- 1CERNA, École des Mines de Paris, Paris, France
- 2CNRS, UMR 8568 CIRED—Centre International de recherche sur l’environnement et le Développement, Paris, France
The study aims at reconciling contrasting productive and environmental goals of agricultural policies at a given budget in the context of climate change. Based on a quantitative bioeconomic model integrating interdependencies between agricultural systems and agroecosystems, we compare the impacts of 4 contrasted public policy scenarios based either on productive (food or energy) or environmental goals (pollution reduction or ecosystem state) on a set of 18 bioeconomic indicators. We run the policy scenarios under two contrasted climate change scenarios to investigate their robustness. We confirm that it is possible to achieve productive and environmental goals with the on-going budget of European agricultural policy. Synergies between productive and environmental performances exist even if they are not trivial nor systematic. More precisely, an agricultural public policy which focuses on energy production might offer a good compromise regarding the different facets of agricultural landscapes. The Pollution scenario constitutes a credible environmentally oriented alternative even if it remains slightly less competitive regarding both ecological and economic sides than an energy-oriented policy. Eventually, our analysis shows that our conclusions are robust to climate change, suggesting that adequate agricultural public policies might attenuate climate change effects when considering intermediary climate change scenarios.
1 Introduction
The degradation of biodiversity constitutes one of the critical global issues for humanity (Convention on Biological Diversity and United Nations Environment Programme, 2014; Loh, 2014; Díaz et al., 2019). Regarding terrestrial ecosystems, land use changes including urbanization, agricultural abandonment or intensification of agricultural landscapes have been identified as the main causes of this erosion in Europe and in France over the last decades (Chamberlain et al., 2000; Donald et al., 2001; Gregory et al., 2017). These changes in land uses lead to a decrease and a degradation of the habitats available for biodiversity, along with their fragmentation (Robinson et al., 1995). Beyond this ecological concern, such an erosion of ecosystems strongly impacts human well-being by interfering with the provision of ecosystem services (MEA, 2005; Costanza et al., 2017; Díaz et al., 2019). Due to these unexpected economic impacts, a debate has emerged about the economic rationale driving changes in land uses.
The European Common Agricultural Policy (CAP) (see Table 1 for the complete list of acronyms) constitutes an interesting case-study for this debate. For 30 years, it has articulated a first set of measures (the “first pillar”) historically dedicated to production with a second set (the “second pillar”) dedicated to rural and environmental development (Swinton et al., 2007). Despite recent developments which tend to include environmental conditions within the first pillar, this dual structure remains at the core of the discussions of CAP (Pe’er et al., 2014; Mouysset, 2014). The concept of ecosystem service brings a new perspective on this duality. Indeed, on one side, the historical economic rationale of agricultural land use changes is related to the provision of ecosystem services, namely food and energy provision (Lowe et al., 2002). On the other side, the negative environmental impacts of land use changes over the last 50 years (such as the impacts on pollinators or pest controls (Bianchi et al., 2006; Ricketts et al., 2008), on carbon and nitrogen cycles (Batlle-Aguilar et al., 2011) and on emblematic species (Tucker and FHeath., 1994; Benton et al., 2002)) involve regulating, supporting and cultural ecosystem services. In this perspective, the challenge of agricultural public policies aiming at articulating productive and environmental objectives consists in providing the whole bundle of ecosystem services (Strijker, 2005; Stoate et al., 2009). To help decision-makers, a literature on ecosystem services has grown significantly in recent years (Bateman et al., 2013; Mouysset et al., 2016; Elalamy et al., 2019; Mouysset et al., 2019). However the identification of a socially acceptable and sustainable trade-off between ecosystem services is still in debate. In such a context, the design of a socially acceptable and sustainable European agricultural public policy remains an open question.
This question takes place in an additional challenging context regarding climate change (Masson-Delmotte et al., 2021). Indeed, the Special Report on Climate Change and Land warns about terrestrial ecosystems and biodiversity vulnerability to climate change and extreme weather conditions (Newbold, 2018; Shukla et al., 2019; Taheri et al., 2021). Climate change directly affects ecosystems. More precisely, the growing pace of isotherms’ displacement leads to a spatial mismatch between bio-climatic niches and available habitat (Devictor et al., 2008) which increases the climatic debt (Kapitza et al., 2021) and the extinction risk (Pearson et al., 2014; Singer et al., 2016). These mismatches emerge from changes in phenology (Gaüzère et al., 2016), habitat quality (Holyoak and Heath, 2016), inter-species interactions (LeBrun et al., 2016; Dalsgaard, 2020) and food ressources (Mantyka-Pringle et al., 2019). Moreover, extreme events during the reproduction period hinder the reproductive success and the survival rate, leading to a population decline (Bellard et al., 2012; Gaüzère et al., 2016).
Beside these direct impacts on ecosystems, climate change creates additional pressures on lands and land use change. More specifically, climate change affects agricultural yields generating an increase or a decrease in land uses depending on the type of crops, the location, the soil quality and the climate conditions (Souty et al., 2013; European Commission Research Centre, Joint et al., 2020). By affecting land use distributions, climate change impacts habitat availability, thus exacerbating existing risks on biodiversity and ecosystem health (Martin et al., 2013; Mantyka-Pringle et al., 2015). In spite of numerous scientific studies, these amplifying mechanisms are still poorly understood (Oliver and Morecroft, 2014). Many interactive effects remain unexpected, generating some “ecological surprises” (Pörtner et al., 2021). Feedback loops, synergistic mechanisms and threshold effects make estimations of the impact of climate change on ecosystems and human well-being imprecise (Elmhagen et al., 2015; Peters et al., 2019).
Despite this complexity, the scientific community agrees that the combined effects of climate change and land-use change are mutually reinforcing (de Chazal and Rounsevell, 2009; Ay et al., 2014; Oliver and Morecroft, 2014). While climate projections for the next few years are already determined due to climate inertia, longer term outcomes given future climate action are less certain. Hence, decision-makers have the opportunity to consider different longer term climate scenarios in designing their agricultural policies (Dunford et al., 2015; Mouchet et al., 2017). It is precisely to reduce or counteract the negative impacts of land use on biodiversity in the context of climate change that Climate and Agri-Environmental Schemes (CAES) have been developed in Europe since 2014. Indeed the IPCC report indicates that maximizing synergies between sustainable and integrated land management on the one hand, and climate change adaptation and mitigation measures on the other, can generate co-benefits for biodiversity, ecosystem services and human well-being (Shukla et al., 2019). In this context, there is an urgent need to explicitly consider different climate change trends when designing agricultural policies.
Interdisciplinary studies based on bioeconomic models integrating complex interdependencies between ecosystems and human society (Jean and Mouysset, 2022) offer the holistic view required to investigate the trade-offs between ecosystem services provided by agricultural landscapes in a context of climate change (Bateman et al., 2013; Oliver and Morecroft, 2014; Mouysset et al., 2019; Rosa et al., 2020). Following this perspective, we developed a quantitative bioeconomic model based on Mouysset et al. (2019), in order to optimize the characterization of productive and environmental objectives of agricultural policies, using synergies between ecosystem services. The bioeconomic model is based on the coupling of an ecological model of bird dynamics with a microeconomic model of land use management. Particular attention is paid to the modeling of ecological dynamics, in agreement with Elmhagen et al. (2015) and Newbold, (2018) who reaffirm the need for mechanistic ecological models. In this study, we investigate 4 contrasted public policy scenarios regarding different productive and environmental goals: food provision, energy provision, pollution decrease and ecosystem preservation. We assess the impact of such policy scenarios on a set of 18 bioeconomic indicators. We evaluate the robustness of these performances in the face of climate change by exploring two contrasted climate change scenarios.
Our study confirms that it is possible to achieve productive and environmental goals with the current European agricultural policy budget. Our study illustrates that synergies between productive and environmental performances do exist even if they are not trivial nor systematic. More precisely, an agricultural public policy which focuses on energy production might offer a compromise regarding the different functions of agricultural landscapes, including productive and environmental ones. The pollution decrease scenario constitutes a credible environment-oriented alternative even if it remains slightly less competitive regarding both ecological and economic sides compared to an energy-oriented policy. Eventually, our analysis shows that our conclusions are robust to different climate change scenarios, suggesting that adequate agricultural public policies might attenuate climate change effects when considering intermediary climate change scenarios.
2 Materials and methods
2.1 The bioeconomic model
2.1.1 The bioeconomic framework
The bioeconomic model presented in Figure 1 is adapted from the bioeconomic framework developed in Mouysset et al. (2019). Similarly, it articulates a national decision maker, who implements national public policies in a context of climate change, with a range of regional planners who optimize their land use distribution, which in turn affect regional birds communities. However, it differs from the initial framework in 3 respects. First, this new model details the agronomic strategies of regional planners: in addition to considering land use distribution, they are now able to choose the level of inputs dedicated to each crop. Second, it integrates feedback impacts from ecosystems on regional planners through ecosystem services affecting the marginal yields of pollination-sensitive crops. In other words, we did not consider biodiversity provisioning per se (as a cultural ecosystem service) but biodiversity provisioning for food production (as a regulating ecosystem service). Third, climate change is not solely taken into consideration in the ecological model but also by the national policy-maker, who investigates different climate scenarios.
2.1.2 The economic model
We consider regional landscapes, each managed by a representative landowner driven by economic rationality. In each period, the regional economic agent determines the surfaces Sr,l dedicated to each land use l and the chemical inputs, namely pesticides Pr,l and fertilizers Fr,l, in order to maximize her regional profit Πr under technical-economic constraints such as in Kapitza et al. (2021). The optimization program in a region r is detailed below.
First, the objective function of the regional land planner is defined as follows:
where
The marginal rent
where pl, cP and cF are respectively the national sale prices for production of land use l, and the costs of pesticides and fertilizers. For the sake of simplicity, these 3 parameters are considered constant over time in this study. The expression ρ(Fr,l(t), Pr,l(t), Er(t), Cr(t)) stands for the agronomic yield function.
Following Souty et al. (2013), the agronomic yields ρ(Pr,l(t), Fr,l(t), Er(t), Cr(t)) are modeled as an increasing function of pesticides and fertilizers. Diminishing yields are approximated by a bounded linear function: the agronomic yields cannot exceed a threshold value for given bioclimatic conditions
with respect to:
Parameters αP,l and αF,l stand for the responses of crops on land use l to pesticides and fertilizers respectively. For the sake of simplicity, we assume that they are constant. Eventually, the range of possible agronomic yields for land use l in region
where
Ecosystems are affected by land uses, pesticides and fertilizers as detailed in the ecological model in Section 2.1.3 thus exhibiting the inter-dependencies between ecological and agro-economic systems.
Second, the maximization of the objective function described in Eq. 1 is subject to 2 technical-economic constraints:
The land availability constraint (Eq. 7) ensures a constant regional area over time. The rigidity constraint (Eq. 8) limits the magnitude of land use change in each period. Similarly to Mouysset et al. (2019), it indirectly accounts for transition costs between two land use classes. As in Mouysset et al. (2019), we do not take into consideration changes in food demand and technological context. Finally, additional technical constraints might regard some specific land uses. In this study, we take two of them in consideration, related to our french case study: the areas of forests and permanent grasslands are bounded by the Natura 2000 network (i.e. a protected area framework in France).
2.1.3 The ecological model
The ecological model is used to predict the overall biodiversity state, proxied by birds population. It is related to a spatially explicit model of metapopulation as in Mouysset et al. (2019). A metapopulation is defined as a network of interconnected subpopulations of a species. Each region r is characterized by a subpopulation driven by two ecological processes: first, the reproduction of individuals within a region, and second, the dispersal between regions. While the dispersion model is strictly identical to Mouysset et al. (2019), innovations are included in the reproduction function due to the effects of pesticides and fertilizers on biodiversity.
The reproduction function (i.e. the intra-regional population dynamics) is modeled using a Verhulst model based on logistic growth:
where Ni,r(t) is the abundance of species i in region r at time t. The parameter ri represents the intrinsic growth rate of species i and is constant for each species at the national scale. The variable Ki,r(t) represents the carrying capacity in region r at time t for species i. The carrying capacity is assumed to be sensitive to land use, inputs and climate variables as follows:
where the parameter
Second, we consider an inter-regional dynamic between connected regions in our spatial framework. Individuals can disperse between two regions according to a national species-specific dispersion rate defined as in Mouysset et al. (2019). We assume the dispersal between two connected regions to be symmetric.
2.2 A case study: Metropolitan France
The bioeconomic model presented in the previous section has been calibrated with a French case study. The territory is divided into 707 small agricultural regions (SARs) exhibiting specific environmental characteristics (land use distributions, input use and climate change), biological states (bird abundance) and economic characteristics (profit per hectare).
2.2.1 Land use and input data
Land uses are defined from the European Environment Agency’s CORINE Land Cover (CLC) project, focusing on metropolitan France for the years 2000, 2006, 2012 and 2018. The CLC project is a biophysical inventory of land use and its evolution, with a detailed nomenclature in 44 categories. According to the methodology developed in Cocco et al. (2021), we aggregated the nomenclature into six main land uses of interest: urban areas, broadleaves forests, coniferous forests, annual crops, perennial crops, and permanent grasslands. Linear extrapolations are used to estimate data in years between 2000, 2006, 2012 and 2018.
This description of land use is then refined using technical and economic data. Annual crops are split into: cereals (excluding maize and rice), maize, oilseeds, protein crops and temporary grasslands. Perennial crops are differentiated between orchards (including olive groves) and vineyards. Information regarding organic versus conventional farming is included for both annual and perennial crops. To do so, we use data from EIDER and Agreste (former and current statistical databases from the French Ministry of Environment) at the department level for the period 2000–2020, and from Eurostat at the NUTS2 level for the 2 years 2013 and 2016. Specific information for forests is taken from sustainable management labels (PEFC or FSC). Agronomic yield and input use (pesticides and fertilizers) data are derived from the Annual Agricultural Statistics of Agreste. Complementary data are obtained from ADEME and FranceAgrimer reports. The ecosystem service function is calibrated with data from numerous works focusing on the impact of pollination on fruits and rapeseed (Klein et al., 2006; Bommarco et al., 2012; Garratt et al., 2014; Lindström et al., 2015; Geslin et al., 2017; Perrot et al., 2019; Kirchweger et al., 2020). Changes in yield due to climate change is taken from the European Joint Research Centre’s technical report1 analyzing the impacts of climate change on European agriculture by 2050 (European Commission Research Centre, Joint et al., 2020).
2.2.2 Economic data
Spatially differentiated economic data are used within our bioeconomic model. Agricultural economic data come from the Annual Agricultural Statistics of Agreste, the European database of Eurostat and the European Farm Accountancy Data Network FADN. Specific additional information regarding forests emerges from the French National Forestry Office (NFO). The rigidity parameters are the same as in Mouysset et al. (2019) when applicable, and the others are set with historical data (time series between 2000 and 2019). Such constraints ensure realistic but flexible dynamics of LUC.
2.2.3 Biodiversity data
Common birds are used as a biodiversity metrics since they exhibit many advantages (Ormerod and Watkinson, 2000; Gregory et al., 2004; Sekercioglu et al., 2004). As predators, they capture many changes in the entire food chain. Moreover, they provide ecosystem services (regulation of invertebrates, rodents and natural pest control agents). Birds are deeply affected by changes in land use proportions and less sensitive to landscape structure. Eventually, their close vicinity to humans makes them a simple and comprehensive example of biodiversity for a large audience of citizens.
Data on bird populations are derived from the French Breeding Bird Survey (FBBS), a monitoring program in which trained ornithological volunteers count birds by visual or auditory contact twice a year on the same plot, following a standardized protocol (Jiguet et al., 2012). The period runs from 2001 to 2019 and the collection is organized by the French National Museum of Natural History. FBBS data have already been used in more than 100 international scientific publications, addressing the impact of global changes on bird communities, and solutions to mitigate these impacts. We consider a large group of species breeding in France based on 60 species, split into 3 functional groups based on their habitat preference (Julliard et al., 2006): 14 generalist species, 23 agricultural specialist species, and 23 forest specialist species. The list of species is available in Mouysset et al. (2019). These common species constitute very abundant populations whose areas of distribution cover the whole French territory. It makes it then possible to conduct our analysis at the national scale.
2.2.4 Climate data
The climate data come from Météo France measurement stations, which are spatially interpolated on a regular 8 km grid using the SAFRAN surface model. We use the average daily temperature and daily rain precipitation data for the 2000–2020 period to create relevant year-SAR-aggregated ecological variables. The forecast data come from DriaslesfutursduclimatⒸ which provides access to the different climate evolutions simulated for the current century in France.
Many explanatory climate variables can be constructed to assess the impact of climate change on biodiversity. Based on a review of the recent literature on the study of the impacts of climate change on the distribution areas of terrestrial biodiversity and the abundance of species, we selected some relevant variables to capture the average climate trend effects and extreme climate events: Mean Annual Temperature, Cumulative Annual Precipitation, Minimum Temperature of the Coldest Month, Maximum temperature of the Warmest Month, Mean Breeding Season Temperature, Cumulative Breeding Season Precipitation, Number of Extreme Hot Days.
2.2.5 Parameters estimation and variable selection
Following the method described in Mouysset et al. (2019) and Cocco et al. (2021), we calibrate the ecological model over a timeline from 2002 to 2018. Bird abundance was described by land use, input and climate variables according to the equations described in the ecological model section. We performed regression based on the least squares method in R-software and estimated the model coefficients for each species i. However since we added new variables compared to Mouysset et al. (2019), our set of land use, input and climate variables may lead all together to an over-specification of the ecological model despite their individual theoretical relevance. Since we aim at using our model to describe current bird population trajectories but also to predict future trends of bird populations, we proceeded to a variable selection by comparing the goodness-of-fit and the predictive power of different sets of explanatory variables.
Following the methodology described in Mouysset et al. (2016) we used the MAPE (Mean Absolute Percentage Error) as a minimizing criterion since it dampens inter-species population size differences. The goodness-of-fit is computed in-sample: the absolute deviation of the predicted values from the actual values is calculated relative to the actual values over the period 2016–2018, for a model calibrated over the period 2002–2018. The predicted values are estimated recursively from the initialization year 2002. The in-sample period is chosen sufficiently distant in time from the initialization year in order to capture the error amplification effects. The predictive power is computed out-sample: the MAPE is calculated on the same period 2016–2018, but for a model calibrated on the period 2002–2015. The initialization year is also 2002. Based on the ols_step_best_subset function in the software R, we developed a new automatic variable selection method adapted to linear panel models, minimizing the MAPEin and MAPEout indicators. The final selection of models depicted among functional groups (namely the generalist, the farmland and forest specialist species) reported in Supplementary Appendix S6.2 and Figure 2 in Supplementary Appendix S6.3 presents some illustrative examples of predicted versus historical bird dynamics.
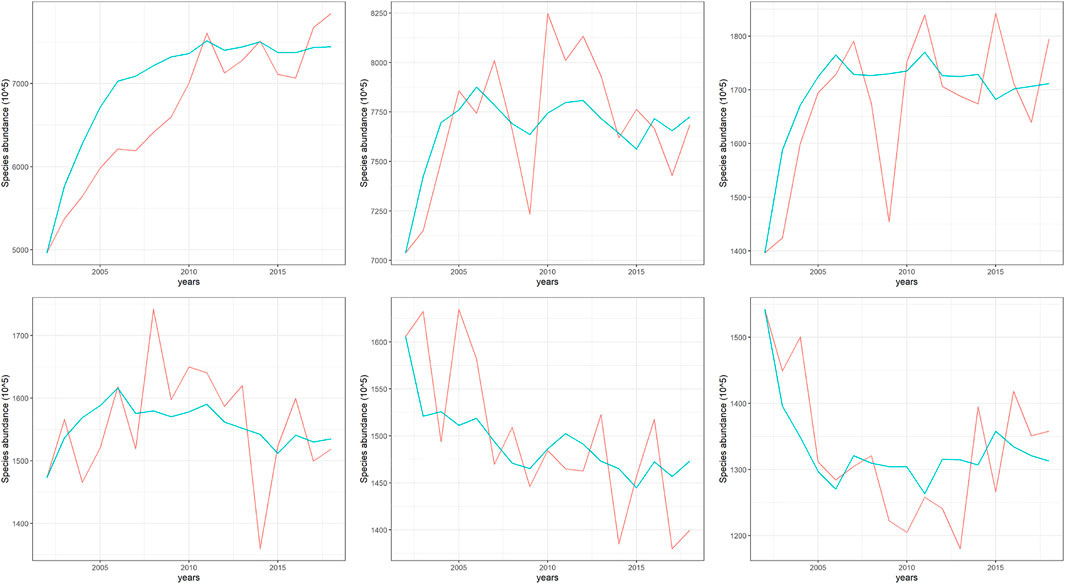
FIGURE 2. Descriptive performance of the predictive ecological model: comparison of predicted bird abundances values (blue line) with historical abundances (red line) for a sample of species. First row: Wood Pigeon Columba palumbus, Chaffinch Fringilla coloebs, Great spotted woodpecker Dendrocopus major, Second row: Green Woodpecker Picus viridis, Dunnock Prunella modularis, Winchat Saxicola torquata.
3 Scenarios and indicators
Using this bioeconomic framework, we assess the economic and ecological performances of agricultural landscapes from 2019 to 2050 according to different public policies and climate change scenarios.
3.1 Public policies scenarios
We investigate 4 contrasted scenarios which are compared to a Business As Usual scenario. The 4 contrasted scenarios follow different objectives, either related to a production objective (food or energy goals) or to an environmental objective (focus on pollution or ecosystem). They are detailed below:
• The “Business As Usual” (BAU) scenario pursues current CAP without any additional changes, meaning that the public incentives are equal to zero: δl = 0 for all land uses l.
• The “Food” scenario follows a food production objective. To do so, it promotes the expansion of intensive annual crops AC (δAC > 0) while temporary grasslands GL_T are taxed (δGL_T < 0) since the high profitability of the latter makes them competitive to food crops expansion.
• The “Energy” scenario promotes energy production from timber and organic biomass, with underlying motivations to decarbonize the energy mix, increase energy independence, reduce vulnerability to international market fluctuations and develop local energy. It is modeled through subsidies dedicated to productive coniferous forests CF and oleaginous biofuel crops Oil (δCF > 0 and δOil > 0).
• The “Pollution” scenario aims at reducing phytosanitary pressure on ecosystems and promotes less intensive management methods for annual AC and perennial PC crops as well as grasslands GL (δAC > 0 if PAC + FAC < T, δPC > 0 if PPC + FPC < T, δGL > 0 if PGL + FGL < T, where T stands for a given threshold).
• The “Nature” scenario aims at preserving biodiversity and ecosystems by expanding permanent grasslands and broadleaves forests semi-natural habitats. The two land uses benefit from subsidies (δGL > 0 and δBF > 0) while temporary grasslands are taxed (δGL_T < 0).
The levels of incentives have been determined in order to obtain a similar inter-annual public budget with the 4 scenarios. It is therefore possible to compare the different scenarios and investigate how to allocate a given public policy envelope to different policy goals. In our study, we chose the overall inter annual envelope of 120 BnEuros, with an average annual budget of 6.5 BnEuros/year. In comparison, nowadays, the French annual CAP budget is 8 BnEuros/year. In our study, we thus investigated a slightly lower budget than the on-going CAP, but we proceeded to a sensitivity analysis with an upper case and an lower case (with higher and lower incentive levels than in the main case).
Table 2 sums up the levels of incentives δl we have tested in our study. Regarding the Pollution scenario, we consider the threshold T to be the first tercile of the pollution rate (sum of phytosanitary products) within the historical data.
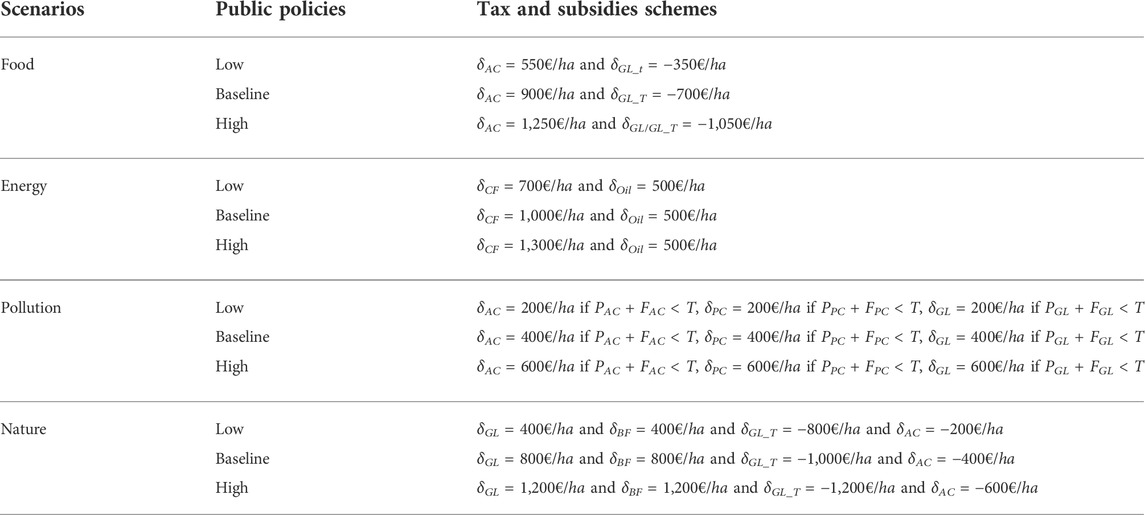
TABLE 2. Detailed description of public incentives for the 4 public policy scenarios: Food, Energy, Pollution and Nature. The baseline scenarios are supplemented with an upper and lower case (i.e. with higher and lower incentive levels than in the main case). Negative incentives are taxes and positive ones subsidies. Only subsidies of the Pollution scenario impose restriction on the use of phytosanitary products. P stand for pesticides, F for fertilizers, AC for annual cultures, Oil for oleaginous crops, GLT for temporary grasslands, GL for permanent grasslands, CF for coniferous forests, BL for broadleaves forests.
3.2 Climate scenarios
We choose two contrasted climate scenarios among the widely used Representative Concentration Pathways (RCP). The RCP4.5 which assumes CO2 concentrations stabilization by the end of the century is our benchmark scenario; the more pessimistic RCP8.5 assumes a growing trajectory of GHG. Data are computed with the model ALADIN 52 from the CNRM3, and we restricted our estimations to the mid-term period 2020–2050. Results are first presented for the climate scenario RCP4.5, then we investigated the robustness of our results to the second climate scenario RCP8.5. Both climate scenarios affect the climate variables that affect bird communities (especially Mean Annual Temperature, Cumulative Annual Precipitations, Maximum Temperature of the warmest month and Cumulative Breeding Season Precipitation) and the ranges of crop yields (ρ0 and ρsup) based on JRC prospective studies.
3.3 Indicators
To assess the impact of public policy scenarios on the landscape, we combined a set of 18 indicators which are merged into 4 classes.
A first group of 4 indicators aims at evaluating the biophysical impacts of scenarios in line with their different objectives, both productive and environmental (these indicators are thus called “target indicators”). In regards with the productive targets, we considered an indicator of food production based on calories produced and an indicator of energy production based on the energy derived from timber and biofuels. In regards with the environmental targets, we computed a pollution indicator based on the use of pesticides and fertilizers, and the ecological Community Specialization Index as it emphasizes the specialist species which are more fragile than generalist species in the face of land use and climate changes.
In order to capture other facets of ecosystems, we added another community indicator related to the mean trophic index in the community (namely the Community Trophic Index) as well as abundance indices related to generalist species, farmland specialist species and woodlands specialist species (Generalist Bird Index GBI, Farmland Bird Index FBI, Woodland Bird Index WBI respectively). The integration of abundance indices is interesting regarding an institutional perspective since the FBI is one of the official ecological indicators of agricultural changes in Europe (Gregory et al., 2005).
A third group of indicators aims at assessing the economic states. We considered both public and private perspectives. Adopting a public point of view, we compute the inter-annual public budget and the inter-annual social welfare of each scenario. By considering the targeted indicators of each scenario, we derived two cost-effectiveness measures of public policies based on budget and social welfare. Following a private perspective, we computed the inter-annual national income of the regional planners over France, their long-term mean income based on their profit in 2050 and the average inequality between regional incomes.
Finally, we investigated a last group of 3 indicators to go further on the climate impacts. In the perspective of a mitigation target, we computed an indicator of carbon stock and an indicator of carbon sequestration flow. We completed these indicators by an ecological indicator related to the community adaptation to temperature change, namely the Community Thermal Index.
Supplementary Appendix S6.4 provides more details about the mathematical computations of these indicators.
4 Results
4.1 Effectiveness of policy scenarios
Table 3 presents the progression of the target indicators for the 4 public policy scenarios (Food, Energy, Pollution and Nature) in comparison with the BAU scenario. We observe that the 4 scenarios are able to improve their related target indicator. In other words, a similar public envelope of 120bn€ is sufficient to increase the 4 objectives. However, the performances are rather heterogeneous among scenarios: the Energy scenario increases bioenergy production by 168% in comparison with BAU, the Food scenario increases food production by 50% in comparison with the BAU, the Pollution scenario diminishes pollution spreading by 21% in comparison with BAU while the Nature scenario improves the specialization of bird communities by 0.94% in comparison with BAU. As a consequence, the public budget cost-effectiveness of the 4 scenarios is strongly contrasted as depicted in row 3 of Table 3.

TABLE 3. Overall effectiveness of the 4 public policy scenarios and the BAU. Highlighted values are computed in comparison to the BAU and in regards with the scenarios target indicators, namely Food production for Food scenario, Energy production for Energy scenario, Pollution spreading for Pollution scenario, and Specialization Community Index for Nature scenario. All other indicators read in the specified units and compare across scenarios. For indicators computation details see 6.4.
As expected, the 4 scenarios exhibit a decrease in social welfare (raw 4 in Table 3) in comparison with the BAU. This decrease is smaller for the Energy scenario (1.7%), than the Pollution scenario (3.2%) and than the Food and Nature scenarios which exhibit similar social welfare decreases (4.3%). However considering these decreases in regards with the target indicator, namely with the cost-effectiveness based on social welfare (row 5 in Table 3), the ranking of the scenarios comes back to the one obtained with the budget-based cost-effectiveness. In other words, the Energy scenario is the most cost-effective, followed by the Food one, then the Pollution one and last, the Nature scenario. The stability of this ranking regarding the two cost-effectiveness indicators suggests that it is more effective to achieve productive goals (related to food and energy) than to achieve environmental goals (related to pollution and nature).
Finally, Table 4 in Supplementary Appendix S6.5 shows a robustness analysis of our results based on a sensitivity analysis. We simulate an upper case (the public policy incentives are Lower than in the scenario depicted in Table 3) and a lower case (the public policy incentives are higher than in the scenario depicted in Table 3) for each scenario. This sensitivity analysis confirms the following observations: all the objectives, productive as well as environmental, are achievable with an adequate public policy, but the productive goals (energy and food) are achieved with more effectiveness than the environmental ones (pollution and nature).

TABLE 4. Sensitivity analysis of overall effectiveness of the 4 public policy scenarios, based on a public policy incentive lower case and a public policy incentive upper case (see tab.2). Highlighted values are computed in comparison to the BAU and in regards with the scenarios target indicators, namely Food production for Food scenario, Energy production for Energy scenario, Pollution spreading for Pollution scenario, and Specialization Community Index for Nature scenario. All other indicators read in the specified units and compare across scenarios.
4.2 Cross effects of policy scenarios
Table 5 presents the cross impacts of the 4 public policy scenarios in regards with BAU on the performances related to the 4 objectives: food (food production), energy (energy production), pollution (pollution indicator) and nature (community specialization index). We observe that no scenario is able to increase simultaneously the whole set of performance indicators.

TABLE 5. Crossed marginal impacts of the 4 public policy scenarios in regards with the BAU on the 4 main objectives, namely: food production, energy production, pollution spread, Community Specialization Index. Indicators levels of the BAU are expressed in the specified unit for reference, while highlighted values are computed in comparison to the BAU. Table reads as follow: food production level in the Food scenario is 50% higher than in the BAU scenario.
However some main results emerge from Table 5. First, food production, except within the Food scenario, always decreases compared to the BAU scenario. This suggests an antagonism between the two productive goals, namely food and energy, as well as an antagonism between the food goal and the two environmental goals (pollution and nature). Second, we observe that one of the production-oriented scenarios, the Energy scenario, is quasi-neutral regarding pollution spread (+0.19% of pollution compared to BAU) and increases the ecological indicator. This second result suggests that productive performance improvement does not systematically jeopardize environmental performance. The positive effect of this production-oriented scenario on the ecological indicator is explained by the fact that forest expansion provides refuges and shelters nesting areas for most vulnerable species. The very slight excess of pollution might come from the relatively higher intensity of the crop mix in phytosanitary products. Forest cover expands predominantly over low intensity farming and subsidized oleaginous crops make an intensive use of pesticides and fertilizers. Third, we exhibit a third result as a corollary of the second result: the Energy scenario avoids the overall largest deterioration of performances of the landscape. Except its negative impact on food production, the Energy scenario looks indeed either positive or neutral. Regarding its negative impact on food production, it is nonetheless the least negative impact among the non-food targeted scenarios. As a consequence, the Energy scenario offers an interesting compromise between the different goals of agricultural public policies.
4.3 Ecosystem focus
To further on the ecological side, Table 6 presents the performances of the BAU and the 4 policy scenarios with respect to our 5 ecological indicators. We observe that no scenario is positive for all the dimensions of ecosystems. Surprisingly, the Nature scenario presents a poor overall ecological performance since 3 ecological indicators are declining and 2 of them (Farmland Bird Index and Generalist Bird Index) do so quite strongly. The decrease in arable land and temporary grassland leading to scarcer food resources in the Nature scenario might explain the decline in generalist and farmland bird species. This result suggests that semi-natural habitats expansion as supported in the Nature scenario succeeds in protecting the most vulnerable species by providing them with refuges but should be thought in relation to other ecosystem facets.

TABLE 6. Crossed marginal impacts of the 4 public policy scenarios in regards with the BAU on ecological indicators. Indicators levels of the BAU are given for reference. Note that all indicators are dimensionless (for indicators computation details see 6.4). Table reads as follow: the Food scenario increases the Community Specialization Index by 1.71% in comparison to the BAU.
On the contrary, the Energy and Pollution scenarios appear as interesting perspectives for ecosystems since they present well-balanced ecological performances. Both of them limit their environmental footprint with the decline of intensive farming, favoring the development of high quality habitats (grasslands for the Pollution scenario and forests for the Energy scenario), along with the massive reduction of phytosanitary products use in the case of the Pollution scenario.
Finally, Table 6 reveals that woodland specialist species seem to benefit from all scenarios. This is explained by the fact that they strongly react to both the decrease in inputs use (pesticides and fertilizers) and forest expansion, whatever its composition.
4.4 Economic focus
Table 7 focuses on economic performances by considering the private agent perspective. As expected with scenarios with overall positive incentives, the intertemporal profit is larger than under BAU for the 4 policy scenarios (+5–6% in average). While this effect exhibits similar intensity for the 4 scenarios, contrasted long-term trends appear. On the one hand, the Food and Nature scenarios generate losses in long-term profit. This is due to the growing impact of taxes: despite a fairly high tax base, the tax is not sufficient to reverse the evolution of temporary grasslands. On the other hand, the Energy and Pollution scenarios both preserve long-term profits. Subsidized land uses had an original marginal profitability not far from the competing areas within the same region. Since subsidies are all the more profitable for private landowners as the original marginal profitability gaps are reduced, this preserves long-term incomes and hampers social welfare loss.

TABLE 7. Marginal impacts of the 4 public policy scenarios on private economic indicators. Indicators levels of the BAU are expressed in the specified unit for reference, while highlighted values are computed in comparison to the BAU. Table reads as follow: Food scenario increases by 4.59% the interannual income in comparison to the BAU. Shades of green indicate a positive evolution while shades of pink indicate a negative evolution.
Contrasted performances are also observed regarding inequalities: while Food and Nature scenarios severely decrease inequalities, the Energy and Pollution scenario both slightly increase inequalities. Regions that were initially the least profitable (usually those with more semi-natural habitats and less crops) benefit from a catch-up effect with the Nature scenario that favors grasslands and broad-leaves forests. In addition, both Food and Nature contribute to regional incomes standardization by a “redistribution process”: taxes affect mainly the originally most profitable regions whereas subsidies are directed towards originally least profitable regions. In contrast, the Energy and Pollution scenarios favor regions whose activities were originally not less profitable than average. Clustering scenarios based on their performances in terms of long-term profit and inequalities yields two groups with opposite behaviors. On the one hand, the Food and Nature scenarios, which decrease long-term profit but reduces inequalities. On the other hand, the Energy and Pollution scenarios, which increase long-term profit but increases inequalities. This observation suggests a trade-off between long-term profit and inequalities.
4.5 Impact of climate change
Table 8 investigates the impact of the public policy scenarios on the climate change adaptation indicators, namely the two climate mitigation indicators: Carbon Storage and Carbon Sequestration Flow, and the Community Thermal Indicator for the ecological side. First, we observe that the Energy and Nature scenarios yield particularly positive results regarding climate change mitigation: the impact on both carbon stock and flow is strongly positive. This is not surprising as these scenarios promote the expansion of grasslands and forests, two land uses with a high positive impact on climate change mitigation. On the contrary, the performances of the Food and Pollution scenarios are worse than in the BAU scenario. Second, regarding the thermal index, all scenarios have a negative impact while remaining weak. This indicates that the impact on the “cold” species is slightly stronger than the impact on the “hot” species. Third, the Nature scenario exhibits good performances on carbon mitigation, intermediary performances on ecological adaptation and small changes on the community thermal index. It might be a better compromise regarding climate change in the RCP 4.5 than the Energy scenario which is quasi-neutral regarding climate issues.

TABLE 8. Marginal impacts of the 4 public policy scenarios on the climate adaption indicators: two climate mitigation indicators and a metric of birds population adaptation to climate change. Indicators levels of the BAU are expressed in the specified unit for reference, while highlighted values are computed in comparison to the BAU. Note that the CTI is dimensionless. Table reads as follow: Food scenario diminishes by 4.59% the carbon stockage in comparison to the BAU. Shades of green indicate a positive evolution while shades of pink indicate a negative evolution.
Table 9 presents the performances of the BAU and the 4 policy scenarios under a more severe climate change scenario assuming higher CO2 emission (RCP8.5). Performances in the opposite direction of those obtained with climate scenario RCP4.5 are in red boxes. Generally speaking, we observe that few performances are going in a different direction under this stronger climate change scenario. More precisely, the 4 policy scenarios improve their target indicator in a similar way with the two climate change scenarios. For the Energy, Pollution and Nature scenarios, the targeted performances are slightly increased with climate scenario RCP 8.5 while they are very slightly decreased for the Food scenario. While the target indicator in the Nature scenario only weakly improves, this maps to a non-negligible marginal impact in terms of budgetary cost-effectiveness: it increases by 57%. Despite this effect, the Nature scenario remains the least cost-effective among the 4 we investigated in this study.
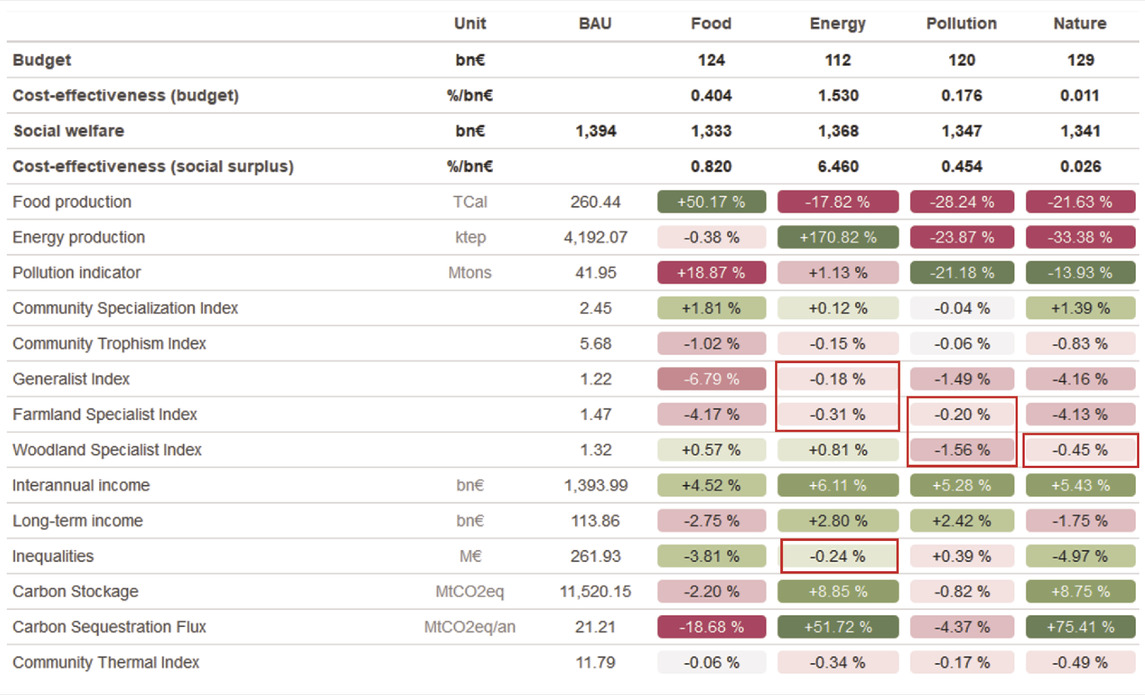
TABLE 9. Overall effectiveness and marginal impacts of the 4 public policy scenarios on the whole set of indicators under the RCP8.5 scenario. Indicators levels of the BAU are expressed in the specified unit for reference, while highlighted values are computed in comparison to the BAU. Red boxes stress values for which the evolution is of the opposite sign of marginal impacts under the RCP4.5 scenario.
The ecological indicators depict the highest differences compared to other indicators. Impacts on ecosystems are contrasted with an overall negative impact on bird abundance and community structure. Especially, the Generalist Bird Index, the Farmland Bird Index and the Community Trophic Index are declining with the RCP8.5 climate scenario whatever the policy scenarios. The impact of the policy scenarios on the Woodland Bird Index, which was always positive with the RCP4.5, becomes more contrasted since the two environment-targeted scenarios (Pollution and Nature) decrease the indicator under the RCP8.5 climate scenario. Generally speaking, climate change does not have monotonous marginal impact on ecological indicators since some of them tend to decrease under RCP8.5 (like the Generalist Bird Index) while some others tends to increase (like the Community Specialization Index). Focusing on economic performances, the impact of climate change is very slight. Even for the impact of the Energy scenario on inequalities, which becomes negative with RCP8.5: the marginal impact is actually small and close to 0.
However, the overall impact of increasing climate change is negative, by decreasing private economic performances. Finally, similar conclusions are obtained regarding the climate change mitigation indicators even if the negative impact of the Food and Pollution scenarios on carbon indicators is less negative under RCP8.5. The impact on thermal indicator remains negative for all scenarios, but we can note that the Nature scenario exhibits the worst results. In a RCP 8.5 context, no scenario looks to be a good compromise to satisfy the 3 climate change mitigation indicators.
5 Discussion
5.1 Synthesis of results
All 4 public policy scenarios succeed in improving their related target indicator with a similar public enveloppe of 120bn€. However, their performance are rather heterogeneous and depict strongly contrasted budget cost-effectiveness. Interestingly, the analysis through budget-based cost-effectiveness and social-based cost-effectiveness rank the scenarios in the same order: Energy and Food first, followed by the Pollution scenario and last, the Nature scenario. This would suggest a greater effectiveness in achieving productive goals than environmental goals. We confirm the robustness of this ranking with a sensitivity analysis based on scenarios with upper and lower public incentives than the initial ones.
The overall good performance of scenarios should not hide that any of them is able to increase simultaneously the whole set of performance indicators. We reveal different antagonisms: productive goals (food and energy) cannot be achieved concomitantly, but, if productive food goals lower environmental outputs, energy productive performance does not systematically jeopardize environmental performance, as witnessed by increases in specific ecological indicators. All in all, the Energy scenario avoids the overall largest deterioration of performances of the landscape and thereby appears to be an interesting compromise between the different goals of agricultural public policies.
The overall positive incentives increase the intertemporal profit from the private agent perspective. Long-term trends in private profits are more contrasted: losses in long-term profits for the Food and Nature scenarios can be explained by the incapacity of taxes to reverse the evolution of the highly profitable temporary grasslands. On the contrary, subsidies schemes of the Pollution and Energy scenarios preserve long-term incomes and hamper social welfare loss. The two same clusters emerge from the contrasted performances in terms of inequalities. However, Food and Nature here contribute to regional incomes standardization by a “redistribution process”, while Energy and Pollution favor regions whose activities were originally not less profitable than average. This would suggest a trade-off between long-term profit and inequalities.
Antagonisms exist among the multiple dimensions of ecosystems and no scenario is able to positively increase all ecological indicators. Surprisingly, the Nature scenario presents a poor overall ecological performance, as food resources allegedly diminish. In contrast, the Energy and Pollution scenarios present well-balanced ecological performances by favoring the expansion of high-quality habitats or achieving massive reductions in phytosanitary products use.
Regarding climate change, scenarios promoting the expansion of high soil-CO2-content land uses, such as Nature and Energy scenarios, unsurprisingly increase carbon storage and carbon sequestration flow, thereby favoring climate change mitigation. For a general assessment, most previous results stand when assessed under a more severe climate change scenario. Notably, the 4 policy scenarios improve their target indicator in a similar way with the two climate change scenarios. Only the ecological indicators show contrasted performance with an overall negative impact on bird abundance and community structure. No scenario can restrain the drop in generalists and farmland birds population, and in the Community Trophic Index. However, climate change does not have a monotonous marginal impact on ecological indicators since some also tend to increase, as the Community Specialization index. Lastly, none of the scenarios prevent the slight decrease in economic performance due to more severe climate conditions, nor appear to be a good compromise to satisfy the 3 climate change mitigation indicators.
5.2 Implications for agricultural public policies
First, our results show that it is possible to achieve productive and environmental goals with the on-going budget of European agricultural policy. This conclusion opens stimulating challenges about which strategy to implement in designing agricultural policies, specifically when considering expectations about biodiversity issues (Pe’er et al., 2019; Pe’er and Lakner, 2020; Pe’er et al., 2020; Candel et al., 2021). As an encouraging perspective, we highlighted that synergies between productive and environmental performances of agricultural landscapes do exist, meaning that they can be improved simultaneously compared to the current situation. These synergies are nevertheless not trivial nor systematic since some productive goals remain antagonistic with some environmental goals. A fine analysis of the variety of agricultural policy goals is thus required.
Our study shows that 1) the food-oriented goal seems to be particularly orthogonal with the other dimensions of agricultural policy targets, namely the energy and environmental ones, and 2) a grassland-oriented policy (here the Nature scenario) is antagonistic to productive goals. These results bring new significant explanations to the current locking of the European agricultural public policy: by coupling a historically food-dedicated first set of incentives (e.g the “first pillar”) with a second set of environment-targeted incentives historically promoting semi-natural habitats (e.g “the second pillar”), the structure of the European CAP looks therefore inevitably inefficient. Our study allows to go a step further on the tension between the two pillars of the current CAP. Indeed, we showed a high contrast in cost-effectiveness to achieve the different public policy targets. More specifically, the food target is achieved with a very high cost-effectiveness while the ecological target driven by semi-natural habitat promotion is achieved with a very small one. Such an imbalance necessarily makes the environmental targets less attractive in regards with the productive ones. It might hamper the re-balancing of the CAP budget from the historical productive incentives towards environmental incentives. We therefore understand how particularly fertile the European agricultural policy is for high frustrations (Erjavec and Erjavec, 2015; Alons, 2017; Hennig and Breustedt, 2018; Bengtsson et al., 2019; Hristov et al., 2020) and hot negotiations (European Commission, 2018; Court of Auditors, 2019). In this context, we support that exploring potential synergies between the alternative productive and environmental goals constitutes the only perspectives to design sustainable agricultural public policies in Europe.
In consistency with two recent European reports (Wissenschaftliche Beiräte, 2017; European Commission et al., 2019), our study highlighted that an agricultural public policy which focuses on energy production might offer an interesting compromise regarding the different facets of agricultural landscapes. Indeed, energy provision performance improvement does not systematically jeopardize environmental performances since our energy-targeted scenario presents well-balanced ecological performances and generates almost no pollution change. Moreover, food production reduction remains limited. Regarding the economic side, the public cost-effectiveness is high and the impact on private income is either positive or neutral. Eventually, this scenario is a satisfying strategy regarding carbon-based climate change mitigation indicators. Our study allowed to complete this result by pointing to a credible environment-oriented alternative to the actual production-oriented policy. Indeed, the pollution scenario constitutes an overall well-balanced strategy suggesting that environmental issues should be faced by the pollution reduction commitment instead of a land use change objective (as in the Nature scenario). Nevertheless, we have to keep in mind that an environment-oriented policy generates smaller synergies than an energy-oriented one since it is slightly less competitive regarding both the ecological and economic sides.
Interestingly, our conclusions look robust to climate change contexts if we consider highly probable climate change scenarios (here RCP 4.5 and RCP 8.5). In other terms, adequate agricultural public policies might attenuate climate change effects when considering intermediary climate change scenarios. Our study specified that this effect might come from either a land use distribution effect or an input use effect, thus highlighting two potential levers to deal with climate issues in agricultural policies (Dunford et al., 2015; Mouchet et al., 2017; Pe’er and Lakner, 2020).
However, we have to note that the climate scenarios investigated in this study are based on moderate climate changes. Due to non-monotonous effects on agricultural systems and ecosystems (Peters et al., 2019; Pörtner et al., 2021), different conclusions might emerge with stronger climate change effects. Eventually, bio economic projections should be conducted at a longer-term simulation window to the 2,100 time horizon. Indeed, these scenarios are expected to exhibit stronger divergences by the end of the century.
5.3 The bioeconomic modeling framework to investigate future global changes
This paper presents an interdisciplinary study based on a bioeconomic model integrating complex interdependencies between ecosystems and human society (Jean and Mouysset, 2022). Despite divergences between economic and ecological disciplines (Drechsler, 2020), this kind of bioeconomic framework offers the holistic view required to investigate the trade-offs between ecosystem services provided by agricultural landscapes in a context of climate change (Bateman et al., 2013; Oliver and Morecroft, 2014; Mouysset et al., 2019; Rosa et al., 2020). Based on mechanistic process-based ecological and economic equations, this approach avoids the monetary evaluation of biodiversity, which is controversial (Diamond and Hausman, 1994). The precision required to integrate agronomic, economic, ecological and climate data compensates for the relatively simple formalism of the model and makes it possible to obtain robust and informative results to investigate prospective scenarios and to understand the future impacts of global changes.
The results are however strongly dependent on methodological choices. For example, the profit-maximizing assumption in our model implies that the most profitable land uses will necessarily be chosen at the expense of the less profitable ones. But in the real world, land use changes are also driven by risk aversion (Lien, 2002). This economic mechanism has shown positive and determinant impact on agricultural bioeconomic performances (Mouysset and Doyen, 2013).
Indeed, diversification driven by risk aversion has positive impacts on ecosystems (Lehman and Tilman, 2000; Roscher et al., 2009; Isbell et al., 2011; Cardinale et al., 2012) and ecosystem services provision (Robinson and Sutherland, 2002; Laiolo, 2005; Quaas et al., 2007). Moreover, our economic model does not include explicit spatial processes such as neighborhood effects, despite some considerations for spatial heterogeneity. From these neighborhood phenomena, we could expect positive effects by expanding good practices, or on the contrary deleterious effects by slowing down their expansion through collective inertia (Wollni and Andersson, 2014). The overall effect on the effectiveness of public policy is thus non-trivial while being possibly determinant.
Regarding the climate change impact, we considered only linear effects between land use and climate changes within ecological models. Yet, the mutual impacts of these two global changes look non-linear and complex with expected threshold effects (Oliver and Morecroft, 2014; Elmhagen et al., 2015; Peters et al., 2019). In this context, complexifying the ecological model would be required by incorporating second order effects of climate variables as well as cross-effects between land use and climate variables. Moreover, the lack of an explicit modeling of the direct effects of climate on carbon sequestration services may lead to an underestimation of climate change impacts. Indeed, climate change modifies land use patterns that indirectly affect carbon stock by replacing high-CO2-content land uses by low ones. But future warming could also directly and significantly reduce the carbon storage capacities of soils and biomass (Álvaro-Fuentes et al., 2012). With a medium-term prospective window, we cannot apprehend the diminishing efficiency of carbon sequestration due to saturation of the carbon sink at some point.
For the agronomic side, the linearity of the crop response function bounded by an upper threshold only approximate the real response function, closer to a concave curve model featuring diminishing marginal yield with respect to inputs. It further implies quasi-perfect substitution between inputs while pesticides and fertilizers serve specific purposes: eliminate weeds and undesirable organisms for the former, and provide plants with nutrients for the latter.
5.4 Future research avenues
We propose here avenues for future research, regarding the 2 facets of our bioeconomic framework, the economic and ecological ones.
Regarding the economic perspective, it would be very informative to connect such nation-based studies with a broader framework. Indeed, both climate issues and market dynamics exhibit large-scale drivers, at least at the European scale. Considering large-scale supply and demand balancing as well as European reallocation of resources by exploiting the comparative advantages of countries might help to design endogenous adaptation to climate change (Dunford et al., 2015; Mouchet et al., 2017; European Commission Research Centre, Joint et al., 2020). At the same time, refining nation-based bioeconomic frameworks to a smaller scale could be required to address the question of inequalities. Spatialized public policies might thus be assessed to take into account the heterogeneous regional adaptation capacity depending on capital and resources (Dunford et al., 2015; Nicholas et al., 2020). Investigations in this direction would be informative for the debate on targeted-area measures such as Climate and Agri-environmental Schemes.
Regarding ecosystems, a natural perspective is to deepen the representation of biodiversity, consistently with Rosa et al. (2020), who calls for the integration of specific functional traits of biodiversity and for an expanded coverage of the multiple dimensions of biodiversity and ecosystem services. As many taxa are impacted by climate change and land use changes (Martin et al., 2013; Visconti et al., 2015), their inclusion would provide more accurate information on the general level of vulnerability of ecosystems. It would notably open the possibility of modeling interspecies interactions within food webs which are determinant regarding the risk of extinction due to climate and land use drivers (Holyoak and Heath, 2016). As some species are more impacted by polluting uses, especially those in freshwater ecosystems (Bayramoglu et al., 2019), their inclusion could be an additional argument in favor of environmentally friendly policies. In regards, the retroactive effects of biodiversity on land use changes (through pollination, natural control of pathogens, and pollution control), could be better modeled if the ecological functionalities of biodiversity (stemming from its abundance, richness and functional diversity) were clarified (Gagic et al., 2017; Dainese et al., 2019; Woodcock et al., 2019).
Data availability statement
The original contributions presented in the study are included in the article/Supplementary Materials, further inquiries can be directed to the corresponding author.
Author contributions
All authors contributed to handling the manuscripts and writing this editorial, and approved it for publication.
Acknowledgments
Such studies would not be feasible without the dedicated help of hundreds of volunteers monitoring bird communities across the country yearly.
Conflict of interest
The authors declare that the research was conducted in the absence of any commercial or financial relationships that could be construed as a potential conflict of interest.
Publisher’s note
All claims expressed in this article are solely those of the authors and do not necessarily represent those of their affiliated organizations, or those of the publisher, the editors and the reviewers. Any product that may be evaluated in this article, or claim that may be made by its manufacturer, is not guaranteed or endorsed by the publisher.
Supplementary material
The Supplementary Material for this article can be found online at: https://www.frontiersin.org/articles/10.3389/fenvs.2022.889506/full#supplementary-material
Footnotes
1https://publications.jrc.ec.europa.eu/repository/
2Aire Limitée Adaptation dynamique Développement InterNational.
3Centre National de la Recherche en Météorologie.
References
Alons, G. (2017). Environmental policy integration in the EU’s common agricultural policy: Greening or greenwashing? J. Eur. Public Policy 24, 1604–1622. doi:10.1080/13501763.2017.1334085
Álvaro-Fuentes, J., Easter, M., and Paustian, K. (2012). Climate change effects on organic carbon storage in agricultural soils of northeastern spain. Agric., Ecosyst. Environ. 155, 87–94. doi:10.1016/j.agee.2012.04.001
Ay, J.-S., Chakir, R., Doyen, L., Jiguet, F., and Leadley, P. (2014). Integrated models, scenarios and dynamics of climate, land use and common birds. Clim. Change 126, 13–30. doi:10.1007/s10584-014-1202-4
Bateman, I. J., Harwood, A. R., Mace, G. M., Watson, R. T., Abson, D. J., Andrews, B., et al. (2013). Bringing ecosystem services into economic decision-making: Land use in the United Kingdom. Science 341, 45–50. doi:10.1126/science.1234379
Batlle-Aguilar, J., Brovelli, A., Porporato, A., and Barry, D. A. (2011). Modelling soil carbon and nitrogen cycles during land use change. Sustain. Agric. 2, 499–527. doi:10.1007/978-94-007-0394-0
Bayramoglu, B., Chakir, R., and Lungarska, A. (2019). Impacts of land use and climate change on freshwater ecosystems in France. Environ. Model. Assess. (Dordr). 25, 147–172. doi:10.1007/s10666-019-09673-x
Bellard, C., Bertelsmeier, C., Leadley, P., Thuiller, W., and Courchamp, F. (2012). Ecol. Lett. 15, 365–377. doi:10.1111/j.1461-0248.2011.01736.x
Bengtsson, J., Bullock, J. M., Egoh, B., Everson, C., Everson, T., O'Connor, T., et al. (2019). Grasslands-more important for ecosystem services than you might think. Ecosphere 10, e02582. doi:10.1002/ecs2.2582
Benton, T. G., Bryant, D. M., Cole, L., and Crick, H. Q. P. (2002). Linking agricultural practice to insect and bird populations: A historical study over three decades. J. Appl. Ecol. 39, 673–687. doi:10.1046/j.1365-2664.2002.00745.x
Bianchi, F., Booij, C., and Tscharntke, T. (2006). Sustainable pest regulation in agricultural landscapes: A review on landscape composition, biodiversity and natural pest control. Proc. R. Soc. B 273, 1715–1727. doi:10.1098/rspb.2006.3530
Bommarco, R., Marini, L., and Vaissière, B. E. (2012). Insect pollination enhances seed yield, quality, and market value in oilseed rape. Oecologia 169, 1025–1032. doi:10.1007/s00442-012-2271-6
Candel, J. J. L., Lakner, S., and Pe’er, G. (2021). Europe’s reformed agricultural policy disappoints. Nature 595, 650. doi:10.1038/d41586-021-02047-y
Cardinale, B. J., Duffy, J. E., Gonzalez, A., Hooper, D. U., Perrings, C., Venail, P., et al. (2012). Biodiversity loss and its impact on humanity. Nature 486, 59–67. doi:10.1038/nature11148
Chamberlain, D., Fuller, R., Bunce, R., Duckworth, J., and Shrubb, M. (2000). Changes in the abundance of farmland birds in relation to the timing of agricultural intensification in england and wales. J. Appl. Ecol. 37, 771–788. doi:10.1046/j.1365-2664.2000.00548.x
Cocco, V., Kervinio, Y., and Mouysset, L. (2021). Enhancing bioeconomic effectiveness of land use policies with spatial targeting and ecological continuities. Master thesis. Paris (France): ENS-PSL.
Convention on Biological Diversity and United Nations Environment Programme, j. (2014). Global biodiversity outlook 4: A mid-term assessment of progress towards the implementation of the strategic plan for biodiversity 2011-2020. Montréal: Secretariat of the Convention on Biological Diversity. 155.
Costanza, R., de Groot, R., Braat, L., Kubiszewski, I., Fioramonti, L., Sutton, P., et al. (2017). Twenty years of ecosystem services: How far have we come and how far do we still need to go? Ecosyst. Serv. 28, 1–16. doi:10.1016/j.ecoser.2017.09.008
Court of Auditors (2019). Opinion no 7/2018 (pursuant to article 322(1)(a) tfeu) concerning commission proposals for regulations relating to the common agricultural policy for the post-2020 period (com(2018) 392, 393 and 394 final). Official J. Eur. Union 62, 1–55.
Dainese, M., Martin, E. A., Aizen, M. A., Albrecht, M., Bartomeus, I., Bommarco, R., et al. (2019). A global synthesis reveals biodiversity-mediated benefits for crop production. Sci. Adv. 5, eaax0121. doi:10.1126/sciadv.aax0121
Dalsgaard, B. (2020). Land-use and climate impacts on plant–pollinator interactions and pollination services. Diversity 12, 168. doi:10.3390/d12050168
de Chazal, J., and Rounsevell, M. D. (2009). Land-use and climate change within assessments of biodiversity change: A review. Glob. Environ. Change 19, 306–315. doi:10.1016/j.gloenvcha.2008.09.007
Devictor, V., Julliard, R., Couvet, D., and Jiguet, F. (2008). Birds are tracking climate warming, but not fast enough. Proc. R. Soc. B 275, 2743–2748. doi:10.1098/rspb.2008.0878
Diamond, P. A., and Hausman, J. A. (1994). Contingent valuation: Is some number better than no number? J. Econ. Perspect. 8, 45–64. doi:10.1257/jep.8.4.45
Díaz, S., Settele, J., Brondízio, E. S., Ngo, H. T., Guèze, M., Agard, J., et al. (2019). Ipbes (2019): Summary for policymakers of the global assessment report on biodiversity and ecosystem services of the intergovernmental science-policy platform on biodiversity and ecosystem services. Bonn, Germany: IPBES secretariat.
Donald, P. F., Green, R. E., and Heath, M. F. (2001). Agricultural intensification and the collapse of europe's farmland bird populations. Proc Biol Sci. 268, 25–29. doi:10.1098/rspb.2000.1325
Drechsler, M. (2020). Model-based integration of ecology and socio-economics for the management of biodiversity and ecosystem services: State of the art, diversity and current trends. Environ. Model. Softw. 134, 104892. doi:10.1016/j.envsoft.2020.104892
Dunford, R. W., Smith, A. C., Harrison, P. A., and Hanganu, D. (2015). Ecosystem service provision in a changing Europe: Adapting to the impacts of combined climate and socio-economic change. Landsc. Ecol. 30, 443–461. doi:10.1007/s10980-014-0148-2
Elalamy, Y., Doyen, L., and Mouysset, L. (2019). Contribution of the land use allocation model for agroecosystems: The case of torrecchia vecchia. J. Environ. Manag. 252, 109607. doi:10.1016/j.jenvman.2019.109607
Elmhagen, B., Eriksson, O., and Lindborg, R. (2015). Implications of climate and land-use change for landscape processes, biodiversity, ecosystem services, and governance. AMBIO 44, 1–5. doi:10.1007/s13280-014-0596-6
Erjavec, K., and Erjavec, E. (2015). ‘greening the CAP’ – just a fashionable justification? A discourse analysis of the 2014–2020 CAP reform documents. Food Policy 51, 53–62. doi:10.1016/j.foodpol.2014.12.006
European Commission for Agriculture, D. G., Development, R., Pražan, J., Nanni, S., and Redman, M. (2019). Evaluation study of the impact of the CAP on climate change and greenhouse gas emissions : Final report. Luxembourg: Publications Office of the European Union, 2019.
European Commission (2018). Proposal for a regulation of the european parliament and of the council establishing rules on support for strategic plans to be drawn up by member states under the common agricultural policy (cap strategic plans) and financed by he european agricultural guarantee fund (eagf) and by the european agricultural fund for rural development (eafrd) and repealing regulation (eu) no 1305/2013 of the european parliament and of the council and regulation (eu) no 1307/2013 of the european parliament and of the council. Brussels. com/2018/392 final - 2018/0216 (cod).
Gagic, V., Kleijn, D., Báldi, A., Boros, G., Jørgensen, H. B., Elek, Z., et al. (2017). Combined effects of agrochemicals and ecosystem services on crop yield across Europe. Ecol. Lett. 20, 1427–1436. doi:10.1111/ele.12850
Garratt, M., Breeze, T., Jenner, N., Polce, C., Biesmeijer, J., Potts, S., et al. (2014). Avoiding a bad apple: Insect pollination enhances fruit quality and economic value. Agric. Ecosyst. Environ. 184, 34–40. doi:10.1016/j.agee.2013.10.032
Gaüzère, P., Princé, K., and Devictor, V. (2016). Where do they go? The effects of topography and habitat diversity on reducing climatic debt in birds. Glob. Chang. Biol. 23, 2218–2229. doi:10.1111/gcb.13500
Geslin, B., Aizen, M. A., Garcia, N., Pereira, A.-J., Vaissière, B. E., Garibaldi, L. A., et al. (2017). The impact of honey bee colony quality on crop yield and farmers’ profit in apples and pears. Agric. Ecosyst. Environ. 248, 153–161. doi:10.1016/j.agee.2017.07.035
Gregory, R. D., David, G., Noble, D., and Custance, J. (2004). The state of play of farmland birds: Population trends and conservation status of lowland farmland birds in the United Kingdom. Ibis. 146, 1–13. doi:10.1111/j.1474-919x.2004.00358.x
Gregory, R. D., van Strien, A., Vorisek, P., Meyling, A. W. G., Noble, D. G., Foppen, R. P., et al. (2005). Developing indicators for European birds. Phil. Trans. R. Soc. B 360, 269–288. doi:10.1098/rstb.2004.1602
Gregory, R., Vorisek, P., Van Strien, A., Gmelig Meuling, A., Jiguet, F., Fornasari, L., et al. (2017). Population trends of widespread woodland birds in Europe. Ibis 149, 78–97. doi:10.1111/j.1474-919x.2007.00698.x
Hennig, S., and Breustedt, G. (2018). The incidence of agricultural subsidies on rental rates for grassland. J. Econ. Statistics (Jahrbuecher fuer Natl. und Statistik) 238, 125–156. doi:10.1515/jbnst-2017-0124
Holyoak, M., and Heath, S. K. (2016). The integration of climate change, spatial dynamics, and habitat fragmentation: A conceptual overview. Integr. Zool. 11, 40–59. doi:10.1111/1749-4877.12167
Hristov, J., Clough, Y., Sahlin, U., Smith, H. G., Stjernman, M., Olsson, O., et al. (2020). Impacts of the EU's common agricultural policy “greening” reform on agricultural development, biodiversity, and ecosystem services. Appl. Econ. Perspect. Policy 42, 716–738. doi:10.1002/aepp.13037
European Commission Research Centre, Joint Hristov, J., Toreti, A., Pérez Domínguez, I., Dentener, F., Fellmann, T., Elleby, C., et al. (2020). Analysis of climate change impacts on EU agriculture by 2050, EUR 30078 EN. Luxembourg: Publications Office of the European Union. doi:10.2760/121115
Isbell, F., Calcagno, V., Hector, A., Connolly, J., Harpole, W. S., Reich, P. B., et al. (2011). High plant diversity is needed to maintain ecosystem services. Nature 477, 199–202. doi:10.1038/nature10282
Jean, S., and Mouysset, L. (2022). Bioeconomic models for terrestrial social-ecological system management: A review. Int. Rev. Environ. Resour. Econ. 16, 43–92. doi:10.1561/101.00000131
Jiguet, F., Devictor, V., Julliard, R., and Couvet, D. (2012). French citizens monitoring ordinary birds provide tools for conservation and ecological sciences. Acta Oecologica. 44, 58–66. doi:10.1016/j.actao.2011.05.003
Julliard, R., Clavel, J., Devictor, V., Jiguet, F., and Couvet, D. (2006). Spatial segregation of specialists and generalists in bird communities. Ecol. Lett. 9, 1237–1244. doi:10.1111/j.1461-0248.2006.00977.x
Kapitza, S., Ha, P. V., Kompas, T., Golding, N., Cadenhead, N. C. R., Bal, P., et al. (2021). Assessing biophysical and socio-economic impacts of climate change on regional avian biodiversity. Sci. Rep. 11, 3304. doi:10.1038/s41598-021-82474-z
Kirchweger, S., Clough, Y., Kapfer, M., Steffan-Dewenter, I., and Kantelhardt, J. (2020). Do improved pollination services outweigh farm-economic disadvantages of working in small-structured agricultural landscapes? – development and application of a bio-economic model. Ecol. Econ. 169, 106535. doi:10.1016/j.ecolecon.2019.106535
Klein, A.-M., Vaissière, B. E., Cane, J. H., Steffan-Dewenter, I., Cunningham, S. A., Kremen, C., et al. (2006). Importance of pollinators in changing landscapes for world crops. Proc. R. Soc. B 274, 303–313. doi:10.1098/rspb.2006.3721
Laiolo, P. (2005). Spatial and seasonal patterns of bird communities in Italian agroecosystems. Conserv. Biol. 19, 1547–1556. doi:10.1111/j.1523-1739.2005.004376.x
LeBrun, J. J., Thogmartin, W. E., Thompson, F. R., Dijak, W. D., and Millspaugh, J. J. (2016). Assessing the sensitivity of avian species abundance to land cover and climate. Ecosphere 7, e01359. doi:10.1002/ecs2.1359
Lehman, C. L., and Tilman, D. (2000). Biodiversity, stability, and productivity in competitive communities. Am. Nat. 156, 534–552. doi:10.1086/303402
Lien, G. (2002). Non-parametric estimation of decision makers' risk aversion. Agric. Econ. 27, 75–83. doi:10.1111/j.1574-0862.2002.tb00106.x
Lindström, S. A. M., Herbertsson, L., Rundlöf, M., Smith, H. G., and Bommarco, R. (2015). Large-scale pollination experiment demonstrates the importance of insect pollination in winter oilseed rape. Oecologia 180, 759–769. doi:10.1007/s00442-015-3517-x
Loh, W. M. e., J. (2014). Living planet report 2014: species and spaces, people and places [R. McLellan, L. Iyengar, B. Jeffries, and N. Oerlemans (Editors)]. Gland, Switzerland: WWF.
Lowe, P., Buller, H., and Ward, N. (2002). Setting the next agenda? British and French approaches to the second pillar of the common agricultural policy. J. Rural Stud. 18, 1–17. doi:10.1016/s0743-0167(01)00025-0
Mantyka-Pringle, C., Leston, L., Messmer, D., Asong, E., Bayne, E. M., Bortolotti, L. E., et al. (2019). Antagonistic, synergistic and direct effects of land use and climate on prairie wetland ecosystems: Ghosts of the past or present? Divers. Distrib. 25, 1924–1940. doi:10.1111/ddi.12990
Mantyka-Pringle, C. S., Visconti, P., Marco, M. D., Martin, T. G., Rondinini, C., Rhodes, J. R., et al. (2015). Climate change modifies risk of global biodiversity loss due to land-cover change. Biol. Conserv. 187, 103–111. doi:10.1016/j.biocon.2015.04.016
Martin, Y., Dyck, H. V., Dendoncker, N., and Titeux, N. (2013). Testing instead of assuming the importance of land use change scenarios to model species distributions under climate change. Glob. Ecol. Biogeogr. 22, 1204–1216. doi:10.1111/geb.12087
Masson-Delmotte, V., Zhai, P., Pirani, A., Connors, S., Péan, C., Berger, S., et al. (2021). IPCC climate change 2021: The physical science basis. contribution of working group I to the sixth assessment report of the intergovernmental panel on climate change. Cambridge, United Kingdom: Cambridge University Press.
Mouchet, M. A., Rega, C., Lasseur, R., Georges, D., Paracchini, M.-L., Renaud, J., et al. (2017). Ecosystem service supply by European landscapes under alternative land-use and environmental policies. Int. J. Biodivers. Sci. Ecosyst. Serv. Manag. 13, 342–354. doi:10.1080/21513732.2017.1381167
Mouysset, L. (2014). Agricultural public policy: Green or sustainable? Ecol. Econ. 102, 15–23. doi:10.1016/j.ecolecon.2014.03.004
Mouysset, L., Assa, C. R., Ay, J.-S., Jiguet, F., Lorrilière, R., Doyen, L., et al. (2019). Bioeconomic impacts of agroforestry policies in France. Land Use Policy 85, 239–248. doi:10.1016/j.landusepol.2019.02.026
Mouysset, L., and Doyen, L. (2013). How does economic risk aversion affect biodiversity? Ecol. Appl. 23, 96–109. doi:10.1890/11-1887.1
Mouysset, L., Miglianico, M., Makowski, D., Jiguet, F., and Doyen, L. (2016). Selection of dynamic models for bird populations in farmlands. Environ. Model. Assess. (Dordr). 21, 407–418. doi:10.1007/s10666-015-9494-y
Newbold, T. (2018). Future effects of climate and land-use change on terrestrial vertebrate community diversity under different scenarios. Proc. R. Soc. B 285, 20180792. doi:10.1098/rspb.2018.0792
Nicholas, K., Villemoes, F., Lehsten, E., and Brady, M.Murray Scown (2020). A harmonized and spatially explicit dataset from 16 million payments from the European Union's Common Agricultural Policy for 2015. Patterns 2, 100236. doi:10.1016/j.patter.2021.100236
Oliver, T. H., and Morecroft, M. D. (2014). Interactions between climate change and land use change on biodiversity: Attribution problems, risks, and opportunities. WIREs Clim. Change 5, 317–335. doi:10.1002/wcc.271
Ormerod, S., and Watkinson, A. (2000). Large-scale ecology and hydrology: An introductory perspective from the editors of the journal of applied ecology. J. Appl. Ecol. 37, 1–5. doi:10.1046/j.1365-2664.2000.00560.x
Pe'er, G., Bonn, A., Bruelheide, H., Dieker, P., Eisenhauer, N., Feindt, P. H., et al. (2020). Action needed for the EU common agricultural policy to address sustainability challenges. People Nat. 2, 305–316. doi:10.1002/pan3.10080
Pe'er, G., Zinngrebe, Y., Moreira, F., Sirami, C., Schindler, S., Müller, R., et al. (2019). A greener path for the EU common agricultural policy. Science 365, 449–451. doi:10.1126/science.aax3146
Pearson, R. G., Stanton, J. C., Shoemaker, K. T., Aiello-Lammens, M. E., Ersts, P. J., Horning, N., et al. (2014). Life history and spatial traits predict extinction risk due to climate change. Nat. Clim. Chang. 4, 217–221. doi:10.1038/nclimate2113
Pe’er, G., Dicks, L., Visconti, P., Arlettaz, R., Báldi, A., Benton, T., et al. (2014). EU agricultural reform fails on biodiversity. Science 344, 1090–1092. doi:10.1126/science.1253425
Pe’er, G., and Lakner, S. (2020). The EU’s common agricultural policy could be spent much more efficiently to address challenges for farmers, climate, and biodiversity. One Earth 3, 173–175. doi:10.1016/j.oneear.2020.08.004
Perrot, T., Gaba, S., Roncoroni, M., Gautier, J.-L., Saintilan, A., Bretagnolle, V., et al. (2019). Experimental quantification of insect pollination on sunflower yield, reconciling plant and field scale estimates. Basic Appl. Ecol. 34, 75–84. doi:10.1016/j.baae.2018.09.005
Peters, M. K., Hemp, A., Appelhans, T., Becker, J. N., Behler, C., Classen, A., et al. (2019). Climate–land-use interactions shape tropical mountain biodiversity and ecosystem functions. Nature 568, 88–92. doi:10.1038/s41586-019-1048-z
Pörtner, H., Scholes, R., Agard, J., Archer, E., Arneth, A., Bai, X., et al. (2021). IPBES-IPCC co-sponsored workshop report on biodiversity and climate. IPBES and IPCC, 19–23. doi:10.5281/zenodo.4782538
Quaas, M. F., Baumgärtner, S., Becker, C., Frank, K., and Müller, B. (2007). Uncertainty and sustainability in the management of rangelands. Ecol. Econ. 62, 251–266. doi:10.1016/j.ecolecon.2006.03.028
Ricketts, T. H., Regetz, J., Steffan-Dewenter, I., Cunningham, S. A., Kremen, C., Bogdanski, A., et al. (2008). Landscape effects on crop pollination services: Are there general patterns? Ecol. Lett. 11, 499–515. doi:10.1111/j.1461-0248.2008.01157.x
Robinson, R. A., and Sutherland, W. J. (2002). Post-war changes in arable farming and biodiversity in great Britain. J. Appl. Ecol. 39, 157–176. doi:10.1046/j.1365-2664.2002.00695.x
Robinson, S. K., Thompson, F. R., Donovan, T. M., Whitehead, D. R., and Faaborg, J. (1995). Regional forest fragmentation and the nesting success of migratory birds. Science 267, 1987–1990. doi:10.1126/science.267.5206.1987
Rosa, I. M., Purvis, A., Alkemade, R., Chaplin-Kramer, R., Ferrier, S., Guerra, C. A., et al. (2020). Challenges in producing policy-relevant global scenarios of biodiversity and ecosystem services. Glob. Ecol. Conservation 22, e00886. doi:10.1016/j.gecco.2019.e00886
Roscher, C., Beßler, H., Oelmann, Y., Engels, C., Wilcke, W., Schulze, E.-D., et al. (2009). Resources, recruitment limitation and invader species identity determine pattern of spontaneous invasion in experimental grasslands. J. Ecol. 97, 32–47. doi:10.1111/j.1365-2745.2008.01451.x
Sekercioglu, C. H., Daily, G. C., and Ehrlich, P. R. (2004). Proc. Natl. Acad. Sci. U. S. A. 101, 18042–18047. doi:10.1073/pnas.0408049101
Shukla, P., Skea, J., Buendia, E. C., Masson-Delmotte, V., Pörtner, H.-O., Roberts, D. C., et al. (2019). Summary for Policymakers: An IPCC Special Report on climate change, desertification, land degradation, sustainable land management, food security, and greenhouse gas fluxes in terrestrial ecosystems. Sous presse, 21–24.
Singer, A., Johst, K., Banitz, T., Fowler, M. S., Groeneveld, J., Gutiérrez, A. G., et al. (2016). Community dynamics under environmental change: How can next generation mechanistic models improve projections of species distributions? Ecol. Model. 326, 63–74. doi:10.1016/j.ecolmodel.2015.11.007
Souty, F., Dorin, B., Brunelle, T., Dumas, P., and Ciais, P. (2013). Modelling economic and biophysical drivers of agricultural land-use change. calibration and evaluation of the nexus land-use model over 1961–2006. Geosci. Model Dev. Discuss. 6, 6975. doi:10.5194/gmdd-6-6975-2013
Stoate, C., Báldi, A., Beja, P., Boatman, N., Herzon, I., van Doorn, A., et al. (2009). Ecological impacts of early 21st century agricultural change in Europe – A review. J. Environ. Manag. 91, 22–46. doi:10.1016/j.jenvman.2009.07.005
Strijker, D. (2005). Marginal lands in Europe—Causes of decline. Basic Appl. Ecol. 6, 99–106. doi:10.1016/j.baae.2005.01.001
Swinton, S. M., Lupi, F., Robertson, G. P., and Hamilton, S. K. (2007). Ecosystem services and agriculture: Cultivating agricultural ecosystems for diverse benefits. Ecol. Econ. 64, 245–252. doi:10.1016/j.ecolecon.2007.09.020
Taheri, S., García-Callejas, D., and Araújo, M. B. (2021). Discriminating climate, land-cover and random effects on species range dynamics. Glob. Chang. Biol. 27, 1309–1317. doi:10.1111/gcb.15483
Tucker, G., and FHeath, M. (1994). Birds in Europe : their conservation status. Conserv. Ser. 3. doi:10.1016/s0006-3207(96)90060-9
Visconti, P., Bakkenes, M., Baisero, D., Brooks, T., Butchart, S. H. M., Joppa, L., et al. (2015). Projecting global biodiversity indicators under future development scenarios. Conserv. Lett. 9, 5–13. doi:10.1111/conl.12159
Wissenschaftliche Beiräte, B. (2017). Climate change mitigation in agriculture and forestry and in the downstream sectors of food and timber use. Berichte über Landwirtsch. - Z. für Agrarpolit. Landwirtsch. Sonderh. 2017, 223. doi:10.12767/BUEL.V1I1.175
Wollni, M., and Andersson, C. (2014). Spatial patterns of organic agriculture adoption: Evidence from Honduras. Ecol. Econ. 97, 120–128. doi:10.1016/j.ecolecon.2013.11.010
Keywords: land-use change, ecosystem service, bioeconomic model, public policy scenario, Europe, terrestrial biodiversity, socioecological system, climate change
Citation: Guillet T and Mouysset L (2022) Productive versus environmental objectives of agricultural policies dealing with climate change: a French case study. Front. Environ. Sci. 10:889506. doi: 10.3389/fenvs.2022.889506
Received: 04 March 2022; Accepted: 11 July 2022;
Published: 20 October 2022.
Edited by:
Nebila Lichiheb, NOAA, United StatesReviewed by:
Neal Eash, The University of Tennessee, United StatesSean Pascoe, Commonwealth Scientific and Industrial Research Organisation (CSIRO), Australia
Copyright © 2022 Guillet and Mouysset. This is an open-access article distributed under the terms of the Creative Commons Attribution License (CC BY). The use, distribution or reproduction in other forums is permitted, provided the original author(s) and the copyright owner(s) are credited and that the original publication in this journal is cited, in accordance with accepted academic practice. No use, distribution or reproduction is permitted which does not comply with these terms.
*Correspondence: Lauriane Mouysset, bW91eXNzZXRAY2VudHJlLWNpcmVkLmZy