- 1State Environment Protection Key Laboratory of Satellite Remote Sensing, Aerospace Information Research Institute, Chinese Academy of Sciences, Beijing, China
- 2University of Chinese Academy of Sciences, Beijing, China
- 3School of Remote Sensing and Information Engineering, North China Institute of Aerospace Engineering, Langfang, China
- 4State Key Laboratory of Remote Sensing Science, Aerospace Information Research Institute, Chinese Academy of Sciences and Beijing Normal University, Beijing, China
Retrieval of aerosol optical depth (AOD) and bidirectional reflectance over Case II waters is a challenging task because of the optical complexity of water and the significant influence of atmospheric scattering. We present a simultaneous retrieval algorithm, coupled with a bidirectional reflectance distribution function (BRDF) model centered on the water’s Inherent Optical Property (IOP), to retrieve AOD and bidirectional reflectance based on multi-angle measurements from the Multi-angle Imaging SpectroRadiometer (MISR) sensor. The algorithm was evaluated through retrievals performed in the proximity of four AERONET sites characterized by various water types. Consistency was observed between the results and in situ measurements. Accurate AODs can be retrieved, and atmospheric overcorrection can be avoided when obtaining remote sensing reflectance. Furthermore, the normalized remote sensing reflectance derived from our results showed a good correlation with the AERONET-OC products derived using the IOP-based correction approach. Our study provides theoretical support for future quantitative remote-sensing studies.
1 Introduction
Depending on their optical properties, natural waters can be divided into Case I and Case II waters (Morel and Prieur, 1977; IOCCG, 2000). Case II (mainly coastal and inland) waters are of interest for remote sensing because of their biological productivity, but require accurate atmospheric correction. Furthermore, the simultaneous determination of aerosol properties is beneficial because of the established connection between air quality and human health (Brunekreef and Holgate, 2002). In the atmosphere-underlying water surface system over clear oceanic waters, atmospheric aerosols contribute the largest uncertainties because most of the signal reaching the sensor is due to atmospheric scattering effects (Gordon and Morel, 1983; Gordon and Wang, 1994). Accurate aerosol optical properties are important for the quantitative remote sensing of ocean color (Gordon, 1997; Lewis et al., 2004; Wang, 2010). Although the significance of aerosol water surfaces has been well recognized in previous studies, it remains a challenging task to constrain the impacts of the atmosphere-underlying water surface system on the climate change modeling and the global carbon budget (Charlson et al., 1992; Bauer et al., 2013; Suzuki and Takemura, 2019).
Observational data at all scales and precise models are required for the validation of aerosol and water properties (Remer et al., 2019a). The AERONET (AErosol RObotic NETwork) and its AERONET-OC (AERONET-Ocean Color) component provide valid examples of data collection containing indispensable and detailed information (Holben et al., 1998; Zibordi et al., 2009). However, these ground-based observations are typically limited geographically, leaving many oceans and lakes under sampled (Smirnov et al., 2009). Only space-based remote sensing satisfies the requirements of frequent and consistent observations.
The accuracy of aerosol property retrieval can exert a notable influence on remote sensing reflectance (Gordon and Morel, 1983; Fukushima and Toratani, 1997; Gordon et al., 1997; Chomko and Gordon, 1998). The atmospheric correction is commonly used to decouple the atmosphere and waters. To retrieve the aerosol properties over waters, there are some algorithms based on the assumption that the water-leaving radiance at a certain band can be neglected (Remer et al., 2005). These algorithms have been improved by the better fitting aerosol models and more suitable channels (Gordon and Wang, 1994; Fukushima et al., 1998; Antoine and Morel, 1999; Gao et al., 2000). To improve the accuracy of remote sensing reflectance, vicarious calibration has been developed using satellite measurements and in situ observations data (Gordon, 1998; Wang and Franz, 2000).However, recent studies suggested that those atmospheric correction methods are still questionable over Case II waters because of the complex aerosol and water conditions (Jamet et al., 2011; Goyens et al., 2013; Fan et al., 2017).
Conversely, the water body contribution to the Top-of-the-Earth Atmosphere (TOA) is non-negligible (Gordon and Wang, 1994; Lavender et al., 2005; Sayer et al., 2010), and the uncertainties in the determination of the BRDF affect the accuracy of the aerosol retrievals. (Morel and Gentili, 1996; Tanré et al., 1997; Yang and Gordon, 1997). Research on the bidirectional effects began with clear Case I waters (mainly clear oceanic) waters, where the optical properties are mainly controlled by phytoplankton and the phase function is related to the concentration of chlorophyll a (Loisel and Morel, 2001). The models were confirmed by measurements (Morel et al., 1995) and remote sensing data (Morel and Gentili, 1996). However, the optical properties of turbid water are more complex. For Case II waters, semi-empirical models can provide physical interpretations with reliable simulations at low computational cost (Hlaing et al., 2012). They are widely used in water color remote sensing algorithms (Gleason et al., 2012). Han et al. (2022)compared five popular semi-empirical BRDF models (Morel et al., 2002; Lee et al., 2004; Park and Ruddick, 2005; Vanderwoerd and Pasterkamp, 2008; Lee et al., 2011) in turbid waters using MISR data, and showed that Lee et al. (2011) IOP-centered model was stable in Case II waters.
As the atmosphere-underlying water surface system for Case II waters is coupled, a rigorous method is required to retrieve the aerosol properties and bidirectional reflectance. Multi-angle sensors provide additional information that cannot be obtained using single-view radiometers. Several sensors are available, such as the ATSR (Along-Track Scanning Radiometer), AATSR (Advanced Along-Track Scanning Radiometer), MISR (Multi-angle Imaging SpectroRadiometer) and POLDER (POLarization and Directionality of the Earth’s Reflectance). Qin et al. (2015) used AATSR data over Australia to simultaneously retrieve the aerosol properties and BRDF. Shi et al. (2017) adopted a gradient optimization method and retrieved AOD and land BRDF from AASTR data. Martonchik et al. (1998) developed an algorithm for retrieving aerosol properties for heterogeneous land based on MISR observations. There are several satellite missions for this purpose. NASA’s PACE (Plankton, Aerosol, Clouds, ocean Ecosystem) mission aims to apply observations of two multi-angle instruments (Remer et al., 2019b). The European Space Agency (ESA) and the European Organization for the Exploitation of Meteorological Satellites (EUMETSAT) developed the Meteorological Operational Satellite Second Generation (MetOp-SG) program, hosting the Multi-Viewing, Multi-Channel, Multi-Polarization imager (3MI) (Chauvigné et al., 2021), which accounts for BRDF effects in AOD retrievals.
Over open ocean, Pepijn Veefkind and de Leeuw (1998) presented a retrieval algorithm to determine the spectral aerosol optical depth from ATSR-2 dual-view data. However, they assumed that the form of the BRDF does not depend on the wavelength, which can lead to large errors in the retrieved spectral AOD over Case II waters. Ahmad et al. (2010) updated aerosol optical models for coastal regions and open oceans. The algorithm is based on the assumption that the water-leaving radiance is negligible for near-infrared wavelengths, which is unsuitable for Case II waters (Bailey et al., 2010). (Limbacher and Kahn, 2014; Limbacher and Kahn, 2017; Limbacher and Kahn, 2018) proposed a joint multi-angle aerosol retrieval algorithm for coastal eutrophic waters. Morel et al. (2002) introduced the bidirectional reflectance model in their study. This model accounts for the contribution of chlorophyll a to water-leaving radiance. Morel et al.‘s model ignores other optically active species in Case II waters, especially inland waters, such as Colored Dissolved Organic Matter (CDOM), Suspended Particulate Matter (SPM), and higher chlorophyll a concentration (Gleason et al., 2012). Shi and Nakajima (2018) presented an optimization approach for the simultaneous determination of AOD and normalized water-leaving radiance
In this study, we describe a simultaneous algorithm for retrieving AOD and bidirectional reflectance over coastal waters and lakes using MISR data. The BRDF model chosen for our algorithm was Lee et al. (2011) model based on IOPs for remote sensing reflectance. The proposed algorithm considers more complex water environments by relating the remote sensing reflectance with the inherent optical properties.
The remainder of this paper is organized as follows: Section 2 introduces the database and provides a description of the method used in the study; Section 3 presents the sensitivity study and validation. The results are discussed in Section 4. Finally, the conclusions are presented in Section 5.
2 Materials and Methods
2.1 Database
Multi-angle data were collected from the spaceborne sensor, MISR. The MISR instrument was launched aboard the Terra satellite in 1999. It provides extensive bidirectional reflectance data and has the advantage of accurate radiometric calibration. Nine cameras record observations in four spectral bands (446, 558, 672, and 867 nm) of the solar spectrum, with spatial sampling distances of 275 and 1100 m. One camera points toward the nadir and is referred to as “An”; another has a forward and aftward view angle of 26.1° and are referred to as “Af” and “Aa,” respectively; and those with view angles at 45.6°, 60.0°, and 70.5° are denoted as “B”, “C”, and “D”, respectively. Single-pixel observations were collected at nine angles over 7 min. Because the observations are collected rapidly, the effect of dynamic inland waters on the results is low. Multi-angle measurements provide the additional angular information which is sensitive to aerosol properties and is important to improve the accuracy of retrieval results. The raw data in this study include the TOA radiance from the MISR Level-1B product from 2015 to 2019, considering the complexity and variations of the atmosperic-underlying water surface system. The data set was selected to obtain a reasonable assessment of the retrieval algorithm.
The most common BRDF products are MODIS BRDF/Albedo Product and MISR Surface Parameter Product. The MODIS BRDF/Albedo Product retrieves measurements at intervals of 8,10 or 16 days (Schaaf et al., 2002), which is not a suitable timeframe for dynamic water. The MISR Surface Parameter Product is designed for retrievals over land (Diner et al., 1999), so it cannot represent the water surface BRDF. The AERONET-based Surface Reflectance Validation Network (ASRVN) is a processing system based on accurate aerosol measurements and is widely used for validation analysis (Yujie Wang et al., 2009). In this study, bidirectional reflectance data from MISR TOA acquired by produced following the ASVRN protocol were used to estimate the accuracy of the BRDF results.
The AERONET program is an international federation established by NASA and LOA-PHOTONS (CNRS) that provides access to a suite of data from established ground-based remote-sensing aerosol networks. AERONET provides detailed aerosol observations and information collected from a CIMEL CE-318 radiometer (Holben et al., 1998). In 2006, a new component (AERONET-OC) was established to support long-term water color research. AOD and normalized water-leaving radiance
ARIAKE_TOWER is an AERONET-OC site located in Ariake Sea, Japan. Ariake Sea is a semi-closed bay with the depth ranging from 50 to 70 m. The rivers carry significant sediment loads from the upstream basins into Ariake Sea. The pollution brought by the fishery production and reclamation of bays is an urgent problem to be solved (Gan et al., 2004).
Lucinda is an AERONET-OC site located in Lucinda Bay in the northeast Australia. Lucinda Bay is a tropical coastal area and is in the south of the Great Barrier Reef. The Great Barrier Reef is a world-famous natural heritage for the beautiful coral scenery and the water is clear. The special water environment also makes it a typical area for coastal research (Li et al., 2021).
Lake _Erie is an AERONET-OC site located in the western Lake Erie, which is one lake of the Great Lakes between United States and Canada. Lake Erie is the typical clear inland waters in studies of water color remote sensing (Mukherjee et al., 2016).
Taihu is an AERONET site is located in the Taihu Lake, which is China’s third-largest fresh water lake. The water in Taihu Lake is constantly turbid and eutrophic with a large area of optically deep waters (Shi et al., 2018). It represents an environment that is very different from clear oceanic waters and turbid coastal waters.
The satellite products are used in this study to evaluate the retrieval results. The MODIS product, MCD19A2, is produced by the Multi-angle Implementation of Atmospheric Correction (MAIAC) algorithm at a 1-km resolution (Lyapustin and Wang, 2007). The fine spatial resolution product is retrieved with parameters of a surface bidirectional reflectance factor (BRF) model developed by the MODIS measurements. The MISR product, MIL2ASAE, is developed by the aerosol retrieval algorithm of Version 23 at a 4.4-km resolution (Garay et al., 2020). The Version 23 data applied two retrieval methods for land surface and dark water, respectively.
The reflectance of optical shallow water with grass bottom is obviously higher than that of optically deep water in the Short Wavelength Infrared (SWIR) band, and the sandy bottom will cause high reflectance in the blue band (Mobley and Sundman, 2003). The MODIS SWIR and blue bands were used to filter out optically shallow waters, and the thresholds for reflectance were set as 0.02
2.2 Radiative Transfer Theory With BRDF Model
An atmospheric radiative transfer (RT) model is essential for this study. Assuming a plane-parallel atmosphere and Lambertian surface, the TOA reflectance can be modelled as follows (Liou, 2002):
where
The angular effect must also be considered because the water surface is not isotropic. The RT model with coupled BRDF can be described as follows (Tanre et al., 1983; Vermote et al., 1997; Vermote et al., 2006):
where
In Eq. 2, the contribution of the target to the TOA signal consists of five terms and is illustrated in Figure 1: (a) light directly transmitted to the water surface and back to the sensor without scattering; (b) light scattered in the atmosphere before reflecting off the water surface and directly transmitted back to the sensor; (c) light directly transmitted to the surface, scattered by the atmosphere and then reached the sensor; (d) light scattered in the atmosphere before reaching the water surface and then scattered on their way to the sensor; and (e) multiple interactions between atmosphere and water surface (Tanre et al., 1983; Shi et al., 2017).
where
BRDF model (Morel et al., 2002) is designed for clear open ocean and is regarded as the standard model for oceanic remote sensing. The model is written as follows:
where
Morel et al.‘s BRDF model accounts for Raman scattering and the variance of particle phase function with the chlorophyll concentration, which is unsuitable for turbid waters (Gleason et al., 2012).
In previous studies, an IOP-centered model was proposed by Lee et al. and validated to process large volumes of satellite data efficiently to describe the bidirectional reflectance of Case II waters surfaces (Lee et al., 2011). This model explicitly accounts for the scattering effects of the molecules and particles. Lee et al.‘s model is defined by a second-order equation as follows:
where
The BRDF coefficients
2.3 Simultaneous Retrieval Method
In the 6S (Second Simulation of a Satellite Signal in the Solar Spectrum) RT algorithm (Vermote et al., 2006), the atmospheric correction (AC) was applied concerning about the angular effects. The simultaneous retrieval method used in this study was developed using a 6S iteration process. A flowchart of the retrieval algorithm is shown in Figure 2.
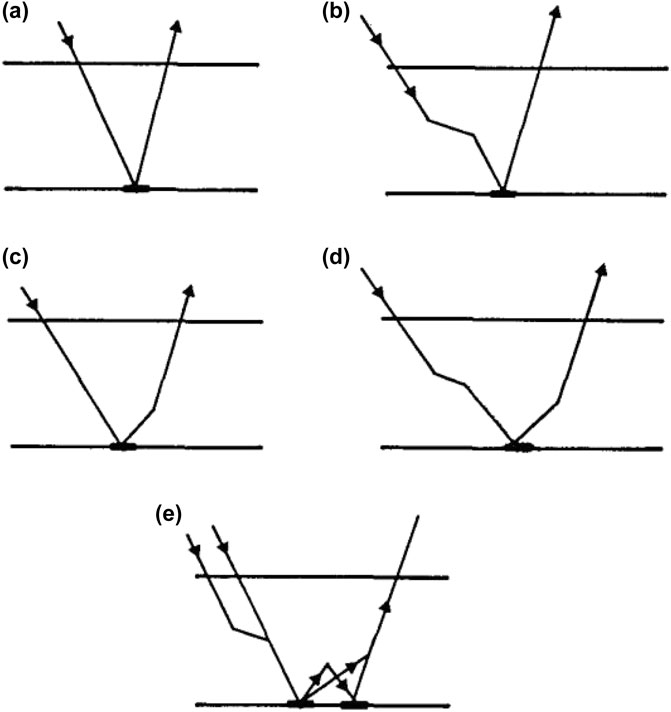
FIGURE 1. Types of radiation interactions in the coupled atmosphere-underlying water surface system (Tanre et al., 1983).
First, the coefficients
The evaluation methods included Pearson correlation coefficient (R), root-mean-square error (RMSE), and average percentage difference (APD). These values were calculated as follows:
where
2.3.1 Data Preprocessing
The dataset of the BRDF coefficients was simulated by Hydorlight with two particle phase functions, and the sampled values is validated in (Lee et al., 2011). The synthetic dataset of IOPs developed by IOCCG (IOCCG, 2006) are the inputs of the simulation. The outputs is a look-up table (LUT) of
The roughness of the water surface can lead to a highly directional signal, so several methods were used to constrain these uncertainties (Harmel and Chami, 2013; Knobelspiesse et al., 2020). In this study, data were filtered with a wind speed of less than 3 m/s, and it was important to apply a glitter test before the iteration (Cox, 1954; Cox and Munk, 1954; Cox and Munk, 1956). We performed the glitter test based on the study in (Limbacher and Kahn, 2017) and assessed the glitter contamination in each camera using a combination of the glitter angle and Rayleigh NIR reflectance.
2.3.2 AOD Retrieval
The current aerosol satellite remote sensing is still limited to aerosol models and optical properties. Considering that aerosol retrievals based on look-up tables (LUTs) are highly dependent on the aerosol models (Lee and Kim, 2010), we use Omar’s six aerosol optical models (Omar et al., 2005) to analyze aerosol over land and generate models over oceans. Omar et al. used the AERONET data to define the speciation of global aerosols and these aerosol optical models have been used widely in previous studies. However, the records from Omar’s models were mainly obtained over land. They are not suitable for the retrieval over oceans. Ahmad et al. (Ahmad et al., 2010) developed a set of aerosols models based on the records of two sites at coastal waters and eight sites at open ocean. However, we focus on Case II waters in this study, and Ahmad’s models might be un able to characterize the complex aerosol conditions over coastal waters, such as the pollution transported from land. Therefore, to generate accurate aerosol models over coastal waters oceans, we used the cluster analysis technique of principal component analysis (PCA) based on the entire archive of AERONET-OC data from 2002 to 2020, which had more than 2,500 valid records for 34 stations located in coastal waters. The aerosol parameters of the records, including aerosol size distributions, complex refractive indices, single scattering albedo (SSA), and asymmetry parameters, were set as the input of PCA technique. Average optical aerosol properties were clustered into four categories. The detailed parameters of the four aerosol models for the ocean are listed in Table 2.
In Table 2, g is the asymmetry parameter;
where
The four clusters in Figure 2 represents the four coastal aerosol models. The Cluster 1-2 are the aerosol models with most records and represent the most common aerosol types. The Cluster 3 characters high value of imaginary part of the complex refractive index and represents the aerosol type with high absorption. The Cluster four shows the high absorption and coarse modes. The records of Cluster four are 1% in the whole dataset and it may be due to the transport dust from land.
The six aerosol models in (Omar et al., 2005) were used as candidate aerosol models for inland waters (Taihu and Lake Erie in this study), and the four cluster models for aerosols over ocean were used for oceanic waters (ARIAKE_TOWER and Lucinda in this study). The LUTs of AOD were generate based on these models, too. We tried these LUTs in the retrieval algorithm and outputted the selected aerosol model with the minimized cost function.
To speed up the algorithm, we generate look-up tables to describe the relationship of
The AC was first performed for a range of AODs to get the bidirectional reflectance of the target pixel. The IOPs were obtained by fitting the bidirectional reflectance with the fixed values of
where
The value of
2.3.3 BRDF Retrieval
As mentioned in Section 2.2,
3 Results
3.1 Theoretical Sensitivity Study
To test the robustness of the simultaneous retrieval method, we conducted the theoretical sensitivity of the BRDF coefficients and aerosol models with the TOA reflectance from the forward simulation.
For the BRDF analysis, the simulation conditions were set as follows: the aerosol particles in the atmosphere were assumed as spherical particles; the solar zenith angle, the view zenith angle and the relative azimuth were set as 50°, 60° and 100°, respectively; the aerosol type was the Cluster 1 of Omar’s models; the true value of BRDF parameters were from Lee et al.‘s LUT; the AOD was set as 0.3. Then, the retrieval test was conducted with the TOA simulation and the relative error of the BRDF input ranges from 0 to 25%.
The relative errors of the retrieval results are shown in Figure 4. The relative errors of the retrieved AOD and
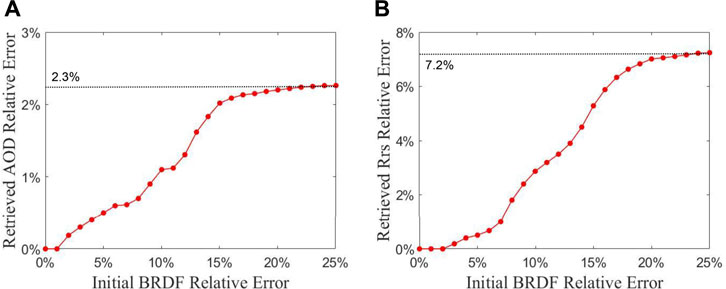
FIGURE 4. Sensitivity analysis of initial BRDF at 558 nm. (A) shows the relative error of the retrieved AOD. (B) shows the relative error of the retrieved
To analyze the sensitivity of different aerosol models, the simulation conditions were set as follows: the aerosol particles in the atmosphere were assumed as spherical particles; the solar zenith angle, the view zenith angle and the relative azimuth were set as 50°, 60° and 100°, respectively; the aerosol type was the Cluster 1 of Omar’s models for inland waters and Cluster 1 of the four categories in Table 2 for coastal waters; the true value of BRDF parameters were from Lee et al.‘s LUT; the AOD was set to range from 0.001 to 1.0. Then, the retrieval test was conducted with the TOA simulation when the candidate aerosol model was set as each category.
The results for inland and coastal waters are shown in Figure 5. The retrieval error increases with the true AOD value owing to the differences between the aerosol models. The deviation of aerosol properties over inland waters affected the retrieval accuracy more than that of coastal waters. Aerosol models have a certain effect on retrieval accuracy, and a more accurate aerosol model is helpful for reducing the relative error.
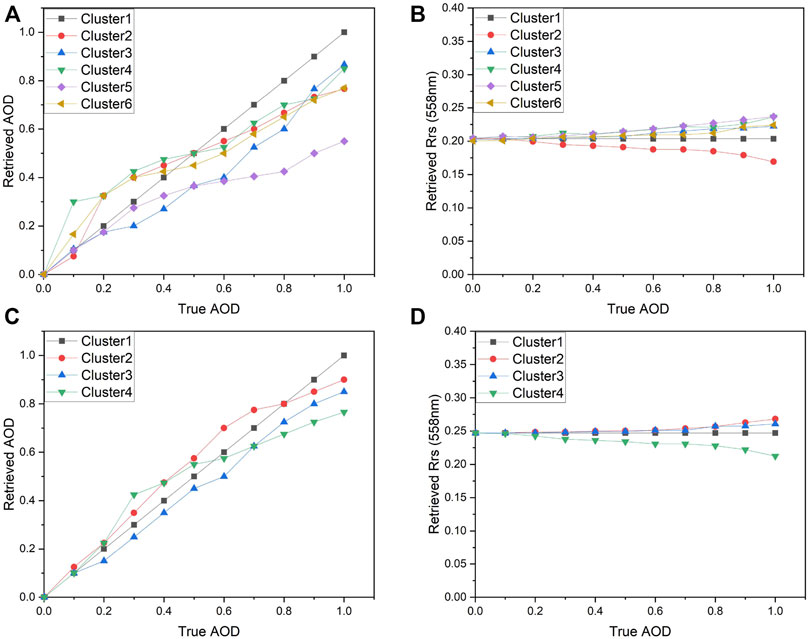
FIGURE 5. Sensitivity analysis of aerosol models. (A) and (B) show the results of retrieved AOD and
3.2 AOD Validation With AERONET Measurements and Satellite Products
We compared the retrieved AOD with AERONET AOD data and satellite products within ±30 min of satellite overpass time. The accuracy of the retrieval results, MODIS AOD product and MISR AOD product was evaluated using in situ data from 2015 to 2019. AERONET AOD measurements were converted to AOD at 558 nm using Angstrom turbidity formula (Holben et al., 2001). Aerosol optical depth data derived from the Terra images were selected for comparison with the retrieval results at 558 nm. We composed a program to obtain the matchups. First, we inputted the data lists of AERONET measurements, the retrieval results, MISR product, MODIS product, and outputted the data lists which all the product are available. Then, we focused on the pixel of the site locations and selected the valid results. Figure 6 shows the scatter plots and time series of the retrieval results and satellite product compared with AERONET measurements at the four sites along with the scatter plots.
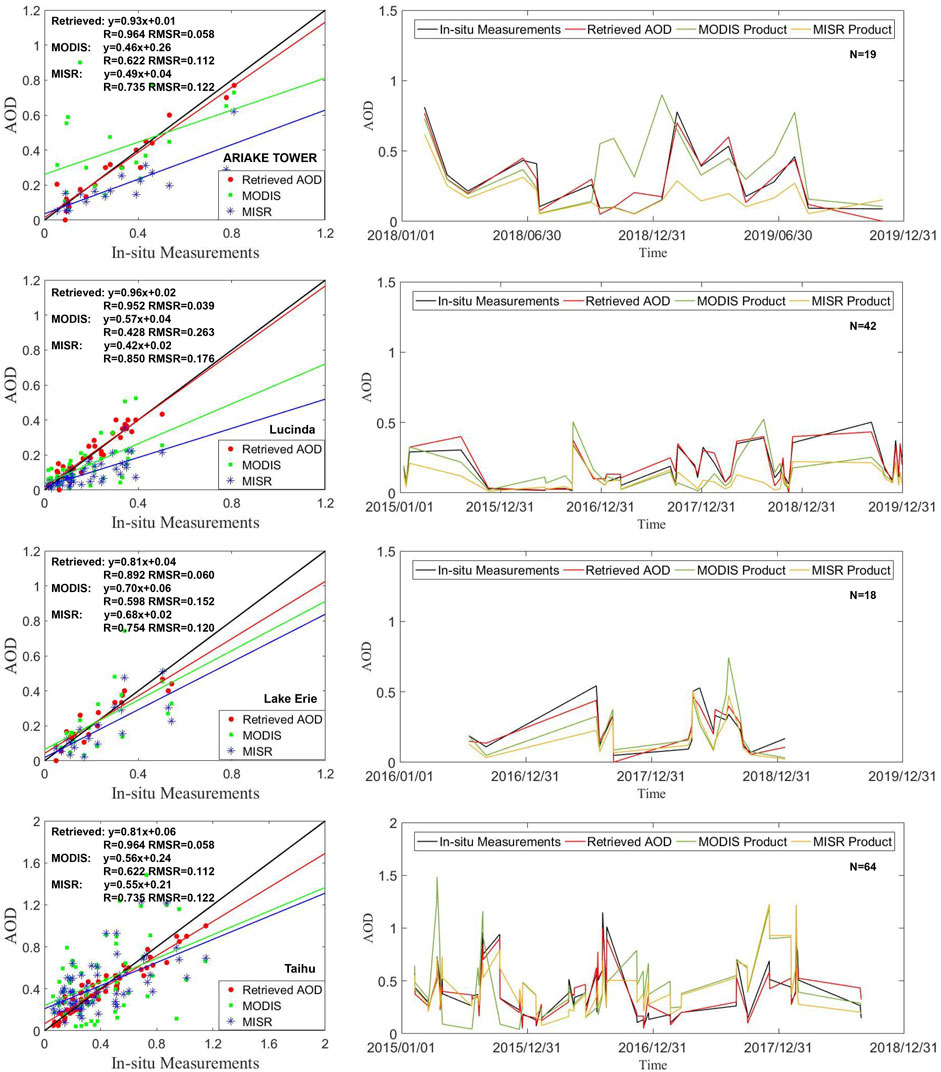
FIGURE 6. Scatter plots and time series of AOD products and AERONET measurements at 558 nm. In the plots, the solid black lines are the reference lines of 1:1; the solid red lines are the fitting lines of retrieved AOD; the solid green lines are the fitting lines of MODIS product; and the solid blue lines are the fitting lines of MISR product.
Our retrieval results show better consistency with the in situ measurements of AERONET than the MODIS and MISR products. The retrieved AOD at sites in coastal waters (ARIAKE TOWER and Lucinda) was more accurate than that of inland waters compared with AERONET data, with 84.2 and 83.3% of the data points falling within the error lines (
Some examples were selected for the comparison of the spatial distribution. The results are shown in Figure 7. The retrieved AOD images exhibit a higher coverage rate and larger spatial resolution.
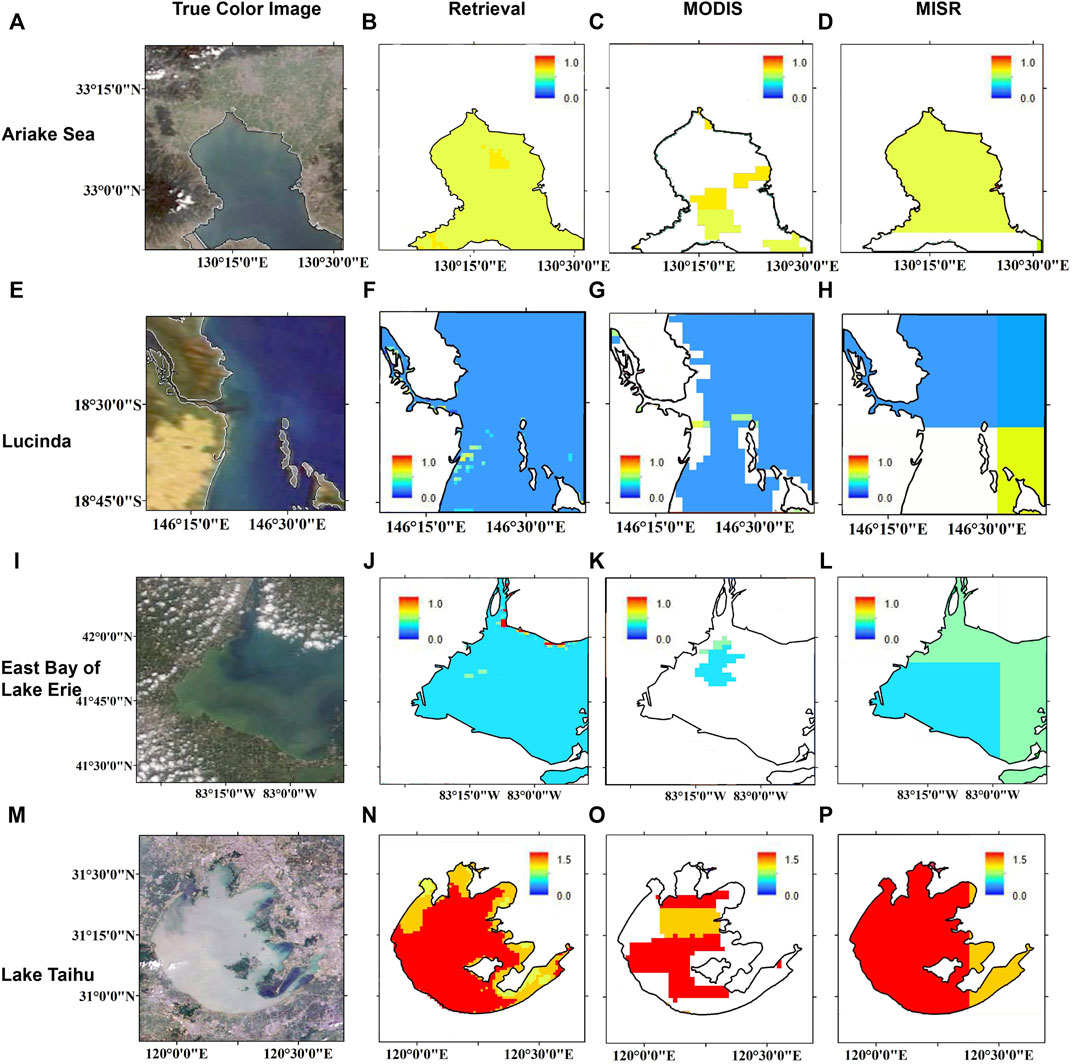
FIGURE 7. Comparison between AOD retrieval results and satellite products at green band. True-color image (A) of Ariake Sea on 25 March 2018, and comparison between the AOD retrieved with the presented method (B) and the products of MODIS (C) and MISR (D), at 558 nm. Panels (E–H), (I–L), and (M–P) are the same but for the Lucinda on 02 September 2018, the Lucinda on 26 August 2017, and the Lucinda on 28 April 2015.
3.3 BRDF Validation With Atmospherically Corrected Surface Reflectance
The MISR Surface Parameter Product is designed for retrievals over land (Diner et al., 1999), so it cannot represent the water surface BRDF. In this study, bidirectional reflectance data from MISR TOA acquired by produced following the ASVRN protocol were used to estimate the accuracy of the BRDF results. We compared directional remote sensing reflectance
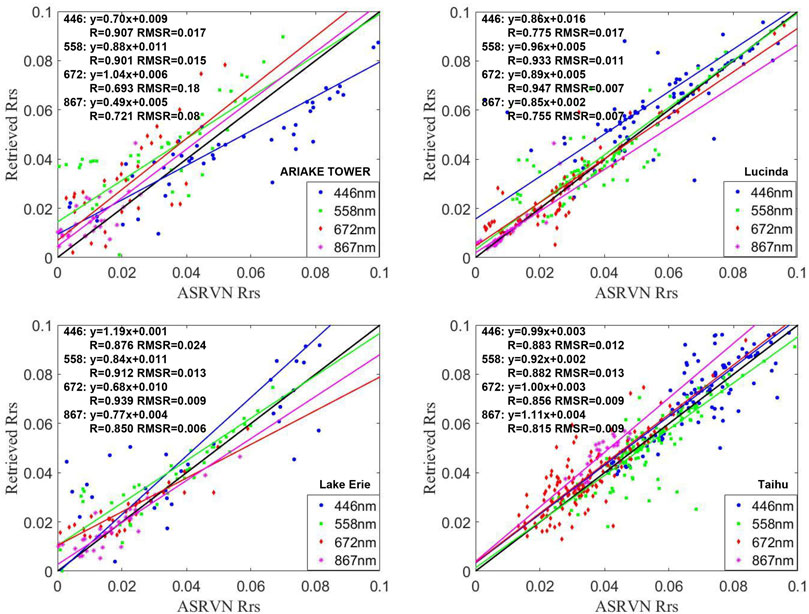
FIGURE 8. Plots of directional remote sensing reflectance and ASRVN. The solid blue lines in the figure is the fitting lines of 446 nm; the solid green lines in the figure is the fitting lines of 558 nm; solid red lines in the figure is the fitting lines of 672 nm; solid magenta lines in the figure is the fitting lines of 867 nm; and the 1:1 reference line is in solid black. The colors indicate the percentages of the points falling in each square.
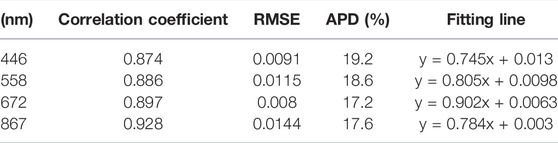
TABLE 3. Statistics for the comparison of directional remote sensing reflectance and ASRVN at each of the four bands.
The relative error between the directional remote sensing reflectance and ASRVN data with respect to the
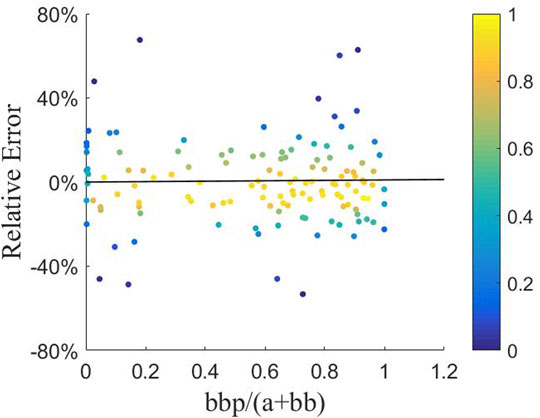
FIGURE 9. Scatter density plots of relative error between the directional remote sensing reflectance and ASRVN results with the ratio of
3.4 BRDF Validation With AERONET-OC Measurements
The in-situ data of normalized water-leaving radiance
The retrieved bidirectional remote sensing reflectance was converted to normalized values to There are two sets of
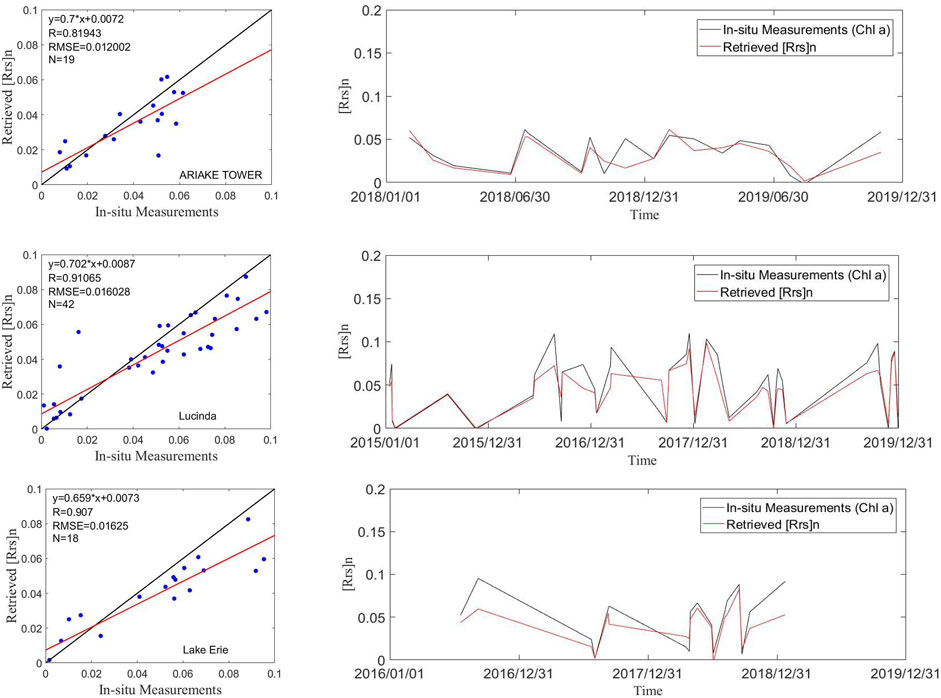
FIGURE 10. Scatter plots and time series of BRDF-derived
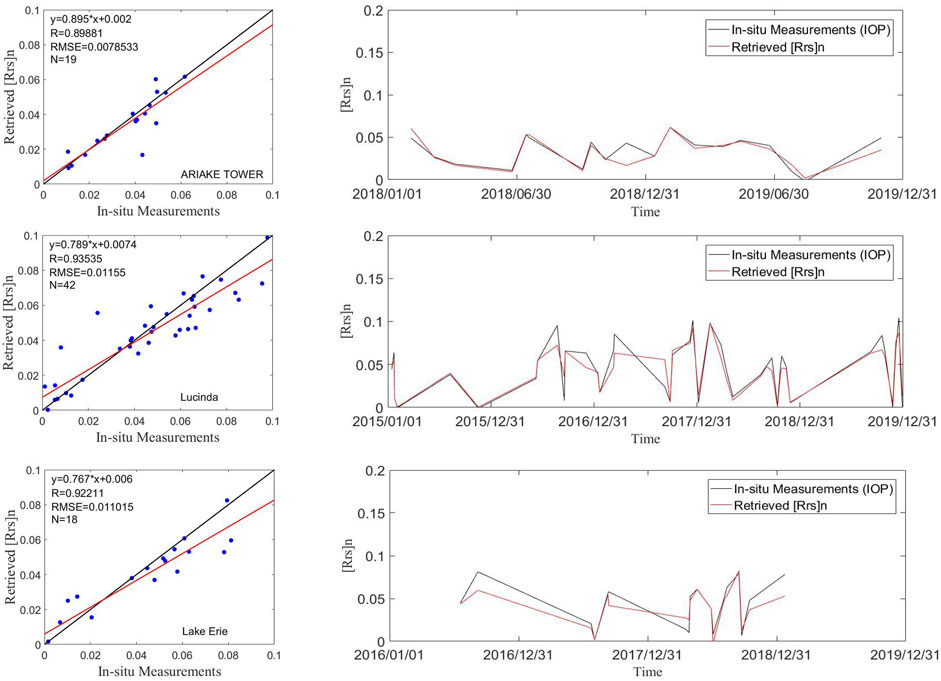
FIGURE 11. Same as in Figure 10, but for AERONET-OC measurements with IOP-based correction.

TABLE 4. Detailed statistics for the comparison of
According to Figures 10, 11, the retrieved
4 Discussion
In this study, the simultaneous underlying water surface - aerosol retrieval method for Case II waters was proven to be insensitive to the initial BRDF errors. However, errors caused by aerosol models cannot be ignored. For coastal waters, the influence of an incorrect aerosol model was limited to retrieval in low aerosol loading cases. However, the relative errors were up to 25% for AOD and 20% for
According to the analysis of AOD results, our retrieval data performed better than the MODIS and MISR products when they were compared with in situ measurements of AERONET. Positive results were obtained for turbid Case II waters, especially in coastal waters. The AOD results for all sites had more than 70% collocations within the error lines. As shown in the comparison of spatial distribution retrieval images and satellite products of MODIS and MISR, the results had an obvious advantage over the MODIS product because of the AOD retrieval algorithm over the lake (He et al., 2014). The MODIS product requires BRF data to retrieve aerosol properties. The BRF product is developed by the MODIS measurements at intervals of 8,10 or 16 days. Water is dynamic and the BRF product might be recognized as invalid. Our results also showed a larger spatial resolution than the MISR product. However, there were insufficient samples in the high aerosol loading cases. An analysis based on additional data is required in the future.
For the BRDF results, directional remote sensing reflectance had a high correlation with the ASRVN results and AERONET-OC data. However, there were differences between the two sets of AERONET-OC with Chl a and IOP-based corrections. One of the reasons is that the Chl a-based correction was determined with the assumption for Case I water conditions. It requires the absorption contribution to co-vary with the concentration of chlorophyll a (Morel and Maritorena, 2001) and ignores other optically active factors such as CDOM, SPM, and higher chlorophyll concentrations (Morel et al., 2002). Another possible reason is that the BRDF model is also based on IOPs in our retrieval. Models based on IOP are more suitable for complex and turbid waters (Han et al., 2022). Based on the analysis of the BRDF results, the simultaneous algorithm exhibits high accuracy and can be applied for quantitative remote sensing over Case II waters. However, the match-ups with all the products available are still not enough in this study. More data over various water conditions is required for the further researches, such as the spatial-temporal analysis, the pollution transport analysis, and so on.
5 Conclusion
A joint algorithm to simultaneously retrieve AOD and water surface BRDF from MISR data for coastal waters and lakes was proposed. As the multi-angle measurements take images of the target within several minutes, the simultaneous retrieval algorithm can produce AOD and bidirectional reflectance over Case II waters. Compared to traditional single-view retrieval, the algorithm can improve the accuracy by considering angular effects. In addition, the short time interval of the images reduced the uncertainties of the BRDF caused by dynamic water. The BRDF model used in this method is an IOP-centered model that expands its application to turbid coastal inland waters.
Four AERONET sites were selected to represent various types of water and the proposed algorithm was tested using the MISR data in the surrounding area. The retrieved AOD was found to be consistent with AERONET measurements and provided a larger spatial resolution than the MODIS product. The bidirectional reflectance was compared with MISR data obtained using the ASRVN AC method, and the results were consistent. Furthermore, the
The algorithm proved suitable for the retrieval of aerosol optical depth and bidirectional reflectance over Case II waters. Our study provides a theoretical basis for future research on quantitative remote sensing and the retrieval using the multi-sensor data synergy. In the future, researches of the bio-optical model and the spatial-temporal analysis should be conducted using more extensive measurements. The applications on other multi-angle and dual-angle sensors are also the subjects in need of future research.
Data Availability Statement
The original contributions presented in the study are included in the article/Supplementary Material, further inquiries can be directed to the corresponding author.
Author Contributions
ZH and TC contributed to conceptualization; ZH and SS contributed to methodology; ZH and KB contributed to software; ZH and XL contributed to investigation; ZH contributed to validation, formal analysis, data curation, writing—original draft preparation, visualization, and supervision; ZH and XG contributed to resources; ZH, TC, and SS contributed to writing—review and editing; ZH and XG contributed to project administration; XG contributed to funding acquisition.
Funding
This research was funded by The National Key Research and Development Program of China (Grant Number: 2020YFE0200700) and the Natural Science Foundation of China (Grant Number: 42005104).
Conflict of Interest
The authors declare that the research was conducted in the absence of any commercial or financial relationships that could be construed as a potential conflict of interest.
Publisher’s Note
All claims expressed in this article are solely those of the authors and do not necessarily represent those of their affiliated organizations, or those of the publisher, the editors and the reviewers. Any product that may be evaluated in this article, or claim that may be made by its manufacturer, is not guaranteed or endorsed by the publisher.
Acknowledgments
We would like to thank NASA for processing the MISR data and MODIS data, and we also thank NCEP for providing global atmospheric reanalysis products. We appreciate the PI investigators from the AERONET and AERONET-OC sites, whose data were used in this paper.
References
Ahmad, Z., Franz, B. A., McClain, C. R., Kwiatkowska, E. J., Werdell, J., Shettle, E. P., et al. (2010). New Aerosol Models for the Retrieval of Aerosol Optical Thickness and Normalized Water-Leaving Radiances from the SeaWiFS and MODIS Sensors over Coastal Regions and Open Oceans. Appl. Opt. 49, 5545. doi:10.1364/ao.49.005545
Antoine, D., and Morel, A. (1999). A Multiple Scattering Algorithm for Atmospheric Correction of Remotely Sensed Ocean Colour (MERIS Instrument): Principle and Implementation for Atmospheres Carrying Various Aerosols Including Absorbing Ones. Int. J. Remote Sens. 20, 1875–1916. doi:10.1080/014311699212533
Bailey, S. W., Franz, B. A., and Werdell, P. J. (2010). Estimation of Near-Infrared Water-Leaving Reflectance for Satellite Ocean Color Data Processing. Opt. Express 18, 7521–7527. doi:10.1364/oe.18.007521
Bauer, J. E., Cai, W.-J., Raymond, P. A., Bianchi, T. S., Hopkinson, C. S., and Regnier, P. A. G. (2013). The Changing Carbon Cycle of the Coastal Ocean. Nature 504, 61–70. doi:10.1038/nature12857
Brunekreef, B., and Holgate, S. T. (2002). Air Pollution and Health. lancet 360, 1233–1242. doi:10.1016/s0140-6736(02)11274-8
Charlson, R. J., Schwartz, S. E., Hales, J. M., Cess, R. D., Coakley, J. A., Hansen, J. E., et al. (1992). Climate Forcing by Anthropogenic Aerosols. Science 255, 423–430. doi:10.1126/science.255.5043.423
Chauvigné, A., Waquet, F., Auriol, F., Blarel, L., Delegove, C., Dubovik, O., et al. (2021). Aerosol Above-Cloud Direct Radiative Effect and Properties in the Namibian Region during the AErosol, RadiatiOn, and CLOuds in Southern Africa (AEROCLO-sA) Field Campaign–Multi-Viewing, Multi-Channel, Multi-Polarization (3MI) Airborne Simulator and Sun Photometer Measurements. Atmos. Chem. Phys. 21, 8233–8253.
Chomko, R. M., and Gordon, H. R. (1998). Atmospheric Correction of Ocean Color Imagery: Use of the Junge Power-Law Aerosol Size Distribution with Variable Refractive Index to Handle Aerosol Absorption. Appl. Opt. 37, 5560–5572. doi:10.1364/ao.37.005560
Cox, C., and Munk, W. (1954). Measurement of the Roughness of the Sea Surface from Photographs of the Sun's Glitter. J. Opt. Soc. Am. 44, 838–850. doi:10.1364/josa.44.000838
Cox, C., and Munk, W. (1956). Slopes of the Sea Surface Deduced from Photographs of Sun Glitter. California: University of California Press.
Diner, D., Martonchik, J., Borel, C., Gerstl, S., Gordon, H., Knyazikhin, Y., et al. (1999). Level 2 Surface Retrieval Algorithm Theoertical Basis. Environmental Science.
Fan, Y., Li, W., Gatebe, C. K., Jamet, C., Zibordi, G., Schroeder, T., et al. (2017). Atmospheric Correction over Coastal Waters Using Multilayer Neural Networks. Remote Sens. Environ. 199, 218–240. doi:10.1016/j.rse.2017.07.016
Fournier, G. R., and Forand, J. L. (1994). “Analytic Phase Function for Ocean Water,” in Proceedings of SPIE - The International Society for Optical Engineering 2258, Bergen, Norway, 194–201.
Fukushima, H., Higurashi, A., Mitomi, Y., Nakajima, T., Noguchi, T., Tanaka, T., et al. (1998). Correction of Atmospheric Effect on ADEOS/OCTS Ocean Color Data: Algorithm Description and Evaluation of its Performance. J. Oceanogr. 54, 417–430. doi:10.1007/bf02742444
Fukushima, H., and Toratani, M. (1997). Asian Dust Aerosol: Optical Effect on Satellite Ocean Color Signal and a Scheme of its Correction. J. Geophys. Res. 102, 17119–17130. doi:10.1029/96jd03747
Gan, T. Y., Kalinga, O. A., Ohgushi, K., and Araki, H. (2004). Retrieving Seawater Turbidity from Landsat TM Data by Regressions and an Artificial Neural Network. Int. J. Remote Sens. 25, 4593–4615. doi:10.1080/01431160410001655921
Gao, B.-C., Montes, M. J., Ahmad, Z., and Davis, C. O. (2000). Atmospheric Correction Algorithm for Hyperspectral Remote Sensing of Ocean Color from Space. Appl. Opt. 39, 887–896. doi:10.1364/ao.39.000887
Garay, M. J., Witek, M. L., Kahn, R. A., Seidel, F. C., Limbacher, J. A., Bull, M. A., et al. (2020). Introducing the 4.4 Km Spatial Resolution Multi-Angle Imaging SpectroRadiometer (MISR) Aerosol Product. Atmos. Meas. Tech. 13, 593–628. doi:10.5194/amt-13-593-2020
Gleason, A. C. R., Voss, K. J., Gordon, H. R., Twardowski, M., Sullivan, J., Trees, C., et al. (2012). Detailed Validation of the Bidirectional Effect in Various Case I and Case II Waters. Opt. Express 20, 7630–7645. doi:10.1364/oe.20.007630
Gordon, H. R. (1997). Atmospheric Correction of Ocean Color Imagery in the Earth Observing System Era. J. Geophys. Res. 102, 17081–17106. doi:10.1029/96jd02443
Gordon, H. R., Du, T., and Zhang, T. (1997). Atmospheric Correction of Ocean Color Sensors: Analysis of the Effects of Residual Instrument Polarization Sensitivity. Appl. Opt. 36, 6938–6948. doi:10.1364/ao.36.006938
Gordon, H. R. (1998). In-orbit Calibration Strategy for Ocean Color Sensors. Remote Sens. Environ. 63, 265–278. doi:10.1016/s0034-4257(97)00163-6
Gordon, H. R., and Morel, A. Y. (1983). Remote Assessment of Ocean Color for Interpretation of Satellite Visible Imagery: A Review. Phys. Earth Planet. Interiors 37, 292. doi:10.1029/ln004
Gordon, H. R., and Wang, M. (1994). Retrieval of Water-Leaving Radiance and Aerosol Optical Thickness over the Oceans with SeaWiFS: a Preliminary Algorithm. Appl. Opt. 33, 443–452. doi:10.1364/ao.33.000443
Goyens, C., Jamet, C., and Schroeder, T. (2013). Evaluation of Four Atmospheric Correction Algorithms for MODIS-Aqua Images over Contrasted Coastal Waters. Remote Sens. Environ. 131, 63–75. doi:10.1016/j.rse.2012.12.006
Han, Z., Gu, X., Zuo, X., Bi, K., and Shi, S. (2022). Semi-Empirical Models for the Bidirectional Water-Leaving Radiance: An Analysis of a Turbid Inland Lake. Front. Environ. Sci. 2022, 767. doi:10.3389/fenvs.2021.818557
Harmel, T., and Chami, M. (2013). Estimation of the Sunglint Radiance Field from Optical Satellite Imagery over Open Ocean: Multidirectional Approach and Polarization Aspects. J. Geophys. Res. Oceans 118, 76–90. doi:10.1029/2012jc008221
He, Q., Li, C., Xu, T., Li, H., and Wu, Y. (2014). Validation of MODIS Derived Aerosol Optical Depth over the Yangtze River Delta in China. Remote Sensing of Environment, 1649–1661.
Hlaing, S., Gilerson, A., Harmel, T., Tonizzo, A., Weidemann, A., Arnone, R., et al. (2012). Assessment of a Bidirectional Reflectance Distribution Correction of Above-Water and Satellite Water-Leaving Radiance in Coastal Waters. Appl. Opt. 51, 220–237. doi:10.1364/ao.51.000220
Holben, B. N., Eck, T. F., Slutsker, I., Tanré, D., Buis, J. P., Setzer, A., et al. (1998). AERONET-A Federated Instrument Network and Data Archive for Aerosol Characterization. Remote Sens. Environ. 66, 1–16. doi:10.1016/s0034-4257(98)00031-5
Holben, B. N., Tanré, D., Smirnov, A., Eck, T. F., Slutsker, I., Abuhassan, N., et al. (2001). An Emerging Ground-Based Aerosol Climatology: Aerosol Optical Depth from AERONET. J. Geophys. Res. 106, 12067–12097. doi:10.1029/2001jd900014
IOCCG, (2006). Remote Sensing of Inherent Optical Properties: Fundamentals, Tests of Algorithms, and Applications. Dartmouth, NS: Reports of the International Ocean-Colour Coordinating Group.
IOCCG, (2000). Remote Sensing of Ocean Colour in Coastal, and Other Optically-Complex, Waters. Dartmouth, NS: Reports of the International Ocean-Colour Coordinating Group.
Jamet, C., Loisel, H., Kuchinke, C. P., Ruddick, K., Zibordi, G., and Feng, H. (2011). Comparison of Three SeaWiFS Atmospheric Correction Algorithms for Turbid Waters Using AERONET-OC Measurements. Remote Sens. Environ. 115, 1955–1965. doi:10.1016/j.rse.2011.03.018
Knobelspiesse, K., Ibrahim, A., Franz, B., Bailey, S., and Kalashnikova, O. (2020). Analysis of Simultaneous Aerosol and Ocean Glint Retrieval Using Multi-Angle Observations. Atmos. Meas. Tech. 14 (5), 3233–3252.
Lavender, S. J., Pinkerton, M. H., Moore, G. F., Aiken, J., and Blondeau-Patissier, D. (2005). Modification to the Atmospheric Correction of SeaWiFS Ocean Colour Images over Turbid Waters. Cont. Shelf Res. 25, 539–555. doi:10.1016/j.csr.2004.10.007
Lee, K. H., and Kim, Y. J. (2010). Satellite Remote Sensing of Asian Aerosols: a Case Study of Clean, Polluted, and Asian Dust Storm Days. Atmos. Meas. Tech. 3, 1771–1784. doi:10.5194/amt-3-1771-2010
Lee, Z., Carder, K. L., and Du, K. (2004). Effects of Molecular and Particle Scatterings on the Model Parameter for Remote-Sensing Reflectance. Appl. Opt. 43, 4957–4964. doi:10.1364/ao.43.004957
Lee, Z. P., Du, K., Voss, K. J., Zibordi, G., Lubac, B., Arnone, R., et al. (2011). An Inherent-Optical-Property-Centered Approach to Correct the Angular Effects in Water-Leaving Radiance. Appl. Opt. 50, 3155–3167. doi:10.1364/ao.50.003155
Lewis, E. R., Lewis, R., Lewis, E. R., and Schwartz, S. E. (2004). Sea Salt Aerosol Production: Mechanisms, Methods, Measurements, and Models. American Geophysical Union.
Li, F., Jupp, D. L. B., Schroeder, T., Sagar, S., Sixsmith, J., and Dorji, P. (2021). Assessing an Atmospheric Correction Algorithm for Time Series of Satellite-Based Water-Leaving Reflectance Using Match-Up Sites in Australian Coastal Waters. Remote Sens. 13, 1927. doi:10.3390/rs13101927
Limbacher, J. A., and Kahn, R. A. (2014). MISR Research-Aerosol-Algorithm Refinements for Dark Water Retrievals. Atmos. Meas. Tech. 7.
Limbacher, J. A., and Kahn, R. A. (2017). Updated MISR Dark Water Research Aerosol Retrieval Algorithm - Part 1: Coupled 1.1 Km Ocean Surface Chlorophyll a Retrievals with Empirical Calibration Corrections. Atmos. Meas. Tech. 10, 1–29. doi:10.5194/amt-10-1539-2017
Limbacher, J. A., and Kahn, R. A. (2018). Updated MISR Over-water Research Aerosol Retrieval Algorithm Part 2: A Multi- Angle Aerosol Retrieval Algorithm for Shallow, Turbid, Oligotrophic, and Eutrophic Waters. Atmos. Meas. Tech. 12, 675–689.
Loisel, H., and Morel, A. (2001). Non-isotropy of the Upward Radiance Field in Typical Coastal (Case 2) Waters. Int. J. Remote Sens. 22, 275–295. doi:10.1080/014311601449934
Lyapustin, A., and Wang, Y. (2007). MAIAC-multi-angle Implementation of Atmospheric Correction for MODIS. AGU Spring Meeting Abstracts, A51B–A05.
Martonchik, J. V., Diner, D. J., Kahn, R. A., Ackerman, T. P., Verstraete, M. M., Pinty, B., et al. (1998). Techniques for the Retrieval of Aerosol Properties over Land and Ocean Using Multiangle Imaging. IEEE Trans. Geosci. Remote Sens. 36, 1212–1227. doi:10.1109/36.701027
Mobley, C. D., and Sundman, L. K. (2003). Effects of Optically Shallow Bottoms on Upwelling Radiances: Inhomogeneous and Sloping Bottoms. Limnology and Oceanography 48 (2003), 329–336.
Mobley, C. D., Sundman, L. K., and Boss, E. (2002). Phase Function Effects on Oceanic Light Fields. Appl. Opt. 41, 1035–1050. doi:10.1364/ao.41.001035
Mobley, C. (1994). Light and Water: Radiative Transfer in Natural Waters. San Diego: Academic Press.
Morel, A., Antoine, D., and Gentili, B. (2002). Bidirectional Reflectance of Oceanic Waters: Accounting for Raman Emission and Varying Particle Scattering Phase Function. Appl. Opt. 41, 6289–6306. doi:10.1364/ao.41.006289
Morel, A., and Gentili, B. (1996). Diffuse Reflectance of Oceanic Waters III Implication of Bidirectionality for the Remote-Sensing Problem. Appl. Opt. 35, 4850. doi:10.1364/ao.35.004850
Morel, A., and Maritorena, S. (2001). Bio-optical Properties of Oceanic Waters: A Reappraisal. J. Geophys. Res. 106, 7163–7180. doi:10.1029/2000jc000319
Morel, A., and Prieur, L. (1977). Analysis of Variations in Ocean Color1. Limnol. Oceanogr. 22, 709–722. doi:10.4319/lo.1977.22.4.0709
Morel, A., Voss, K. J., and Gentili, B. (1995). Bidirectional Reflectance of Oceanic Waters: a Comparison of Modeled and Measured Upward Radiance Fields. J. Geophys. Res. 100, 13143–13150. doi:10.1029/95jc00531
Mukherjee, M., Ray, A., Post, A. F., Mckay, R. M., and Bullerjahn, G. S. (2016). Identification, Enumeration and Diversity of Nitrifying Planktonic Archaea and Bacteria in Trophic End Members of the Laurentian Great Lakes. J. Gt. Lakes. Res. 42, 39–49. doi:10.1016/j.jglr.2015.11.007
Omar, A. H., Won, J. G., Winker, D. M., Yoon, S. C., Dubovik, O., and Mccormick, M. P. (2005). Development of Global Aerosol Models Using Cluster Analysis of Aerosol Robotic Network (AERONET) Measurements. J. Geophys. Res. Atmos. 110.
Park, Y.-J., and Ruddick, K. (2005). Model of Remote-Sensing Reflectance Including Bidirectional Effects for Case 1 and Case 2 Waters. Appl. Opt. 44, 1236–1249. doi:10.1364/ao.44.001236
Pepijn Veefkind, J., and de Leeuw, G. (1998). A New Algorithm to Determine the Spectral Aerosol Optical Depth from Satellite Radiometer Measurements. J. Aerosol Sci. 29, 1237–1248. doi:10.1016/s0021-8502(98)00032-9
Qin, Y., Mitchell, R., and Forgan, B. W. (2015). Characterizing the Aerosol and Surface Reflectance over Australia Using AATSR. IEEE Trans. Geosci. Remote Sens. 53, 6163–6182. doi:10.1109/tgrs.2015.2433911
Remer, L. A., Davis, A. B., Mattoo, S., Levy, R. C., Kalashnikova, O. V., Coddington, O., et al. (2019). Retrieving Aerosol Characteristics from the PACE Mission, Part 1: Ocean Color Instrument. Front. Earth Sci. 7, 152. doi:10.3389/feart.2019.00152
Remer, L. A., Kaufman, Y. J., Tanré, D., Mattoo, S., Chu, D. A., Martins, J. V., et al. (2005). The MODIS Aerosol Algorithm, Products, and Validation. J. Atmos. Sci. 62, 947–973. doi:10.1175/jas3385.1
Remer, L. A., Knobelspiesse, K., Zhai, P.-W., Xu, F., Kalashnikova, O. V., Chowdhary, J., et al. (2019). Retrieving Aerosol Characteristics from the PACE Mission, Part 2: Multi-Angle and Polarimetry. Front. Environ. Sci. 7, 94. doi:10.3389/fenvs.2019.00094
Sayer, A. M., Thomas, G. E., and Grainger, R. G. (2010). A Sea Surface Reflectance Model for (A)ATSR, and Application to Aerosol Retrievals. Atmos. Meas. Tech. 3, 813–838. doi:10.5194/amt-3-813-2010
Schaaf, C. B., Gao, F., Strahler, A. H., Lucht, W., Li, X., Tsang, T., et al. (2002). First Operational BRDF, Albedo Nadir Reflectance Products from MODIS. Remote Sens. Environ. 83, 135–148. doi:10.1016/s0034-4257(02)00091-3
Shi, C., and Nakajima, T. (2018). Simultaneous Determination of Aerosol Optical Thickness and Water-Leaving Radiance from Multispectral Measurements in Coastal Waters. Atmos. Chem. Phys. 18, 3865–3884. doi:10.5194/acp-18-3865-2018
Shi, K., Zhang, Y., Zhu, G., Qin, B., and Pan, D. (2018). Deteriorating Water Clarity in Shallow Waters: Evidence from Long Term MODIS and In-Situ Observations. Int. J. Appl. Earth Observation Geoinformation 2018, S0303243417303355. doi:10.1016/j.jag.2017.12.015
Shi, S., Cheng, T., Gu, X., Chen, H., Guo, H., Wang, Y., et al. (2017). Synergy of MODIS and AATSR for Better Retrieval of Aerosol Optical Depth and Land Surface Directional Reflectance. Remote Sens. Environ. 195, 130–141. doi:10.1016/j.rse.2017.04.010
Smirnov, A., Holben, B., Slutsker, I., Giles, D., McClain, C., Eck, T., et al. (2009). Maritime Aerosol Network as a Component of Aerosol Robotic Network. J. Geophys. Res. Atmos. 114. doi:10.1029/2008jd011257
Suzuki, K., and Takemura, T. (2019). Perturbations to Global Energy Budget Due to Absorbing and Scattering Aerosols. J. Geophys. Res. Atmos. 124, 2194–2209. doi:10.1029/2018jd029808
Tanre, D., Herman, M., and Deschamps, P. Y. (1983). Influence of the Atmosphere on Space Measurements of Directional Properties. Appl. Opt. 22, 733–741. doi:10.1364/ao.22.000733
Tanré, D., Kaufman, Y., Herman, M., and Mattoo, S. (1997). Remote Sensing of Aerosol Properties over Oceans Using the MODIS/EOS Spectral Radiances. J. Geophys. Res. Atmos. 102, 16971–16988.
Vanderwoerd, H., and Pasterkamp, R. (2008). HYDROPT: A Fast and Flexible Method to Retrieve Chlorophyll-A from Multispectral Satellite Observations of Optically Complex Coastal Waters. Remote Sens. Environ. 112, 1795–1807. doi:10.1016/j.rse.2007.09.001
Vermote, E. F., Tanre, D., Deuze, J. L., Herman, M., and Morcette, J.-J. (1997). Second Simulation of the Satellite Signal in the Solar Spectrum, 6S: An Overview. IEEE Trans. Geosci. Remote Sens. 35, 675–686. doi:10.1109/36.581987
Vermote, E., Tanré, D., Deuzé, J., Herman, M., Morcrette, J., and Kotchenova, S. (2006). Second Simulation of a Satellite Signal in the Solar Spectrum-Vector (6SV). 6S User Guide Version 3, 1–55.
Wang, M. (2010). “Atmospheric Correction for Remotely-Sensed Ocean-Colour Products,” in Reports and Monographs of the International Ocean-Colour Coordinating Group (Dartmouth, NS: IOCCG).
Wang, M., and Franz, B. A. (2000). Comparing the Ocean Color Measurements between MOS and SeaWiFS: A Vicarious Intercalibration Approach for MOS. IEEE Trans. Geosci. Remote Sens. 38, 184–197. doi:10.1109/36.823911
Wang, S., Li, J., Zhang, B., Spyrakos, E., Tyler, A. N., Shen, Q., et al. (2018). Trophic State Assessment of Global Inland Waters Using a MODIS-Derived Forel-Ule Index. Remote Sens. Environ. 217, 444–460. doi:10.1016/j.rse.2018.08.026
Yang, H., and Gordon, H. R. (1997). Remote Sensing of Ocean Color: Assessment of Water-Leaving Radiance Bidirectional Effects on Atmospheric Diffuse Transmittance. Appl. Opt. 36, 7887–7897. doi:10.1364/ao.36.007887
Yujie Wang, Y., Lyapustin, A. I., Privette, J. L., Morisette, J. T., and Holben, B. (2009). Atmospheric Correction at AERONET Locations: A New Science and Validation Data Set. IEEE Trans. Geosci. Remote Sens. 47, 2450–2466. doi:10.1109/tgrs.2009.2016334
Zibordi, G., Holben, B. N., Talone, M., D'Alimonte, D., and Sorokin, M. G. (2020). Advances in the Ocean Color Component of the Aerosol Robotic Network (AERONET-OC). J. Atmos. Ocean. Technol. 38, 4. doi:10.1175/JTECH-D-20-0085.1
Keywords: aerosol optical depth, bidirectional reflectance, case II waters, simultaneous retrieval, multi-angle measurement
Citation: Han Z, Cheng T, Gu X, Shi S, Li X and Bi K (2022) A Multi-Angle Method for Simultaneous Retrieval of Aerosol Optical Depth and Bidirectional Reflectance Over Case II Waters. Front. Environ. Sci. 10:900694. doi: 10.3389/fenvs.2022.900694
Received: 21 March 2022; Accepted: 29 April 2022;
Published: 24 May 2022.
Edited by:
Dmitry Efremenko, German Aerospace Center (DLR), GermanyReviewed by:
Cédric Jamet, UMR8187 Laboratoire d’Océanologie et de Géosciences (LOG), FranceHui Xu, University of Maryland, United States
Copyright © 2022 Han, Cheng, Gu, Shi, Li and Bi. This is an open-access article distributed under the terms of the Creative Commons Attribution License (CC BY). The use, distribution or reproduction in other forums is permitted, provided the original author(s) and the copyright owner(s) are credited and that the original publication in this journal is cited, in accordance with accepted academic practice. No use, distribution or reproduction is permitted which does not comply with these terms.
*Correspondence: Tianhai Cheng, Y2hlbmd0aEByYWRpLmFjLmNu