- 1Department of Ecology, Evolution and Behavior, University of Minnesota Twin Cities, Saint Paul, MN, United States
- 2Department of Biological Sciences, University of Bergen, Bergen, Norway
Freshwaters are significant contributors of greenhouse gases to the atmosphere, including carbon dioxide (CO2), methane (CH4), and nitrous oxide (N2O). Small waterbodies such as ponds are now recognized to have disproportionate greenhouse gas emissions relative to their size, but measured emissions from ponds have varied by several orders of magnitude. To assess drivers of variation in pond greenhouse gas dynamics, we measured concentrations and emissions of CO2, CH4, and N2O across 26 ponds in Minnesota, United States, during the ice-free season. The studied ponds differed in land-use, from urban stormwater ponds to natural forested ponds. The ponds were all sources of greenhouse gases, driven by large CH4 emissions (mean 704 [sd 840] mg CH4-C m−2 d−1). CO2 fluxes were variable, but on average a sink (mean −25.9 [sd 862] mg CO2-C m−2 d−1), and N2O emissions were generally low (mean 0.398 [sd 0.747] mg N2O-N m−2 d−1). Duckweed coverage on the water surfaces ranged from 0% to 100% coverage, and had the largest influence on water chemistry and greenhouse gas dynamics across the ponds. Duckweed covered ponds (ponds with greater than 85% coverage) had higher phosphorus levels and increased anoxia compared to ponds without duckweed (ponds with less than 12% coverage), leading to higher CH4 concentrations and overall greenhouse gas emissions in the duckweed ponds. Duckweed ponds had a mean emission rate in CO2 equivalents of 30.9 g C m−2 d−1 compared to 11.0 g C m−2 d−1 in non-duckweed ponds.
Introduction
Freshwater lakes and reservoirs are important sites of biogeochemical activity and emissions of the major greenhouse gases carbon dioxide (CO2), methane (CH4), and nitrous oxide (N2O). While freshwater lakes cover less than 4% of the earth’s non-glaciated land area (Verpoorter et al., 2014), they are estimated to annually emit over 0.5 Pg C of CO2 (DelSontro et al., 2018) and 0.3 Pg C of CH4 (Rosentreter et al., 2021) to the atmosphere. However, small waterbodies such as ponds remain a major uncertainty in freshwater emission estimates, and ponds are increasingly recognized as disproportionately large emitters of greenhouse gases relative to their size (Holgerson & Raymond, 2016). Ponds, which tend to be < 5 ha in size (Richardson et al., 2022), comprise over 90% of freshwater lakes by number (Downing et al., 2006) and 20% by surface area (Verpoorter et al., 2014). This makes them one of the most common terrestrial-aquatic interfaces on the landscape, and thus key hotspots of biogeochemical cycling (McClain et al., 2003).
Ponds can receive high loads of allochthonous organic carbon from the watershed relative to their small volume, and this coupled with autochthonous carbon from production can lead to high decomposition and respiration rates (van Bergen et al., 2019). High respiration rates in the water column and the sediments can consume oxygen and create anoxia, which can lead to anaerobic CO2 and CH4 production (Duc et al., 2010). Ponds tend to be especially prolific sources of CH4 emissions, given that anoxia and shallow depths can limit CH4 water-column oxidation and increase ebullition (Lazar et al., 2014; Kifner et al., 2018), a process whereby CH4 bubbles are released from the sediment. CH4 can account for much of the warming potential of pond emissions (Holgerson & Raymond, 2016; Gorsky et al., 2019), especially given that over a 100-year period CH4 has a warming potential about 37 times higher than CO2 (Derwent, 2020), or 84 times higher on a 20-years time period (Myhre et al., 2013). Denitrification under hypoxic and anoxic conditions can produce N2O, a greenhouse gas with 265 times the warming potential of CO2 over a 100-year period (Myhre et al., 2013). Denitrification potential can be high in ponds with large nitrogen inputs (Bettez & Groffman, 2012), though this may not always lead to elevated N2O production as complete denitrification can consume N2O while producing N2 (Blaszczak et al., 2018).
While recent studies have shown ponds to have the potential for extremely high greenhouse gas emissions, pond emission rates vary by several orders of magnitude, even within individual studies (Grinham et al., 2018; Gorsky et al., 2019; Peacock et al., 2019; Audet et al., 2020). This extreme variation hinders evaluating pond emissions at larger scales, and thus their role in freshwater emissions as a whole. Mechanisms driving variation in pond gas emissions are largely not understood, and there remains a lack of spatially and temporally representative pond greenhouse gas measurements (Koschorreck et al., 2020).
Much of the variation in pond greenhouse gas dynamics could stem from the many physical and biological differences in ponds across different environments. Dominant macrophyte and algal communities can vary significantly across pond ecosystems, and macrophytes are known to play a significant role in both CO2 and CH4 fluxes in aquatic ecosystems. Emergent macrophytes can directly transport gases from the sediments to the atmosphere, and can increase CH4 emissions (Desrosiers et al., 2022). Submersed macrophytes can also increase CH4 emissions by influencing methanogenesis and CH4 ebullition, even in oxygenated waters (Hilt et al., 2022). The effect of floating macrophytes on greenhouse gas emissions has not been well-studied, though large floating macrophytes such as water hyacinth have been shown to reduce CO2 emissions through increased photosynthesis (Attermeyer et al., 2016). While water hyacinth largely grows in tropical and sub-tropical waterbodies, small floating macrophytes, such as duckweed (family Lemnaceae) are practically ubiquitous in waterbodies worldwide (Tippery & Les, 2020), and are especially prevalent in small pond ecosystems (Hillman, 1961). Duckweed can form mats covering the entire surface of small ponds, and shade out other rooted macrophytes (Hillman, 1961). Despite the effects duckweed can have in aquatic ecosystems, little is known about how duckweed may directly influence greenhouse gas production and emissions in ponds.
In addition to differing macrophyte communities among different ponds, ponds can vary greatly in land-use from human-constructed ponds deigned for certain uses, to natural ponds in pristine watersheds. While natural ponds (Holgerson, 2015) and urban constructed ponds (Herrero Ortega et al., 2019; Audet et al., 2020; Goeckner et al., 2022) have been evaluated separately, no studies have compared natural and constructed ponds directly in the same study. Urban ponds often receive more nutrients and particles transported in stormwater runoff compared to natural ponds, which increases autochthonous production and eutrophication. Land-use and watershed properties may also directly impact the macrophyte communities of ponds, with eutrophication leading to the disappearance of submersed aquatic vegetation and the dominance of floating macrophytes (Khan & Ansari, 2005).
To evaluate the effects of floating macrophytes and environmental characteristics on pond greenhouse gas dynamics, this study measured both daytime concentrations and emissions of CO2, CH4, and N2O in 26 ponds during the growing season. It was hypothesized that factors influencing oxygen loss, such as surface duckweed coverage, would have the largest impact on pond greenhouse gas emissions, given the particular importance of anaerobic processes for the formation of CH4 and N2O. Physical and chemical properties were measured in ponds with and without duckweed coverage, as well as both constructed and natural ponds. To gain a complete view of greenhouse gas dynamics in the ponds, surface and bottom concentrations of CO2, CH4, N2O were measured, as well as emission rates from floating chamber measurements for CO2 and CH4.
Materials and methods
Study sites
The 26 ponds in this study were all located within a 40-mile radius within the metropolitan area of St. Paul and Minneapolis, Minnesota, United States. The ponds varied in land use type, from stormwater ponds in urban environments to natural forested ponds in preserves and parks. Ponds were divided into two categories based on origin, “natural” ponds and “constructed” ponds. While the complete history of some ponds was unknown, ponds were classified as constructed if there were known human alterations to the pond. All constructed ponds in this study are functioning stormwater ponds, managed for water regulation or the reduction of nutrient and metal exports. Daily aerial imagery at 3 m resolution from Planet Labs (Planet Team, 2017) was used to examine pond inundation, surface area, canopy cover, and floating macrophyte coverage. All ponds remain inundated year-round, and pond surface area did not detectably change within the study period. Canopy cover within a 50 m buffer around each pond was estimated using the area covered by mature trees within the buffer, using imagery from the same day each pond was sampled. Pond surface area ranged from 0.04 to 1.5 ha and pond max depth ranged from 0.3 to 1.8 m deep (Table 1).
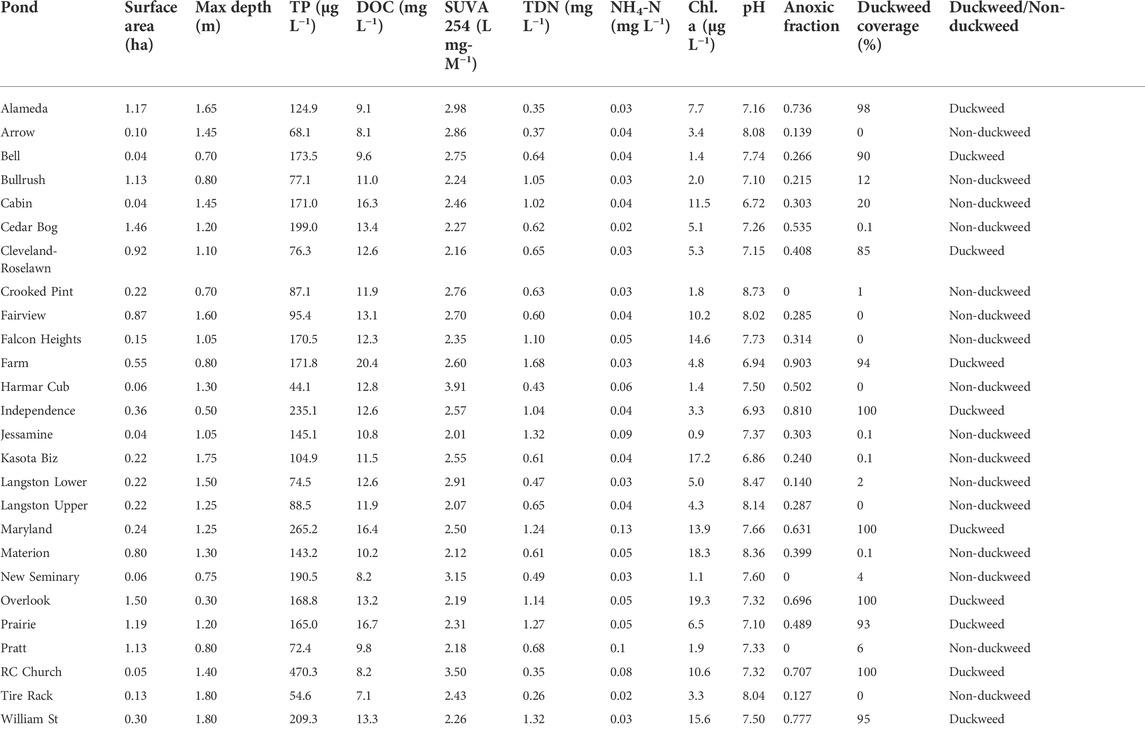
TABLE 1. Physical, chemical, and biological characteristics of the 26 study ponds. If duckweed species were present but covered negligible pond surface area, duckweed coverage was listed as 0.1%. Anoxic fraction is the anoxic sediment area divided by the pond surface area.
The ponds were all sampled once between mid-July and mid-August 2021. Ponds were sampled between 10:30 in morning and 14:00 in the afternoon, to remove as much hourly variation as possible. While diel cycles have been shown to influence both CO2 and CH4 emissions, both sample time and sample date did not show a significant trend with measured emissions (Supplementary Figure S1). Nonetheless, diel studies have shown that daytime fluxes of CO2 underestimate total daily fluxes while overestimating total CH4 fluxes (van Bergen et al., 2019; Sieczko et al., 2020). Percent cover of floating macrophytes on each pond was estimated using aerial imagery. Since surface area coverage of macrophytes can change day to day with wind speed and direction, the maximum percent cover within a week period around the sample date for each pond was used. Visual estimates of floating macrophyte coverage were also taken onsite during sampling to verify the areal estimates and assess types of floating macrophytes. Floating macrophytes were almost exclusively duckweed (lemna sp.) and watermeal (wolffia sp.), here-on referred to as “duckweed”.
Surface water samples were collected in the middle of each pond and used to measure total phosphorus (TP), dissolved organic carbon (DOC), total dissolved nitrogen (TDN), nitrate/nitrite (NO3/NO2), and ammonium (NH4). TP was measured using the molybdenum blue reaction with acid-persulfate digestion (Murphy & Riley, 1962). For DOC, TDN, NO3/NO2 and NH4 analysis water samples were passed through a muffled 0.45 μm filter. DOC and TDN was measured using a Shimadzu TOC-L model high temperature carbon-analyzer with a TNM-L module (Shimadzu Corp., Kyoto, Japan). NO3/NO2 was measured using colorimetric analysis by the cadmium reduction method, and NH4 was measured using colorimetric analysis by the salicylate/nitroprusside method using a Lachat 8500 FIA (Lachat Instruments, Loveland Colorado, United States). Spectral scans of water samples and DOC concentrations were also used to calculate specific ultraviolet absorbance at 254 nm (SUVA254) as a measure of DOC aromaticity (Weishaar et al., 2003).
Profiles were taken at the deepest point of each pond to measure water temperature, dissolved oxygen (DO), pH, chlorophyll a (chl a), and conductivity (Manta probe, Eureka Water Probes, Austin Texas, United States). Measurements were taken every 5 s, and profiles were taken slow enough to allow for measurements every 0.05–0.1 m. DO profiles were used to calculate the anoxic fraction of the pond, defined as the fraction of sediment exposed to anoxic conditions (anoxic sediment area/pond surface area) (Nürnberg, 1995). DO values under 2 mg L−1 were considered anoxic (Nürnberg, 1995). The anoxic sediment area was determined using DO profiles and pond hypsographic curves determined from bathymetry. As an example, in a 1.5 m-deep pond in which the DO profile was less than 2 mg L−1 within 0.5 m of the bottom of the pond, all sediment at a depth of 1 m or greater was considered the anoxic sediment area. Bathymetry of ponds was acquired from maps or previous measurements, however for six ponds bathymetry was not available. For these ponds bathymetry was estimated using functions in the LakeAnalyzer R package (Read et al., 2011).
Water temperature profiles were used to calculate relative thermal resistance to mixing (RTRM) as a measure of stratification strength. RTRM (unitless value) was calculated for the whole water column by taking the difference of the densities of the surface water layer (using surface water temperature) and bottom water layer (using temperature ∼5 cm above sediment) and dividing by the difference in densities of water at 4 and 5°C (Birge, 1916). An RTRM >1 implies that the water column is stably stratified (more stable at higher values), while an RTRM ≤1 implies the water column is unstable and will mix (i.e. the density difference between water layers is less than the density difference between water at 4 and at 5°C). A value of zero implies the water layers are the same density.
Greenhouse gas sampling
Greenhouse gas emissions of CO2 and CH4 were measured using a floating chamber technique (Erkkilä et al., 2018; Grinham et al., 2018; Gorsky et al., 2019). N2O fluxes proved to be too low to get reliable emission rates using the floating chamber, and therefore N2O emissions were estimated from surface water and atmospheric concentrations. The floating chamber was constructed from an inverted white 5-gallon bucket, with a headspace volume of 10.02 L within the chamber. The chamber was wrapped in Styrofoam to float on the surface of the water, with 3 cm extending below the water surface to reduce turbulence. The floating chamber was connected directly to a portable greenhouse gas analyzer (DX4040 FTIR Gas Analyzer, Gasmet Technologies Oy, Vantaa, Finland) via inlet and outlet tubing to create a closed loop system. To measure CH4 and CO2 emissions, the float was placed on the surface of the water and the pressure was allowed to equilibrate through the outlet port. The float was then connected to the gas analyzer, and the analyzer began pulling air through the loop with an approximate pumping rate of 1.5 L min−1. No alterations were made to the water surface before placing the chamber, and the chamber was placed on top of any floating macrophytes (such as duckweed) or algal mats on the surface of the pond as well as open water. Gas measurements were taken every 5 s, and incubations lasted at least 5 min, and up to 10 min if rates were low. Chamber incubations were taken at three locations on each pond, one near the shoreline, one at the deep spot, and one in between those two points. Ponds were sampled with either a kayak or canoe, with the chamber floating freely connected by approximately 2 m of tubing. If the boat had to be anchored due to wind, the anchor was placed far away from the floating chamber to avoid ebullition events caused by disturbing the sediment.
Concentrations of CO2, CH4, and N2O in both the surface water and bottom water were measured using the headspace technique (McAuliffe, 1971). Surface samples were collected 5–10 cm below the surface, and bottom water samples were collected approximately 10 cm above the sediment using a Van Dorn water sampler. Van Dorn sampling was done slowly and carefully to not produce bubbles or cause turbulence, and after retrieval one end was opened to directly collect the bottom water sample. For all samples, 125 ml of water was collected in a 140 ml plastic syringe. Any bubbles formed when drawing water collected in the top of the syringe, and then were removed by reducing the syringe volume to 105 ml. Atmospheric air (32.5 ml) was introduced into the syringe and then vigorously shaken for 2 minutes. Thereafter, 30 ml of the headspace was transferred into a separate syringe, and immediately injected onsite into the Gasmet portable gas analyzer, equipped with a closed loop injection system (Wilkinson et al., 2019).
Greenhouse gas calculations
The diffusive flux for all gases across the air-water interface can be expressed as:
Where F is the gas flux, k is the gas transfer velocity, Cw is the concentration of gas in the surface water, Csat is the concentration of gas in the surface water at equilibrium with the overlying atmosphere. For CO2 and CH4, gas flux (F) was calculated directly with the floating chamber measurements. For CO2, concentrations always increased or decreased linearly over time, and a simple linear regression method was used to calculate the flux based on the slope of linear increase (Xiao et al., 2014). For CH4, ebullition led to a non-linear increase in CH4 concentration when bubbling occurred (example shown in Supplementary Figure S2). With the 5 s measurement resolution, emission from ebullition events could be separated from the diffusive flux due to the high sampling frequency, as shown by Xiao et al. (2014). First, the CH4 diffusion rate was calculated by fitting a linear regression to a long straight segment of the sample curve (Supplementary Figure S2). Multiplying this diffusion rate by the total sample time gave the CH4 concentration in the chamber due to diffusion. CH4 concentration due to ebullition was the surplus CH4 concentration, calculated by subtracting the diffusion concentration and original background concentration from the total concentration at the sampling endpoint (Supplementary Figure S2). The Ideal Gas Law was used to convert concentrations to mass, and all emission rates for CO2 and CH4 are expressed in mg of carbon (i.e. CO2-C, CH4-C).
As shown by Eq. 1, the rate of gas flux will change over time as the concentration changes inside the floating chamber (as Csat increases or decrease). While linear models are often used calculating gas fluxes from floating chambers (e.g. Xiao et al., 2014; Attermeyer et al., 2016; Gorsky et al., 2019), it has been shown that exponential models may be more appropriate, and linear models may underestimate fluxes by 10—30% for short incubations (5—25 min), and by over 50% for hour long incubations (Xiao et al., 2016). To test the use of a linear or exponential model, emission rates were calculated using both linear and exponential models for every CO2 incubation (n = 75) and every CH4 incubation where ebullition did not occur (n = 26), and model goodness of fits were compared using coefficient of determination R2. Contrary to the results from Xiao et al. (2016), the linear models had a higher R2 value for most incubations, and initial slope values did not significantly differ between linear and exponential models (Supplementary Table S1). Therefore, linear models were chosen to calculate CO2 and CH4 emission rates from the float chambers.
To calculate the concentration of CO2, CH4, and N2O in the surface and bottom water, gas concentrations in the sample headspace were first calculated using the following equation (Wilkinson et al., 2019):
Where Xheadspace is the concentration of the sample headspace, Vl is the volume of the closed loop system, Vs is the volume of the injected sample, ∆X is the change in gas concertation after sample injection, and X0 is the initial gas concentration. Inputs from the added atmospheric air was subtracted out from the headspace concentration using onsite measurements of atmospheric gas concentrations (usually around 400 ppm CO2, 2 ppm CH4 and 0.33 ppm N2O). The partial pressure of gas in the headspace was used to calculate the moles of dissolved gas in the water (molaq) according to Henry’s law, and this was added to the moles of gas in the headspace (molheadspace) and divided by the original sample volume to find the concentration of gas in the original sample (Cw) (Johnson et al., 1990):
Where Pheadspace is the partial pressure of gas in the headspace (atm), KH is Henry’s constant (mol l−1 atm−1), R is the universal gas constant (0.082, L∙atm mol−1 K−1), T is the temperature (K), Vheadspace is the volume of the headspace (L), and Vw is the volume of the water sample (L). Henry’s constants were calculated for each gas: CO2 (Weiss, 1974), CH4 (Wiesenburg & Guinasso, 1979), and N2O (Weiss & Price, 1980) and corrected for water temperature and pressure. Constants were also corrected for salinity, as particularly the bottom waters of urban ponds can have high salinity due to road salt inputs. The highest recorded conductivity in the study ponds was 10,000 μS cm−1. Conductivity for each sample was converted to salinity using equations from Fofonoff and Millard Jr (1983), and Hill et al. (1986). Molar concentrations for all gases were then expressed in mg of carbon or nitrogen (i.e. CO2-C, CH4-C, N2O-N).
Using Eq. 1, the estimate Cw, and calculating Csat according to Henry’s law, the gas transfer velocity (k) was estimated for each pond. Since CO2 chamber fluxes were often influenced by floating macrophyte photosynthesis, CH4 diffusion fluxes and surface concentrations were used with Eq. 1 to calculate k values specific to CH4 and the sample temperature. k coefficients were then normalized to k600 values for comparison among gases and at different water temperatures, with the following equation (Jähne et al., 1987):
Where kg,T and Scg,T are the gas transfer velocity and Schmidt number of a given gas (in this case CH4) and temperature (Wanninkhof, 1992). Temperature specific Sc values were calculated using equations in Wanninkhof (1992), and an n of 2/3 was used for all calculations, as this factor is appropriate for a smooth liquid surface (Deacon, 1981). These site-specific k600 values were then used to estimate emissions for N2O, based on N2O surface concentrations and using Eq. 1.
For each site, equilibrium concentrations of each gas were calculated with Eq. 3, using the gas and temperature specific KH (mol l−1 atm−1) and the partial pressure of the gas in the atmosphere measured at each site.
To evaluate the total greenhouse gas effect of emitted gases, gas emissions (floating chamber method for CO2, CH4, concentration method of N2O) were then converted to CO2eq (mg C m−2 d−1) on a mass basis by multiplying the CH4 emissions by 37 (Derwent, 2020) and N2O emissions by 265 (Myhre et al., 2013).
Data analysis and statistics
To test for differences in the chamber emission estimates among the three sample sites (near-shore, intermediate point, and the deep point) on each pond, linear mixed effect models were used with ponds included as a random effect (Zuur et al., 2009). For the rest of analyses, chamber emission estimates from the three sample sites were averaged, to provide a single mean CO2 and CH4 emission rate for each pond. To evaluate individual relationships among all greenhouse gas metrics and environmental variables, a Pearson correlation matrix was used. Relationships among all variables were first visually inspected with scatter plots to check for a linear relationship, and then correlations were calculated with the raw values. Environmental variables included surface area, max depth, TP, DOC, SUVA254, TDN, NH4, chl. a, anoxic fraction, RTRM, duckweed coverage, and canopy coverage.
To further investigate the effect of duckweed on pond greenhouse gas emissions, ponds were classified as “duckweed ponds” and “non-duckweed ponds” using a k-means cluster analysis of pond duckweed coverage, resulting in 10 duckweed ponds and 16 non-duckweed ponds. Unequal variance t-tests were used for all comparisons between natural/constructed categories and duckweed/non-duckweed categories, as variances were not homogeneous between categories for many variables. To test for interactions among the categorical variables (natural/constructed and duckweed/non-duckweed), two-way anova tests were used. p-values < 0.05 were considered significant for all analyses. All statistics were performed using R statistical software (R 4.4.1, R Core Team 2021). The lme4 package was used for mixed effect models along with the lmerTest package to test for significant differences between sampling sites, and the stats package was used for the unequal variance t-tests, k-means cluster analysis, and two-way anova tests.
Results
Pond characteristics
The 26 ponds varied widely in water chemistry and physical characteristics (Table 1). The ponds were generally small and shallow with a mean (range) surface area of 0.51 (0.04–1.5) ha and a mean max depth of 1.2 m (0.3–1.8). Most ponds were high in total phosphorus (TP) (mean 150 [44—470] μg L−1), dissolved organic carbon (DOC) (mean 12 [7.1–20] mg L−1)), and total dissolved nitrogen TDN) (mean 0.79 [0.26–1.7] mg L−1). All but one pond (New Seminary) had undetectable levels of NO2/NO3 (below 10 μg L−1). Most ponds showed evidence of stratification, with relative thermal resistance to mixing (RTRM) greater than one in all ponds (mean RTRM of 77, range of 6.8–180).
Natural ponds and constructed ponds did not significantly differ in TP levels (p = 0.66), but natural ponds did have significantly higher levels of DOC and TDN (p = 0.045, 0.016). On average, natural ponds had a larger surface area than constructed ponds (0.92 vs. 0.32 ha, p = 0.017), but constructed ponds had a deeper max depth (1.3 vs. 0.97 m, p = 0.14), though not significantly.
Duckweed coverage on the ponds ranged from 0 to 100%, though most ponds had either close to no duckweed present or almost full duckweed coverage (Table 1). Ponds were split into two duckweed categories using k-means cluster analysis, and duckweed ponds had an average duckweed coverage of 95.5% (n = 10), while non-duckweed ponds had an average duckweed coverage of 2.84% (n = 16). Compared to non-duckweed ponds, duckweed ponds had significantly higher TP concentrations (p = 0.023), significantly lower surface oxygen concentrations (p = 0.037), and a significantly larger anoxic fraction (p < 0.001; Table 2). There were no significant interactions for environmental variables or greenhouse gas metrics between the natural/constructed and duckweed/non-duckweed categories.
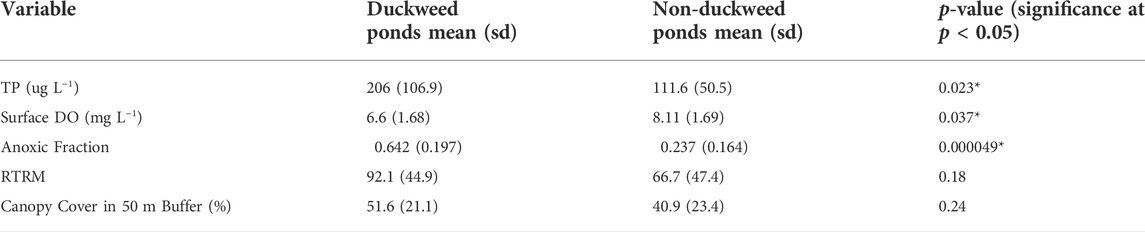
TABLE 2. Differences in environmental variables between duckweed ponds and non-duckweed ponds. Ponds were categorized as duckweed or non-duckweed by k-means clustering, with duckweed ponds having an average coverage of 96%, and non-duckweed ponds having an average coverage of 3%.
Greenhouse gas concentrations and emissions
Both concentrations in the surface and bottom water and emissions varied by several orders of magnitude across the ponds for each greenhouse gas (Table 3). In the surface water, mean concentrations of gases across all ponds were 2.03 mg CO2-C L−1, 0.250 mg CH4-C L−1, and 0.501 µg N2O-N L−1. Mean concentrations in the bottom water were 10.3 mg CO2-C L−1, 1.38 mg CH4-C L−1, and 0.313 µg N2O-N L−1 (Table 3). The difference between surface and bottom concentrations was significant for each gas, with CO2 concentrations significantly higher in the bottom water (mean difference 8.24 mg CO2-C L−1, p = 0.002), CH4 concentrations significantly higher in the bottom water (mean difference 1.13 mg CH4-C L−1, p = 0.002), and N2O concentrations significantly lower in the bottom water (mean difference -0.188 µg N2O-N L−1, p = 0.017). Equilibrium concentrations were on average 162 μg L−1 for CO2-C, 0.034 μg L−1 for CH4-C, and 0.24 μg L−1 for N2O-N, meaning that the ponds were generally super saturated with all three greenhouse gases. In the surface water, CO2 concentrations were on average 11-fold supersaturated, CH4 concentrations were on average 7,000-fold supersaturated, and N2O concentrations were on average 2-fold supersaturated.
Emission rates using the floating chamber method did not significantly vary by location within each pond (Supplementary Figure S3), with no significant differences between the shallow, middle and deep locations (p = 0.245, 0.782, and 0.315 for CO2 emission, CH4 diffusion, and CH4 ebullition, respectively). Though not significant, CH4 ebullition emissions were highest at the near-shore shallow location, while CH4 diffusion emissions were highest at the deep point location (Supplementary Figure S3). When all three floating chamber incubations were averaged to get a mean rate for each pond the mean emission rates for CO2 and CH4 were −25.9 mg CO2-C m−2 d−1 and 705 mg CH4-C m−2 d−1, with mean rates of 219 mg CH4-C m−2 d−1 for diffusion and 484 mg CH4-C m−2 d−1 for ebullition. Using surface concentrations and calculated k600 values, the average emission rate for N2O was 0.398 mg N2O-N m−2 d−1. The mean k600 across all ponds was 1.12 m d−1 but ranged from 0.240—2.82 m d−1 (Table 3). k600 did not significantly correlate with any environment variables but was lower in duckweed ponds compared to non-duckweed ponds (0.924 vs 1.24 m d−1), though not significantly (p = 0.251).
The mean total daytime emission rate for all three greenhouse gases in CO2 equivalents was 26,100 mg C m−2 d−1, with CH4 accounting for over 94% of the total emissions in CO2 equivalents on average. CO2 accounted for about 4% of the total emissions in CO2 equivalents, but ranged from a sink of −12%–57%. N2O accounted for 2.4% of the total emissions in CO2 equivalents, ranging from a sink of −2.2%–11% (Table 3).
Predictors of greenhouse gases
CO2 emission rates from the floating chamber method was significantly negatively correlated with TP, chl. a, anoxic fraction, RTRM, and duckweed coverage, with the strongest correlations being duckweed, and chl. a (Table 4; Figure 1). However, surface concentrations of CO2 were significantly positively correlated with TP and anoxic fraction, as well as ammonium concentration (Table 4; Figure 1).
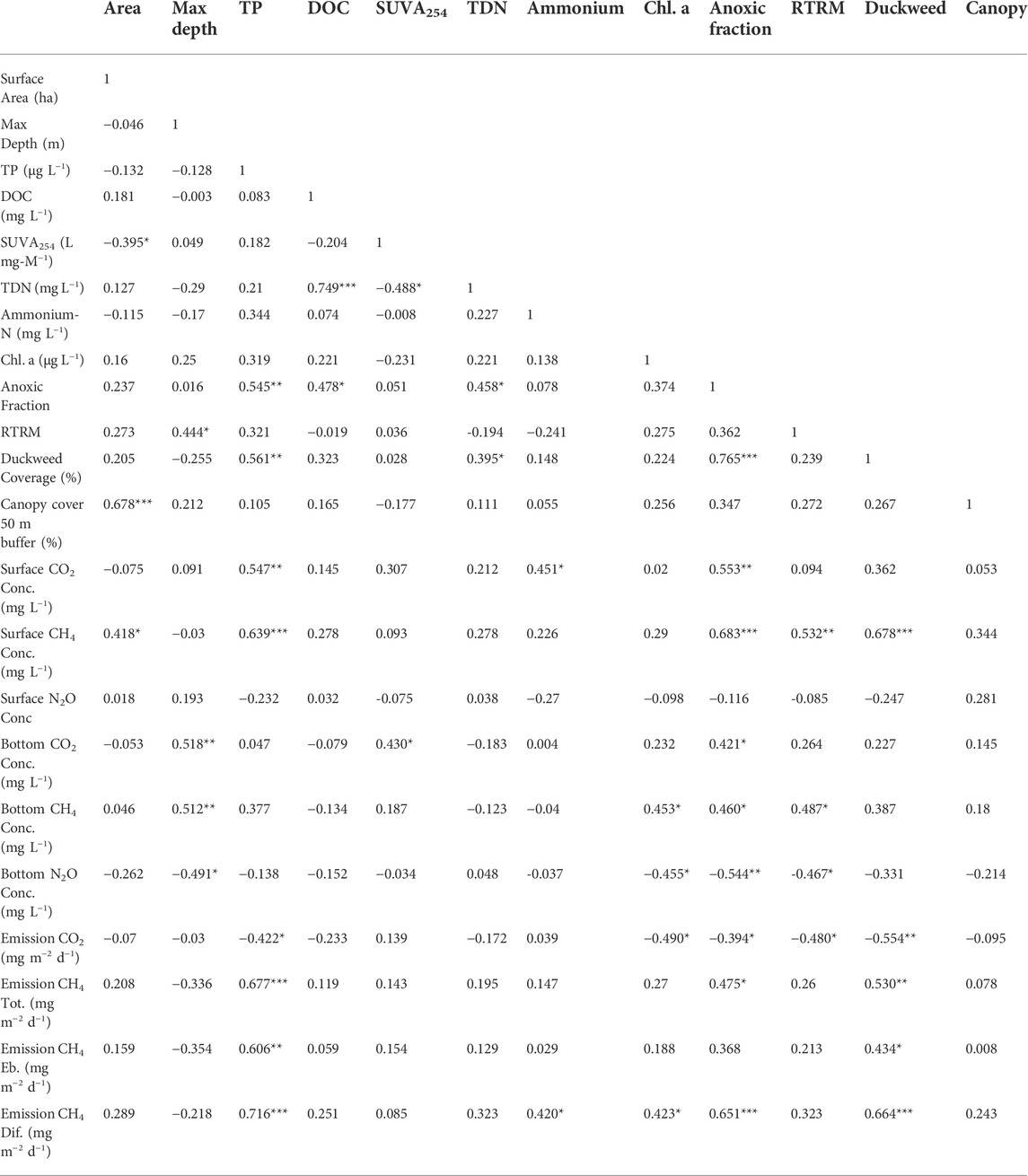
TABLE 4. Pearson correlation coefficients between environmental variables and greenhouse gas metrics for all ponds (n = 26 for all variables). * indicates significance (*p < 0.05, **p < 0.01, ***p < 0.001).
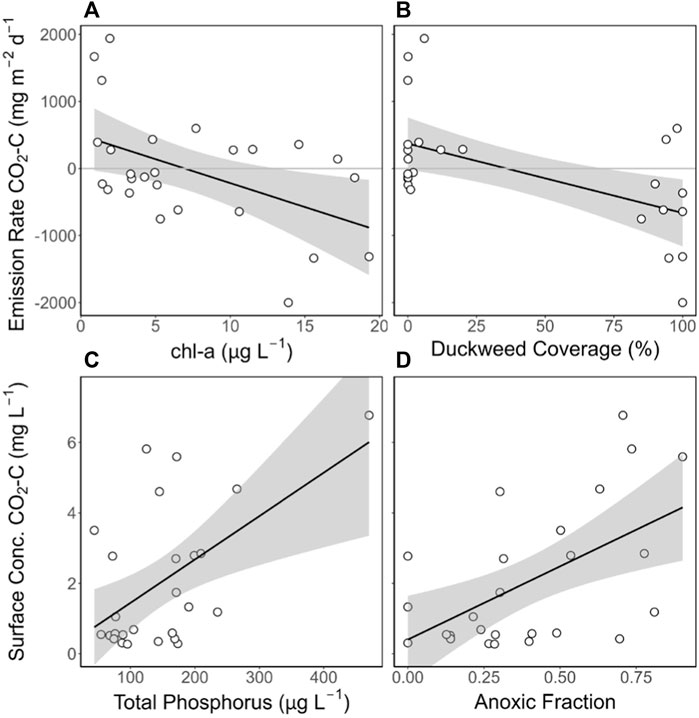
FIGURE 1. CO2 emissions from the floating chamber and CO2 surface concentrations were significantly correlated with different variables. Scatterplots show significant correlations for all ponds (n = 26) between (A) CO2 emission rate and chl-a (y = -71.28 + 496.25, R2 = 0.22, p = 0.016) (B) CO2 emission rate and duckweed coverage (y = -10.34 + 371.83, R2 = 0.31, p = 0.0033) (C) CO2 surface concentration and total phosphorus (y = 0.01x + 0.21, R2 = 0.30, p = 0.0039), and (D) CO2 surface concentration and anoxic fraction (y = 4.15x + 0.41, R2 = 0.38, p = 0.001).
CH4 emission rates and surface concentrations correlated with similar variables, with surface CH4 concentrations significantly positively correlated with surface area, TP, RTRM, anoxic fraction, and duckweed coverage. For emission rates of CH4, total emission, ebullition, and diffusion were all significantly positively correlated with TP, anoxic fraction, and duckweed coverage, with ebullition also negatively correlated with maximum depth, and diffusion also positively correlated with ammonium and chl-a (Table 4; Figure 2).
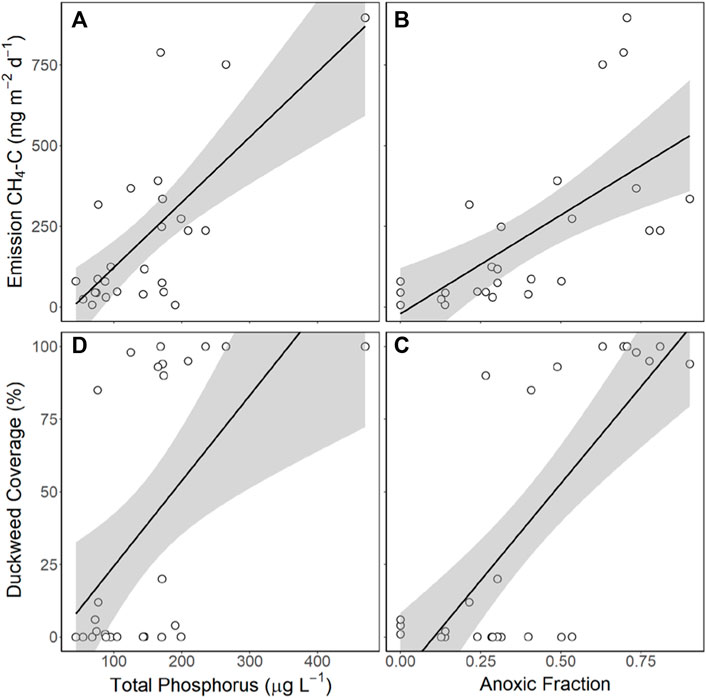
FIGURE 2. CH4 emissions and duckweed coverage were both significantly correlated with total phosphorus and anoxic fraction. Scatterplots show significant correlations for all ponds (n = 26) between (A) CH4 emission rate and total phosphorus (y = 2.02x—79.33, R2 = 0.51, p < 0.0001) (B) CH4 emission rate and anoxic fraction (y = 611.34x—20.85, R2 = 0.44, p = 0.0002 (C) duckweed coverage and total phosphorus (y = 0.29x—4.94, R2 = 0.32, p = 0.0028), and (D) duckweed coverage and anoxic fraction (y = 133.3x - 13.87, R2 = 0.51, p < 0.0001).
N2O surface concentrations were not significantly correlated with any environmental variables. All three greenhouse gas bottom concentrations were correlated with maximum depth (positively for CO2 and CH4, negatively for N2O). CO2 bottom concentration was also positively correlated with SUVA values, and N2O bottom concentration was negatively correlated with chl-a, anoxic fraction, and RTRM (Table 4).
Duckweed coverage was significantly correlated with many of the greenhouse gas metrics, as well as significantly positively correlated with TP and anoxic fraction (Table 4; Figure 2). Duckweed ponds had significantly higher CH4 emissions for both total emission (p = 0.04) and diffusion (p = 0.0085), but not ebullition (p = 0.095). Duckweed ponds also had significantly lower CO2 emissions (p = 0.0055; Figure 3). For greenhouse gas concentrations, duckweed ponds had significantly higher CH4 surface concentrations (p = 0.004), and significantly lower N2O bottom concentrations (p = 0.043; Figure 4).
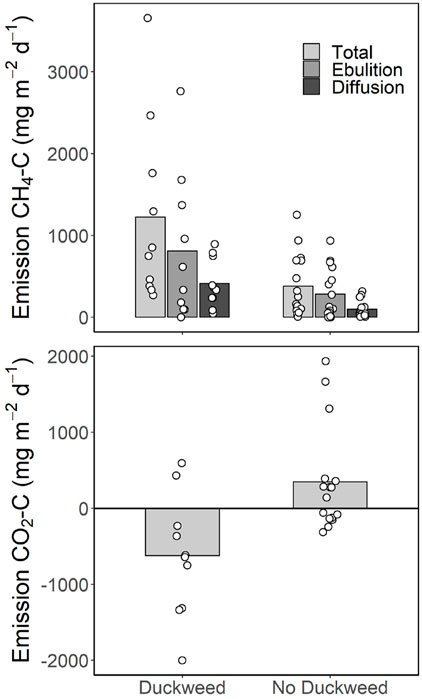
FIGURE 3. Daily emission rates of CH4 and CO2 for duckweed and non-duckweed ponds. Significant differences between duckweed ponds and non-duckweed ponds are total CH4 emission (p = 0.04), diffusion CH4 emission (p = 0.0085), and CO2 emission (p = 0.0055).
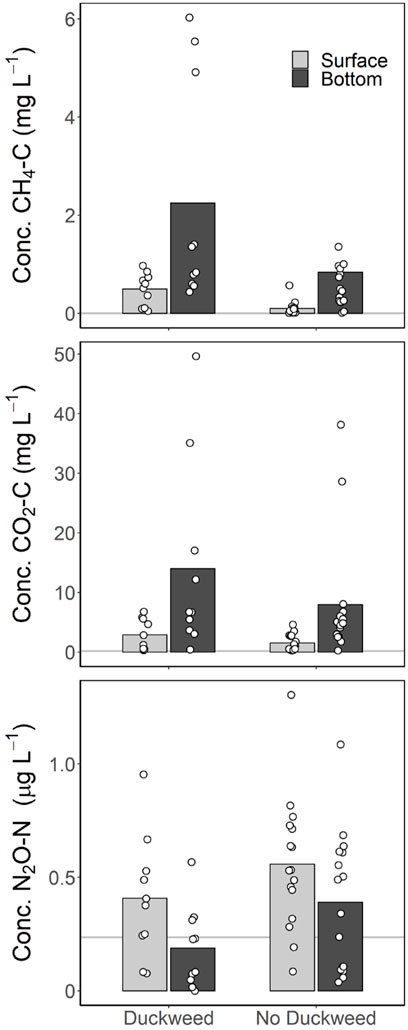
FIGURE 4. Surface and bottom concentrations of CH4, CO2, and N2O for duckweed and non-duckweed ponds. Significant differences between duckweed ponds and non-duckweed ponds are surface CH4 concentration (p = 0.0040), and bottom N2O concentration (p = 0.043). Grey horizontal lines represent the equilibrium concentration.
Discussion
Greenhouse gas concentrations and emissions
Most of the 26 ponds in this study were super-saturated with all three greenhouse gases during the study period. Concentrations of greenhouse gases in the surface water were comparable to other recent studies of pond greenhouse gases, including ponds in Connecticut (mean of 4.32 mg CO2-C L−1 and 0.396 mg CH4-C L−1 (Holgerson, 2015)), and ponds in Denmark (mean of 1.94 mg CO2-C L−1, 0.044 mg CH4-C L−1, and 0.8 µg N2O-N L−1 (Audet et al., 2020)). However, surface concentrations of CO2 and CH4 were higher than values typically reported for lakes. In a survey of 1,835 lakes, the mean CO2 concentration of the upper 10% of the samples were 16-fold above atmospheric equilibrium, while the average of all lakes was about 2-fold above equilibrium (Cole et al., 1994). For the ponds in this study, the highest surface CO2 concentration was 40-fold above atmospheric equilibrium, while the average of all 26 ponds was 11-fold above equilibrium. For CH4, a survey of surface methane concentrations from 48 lakes had a mean concentration of 8.3 µg CH4-C L−1 with 27.9 µg CH4-C L−1 as the highest concentration (Bastviken et al., 2004). The ponds in this study had an average surface concentration of 250 µg CH4-C L−1, showing ponds can be extreme hotspots of CH4 production. These high CH4 concentrations may also signal the lack of CH4 oxidation in ponds, potentially driven by anoxia and/or a lack of other oxidants such as nitrate, or shallow depth that allows for rapid transport of CH4 from the sediment to the surface (Bastviken et al., 2004). Surface N2O concentrations were not much higher than atmospheric equilibrium, and four of the 26 ponds were undersaturated in surface N2O. These ponds overall had lower surface N2O concentrations compared to many lakes. A study of 15 Swiss lakes found an average of 434% saturation for surface N2O (Mengis et al., 1997), while the ponds in this study had an average saturation of 209%.
Bottom water concentrations of CO2 and CH4 were on average much higher than surface concentrations, while N2O concentrations were often lower in the bottom waters. While all ponds were less than 1.8 m deep, all ponds still showed evidence of stratification, with relative thermal resistance to mixing (RTRM) greater than one in all ponds during the day. Thus, many ponds had substantial storage of CO2 and CH4 in the bottom waters, as well as undersaturation of N2O. Bottom water concentrations of greenhouse gases are less often measured or reported for lakes and ponds, but the ponds in this study had high concentrations of CO2 and CH4 that compare to or exceeded anoxic hypolimnion concentrations of stratified lakes. Hypolimnion concentrations of CO2 from northern lakes can reach 3.6 to 5.4 mg CO2-C L−1 (Ducharme-Riel et al., 2015), while these study ponds had a maximum bottom CO2 concentration of 49 mg CO2-C L−1 (mean 10.3 mg CO2-C L−1). The highest hypolimnion CH4 concentrations in a study of three Wisconsin lakes in late summer ranged from 3.6—8.4 mg CH4-C L−1 (Bastviken et al., 2008), while the highest bottom concentrations in these study ponds was 6 mg CH4-C L−1 (mean 1.38 mg CH4-C L−1). This shows that some small ponds can still build up large concentrations of CO2 and CH4 in the bottom waters, which likely are emitted during fall turnover or other mixing events. Intermittently mixed ponds may mix during the night or early morning when the surface water cools (Holgerson et al., 2022), and nighttime emissions could be higher due to mixing events in some of these ponds. N2O concentrations were on average lower in the bottom waters compared to the surface waters, and 14 of the 26 ponds were undersaturated in the bottom waters, compared to only four ponds undersaturated in the surface waters. This pattern agrees with many findings for lakes and reservoirs, where N2O concentrations peak in the surface water or oxic-anoxic interface, and are undersaturated in the permanently anoxic part of the hypolimnion (Mengis et al., 1997; Beaulieu et al., 2015).
CO2 using floating chamber estimates suggested that the ponds are a net sink (Table 3), though these ponds were sampled during the day and in the peak growing season and this likely does not reflect overall daily or annual CO2 fluxes from these ponds. Fluxes of CO2 would likely be the lowest during day when photosynthesis is high and would peak during the night when respiration predominates. Therefore, the daily CO2 fluxes measured in these ponds are likely underestimated due to the lack of nighttime measurements. The negative fluxes of CO2 contrasted with the CO2 supersaturation in the surface waters of most ponds, due to duckweed creating a CO2 sink above the surface of the duckweed covered ponds. Despite middle floating chamber incubations and surface concentrations being measured in the same spots, using surface concentrations to estimate CO2 fluxes would have greatly overestimated daytime CO2 emissions for the duckweed covered ponds.
Fluxes of CH4 were always positive, and the CH4 emission rates from these ponds were extremely high for freshwater ponds and lakes. CH4 emissions have been shown to peak during the day and regress at night (Sieczko et al., 2020), and daytime sampling of these ponds may overestimate daily emission rates. Nonetheless, ponds may mix at night due to convective cooling unlike larger lakes (Andersen et al., 2017), which may lead to higher emissions during nighttime as gases escape from the bottom waters. CH4 emissions also peak during the growing season (van Bergen et al., 2019), and therefore the CH4 emission rates found in these study ponds may represent the peak emission rates that these ponds experience. However, mixing during the shoulder seasons could also lead to the release of CH4 from the bottom waters, and fall and spring CH4 emission rates can be higher than other seasons (Riera et al., 1999; Jansen et al., 2019). Mean total CH4 emissions from these ponds were among the highest reported for studies involving multiple ponds (Table 5). Diffusive CH4 emission were high compared to a global assessment of lakes and ponds, where waterbodies less than 1 ha in size had a mean diffusive emission rate of 21.8 mg CH4-C m−2 d−1 (Holgerson & Raymond, 2016). However, emissions were similar to diffusive CH4 emissions found in Virginia stormwater ponds (mean 271.8 mg CH4-C m−2 d−1) (Gorsky et al., 2019). Ebullition CH4 emissions are less often measured in ponds, and ebullition rates are highly variable both spatially and temporally. Ebullition CH4 emissions from these study ponds were higher than mean ebullition fluxes in Canadian ponds (mean 55.2, max 204 mg CH4-C m−2 d−1) (DelSontro et al., 2016), but within a similar range of ponds across Canada and Missouri (highest median pond ebullition rate of 485 mg CH4-C m−2 d−1) (Baron et al., 2022). Ebullition rates can be strongly correlated with temperature (DelSontro et al., 2016), and sampling in mid-summer during the day likely facilitated the peak ebullition rates observed from these ponds.
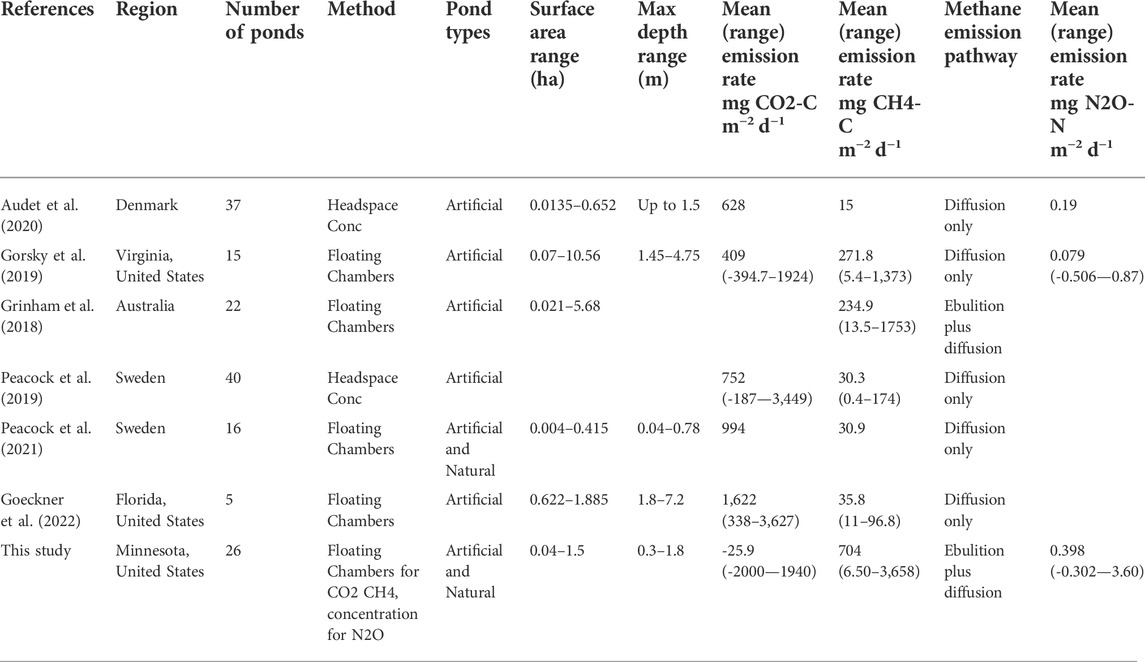
TABLE 5. Average daily greenhouse gas emissions reported from recent studies including multiple ponds. All rates converted to mg C/N m−2 d−1, for comparison.
The mean gas transfer velocity, k600, of the study ponds was high but many of the ponds fell within the range of other measurements of k600 in small, shallow waterbodies (Xiao et al., 2014; Holgerson et al., 2017). Many studies that use a literature value of k600 to calculate greenhouse gas fluxes based on surface concentrations use a k600 value of less than 1 m d−1 for small waterbodies, such as the 0.36 m d−1 used by Holgerson and Raymond (2016). The high average k600 in these ponds of 1.12 m d−1 was driven by a few ponds with exceptional large k600 values (up to 2.82 m d−1), and the median value of 0.89 m d−1 is closer to what studies assuming a constant k600 may use for small waterbodies. Gas transfer velocities can be extremely variable, and the high k600 values seen in these ponds may mean that assuming a low constant k600 could underestimate greenhouse gas emissions based on surface water concentrations.
Environmental predictors of greenhouse gases
CO2 concentrations and emissions correlated with similar variables, but in opposite directions. While surface CO2 concentrations were significantly positively correlated with TP, CO2 emissions were significantly negatively correlated with TP. This is likely due to a relationship between duckweed and TP, with phosphorus facilitating duckweed growth (Lasfar et al., 2007), that then reduces CO2 emissions. Neither CO2 concentrations nor emissions were correlated with DOC concentration, a relationship that has been found in many lakes (Raymond et al., 2013). Surface CO2 concentrations did correlate negatively with surface oxygen concentrations (r2 = 0.46, p = 0.00014), a relationship that has been shown to best predict CO2 concentrations in ponds (Kankaala et al., 2013; Holgerson, 2015). Low oxygen is likely consequence of the combination of high rates of aerobic respiration in ponds, where sediment respiration can affect the whole water column (Holgerson, 2015), and low ventilation rates, especially in the systems that were protected from wind and with high abundance of duckweed.
Both CH4 concentrations and emissions were predicted by similar variables. CH4 diffusion and TP concentration was the strongest correlation among any greenhouse gas metric and any environmental variable, and TP also correlated with ebullition rates and surface CH4 concentrations. This trend agrees with previous research that showed higher CH4 emissions in more eutrophic lakes and ponds (DelSontro et al., 2016, 2018; Beaulieu et al., 2019). While it is unknown if high phosphorus levels stimulate CH4 production directly, excess phosphorus likely helps create conditions conducive to CH4 production, including stimulating algal growth, which when degraded can lead to more labile organic matter and increased anoxia (Davidson et al., 2018). The pond anoxic fraction was also significantly positively correlated with all CH4 gas metrics. As methanogenesis is primarily an anoxic process, more anoxic water can lead to increased CH4 formation in the water column and the sediments, as well as decreased methane oxidation (Bastviken et al., 2004).
While no environmental variables correlated with surface N2O, N2O concentrations from the ponds in this study compare well to other studies that have not found significant N2O emissions from ponds (Singh et al., 2005; Audet et al., 2020). Despite high nutrients in these ponds, many which are hyper-eutrophic based on phosphorus concentrations (Carlson, 1977), there was almost no detectable levels of NO3/NO2 in any of the ponds, which can be a strong driver of N2O emissions (Mccrackin & Elser, 2010; Beaulieu et al., 2015; Wang et al., 2021). Furthermore, almost all of the ponds were stratified to some degree during the day, similar to conditions in Canadian farm ponds that became N2O sinks in late summer (Webb et al., 2019).
There was no significant difference in greenhouse gas concentrations or emissions between constructed ponds and natural ponds, which was likely due to very similar water chemistry on average between natural and constructed ponds. There were no significant differences in TP among constructed and natural ponds, highlighting that most ponds are often highly eutrophic, even among different land use types. While there can be many reasons why constructed ponds may function differently compared to natural ponds (Clifford & Heffernan, 2018), constructed ponds can vary greatly in physical and chemical characteristics just like natural ponds, potentially mitigating differences between the two at larger scales.
Impacts of duckweed
Duckweed coverage had a discernable effect on the physical and chemical states among ponds, as well as a significant effect on all three greenhouse gases. Among the 26 ponds, conditions either supported the complete coverage of duckweed over the pond surface, or ponds were not suitable for duckweed to have significant growth. Conditions for duckweed growth may depend on terrestrial canopy cover to block wind, as well as available nutrients (Hillman, 1961) (Table 4). Very few ponds had only partial duckweed coverage, though larger ponds with duckweed often had some open water if there was any wind. Duckweed coverage had clear effects on oxygen levels, with duckweed ponds having significantly less surface oxygen and a larger anoxic fraction (Tables 3, 4). Duckweed mats are known to reduce oxygen levels in small waterbodies (Pokorny & Rejm, 1983; Ceschin et al., 2019), likely due to duckweed shading out photosynthetic organisms in the water column and leading to almost no oxygen production within the pond. A blanket of duckweed coverage could also reduce atmospheric exchange of oxygen, and duckweed ponds did have slightly lower mean gas transfer velocity, though not significantly. Stratification was also stronger in duckweed ponds, though not significantly (Table 4), and this could also play a role in developing a more anoxic hypolimnion. Duckweed ponds were also significantly higher in TP (Tables 3, 4), likely due to increased anoxia which led to a positive feedback loop by stimulating further duckweed growth (Lasfar et al., 2007). Greater anoxia can stimulate higher rates of internal loading of phosphorus from the sediments (Taguchi et al., 2020) (Figure 5).
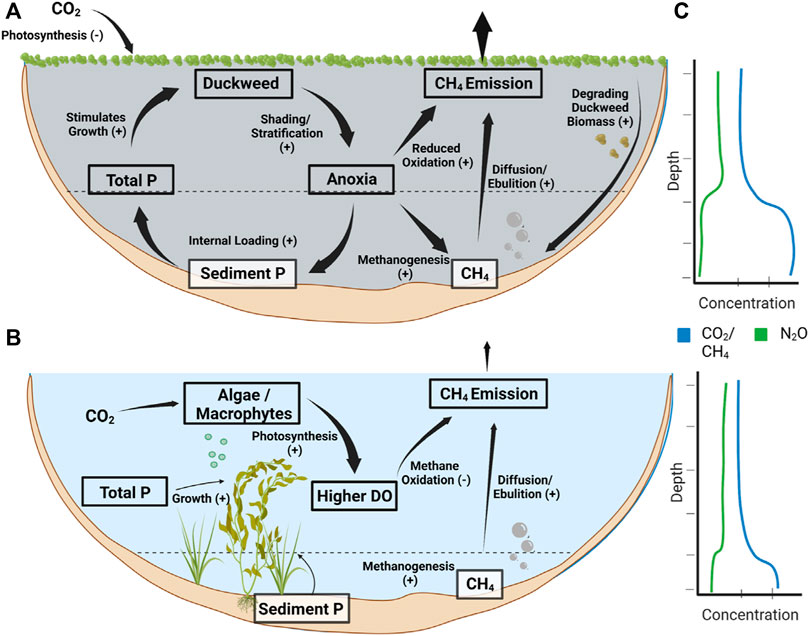
FIGURE 5. A conceptual diagram of the effect of duckweed on pond greenhouse gas emissions. (A) Duckweed can create a positive feedback loop that promotes anoxia, phosphorus loading, and further duckweed growth. While duckweed can consume CO2, high phosphorus levels and increased anoxia can promote CH4 production, reduce CH4 oxidation, and lead to high CH4 emissions. (B) Non-duckweed ponds have photosynthesis occurring within the water column, leading to higher DO, more methane oxidation, and less methane production. (C) Conceptual profiles of dissolved gases in duckweed and non-duckweed ponds.
Through these physical and chemical effects on the water column, duckweed coverage created two distinct states among the 26 ponds in this study. The increased anoxia and high phosphorus concentrations promoted by duckweed coverage also coincided with increased CH4 emissions from duckweed ponds (Figure 2), and duckweed ponds had mean CH4 emissions over twice that of non-duckweed ponds for each of total, ebullitive, and diffusive emissions (Figure 3). Degrading duckweed may also create a large pool of labile organic matter in duckweed ponds similar to submersed macrophytes (Hilt et al., 2022), which could further promote methanogenesis and ebullition (West et al., 2012; Zhou et al., 2019). Duckweed ponds were on average CO2 sinks, despite having higher CO2 concentrations in the surface waters compared to non-duckweed ponds (Figures 3, 4). Though duckweed ponds were a daytime CO2 sink, higher CO2 concentrations may mean that these ponds release more CO2 during the night, potentially offsetting the CO2 captured by the duckweed. Further, CH4 accounted for over 95% of the total emission rate in CO2eq on average for these ponds, showing that CH4 dominates the emissions in small ponds. Overall duckweed ponds had about three times the emission rate in CO2 equivalents compared to non-duckweed ponds (mean 31 vs. 11 g C m−2 d−1).
Duckweed ponds also had higher concentrations of CH4 and CO2 in the bottom waters, though not significantly. Duckweed ponds did have significantly lower concentrations of N2O in the bottom waters, with most bottom waters of duckweed ponds undersaturated. This could again be due to anoxic conditions in the hypolimnion of duckweed ponds, which has been linked to undersaturated N2O (Beaulieu et al., 2015).
Implications and management
Floating macrophyte coverage, including duckweed, is increasing worldwide in recent decades, correlated with expanding urbanization and increased eutrophication of aquatic systems (Kleinschroth et al., 2021). While not all floating macrophytes may create similar dynamics, other floating macrophytes that shade out water column oxygen production could lead higher anoxia, higher phosphorus concentrations, and higher CH4 emissions as seen in the ponds in this study. For urban stormwater ponds, floating macrophytes may undermine the goal of capturing and preventing nutrients from flowing into downstream waterways by increasing internal loading of from phosphorus-rich sediments (Taguchi et al., 2020). Further, duckweed and other floating macrophytes may be causing an unforeseen increase in greenhouse gas emissions from both constructed and natural ponds.
In constructed ponds, management of duckweed and other floating macrophytes can be difficult, as weed control can be costly and labor intensive (Kleinschroth et al., 2021). Duckweed may be utilized as an obvious “indicator” of degraded conditions in constructed ponds, likely signaling high nutrients, poor water quality, and high greenhouse gas emissions, and duckweed has already been used as an indicator of pollution and heavy metal contamination (Garg & Chandra, 1994). While canopy cover blocking wind may be an important influencer of duckweed growth, data for this study suggest phosphorus levels may be one of the most important drivers of duckweed coverage. While management of stormwater ponds already often focuses on reducing nutrient levels, the duckweed state in ponds may act similarly to stable states seen in shallow lakes (Scheffer et al., 1993), where duckweed takes over as the dominant primary producer, and the ensuing degraded water quality state is more resistant to reduced nutrient levels. Oxygen depletion may be the largest factor in increasing CH4 emissions caused by duckweed, and increasing oxygenation in ponds may limit phosphorus loading as well as reduce greenhouse gas emissions.
Conclusion
The ponds in this study had high concentrations and emissions of greenhouse gases, especially CH4, that were similar to other recent studies of ponds. While no differences in nutrient levels or greenhouse gas concentrations were found between natural and constructed ponds, duckweed had a distinct impact on pond physical and chemical characteristics, as well as greenhouse gas dynamics. Duckweed ponds had CH4 emission rates nearly three times as high as non-duckweed ponds. Duckweed ponds had lower CO2 and N2O emissions, but overall had higher emissions in CO2eq due to methane. Globally increasing duckweed and other floating macrophyte coverage worldwide may be increasing greenhouse gas emissions from ponds and can be an indicator of degraded water quality conditions.
Data availability statement
The datasets presented in this study are located in the Environmental Data Initiative (EDI) Repository. (Rabaey and Cotner, 2022) https://doi.org/10.6073/pasta/370cca808d5d1991ddb7f2b9f2b6c107.
Author contributions
JR and JC conceived and designed the analysis, JR completed data collection, performed data analysis, and drafted the manuscript. JC aided in data interpretation and revising the manuscript.
Funding
Research funding was provided by the University of Minnesota College of Biological Sciences, the Bell Museum of Natural History, and the NSF Department of Environmental Biology (DEB), grant number 1722507.
Acknowledgments
Thank you to Miriam Arroyo for aiding in fieldwork and data collection. Thank you to the Belwin Conservancy, Dodge Nature Center, Ordway Field Station, and Cedar Creek Ecosystem Reserve for access and use of study ponds. Research funding was provided by the University of Minnesota College of Biological Sciences, as well as the Bell Museum of Natural History.
Conflict of interest
The authors declare that the research was conducted in the absence of any commercial or financial relationships that could be construed as a potential conflict of interest.
Publisher’s note
All claims expressed in this article are solely those of the authors and do not necessarily represent those of their affiliated organizations, or those of the publisher, the editors and the reviewers. Any product that may be evaluated in this article, or claim that may be made by its manufacturer, is not guaranteed or endorsed by the publisher.
Supplementary material
The Supplementary Material for this article can be found online at: https://www.frontiersin.org/articles/10.3389/fenvs.2022.889289/full#supplementary-material
References
Andersen, M. R., Kragh, T., Sand-jensen, K., and Andersen, M. R. (2017). Extreme diel dissolved oxygen and carbon cycles in shallow vegetated lakes. The Royal Society B, 284.
Attermeyer, K., Flury, S., Jayakumar, R., Fiener, P., Steger, K., Arya, V., et al. (2016). Invasive floating macrophytes reduce greenhouse gas emissions from a small tropical lake. Sci. Rep. 6, 20424. doi:10.1038/srep20424
Audet, J., Carstensen, M. V., Hoffmann, C. C., Lavaux, L., Thiemer, K., and Davidson, T. A. (2020). Greenhouse gas emissions from urban ponds in Denmark. Inland Waters 10 (3), 373–385. doi:10.1080/20442041.2020.1730680
Baron, A. A. P., Dyck, L. T., Amjad, H., Bragg, J., Kroft, E., Newson, J., et al. (2022). Differences in ebullitive methane release from small, shallow ponds present challenges for scaling. Sci. Total Environ. 802, 149685. doi:10.1016/j.scitotenv.2021.149685
Bastviken, D., Cole, J. J., Pace, M. L., and van de-Bogert, M. C. (2008). Fates of methane from different lake habitats: Connecting whole-lake budgets and CH4 emissions. J. Geophys. Res. 113 (2), 1–13. doi:10.1029/2007JG000608
Bastviken, D., Cole, J., Pace, M., and Tranvik, L. (2004). Methane emissions from lakes: Dependence of lake characteristics, two regional assessments, and a global estimate. Glob. Biogeochem. Cycles 18 (4), 1–12. doi:10.1029/2004GB002238
Beaulieu, J. J., DelSontro, T., and Downing, J. A. (2019). Eutrophication will increase methane emissions from lakes and impoundments during the 21st century. Nat. Commun. 10 (1), 1375–1377. doi:10.1038/s41467-019-09100-5
Beaulieu, J. J., Nietch, C. T., and Young, J. L. (2015). Controls on nitrous oxide production and consumption in reservoirs of the Ohio River Basin. J. Geophys. Res. Biogeosci. 120 (10), 1995–2010. doi:10.1002/2015JG002941
Bettez, N. D., and Groffman, P. M. (2012). Denitrification potential in stormwater control structures and natural riparian zones in an urban landscape. Environ. Sci. Technol. 46 (20), 10909–10917. doi:10.1021/es301409z
Birge, E. A. (1916). The work of the wind in warming a lake. Notes from the Laboratory of the Wisconsin Geological and Natural History Survey.
Blaszczak, J. R., Steele, M. K., Badgley, B. D., Heffernan, J. B., Hobbie, S. E., Morse, J. L., et al. (2018). Sediment chemistry of urban stormwater ponds and controls on denitrification. Ecosphere 9 (6). doi:10.1002/ecs2.2318
Carlson, R. E. (1977). A trophic state index for lakes1. Limnol. Oceanogr. 22 (2), 361–369. doi:10.4319/lo.1977.22.2.0361
Ceschin, S., Abati, S., Traversetti, L., Spani, F., del Grosso, F., and Scalici, M. (2019). Effects of the invasive duckweed lemna minuta on aquatic animals: Evidence from an indoor experiment. Plant Biosyst. - Int. J. Deal. All Aspects Plant Biol. 153 (6), 749–755. doi:10.1080/11263504.2018.1549605
Clifford, C. C., and Heffernan, J. B. (2018). Artificial aquatic ecosystems. Water 10, 1096. doi:10.3390/w10081096
Cole, J. J., Caraco, N. F., et al. Cole, J. J., Caraco, N. F., Kling, G. W., and Kratz, T. K. (1994). Carbon dioxide supersaturation in the surface waters of lakes. Science 265 (5178), 1568–1570. doi:10.1126/science.265.5178.1568
Davidson, T. A., Audet, J., Jeppesen, E., Landkildehus, F., Lauridsen, T. L., Søndergaard, M., et al. (2018). Synergy between nutrients and warming enhances methane ebullition from experimental lakes. Nat. Clim. Chang. 8 (2), 156–160. doi:10.1038/s41558-017-0063-z
Deacon, E. L. (1981). Sea-air gas transfer: The wind speed dependence. Bound. Layer. Meteorol. 21, 31–37. doi:10.1007/bf00119365
DelSontro, T., Beaulieu, J. J., and Downing, J. A. (2018). Greenhouse gas emissions from lakes and impoundments: Upscaling in the face of global change. Limnol. Oceanogr. Lett. 3 (3), 64–75. doi:10.1002/lol2.10073
DelSontro, T., Boutet, L., St-Pierre, A., del Giorgio, P. A., and Prairie, Y. T. (2016). Methane ebullition and diffusion from northern ponds and lakes regulated by the interaction between temperature and system productivity. Limnol. Oceanogr. 61, S62–S77. doi:10.1002/lno.10335
Derwent, R. G. (2020). Global warming potential (GWP) for methane: Monte Carlo analysis of the uncertainties in global tropospheric model predictions. Atmosphere 11 (5), 486. doi:10.3390/ATMOS11050486
Desrosiers, K., Delsontro, T., and del Giorgio, P. A. (2022). Disproportionate contribution of vegetated habitats to the CH4 and CO2 budgets of a boreal lake. Ecosystems. doi:10.1007/s10021-021-00730-9
Downing, J. A., McDowell, W. H., Kortelainen, P., Caraco, N. F., Tranvik, L. J., Cole, J. J., et al. (2006). The global abundance and size distribution of lakes, ponds, and impoundments. Limnol. Oceanogr. 51 (5), 2388–2397. doi:10.4319/lo.2006.51.5.2388
Duc, N. T., Crill, P., and Bastviken, D. (2010). Implications of temperature and sediment characteristics on methane formation and oxidation in lake sediments. Biogeochemistry 100 (1), 185–196. doi:10.1007/s10533-010-9415-8
Ducharme-Riel, V., Vachon, D., del Giorgio, P. A., and Prairie, Y. T. (2015). The relative contribution of winter under-ice and summer hypolimnetic CO2 accumulation to the annual CO2 emissions from northern lakes. Ecosystems 18 (4), 547–559. doi:10.1007/s10021-015-9846-0
Erkkilä, K. M., Ojala, A., Bastviken, D., Biermann, T., Heiskanen, J., Lindroth, A., et al. (2018). Methane and carbon dioxide fluxes over a lake: Comparison between eddy covariance, floating chambers and boundary layer method. Biogeosciences 15 (2), 429–445. doi:10.5194/bg-15-429-2018
Fofonoff, N. P., and Millard, R. D. (1983). Algorithms for computation of fundamental properties of seawater. Unesco Tech. Pap. Mar. Sci. 44, 44.
Garg, P., and Chandra, P. (1994). The duckweed wolffia globosa as an indicator of heavy metal pollution: Sensitivity to cr and cd. Environ. Monit. Assess. 29, 89–95. doi:10.1007/bf00546781
Goeckner, A. H., Lusk, M. G., Reisinger, A. J., Hosen, J. D., and Smoak, J. M. (2022). Florida’s urban stormwater ponds are net sources of carbon to the atmosphere despite increased carbon burial over time. Commun. Earth Environ. 3 (1), 53. doi:10.1038/s43247-022-00384-y
Gorsky, A. L., Racanelli, G. A., Belvin, A. C., and Chambers, R. M. (2019). Greenhouse gas flux from stormwater ponds in southeastern Virginia (USA). Anthropocene 28, 100218. doi:10.1016/j.ancene.2019.100218
Grinham, A., Albert, S., Deering, N., Dunbabin, M., Bastviken, D., Sherman, B., et al. (2018). The importance of small artificial water bodies as sources of methane emissions in Queensland, Australia. Hydrol. Earth Syst. Sci. 22 (10), 5281–5298. doi:10.5194/hess-22-5281-2018
Herrero Ortega, S., Romero González-Quijano, C., Casper, P., Singer, G. A., and Gessner, M. O. (2019). Methane emissions from contrasting urban freshwaters: Rates, drivers, and a whole-city footprint. Glob. Chang. Biol. 25 (12), 4234–4243. doi:10.1111/gcb.14799
Hill, K. D., Dauphinee, T. M., and Woods, D. J. (1986). The extension of the practical salinity scale 1978 to low salinities. IEEE J. Ocean. Eng. 11 (1), 109–112. doi:10.1109/JOE.1986.1145154
Hillman, W. S. (1961). The Lemnaceae, or duckweeds. A review of the descriptive and experimental literature. Bot. Rev. 27, 221–287. doi:10.1007/bf02860083
Hilt, S., Grossart, H., McGinnis, D. F., and Keppler, F. (2022). Potential role of submerged macrophytes for oxic methane production in aquatic ecosystems. Limnol. Oceanogr. doi:10.1002/lno.12095
Holgerson, M. A. (2015). Drivers of carbon dioxide and methane supersaturation in small , temporary ponds. Biogeochemistry 124, 305–318. doi:10.1007/s10533-015-0099-y
Holgerson, M. A., Farr, E. R., and Raymond, P. A. (2017). Gas transfer velocities in small forested ponds. JGR. Biogeosciences 122 (5), 1011–1021. doi:10.1002/2016JG003734
Holgerson, M. A., and Raymond, P. A. (2016). Large contribution to inland water CO 2 and CH 4 emissions from very small ponds. Nat. Geosci. 9, 222–226. doi:10.1038/NGEO2654
Holgerson, M. A., Richardson, D. C., Roith, J., Bortolotti, L. E., Finlay, K., Hornbach, D. J., et al. (2022). Classifying mixing regimes in ponds and shallow lakes. Water Resour. Res. 58 (7). doi:10.1029/2022WR032522
Jähne, B., Münnich, K. O., Bösinger, R., Dutzi, A., Huber, W., and Libner, P. (1987). On the parameters influencing air‐water gas exchange. J. Geophys. Res. 92, 1937–1949. doi:10.1029/jc092ic02p01937
Jansen, J., Thornton, B. F., Jammet, M. M., Wik, M., Cortés, A., Friborg, T., et al. (2019). Climate‐sensitive controls on large spring emissions of CH 4 and CO 2 from northern lakes. J. Geophys. Res. Biogeosci. 124, 2379–2399. doi:10.1029/2019jg005094
Johnson, K., Hughes, J., Donaghay, P., and Sieburthm, John. (1990). Bottle-calibration static head space method for the determination of methane dissolved in seawater. Anal. Chem. 62, 2408–2412. doi:10.1021/ac00220a030
Kankaala, P., Huotari, J., Tulonen, T., and Ojala, A. (2013). Lake-size dependent physical forcing drives carbon dioxide and methane effluxes from lakes in a boreal landscape. Limnol. Oceanogr. 58 (6), 1915–1930. doi:10.4319/lo.2013.58.6.1915
Khan, F. A., and Ansari, A. A. (2005). Eutrophication: An ecological vision. Botanical Rev. 71 (4), 449–482. doi:10.1663/0006-8101(2005)071[0449:eaev]2.0.co;2
Kifner, L. H., Calhoun, A. J. K., Norton, S. A., Hoffmann, K. E., and Amirbahman, A. (2018). Methane and carbon dioxide dynamics within four vernal pools in Maine, USA. Biogeochemistry 139 (3), 275–291. doi:10.1007/s10533-018-0467-5
Kleinschroth, F., Winton, R. S., Calamita, E., Niggemann, F., Botter, M., Wehrli, B., et al. (2021). Living with floating vegetation invasions. Ambio 50 (1), 125–137. doi:10.1007/s13280-020-01360-6
Koschorreck, M., Downing, A. S., Hejzlar, J., Marcé, R., Laas, A., Arndt, W. G., et al. (2020). Hidden treasures: Human-made aquatic ecosystems harbour unexplored opportunities. Ambio 49 (2), 531–540. doi:10.1007/s13280-019-01199-6
Lasfar, S., Monette, F., Millette, L., and Azzouz, A. (2007). Intrinsic growth rate: A new approach to evaluate the effects of temperature, photoperiod and phosphorus-nitrogen concentrations on duckweed growth under controlled eutrophication. Water Res. 41 (11), 2333–2340. doi:10.1016/j.watres.2007.01.059
Lazar, J. G., Addy, K., Welsh, M. K., Gold, A. J., and Groffman, P. M. (2014). Resurgent beaver ponds in the northeastern United States: Implications for greenhouse gas emissions. J. Environ. Qual. 43 (6), 1844–1852. doi:10.2134/jeq2014.02.0065
McAuliffe, C. (1971). Cas chromatographic determination of solutes by multiple phase equilibrium. Chem. Technol. 1, 46–51.
McClain, M. E., Boyer, E. W., Dent, C. L., Gergel, S. E., Grimm, N. B., Groffman, P. M., et al. (2003). Biogeochemical hot spots and hot moments at the interface of terrestrial and aquatic ecosystems. Ecosystems 6 (4), 301–312. doi:10.1007/s10021-003-0161-9
Mccrackin, M. L., and Elser, J. J. (2010). Atmospheric nitrogen deposition influences denitrification and nitrous oxide production in lakes. Ecology 91 (2), 528–539. doi:10.1890/08-2210.1
Mengis, M., Gächter, R., and Wehrli, B. (1997). Sources and sinks of nitrous oxide (N2O) in deep lakes. Biogeochemistry 38 (3), 281–301. doi:10.1023/A:1005814020322
Murphy, J., and Riley, J. P. (1962). A modified single solution method for the determination of phosphate in natural waters. Anal. Chim. Acta 27, 31–36. doi:10.1016/s0003-2670(00)88444-5
Myhre, G., Shindell, D., Bréon, F.-M., Collins, W., Fuglestvedt, J., Huang, J., et al. (2013). “Anthropogenic and natural radiative forcing,” in Climate change 2013 the physical science basis: Working group I contribution to the fifth assessment report of the intergovernmental panel on climate change. Editors T. F. Stocker, D. Qin, G.-K. Plattner, M. Tignor, S. K. Allen, J. Boschunget al. (Cambridge: Cambridge University Press), 659–740. doi:10.1017/CBO9781107415324.018
Nürnberg, G. K. (1995). Quantifying anoxia in lakes. Limnol. Oceanogr. 40 (6), 1100–1111. doi:10.4319/lo.1995.40.6.1100
Peacock, M., Audet, J., Bastviken, D., Cook, S., Evans, C. D., Grinham, A., et al. (2021). Small artificial waterbodies are widespread and persistent emitters of methane and carbon dioxide. Glob. Chang. Biol. 27 (20), 5109–5123. doi:10.1111/gcb.15762
Peacock, M., Audet, J., Jordan, S., Smeds, J., and Wallin, M. B. (2019). Greenhouse gas emissions from urban ponds are driven by nutrient status and hydrology. Ecosphere 10 (3), e02643. doi:10.1002/ecs2.2643
Planet Team (2017). In Planet application program interface: In space for life on earth. Available at https://api.planet.com.
Pokorny, J., and Rejm, E. (1983). Oxygen regime in A fishpond with duckweeds (Lemnaceae) and ceratophyllum. Aquatic Botany, 17.
Rabaey, J. S., and Cotner, J. B. (2022). Greenhouse gas and water chemistry data from ponds in the twin-cities area of Minnesota, 2021 ver 1. Environmental Data Initiative.
Raymond, P. A., Hartmann, J., Lauerwald, R., Sobek, S., McDonald, C., Hoover, M., et al. (2013). Global carbon dioxide emissions from inland waters. Nature 503 (7476), 355–359. doi:10.1038/nature12760
Read, J. S., Hamilton, D. P., Jones, I. D., Muraoka, K., Winslow, L. A., Kroiss, R., et al. (2011). Derivation of lake mixing and stratification indices from high-resolution lake buoy data. Environ. Model. Softw. 26 (11), 1325–1336. doi:10.1016/J.ENVSOFT.2011.05.006
Richardson, D. C., Holgerson, M. A., Farragher, M. J., Hoffman, K. K., King, K. B. S., Alfonso, M. B., et al. (2022). A functional definition to distinguish ponds from lakes and wetlands. Sci. Rep. 12 (1), 10472. doi:10.1038/s41598-022-14569-0
Riera, J. L., Schindler, J. E., and Kratz, T. K. (1999). Seasonal dynamics of carbon dioxide and methane in two clear-water lakes and two bog lakes in northern Wisconsin, U.S.A. Can. J. Fish. Aquat. Sci. 56 (2), 265–274. doi:10.1139/f98-182
Rosentreter, J. A., Borges, A. v., Deemer, B. R., Holgerson, M. A., Liu, S., Song, C., et al. (2021). Half of global methane emissions come from highly variable aquatic ecosystem sources. Nat. Geosci. 14, 225–230. doi:10.1038/s41561-021-00715-2
Scheffer, M., Hosper, S. H., Meijer, M.-L., Moss, B., and Jeppesen, E. (1993). Alternative Equilibria in Shallow Lakes. Reviews 11 (3), 220–226.
Sieczko, A. K., Thanh Duc, N., Schenk, J., Pajala, G., Rudberg, D., Sawakuchi, H. O., et al. (2020). Diel variability of methane emissions from lakes. Proc. Natl. Acad. Sci. U. S. A. 117 (35), 21488–21494. doi:10.1073/pnas.2006024117
Singh, V. P., Dass, P., Kaur, K., Billore, S. K., Gupta, P. K., and Parashar, D. C. (2005). Nitrous oxide fluxes in a tropical shallow urban pond under influencing factors. Curr. Sci. 88 (3), 478–483.
Taguchi, V. J., Olsen, T. A., Natarajan, P., Janke, B. D., Gulliver, J. S., Finlay, J. C., et al. (2020). Internal loading in stormwater ponds as a phosphorus source to downstream waters. Limnol. Oceanogr. Lett. 5 (4), 322–330. doi:10.1002/lol2.10155
Tippery, N. P., and Les, D. H. (2020). Tiny plants with enormous potential: Phylogeny and evolution of duckweeds, 19–38. doi:10.1007/978-3-030-11045-1_2
van Bergen, T. J. H. M., Barros, N., Mendonça, R., Aben, R. C. H., Althuizen, I. H. J., Huszar, V., et al. (2019). Seasonal and diel variation in greenhouse gas emissions from an urban pond and its major drivers. Limnol. Oceanogr. 64 (5), 2129–2139. doi:10.1002/lno.11173
Verpoorter, C., Kutser, T., Seekell, D. A., and Tranvik, L. J. (2014). A global inventory of lakes based on high-resolution satellite imagery. Geophys. Res. Lett. 41 (18), 6396–6402. doi:10.1002/2014GL060641
Wang, G., Xia, X., Liu, S., Zhang, S., Yan, W., and McDowell, W. H. (2021). Distinctive Patterns and Controls of Nitrous Oxide Concentrations and Fluxes from Urban Inland Waters. Environ. Sci. Technol. 55, 8422–8431. doi:10.1021/acs.est.1c00647
Wanninkhof, R. (1992). Relationship between wind speed and gas exchange over the ocean. J. Geophys. Res. 97 (C5), 7373–7382. doi:10.1029/92JC00188
Webb, J. R., Hayes, N. M., Simpson, G. L., Leavitt, P. R., Baulch, H. M., and Finlay, K. (2019). Widespread nitrous oxide undersaturation in farm waterbodies creates an unexpected greenhouse gas sink. Proc. Natl. Acad. Sci. U. S. A. 116 (20), 9814–9819. doi:10.1073/pnas.1820389116
Weishaar, J. L., Aiken, G. R., Bergamaschi, B. A., Fram, M. S., Fujii, R., and Mopper, K. (2003). Evaluation of specific ultraviolet absorbance as an indicator of the chemical composition and reactivity of dissolved organic carbon. Environ. Sci. Technol. 37 (20), 4702–4708. doi:10.1021/es030360x
Weiss, R. F. (1974). Carbon Dioxide in Water and Seawater: The Solubility of a Non-Ideal Gas. Mar. Chem. 2, 203–215. doi:10.5194/bg-13-841-2016
Weiss, R. F., and Price, B. A. (1980). Nitrous Oxide Solubility in Water and Seawater. Mar. Chem. 8, 347–359.
West, W. E., Coloso, J. J., and Jones, S. E. (2012). Effects of algal and terrestrial carbon on methane production rates and methanogen community structure in a temperate lake sediment. Freshw. Biol. 57 (5), 949–955. doi:10.1111/j.1365-2427.2012.02755.x
Wiesenburg, D. A., and Guinasso, N. L. (1979). Equilibrium Solubilities of Methane, Carbon Monoxide, and Hydrogen in Water and Sea Water. J. Chem. Eng. Data 24 (4), 356–360. doi:10.1021/je60083a006
Wilkinson, J., Bors, C., Burgis, F., Lorke, A., and Bodmer, P. (2019). Measuring CO2 and CH4 with a portable gas analyzer: Closed-loop operation, optimization and assessment. PLoS ONE 13 (4), e0193973. doi:10.1371/journal.pone.0193973
Xiao, S., Wang, C., Wilkinson, R. J., Liu, D., Zhang, C., Xu, W., et al. (2016). Theoretical model for diffusive greenhouse gas fluxes estimation across water-air interfaces measured with the static floating chamber method. Atmos. Environ. 137, 45–52. doi:10.1016/j.atmosenv.2016.04.036
Xiao, S., Yang, H., Liu, D., Zhang, C., Lei, D., Wang, Y., et al. (2014). Gas transfer velocities of methane and carbon dioxide in a subtropical shallow pond. Tellus B Chem. Phys. Meteorology 66 (1), 23795. doi:10.3402/tellusb.v66.23795
Zhou, Y., Zhou, L., Zhang, Y., Garcia de Souza, J., Podgorski, D. C., Spencer, R. G. M., et al. (2019). Autochthonous dissolved organic matter potentially fuels methane ebullition from experimental lakes. Water Res. 166, 115048. doi:10.1016/j.watres.2019.115048
Keywords: floating macrophytes, stormwater ponds, gas fluxes, carbon dioxide (CO2), methane (CH4), nitrous oxide (N2O), freshwaters, climate change
Citation: Rabaey J and Cotner J (2022) Pond greenhouse gas emissions controlled by duckweed coverage. Front. Environ. Sci. 10:889289. doi: 10.3389/fenvs.2022.889289
Received: 04 March 2022; Accepted: 14 September 2022;
Published: 05 October 2022.
Edited by:
Tonya DelSontro, University of Waterloo, CanadaReviewed by:
Pascal Bodmer, Université du Québec à Montréal, CanadaRoberta Bittencourt Peixoto, Federal University of Rio de Janeiro, Brazil
Copyright © 2022 Rabaey and Cotner. This is an open-access article distributed under the terms of the Creative Commons Attribution License (CC BY). The use, distribution or reproduction in other forums is permitted, provided the original author(s) and the copyright owner(s) are credited and that the original publication in this journal is cited, in accordance with accepted academic practice. No use, distribution or reproduction is permitted which does not comply with these terms.
*Correspondence: Joseph Rabaey, Rabae005@umn.edu