center-of-gravity moved remarkably from the south and east to the north and west of China, and the moving speed was increasing (Zhang et al., 2018). Alone with the water scarcity in northern China in recent years, the shift of grain production centers to the northwest may put more pressure on the water supply in northern China. Exhausting the agricultural potential of northern China too quickly could also bring agricultural products decreasing when the water resource reaches their critical point.
The objectives of this study are to 1) observe the trends of changes in grain production and local water storage at the raster scale using satellite data, and 2) reveal the contradiction between water demand and water supply due to the changing grain production pattern in China. We first introduced the center-of-gravity analysis to explore the trend of CNDVI and TWS at the national scale. Then we discuss the trends of the time series of the two data sets in nine agricultural regions. Finally, we mapped the spatial correlation of these two datasets pixelwise.
This research focused on mainland China. Mainland China exhibits strong monsoonal and continental climatic characteristics. The topography of China is diverse, with higher elevations in the west and lower elevations in the east. A temperate continental climate dominates the northwest, while a temperate monsoon climate dominates the Northeast. A subtropical monsoon climate is prevalent in southern China, except for the southernmost part of southern China, where a tropical monsoon climate dominates. A highland alpine climate dominates the Qinghai-Tibet Plateau. Most of mainland China is located in the East Asian monsoon region, and there are significant monthly and annual variations in temperature and precipitation.
According to the Comprehensive Agricultural Zoning of China (National Agricultural Zoning Committee, 1981), this study divided mainland China into nine agricultural regions for discussion. These regions have different climatic conditions, soil conditions and farming systems. They are A: Northeast China Plain, B: Northern arid and semi-arid region, C: Huang-Huai-Hai Plain, D: Loess Plateau, E: Qinghai Tibet Plateau, F: Middle-lower Yangtze Plain, G: Sichuan Basin and surrounding regions, H: Southern China, I: Yunnan-Guizhou Plateau. Figure 1 shows the study area and zoning area. More importantly, these regions face different pressures on water resources. Northwestern China is a relatively water-scarce area compared to the southeast. In these regions, water is a limiting factor in the ecosystem. These areas are classified as water-limited areas.
FIGURE 1. Mainland China with Agricultural Zoning [(A): Northeast China Plain, (B): Northern arid and semi-arid region, (C): Huang-Huai-Hai Plain, (D): Loess Plateau, (E): Qinghai Tibet Plateau, (F): Middle-lower Yangtze Plain, (G): Sichuan Basin and surrounding regions, (H): Southern China, (I): Yunnan-Guizhou Plateau].
In this study, we used the GRCTellus Land grids Level-3 GRACE data product processed by the Jet Propulsion Laboratory (Landerer and Swenson, 2012) to provide estimates of changes in total water storage. The units of Level-3 data are centimeters of equivalent water thickness.
Before calculating the center of gravity for the GRACE data, we applied the following equation to convert the equivalent water thickness provided by the GRACE data to total water storage, for each pixel in the raster of the GRACE data:
where tEWT is the equivalent water thickness of the pixel and A is the area represented by the same pixel.
Since the spatial resolution of GRACE data is in degree, the area represented by each cell is different at different latitudes, we applied some formulas to simply estimate the area represented by each grid. There is a relatively simple and exact formula for the area of any spherical quadrilateral defined by parallels (latitude) and meridians (longitude). The ellipse can be derived directly by using the basic properties of the ellipse (long axis a and short axis b) rotated about its short axis to produce the ellipsoid.
The formula can be simplified by breaking down the calculation into basic steps. First, the distance between the east-west boundary (meridians l0 and l1) is part of the whole circle and is equal to q = (l1−l0)/360 (when the meridian is in degrees). Find the area of the entire slice located between the parallel lines f0 and f1 and multiply it by q. Next, we will apply a formula for an elliptical horizontal slice defined by the equator (at f0 = 0) and an ellipse parallel to it at latitude f = f1. The area of the slice between any two latitudes f0 and f1 (located on the same hemisphere) will be the difference between the larger area and the smaller area. Finally, assuming that the model is a true ellipsoid (and not a sphere), the area of such a slice between the equator and a parallel of latitude f is:
where a and b are the lengths of the major and minor axes of the generating ellipse, respectively (in WGS84, a = 6,378,137 m and b = 6,356,752.3142 m); f is the center of latitude.
Due to instrument issues and calibration campaigns, and the GRACE program’s implementation of GRACE satellite battery management starting in 2011, part of GRACE data from data centers were missing during the observation period (Rodell et al., 2004; Cooley and Landerer, 2019). Table 1 shows the index of the month with missing GRACE data during the whole observation period.
As shown in Table 1, the dates of missing data do not follow a specific pattern. GRACE data are monthly data and possess significant seasonality. We grouped the data by month and calculate the mean value in each group. We inserted the averages according to months with missing data. After forming a complete set of Monthly GRACE data from June 2003 to December 2016, we calculated the annual average of GRACE data.
The Normalized Difference Vegetation Index (NDVI) is a normalized index for highlighting vegetation features in images. Many studies have shown that the NDVI can be a helpful tool in measuring the fraction of absorbed photosynthetically active radiation, extracting vegetation classes, or estimating green biomass (Colwell, 1974; Baret and Guyot, 1991; Goward and Huemmrich, 1992; Thenkabail et al., 2000; Ahmed and Akter, 2017). Plant leaf tissue strongly absorbs blue and red light, while it strongly reflects it in the green and infrared bands. From red light to infrared light, the reflection of bare ground is higher, but the increase is slight. The higher the vegetation cover, the smaller the reflection of red light and the greater the reflection in the near-infrared band (NIR). Therefore, NDVI was introduced as a mathematical transformation to enhance the difference between the RED and NIR bands. As a result, researchers can separate land covered by vegetation from other types of land cover. Furthermore, NDVI was defined as:
where NIR indicate the spectral reflectance at the near-infrared and red band separately. Although many studies have found and established the relationship between NDVI and grain yield, these studies have also found that the relationship between NDVI and grain yield varies with different study areas, and therefore the use of time-integrated NDVI values to directly refer to grain yield may be subject to large errors in different regions. Such errors may be caused by local climatic conditions or changes in the type of crops. However, the purpose of this study is to reveal the contradiction between agricultural water use and water availability under the changing grain pattern in China, Responding to crop growth and biomass accumulation through cumulative NDVI. The use of NDVI has some advantages. For example, although the use of NDVI to predict grain yield is not stable, some studies have found that the relationship between NDVI and biomass accumulation in crops is more apparently stable and pronounced (Wang et al., 2005; le Maire et al., 2011). Therefore, crop biomass accumulation is a more appropriate indicator of water consumption in agriculture than yield when revealing water crises under changing food production patterns. CNDVI is also more suitable for this study relative to the crop yield indicator based on field surveys or statistics.
NASA’S Moderate Resolution Imaging Spectroradiometer Vegetation Indices (MODIS VIs) provided consistent spatial time-series information about global vegetation conditions. The rasterized vegetation index map depicts the temporal and spatial changes of vegetation activity, once every 16 days and every month. To be consistent with the GRACE data, we resampled the NDVI data to 1° × 1°.
Normalized Difference Vegetation Index is widely used to monitor vegetation stress, measuring NDVI throughout a growing season helps to evaluate the effect of continuous phenological and morphological changes on grain yield. To quantify the biomass accumulation by the growth of crops, we introduced the Cumulated Normalized difference vegetation index to this study as an indicator of water consumption for grain production.
Directly predicting grain yields from satellite data has proven to be more difficult. However, some studies have achieved some results using NDVI data (Prasad et al., 2006; Ren et al., 2008; Panek and Gozdowski, 2020). A study by Ren et al. (2008) found that it is more reasonable and accurate to calculate the accumulation of NDVI and use it to predict yields (Lopresti et al., 2015). Based on the assumption that plants grow at temperatures above 10°C, we applied the cumulative temperatures method to the NDVI data. Thus, we found the longest period of the year when the temperature is above 10°C and sum up the NDVI values for that period. This sum calculated by this method was used to characterize the cumulative increase in NDVI of the plant during its growing period. Finally, we extract the cumulated NDVI in cropland by using Land Cover data as masks. NDVI data was provided by NASA’s MODIS Satellites. Cropland masks were collected from the EOS CCI land cover datasets. Also, for the correlation test, we subtracted the multiyear average of the same period of TWS data as the baseline for CNDVI to ensure that the two variables were taken the same treatment.
The cropland data were extracted from the land cover maps of The European Space Agency (ESA) CCI projects. The data version is 2.0.7, contains global land cover maps 1992–2015, and 2016 at 300 m spatial resolution (ESA, 2017). CCI-LC maps use the United Nations (UN) Land Cover Classification System (LCCS) to describe the Landcover state with 24 different classes. Bontemps et al. evaluated the accuracy of the 2010 ESA CCI LC product. Using all points interpreted by experts as “definite,” either as a single land cover or consisting of multiple mosaics, an overall accuracy of 71.5% was found. Referring to the homogeneous points, the overall accuracy increased to 75.4% (Bontemps et al., 2011). The highest user accuracy values were found in the categories of rainfed farmland, irrigated farmland, broadleaf evergreen forest, urban areas, bare ground, water bodies, and permanent snow and ice. In contrast, the mosaic category of natural vegetation was associated with the lowest user accuracy values as well as the three categories of lichens and mosses, sparse vegetation, and swamp forests. In calculating the center of gravity shift, we used a continuous Land Cover map to extract cropland. In the TWS-CNDVI spatial correlation analysis, the land cover map at the beginning of the observation was used.
The concept of center of gravity originated in physics. It is an imagery point in which the force of gravity appears to act. In the Geographic information system, a method of calculating the ideal location for warehousing facilities by calculating the transport costs to each of the outlets to be served by the facility (Chen et al., 2011). The center of gravity model is an essential analytical tool to study the spatial changes of factors in regional development (Wang et al., 2018). As an important tool in spatial analysis, center of gravity method is widely used in population barycenter, economic barycenter and energy barycenter model (Smith and Dicken, 2000; Zhang et al., 2012; Bigot and Klein, 2018). The movement of the center of gravity of factors reflects the spatial trajectory of regional development. The analytical model of the center of gravity constructed in this study is expressed as:
where n is the total number of cells in each raster; Point P (X, Y) represents the coordinate of the center of gravity; Gi is the ith sample values; Xi and Yi are the coordinates of the geographic center of the evaluation unit. Next, we calculated the coordinate of the center of gravity for each year. Finally, we plotted the center points’ trajectory map to visualize the pattern of changes in GRACE data and Cumulated NDVI data.
In statistics, the Pearson product-moment correlation coefficient is used to measure the degree of linear correlation between two sets of variables X and Y, with a value between −1 indicates a totally negative linear correlation and 1 represents a totally positive linear correlation.
If X and Y are stationary, the Pearson correlation coefficient is built as follows:
where r is the Pearson correlation coefficient; x and y represent the values in the first and second sets of data separately; n is the total number of data sets.
The trajectory of the center of gravity can indicate the tendency of the variable to move in space. Figure 2A shows that the center of gravity of water resources generally moves to the south and east during the observation period. The other side of this occurrence is that TWS data in western and northern China are decreasing. The movement of the center of gravity in the GRACE TWS can be broadly divided into several phases. First, 2004–2008 saw a continuous movement of gravity to the south, followed by a shift to the west from 2009 to 2011. Then, immediately after 2011, the center of gravity began to move significantly to the east, and this trend continued until 2013. After that, from 2013 to 2016, the center of gravity of GRACE data started to move southward.
FIGURE 2. (A) The Trajectory Plot of TWS’s center of gravity from 2003 to 2016 and (B) The Trajectory Plot of Cumulated NDVI’s center of gravity from 2003 to 2016.
Figure 2B shows the movement of CNDVI’s center of gravity from 2003 to 2016. Throughout the observation interval, CNDVI shows the opposite motion trajectory to TWS, from southeast to northwest. However, at each stage, the trajectory of the center of gravity movement of CNDVI does not overlap with that of TWS. In order to make the trajectory of the center of gravity more prominent, we divided the whole interval into several phases. 2003–2006 was the first phase, and 2007–2009 was the second phase when the center of gravity moved more to the west and slightly to the south. After that, the center of gravity continues to move northward from 2009 until 2012. And after that, it continues until the end of the observation period, the center of gravity continues to move sharply to the west and slightly to the south.
Although the interannual trend of the CNDVI center of gravity is not the same as that of TWS’s, this fact that the two do not overlap may be due to a variety of reasons, which may include: a delay between changes in grain production and changes in water resources, the cyclic flow characteristics of water that cause agricultural development to affect water resources regionally rather than point-to-point, and changes in water resources that also incorporate the effects of other natural and human activities. Figure 4 shows the correlation coefficients of GRACE and CNDVI in space. Each pixel contains two sets of data, one set of GRACE data from 2003 to 2016, and CNDVI data for the corresponding period. We matched the two sets of data and calculated the correlation coefficients. For each pixel, the correlation coefficients have large uncertainties. However, when the target is the whole region, the overall trend obtained from the analysis of the correlation coefficients is still credible. The correlation analysis between GRACE data and NDVI data at the spatial scale corroborates the previous analysis more intuitively. The TWS data and CNDVI show the exact opposite relationship in water-limited areas (Northern arid and semi-arid region, Huang-Huai-Hai Plain, Loess Plateau) and other areas where water resources are not a limiting factor.
From January 2002 to December 2016, GRACE satellites observed the changes in water resources all over mainland China. As shown in Figure 3, in the Northern arid and semi-arid region of China (Inner Mongolia, Xinjiang, Gansu, and Ningxia province), the Huang-Huai-Hai Plain (Northern China plain), and the Loess Plateau, TWS show a clear trend of annual decline. In Northeast China, GRACE satellites monitored fluctuations in TWS with three peaks and three troughs throughout the observation period. TWS value of this area reaches a maximum value in 2005, 2010, and 2013, respectively, followed by a rapid decline, reaching its valley in 2008, 2011, and 2015. In the water-limited area, the annual yearly TWS value showed a continuous decline. The data increase in these areas during the first 2 to 3 years of the observation period, and then show an almost continuous decrease during the subsequent observation period. Specifically, the TWS in the northern arid and semi-arid region starts to show a steep decline in 2005, fluctuates between −500 and 0 m3 × 1,000 m3 between 2009 and 2013, and then starts to show a continuous decline again. In other water-limited regions, TWS was stable from 2008 to 2012 and declined continuously during the rest of the period. The overall trend of TWS for the Qinghai-Tibet Plateau is decreasing. Because GRACE TWS Data contains ice, the melting of snow and ice and its inflow into the immediate area will also cause a reduction in TWS. In the southern region, the overall trend of TWS is quite different from that in the north. In and around the Sichuan basin, monthly TWS shows significant interannual variation, but the trend is stable throughout the period. In contrast, in the middle and lower reaches of the Yangtze River plain, southern China, and the Yunnan-Guizhou plateau, TWS remains stable in the early part of the observation period and starts to show a continuous increase around 2010. The annual TWS in the non-water-limited area generally shows an increasing trend. In the south, TWS showed a different trend from the north. TWS showed a growing trend in the middle-lower Yangtze Plain, Sichuan Basin and surrounding regions, Southern China, and Yunnan-Guizhou Plateau. In the middle and lower reaches of the Yangtze River, TWS increased from −161 m3 × 1,000 m3 at the beginning of the observation to 374 m3 × 1,000 m3 at the end of the observation. The trend line of TWS in the Sichuan basin region shows that the value does not vary much during the observation period, slowly increasing from slightly below the zero line at the beginning to slightly above the zero line at the end. The trends of TWS are similar in South China and the Yunnan-Guizhou plateau, where the value remains relatively small from 2003 to 2010, while showing an increase after this period. The annual TWS value in South China increased from near the zero line to near 270 m3 × 1,000 m3, while the value in the Yunnan-Guizhou plateau was about 512 m3 × 1,000 m3.
FIGURE 3. GRACE time series plot of yearly sum with Trend Line from 2003 to 2016 in nine agricultural regions. [(A) Northeast China Plain, (B) Northern arid and semi-arid region, (C) Huang-Huai-Hai Plain, (D) Loess Plateau, (E) Qinghai Tibet Plateau, (F) Middle-lower Yangtze Plain, (G) Sichuan Basin and surrounding regions, (H). Southern China, (I) Yunnan-Guizhou Plateau].
Figure 4 shows the annual trend of CNDVI data with an increasing trend in most agricultural zones of China, except in the Qinghai-Tibet Plateau. During the observation interval, the CNDVI of the Qinghai-Tibet Plateau region showed an increasing yearly trend from 2003 to 2010 and decreased after reaching the peak in 2010. The decreasing trend continued until the end of the observation. Northeast China is an important center of grain production in China. In terms of CNDVI, the value of CNDVI in Northeast China is relatively high. Compared to other regions, although the growth trend in the Northeast is stable, the absolute value of the growth is smaller compared to the Northeast. At the end of the observation period, the growth trend of the CNDVI value in Northeast China was stopped, and its value floated around 7.65/million. The CNDVI of the Huang-Huai-Hai Plain and Loess Plateau showed similar trends and increased steadily throughout the observation period. However, the base values of the two regions were different, with the CNDVI in the Huang-Huai-Hai Plain increasing from 7.8 million at the beginning to about 8.3 million at the end of the observation period, while the CNDVI in the Loess Plateau increased from 2.3 million to 2.7 million. In the southern part of China, CNDVI generally tends to level off gradually in the early part of the observation and rises in the late part of the observation. For the middle and lower reaches of the Yangtze River, the Sichuan Basin and the Yunnan-Guizhou Plateau, this plateau period occurred between 2008 and 2012. For southern China, CNDVI only stagnated between 2003 and 2008 and then showed the same steady increase until the end of the observation period.
FIGURE 4. Yearly CNDVI data Times Series plot with trendline from 2004 to 2016 in nine agricultural subdivisions of China in nine agricultural area [(A) Northeast China Plain, (B) Northern arid and semi-arid region, (C) Huang-Huai-Hai Plain, (D) Loess Plateau, (E) Qinghai Tibet Plateau, (F) Middle-lower Yangtze Plain, (G) Sichuan Basin and surrounding regions, (H) Southern China, (I) Yunnan-Guizhou Plateau].
Figure 5 shows the correlation coefficients of TWS and GRACE. In the Northeast Plain, the correlation coefficients generally fluctuate around 0. In the northern arid and semi-arid region, the part near the Loess Plateau and the northwest border, TWS and CNDVI show a negative correlation, and the correlation coefficients in the rest of the region are near zero. The central area of the Yellow-Huai-Hai Plain and all parts of the Loess Plateau also show negative correlations between TWS and CNDVI. In the center of the Qinghai-Tibet Plateau region, there is no significant trend in the correlation coefficients. However, on the edges of the region, positive correlations were mainly distributed. In the Sichuan Basin and surrounding area and the middle and lower reaches of the Yangtze River region, the correlation between CNDVI and TWS is not obvious in the north of these two regions, and the correlation coefficients begin to move toward positive correlation as it advances to the south. By the south of China and the Yunnan-Guizhou plateau, these regions have been dominated by positive correlations.
FIGURE 5. Spatial Correlation between TWS and CNDVI with p-value under 0.05 [(A) Northeast China Plain, (B) Northern arid and semi-arid region, (C) Huang-Huai-Hai Plain, (D) Loess Plateau, (E) Qinghai Tibet Plateau, (F) Middle-lower Yangtze Plain, (G) Sichuan Basin and surrounding regions, (H) Southern China, (I) Yunnan-Guizhou Plateau].
The correlations between TWS and CNDVI showed a clear regional trend. In the water-limited area, the correlations were mainly negative. These areas form a band shape from the central part of the Huang-Huai-Hai Plain in the east to the western boundary of the northern semi-arid region in the west. The negative correlations are mainly spread in this belt area. Another distinct regional trend appears in the south, starting from the southern part of the Sichuan basin and the middle and lower reaches of the Yangtze River region, and covering the whole of southern China and the Yunnan-Guizhou plateau, where positive correlations dominate. Positive correlations also appear in the northern part of the Tibetan Plateau, but the TWS data include the influence of melting snow and ice, and it is unclear whether the trend is due to the influx of melting snow and ice into the region. As can be seen in Figure 5, the results of the correlation analysis are consistent with statistical significance in the two regions with significant positive and negative correlations. In the other regions, the correlation analysis could not pass the test. NA values indicate that there is no cropland in the area.
The shift in the center of gravity of CNDVI provides evidence of a shift in China’s grain production pattern. The absolute value of CNDVI cannot accurately predict grain production, but the direction of change can indicate relative changes in production patterns. Wang et al. (2018) collected grain production data from the China Statistical Yearbook and field research data, found a similar pattern. From 1996 to 2005, the center of gravity of grain production showed a “northward and westward” trend. The center of gravity moved faster and faster, and according to Wang et al. (2018) estimate, the center of gravity moved from 7.6 km/a in the early period to 41.2 km/a at the end of the observation period (Jieyong and Yansui, 2009). They explain this phenomenon from several aspects. In terms of water resources, drilling of wells to develop groundwater resources has been vigorously developed in the north since the 1980’s (Wang et al., 2018). On the other hand, the promotion of agricultural films has caused a much higher replanting index in many northern areas, and many 1-year maturity areas can reach 2-year maturity and 2-year maturity (Ye et al., 2012).
The TWS extracted from the GRACE program provided results consistent with Common sense. The GRACE satellite data shows a situation that coincides with the distribution of China’s water resources, that is, the total amount of water resources, although significant, is unevenly distributed in the south and north part of this country. The three regions where the GRACE data shows a significant decline: The North China Plain, the Loess Plateau, and the desert-strewn northwest are precisely the regions where water resources face a considerable shortage. In these regions, where water resources are less distributed, the continuous decline of water resources releases a danger signal. In the water-rich southern and northeastern regions, which are also experiencing rapid socioeconomic development, water resources are not significantly affected. Unlike water resources, all nine agricultural regions showed steady growth in food production, except for the Qinghai-Tibet Plateau region.
Although water resources show large fluctuations during the observation period in the Northeast Plain, there is no significant decrease in CNDVI during the whole period. Combined with the CNDVI data, agricultural activities on water resources are relatively small in the northeastern region in the short term, and it is still a stable grain production center in China. Although there was no significant decrease in TWS data before and after the observation period, Zhang et al. (2018) used the Weather Research and Forecasting (WRF) model to simulate the water balance and assess the impact of agriculture on water resources for each decade from 1910 to 2010 based on a series of interdecadal land use datasets. They found no significant trend in precipitation in the Northeast Plain. However, canopy transpiration and interception evaporation increased and runoff and infiltration decreased. This suggests that agricultural expansion in the region may put significant pressure on this vital food production base in the long term.
The Loess Plateau is the most eroded area in China (Liu and Diamond, 2005). There are four leading causes of soil erosion on the Loess Plateau: topography, soil type, climate, and vegetation. The concentrated rainfall in July, August, and September and the erosion-prone loess have caused severe soil erosion on the Loess Plateau. Soil erosion takes away local water resources not only directly but also indirectly leads to the rapid loss of water resources in this region due to the lack of soil as a carrier. Furthermore, the expansion of agriculture will undoubtedly exacerbate this trend. As more and more land is cleared for agriculture, irrigation water and crop evapotranspiration will increase the water shortage in the region.
The Huang-Huai-Hai Plain is also facing severe water shortages. Groundwater Funnel has been used to describe the increasing water shortage in northern China (Chen et al., 2011). Many researchers have conducted studies on this phenomenon, and the over-exploitation of groundwater is the likely culprit (Changming et al., 2001; Yuan et al., 2004; Zhang et al., 2004). In the Beijing-Tianjin-Hebei region, agriculture accounts for over 70% of total water use (National Bureau of Statistics of China, 2020). The primary cropping system in the region is the winter wheat-summer corn annual rotation (double cropping). The production of winter wheat in the region is increasing year by year. However, in terms of climatic factors, the annual precipitation only meets about 65% of the region’s agricultural water needs (Yuan et al., 2004). Further, during the growing period of winter wheat, precipitation is even more scarce and the deficit is mainly supplemented by groundwater. Over-dependence on groundwater water resources and uncontrolled exploitation of groundwater, in turn, destabilize the local water cycle and accelerate the loss of water resources. Taken together, water resource management in these water-limited areas is unsustainable. Consequently, the growth dynamics of grain production are also unsustainable in the long run.
The Northern arid and semi-arid region, as the name of the region describes, has less annual precipitation than potential evaporation. In the western part of the region, the average annual rainfall in the arid region was between 70 and 100 mm between 1990 and 2010, while in the semi-arid region in the eastern part, the average annual rainfall during the same period was between 300 and 440 mm (National Bureau of Statistics of China, 2020). Grasslands and deserts cover the entire region, and rainfall decreases from east to west (John et al., 2009). Water resources here are also increasingly challenged by GRACE data. In these areas, water resources are clearly a limiting factor for ecosystem development. The average annual available water resource in the region is only 1,275.8 km2, or 4.6% of the national total, with an average annual deficit of at least 36.53 km2 (Xia et al., 2017).
Due to abundant rainfall and rich water reserves, the overall trend of water resources in the south is stable, and even some areas show growth at the end of the observation period compared to the beginning. Natural conditions indicate that these areas still have an enormous potential for grain production.
Water, an essential input in agricultural production, can also eventually lead to the suspension of the entire grain production process after it is continuously outflowed from the region. The correlation between TWS and CNDVI releases a potential danger signal. Due to a variety of factors such as cost and uneven economic development, the northern region is beginning to assume a larger share of food production. Such a production pattern is not in line with the water distribution pattern of China. The region, represented by the Huang-Huai-Hai plain, suffers from perennial water shortages and over-exploits groundwater to irrigate farmland. Other water-scarce northern regions are facing similar dilemmas. This situation may eventually lead to the depletion of the agricultural production potential of these regions and, at a certain point, to a significant decrease in grain production in these regions. Kang et al. (2009) found that although crop yield increases with expanded or intensified irrigation, this may increase the rate of environmental degradation. Also they found through modeling that crop yields are more sensitive to precipitation than temperature. Shu et al. (2021) observed a similar water dilemma by analyzing the water footprint of all Chinese provinces. Green water scarcity poses a challenge to agricultural water management in most regions, with important areas for crop export in the north such as the North China Plain, and the Northeast Plain showing high growth in blue, green and gray water footprints. In contrast, in the southwest and southeast, relatively abundant water resources meet the water requirements for crop growth, but green water scarcity limits the long-term development of agriculture (Shu et al., 2021; Zhai et al., 2021).
From the results, GRACE data and CNDVI data exhibit linear correlation in some areas, and this correlation has a clear spatial distribution. In general, TWS and CNDVI are negatively correlated in the western and northern parts of China. In the east and south of China, where water resources are more abundant compared to the North, TWS and CNDVI simultaneously increase steadily and show a positive correlation over the observation period.
The trajectory of center-of-gravity and spatial correlation analysis detected the coupling between changes in grain production patterns and changes in water resources in China at the overall and regional levels, respectively. However, behind the continuous years of grain production increase, there is also a possible crisis. Due to the rapid progressing of urbanization and economic development in the south, agriculture has not developed as fast as in the north. This development reversal and the spatial distribution of water resources have created a contradiction. Compared to the southeast, where rainfall is plentiful, water resources become increasingly scarce as the perspective shifts to the west and north inland. This contradiction lays the groundwork for China’s future agricultural development. Whether it is a shift in center of gravity or spatial correlation, the satellite data may reveal the internal-logic changes in China’s agricultural development behind the scenes. On the one hand, the uneven distribution of water resources in China has been a nuisance for many years. The pattern of China’s food production under the influence of various factors such as climate shows a worrying change.
The endless demand for water from natural bodies of water, including groundwater, to irrigate farmland will sooner or later trigger a water shortage at some point in the future. This will not only force agricultural development in these areas to stagnate but also affect water for domestic and industrial use, ultimately hindering the development of society as a whole. Therefore, policy makers must balance the agricultural development between the north and the south, give full play to the advantages of abundant water resources in the south, and reasonably arrange the spatial pattern of agricultural development. Potential measures include: 1) limiting land reclamation in northern regions; 2) promoting water conservation systems to slow down the exploitation of groundwater resources; 3) promoting agricultural technology innovation, introducing drought-tolerant varieties; appropriately encouraging agricultural reclamation in southern regions.
This study examines the use of satellite data for large scale water resources (including groundwater) and its possible applications. At this stage, satellite data still have some limitations, taking GRACE satellite data as an example, the twin satellite has only collected 18 years of data since it was first launched in 2004, and only up to 18 matched pairs can be generated when performing interannual analysis. Also, due to the low spatial resolution of GRACE’s data, only 1° and 0.5° resolutions are available. Two characteristics of GRACE satellite products cause limitations in using this data for use at small scales. Therefore, future research could focus on using data fusion techniques to provide time series of sufficient density to provide more refined and reliable statistical analysis support for water resources management in an agricultural context.
The original contributions presented in the study are included in the article/Supplementary Material, further inquiries can be directed to the corresponding author.
Conceptualization, YF and JW. Methodology, YF and JW. Software, YF. Validation, YF. Formal analysis, YF. Data curation, YF. Writing—original draft preparation, YF. Writing—review and editing, YF and JW. Visualization, YF. Supervision, JW. Project administration, JW. Funding acquisition, JW. All authors have read and agreed to the published version of the manuscript.
This research was funded by the National Natural Science Foundation of China (Grant No. 42171266), and by the Strategic Priority Research Program of the Chinese Academy of Sciences (Grant No. XDA28130400).
The authors declare that the research was conducted in the absence of any commercial or financial relationships that could be construed as a potential conflict of interest.
All claims expressed in this article are solely those of the authors and do not necessarily represent those of their affiliated organizations, or those of the publisher, the editors and the reviewers. Any product that may be evaluated in this article, or claim that may be made by its manufacturer, is not guaranteed or endorsed by the publisher.
CNDVI, Cumulated Normalized Difference Vegetation Index; ESA, European Space Agency; GLR, German Aerospace Center; GRACE, Gravity Recovery and Climate Experiment; TWS, Total Water Storage; LCCS, Land Cover Classification System; NDVI, Normalized Difference Vegetation Index.
Ahmed, K. R., and Akter, S. (2017). Analysis of Landcover Change in Southwest Bengal Delta Due to Floods by NDVI, NDWI and K-Means Cluster with Landsat Multi-Spectral Surface Reflectance Satellite Data. Remote Sens. Appl. Soc. Environ. 8, 168–181. doi:10.1016/j.rsase.2017.08.010
Baret, F., and Guyot, G. (1991). Potentials and Limits of Vegetation Indices for LAI and APAR Assessment. Remote Sens. Environ. 35, 161–173. doi:10.1016/0034-4257(91)90009-U
Bigot, J., and Klein, T. (2018). Characterization of Barycenters in the Wasserstein Space by Averaging Optimal Transport Maps. Esaim Ps. 22, 35–57. doi:10.1051/ps/2017020
Bonell, M. (2002). Ecohydrology-a Completely New Idea? Hydrological Sci. J. 47, 809–810. doi:10.1080/02626660209492984
Bontemps, S., Herold, M., Kooistra, L., van Groenestijn, A., Hartley, A., Arino, O., et al. (2011). Revisiting Land Cover Observations to Address the Needs of the Climate Modelling Community. Biogeosciences Discuss. 8, 7713–7740. doi:10.5194/bgd-8-7713-2011
Cao, Y., Nan, Z., and Cheng, G. (2015). GRACE Gravity Satellite Observations of Terrestrial Water Storage Changes for Drought Characterization in the Arid Land of Northwestern China. Remote Sens. 7, 1021–1047. doi:10.3390/rs70101021
Changming, L., Jingjie, Y., and Kendy, E. (2001). Groundwater Exploitation and its Impact on the Environment in the North China Plain. Water Int. 26, 265–272. doi:10.1080/02508060108686913
Chen, B., Gong, H., Li, X., Lei, K., Zhang, Y., Li, J., et al. (2011). Spatial-temporal Characteristics of Land Subsidence Corresponding to Dynamic Groundwater Funnel in Beijing Municipality, China. Chin. Geogr. Sci. 21, 753–764. doi:10.1007/s11769-011-0509-6
Colwell, J. E. (1974). Vegetation Canopy Reflectance. Remote Sens. Environ. 3, 175–183. doi:10.1016/0034-4257(74)90003-0
Elahi, E., Khalid, Z., Tauni, M. Z., Zhang, H., and Lirong, X. (2021a). Extreme Weather Events Risk to Crop-Production and the Adaptation of Innovative Management Strategies to Mitigate the Risk: A Retrospective Survey of Rural Punjab, Pakistan. Technovation, 102255. In Press. doi:10.1016/j.technovation.2021.102255
Elahi, E., Khalid, Z., and Zhang, Z. (2022a). Understanding Farmers' Intention and Willingness to Install Renewable Energy Technology: A Solution to Reduce the Environmental Emissions of Agriculture. Appl. Energy 309, 118459. doi:10.1016/j.apenergy.2021.118459
Elahi, E., Zhang, H., Lirong, X., Khalid, Z., and Xu, H. (2021b). Understanding Cognitive and Socio-Psychological Factors Determining Farmers' Intentions to Use Improved Grassland: Implications of Land Use Policy for Sustainable Pasture Production. Land use policy 102, 105250. doi:10.1016/j.landusepol.2020.105250
Elahi, E., Zhang, Z., Khalid, Z., and Xu, H. (2022b). Application of an Artificial Neural Network to Optimise Energy Inputs: An Energy- and Cost-Saving Strategy for Commercial Poultry Farms. Energy 244, 123169. doi:10.1016/j.energy.2022.123169
ESA (2017). Land Cover CCI Product User Guide Version 2. Available at: https://maps.elie.ucl.ac.be/CCI/viewer/download/ESACCI-LC-Ph2-PUGv2_2.0.pdf (Accessed November 23, 2021).
Famiglietti, J. S., Lo, M., Ho, S. L., Bethune, J., Anderson, K. J., Syed, T. H., et al. (2011). Satellites Measure Recent Rates of Groundwater Depletion in California's Central Valley. Geophys. Res. Lett. 38, L03403. doi:10.1029/2010GL046442
Feng, W., Zhong, M., Lemoine, J.-M., Biancale, R., Hsu, H.-T., and Xia, J. (2013). Evaluation of Groundwater Depletion in North China Using the Gravity Recovery and Climate Experiment (GRACE) Data and Ground-Based Measurements. Water Resour. Res. 49, 2110–2118. doi:10.1002/wrcr.20192
Goward, S. N., and Huemmrich, K. F. (1992). Vegetation Canopy PAR Absorptance and the Normalized Difference Vegetation Index: An Assessment Using the SAIL Model. Remote Sens. Environ. 39, 119–140. doi:10.1016/0034-4257(92)90131-3
Iqbal, N., Hossain, F., Lee, H., and Akhter, G. (2016). Satellite Gravimetric Estimation of Groundwater Storage Variations over Indus Basin in Pakistan. IEEE J. Sel. Top. Appl. Earth Obs. Remote Sens. 9, 3524–3534. doi:10.1109/JSTARS.2016.2574378
Jieyong, W., and Yansui, L. (2009). The Changes of Grain Output Center of Gravityand its Driving Forces in China since 1990. Resour. Sci. 31, 1188–1194.
John, R., Chen, J., Lu, N., and Wilske, B. (2009). Land Cover/land Use Change in Semi-arid Inner Mongolia: 1992-2004. Environ. Res. Lett. 4, 045010. doi:10.1088/1748-9326/4/4/045010
Kang, Y., Khan, S., and Ma, X. (2009). Climate Change Impacts on Crop Yield, Crop Water Productivity and Food Security - A Review. Prog. Nat. Sci. 19, 1665–1674. doi:10.1016/j.pnsc.2009.08.001
Landerer, F. W., and Swenson, S. C. (2012). Accuracy of Scaled GRACE Terrestrial Water Storage Estimates. Water Resour. Res. 48, 1–11. doi:10.1029/2011WR011453
le Maire, G., Marsden, C., Nouvellon, Y., Grinand, C., Hakamada, R., Stape, J.-L., et al. (2011). MODIS NDVI Time-Series Allow the Monitoring of Eucalyptus Plantation Biomass. Remote Sens. Environ. 115, 2613–2625. doi:10.1016/j.rse.2011.05.017
Liu, J., and Diamond, J. (2005). China's Environment in a Globalizing World. Nature 435, 1179–1186. doi:10.1038/4351179a
Lopresti, M. F., Di Bella, C. M., and Degioanni, A. J. (2015). Relationship between MODIS-NDVI Data and Wheat Yield: A Case Study in Northern Buenos Aires Province, Argentina. Inf. Process. Agric. 2, 73–84. doi:10.1016/j.inpa.2015.06.001
Ministry of Water Resources (2010). Bulletin of Flood and Drought Disasters in China 2010. Beijing: China Water Power Press.
Cooley, S. S., and Landerer, F. W. (2019). “GRACE D-103133 Gravity Recovery and Climate Experiment Follow-on (GRACE-FO) Level-3 Data Product User Handbook,” in NASA Jet Propulsion Laboratory (Pasadena, CA: California Institute of Technology).
National Agricultural Zoning Committee (1981). Comprehensive Agricultural Zoning of China. Beijing: China Agriculture Press. The map is available at https://www.resdc.cn/data.aspx?DATAID=275.
National Bureau of Statistics of China (2020). China Statistical Yearbook. Beijing: China Agriculture Press. Available at http://www.stats.gov.cn/tjsj./ndsj/(Accessed November 23, 2021).
Panek, E., and Gozdowski, D. (2020). Analysis of Relationship between Cereal Yield and NDVI for Selected Regions of Central Europe Based on MODIS Satellite Data. Remote Sens. Appl. Soc. Environ. 17, 100286. doi:10.1016/j.rsase.2019.100286
Prasad, A. K., Chai, L., Singh, R. P., and Kafatos, M. (2006). Crop Yield Estimation Model for Iowa Using Remote Sensing and Surface Parameters. Int. J. Appl. Earth Observation Geoinformation 8, 26–33. doi:10.1016/j.jag.2005.06.002
Ren, J., Chen, Z., Zhou, Q., and Tang, H. (2008). Regional Yield Estimation for Winter Wheat with MODIS-NDVI Data in Shandong, China. Int. J. Appl. Earth Observation Geoinformation 10, 403–413. doi:10.1016/j.jag.2007.11.003
Rodell, M., and Famiglietti, J. S. (2001). An Analysis of Terrestrial Water Storage Variations in Illinois with Implications for the Gravity Recovery and Climate Experiment (GRACE). Water Resour. Res. 37, 1327–1339. doi:10.1029/2000WR900306
Rodell, M., Houser, P. R., Jambor, U., Gottschalck, J., Mitchell, K., Meng, C.-J., et al. (2004). The Global Land Data Assimilation System. Bull. Amer. Meteor. Soc. 85, 381–394. doi:10.1175/BAMS-85-3-381
Shu, R., Cao, X., and Wu, M. (2021). Clarifying Regional Water Scarcity in Agriculture Based on the Theory of Blue, Green and Grey Water Footprints. Water Resour. Manage 35, 1101–1118. doi:10.1007/s11269-021-02779-6
Smith, M. R., and Dicken, P. (2000). Global Shift: Transforming the World Economy. Can. J. Sociol./Cahiers Can. de Sociol. 25, 399. doi:10.2307/3341650
Thenkabail, P. S., Smith, R. B., and De Pauw, E. (2000). Hyperspectral Vegetation Indices and Their Relationships with Agricultural Crop Characteristics. Remote Sens. Environ. 71, 158–182. doi:10.1016/S0034-4257(99)00067-X
Wahr, J., Swenson, S., Zlotnicki, V., and Velicogna, I. (2004). Time-variable Gravity from GRACE: First Results. Geophys. Res. Lett. 31. doi:10.1029/2004GL019779
Wang, J., Rich, P. M., Price, K. P., and Kettle, W. D. (2005). Relations between NDVI, Grassland Production, and Crop Yield in the Central Great Plains. Geocarto Int. 20, 5–11. doi:10.1080/10106040508542350
Wang, J., Zhang, Z., and Liu, Y. (2018). Spatial Shifts in Grain Production Increases in China and Implications for Food Security. Land use policy 74, 204–213. doi:10.1016/j.landusepol.2017.11.037
Xia, J., Ning, L., Wang, Q., Chen, J., Wan, L., and Hong, S. (2017). Vulnerability of and Risk to Water Resources in Arid and Semi-arid Regions of West China under a Scenario of Climate Change. Clim. Change 144, 549–563. doi:10.1007/s10584-016-1709-y
Yang, Y., Long, D., Guan, H., Scanlon, B. R., Simmons, C. T., Jiang, L., et al. (2014). GRACE Satellite Observed Hydrological Controls on Interannual and Seasonal Variability in Surface Greenness over Mainland Australia. J. Geophys. Res. Biogeosci. 119, 2245–2260. doi:10.1002/2014JG002670
Ye, T., Shi, P., Wang, J. a., Liu, L., Fan, Y., and Hu, J. (2012). China's Drought Disaster Risk Management: Perspective of Severe Droughts in 2009-2010. Int. J. Disaster Risk Sci. 3, 84–97. doi:10.1007/s13753-012-0009-z
Yuan, G., Luo, Y., Sun, X., and Tang, D. (2004). Evaluation of a Crop Water Stress Index for Detecting Water Stress in Winter Wheat in the North China Plain. Agric. Water Manag. 64, 29–40. doi:10.1016/S0378-3774(03)00193-8
Zhai, Y., Zhang, T., Bai, Y., Ji, C., Ma, X., Shen, X., et al. (2021). Energy and Water Footprints of Cereal Production in China. Resour. Conservation Recycl. 164, 105150. doi:10.1016/j.resconrec.2020.105150
Zhang, L., Wang, C., Li, X., Zhang, H., Li, W., and Jiang, L. (2018). Impacts of Agricultural Expansion (1910s-2010s) on the Water Cycle in the Songneng Plain, Northeast China. Remote Sens. 10, 1108. doi:10.3390/rs10071108
Zhang, Y., Kendy, E., Qiang, Y., Changming, L., Yanjun, S., and Hongyong, S. (2004). Effect of Soil Water Deficit on Evapotranspiration, Crop Yield, and Water Use Efficiency in the North China Plain. Agric. Water Manag. 64, 107–122. doi:10.1016/S0378-3774(03)00201-4
Keywords: grain production pattern, spatial mismatch, water resource, satellite data, China
Citation: Feng Y and Wang J (2022) Detecting the Spatial Mismatch of Water Resources and Grain Planting Pattern Changes in China Based on Satellite Data. Front. Environ. Sci. 10:904779. doi: 10.3389/fenvs.2022.904779
Received: 25 March 2022; Accepted: 06 May 2022;
Published: 16 June 2022.
Edited by:
Jinlong Gao, Nanjing Institute of Geography and Limnology (CAS), ChinaReviewed by:
Ehsan Elahi, Shandong University of Technology, ChinaCopyright © 2022 Feng and Wang. This is an open-access article distributed under the terms of the Creative Commons Attribution License (CC BY). The use, distribution or reproduction in other forums is permitted, provided the original author(s) and the copyright owner(s) are credited and that the original publication in this journal is cited, in accordance with accepted academic practice. No use, distribution or reproduction is permitted which does not comply with these terms.
*Correspondence: Jieyong Wang, d2p5QGlnc25yci5hYy5jbg==
Disclaimer: All claims expressed in this article are solely those of the authors and do not necessarily represent those of their affiliated organizations, or those of the publisher, the editors and the reviewers. Any product that may be evaluated in this article or claim that may be made by its manufacturer is not guaranteed or endorsed by the publisher.
Research integrity at Frontiers
Learn more about the work of our research integrity team to safeguard the quality of each article we publish.