- Laboratório de Ficologia, Departamento de Botânica, Museu Nacional, Universidade Federal do Rio de Janeiro, Rio de Janeiro, Brazil
Understanding the influence of environmental conditions on biodiversity is a major task in ecology. We investigated how phytoplankton taxonomic (TD) and functional (FD) diversities vary with environmental factors in eight subtropical and tropical reservoirs. We hypothesized that i) environmental variables affect phytoplankton TD and FD; ii) FD provides better relationships to environmental changes than TD, and; iii) indices based on biomass are better related to the environment than those based on identities. The relationships between phytoplankton diversities and environmental drivers were assessed through generalized linear models. Our hypotheses were partly confirmed. TD and FD were, in fact, dependent on the environment, with higher values occurring in warmer, clearer, and more enriched systems, under lower zooplankton grazing pressure; but FD based on identities was not predicted better from environmental conditions than TD based on identities. As expected, indices based on biomass are better related to the environment than their counterpart based on identities.
Introduction
Except for the neutral theory (Hubbel, 2001), the ability to predict patterns of species richness is based on the assumption that different species will respond differently to environmental gradients (Tilman, 2004; Cadotte et al., 2011). Species richness has been used as a primary measure of biodiversity to study its relationships to ecosystem functioning (Díaz & Cabido, 2001; Magurran, 2005; Vogt et al., 2010; Magurran and Vianna, 2011). However, this measure provides incomplete information about the function of individual species in each community (Petchey, 2004; Villéger et al., 2010), and so does little to explain why biodiversity might be necessary for ecosystem functioning (Díaz & Cabido, 2001). To have rapid and consistent responses across ecosystem gradients (Mouillot et al., 2013) and to increase the explanatory power (Petchey et al., 2004), an alternative approach - functional diversity (FD) - has been proposed to assess the functional structure of communities. FD diversity is generally defined as the extent of trait differences between species (Petchey & Gaston, 2002; De Bello et al., 2016). Functional traits are morphological, physiological, and behavioral features, measured at the individual level, which influence the performance of an individual to the environment and other species (Díaz and Cabido, 2001; Weithoff, 2003; Violle et al., 2007). The use of abundance (or biomass), an essential aspect of ecosystem functioning—the niche size (De Bello et al., 2007; Magurran and Vianna, 2011)—can increase the prediction of biodiversity and environmental conditions (De Bello et al., 2006; Vogt et al., 2010).
Some studies have examined phytoplankton patterns and mechanisms underlying FD compare to taxonomical diversity (TD), although differences in functionality are easily distinguishable from morphological traits (Weithoff, 2003; Kruk et al., 2010; Wang et al., 2020). The majority of research on phytoplankton FD is based on identities (presence-absence, FDPA, e.g., Bergkemper et al., 2018; Santos et al., 2015; Cardoso et al., 2017; Ye et al., 2019) and, in minor extension, on abundance or biovolume, FDBV (Santos et al., 2015; Graco-Roza et al., 2020; Wang et al., 2020). FDPA and FDBV have been indicated as driven by similar environmental variables, such as water temperature, productivity, light, flushing, grazing, or macrophytes, that can mediate changes in phytoplankton assemblages. Although, the discussion about how these mechanisms influence phytoplankton diversity still requires more attention.
Regarding functional diversity based on identities, FDPA may be negatively affected by water temperature, as shown in observational studies for shallow lakes in South America, using the richness of functional groups (Kruk et al., 2017) and Amazonian floodplain lakes during high water using traits (Cardoso et al., 2017). Water temperature negatively drove FDPA also in experimental studies (e.eg. Bergkemper et al., 2018). However, positive temperature influences FDPA, as in some temperate lakes (Palffy et al., 2013). Besides, high FDPA is supported by low productivity (Vogt et al., 2010) and weak stratification (Longhi & Beisner, 2010) in Canadian shallow lakes. High FDPA was related to high light intensity and zooplankton biomass (low water level) and to low productivity (high water level) in floodplain lakes (Cardoso et al., 2017). If the FDBV is considered, a positive correlation with light is provided in an eutrophic lake (Wang et al., 2020). The functional phytoplankton composition based on biovolume changed more than 200 km downstream in a tropical river, suggesting that damming can filter species by their traits Graco-Roza et al. (2020). An experimental study (Barrow et al., 2019) showed the influence of the macrophyte abundance in shaping phytoplankton functional diversity, based on density/biovolume; it indicated that competition for light, grazing, and probably, allelochemicals were mechanisms through which macrophytes mediated changes in the phytoplankton community. A recent experimental study accessed the relative effects of spatial overlap, grazing, and the physical environment on multiple dimensions of phytoplankton diversity (functional and taxonomical). Those authors revealed the physical structure, and top-down cascading interactions as the potential strongest drivers of phytoplankton diversity, probably because the forced coexistence allowed niche differentiation along trait axes to alleviate interspecific competition and might have avoided this effect on taxonomic diversity (Le Noac’h et al., 2021).
Regarding taxonomic diversity, phytoplankton diversity has traditionally been assessed through identities (species richness, DPA). Their main ecological drivers are temperature (positive relationship; Weyhenmeyer et al., 2013; Kruk et al., 2017; Cardoso et al., 2017), system area (positive relationship; Smith et al., 2005), and productivity (unimodal relationship; Dodson et al., 2000; Smith, 2007; Santos et al., 2015). Light availability is an essential control of TDPA (positive relationship; Weyhenmeyer et al., 2013; Stomp et al., 2011; Wang et al., 2020) by favoring growth and increasing the number of available niches due to stratification. Although less frequently assessed, relationships of TDPA to hydraulic flow (negative relationship, Borges & Train, 2009; Nabout et al., 2007; Huszar et al., 1998; or positive, Train & Rodrigues, 1998) and to grazing pressure (negative relationship, Muylaert et al., 2010; Kruk et al., 2017) have also been noted as important factors for controlling phytoplankton TDPA. The patterns of TDBV based on biovolume are similar to TDPA (Huszar et al., 1998; Borges & Train, 2009). Both diversity dimensions increase in warmer systems and decrease in strongly selective environments, e.g., highly flushed systems or lakes with extreme acidity, alkalinity, turbidity, oligotrophy, or physical constancy (save for spatial niche differentiation) (Reynolds et al., 1993). Additional studies that combine both diversities and their relationships to environmental conditions are needed to provide deeper insights into phytoplankton diversity mechanisms (Vogt et al., 2010; Pálffy et al., 2013; Dunck et al., 2015; Weithoff et al., 2015).
Using data from eight large subtropical and tropical reservoirs, we investigated how local phytoplankton diversity (taxonomic and functional) varies with productivity, water temperature, hydraulic flow, turbidity, and grazing pressure. We hypothesized that: i) environmental variables (water temperature, productivity, etc.) affect the taxonomic and functional diversity of phytoplankton; ii) functional diversity provides better species-environment relationships than taxonomic diversity since they are assumed to be mediated by functional traits; and iii) within each diversity dimension (taxonomic and functional), indices based on biomass are better related to the environment than their counterpart based on identities.
Materials and Methods
Study Area and Sampling
The study was conducted in eight hydroelectric reservoirs that differ in morphometry, hydrology, and trophic state (Balbina, Tucuruí, Xingó, Serra da Mesa, Três Marias, Funil, Salto Segredo, and Itaipu), located in different Brazilian biomes (Figure 1; Table 1). Most of them are stratified, at least during the warmer months (Esteves et al., 1985; De Fillipo et al., 1999; Tundisi et al., 2005; Borges et al., 2008; Soares et al., 2009; Kemenes et al., 2011). As found in many reservoirs (Tundisi & Matsumura-Tundisi, 2008), spatial heterogeneity in limnological conditions and plankton communities occurs in these reservoirs (Soares et al., 2012; Brasil-MME, 2014).
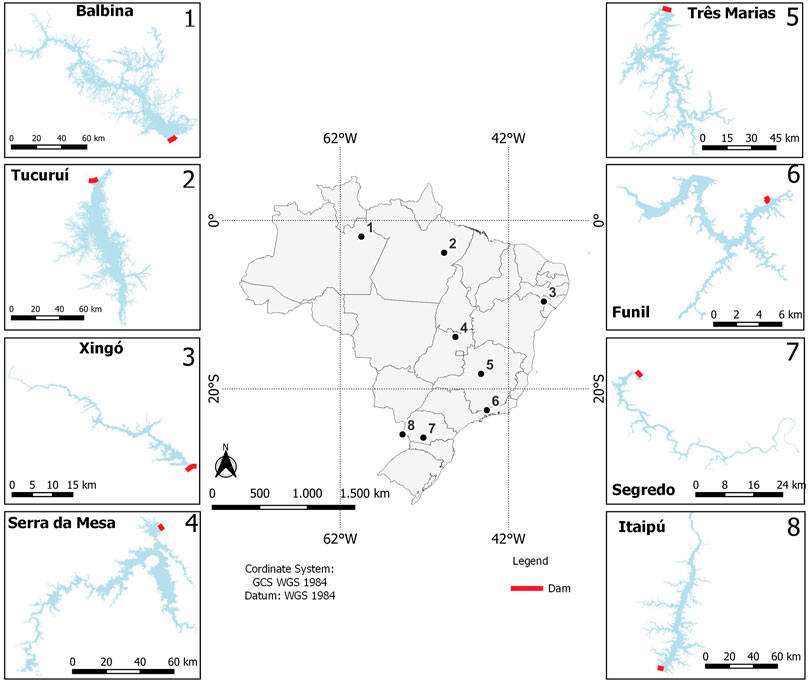
FIGURE 1. Map of Brazil showing the locations of eight hydroelectric reservoirs indicated with numbers. Water limits from Brasil (2019).
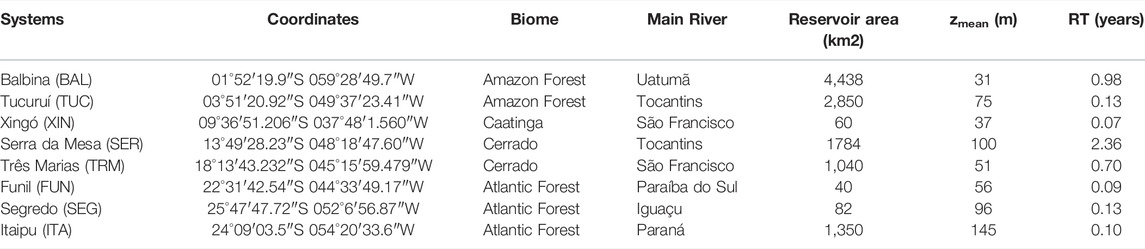
TABLE 1. Location and main features of the eight hydroelectric reservoirs (zmean = mean depth; RT = water residence time). Caatinga is a type of shrub vegetation, and Cerrado is a type of savanna.
Each reservoir was sampled quarterly over a year, between 2011 and 2012. Water samples for nutrient, chlorophyll-a, and phytoplankton analyses (n = 255) were taken using a sampling tube (1.5 m long) at the water surface of the sampling stations (8–12 per reservoir, in the pelagic zone) (Supplementary Table S1). Phytoplankton samples were fixed with Lugol’s solution. Zooplankton samples were collected with a plankton net (mesh = 50 μm, Ø = 30 cm) by vertical hauls, mainly in the mixing zone (1.2–18.0 m), and were immediately fixed with 4% formalin. For analyses of total phosphorus (TP) and total nitrogen (TN) concentrations, unfiltered samples were used. For dissolved-nutrient concentrations (ammonium, N-NH4+; nitrite, N-NO2– and nitrate, N-NO3–), samples were filtered through Whatman GF/C filters and immediately frozen. Water transparency was measured with a Secchi disk; water temperature, pH, and electrical conductivity with a YSI 6000 multiparameter probe; and turbidity with a La-Motte 2020 turbidimeter. Seasonal measurements for water-residence time were obtained from the Agência Nacional de Energia Elétrica (ANEEL), with daily values, and averaged for each sampling month.
Sample Analyses
N-NO3– and N-NO2− were analyzed through cadmium reduction of the samples and N-NH4+ through the colorimetric phenol-hypochlorite method (Wetzel & Likens, 2000). Dissolved inorganic nitrogen (DIN) was defined as the sum of N-NO3−, N-NH4+, and N-NO2−. TN was analyzed by the colorimetric method, using sulfuric acid (Crumpton et al., 1992); SRSi by the molybdate method (Wetzel & Likens, 2000); TP by sodium persulfate digestion (Mackereth et al., 1978); and SRP by the ascorbic-acid method (Wetzel & Likens, 2000). Chlorophyll-a was extracted in cold ethanol and read in a fluorometer (APHA, 2005). Phytoplankton population density (individuals mL−1) was enumerated in random fields (Uhelinger, 1964), using the sedimentation technique (Utermöhl, 1958) under a Zeiss Axiovert 10 inverted microscope (Germany), at 400 ×. As far as possible, the units (cells, colonies, and filaments) were enumerated to at least 100 specimens of the most frequent species (Lund et al., 1958). Specific phytoplankton biomass was evaluated as biovolume (mm3 L−1), according to Hillebrand et al. (1999), based on geometric equations. Individual phytoplankton biovolume (V, µm3) and surface area (S, µm2) were estimated according to geometric equations (Hillebrand et al., 1999). When possible, calculations were based on measurements of at least 25–30 organisms. Phytoplankton individuals were identified, when possible, to the lowest taxonomic unit. The presence of aerotopes, flagella, mucilage, heterocytes, siliceous and non-siliceous walls, and life-forms were noted for each organism. Zooplankton population density (individuals L−1) was estimated by enumerating organisms in a Sedgwick-Rafter counting chamber. Aliquots for counting were removed from a well-mixed sample using a Hensen-Stempel pipette. At least 250 individuals were counted in each of three sequential subsamples, and 100 individuals of the most abundant species, with a coefficient of variation <10%. When this minimum was not obtained, the sample was counted entirely.
Rotifer biomass was estimated from the mean weight of each species as given in the literature (Wetzel & Likens, 1991), multiplied by its density in each sample (Wetzel & Likens, 1991), and the biomass of nauplii was estimated according to allometric formulas (Culver et al., 1985). Biomass of microcrustaceans (cladocerans and copepods) was measured as dry weight (µg) of the first 20 individuals taken from the samples, in a Mettler UMT2 analytical microbalance, after drying in an oven at 60°C for 24 h. The ratio of zooplankton biomass (dry weight) to algal biomass (dry weight, as chlorophyll-a multiplied by 66) was used as a proxy for grazing pressure (GP) (Jeppesen et al., 1997, modified by using medium-sized filter-feeders, i.e., cladocerans + calanoid copepods), assuming inefficient predation by rotifers on phytoplankton (Muylaert et al., 2010). This ratio gives a rough indication of the percentage of phytoplankton standing stock grazed away per day (assuming that the zooplankton consumes an amount equal to its biomass per day) and that phytoplankton is the only food source for the zooplankton (Jeppesen et al., 1994).
Phytoplankton Diversity
Phytoplankton diversity in each sample (8–12 per reservoir) was assessed through: i) taxonomic diversity, as presence and absence of species (species richness, TDPA) and the Gini-Simpson index in biovolume (TDBV); and ii) functional diversity, calculated as Rao’s quadratic entropy index based on presence/absence (FDPA) and relative biovolume (FDBV) (Rao, 1982; Botta-Dukat, 2005; Ricotta & Moretti, 2011).
Traits for phytoplankton FD were: a) continuous traits i) individual size (maximum linear dimension, MLD; volume, V; surface, S; and the ratio of surface area:volume, S/V); and b) binary traits ii) capacity for nitrogen fixation, through the presence of heterocytes; iii) silica demand; iv) life forms, i.e., unicells, chain-filaments, and colonies; v) cell motility, i.e., by aerotopes and flagella; and (vi) pigment composition, i.e. blue, green, brown or mixed (Longhi & Beisner, 2010). For mucilaginous colonial organisms, V (volume) and S (surface area) were calculated for the whole colony, including mucilage. These traits are related to the main processes of phytoplankton growth and reproduction: resource acquisition, i.e., nutrients and light (traits i–iv), predator avoidance (traits iv and v), and reproduction (trait i) (Litchman & Klausmeier, 2008). Besides, they can be easily measured or obtained from the literature (Weithoff, 2003). The continuous traits (MDL, V, S, and S/V) were measured for individuals, and therefore we calculated the mean for each species. We opted to use all possible phytoplankton traits available in the literature for this purpose.
Rao’s index can be understood as the mean of all dissimilarities in the dissimilarity matrix. It expresses the mean difference between two randomly selected individuals, with replacement, including the diagonal, which represents the dissimilarity between one species and itself (generally assumed to be zero in most existing approaches) (Botta-Dukát, 2005). This mean value can be weighted or not by species relative biovolume. We used the presence and absence (identities) and the relative species biovolume here. TDBV, FDPA and FDBV range from zero to 1, but TDPA does not. To run the models, TDPA values were standardized for a range from 0 to 1 in the {vegan} package, using range methods in the decostand function of R software (R Development Core Team 2011).
We also estimated the functional redundancy (FR), i.e., the fraction of species diversity not expressed by functional diversity, following the procedures described by Pillar et al. (2013) and De Bello et al. (2007). FR was calculated as the difference between TDBV (Gini-Simpson index) and FDBV (Rao index; Pillar et al., 2013; De Bello et al., 2007). If FR = 0, the species have completely different traits; if FR = 1, all the species have identical traits. FRs close to 1 should be expected in redundant assemblages (Pillar et al., 2013).
TDBV, FDPA, FDBV, and FR were calculated with the melodic function proposed by De Bello et al. (2016) in the {picante} package of R software (R Development Core Team 2011).
Data Analyses
We used environmental variables related to temperature, hydraulic flow, turbidity, productivity, and grazing pressure gradients to investigate the relationships between phytoplankton diversity and environmental gradients. Water temperature, WT; seasonal water-residence time, RT; turbidity, TU; total nitrogen, TN and total phosphorus, TP; and grazing pressure of medium-sized filter-feeders, GP were compiled from the measured dataset initially 21 environmental variables (Supplementary Table S1). Three selection criteria were used: i) VIF (variation inflation factors)—only variables with VIF values < 20 were kept (Ter Braak, 1986); ii) PCA (principal components analysis) to choose the strength of correlation among variables; and iii) known or presumed importance of variables for phytoplankton in reservoirs, based on previous knowledge. Six variables were selected: RT, WT, TU, TN, TP, and GP. To summarize the phytoplankton habitat, we performed another principal components analysis (PCA) with the six selected variables, using canonical ordination software CANOCO 5. Data were previously log 10-transformed. Axis retention was evaluated under the Broken-Stick criterion (Jackson, 1993).
To identify the primary relationships between environmental gradients and phytoplankton traits mediated by phytoplankton species biovolume, we conducted a co-inertia analysis that couples multiple matrices and identifies co-relationships between them (RLQ, Dolédec, et al., 1996), and fourth-corner analyses (Legendre et al., 1997). Both methods are based on the three separate matrices: R matrix, environmental characteristics (6 columns) of sampling locations (255 lines); L matrix, species biovolume (469 columns) distribution across sampling locations (255 lines); and Q matrix, continuous and categorical traits (9 columns) of species (469 lines). L matrix was logx+1 transformed to prevent zero values. Q and R matrices were standardized (mean 0, standard deviation 1) to allow comparisons of variables measured at different scales (Melo & Hepp, 2008).
The analysis was conducted in three steps. In the first step, the matrices were submitted to ordination analyses (Vallet et al., 2010): i) correspondence analysis (CA) with the L matrix; ii) principal components analysis (PCA) with the R matrix, and scores obtained from the CA, and iii) principal coordinates analysis (PCoA) with the Q matrix using the Euclidean Distance. In the second step, the RLQ was performed based on the scores obtained from the ordination analysis in the first step, which allowed us to obtain new site and species scores with the maximum covariance (Dolédec et al., 1996), followed by a Monte Carlo permutation test (9,999 iterations with a sequential approach; Ter Braak et al., 2012). In the third step, the fourth-corner analysis was performed; this analysis tests the relationship between one trait and one environmental variable at a time, allowing the evaluation of individual trait-environmental relationships (Legendre et al., 1997). This method has been used to test the significance of bivariate associations. We used 9,999 permutations in all randomization procedures, and the false discovery rate method (FDR; Benjamini & Hochberg, 1995) to adjust p values for multiple testing.
RLQ and fourth-corner are complementary methods, and their combined use may strongly improve the study of trait-environment associations (Dray et al., 2014). For that, we used the package ADE-4 (Dray & Dufour, 2007) in the R software (R Development Core Team, 2014).
To elucidate how phytoplankton diversity (taxonomic and functional) is affected by changes in the environmental conditions, we generated generalized linear models (GLM). To remove the spatial dependence of samples, we performed multiple regressions among the response variables (TDPA, TDBV; FDPA, FDBV) and spatial variables (latitude and longitude), and we used the residuals generated from these regressions as our response variables. For each response variable, the best-approximation GLM was selected based on the Akaike Information Criterion, corrected by the sample size and the number of parameters in the model (AICc; Burnham & Anderson, 2002). All the six previously selected environmental variables were initially included in a full model, and a stepwise selection was used to find the optimal models by AICc. The variance explained by the model was defined by deviance (D2), an analog to the R2 determination coefficient. We used R software version 3.0.1 (R Development Core Team, 2013) to perform GLM and AICc, using the {MASS} and {AICcmodavg} packages.
Results
Response Variables
A total of 469 phytoplankton species (39% chlorophyceans, 26% cyanobacteria) were recorded in the eight systems during the study period. The remaining species were chrysophyceans (11%), cryptomonads (7%), diatoms (7%), desmids (6%), dinoflagellates, xanthophyceans, and euglenoids (2% each). Total biovolume values ranged from 0.02 to 28.80 mm3 L−1 with high TP, TN, TU, and low GP.
Across all reservoirs, TDPA ranged from 5 to 85 species/sample, with higher median values in the reservoirs located in the Amazon region (TUC and BAL, 44 and 43 species/sample, respectively) than in the southern region (ITA and SEG, 10 and 12 species/sample, respectively). TDPA was standardized (0–1) and ranged from 0.06 to 0.93. TDBV ranged from 0.15 to 0.94, with higher median values in the Amazonian TUC and BAL (0.89 and 0.86, respectively) than in the others (0.42–0.79). FDPA ranged from 0.49 to 0.83, with higher median FUN, XIN, and TUC (0.77–0.79) than in the remaining reservoirs (0.59–0.68). FDBV ranged from 0.04 to 0.77, with higher median values in TUC (0.68) and SER (0.67) than in the other systems (0.33–0.61). Therefore, most reservoirs showed intermediate to high median values of diversity, except TDPA (standardized), which ranged from low to high (Figure 2).
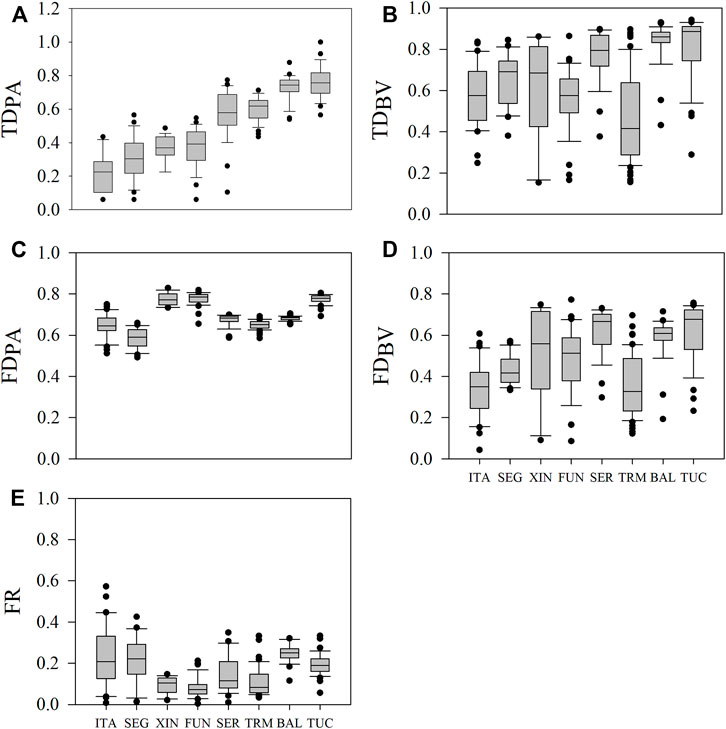
FIGURE 2. Variations in phytoplankton diversity indices and functional redundancy in the reservoirs studied. In each reservoir, the variation for a given variable is caused by seasonal and spatial variation. The horizontal line inside the boxes indicates the median, and the boundaries indicate the 25th and 75th percentiles. Whiskers indicate the 90th and 10th percentiles, and dots indicate outliers. (A) TDPA = taxonomic diversity based on presence and absence (species richness); (B) TDBV = taxonomic diversity based on biovolume (Gini-Simpson Index); (C) FDPA = functional diversity based on presence and absence (Rao index); (D) FDBV = functional diversity based on biovolume (Rao Index); (E) FR = functional redundancy (TDBV—FDBV). Reservoirs were ordered according to the increase in TDPA. Abbreviations of reservoir names as in Table 1.
Median values of FR were low in TMA, FUN, XIN, and SER (0.07–0.11) and intermediate in ITA, TUC, BAL, and SEG (0.19–0.25) (Figure 2). About 75% of the samples in ITA and SEG and about 90% in all other reservoirs showed functional redundancy values lower than 0.3 (Figure 2), which indicates a high number of species with different traits, i.e., a low number of redundant species in these samples.
Explanatory Variables
This section describes the six selected environmental variables included in the general linear models to explain the diversity metrics (Table 3, next section). Seasonal water-residence time ranged from 0.1 to 1,577 days (Table 2). At each sampling station, the subsurface water temperature ranged from 16.2 to 34.8°C, with high median values in systems located at lower latitudes. Turbidity was generally low and went from 0.1 to 7.4 NTU (Table 2). TP ranged from 6.0 to 247.6 μg L−1, and TN from 235.0 to 5,415.8 μg L−1. The total zooplankton biomass at each sampling station went from zero to 4,535 µg dry weight L−1 among systems (data not shown). Medium-sized filter-feeders were the most important fraction of zooplankton (39% of the total zooplankton biomass). Grazing pressure estimated for medium-sized filter-feeders ranged from zero to 42 in the whole dataset, but the median value was only 0.2 (Table 2).
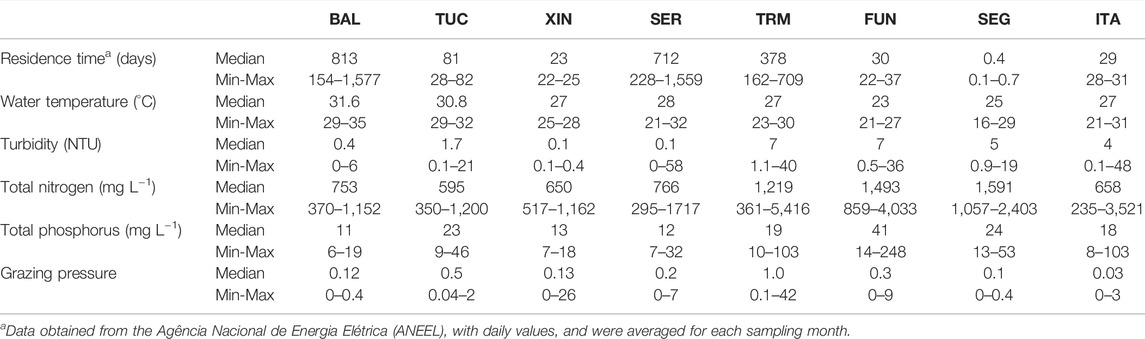
TABLE 2. Median, minimum (Min) and maximum (Max) values of the main explanatory variables in the reservoirs during the study period. Reservoirs name abbreviations as in Table 1.
The PCA using six environmental variables explained 61.6% of data variability in the first two axes (axis 1 = 41.2%; axis 2 = 20.4%). The first axis was positively related to TP (0.68), TN (0.76), turbidity (0.74), and negatively to water temperature (0.74). The second axis was positively associated with grazing pressure (0.87) and seasonal water residence (0.60) (Figure 3).
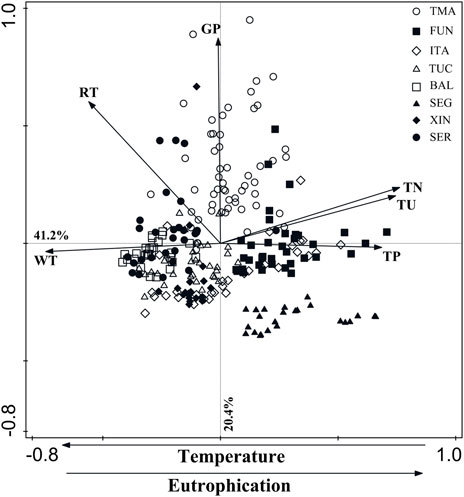
FIGURE 3. Plot of the Principal Components Analysis showing the distribution of the eight hydroelectric reservoirs studied, according to the environmental gradients. RT = seasonal water residence time; WT = water temperature; TU = turbidity; TN = total nitrogen; TP = total phosphorus; GP = grazing pressure. Abbreviations of reservoir names as in Table 1.
Relationships Among Functional Traits and Environmental Variables
We performed an RLQ analysis to determine the relationship between the phytoplankton traits and the environmental variables based on the species’ biovolume. The results of the RLQ indicated a significant relationship between phytoplankton functional traits and environmental variables (p = 0.0001), with the first two axes accounting for 98.6% of the trait-environment relationship (axis 1: 79.8%; axis 2: 18.8%), showed that phytoplankton traits are dependent of the environmental variables.
Only significant correlations among traits and environmental variables were considered in this section. Traits related to body size (MDL), the presence of mucilage, aerotopes, and blue pigments were positively related to turbidity and TN. While water temperature was positively related to single-celled species and negatively related to phytoplankton species with heterocytes, aerotopes, and blue pigment (Figure 4; Supplementary Figure S1). These associations among traits and increased water temperature, turbidity, TN, and TP were associated mainly with the sample points of SER, XNG, ITA, and FUN reservoirs (data not shown).
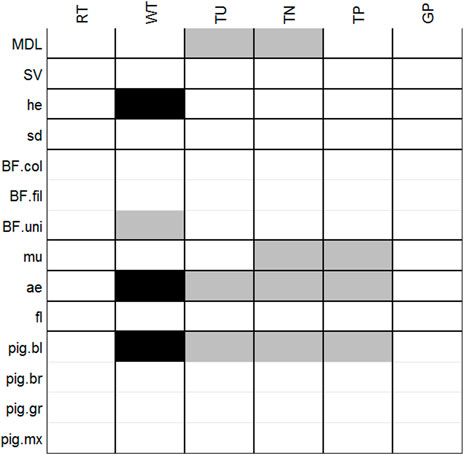
FIGURE 4. Results of the fourth-corner tests. Significant (p < 0.05) positive associations are represented by Gy cells and negative associations by black cells. Non-significant associations are in white. Black lines separate different variables; light gray lines separate modalities for categorical variables. p values were adjusted using the FDR (false discovery rate) procedure for multiple comparisons. MLD, maximum linear dimension; SV, surface volume ratio; he, presence of heterocyte; sd, silica demand; BF. col, biological form—colonies; BF. fil, biological form—filaments; BF. uni, biological form—unicells; mu, mucilage presence; ae, aerotopes; fl, flagella; pig. bl, blue pigment; pig. gr, green pigment; pig. br, brown pigment; and pig. mx, mixed pigment. Abbreviations of environmental variables as in Figure 2.
Relationship Between Taxonomic and Functional Diversity Versus Environmental Variables
The relationships between diversity indices and environmental variables were assessed with multiple generalized linear models (GLM). The variance explained by environmental conditions was higher for diversity indices based on biovolume than for identities (Table 3), fluctuating between 4% (D2 for TDPA and FDPA) and 11% of the explained variance (D2 for FDBV). Most diversity indices were positively associated with water residence time, water temperature, and, concerning productivity, TN and TP. The indices were negatively related to turbidity (except TDPA, positively) and grazing pressure (except TDPA, not related). Functional redundancy was negatively associated with water residence time and TP concentrations (Table 3).
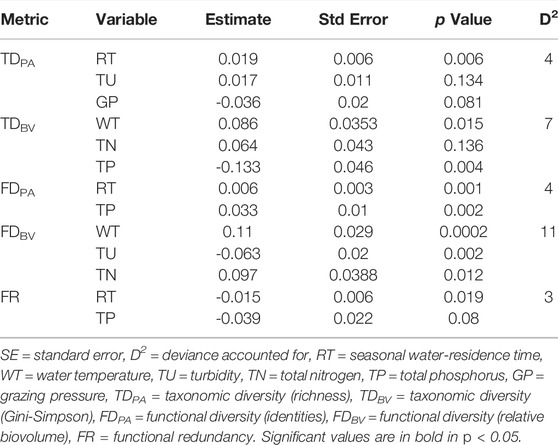
TABLE 3. Best multiple general linear models (GLM) for the relationships between diversity and environmental explanatory variables, using Akaike Information Criterion.
Discussion
In this study, we investigated the responses of phytoplankton diversity to environmental gradients. Functional and taxonomical diversity were affected by changes in environmental conditions, confirming our first hypothesis. We found that taxonomic (TD) and functional (FD) diversities were explained mainly by the same physical (water temperature, turbidity, and residence time) and chemical (TP and TN) variables. Higher TD and FD occurred in high-temperature conditions, high and low turbidity, and high TP. In addition, our findings indicated significant correlations between functional traits and the variation of environmental conditions. Our second hypotheses was, however, only partially confirmed. Although FD based on biomass has been, in fact, better predicted by environmental variables than TD also based on biomass, the variance explained in FD based on identities was equal to species richness. Confirming our third hypothesis, within each diversity dimension (taxonomic and functional), indices based on biomass were better related to the environment than their counterpart based on identities.
In the context of the niche theory, our study is more in line with Grinnellian concept, which describes the myriad of environmental requirements of species (i.e., abiotic factors, resources, and predators), than Eltonian concept of the niche, which represents the effect that a given species has on the community (i.e., trophic level in a food chain) (Leibold, 1995; Soberón, 2007). Grinnell’s view of the niche is similar to the concept of fundamental niche proposed by Hutchinson (1957), the hypervolume n-dimensional whose axes are the environmental variables affecting the species.
The positive influence of temperature is well recognized as a determinant for diversity in macroorganisms (Allen et al., 2002; Currie et al., 2004; Mittelbach et al., 2007). Particularly for phytoplankton, the temperature is among the major determinants of growth rates, nutrient stoichiometry, and the mixing regime of the waterbody, shaping the spatial and temporal distribution of populations in freshwater systems (Schabhüttl et al., 2013; Kruk et al., 2017). Several phytoplankton studies have shown positive relationships between taxonomic diversity and temperature (Ptacnick et al., 2010; Thomas et al., 2012; Weyhenmeyer et al., 2013; Kruk et al., 2017). Our findings agree with the primary literature indicating that taxonomic diversity, based on biovolume, is higher in warmer temperatures. We can relate our results for taxonomic diversity (species richness) to a study by Kruk et al. (2017) of a latitudinal gradient in shallow lakes along a broad latitudinal gradient (6,000 km). These authors found an increase in species richness from subpolar to subtropical and tropical regions, with no changes in functional diversity (as the richness of functional groups). They also found a higher functional redundancy in warmer areas due to the increase in the number of species in each functional group, which is related to lower and less-variable grazing pressure (Kruk et al., 2017). In our reservoirs, functional diversity (biovolume) was positively related to water temperature. This finding was related to unicellular species (biological forms) in warmer systems and the presence of heterocytes, aerotopes, and blue pigment in systems with lower water temperatures. We recorded a higher biovolume of different traits in warmer systems—high FDBV—which was positively related to temperature, enabling us to connect this result to high trait complementarity, which allows species to exploit different resources (Loreau, 2004; Petchey, 2004; Mason et al., 2005). The increase in temperature can result in the formation of several habitat layers for phytoplankton through stratification of the water column, contributing to the rise in species richness and traits to occupy different niches (Longhi & Beisner, 2010). Therefore, temperature acts as an essential selective agent in species adaptation, influencing the occurrence of particular traits (Schabhüttl et al., 2013) and maximizing the performance and maintenance of species in the system (Thomas et al., 2012; Schabhüttl et al., 2013).
Another critical variable acting upon phytoplankton diversity is the seasonal water-residence time, which affects the system volume and area (Thomaz et al., 2004), and the availability of light and nutrients (Descy, 1993; Schindler, 2006; Obertergger et al., 2007). In general, a higher water flow is an additional factor in removing phytoplankton biomass and populations from reservoirs with a shorter residence time (Obertergger et al., 2007; Rangel et al., 2012). However, systems with a long seasonal water-residence time have patches with greater physical stability, which leads to the formation of horizontal (Kalff, 2002) and vertical gradients (Longhi & Beisner, 2010), and allows increases in species richness and abundance (Dunck et al., 2013; Stević et al., 2013). Our results showed greater taxonomic and functional diversity, in identities, in our mainly stratified systems with longer residence times. It suggests that more-stable conditions might favor the differentiation of niches that different species can fill, with less competition and greater efficiency in resource use (Loreau, 2004; Mason et al., 2005; Kruk et al., 2017).
Beyond temperature and water residence time, resources such as light and nutrients, bottom-up controls on phytoplankton growth also affect species richness (Tilman & Pacala, 1993; Rosenzweig, 1995; Worm et al., 2002). Taxonomic diversity, i.e., species richness, and the system productivity relationships depend on the range of productivity, which is usually recorded in only part of a gradient (Smith, 2007). For a broad trophic gradient - from oligotrophic to hypereutrophic - a unimodal relationship between species richness and productivity is expected, as recorded for phytoplankton in continental aquatic systems (Dodson et al., 2000; Smith, 2007; Stomp et al., 2011; Soininen & Luoto, 2012), as well as for terrestrial and marine communities (Grime, 1973; Rosenzweig, 1995; Mittelbach et al., 2001; Irigoien et al., 2004). Most of our reservoirs are mesotrophic, based on the nitrogen and phosphorus concentrations (Nürnberg, 1996), except Funil, which is eutrophic. Although the reservoirs showed a range of productivity, this was not sufficiently wide to capture the unimodal variation in diversity. Instead, high values of functional diversity (identities and biomass) were found in the higher TP and TN ranges, respectively. These high values mean that most species have different traits, which indicates a complementarity of species in those conditions (Loreau, 2004; Petchey et al., 2007; Kruk et al., 2017). Surprisingly, taxonomic diversity based on biovolume was negatively related to phosphorus concentrations and indicated the decrease of total phytoplankton biomass in this condition, but the trend was unclear.
Light availability is essential for phytoplankton and can modify the community composition in the pelagic zone (Loiselle et al., 2007; Stomp et al., 2007; Filstrup et al., 2016). In experimental studies, the most efficient use of light was reported in cultures with a high richness of both species and functional groups (Stockenreiter et al., 2013). Only a few species can survive in systems with high turbidity (Weithoff et al., 2015), which should favor species with large, elongated bodies and a high surface-volume ratio that deviates from the spherical shape, making them good light antennae (Reynolds, 2006; Kruk et al., 2010; Colina et al., 2015). In this study, we could observe that traits associated with high productivity and low light availability were particular to cyanobacteria (blue pigment, aerotopes, mucilage, and large body size). Therefore, high turbidity should lead to low functional diversity, with species shared traits common to a single group—Cyanobacteria, as confirmed by the negative relationship between turbidity versus functional diversity (biovolume) in our GLM models.
Biotic interactions also determine the phytoplankton diversity (Vogt et al., 2010; Kruk et al., 2017). A negative relationship of both taxonomic and functional richness to grazing pressure is expected (Padisák, 2003; Muylaert et al., 2010; Özkan et al., 2013; Gianuca et al., 2016), because grazing affects the body-size structure of the community, as a large maximum linear dimension serves as an obstacle to grazing (Vanni, 1987). We observed a negative relationship between grazing pressure and taxonomic diversity (identities), but this predictor did not significantly affect the traits in our systems.
Despite a significant part of the variation in taxonomic and functional diversity of phytoplankton in our datasets could be explained by environmental variables, the largest proportion of the variation in phytoplankton diversity (89–96%) remained unexplained. A potential explanation for those high residual values may be due unmeasured environmental variables, and the inherent chaotic behavior of phytoplankton communities (Beninca et al., 2008; Kosten et al., 2012).
The increase in FD is usually related to a higher degree of niche differentiation, lower resource competition, and more efficient use of resources (Mason et al., 2005; De Bello et al., 2006). As found in previous studies (Petchey et al., 2004; De Bello et al., 2006; Mouchet et al., 2010), the strength of the relationships was usually greater for functional (D2 = 4 and 11%, identities and biomass, respectively) than for taxonomic diversity (D2 = 4 and 7%, identities and biomass, respectively), when biomass was considered responded more closely to environmental gradients. Assuming that species are not equal in their effects on ecosystem functioning (Mouchet et al., 2010), the trait responses express species interactions with ecosystem processes and the optimum use of resources (McGill et al., 2006; Westoboy & Wright, 2006), leading to a closer correlation between functional diversity and environmental gradients. Additionally, mechanisms that support the coexistence of many species do not necessarily support functional differentiation among those species (Fukami et al., 2005; De Bello et al., 2006; Guareshi et al., 2015). According to De Bello et al. (2006), environmental gradients may limit the species composition to a specific range of functional characteristics, thus limiting the degree of variation of functional diversity concerning taxonomic diversity.
One criterion that ecologists often use to organize the various diversity measurements is whether the index is based on species identities only or if the species abundances (or biomass) are incorporated into the index. The idea behind the identity index is that each species may strongly influence ecosystem functioning and that rare species could have dominant effects on ecosystem functioning. In contrast, indices based on species abundance emphasize the role of dominant species, which are assumed to have a more significant impact on ecosystem functioning than do rare species (see Lohbeck et al., 2012 and references therein).
The patterns of the relationship between functional redundancy and environmental conditions are not yet clear because species that are functionally redundant in one environment may become pivotal in another (Fetzer et al., 2015). It has been suggested that in the tropics, the species are primarily ¨neutral¨, and therefore, the number of redundant species is high (Fauset et al., 2015; Scheffer et al., 2015). There is no clear explanation for this high functional redundancy in the tropics. Still, some authors have related this finding to the increase of rare species and high trait similarity, which indicate potential neutrality among species (Kruk et al., 2017). Therefore further studies in different environmental situations are needed, to understand this relationship.
Particularly for phytoplankton, more redundant species were found during the low-water season, when productivity in Amazonian floodplain lakes is higher, compared to the high-water season when complementarity increases and grazing pressure decreases (Cardoso et al., 2017). High complementarity was also recorded in temperate shallow lakes sheltered from wind mixing (Longhi & Beisner, 2010). In a different approach, a higher functional redundancy for subtropical and tropical shallow lakes has been related to a combination of environmental stability (high temperature), lower grazing pressure, and lower competition, which lead to a smaller variance in traits and a higher species richness in warmer regions (Kruk et al., 2017). In contrast to other results for tropical areas (Cardoso et al., 2017; Kruk et al., 2017), we found a high complementarity in our large subtropical and tropical reservoirs, rather than a low functional redundancy of species. High complementarity may occur in systems at larger spatial and environmental scales, allowing species to coexist in systems with high environmental heterogeneity (Loreau, 2004). Therefore, our stratified and horizontally heterogeneous systems (e.g., Borges et al., 2008; Soares et al., 2009; Kemenes et al., 2011; Brasil-MME, 2014) may have high niche differentiation, enabling the coexistence of complementary species with high dissimilarity among traits to more-efficiently exploit different resources (Loreau, 2004; Petchey et al., 2007).
Conclusion
In summary, our results show that phytoplankton diversity, both taxonomic and functional, is dependent on environmental conditions. High functional and taxonomic diversities occur in warm, clear, and enriched systems with low zooplankton grazing pressure. Our results corroborate with other studies finding that functional diversity may be better related to environmental conditions than taxonomic diversity, at least when using indices based on biomass. Our study also suggests that diversity metrics (taxonomic or functional) based on biomass may be more suitable for predicting changes in diversity from environmental conditions.
Data Availability Statement
The original contributions presented in the study are included in the article/Supplementary Material, further inquiries can be directed to the corresponding author.
Author Contributions
JS, JB, and VH contributed to conception and design of the study. JS organized the database, performed the statistical analysis and wrote the manuscript. All authors contributed to manuscript revision, read, and approved the submitted version.
Funding
JS and VH were partially supported by the Conselho Nacional de Desenvolvimento Científico e Tecnológico (CNPq, Brazil) (grants 141650/2010-9 and 309700/2013-2, respectively). This research was financially supported by ANEEL (National Electrical Energy Agency, Brazil).
Conflict of Interest
The authors declare that the research was conducted in the absence of any commercial or financial relationships that could be construed as a potential conflict of interest.
Publisher’s Note
All claims expressed in this article are solely those of the authors and do not necessarily represent those of their affiliated organizations, or those of the publisher, the editors and the reviewers. Any product that may be evaluated in this article, or claim that may be made by its manufacturer, is not guaranteed or endorsed by the publisher.
Acknowledgments
We are grateful to the BALCAR/ANEEL project team; to Fábio Roland from the Federal University of Juiz de Fora for sampling and limnological analyses; to Lúcia Helena Sampaio da Silva, Carolina Domingues, and Leonardo de Magalhães for the phytoplankton analyses in part; to Marcus Cianciaruso from the Federal University of Goiás and João Carlos Nabout from the State University of Goiás for fruitful discussions on functional diversity indices; and to Janet W. Reid (JWR Associates) for revising the English text.
Supplementary Material
The Supplementary Material for this article can be found online at: https://www.frontiersin.org/articles/10.3389/fenvs.2022.899571/full#supplementary-material
References
Allen, A. P., Brown, J. H., and Gillooly, J. F. (2002). Global Biodiversity, Biochemical Kinetics, and the Energetic-Equivalence Rule. Science 297 (5586), 1545–1548. doi:10.1126/science.1072380
APHA (2005). Standard Methods for the Examination of Water and Wastewater. Washington, DC: American Public Health Association.
Barrow, J. L., Beisner, B. E., Giles, R., Giani, A., Domaizon, I., and Gregory‐Eaves, I. (2019). Macrophytes Moderate the Taxonomic and Functional Composition of Phytoplankton Assemblages during a Nutrient Loading Experiment. Freshw. Biol. 64, 1369–1381. doi:10.1111/fwb.13311
Bello, F., Lepš, J., Lavorel, S., and Moretti, M. (2007). Importance of Species Abundance for Assessment of Trait Composition: an Example Based on Pollinator Communities. Community Ecol. 8, 163–170. doi:10.1556/comec.8.2007.2.3
Benincà, E., Huisman, J., Heerkloss, R., Jöhnk, K. D., Branco, P., Van Nes, E. H., et al. (2008). Chaos in a Long-Term Experiment with a Plankton Community. Nature 451, 822–825. doi:10.1038/nature06512
Benjamini, Y., and Hochberg, Y. (1995). Controlling the False Discovery Rate: a Practical and Powerful Approach to Multiple Testing. J. R. Stat. Soc. Ser. B Methodol. 57, 289–300. doi:10.1111/j.2517-6161.1995.tb02031.x
Bergkemper, V., Stadler, P., and Weisse, T. (2018). Moderate Weather Extremes Alter Phytoplankton Diversity-A Microcosm Study. Freshw. Biol. 63, 1211–1224. doi:10.1111/fwb.13127
Borges, P. A. F., Train, S., and Rodrigues, L. C. (2008). Spatial and Temporal Variation of Phytoplankton in Two Subtropical Brazilian Reservoirs. Hydrobiologia 607, 63–74. doi:10.1007/s10750-008-9367-3
Borges, P., and Train, S. (2009). Phytoplankton Diversity in the Upper Paraná River Floodplain during Two Years of Drought (2000 and 2001). Braz. J. Biol. 69, 637–647. doi:10.1590/S1519-69842009000300018
Botta‐Dukát, Z. (2005). Rao's Quadratic Entropy as a Measure of Functional Diversity Based on Multiple Traits. J. Veg. Sci. 16, 533–540. doi:10.1111/j.1654-1103.2005.tb02393.x
Brasil (2019). Bases Cartográficas Contínuas 1:250.000. Rio de Janeiro, Brazil: Instituto Brasileiro de Geografia e Estatística. Available at: https://portaldemapas.ibge.gov.br/portal.php#mapa222602 (Accessed 04 27, 2022).
Brasil-MME (Ministério de Minas e Energia) (2014). Emissões de Gases de Efeito Estufa em Reservatórios de Centrais Hidrelétricas. Rio de Janeiro: CEPEL.
Burnham, K. P., and Anderson, D. R. (2002). Model Selection and Multimodel Inference: A Practical Information-Theoretic Approach. New York: Springer-Verlag.
Cadotte, M. W., Carscadden, K., and Mirotchnick, N. (2011). Beyond Species: Functional Diversity and the Maintenance of Ecological Processes and Services. J. Appl. Ecol. 48, 1079–1087. doi:10.1111/j.1365-2664.2011.02048.x
Cardoso, S. J., Nabout, J. C., Farjalla, V. F., Lopes, P. M., Bozelli, R. L., Huszar, V. L. M., et al. (2017). Environmental Factors Driving Phytoplankton Taxonomic and Functional Diversity in Amazonian Floodplain Lakes. Hydrobiologia 802, 115–130. doi:10.1007/s10750-017-3244-x
Colina, M., Calliari, D., Carballo, C., and Kruk, C. (2015). A Trait-Based Approach to Summarize Zooplankton-Phytoplankton Interactions in Freshwaters. Hydrobiologia 767, 221–233. doi:10.1007/s10750-015-2503-y
Crumpton, W. G., Isenhart, T. M., and Mitchell, P. D. (1992). Nitrate and Organic N Analyses with Second-Derivative Spectroscopy. Limnol. Oceanogr. 37, 907–913. doi:10.4319/lo.1992.37.4.0907
Culver, D. A., Boucherle, M. M., Bean, D. J., and Fletcher, J. W. (1985). Biomass of Freshwater Crustacean Zooplankton from Length-Weight Regressions. Can. J. Fish. Aquat. Sci. 42, 1380–1390. doi:10.1139/f85-173
Currie, D. J., Mittelbach, G. G., Cornell, H. V., Field, R., Guegan, J.-F., Hawkins, B. A., et al. (2004). Predictions and Tests of Climate-Based Hypotheses of Broad-Scale Variation in Taxonomic Richness. Ecol. Lett. 7, 1121–1134. doi:10.1111/j.1461-0248.2004.00671.x
De Bello, F., Carmona, C. P., Lepš, J., Szava-Kovats, R., and Pärtel, M. (2016). Functional Diversity through the Mean Trait Dissimilarity: Resolving Shortcomings with Existing Paradigms and Algorithms. Oecologia 180, 933–940. doi:10.1007/s00442-016-3546-0
De Bello, F., Lepš, J., and Sebastià, M.-T. (2006). Variations in Species and Functional Plant Diversity along Climatic and Grazing Gradients. Ecography 29, 801–810. ISSN: 0906-7590. doi:10.1111/j.2006.0906-7590.04683.x
De Fillipo, R., Gomes, E. L., Lenz-César, J., Soares, C. B. P., and Menezes, C. F. S. (1999). “As alterações na qualidade da Água durante o enchimento Do Reservatório de UHE Serra da Mesa – GO,” in Ecologia de Reservatórios: Estrutura, Função e Aspectos Sociais. Editor R. Henry (São Paulo, Brazil: FUNDIBIO: FAPESP), 321–346.
Descy, J.-P. (1993). Ecology of the Phytoplankton of the River Moselle: Effects of Disturbances on Community Structure and Diversity. Hydrobiologia 249, 111–116. doi:10.1007/BF00008847
Díaz, S., and Cabido, M. (2001). Vive la différence: plant functional diversity matters to ecosystem processes. Trends Ecol. Evol. 16, 646–655. doi:10.1016/S0169-5347(01)02283-2
Dodson, S. I., Arnott, S. E., and Cottingham, K. L. (2000). The Relationship in Lake Communities between Primary Productivity and Species Richness. Ecology 81, 2662–2679. doi:10.1890/0012-9658(2000)081[2662:TRILCB]2.0.CO;2
Dolédec, S., Chessel, D., ter Braak, C. J. F., and Champely, S. (1996). Matching Species Traits to Environmental Variables: a New Three-Table Ordination Method. Environ. Ecol. Stat. 3, 143–166. doi:10.1007/BF02427859
Dray, S., Choler, P., Dolédec, S., Peres-Neto, P. R., Thuiller, W., Pavoine, S., et al. (2014). Combining the Fourth‐corner and the RLQ Methods for Assessing Trait Responses to Environmental Variation. Ecology 95, 14–21. doi:10.1890/13-0196.1
Dray, S., and Dufour, A.-B. (2007). Theade4Package: Implementing the Duality Diagram for Ecologists. J. Stat. Soft. 22, 1–20. doi:10.18637/jss.v022.i04
Dunck, B., Bortolini, J. C., Rodrigues, L., Rodrigues, L. C., Jati, S., and Train, S. (2013). Functional Diversity and Adaptative Strategies of Planktonic and Periphytic Algae in Isolated Tropical Floodplain Lake. Braz. J. Bot. 36, 257–266. doi:10.1007/s40415-013-0029-y
Dunck, B., Schneck, F., and Rodrigues, L. (2015). Patterns in Species and Functional Dissimilarity: Insights from Periphytic Algae in Subtropical Floodplain Lakes. Hydrobiologia 763, 237–247. doi:10.1007/s10750-015-2379-x
Esteves, F. D. A., Amorim, J. C., Cardoso, E. L., and Barbosa, F. A. R. (1985). Caracterização limnológica preliminar da represa de Três Marias (MG) com base em alguns parâmetros ambientais básicos. Ciência Cult. 37, 608–617.
Fauset, S., Johnson, M. O., Gloor, M., Baker, T. R., Monteagudo M, A., Brienen, R. J., et al. (2015). Hyperdominance in Amazonian Forest Carbon Cycling. Nat. Commun. 6, 6857. doi:10.1038/ncomms7857
Fetzer, I., Johst, K., Schäwe, R., Banitz, T., Harms, H., and Chatzinotas, A. (2015). The Extent of Functional Redundancy Changes as Species' Roles Shift in Different Environments. Proc. Natl. Acad. Sci. U.S.A. 112, 14888–14893. doi:10.1073/pnas.1505587112
Filstrup, C., Heathcote, A., Kendall, D., and Downing, J. (2016). Phytoplankton Taxonomic Compositional Shifts across Nutrient and Light Gradients in Temperate Lakes. Iw 6, 234–249. doi:10.5268/IW-6.2.939
Fukami, T., Martijn Bezemer, T., Mortimer, S. R., and Putten, W. H. (2005). Species Divergence and Trait Convergence in Experimental Plant Community Assembly. Ecol. Lett. 8, 1283–1290. doi:10.1111/j.1461-0248.2005.00829.x
Gianuca, A. T., Pantel, J. H., and De Meester, L. (2016). Disentangling the Effect of Body Size and Phylogenetic Distances on Zooplankton Top-Down Control of Algae. Proc. R. Soc. B 283, 20160487. doi:10.1098/rspb.2016.0487
Graco-Roza, C., Soininen, J., Corrêa, G., Pacheco, F. S., Miranda, M., Domingos, P., et al. (2021). Functional rather Than Taxonomic Diversity Reveals Changes in the Phytoplankton Community of a Large Dammed River. Ecol. Indic. 121, 107048. doi:10.1016/j.ecolind.2020.107048
Grime, J. P. (1973). Competitive Exclusion in Herbaceous Vegetation. Nature 242, 344–347. doi:10.1038/242344a0
Guareschi, S., Bilton, D. T., Velasco, J., Millán, A., and Abellán, P. (2015). How Well Do Protected Area Networks Support Taxonomic and Functional Diversity in Non-target Taxa? the Case of Iberian Freshwaters. Biol. Conserv. 187, 134–144. doi:10.1016/j.biocon.2015.04.018
Hillebrand, H., Dürselen, C.-D., Kirschtel, D., Pollingher, U., and Zohary, T. (1999). Biovolume Calculation for Pelagic and Benthic Microalgae. J. Phycol. 35, 403–424. doi:10.1046/j.1529-8817.1999.3520403.x
Hubbell, S. P. (2011). The Unified Neutral Theory of Biodiversity and Biogeography (MPB-32). Princeton, NJ: Princeton University Press. doi:10.1515/9781400837526
Huszar, V. L. M., Silva, L. H. S., Domingos, P., Marinho, M., and Melo, S. (1998). Phytoplankton Species Composition Is More Sensitive Than OECD Criteria to the Trophic Status of Three Brazilian Tropical Lakes. Hydrobiologia 369/370, 59–71. doi:10.1023/A:1017047221384
Hutchinson, G. E. (1957). Concluding Remarks. Cold Spring Harb. Symposia Quantitative Biol. 22, 415–427. doi:10.1101/sqb.1957.022.01.039
Irigoien, X., Huisman, J., and Harris, R. P. (2004). Global Biodiversity Patterns of Marine Phytoplankton and Zooplankton. Nature 429, 863–867. doi:10.1038/nature02593
Jackson, D. A. (1993). Stopping Rules in Principal Components Analysis: a Comparison of Heuristical and Statistical Approaches. Ecology 74, 2204–2214. doi:10.2307/1939574
Jeppesen, E., Søndergaard, M., Kanstrup, E., Petersen, B., Eriksen, R. B., Hammershøj, M., et al. (1994). Does the Impact of Nutrients on the Biological Structure and Function of Brackish and Freshwater Lakes Differ? Hydrobiologia 275-276, 15–30. doi:10.1007/BF00026696
Jeppesen, E., Søndergaard, M., Søndergaard, M., and Christofferson, K. (1997). The Structuring Role of Submerged Water Plants in Lakes. New York: Springer-Verlag.
Kalff, J. (2002). Limnology: Inland Water Ecosystems. Upper Saddle River, NJ: Prentice-Hall. ISBN: 0-13-033775-7.
Kemenes, A., Forsberg, B. R., and Melack, J. M. (2011). CO2emissions from a Tropical Hydroelectric Reservoir (Balbina, Brazil). J. Geophys. Res. 116, G03004. doi:10.1029/2010JG001465
Kosten, S., Huszar, V. L. M., Bécares, E., Costa, L. S., Donk, E., Hansson, L.-A., et al. (2012). Warmer Climates Boost Cyanobacterial Dominance in Shallow Lakes. Glob. Change Biol. 18, 118–126. doi:10.1111/j.1365-2486.2011.02488.x
Kruk, C., Huszar, V. L. M., Peeters, E. T. H. M., Bonilla, S., Costa, L., Lürling, M., et al. (2010). A Morphological Classification Capturing Functional Variation in Phytoplankton. Freshw. Biol. 55, 614–627. doi:10.1111/j.1365-2427.2009.02298.x
Kruk, C., Segura, A. M., Costa, L. S., Lacerot, G., Kosten, S., Peeters, E. T. H. M., et al. (2017). Functional Redundancy Increases towards the Tropics in Lake Phytoplankton. J. Plankton Res. 39, 518–530. doi:10.1093/plankt/fbw083
Le Noac’h, P., Jobin, V. O., and Beisner, B. E. (2021). Effects of Vertical Spatial Overlap on Phytoplankton Diversity under Experimentally Altered Lake Stratification Regimes. Microorganisms 9, 2447. doi:10.3390/microorganisms912244/
Legendre, P., Galzin, R., and Harmelin-Vivien, M. L. (1997). Relating Behavior to Habitat: Solutions to Thefourth-Corner Problem. Ecology 78, 547–562. doi:10.1890/0012-9658(1997)078[0547:RBTHST]2.0.CO;2
Leibold, M. A. (1995). The Niche Concept Revisited: Mechanistic Models and Community Context. Ecology 76, 1371–1382. doi:10.2307/1938141
Litchman, E., and Klausmeier, C. A. (2008). Trait-based Community Ecology of Phytoplankton. Annu. Rev. Ecol. Evol. Syst. 39, 615–639. doi:10.1146/annurev.ecolsys.39.110707.173549
Lohbeck, M., Poorter, L., Paz, H., Pla, L., van Breugel, M., Martínez-Ramos, M., et al. (2012). Functional Diversity Changes during Tropical Forest Succession. Perspect. Plant Ecol. Evol. Syst. 14, 89–96. doi:10.1016/j.ppees.2011.10.002
Loiselle, S. A., Cózar, A., Dattilo, A., Bracchini, L., and Gálvez, J. A. (2007). Light Limitations to Algal Growth in Tropical Ecosystems. Freshw. Biol. 52, 305–312. doi:10.1111/j.1365-2427.2006.01693.x
Longhi, M. L., and Beisner, B. E. (2010). Patterns in Taxonomic and Functional Diversity of Lake Phytoplankton. Freshw. Biol. 55, 1349–1366. doi:10.1111/j.1365-2427.2009.02359.x
Loreau, M. (2004). Does Functional Redundancy Exist? Oikos 104, 606–611. doi:10.1111/j.0030-1299.2004.12685.x
Lund, J. W. G., Kipling, C., and Le Cren, E. D. (1958). The Inverted Microscope Method of Estimating Algal Numbers and the Statistical Basis of Estimations by Counting. Hydrobiologia 11, 143–170. doi:10.1007/BF00007865
Mackereth, F. J. H., Heron, J., and Talling, J. F. (1978). Water Analysis: Some Revised Methods for Limnologists. Far Sawrey, Ambleside: Freshwater Biological Association Scientific Publication No. 36.
Magurran, A. E. (2005). Biological Diversity. Curr. Biol. 15, 116–118. ISBN: 978-1-118-68792-5. doi:10.1016/j.cub.2005.02.006
Magurran, A. E. (2011). Medindo a Diversidade Biológica. (Translator: D.M. Vianna). Curitiba: Editora UFPR. ISBN: 9788573352788.
Mason, N. W. H., Mouillot, D., Lee, W. G., and Wilson, J. B. (2005). Functional Richness, Functional Evenness and Functional Divergence: the Primary Components of Functional Diversity. Oikos 111, 112–118. doi:10.1111/j.0030-1299.2005.13886.x
McGill, B., Enquist, B., Weiher, E., and Westoby, M. (2006). Rebuilding Community Ecology from Functional Traits. Trends Ecol. Evol. 21, 178–185. doi:10.1016/j.tree.2006.02.002
Melo, A. S., and Hepp, L. U. (2008). Ferramentas Estatísticas Para Análises De Dados Provenientes De Biomonitoramento. Oecol. Austr. 12, 463–486. doi:10.4257/oeco.2008.1203.07
Mittelbach, G. G., Schemske, D. W., Cornell, H. V., Allen, A. P., Brown, J. M., Bush, M. B., et al. (2007). Evolution and the Latitudinal Diversity Gradient: Speciation, Extinction and Biogeography. Ecol. Lett. 10, 315–331. doi:10.1111/j.1461-0248.2007.01020.x
Mittelbach, G. G., Steiner, C. F., Scheiner, S. M., Gross, K. L., Reynolds, H. L., Waide, R. B., et al. (2001). What Is the Observed Relationship between Species Richness and Productivity? Ecology 82, 2381–2396. doi:10.1890/0012-9658(2001)082[2381:WITORB]2.0.CO;2
Mouchet, M. A., Villéger, S., Mason, N. W. H., and Mouillot, D. (2010). Functional Diversity Measures: an Overview of Their Redundancy and Their Ability to Discriminate Community Assembly Rules. Funct. Ecol. 24, 867–876. doi:10.1111/j.1365-2435.2010.01695.x
Mouillot, D., Graham, N. A. J., Villéger, S., Mason, N. W. H., and Bellwood, D. R. (2013). A Functional Approach Reveals Community Responses to Disturbances. Trends Ecol. Evol. 28, 167–177. doi:10.1016/j.tree.2012.10.004
Muylaert, K., Pérez-Martínez, C., Sánchez-Castillo, P., Lauridsen, T. L., Vanderstukken, M., Declerck, S. A. J., et al. (2010). Influence of Nutrients, Submerged Macrophytes and Zooplankton Grazing on Phytoplankton Biomass and Diversity along a Latitudinal Gradient in Europe. Hydrobiologia 653, 79–90. doi:10.1007/s10750-010-0345-1
Nabout, J. C., de Nogueira, I. S., de Oliveira, L. G., and Morais, R. R. (2007). Phytoplankton Diversity (Alpha, Beta, and Gamma) from the Araguaia River Tropical Floodplain Lakes (Central Brazil). Hydrobiologia 575, 455–461. doi:10.1007/s10750-006-0393-8
Nürnberg, G. K. (1996). Trophic State of Clear and Colored, Soft- and Hardwater Lakes with Special Consideration of Nutrients, Anoxia, Phytoplankton and Fish. Lake Reserv. Manag. 12, 432–447. doi:10.1080/07438149609354283
Obertegger, U., Flaim, G., Braioni, M. G., Sommaruga, R., Corradini, F., and Borsato, A. (2007). Water Residence Time as a Driving Force of Zooplankton Structure and Succession. Aquat. Sci. 69, 575–583. doi:10.1007/s00027-007-0924-z
Özkan, K., Jeppesen, E., Søndergaard, M., Lauridsen, T. L., Liboriussen, L., and Svenning, J.-C. (2013). Contrasting Roles of Water Chemistry, Lake Morphology, Land-Use, Climate and Spatial Processes in Driving Phytoplankton Richness in the Danish Landscape. Hydrobiologia 710, 173–187. doi:10.1007/s10750-011-0996-6
Padisák, J. (2003). “Phytoplankton,” in The Lakes Handbook 1. Limnology and Limnetic Ecology. Editors P. E. O'Sullivan, and C. S. Reynolds (Oxford, UK: Blackwell Publishing), 251–308. ISBN: 978-0-632-04797-0.
Pálffy, K., Présing, M., and Vörös, L. (2013). Diversity Patterns of Trait-Based Phytoplankton Functional Groups in Two Basins of a Large, Shallow Lake (Lake Balaton, Hungary) with Different Trophic State. Aquat. Ecol. 47, 195–210. doi:10.1007/s10452-013-9434-3
Petchey, O. L., Evans, K. L., Fishburn, I. S., and Gaston, K. J. (2007). Low Functional Diversity and No Redundancy in British Avian Assemblages. J. Anim. Ecol. 76, 977–985. doi:10.1111/j.1365-2656.2007.01271.x
Petchey, O. L., and Gaston, K. J. (2002). Functional Diversity (FD), Species Richness and Community Composition. Ecol. Lett. 5, 402–411. doi:10.1046/j.1461-0248.2002.00339.x
Petchey, O. L., Hector, A., and Gaston, K. J. (2004). How Do Different Measures of Functional Diversity Perform? Ecology 85, 847–857. doi:10.1890/03-0226
Petchey, O. L. (2004). On the Statistical Significance of Functional Diversity Effects. Funct. Ecol. 18, 297–303. doi:10.1111/j.0269-8463.2004.00852.x
Pillar, V. D., Blanco, C. C., Müller, S. C., Sosinski, E. E., Joner, F., and Duarte, L. D. S. (2013). Functional Redundancy and Stability in Plant Communities. J. Veg. Sci. 24, 963–974. doi:10.1111/jvs.12047
Ptacnik, R., Andersen, T., Brettum, P., Lepistö, L., and Willén, E. (2010). Regional Species Pools Control Community Saturation in Lake Phytoplankton. Proc. R. Soc. B 277, 3755–3764. doi:10.1098/rspb.2010.1158
Rangel, L. M., Silva, L. H. S., Rosa, P., Roland, F., and Huszar, V. L. M. (2012). Phytoplankton Biomass Is Mainly Controlled by Hydrology and Phosphorus Concentrations in Tropical Hydroelectric Reservoirs. Hydrobiologia 693, 13–28. doi:10.1007/s10750-012-1083-3
Rao, C. R. (1982). Diversity and Dissimilarity Coefficients: a Unified Approach. Theor. Popul. Biol. 21, 24–43. doi:10.1016/0040-5809(82)90004-1
Reynolds, C. S., Padisák, J., and Sommer, U. (1993). “Intermediate Disturbance in the Ecology of Phytoplankton and the Maintenance of Species Diversity: a Synthesis,” in Intermediate Disturbance Hypothesis in Phytoplankton Ecology. Developments in Hydrobiology 81. Editors J. Padisák, C. S. Reynolds, and U. Sommer (Dordrecht: Springer), 183–188. doi:10.1007/978-94-017-1919-3_17
Reynolds, C. S. (2006). The Ecology of Phytoplankton. Cambridge, UK: Cambridge University Press. doi:10.1017/CBO9780511542145
Ricotta, C., and Moretti, M. (2011). CWM and Rao's Quadratic Diversity: a Unified Framework for Functional Ecology. Oecologia 167, 181–188. doi:10.1007/s00442-011-1965-5
Rosenzweig, M. L. (1995). Species Diversity in Space and Time. Cambridge, UK: Cambridge University Press. doi:10.1017/CBO9780511623387
Santos, A. M. C., Carneiro, F. M., and Cianciaruso, M. V. (2015). Predicting Productivity in Tropical Reservoirs: The Roles of Phytoplankton Taxonomic and Functional Diversity. Ecol. Indic. 48, 428–435. doi:10.1016/j.ecolind.2014.08.033
Schabhüttl, S., Hingsamer, P., Weigelhofer, G., Hein, T., Weigert, A., and Striebel, M. (2013). Temperature and Species Richness Effects in Phytoplankton Communities. Oecologia 171, 527–536. doi:10.1007/s00442-012-2419-4
Scheffer, M., Vergnon, R., Van Nes, E. H., Cuppen, J. G. M., Peeters, E. T. H. M., Leijs, R., et al. (2015). The Evolution of Functionally Redundant Species; Evidence from Beetles. PLoS ONE 10, e0137974. doi:10.1371/journal.pone.0137974
Schindler, D. W. (2006). Recent Advances in the Understanding and Management of Eutrophication. Limnol. Oceanogr. 51, 356–363. doi:10.4319/lo.2006.51.1_part_2.0356
Smith, V. H., Foster, B. L., Grover, J. P., Holt, R. D., Leibold, M. A., and Denoyelles, F. (2005). Phytoplankton Species Richness Scales Consistently from Laboratory Microcosms to the World's Oceans. Proc. Natl. Acad. Sci. U.S.A. 102, 4393–4396. doi:10.1073/pnas.0500094102
Smith, V. H. (2007). Microbial Diversityâ€"productivity Relationships in Aquatic Ecosystems. FEMS Microbiol. Ecol. 62, 181–186. doi:10.1111/j.1574-6941.2007.00381.x
Soares, M. C. S., Marinho, M. M., Azevedo, S. M. O. F., Branco, C. W. C., and Huszar, V. L. M. (2012). Eutrophication and Retention Time Affecting Spatial Heterogeneity in a Tropical Reservoir. Limnologica 42, 197–203. doi:10.1016/j.limno.2011.11.002
Soares, M., de A Rocha, M., Marinho, M., Azevedo, S., Branco, C., and Huszar, V. (2009). Changes in Species Composition during Annual Cyanobacterial Dominance in a Tropical Reservoir: Physical Factors, Nutrients and Grazing Effects. Aquat. Microb. Ecol. 57, 137–149. doi:10.3354/ame01336
Soberón, J. (2007). Grinnellian and Eltonian Niches and Geographic Distributions of Species. Ecol. Lett. 10, 1115–1123. doi:10.1111/j.1461-0248.2007.01107.x
Soininen, J., and Luoto, M. (2012). Is Catchment Productivity a Useful Predictor of Taxa Richness in Lake Plankton Communities? Ecol. Appl. 22, 624–633. doi:10.1890/11-1126.1
Stević, F., Mihaljević, M., and Špoljarić, D. (2013). Changes of Phytoplankton Functional Groups in a Floodplain Lake Associated with Hydrological Perturbations. Hydrobiologia 709, 143–158. doi:10.1007/s10750-013-1444-6
Stockenreiter, M., Haupt, F., Graber, A.-K., Seppälä, J., Spilling, K., Tamminen, T., et al. (2013). Functional Group Richness: Implications of Biodiversity for Light Use and Lipid Yield in Microalgae. J. Phycol. 49, 838–847. doi:10.1111/jpy.12092
Stomp, M., Huisman, J., Mittelbach, G. G., Litchman, E., and Klausmeier, C. A. (2011). Large-scale Biodiversity Patterns in Freshwater Phytoplankton. Ecology 92, 2096–2107. doi:10.1890/10-1023.1
Stomp, M., Huisman, J., Vörös, L., Pick, F. R., Laamanen, M., Haverkamp, T., et al. (2007). Colourful Coexistence of Red and Green Picocyanobacteria in Lakes and Seas. Ecol. Lett. 10, 290–298. doi:10.1111/j.1461-0248.2007.01026.x
Ter Braak, C. J. F. (1986). Canonical Correspondence Analysis: a New Eigenvector Technique for Multivariate Direct Gradient Analysis. Ecology 67, 1167–1179. doi:10.2307/1938672
Ter Braak, C. J. F., Cormont, A., and Dray, S. (2012). Improved Testing of Species Traits-Environment Relationships in the Fourth‐corner Problem. Ecology 93, 1525–1526. doi:10.1890/12-0126.1
Thomas, M. K., Kremer, C. T., Klausmeier, C. A., and Litchman, E. (2012). A Global Pattern of Thermal Adaptation in Marine Phytoplankton. Science 338 (6110), 1085–1088. doi:10.1126/science.1224836
Thomaz, S. M., Pagioro, T. A., Bini, L. M., Roberto, M. C., and Rocha, R. R. A. (2004). “Limnological Characterization of the Aquatic Environments and the Influence of Hydrometric Levels,” in The Upper Paraná River and its Floodplain: Physical Aspects, Ecology and Conservation. Editors S. M. Thomaz, A. A. Agostinho, and N. S. Hann (Leiden: Backhuys), 75–102.
Tilman, D. (2004). Niche Tradeoffs, Neutrality, and Community Structure: A Stochastic Theory of Resource Competition, Invasion, and Community Assembly. Proc. Natl. Acad. Sci. U.S.A. 101, 10854–10861. doi:10.1073/pnas.0403458101
Tilman, D., and Pacala, S. (1993). “The Maintenance of Species Richness in Plant Communities,” in Species Diversity in Ecological Communities. Editors R. E. Ricklefs, and D. Schluter (Chicago: University of Chicago Press), 13–25.
Train, S., and Rodrigues, L. C. (1997). Temporal Fluctuations of the Phytoplankton Community of the Baía River in the Upper Paraná River Floodplain, Mato Grosso Do Sul, Brazil. Hydrobiologia 361, 125–134. doi:10.1023/A:1003118200157
Tundisi, J. G., Santos, M. A., and Menezes, C. F. S. (2005). Tucurui Reservoir: Experience and Lessons Learned Brief. (World Lake Basin Management Initiative 1–20.). Available at: www.worldlakes.org/uploads/26_Tucurui_Reservoir_27February2006.pdf.
Uhelinger, V. (1964). Étude statistique des méthodes de dénombrement planctonique. Arch. Des. Sci. 17, 121–123.
Utermöhl, H. (1958). Zur Vervollkommnung der quantitativen Phytoplankton-Methodik. SIL Commun. 1953-1996 9, 1–38. doi:10.1080/05384680.1958.11904091
Vallet, J., Daniel, H., Beaujouan, V., Rozé, F., and Pavoine, S. (2010). Using Biological Traits to Assess How Urbanization Filters Plant Species of Small Woodlands. Appl. Veg. Sci. 13, 412–424. doi:10.1111/j.1654-109X.2010.01087.x
Vanni, M. J. (1987). Effects of Nutrients and Zooplankton Size on the Structure of a Phytoplankton Community. Ecology 68, 624–635. doi:10.2307/1938467
Villéger, S., Miranda, J. R., Hernández, D. F., and Mouillot, D. (2010). Contrasting Changes in Taxonomic vs. Functional Diversity of Tropical Fish Communities after Habitat Degradation. Ecol. Appl. 20, 1512–1522. doi:10.1890/09-1310.1
Violle, C., Navas, M.-L., Vile, D., Kazakou, E., Fortunel, C., Hummel, I., et al. (2007). Let the Concept of Trait Be Functional!. Oikos 116, 882–892. doi:10.1111/j.0030-1299.2007.15559.x
Vogt, R. J., Beisner, B. E., and Prairie, Y. T. (2010). Functional Diversity Is Positively Associated with Biomass for Lake Diatoms. Freshw. Biol. 55, 1636–1646. doi:10.1111/j.1365-2427.2010.02397.x
Wang, H., Zhao, D., Chen, L., Giesy, J. P., Zhang, W., Yuan, C., et al. (2020). Light, but Not Nutrients, Drives Seasonal Congruence of Taxonomic and Functional Diversity of Phytoplankton in a Eutrophic Highland Lake in China. Front. Plant Sci. 11, 179. doi:10.3389/fpls.2020.00179
Weithoff, G., Rocha, M. R., and Gaedke, U. (2015). Comparing Seasonal Dynamics of Functional and Taxonomic Diversity Reveals the Driving Forces Underlying Phytoplankton Community Structure. Freshw. Biol. 60, 758–767. doi:10.1111/fwb.12527
Weithoff, G. (2003). The Concepts of 'plant Functional Types' and 'functional Diversity' in Lake Phytoplankton - a New Understanding of Phytoplankton Ecology? Freshw. Biol. 48, 1669–1675. doi:10.1046/j.1365-2427.2003.01116.x
Westoby, M., and Wright, I. J. (2006). Land-plant Ecology on the Basis of Functional Traits. Trends Ecol. Evol. 21, 261–268. doi:10.1016/j.tree.2006.02.004
Weyhenmeyer, G. A., Peter, H., and Willén, E. (2013). Shifts in Phytoplankton Species Richness and Biomass along a Latitudinal Gradient - Consequences for Relationships between Biodiversity and Ecosystem Functioning. Freshw. Biol. 58, 612–623. doi:10.1111/j.1365-2427.2012.02779.x
Worm, B., Lotze, H. K., Hillebrand, H., and Sommer, U. (2002). Consumer versus Resource Control of Species Diversity and Ecosystem Functioning. Nature 417, 848–851. doi:10.1038/nature00830
Keywords: rao quadratic entropy, functional redundancy, species richness, functional traits, freshwater phytoplankton
Citation: Santos JBO, Brasil J and Huszar VLM (2022) Responses of Functional and Taxonomic Phytoplankton Diversity to Environmental Gradients in Subtropical and Tropical Reservoirs. Front. Environ. Sci. 10:899571. doi: 10.3389/fenvs.2022.899571
Received: 18 March 2022; Accepted: 03 June 2022;
Published: 22 July 2022.
Edited by:
Juliana Dias, Federal University of Rio Grande do Norte, BrazilReviewed by:
Luciane Oliveira Crossetti, Federal University of Rio Grande do Sul, BrazilYongjiu Cai, Nanjing Institute of Geography and Limnology (CAS), China
Copyright © 2022 Santos, Brasil and Huszar. This is an open-access article distributed under the terms of the Creative Commons Attribution License (CC BY). The use, distribution or reproduction in other forums is permitted, provided the original author(s) and the copyright owner(s) are credited and that the original publication in this journal is cited, in accordance with accepted academic practice. No use, distribution or reproduction is permitted which does not comply with these terms.
*Correspondence: Juliana B. O. Santos, anVsaWFuYWJvc2FudG9zQGdtYWlsLmNvbQ==