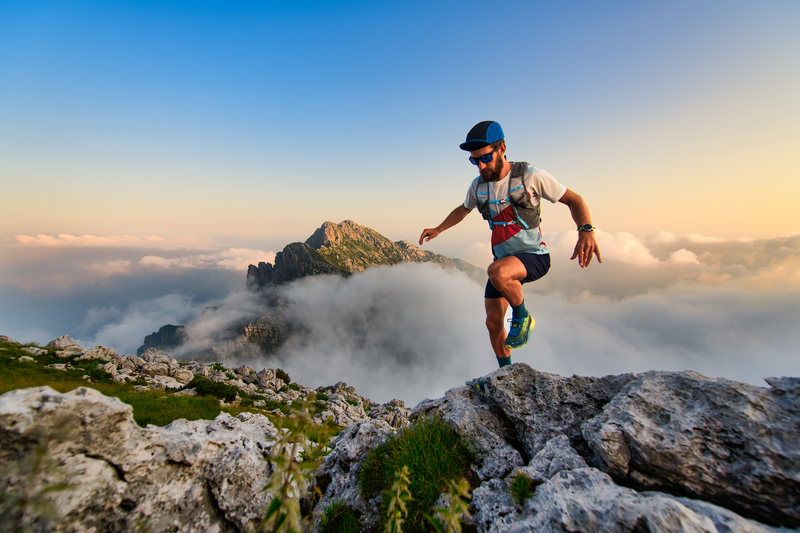
95% of researchers rate our articles as excellent or good
Learn more about the work of our research integrity team to safeguard the quality of each article we publish.
Find out more
ORIGINAL RESEARCH article
Front. Environ. Sci. , 27 April 2022
Sec. Environmental Economics and Management
Volume 10 - 2022 | https://doi.org/10.3389/fenvs.2022.894328
This article is part of the Research Topic Land Governance, Integrated Socio-Ecosystem and Sustainable Development View all 33 articles
On the basis of a production function, an economic model consisting of three regions and two departments (resources and conventional departments) is constructed to explore the effect of natural resources on economic development and their spatial correlation. Then, we construct resource abundance and resource dependence indicators. Based on panel data of 30 provinces in China from 2004 to 2020, a spatial Durbin model is used to empirically test the theoretical hypothesis. The results demonstrate that the resource curse exists objectively, and its root lies in resource dependence; increased dependence on resource industries in a region can improve the economic situation of its surrounding regions, and the spatial spillover effect demonstrates obvious nearby features. The marginal measurement result of spatial spillover indicates that the economic effects of local resource dependence on surrounding areas peaks at 250 km. Further categorization discussions confirm that the resource curse in China is mainly the energy curse.
Conventional economics posits that abundant natural resources can provide a strong impetus for economic development. During the Industrial Revolution, the rapid rise of veteran capitalist countries such as the United Kingdom and the United States depended to a certain degree on the dividends of natural resources (Wright, 1990; Sachs and Warner, 2001; David and Wright, 2002). However, since the mid-20th century, the economies of many resource-rich countries have been gradually declining, and countries such as Iran have even experienced negative growth in GDP t (gross domestic produc). By contrast, some countries with relatively few resources have exhibited surprising growth rates. For example, the economies of Japan, Singapore, and Hong Kong have achieved remarkable results. Current empirical data have reversed attitudes toward natural resources, and scholars have begun to reexamine the essential link between natural resources and economic growth.
With vast territory, China’s resource endowments vary greatly by region, and its economic development is not well balanced. Western China is rich in energy reserves but is becoming increasingly poor. In the past 10 years, apart from Inner Mongolia and Chongqing, the per capita GDP of the western provinces did not reach the national average, and the phenomenon of “rich poverty” was obvious. The southern region has a dense water network, but relatively abundant water resources have not hindered its economic development. The prosperity of the Yangtze River Delta and the Pearl River Delta regions is obvious. From this perspective, both the resource curse and the resource gospel appear to be confirmed in China. The reexamination of the resource curse proposition still has practical implications.
With the advancement of resource allocation projects such as the West–East Gas Pipeline and South–North Water Transfer, the liquidity of natural resources has been greatly enhanced, and the influence of resources on the economy is no longer tied to region. Throughout China, the distribution of resource industries has a clear agglomeration effect, and economic development has spatial dependence (Huajun and Zai, 2014). In this context, investigating the spatial spillover effects of natural resources on the economy is imperative. What is the effect of Chinese natural resources on economic development? What is the spatial correlation characteristic of this effect?
On the basis of the apparent divergence between the degree of resource abundance and the level of economic development, Auty proposed the resource curse hypothesis, arguing that abundant natural resources reduces the competitiveness of the agricultural and manufacturing sectors, which leads to the stagnation of their long-term growth (Auty, 1993). Subsequent researchers have conducted empirical analyses on the relationship between natural resources and economic growth at the transnational、interregional and microcosmic levels, demonstrating the existence of the resource curse effect (Badeeb et al., 2017; Jiang et al., 2021; Shuai et al., 2021). They explain its causes in terms of trade, government rent-seeking corruption, and the effect of the Dutch disease (Corden and Neary, 1982; Auty, 2001; Adams et al., 2019). Simultaneously, the voice of doubt is endless. Opponents question the measurement of natural resources or refute the universality of the resource curse with the success of resource-oriented economies (Larsen, 2006; Brunnschweiler, 2008). The academic community has not been able to reach a consensus on whether natural resources are a curse or a blessing.
As related research continues to advance, the work of scholars is gradually coming to be no longer limited to confirming or denying the resource curse proposition, and they have begun to realize the heterogeneity of time and region between natural resources and economic development. On the basis of questions regarding natural resource measurement, Feng et al. analyzed the concepts of “point” and “scattered” resources and argued that mineral resources are only part of natural resources and the resource curses demonstrated in past studies are essentially energy curses (Zongxian et al., 2010). Shao and Yang clearly defined the abundance of resources and the degree of resource dependence (Shuai and Lili, 2018). They argued that abundant natural resources by themselves are conducive to economic development and the resource curse stems from excessive dependence on the resource industry. Following this thought, Li and Xu discussed the relationship between the abundance of resources and the development of green economy further (Jianglong and Bin, 2018). These studies have shed light on why countries such as the United Kingdom and the United States have been able to quickly accumulate capital from abundant natural resources to grow their economies, whereas the members of the Organization of Petroleum Exporting Countries (OPEC) are mired in a resource curse. The United Kingdom and United States simultaneously have rich mineral and green resources and do not use resource-based industries as a pillar of their national economies, whereas the OPEC countries are the opposite. By contrast, an increasing number of scholars argue that the effect of natural resources on the economy is variable and actively seek ways to transform the resource curse into a resource gospel. By examining the threshold effect of social capital and technological innovation, Wan and Wang concluded that the accumulation of social capital can weaken the crowding effect of resource development on technological innovation, thus breaking the resource curse (Jianxiang and Shouyang, 2016). Wang et al. believe that the most important reasons for the curse of resources are unreasonable mining, allocation distortion effects of natural rents, and crowding out effects, and the key to realizing the gospel of resources is to adopt a prudent and transparent management method for the mining industry. (Yanli et al., 2021). Zhang et al. attribute the difference between resource curse and resource gospel to the life cycle of resource-based cities (Zilong et al., 2021). These studies have effectively explained that the same economy presents perspectives of the resource curse and resource gospel in different sample ranges.
The aforementioned studies have implied a default condition that natural resources have an effect on only the local economy. Natural resources have characteristics that are different from those of capital and labor. In the era of underdeveloped transportation, natural resources are relatively difficult to transport across regions. In addition, most of the neighboring areas have similar resource endowments, and thus the resource characteristics reflected in the economic development of a region have often been attributed to the local resource situation.1 The research of Song and Chen, which was conducted at the empirical level, also proves that the natural resource endowment in adjacent areas does not have a significant effect on local economic efficiency (Ying and Jiping, 2014). However, we must acknowledge the spatial spillover effect of the resource curse (resource gospel). In fact, spatial spillovers are crucial in regional economic development, and resource industries have obvious spatial agglomeration (Wenqing, 2012). The long-term development of economies is likely linked to the resource endowment situation in surrounding areas (Weiya et al., 2016). Fang et al. found that the richness of natural resources of a city has a positive spillover effect on the economic development of other cities in the province by adding provincial-level dummy variables that reflect natural resource abundance (Ying et al., 2011). Xue et al. used the spatial agglomeration degree of the resource industry to replace the resource dependence index, and found that there is an “inverted U-shaped” relationship between the spatial dependence of the resource industry and economic growth (Weiya et al., 2019).
The discussion of the economic effect of natural resources on surrounding areas remains in its infancy. The relevant research is nonexistent or involves only mechanism analysis at the theoretical level, and mathematical models supporting the hypothesis are lacking. Empirical analysis is also limited to investigating resource endowment status, and the spatial correlation model is extremely simple. The first law of geography states that everything is related to everything else, but the connections between nearer things are much closer (Tobler, 1970). According to this, effectively investigating the spatial dependence of natural resources’ economic benefits by including the resource status of neighbors is impossible. Yang (Lili et al., 2014) applied a spatial measurement method to the resource curse for the first time by using spatial lag and error models to analyze the effect of resource dependence on Chinese provinces’ economic development and demonstrated the positive spatial dependence among provincial economies. Qin and Guo focused on the internal situation of Shanxi Province and analyzed the representation of the resource curse effect of regional spatial structure from the perspectives of spatial differentiation and spatial connection (Zhiqin and Wenjiong, 2016). Although these studies are either limited to one province or focus on economic growth itself (rather than the spatial spillover effect of natural resources on economic growth), it demonstrated the necessity of examining resource curse propositions from a spatial economic perspective.
Current research on the resource curse has the following characteristics: First, the spatial spillover effect of natural resources on economic growth has been neglected by scholars. The few related studies are empirical analyses and are limited to adjacent areas. No systematic investigations of the resource curse involving spatial association have used appropriate measurement models, and even fewer have used mathematical models. Second, by drawing from Cerny and Filer (Cerny and Filer, 2007), the academic community has gradually recognized resource dependence as the core explanatory variable with which to verify the resource curse proposition. However, resource storage is difficult to measure and should not be used as a measure of resource status. This does not imply that resource storage has no effect on economic growth. Removing it from the model is too arbitrary. Finally, although the academic community has recognized that natural resources are not limited to mineral resources, resource dependence differs from energy dependence, and studies have indicated that “distributed resources” such as land also affect the economy (Birdsall et al., 2000), Some scholars have empirically proved the relationship between arable land resources and economic growth (Zhigang, 2018). However, most of the research, on the basis of data availability, still insists on using the relevant indicators of the mining industry to measure overall resource industry dependence while ignoring the prominence of green resources.
In view of this, this paper innovates mainly in the following aspects: First, at the theoretical level, this paper starts with the most basic production function, considers the development of natural resources to be a relatively independent department, and builds three regions and two departments (product departments). The resource sector’s economic growth model clarifies the effect of natural resources on the economic development of the surrounding areas. Second, at the empirical level, this paper introduces a spatial weight matrix and examines the resource curse hypothesis from the perspective of spatial correlation. Third, we consider mineral resources and green resources while measuring resource dependence; construct a comprehensive index involving the mining, agriculture, forestry, animal husbandry, and fishery industries; improve the scientific and systematic nature of indicators; and measure energy extraction to measure resource storage.
Assume that the whole society produces only one product Y, with A for technical level, K for physical capital, L for labor, H for human capital, N for natural resource storage, and technical level for exogeneity. We can then use the production function described as follows to describe the state of input and output of the whole society:
When this model incorporates natural resources, they are simply regarded as a factor of production. In other words, natural resources are beneficial, and they can enter the production process without investments in physical capital or labor for development and processing. However, the research in this paper focuses on the effect of natural resources on economic development, and adopting this oversimplified setting is unreasonable. Therefore, we draw from the ideas of Wan and Wang (Jianxiang and Shouyang, 2016) to establish a relatively independent resource department. The resource department is engaged in only natural resource development and use and has no technological progress independent of the product department. We let R represent its output, and the department’s production function can be expressed as
In Eq. 2, we let KR, LR, and HR represent the amount of material capital, labor, and human capital used in the resource sector, respectively, and N still represents the storage of natural resources. Similarly, using KY, LY, and HY to represent the amount of material capital, labor, and human capital used in the product sector and combining Eq. 2 with Eq. 1, a new production function can be obtained:
To differentiate Eq. 3, the superscript “^” indicates the rate of change, and ρ indicates the elasticity:
By simplifying Eq. 4, we can obtain
To simplify the analysis, regardless of special situations such as labor surplus and labor inertia, thus reducing the output level, that is, if the increase of any factor input leads to an increase in output, then the correlation coefficient ρ is always greater than zero. Therefore, we can obtain
By using Eq. 6, we analyze the two different values of the subscript n. Abundant natural resource storage, as with increased physical capital, labor, intellectual capital, and technological progress, plays a positive role in economic development. However, the effect of increased input of physical capital, labor, and human capital in the resource sector on the economy is uncertain. Investing more material capital, labor, or human capital in the resource sector certainly increases the output of the sector, but it will exhaust the input of the corresponding elements in the production sector. Because knowing the exact values of the correlation coefficients is impossible, determining how the output changes under the superposition of the two effects requires further research. In light of this analysis, we postulate Hypothesis 1:
Hypothesis 1. : Abundant natural resources cannot hinder economic development. If the resource curse exists, then the curse should be attributed to excessive dependence on the resource industry.This inference effectively explains why some countries in regions with abundant natural resources can seize the fact that the resource dividend can be used to inform development, while some countries will fall into the quagmire of resource curse. The fundamental reason lies in whether the region takes natural resources as a factor of production to participate in the entire social production, or regards resource exploitation as a pillar industry of the national economy, and invests a large amount of material and human capital in it, so as to crowd out other high-efficiency industries’ industry resources.The above discussion was conducted within the framework of a closed economy. To enhance the effect of the resource curse or gospel, we draw upon the ideas of Levinson and Taylor (Levinson and Taylor, 2008) and construct a three-region partial equilibrium model. According to H1, the following analysis only addresses the dependence of the resource industry and does not consider the problem of changes in the amount of original natural resources.Assume that the country has only three regions (disregarding cross-border trade), which are represented by a, b, and c, and the prices of production factors are given exogenously and consistently. In the initial closed state, region a holds abundant natural resource reserves and developed resource industries, whereas regions b and c have no natural resource storage, and their resource sectors have not yet developed.First, assume that regions b and c are homogeneous. For these regions, under the premise of the previous hypothesis, R produced by the resource department accounts for a small portion of the production sector input. Increasing the input of this factor leads to a large increase in the marginal output. Therefore, on the basis of considerable marginal compensation, regions b and c increase the input of R.Increasing the input of R can occur by two means. One is importing natural resources N to the country and then processing them,2 and the other is importing products from the resource sector directly. We let p represent the price, and
In Eq. 7, i represents the region type, and
Hypothesis 2. : Regardless of whether a region has a resource curse or a resource gospel, a spatial spillover effect occurs. Resource abundance is not only closely related to the local economic situation but also affects resource industry dependence in the surrounding area, in turn affecting its economic development level.Next, we refer to the study of Shen et al. (Kunrong et al., 2017) and relax the homogeneity hypothesis of regions b and c. Region b is assumed to be closer to region a than region c is. The difference in geographical distance directly affects the transportation of unprocessed natural resources. For the convenience of discussion, we use extremes for this distance difference:
Because the resource departments in regions b and c are not developed at the initial stage, even if trade is vigorously developed after opening up, it is backward compared with region a, relatively. The labor and material resources required to produce the finished product R of one resource department unit are greater than those in region a, indicating that
Therefore, the selection of means of increasing the input of R in regions b and c depends on the transportation cost. The specific judgment conditions are as follows:
By simultaneously considering Eqs 8 and 9, and 10, we can conclude that
In accordance with Eq. 11, region b, which is closer to region a, elects to import the finished products of the resource department directly, whereas region c is more inclined to import natural resources for processing. This demonstrates that a large resource endowment in a certain place reduces the dependence of the surrounding area on the resource industry while expanding the local resource industry. Areas closer to the abundant resources enjoy the “revenue” of the resource curse to an extent (the “cost” of the resource gospel), and the areas farther away cannot profit (can be spared). On this basis, we can formulate the third hypothesis:
Hypothesis 3. : Because of the difference in transportation costs, the spatial spillover effect of natural resources on economic development presents a negative and near-trend characteristic.
Although the resource curse is concentrated in the energy sector, studies have shown that excessive dependence on green resources (such as forest resources) also has an adverse effect on the economy. Therefore, we use the status of the mining industry and agriculture, forestry, animal husbandry, and to measure mineral and green resources. By using Eq. 5 in the theoretical model, the specific indicators are determined as the proportion of employment in the resource industry to the total number of employed persons, the proportion of fixed asset investment in resource industries to the total stock of fixed asset investment in the whole society, and the ratio of human capital in resource industries to the total human capital stock. Resource industries include the mining, agriculture, forestry, animal husbandry, and fishery industries. These subindicators are used to construct a comprehensive indicator with which to comprehensively examine the degree of dependence of the economy on the resource industry.3 The calculation formula is
In this formula, xi is the indicator, wi is the weight, and the index i is the indicator category. We use principal component analysis to calculate the weight of each indicator. Before calculating the weight, we perform nonclass quantization processing on the data and convert all of them into values between 0 and 100. The formula is
The steps of weight calculation are as follows: First, we calculate the variance contribution rate (varn), the eigenvalue (λn), and the load factor (Ain). The subscripts n and i represent the categories of the principal components and indicators, respectively. The calculation results demonstrate that the cumulative variance contribution rate of the first five principal components is greater than 95% and can replace the original five indicators. Second, we calculate the coefficients (βin) of each index in the linear combination of the four principal components. Third, we calculate coefficients (γi) in the comprehensive score model. Finally, γi is normalized to obtain the weights. The specific calculation formula is
In addition, because the cumulative variance contribution rate of the first four principal components exceeds 90% and can reflect the status of each indicator, resource dependence is calculated using the weights obtained using the first four principal components (using the same calculation method) as an indicator of the robustness test, expressed as R2. According to H3, the signs of R and WR are predicted to be opposite. Some calculation results are shown in Table 1.
TABLE 1. Calculation results of resource dependence degree of each province in China from 2004 to 2020.
From Table 1, we can easily capture the spatiotemporal heterogeneity of the dependence status of China’s resource industries. From the perspective of time, in the early years, China’s economic growth was still largely at the expense of resource consumption (including mineral resources and green resources), and resource dependence gradually increased and reached its peak around 2008. Since then, with the increasingly serious environmental and social problems derived from long-term high-load economic growth, extensive economic growth methods have been banned. Under the guidance of the concept of sustainable development, the dependence of economic development on the resource industry has been continuously weakened. From a spatial point of view, Beijing, Shanghai, Guangdong and other places all maintain a reduced degree of resource dependence, while the northeastern and western regions with relatively lagging development show more obvious resource dependence, which confirms the resource curse from the level of economic facts. the existence of a hypothesis.
The review of the previous literature review and the analysis of theoretical models both show that although resource storage is not suitable for testing the resource curse hypothesis, it is still one of the influencing factors of economic growth. In accordance with the definition of resource abundance, we should measure natural resource storage. However, natural resource storage is difficult to measure before it is fully developed. Because energy is a representative natural resource, and its production volume is closely related to its storage capacity, we use energy production to measure the resource abundance. For a unified caliber, all energy is converted to standard coal. Relevant data were obtained from the China Energy Statistics Yearbook. On the basis of Eq. 6, this variable is predicted to be positive.
Figures 1, 2 show the resource dependence and energy extraction of each province in China during the sample period, respectively. By comparison, it can be found that the resource dependence and resource endowment of a place do not completely match. Shanxi, Inner Mongolia and other places with the most abundant natural resources also have a high degree of resource dependence, but they are still significantly lower than Heilongjiang, Xinjiang and other provinces; difference. This feature justifies the necessity of incorporating both resource dependence and resource abundance indicators into subsequent measurement models.
On the basis of the theoretical analysis, we research the effect of natural resources on economic growth and its spatial spillover effects to test H1–H3 empirically. On the basis of H1 and Eq. 5, the following spatial Durbin model is created:
In Eq. 12, the subscripts i and t represent province and time, respectively. The level of economic development (gdp) is the explanatory variable, and R and WR are the core explanatory variables, which indicate the resource dependence of local and neighboring countries, respectively. We use these variables to examine the effect of natural resources on economic development and test H2 and H3. Technical level (tech), fixed asset investment stock (k), employment number (L), human capital stock (hc), and energy production (N) correspond to the technology, material capital, labor, human capital, and natural resource storage described in Eq. 5, respectively. The theoretical model discusses inter-regional trade (rather than cross-border trade), and the analysis is carried out under laissez-faire conditions without considering government factors. However, previous research experience and objective facts show that opening-up and policy institutions There is an important impact on the relationship between resource dependence and economic development. Therefore, based on the theoretical model, we have added openness (open) and government intervention (g) to measure openness and fiscal policies, respectively. Moreover, eit is a random disturbance term, interprovincial effect (ui) controls individual difference factors, and time effect (λi) controls time factors. To balance the reduction of heteroscedasticity and the maintenance of data difference, we perform logarithmization on the absolute number variable, and the relative number variable maintains the original form.
In this paper, we select panel data from 30 provinces in China (excluding Tibet, Hong Kong, Macao, and Taiwan) from 2004 to 2020 as the empirical research sample. The data without special instructions are from the China Statistical Yearbook and the National Bureau of Statistics. Indicator selection and measurement details are described as follows:
(1) Economic development level (gdp). We select GDP to measure the economic development level. As an indicator of the stability test, we also use per capita GDP to indicate the economic development level, expressed as gdp2. To eliminate the effect of price factors, we use the consumer price index to reduce the two (2004 constant price).
(2) Spatial weight matrix (W). We select the spatial weight matrix on the basis of the reciprocal of geographical distance—the shorter the geographical distance is, the closer the correlation between the correlations is and the greater the weight in the spatial weight matrix is. Because a robustness test is an indicator, we also use different methods (based on the square of the geographic distance derivative) to measure the spatial weight matrix, expressed as W2, both of which are line normalized.
(3) Technology level (tech). On the basis of the theoretical model, energy efficiency is used to measure the technical level.4 The calculation formula is GDP/standard coal consumption. The direction is predicted to be positive. The conversion coefficient of standard coal for all types of energy is adopted from the China Energy Statistical Yearbook.
(4) Fixed capital stock (k). We use the perpetual inventory method to estimate the stock of research and development capital. The formula is
(5) Number of employed people (L). Compared with the population indicator, the number of employed people can more accurately reflect the status of labor. Data on the number of employed people were obtained from the China Labor Statistics Yearbook. On the basis of Eq. 6, this variable is predicted to be positive.
(6) Human capital stock (hc). In this paper, years of education (no schooling, elementary school, junior high school, high school, and college or above) are set to 0, 6, 9, 12, and 16 years, respectively. The formula is primary school proportion × 6 + junior high school proportion × 9 + high school proportion × 12 + college or above proportion × 16. On the basis of Eq. 6, this variable is predicted to be positive.
(7) The level of openness to the outside world (open). This article uses import and export trade volume to indicate openness level. The relevant data were converted from US dollars to renminbi by using the exchange rate (annual average price) of each year.
(8) Degree of government intervention (g). Drawing upon the idea of Ding and Deng (Junhong and Kebing, 2007), we use the proportion of fiscal expenditures deducting science and education expenditures as a percentage of GDP to measure the degree of government intervention in the economy. Table 2 presents detailed information of all variables.
In China, economic growth exhibits obvious spatial dependence, and provinces with geographical distances tend to have similar development levels. Based on this fact a nd related research (Ying et al., 2011), we postulate that economic development level has a positive spatial autocorrelation. To confirm this inference, we use the Moran index to test the global autocorrelation of the interpreted variables.5
In Table 3, between 2004 and 2020, the Moran index of the global spatial autocorrelation of the two explained variables rejected the null hypothesis of spatial autocorrelation. Therefore, we can conclude that economic development among provinces has spatial dependence. In addition, the per capita GDP indicators have more obvious and extensive spatial dependence characteristics than the overall indicators do.
On the basis of the previous analysis, we modify the measurement model established previously in the paper and combine the spatial autoregressive model with the spatial Durbin model to construct the following generalized spatial Durbin model:
Because the random disturbances of different periods in different provinces may be autocorrelated, we select the clustering robust standard error (rather than the common standard error) for regression. In addition, we select a spatial Durbin model that includes both individual and time effects; hence, the year dummy variables are no longer required. The regression results are presented in Table 4.
Resource industry dependence has a significant negative effect on economic growth, whereas the economic effect of energy extraction is the exact opposite. This indicates that abundant natural resources not only hinder economic development but also promote economic growth to an extent, in turn creating resource dividends. However, if a place is relatively resource rich, it invests too many production factors in the resource industry, leading to resource industry dominance, which significantly limits economic growth. This conclusion is consistent with the views of Shao and Yang (Shuai and Lili, 2018) and effectively confirms H1. In China, resource curses exist, but their roots lie in resource dependence rather than resource endowment.
The spatial lag term coefficient of resource dependence is significantly positive, which is consistent with H2 and H3. If other regions’ resource dependence Sincreases, the local economic situation will improve significantly, indicating that the spatial spillover of the resource curse is negative. However, more distant areas have more advantages in terms of transportation costs and occupy a larger proportion of the spatial weight matrix. Therefore, the positive effect on the local economic situation is greater than that of distant regions, and the negative spatial spillover effect of the resource curse has the closest feature. In addition, the positive spatial autocorrelation of the economic development level is confirmed in the model, and the synergy effect of regional development is significant. This is consistent with China’s development trend this year. Provincial economic growth has begun to rely more on the spillover effects of external regions than on local output (Levinson and Taylor, 2008).
Among the control variables, technical level, fixed capital stock, human capital stock, employment and the regression coefficient of openness level are all significantly positive; that is, technological advancement, the increase of labor, the accumulation of fixed and human capital, and the improvement of openness level can all contribute to economic growth. These conclusions are basically consistent with those of Shuai et al. (2013) and our previous predictions. However, because of differences in sample selection and index construction, these results differ from those of Zhang et al. (Wenqing, 2015). Finally, government intervention is negatively correlated with economic growth, indicating that the current government fiscal intervention is inefficient and cannot play a positive role in the economy for the time being. This conclusion is inconsistent with the views of Song and Yang (Zaixu et al., 2015), which may be caused by the difference in data selection and index measurement. Future research will conduct more in-depth exploration of related issues in this field.
To avoid the adverse effects of endogenous problems on the validity of OSL estimation, we examine the endogeneity of the model. First, regarding the two-way causal relationship, because a higher level of economic development can promote the prosperity of high-technology industries (often nonresource industries) and promote technological progress, this paper argues that economic growth and resource dependence and technology may have a two-way causal relationship. Therefore, we use the Davidson–MacKinnon (DM) test and a generalized method of moments (GMM) distance test (also known as a C test) to examine the endogenous problems that may exist in the model (Mackinnon, 1993; Baum et al., 2003). The first- and second-stage lags of resource dependence and technical level are used as instrumental variables (IV) for IV–GMM estimation, and DM and C-test statistics are calculated. The estimated results of the spatial GMM are displayed in the first column of Table 5. Second, to avoid omitting crucial explanatory variables and measurement errors, we expand the benchmark model. The second column of Table 5 adds a lag in GDP to characterize the possible dynamics of economic development. Specifically, we adopt a differential GMM estimation spatial dynamic panel model with a maximum lag period of 4, an Arellano–Bond test, and a Sargan test. The third column of Table 5 presents the spatial overflow of all control variables, whereas the fourth column only displays the spatial lag term for the control variables with spatial spillover effects. The addition of the spatial lag term facilitates a more comprehensive study of the spatial characteristics of economic development, thereby effectively reducing the potential of set bias in the model.
We conduct a robustness test using the following aspects. First, the spatial lag term is constructed with the spatial weight matrix (W2) based on the square of the geographic distance derivative. The result of the replacement is displayed in the second column of Table 4. Second, per capita GDP (gdp2) and the first four principal components are used in turn. Resource dependence (R2), calculated using the components, replaces the original interpreted variable and the core explanatory variable. The result of the replacement is displayed in the third and fourth columns of Table 4. Finally, 1% of all data are tail finished. Excluding the effect of outliers on the estimation results, the processed regression results are presented in the fifth column of Table 4. The empirical results after the index change and tail-finishing treatment have only slight changes in specific values and significance levels and have not changed the basic conclusions of this article; thus, the regression results are robust.
A placebo test is performed because the previous conclusions may be artificially fixed or affected by missing variables. We construct pseudogeographic distances, pseudoadjacent matrices, and random matrices6 and replace them with the original spatial weight matrices for regression. The regression results are presented in Table 6. If the influence of resource dependence on the surrounding area is not the result of artificial setting, then the regression coefficient should be nonsignificant when using the aforementioned spatial weight matrix that deviates from the geographical law. The placebo test effectively supports the spatial spillover effect of the resource curse and its proximity characteristics.
To further investigate the ubiquity and change trend of resource curse spatial spillover and test the geography law of spatial attenuation, we re-estimate the effect of natural resources on the surrounding economy based on various distance thresholds. Specifically, we start at 100 km and regress every 100 km. The resource dependence coefficient lags in each distance threshold and is significant at the 1% level. Figure 3 illustrates the proximity of the source curse spatial spillover effect.
In Figure 3, the spatial spillover effect of the resource curse fluctuates as geographical distance increases, which essentially conforms to the general law of spatial attenuation and is consistent with the inferences of the theoretical model. The undersmoothing of the curve may occur because this paper is based on available interprovincial (rather than prefecture-level city) data that cannot capture resource associations within provinces. This limitation can be improved in future research. The economic effect of resource dependence on the surrounding area peaked at 250 km and gradually disappears after 700 km. Although resource-abundant places have no incentive to develop resource industries independently, they often develop industries derived from resource development industries in the region, thereby indirectly increasing resource dependence and resource curse space. The role of spillovers in promoting the economy was partially offset (the distance of 250 km is basically no longer an adjacent province). However, areas with long distances are more inclined to develop resource industries locally (rather than directly importing resources and finished products) because of higher transportation costs; thus, enjoying the “revenue” of resource-rich resource curses is increasingly difficult (the 700 km distance in China often spans several provinces).
In China, is the resource curse fully reflected in the energy curse? To answer this question, this paper discusses the heterogeneity of the economic effect of mineral and green resources. We calculate the dependence of mineral resources and green resources in China’s provinces and regress them separately.7 The regression results are presented in Table 7.
TABLE 7. Regression results of Classification discussion and robustness test (Green resources and mineral resources).
The increase in mineral resource dependence hinders economic development, whereas the economic effect of green resource dependence is nonsignificant. We can explain the heterogeneity by combining the previous theoretical model with current development in China. First, regarding the composition of human capital in the mining, agriculture, forestry, animal husbandry, and fishery industries, the average years of education for personnel engaged in the agriculture, forestry, animal husbandry, and fishery industries are below average, whereas those in the mining industry are the opposite. In other words, the development of the agriculture, forestry, animal husbandry, and fishery industries has a relatively small number of highly skilled workers (
The regression results of the spatial lag term show that the impact of mineral resources on the economy has a positive spatial spillover, while the impact of green resources on the economy has a negative spatial spillover. This shows that the negative space overflow of the resource curse at the overall level is actually the result of the two offsetting each other. Whether a place’s resource dependence is a curse or a boon to the economy of the surrounding area depends on whether it depends on mining or the curse of agriculture, forestry, animal husbandry and fishery. As far as the mining industry is concerned, excessive reliance on production and mining in one place will not only create an existence for local economic growth, but also hinder the development of surrounding areas. This may be caused by the particularity of mineral resources. The mining industry is less limited by time, and can mobilize a large amount of capital and labor from the local and surrounding areas for production in a short period of time, thus occupying the resources of other industries in the surrounding areas. However, this high-intensity mining cannot be sustained for a long time, and will generate Structural unemployment and other issues have resulted in idle local and neighboring resources, and ultimately affected economic development.
The situation of agriculture, forestry, animal husbandry and fishery is much more optimistic. If local resource industry dependence is limited to the agriculture, forestry, animal husbandry, and fishery industries (rather than mining), then economic improvement in surrounding areas no longer occurs at the expense of economic losses in the region. We attempt to explain this phenomenon from the perspective of industrial agglomeration to improve resource allocation. Generally, areas in the early stages of vigorous agriculture, forestry, animal husbandry, and fishery industrial development often have relatively abundant green resources. Further agglomeration can provide a more favorable environment for the development of local green resource industries, ameliorate labor outcomes and capital thresholds, and thus improve the efficiency of resource allocation (Shuhan et al., 2016). Correspondingly, the surrounding areas (typically also regions with relatively disadvantaged resource endowments) are more inclined to obtain resource products directly from the resource-rich region (rather than self-developing resource industries) because of the geographical relationship, thereby guiding the inflow of production factors. Currently, the output of each region has increased (or at least not decreased), and cooperation between regions has been achieved.
In addition, in the empirical analysis based on mineral and green resource dependence, the direction and significance of each control variable are consistent with the overall level of investigation, further demonstrating the robustness of the previous regression results.
The previous theoretical analysis has shown that the resource curse originates from the resource industry’s crowding out of fixed capital, labor and human capital in other industries. So, will this crowding be concentrated in a certain dimension? To answer this question, this paper discusses the heterogeneity of the resource curse in the three domains of fixed capital investment, employment and human capital. We calculated the resource dependence of fixed asset investment, employment, and human capital in each province in China (taking the average values of agriculture, forestry, animal husbandry, fishery, and mining) and regressed them respectively. The regression results are shown in Table 8.
TABLE 8. Regression results of Classification discussion. (Fixed Asset Investment, Employment and Human Capital).
Empirical results show that the excessive injection of fixed assets and labor into resource industries will hinder economic development, but human capital will not. This may be because the entry of high-quality talents into the agriculture, forestry, animal husbandry, fishery or mining industries can greatly improve their business models and production efficiency, thereby making up for their adverse effects on the economy. In addition, looking at the spatial lag term, it can be found that there is no spatial overflow of the resource curse in the employment field, that is, the influx of a large number of local labor into the resource industry will not improve the economic conditions of neighboring areas. caused by restrictions.
On the basis of a production function, we construct a partial equilibrium model covering three regions and two departments (resources and conventional departments) and discuss the effect of natural resources on economic development and its spatial characteristics. Abundant resource reserves can boost economic development. The reason for the resource curse is excessive resource dependence. However, the effect of resource abundance on economic status is not confined to the local economy, the development of which affects surrounding areas. Development levels are also closely related, and this spatial spillover effect exhibits a negative near-trend feature.
Subsequently, this paper uses panel data of China’s provinces from 2004 to 2020 to construct a spatial Durbin model and validate the proposed theoretical hypothesis. The study demonstrated the following: First, given the interregional spatial correlation, the resource curse hypothesis remains confirmed in China, and the resource-oriented development model curbs economic development. Second, natural resources have a significant negative spatial spillover effect on economic development. If a local economy is dominated by the resource industry, then the surrounding areas reduce their own resource dependence, thus stimulating economic growth in the region. Third, the spatial association of the resource curse has the nearest feature, which conforms to the universal law of spatial attenuation, and the negative spatial spillover of natural resources to economic development is most pronounced in the area of 250 km. Fourth, China’s current resource curse is embodied in the energy curse. In the field of mineral resources, the resource curse hinders economic development in both the local and surrounding areas. In the green resources industry, industrial agglomeration is conducive to Pareto improvement. In addition, the development of various provinces in China shows obvious spatial dependence, and the economic relations between regions are becoming more and more closely. Among the control variables, government intervention has an adverse impact on GDP, and the level of government intervention needs to be further improved; technical level and other controls The impact of variables on economic development is basically in line with the logic of classical economic growth theory.
Based on the aforementioned research results, we conclude the following: First, resource-driven development is not a long-term strategy. Resource-rich regions should gradually reduce their dependence on resource industries and promote diversified industry development. In addition, we must make full use of local resource advantages, actively use resource endowments to resolve resource dependence, and strive to turn the resource curse into a resource gospel. Moreover, based on the effect of natural resources on the economy and its spatial characteristics, China’s current resource industry (particularly the energy industry) still has a beggar-thy-neighbor phenomenon. To avoid blindly abandoning the resource industry and prevent vicious competition between regions, strengthening economic policy coordination in the provinces, considering the role of the resource industry more rationally, and promoting coordinated development among regions are necessary. Finally, the agglomeration of agriculture, forestry, animal husbandry, and fishery industries can effectively improve resource allocation and overall economic status and deepen spatial agglomeration and improve the efficient use of green resources. Thus, we can achieve a win–win situation for economic and ecological civilization development.
The raw data supporting the conclusion of this article will be made available by the authors, without undue reservation.
XM: establish topics, collect data and materials, build theoretical models, empirical analysis.
The author declares that the research was conducted in the absence of any commercial or financial relationships that could be construed as a potential conflict of interest.
All claims expressed in this article are solely those of the authors and do not necessarily represent those of their affiliated organizations, or those of the publisher, the editors and the reviewers. Any product that may be evaluated in this article, or claim that may be made by its manufacturer, is not guaranteed or endorsed by the publisher.
1Countries such as Saudi Arabia, Iran, and Iraq are close geographically, and their economic development is plagued by abundant oil reserves. However, discussions of the resource curse faced by these countries often involve only the impact mechanism of local natural resources on economic development and ignore the resources of the surrounding areas. Similarly, when studying the role of rich green resources in the United States and Canada in growing the economy, the possibility of neighboring resources as the engine of economic growth is rarely considered.
2Because the threshold of the resource industry is relatively low, here we assume that both regions b and c can independently develop resource industries and need not rely on the assistance of or attract the resource industry of a place to move into the region.
3Because human capital data for the provincial subsectors are unavailable, the allocation of human capital stocks in the provinces is assumed to be homogeneous in the industry. The relevant provincial data are uniformly replaced by the proportion of the national human capital stock in the total human capital stock of the whole society.
4The technical variables in the theoretical model are technical efficiency rather than technical input; hence, using R&D investment to measure the technical level is unsuitable.
5Because the spatial autocorrelation test applies only to cross-sectional data, this paper examines the GDP of each province (per capita). In addition, because the Moran index calculation must use a binary symmetric matrix, the spatial weighting matrix based on the reciprocal of the geographical distance, which was previously standardized by the line, is no longer applicable. To this end, we constructed a 0-1-type spatial weight matrix based on geographical proximity (not standardized).
6Pseudogeographic distance was created by randomly generating the geographical distance of each section and obtaining the reciprocal. The pseudoadjacent matrix was generated by randomly setting the adjacent conditions between provinces (adjacent: 1; not adjacent: 0). The elements of the random matrix are randomly generated natural numbers. This part draws from Deng and Zhao (Huihui and Jialing, 2018).
7The measurement methods of mineral resources and green resource dependence are consistent with the overall resource dependence, involving employment, fixed asset investment, and human capital. The weight of each part is determined by principal component analysis (the first and third columns are the resource dependencies calculated using the first three principal components, and the second and fourth columns are the resource dependencies calculated using the first two principal components).
Adams, D., Adams, K., Ullah, S., and Ullah, F. (2019). Globalisation, Governance, Accountability and the Natural Resource 'Curse': Implications for Socio-Economic Growth of Oil-Rich Developing Countries. Resour. Pol. 61 (2), 128–140. doi:10.1016/j.resourpol.2019.02.009
Badeeb, R. A., Lean, H. H., and Clark, J. (2017). The Evolution of the Natural Resource Curse Thesis: A Critical Literature Survey. Resour. Pol. 51 (5), 123–134. doi:10.1016/j.resourpol.2016.10.015
Baum, C. F., Schaffer, M. E., and Stillman, S. (2003). Instrumental Variables and GMM: Estimation and Testing. Stata J. 3, 1–31. doi:10.1177/1536867x0300300101
Birdsall, N., Pinckney, T., and Sabot, R. (2000). Natural Resources, Human Capital, and Growth. Resource Abundance Econ. Develop. 7, 57–76. doi:10.1093/0199275785.003.0004
Brunnschweiler, C. N. (2008). Cursing the Blessing? Natural Resource Abundance, Institutions, and Economic Growth. Ssrn Electron. J. 36, 99–419. doi:10.1016/j.worlddev.2007.03.004
Cerny, A., and Filer, R. K. (2007). Natural Resources: Are They Really a Curse? SSRN Electron. J. 11, 291–320. doi:10.2139/ssrn.1114352
Corden, W. M., and Neary, J. P. (1982). Booming Sector and De-Industrialisation in a Small Open Economy. Econ. J. 92, 825–848. doi:10.2307/2232670
David, A. P., and Wright, G. (2002). Increasing Returns and the Genesis of American Resource Abundance. Ind. Corporate Change 6, 203–245. doi:10.1093/icc/6.2.203
Huajun, L., and Zai, Y. (2014). Environmental Pollution, Temporal-Spatial Dependence, and Economic Growth. Growth. Monthly Labor Review, 13, 81–91. doi:10.13269/j.cnki.ier.2014.01.009
Huihui, D., and Jialing, Z. P. (2018). Effects in Economic Decision-making of China’s Local Governments. China Ind. Economy 4, 59–78. doi:10.19581/j.cnki.ciejournal.2018.04.004
Jiang, C., Zhang, Y., and Kamran, H. W. (2021). Understanding the Dynamics of the Resource Curse and Financial Development in China? A Novel Evidence Based on QARDL model. Resour. Pol. 72 (12), 342–351. doi:10.1016/j.resourpol.2021.102091
Jianglong, Li., and Bin, X. (2018). Curse or Blessing: How Does Natural Resource Abundance Affect Green Economic Growth in China? Econ. Res. J. 9, 151–167.
Jianxiang, W., and Shouyang, W. (2016). Can Social Capital,Technology Innovation Break Resource Curse:Based on Panel Threshold Effect. Econ. Res. J. 51, 76–89.
Junhong, D., and Kebing, D. (2007). Government Intervene, Natural Resource and Economy Development: Based on Regional Panel Data Study of China. China Ind. Economy 7, 56–64. doi:10.19581/j.cnki.ciejournal.2007.07.007
Kunrong, S., Gang, G., and Xian, F. (2017). Does Environmental Regulation Cause Pollution to Transfer Nearby? Econ. Res. J. 52, 44–59.
Larsen, E. R. (2006). Escaping the Resource Curse and the Dutch Disease? When and Why Norway Caught up with and Forged Ahead of Its Neighbors. Discussion Papersy 65, 605–640. doi:10.1111/j.1536-7150.2006.00476.x
Levinson, A., and Taylor, M. S. (2008). Unmasking the Pollution Haven Effect. Int. Econ. Rev 49, 223–254. doi:10.1111/j.1468-2354.2008.00478.x
Lili, Y., Shuai, S., and Jianhua, C. (2014). The Effects of the Dependence on Resource—Based Industries on Provincial Economic Growth in China and Its Transmission Mechanism: Empirical Analysis Based on Spatial Panel Model. J. Finance Econ. 40, 4–16. doi:10.16538/j.cnki.jfe.2014.03.003
MaCkinnon, G. J. (1993). Estimation and Inference in Econometrics. Ontario: Oxford University Press.
Sachs, J. D., and Warner, A. M. (2001). The Curse of Natural Resources. Eur. Econ. Rev. 45, 827–838. doi:10.1016/s0014-2921(01)00125-8
Shuai, S., Junya, Y., Hai, W., and Lili, Y. (2021). Induced Effect of Resource-Based Industry Dependence on Zombie Firms. Econ. Res. J. 56 (11), 138–154.
Shuai, S., and Lili, Y. (2018). Abundant Natural Resources, Resource Industry Dependence and China's Regional Economic Growth. Manage. World 53 (09), 151–167. doi:10.19744/j.cnki.11-1235/f.2010.09.002
Shuai, S., Meiting, F., and Lili, Y. (2013). How Does Resource Industry Dependence Affect the Efficiency of Economic Development?——Test and explanation of the conditional resource curse hypothesis. Manage. World 2, 32–63. doi:10.19744/j.cnki.11-1235/f.2013.02.005
Shuhan, G., Yingming, Z., and Xin, Z. (2016). Study of Industrial Agglomeration Improving Misallocation of Resources. China Ind. Economy 6, 73–90. doi:10.19581/j.cnki.ciejournal.2016.06.007
Tobler, W. R. (1970). A Computer Movie Simulating Urban Growth in the Detroit Region. Econ. Geogr. 46, 234–240. doi:10.2307/143141
Weiya, X., Jian, Z., and Lexin, Y. (2019). Spatial Agglomeration of Resource Industry, Transmission Factor Extraction and Mediator Effect of Resource Curse. Chin. J. Manage. Sci. 27 (06), 179–190. doi:10.16381/j.cnki.issn1003-207x.2019.06.017
Weiya, X., Zaixu, Z., H ongxun, L., and Junyu, N. (2016). Spatial agglomeration of resource industry and regional economic growth: an empirical study of "resource curse. China Popul. Resour. Environ. 26, 25–33. doi:10.3969/j.issn.1002-2104.2016.08.005
Wenqing, P. (2015). Regional Economic Development in China: Analysis Based on Spatial Spillover Effect. The J. World Economy 38, 120–142.
Wenqing, P. (2012). Regional Linkage and the Spatial Spillover Effects on Regional Economic Growth in China. Econ. Res. J. 47, 54–65.
Wright, G. (1990). The Origins of American Industrial Success, 1879-1940. Am. Econ. Rev. 80, 651–668.
Yanli, W., Jingxuan, X., and Guangrong, M. (2021). Decording the Economic Development of Resource Areas: Mechanism Evolution and Prospects. Economist 44 (09), 109–118. doi:10.16158/j.cnki.51-1312/f.2021.09.013
Ying, F., Kan, G., and Yang, Z. (2011). Does China Exist "Resource Curse"? J. World Economy 34, 144–160. doi:10.16538/j.cnki.jfe.2014.03.003
Ying, S., and Jiping, C. (2014). Government Dominance,Market Segmentation and Resource Curse: A Study on Influence of Natural Resources Endowment to Economic Growth. China Popul. Resour. Environ. 24, 156–162. doi:10.3969/j.issn.1002-2104.2014.09.022
Zaixu, Z., Yawei, X., Zengliang, H., and Ying, M. (2015). An Empirical Study on Resource Curse Effect of China’s Oil and Gas Resources City. China Popul. Resour. Environ. 25, 79–86. doi:10.3969/j.issn.1002-2104.2015.10.011
Zhigang, Z. (2018). Study on the Relationship Between Cultivated Land Resources and Economic Growth ———An Empirical Test Based on the “Resource Curse” Hypothesis. J. Agrotechnical Econ. 37 (06), 127–135. doi:10.13246/j.cnki.jae.2018.06.011
Zhiqin, Q., and Wenjiong, G. (2016). Resource Curse" Effect of Regional Spatial Structure: Empirical Study on Shanxi Province. China Popul. Resour. Environ. 26 (09), 110–115. doi:10.3969/j.issn.1002-2104.2016.09.013
Zilong, Z., Bo, W., Zhi, L., and Yanbi, C. (2021). Fiscal Decentralization, Industrial Upgrading, Technological Progress and“Resource Curse” —Empirical Analysis of Resource-based Cities in the Yellow River Basin. Econ. Surv. 38 (03), 133–141. doi:10.15931/j.cnki.1006-1096.20210409.005
Keywords: energy curse, resource curse, spatial spillover, spatial durbin model, nearby features
Citation: Men X (2022) Spatial Spillover Effects of the Resource Curse: An Empirical Analysis in China. Front. Environ. Sci. 10:894328. doi: 10.3389/fenvs.2022.894328
Received: 11 March 2022; Accepted: 29 March 2022;
Published: 27 April 2022.
Edited by:
Qingsong He, Huazhong University of Science and Technology, ChinaReviewed by:
Qing Wan, Wuhan Institute of Technology, ChinaCopyright © 2022 Men. This is an open-access article distributed under the terms of the Creative Commons Attribution License (CC BY). The use, distribution or reproduction in other forums is permitted, provided the original author(s) and the copyright owner(s) are credited and that the original publication in this journal is cited, in accordance with accepted academic practice. No use, distribution or reproduction is permitted which does not comply with these terms.
*Correspondence: Xiaolu Men, NTY3NjMzNkBxcS5jb20=
Disclaimer: All claims expressed in this article are solely those of the authors and do not necessarily represent those of their affiliated organizations, or those of the publisher, the editors and the reviewers. Any product that may be evaluated in this article or claim that may be made by its manufacturer is not guaranteed or endorsed by the publisher.
Research integrity at Frontiers
Learn more about the work of our research integrity team to safeguard the quality of each article we publish.