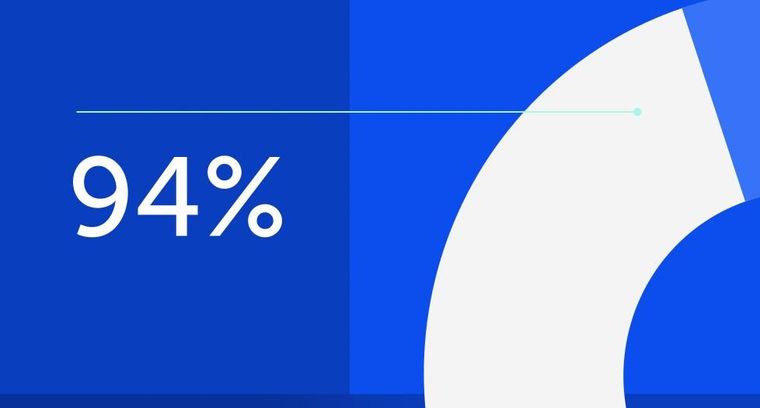
94% of researchers rate our articles as excellent or good
Learn more about the work of our research integrity team to safeguard the quality of each article we publish.
Find out more
HYPOTHESIS AND THEORY article
Front. Environ. Sci., 08 April 2022
Sec. Environmental Economics and Management
Volume 10 - 2022 | https://doi.org/10.3389/fenvs.2022.885933
This article is part of the Research TopicGreen Innovation and Industrial Ecosystem Reconstruction in Achieving Environmental SustainabilityView all 34 articles
Facing of increasingly serious resource and environmental constraints, the adoption of green technology has become the key to driving the green production transformation of farmers under the constraints of environmental regulation. Whether environmental regulation can improve the adoption of green technology in breeding industry still needs to be further tested. Based on the survey data of 544 pig farmers in Sichuan, Shandong, Henan and Hebei provinces, this paper first measures the adoption degree of green breeding technology by using the coefficient of variation method. Then, the econometric model is used to empirically test the relationship between environmental regulation and farmers’ adoption of green technology. The results show that: firstly, environmental regulation can improve the adoption of green technology by farmers; Economic incentive regulation and educational guidance regulation can significantly improve the adoption of green technology by farmers from the perspective of environmental regulation. Secondly, environmental regulation has different effects on the adoption of green technology of different attributes of farmers. Its effect on the adoption of resource utilization technology is higher than that of harmless treatment technology and higher than that of reduction technology. Different dimensions of environmental regulation have different effects on the adoption of green technology by farmers with different attributes. Thirdly, the impact of environmental regulation on the adoption of green technology by different scale farmers is different, and its impact on high-scale farmers is more obvious. At the same time, different dimensions of environmental regulation have different effects on the adoption of green technology by farmers of different sizes.
Over the past 40 years of reform and opening up, with the growth of demand and the promotion of policies, China’s animal husbandry industry has shown a rapid growth trend. By 2020, the output value of animal husbandry exceed 4 trillion yuan, accounting for 34% of the total agricultural output value2, with more than 100 million employees. It has become an important pillar industry for rural development. However, in the process of rapid development, the emission of livestock and poultry breeding waste is increasing day by day, and the amount of livestock and poultry feces produced every year is up to 3.8 billion tons. The comprehensive utilization rate of livestock and poultry manure is only 64%, and the problem of environmental pollution is becoming more and more serious (Yin, 2019). Extensive development has increased the pressure on carbon emission reduction. In 2016, China’s agricultural non energy CO2 emission equivalent was 780.22 million tons. Carbon emissions from livestock manure and intestinal fermentation account for 38.76% (Tian and Chen, 2021).
Taking into account the effective supply of livestock products and ecological and environmental protection, promoting the green transformation and upgrading of livestock and poultry breeding is an important way to reduce pollution and carbon in animal husbandry. Its core is the adoption of green breeding technology (Si et al., 2020). In view of this, the Chinese government has successively implemented a series of environmental regulation measures, such as the regulations on the prevention and control of pollution in large-scale livestock and poultry breeding issued in 2013, the technical guide for the delimitation of livestock and poultry breeding prohibited areas issued in 2016, and the environmental protection tax law officially implemented in January 2018. In addition, local governments at all levels have successively issued corresponding local regulations and environmental protection standards according to their own economic development and environmental protection requirements.
Many studies have shown that environmental regulation plays an important role as an external institutional element in the adoption of green technologies by farmers. Moser et al., 2008) empirically showed that media campaigns have an incentive effect on farmers’ adoption of biocontrol technologies. Mueller, 2013) found that government incentive-based regulations have a better effect than constraint-based regulations and that incentive-based regulations have the most significant effect on farmers’ adoption behavior of manure resource utilization technologies. Haghjou et al., 2014) found that government subsidies imposed a positive impact and incentive effect on farmers’ green technology adoption behavior. Abate et al., 2016) concluded that the level of support from government rural financial institutions was positively associated with the adoption of green technologies and the degree of adoption by farmers. Hou et al., 2019) found that mandatory burning bans had no significant ef fect on the use of straw-returning technologies, while the establishment of demonstration projects had a significant contribution to the adoption of straw-returning technologies. A study by Kolady et al., 2021) on the behavior of green fertilization technology use found that environmental regulation has a significant positive effect on farmers’ green technology adoption behavior through three paths: guidance, incentive and constraint. It has also been pointed out that the use of various types of policies in combination has a better effect on farmers’ green production technology adoption behavior (Jacquet et al., 2011; Zheng et al., 2014). However, the research on the impact of environmental regulation on farmers’ green technology choices mainly focuses on the research on farmers’ green production technology adoption behavior, lacks research on the capital- and labor-intensive livestock and poultry industries, and on the correlation between multiple technology adoption decisions Relationships are less discussed.
Farmers’ green technology adoption is a careful trade-off between risk minimization and profit maximization, and generally consider technology attributes that match their own endowments and have lower technology risks, and then combine with policy environment and capital endowment factors to show a certain technology adoption bias (Barnes et al., 2019). Green technology is a technology package composed of several technologies, and the technologies can be divided into different categories according to their technical attributes, for example, they can be divided into capital-increasing and risk-increasing technologies according to their technical functions, and capital-intensive and labor-intensive technologies according to their factor intensity (Man et al., 2010). However, due to the limitations of China’s incomplete factor market and the differentiation of farmers’ subjects, the initial endowments and risk preferences of farmers of different scales differ, resulting in large differences in the demand for different attribute technologies and different technology adoption biases among farming subjects. Although some studies have noted the strong heterogeneity of farmers’ adoption of different attribute technologies and studied them by technology attributes (Kolady et al., 2021), they only considered a prominent attribute of the technology and ignored the multidimensional attribute characteristics of the technology; for example, Xu et al., 2018) only explored the intertemporal selection attribute of direct straw return technology. The different degrees of constraints, incentives and publicity of environmental regulation policies on different attribute technologies lead to different biases of environmental regulation on farmers’ choice of different attribute green technologies. Then, can a series of environmental regulation policies implemented by the government promote the adoption of green technology by farmers? Do different attribute technologies show different adoption effects? Is there a significant difference in the behavioral response of farmers with endowment heterogeneity? The empirical test of these problems can provide a basis for formulating the green transformation policy of animal husbandry.
This paper uses the survey data of 544 pig farmers in Sichuan, Shandong, Henan and Hebei provinces to empirically study the impact of environmental regulation on the adoption of breeding technology using two-stage least squares (2SLS) and Multivariate Probit models. The structure of the article is arranged as follows, the second part is theoretical analysis and research hypothesis, the third part is data source, variable setting and model construction, the fourth part is empirical test and result analysis, the fifth part is heterogeneity analysis, the sixth part is are the main conclusions and policy recommendations.
Environmental regulation belongs to the category of social regulation, and as administrative regulations to restrain the negative externality behavior of micro subjects, it can be divided into command-and-coercion type regulation with reverse supervision and economic incentive type and educational guidance type regulation with proactive promotion (Huang et al., 2016). At present, the implementation of environmental regulation is in a state of economic incentive regulation, supplemented by command-and-force regulation of punishment and supervision, and education-guided regulation running through it. Environmental regulation mainly affects the adoption of green technology in breeding industry through two mechanisms. The first is the compensation effect of innovation. Porter hypothesis points out that appropriate environmental regulation can force the economic subject to green technology innovation and produce compensation effect. Furthermore, environmental regulation can partially or completely make up for or even exceed the internalized environmental governance cost (Ge et al., 2021) The second is the cost offset effect. Environmental regulation will increase the access threshold of livestock and poultry breeding for farmers. The breeding that fails to meet the standard in the breeding link will not only reduce the production capacity, but also withdraw from production, resulting in serious economic losses (Sun et al., 2021a). The adoption of green technology by farmers requires a large amount of capital investment, which not only squeezes the capital investment in other links of farmers and weakens the competitiveness of farmers in market competition, but also reduces the capital turnover rate of farmers and occupies the supplementary column resources (Han Li et al, 2021; Han et al, 2021b), resulting in the squeeze of profit space. Third, the information rationing effect. The adoption of green technology by farmers is a dynamic process, and information, as an intangible element attached to the technology itself, also influences farmers’ production decision making behavior (Wei and Xu, 2017). Education-led regulation, including government demonstration, policy advocacy guidance and technical training, plays an important role in information rationing. On the one hand, it can alleviate the constraints of farmers’ access to green technology information, break the information barriers, realize the accumulation of technical knowledge and experience (Sun et al., 2021b), and promote their green technology choice; on the other hand, it can reduce information inconsistency, reduce the information search and transaction costs in the green technology choice process, and thus enhance the initiative of farmers’ green technology choice (Fen et al., 2019). In addition, the information sharing and“lock-in effect” of the guiding regulation can provide safety guarantee for green and high-quality livestock products, and can force farmers to choose green technology. Therefore, the impact of environmental regulation on the adoption of green technology by farmers is the result of the combination of two effects. This depends on the comparison of the compensation effect and offset effect brought by environmental regulation to farmers. If the former is greater than the latter, environmental regulation will promote the adoption of green technology by farmers. Based on this, the following hypotheses are proposed:
H1: Environmental regulation has a significant impact on the adoption of green technology by farmers.
When mandatory regulation regulates the production behavior of farmers through environmental protection measures such as supervision and punishment and pollution discharge standards, it needs to bear not only the corresponding factor input cost, but also the internalized environmental cost (pollution discharge and pollution control cost), which will undoubtedly increase the Cost Stickiness of farmers. The increase of Cost Stickiness will accelerate the optimal allocation of factor resources of farmers and force them to adopt green technology to absorb non- productive costs (Yu et al., 2019). At the same time, the spillover effect of green technology is conducive to slowing down or offsetting the decline in total income caused by regulation costs. Therefore, this paper puts forward the following hypotheses:
H2: Mandatory regulation can promote the adoption of green technology by farmers.
The incentive environmental regulation composed of financial subsidies, insurance linkage, facility subsidies and loan interest discount can not only alleviate the cost pressure and “production reduction” risk of green technology adoption by farmers, but also provide necessary conditions for technology adoption; It can also enhance the risk prevention and control ability of farmers, improve the risk awareness level of the main body, reduce the uncertainty of expected income, and provide risk guarantee for technology adoption; It can also alleviate the financing constraints of green breeding, virtually introduce green technology into the livestock and poultry breeding process, and drive farmers without technology to adopt green breeding technology (Li, 2019). Therefore, this paper puts forward the following hypotheses:
H3: Economic incentive regulation can promote the adoption of green technology by farmers.
The educational guidance regulation of government demonstration, policy publicity and guidance and technical training plays an important role in information rationing. It can alleviate the constraints of farmers’ access to green technology information, break the information barrier, realize the accumulation of technical knowledge and experience, and promote the adoption of green technology; It can also reduce information inaccuracy, reduce the information search and transaction cost in the process of green technology adoption, and then improve the initiative of farmers in green technology adoption. In addition, the information sharing and “lock-in effect” of guiding regulation provide safety guarantee for green and high-quality livestock products, which can force farmers to adopt green technology. Therefore, this paper puts forward the following hypotheses:
H4: Educational guidance regulation can promote the adoption of green technology by farmers.
The data used in this paper comes from the research group of “green transformation and production recovery of animal husbandry under the complex scenario of environmental regulation and epidemic impact”. From October to December 2019, the research group conducted a survey on Sichuan Province (Anyue County and Renshou County), Henan Province (Tanghe County, Xinxiang City) Field investigation on pig breeding in 4 provinces and 8 counties of Hebei Province (Luannan County and Funing County) and Shandong Province (Zhucheng city and Laixi county). The selection of these four provinces is mainly based on the following considerations: first, the four provinces are the national pig breeding intensive areas, with superior geographical location, strong environmental carrying capacity and large-scale breeding volume. The pig industry has become the pillar industry of the agricultural and rural economy of the four provinces. Second, the four provinces are located in the western, central and eastern regions of China, with relatively different levels of economic development and obvious ladder characteristics, which can better describe the production and operation of farmers at different levels in the main pig breeding areas in China (see Table 1) Third, the four provinces belong to different pig production areas, Shandong Province and Hebei Province belong to the main pig production areas, Sichuan Province and Henan Province are both the main pig production areas and the main pig sales areas, and the four provinces belong to the main distribution areas of leading pig enterprises such as Wen’s group and new hope group. Therefore, the four provinces are selected as the research area, and the research conclusions are more typical and representative.
The survey follows the principle of combining multi-stage stratified sampling and random sampling. The specific sampling process is as follows: for the selected four provinces, combined with the geographical environment and regional economic development level, first randomly select two counties (county-level districts and cities) in each province. Secondly, according to the research purpose and the introduction of the heads of relevant departments of the animal husbandry bureau, three townships were randomly selected from each county (county-level district and city). Finally, 10–15 farmers were randomly selected in each township for face-to-face in-depth interviews to obtain first-hand information. In order to ensure the validity of the questionnaire, the research group selected Xianyang City, Shaanxi Province to carry out a small-scale pre survey before the formal survey. On this basis, the questionnaire is modified and improved. The respondents were family members who directly played a decision-making role in pig breeding, so as to ensure the accuracy and response rate of the questionnaire. The survey mainly includes the basic characteristics of farmers, family management characteristics, green production cognition, green technology participation, production transformation, environmental regulation, government promotion, production recovery and social capital. A total of 600 questionnaires were distributed in this survey. After excluding the questionnaires such as illogical and abnormal values, 544 valid questionnaires highly related to this study were obtained, and the effective rate of the questionnaire was 90.67%. Among them, there are 162 households in Sichuan Province, 122 households in Henan Province, 146 households in Hebei Province and 114 households in Shandong Province. Questionnaire Cronbach ' α) The reliability test value is 0.786, indicating that the overall validity of the questionnaire is good. The main research was conducted in 24 townships involving 48 administrative villages in 8 counties and cities, and the county distribution of specific samples is shown in Table 2.
degree of adoption of green technology by farmers. Due to the different costs and benefits of different types of technologies, the adoption of green technology by farmers is more complex. In this paper, the weighted average of the three technologies is used to measure the degree of adoption of green technology by farmers, that is, whether farmers adopt green breeding technology or not is a binary variable. If adopted, the value is 1, otherwise, the value is 0. However, considering the different promotion and applicability of farmers’ green production technology in different provinces, equal weight estimation will lead to inaccurate calculation results. Therefore, based on the practice of Xiao et al., 2020), this paper uses the coefficient of variation method to determine the weight coefficients of three green breeding technologies on the basis of measuring the benefits of green production technology from the three-dimensional aspects of technical and economic benefits, environmental benefits and livestock product quality and safety.
Coefficient of variation is a statistic to measure the degree of variation of the observed values of each index, which belongs to the objective weighting method of index weight. Different from chromatographic analysis method and entropy method, coefficient of variation method directly uses the information contained in each index data. After dimensionless processing, the coefficient of variation method reflects the degree of variation by calculating the ratio of standard error to average value of index data, and weights it. In this method, the greater the degree of variation between indicators, the greater the weight. On the contrary, the smaller the assignment (Chen et al., 2021). First, calculate the variation coefficient of each dimension under each green breeding sub technology, and the expression is:
In Equation 1
Secondl, the coefficient of variation obtained by formula (1) is normalized. Then, this study selects the proportion of index variation coefficient in the sum of all index variation coefficients to obtain the secondary index weight of each dimension of green breeding technology, and the expression is:
In Equation 2,
Finally, the weight coefficients of the three green breeding sub technologies can be obtained by summing up the secondary index weights of the three dimensions of each green breeding sub technology respectively. The specific calculation results are as follows.
In order to effectively describe the technical attributes of green breeding technology, according to the subjective evaluation data of pig farmers, this paper uses Likert five level scale to measure the technical and economic benefit attributes, environmental protection attributes and product quality and safety attributes of the three technologies. The results of farmers’ adoption of green technology calculated by coefficient of variation method are shown in Table 3.
environmental regulation and its dimensions. Environmental regulation has multi-dimensional attributes, and its connotation is relatively rich. The academic research on environmental regulation mainly focuses on the related fields of industry and enterprises. However, there is a relative lack of research in the field of agriculture, especially in the field of animal husbandry. At present, there is no unified standard for the measurement of environmental regulation. The existing research basically selects the corresponding indicators according to the needs. This paper draws on the comprehensive index method and classified investigation method of environmental regulation proposed by Zhang, 2016) and Mei et al., 2018). This study constructs a comprehensive system of environmental regulation, including command and compulsion, economic incentive and educational guidance, to measure the intensity of environmental regulation. See Table 4 for specific indicator setting and dimension division. According to the requirements of the comprehensive index method, the regulation intensity of various policies or systems is measured or objective valued through the psychological response of farmers. The subjective values are assigned 1 ∼ 5 respectively, where 1 means no influence at all and 5 means great influence. In this paper, the dimensionality of 15 specific indicators is reduced by factor analysis. The results show that the KMO statistic is 0.80 and the p value of Bartlett spherical test is 0.00. This shows that the sample data is suitable for factor analysis. Three common factors are extracted according to the principle that the characteristic root is greater than 1, and the common factors are named command mandatory regulation, economic incentive regulation and education guidance regulation respectively. The cumulative variance contribution rate is 78.21%. Then, the value of the obtained factor variables is standardized factor score. According to the factor score and its variance contribution rate, the weighted average method is used to calculate the environmental regulation intensity index. The Cronbach coefficient of the selected index is 0.82, indicating that the extraction of common factor is well representative.
In empirical research, there may be endogenous problems caused by measurement errors and missing variables. In order to eliminate the estimation error caused by endogeneity, the average environmental regulation intensity of other respondents except the respondent but with the same income level as the respondent is selected as the environmental regulation tool variable of the respondent. Mainly based on the following considerations: within the same village, the environmental regulation intensity of an individual farmer is highly related to the environmental level of other farmers with the same income level in the village; At the same time, the environmental regulation intensity of other farmers is not directly related to the green technology adoption decision of the individual farmers, so it is strictly exogenous. Similarly, this paper selects “the average value of mandatory environmental regulation of other respondents in the same village with the same income level as the respondents except the respondents”, “the economic incentive mean value of other respondents in the same village except the protected but with the same income level as the respondents” and “the educational guidance mean value of other respondents in the same village except the protected but with the same income level as the respondents” are used as the instrumental variables of the three regulations of farmers respectively.
In this study, 14 variables that have an important impact on the adoption of green breeding technology by farmers are selected as control variables in three categories: respondents’ individual characteristics, family characteristics and external environment.
Among them, the individual characteristics of the respondents include:①Age of the household head. The older the household head, the more likely he or she is to be influenced by traditional habits and experiences, the more inclined he or she is to follow traditional farming patterns, and the less likely he or she is to adopt green farming techniques. ②Years of education. The higher the education level of the household head, the stronger the learning and understanding ability, the more comprehensive the understanding of green production, so the more inclined to adopt green farming technology, and improve its adoption level. ③Health status of the household head. The health status of the household head reflects the quality of the household labor force, and under the current situation of low mechanization level in pig farming, farmers with better hyperthyroidism are more likely to choose to adopt green farming technology (Zhang et al., 2015). ④Degree of risk preference. According to prospect theory, individual decision-making behavior is determined by a combination of factors such as the degree of risk preference and subjective judgment of objective probability (Qiu et al., 2020). In order to avoid production risks and market risks, farmers with a high degree of risk aversion are more inclined to adopt green farming techniques to improve farming efficiency. ⑤Green production perception. Green production perception is the evaluation and perception of green production costs and benefits formed by farmers under the combined effect of their own endowment characteristics and the external policy environment. Farmers’ green farming technology selection behavior is also a technology-integrated investment behavior, and clear value perceptions play an important role in farmers’ green technology selection behavior in the face of large technical risks and unknown factors before they personally practice the behavior.
Individual household characteristics of the respondents included. ①The percentage of farming income. The proportion of farming income reflects the dependence of farmers on pig farming and the degree of specialization, the more dependent the households are on farming income, the more likely they are to adopt green farming technologies with higher benefits. ②The ratio of farming labor. The more the farming labor force, the more the farmers rely on pig farming, but at the same time, since the household labor force has a substitution relationship with technology adoption, the influence of the farming labor force ratio on the adoption of green technology by farmers is uncertain. ③Years of farming. The longer the years of pig farming, the richer the experience0, the more susceptible to traditional thinking and the more accustomed to follow the previous farming model, the less likely the farmers will adopt green farming technology. ④The scale of farming. The larger the scale of the farmer, driven by the scale effect, the more motivated to switch farming mode and more inclined to choose to adopt green farming technology.
External environmental variables include: ①Contract farming participation. Contract farming participation farmers can get timely material support and tool support from contract organizations, and get effective information and technical guidance on green farming technology, so the adoption of green farming technology is higher. ②Group norms. Farming subjects as a member of a specific living environment, members of the surrounding environment have a supervisory and restraining role in their production decision-making behavior, which can lead to the adoption of green pro-environmental behavior of farmers (Xu et al., 2021). ③Social network. In a cognitively limited context, the peer effect of social networks can improve farmers’ ability to obtain information and funds, gain emotional support, and reduce search costs, which are socially critical factors influencing individual decisions. ④Distance from village to livestock station. The more distant villages are generally relatively poor in socioeconomic and informational conditions, and it is difficult to obtain farming-related information. ⑤Neighborhood emulation. Farmers’ new technology adoption behavior decision has a “follow strategy” (Mao et al., 2018), that is, they observe the adoption effect of others before deciding whether they choose to adopt it, and the more opportunities they have to exchange green farming technology with surrounding farmers, the greater the probability of adopting green farming technology.
In addition, the natural resource environment, infrastructure conditions, relevant agricultural policies and market environment in each region will affect the adoption behavior of green breeding technology by farmers, and provincial dummy variables are introduced to control the regional fixed effect. See Table 5 for specific variables and descriptive statistics.
The degree of adoption of green breeding technology by farmers is a continuous variable calculated by the coefficient of variation method, and the following model is constructed:
In formula (3),
Green technology adoption behaviors are not independent, and the three technologies may have complementary or alternative relationships. If a single model is used for step-by-step analysis, it will lead to estimation bias and invalid estimation. The Multivariate Probit (MVP) model allows correlation between multiple decision models, which not only reflects the impact of environmental regulations and their sub-dimensions on the adoption of green technologies by farmers, but also can use the error term correlation coefficient of the regression results of each decision to judge each Substitution or complementarity between technical decision-making behaviors. In order to control the endogenous problems caused by joint decision-making, a ternary probit model is constructed to estimate the farmers’ adoption decisions and related relationships of factor reduction technology (
In Equations 4, 5,
In Equation 6,
Therefore, when farmers are faced with factor input reduction technology, waste harmless treatment technology and fecal sewage resource utilization, depending on one of the three green breeding technologies, the adoption probability can be expressed as
When farmers are faced with the adoption of two of the three green breeding technologies, the adoption probability can be expressed as
When farmers are faced with the adoption of three of the green breeding technologies, the adoption probability can be expressed as:
In order to avoid multicollinearity, stepwise regression is adopted to investigate the impact of environmental regulation intensity on the adoption of green technology by farmers. The regression results of the model are shown in Table 6, model 1) ∼ model (3). According to the results of model 1) and model (2), the R2 and F values adjusted by the model gradually increase with the addition of control variables, indicating that the fitting degree and interpretation strength of the model are gradually improving, and the overall simulation effect is good. The OLS estimation results show that the environmental regulation intensity is significantly positive to the adoption of green technology by farmers at the level of 1%, and the adoption of green technology by farmers increases by 34.50% for each unit of environmental regulation intensity. The 2SLS estimation results considering the reverse causal relationship between the intensity of environmental regulation and the adoption of green technology by farmers show that the intensity of environmental regulation has a positive impact on the adoption of green technology by farmers at a significant level of 1%, that is, after the endogenous is eliminated by the instrumental variable method, the conclusion that environmental regulation significantly improves the adoption of green technology by farmers is still valid, and the adoption of green technology by farmers will increase by 27.04% every time the intensity of environmental regulation increases by 1 unit. This is consistent with the indispensable theory of policy environment under the positive externality of green production. The goal of “ensuring supply, safety and ecology” of environmental regulation induces farmers to change to green production mode to comply with the goal of regulation. Hypothesis H1 was tested.
In terms of control variables, the years of education of the household head had a significant contribution to the degree of green technology choice of the farming households. health status of the household head has a significant positive effect on the degree of green technology choice of the farmers. a significant boosting effect of the percentage of farming income on the degree of green technology selection by farmers a significant contribution of the percentage of farming labor to the degree of green technology choice of farmers social network has a significant positive effect on the degree of green technology choice of farmers; distance to livestock station has a significant negative effect on the degree of green technology choice of farmers. Neighborhood emulation has a significant positive effect on the degree of green technology choice of farmers. In addition, the province dummy variable was partially significant, indicating that there were significant regional differences in the degree of farmers’ green technology choice, probably because the effect of farmers’ green technology choice had different performance in different regions.
The regression results of the impact of environmental regulation on the adoption of green technology by farmers are shown in Table 6, model 4) ∼ model (6). OLS regression results show that mandatory regulation, economic incentive regulation and educational guidance regulation have a significant positive impact on the adoption of green technology by farmers at the statistical level of more than 5%. However, the OLS model does not consider the endogenous problems caused by the reverse causal relationship between the sub dimension of environmental regulation and the degree of green technology adoption of farmers, missing variables or variable measurement deviation. Model 6) is the regression result of instrumental variables. From the regression result of one stage, it can be seen that the village average command mandatory regulation, village average economic incentive regulation and village average education guidance regulation are all significant at more than 10%, indicating that the sub dimension of village average environmental regulation, as the instrumental variable of the sub dimension of environmental regulation, does not have the problem of weak instrumental variables. At the same time, the value of F in the first stage is 32.19, which is greater than the critical value of 16.38 at the 10% level recommended by Andrews and Stock, 2007), indicating that the instrumental variable is reasonable. In addition, from the analysis of exogenous Wald test and DWH test of environmental regulation, it is concluded that environmental regulation has endogeneity in the degree of green technology adoption of farmers, indicating that this endogeneity is the main reason for the bias of OLS estimation results and the change of influence coefficient. Therefore, the regression results are subject to 2SLS regression results.
Table 6 model 6) 2SLS estimation results show that mandatory regulation has a positive impact on the adoption of green technology by farmers at a significant level of 1%. That is to say, after using instrumental variable method to eliminate endogeneity, the conclusion that mandatory regulation significantly improves the technology adoption of farmers is still valid. Moreover, the adoption of green technology by farmers will increase by 3.68% every time the intensity of environmental regulation increases by 1 unit. This is consistent with the research conclusions of Qian and Wang, 2018). Government binding regulation will increase the production cost and punishment risk of farmers. In order to avoid risks, the adoption of green technology by farmers has been improved. Economic incentive regulation has a significant positive impact on the adoption of green technology by farmers at the 1% statistical level. And for every unit of economic incentive regulation, the adoption of green technology by farmers will increase by 6.90%. Incentive regulation includes compensation for green farming, loan support and tax relief. The main reason why farmers are unwilling to adopt green breeding technology is that the environmental improvement they have adopted is shared by others free of charge, and they have not received any compensation. Economic incentive regulation alleviates the cost of green technology adoption by farmers and increases the income of technology adoption through various forms of subsidies and cost reduction. Incentive regulation internalizes the cost of environmental governance to improve the lack of market mechanism and improve the efficiency of resource allocation. Education guided regulation has a significant positive impact on the adoption of green technology by farmers at the statistical level of 5%. And for every unit of education guided regulation, the adoption of green technology by farmers will increase by 7.51%. Educational guidance regulation aims to guide people to form correct ecological and economic values and strengthen people’s awareness of green production through publicity, training and guidance. By improving people’s awareness and understanding of green production, we can promote the adoption of green technology by farmers. The research hypotheses H2, H3 and H4 were verified.
In terms of control variables, in general agreement with the estimated results of the effect of environmental regulation intensity on the degree of green technology choice of farmers, variables such as years of education of the household head, health status of the household head, perception of green production, share of farming income, share of farming labor, social network and neighborhood emulation had significant effects on the degree of green technology choice of farmers.
Green technology is a technology package composed of multiple technologies. According to different technical attributes, technologies can be divided into different types. Based on the classification method of technical attributes by Mao, 2018), this paper divides green breeding technology into three categories: reduction technology, harmless treatment technology and resource utilization technology. Multivariate probit model is used to test the impact of environmental regulation intensity on the technology adoption bias of farmers with different attributes. The results are shown in Table 8. The correlation coefficients of error terms of reduction technology, harmless treatment technology and resource utilization technology are significantly positive at the statistical level of 1%. This shows that there is a significant positive correlation between the adoption of three green technologies by farmers under unobservable factors. The three technologies are more complementary and synergistic, and farmers are more inclined to adopt a variety of green technologies at the same time.
Models 1) to 3) in Table 7 are the MVP estimation results of the bias of environmental regulation intensity towards the adoption of green technologies with different attributes of farmers. According to the estimation results of the model, the intensity of environmental regulation has a significant positive impact on Farmers’ reduction technology, harmless treatment technology and resource utilization technology. However, from the perspective of marginal effect, the impact of environmental regulation intensity on Farmers’ resource utilization technology is higher than that of harmless treatment technology. And its impact on resource utilization technology and harmless treatment technology is higher than that of reduction technology. Under the circumstance of increasingly strict environmental regulation constraints, farmers mainly alleviate environmental pollution in the process of livestock and poultry breeding by means of resource utilization of fecal sewage and harmless treatment of waste.
Models 4) to 6) are the biased estimation results of different attributes of green technology adopted by farmers in different dimensions of environmental regulation. Different dimensions of environmental regulation have different preferences for the adoption of green technology with different attributes of farmers. Among them, the impact of mandatory regulation on Farmers’ harmless treatment technology is significantly higher than that on resource utilization technology, but it has the least impact on reduction technology. This may be related to the authority and irrefutability of mandatory regulation. Limited by the risk of punishment, farmers tend to adopt harmless treatment technologies with low cost and less technical uncertainty. The impact of economic incentive regulation on Farmers’ reduction technology is significantly higher than that on resource utilization technology, and higher than that on harmless treatment technology. The possible reason is that the adoption of capital intensive and environment-friendly technologies has high costs and slow benefits. Economic incentive regulation can not only alleviate the cost of farmers’ adoption of this attribute technology, but also improve the income space of farmers and promote the adoption of green technology. The impact of educational guidance regulation on Farmers’ harmless treatment technology is higher than that on resource utilization technology, but it has the least impact on reduction technology. The possible explanation is that there are many educational guidance regulations to improve the farmers’ awareness of green production and promote the adoption of green technology. Compared with reduction technology and resource utilization technology, which are stricter in capital and technology operation, harmless treatment technology belongs to stable capital and simple operation technology. These safe production technologies include harmless treatment technologies such as incineration and burial of dead pigs and other wastes. Under the influence of the government’s educational guidance regulation of “moistening things silently”, farmers tend to adopt harmless treatment technology in order to pursue production safety and “feel at ease".
China’s livestock and poultry breeding is still dominated by free range farmers and small and medium-sized farms, showing a trend of general part-time, large-scale and professional division of labor (Hu, 2019). Small and medium-sized farmers are often unable to independently purchase or adopt green production machinery and breeding technology due to lack of self-owned funds, limited production scale, technical obstacles and imperfect facilities. Even if some farmers can purchase and use it, it is difficult to give play to the effect of economies of scale (Sun and Liu, 2019). In addition, the ability of information acquisition and policy application is weak, and there are constraints on the production behavior of farmers. These weaknesses reduce the willingness and initiative of farmers for green production. Scale farming can produce economies of scale. Relying on the advantages of economies of scale, farmers can promote the re integration and allocation of capital, labor and other factors in various production links, improve the contribution and synergy of factor input, and promote their technology adoption. In order to test the impact of environmental regulation and its sub dimensions on the adoption of green technology by farmers, taking the mean value of breeding scale as the dividing point, this study divides farmers into “above mean” and “below mean” groups for comparative analysis. The specific results are shown in Table 8.
TABLE 8. Estimation results of the impact of environmental regulation on the adoption of green technology by farmers of different sizes.
According to the estimation results of model 1) and model 3) in Table 8, the intensity of environmental regulation has a significant positive impact on the adoption of green technology by farmers of different sizes. After no correlation test, the p value can be obtained, and its impact on high-scale farmers is more obvious. The high-scale group farmers are more cautious about the adjustment and change of green production decision-making behavior due to the influence of breeding scale. If the external policy environment changes, it will always change its green technology adoption behavior at the first time. However, due to the limitation of capital and scale, the free range farmers households are always in a wait-and-see state to the change of policy environment. Therefore, the intensity of environmental regulation has a more obvious impact on the adoption of green technology by high-scale group farmers.
According to the estimation results of model 2) and model 4) in Table 8, the impact of mandatory regulation on the adoption of green technology by low-scale farmers is more significant. Low scale farmers are generally professional farmers and free range farmers. This part of farmers have higher risk aversion tendency and more obvious response to restrictive regulation. The impact of economic incentive regulation on the adoption of green technology by high-scale farmers is significantly greater than that of low-scale farmers. Economic incentive regulation is non-fixed amount subsidy, and its setting is based on the breeding scale. Only when it reaches a certain breeding scale can it obtain economic incentives and subsidies. This “more work, more pay” economic incentive mechanism for scale operation is more conducive to the adoption of green production behavior by farmers. There is no significant difference in the impact of education guided regulation on the adoption of green technology between high-scale group and low-scale group. The imperceptible role of such government led “top-down” publicity, promotion and training is still the main mode of government promotion.
Using micro survey data, this paper empirically analyzes the impact of environmental regulation intensity and its sub dimensions on the adoption of green technology by farmers. And further discusses the impact of environmental regulation intensity on the endowment heterogeneity of farmers and the adoption of different attribute technologies. The main conclusions are as follows: first, environmental regulation can significantly improve the adoption of green technology by farmers. Every time the intensity of environmental regulation is increased by one unit, the adoption of green technology by farmers will increase by 27.04%; From the perspective of environmental regulation, mandatory regulation, economic incentive regulation and educational guidance regulation can significantly promote the adoption of green technology by farmers. Second, the intensity of environmental regulation can significantly promote the adoption bias of farmers’ reduction technology, harmless treatment technology and resource utilization technology. The impact intensity of environmental regulation intensity on Farmers’ green technology adoption bias is as follows: resource utilization technology is higher than harmless treatment technology and higher than reduction technology; From the perspective of environmental regulation, mandatory regulation, economic incentive regulation and educational guidance regulation can significantly promote farmers’ reduction technology, harmless treatment technology and resource utilization technology. Third, the intensity of environmental regulation has a significant effect on the adoption of green technology by farmers of different sizes, especially for high-scale farmers. At the same time, the sub dimension of environmental regulation has a significant positive impact on the adoption of green technology by farmers. Among them, the impact of mandatory regulation on the adoption of green technology by low-scale farmers is more obvious; The impact of economic incentive regulation on the adoption of green technology by high-scale farmers is significantly greater than that of low-scale farmers; There is no significant difference in the impact of education guided regulation on the adoption of green technology between high-scale group and low-scale group.
Based on the above research conclusions, this paper draws the following policy enlightenment: first, strengthen the role of environmental regulation policy in the adoption of green technology by farmers. The formulation and implementation of environmental regulation should be integrated with local village regulations and cultural customs according to local conditions, so as to ensure the stability and sustainability of the implementation of environmental regulation policies; At the same time, we should strengthen the publicity and promotion of environmental regulation policies. Improve farmers’ awareness and understanding of environmental regulation. Let environmental regulation become a “household name” policy, so as to strengthen the restrictive, incentive and guiding role of environmental regulation on the adoption of green technology by farmers. Give full play to its role in promoting the transformation of old and new kinetic energy of animal husbandry. Second, encourage farmers to continue to adopt green breeding technology. Green technology adoption is the key to breaking through the constraints of green production transformation of farmers. The promotion and restriction factors affecting the adoption of green technology by farmers should be identified at multiple levels and in an all-round way. Rely on the government to increase the popularization and promotion of the necessity of adopting green technology. Create a green production awareness and environment to mobilize the initiative and enthusiasm of farmers in the adoption of green technology, and improve the durability and stability of farmers’ adoption of green breeding technology. The third is to improve the differential impact of capital endowment heterogeneity on the adoption of green technology by farmers. Establish and improve the three major markets of rural capital, labor and insurance. Through the improvement of factor market, the heterogeneity of farmers’ endowment can be alleviated. Combined with the capital endowment differences of farmers of different sizes, formulate differentiated green breeding technology promotion strategies and support policies. For example, for farmers without endowment constraints, focus on the availability and operability of technology; Reduce the access threshold of green production for farmers; Improve the “disconnection” between farmers’ willingness to adopt green technology and behavior.
The original contributions presented in the study are included in the article/Supplementary Materials, further inquiries can be directed to the corresponding authors.
YL: Data Collection, initial version writing; YT: Data curation, Writing- Reviewing and Editing; HW: Conceptualization, Methodology, supervision.
This study is supported by The Ministry of education of Humanities and Social Science project ( No. 20YJA790089); Natural Science Basic Research Program of Shaanxi (No. 2020JZ-17); Shaanxi Soft Science Project (No. 2021KRM051); Shaanxi Social Science Major Theoretical and Practical Issues Research Project (No. 2021ZD1055).
The authors declare that the research was conducted in the absence of any commercial or financial relationships that could be construed as a potential conflict of interest.
All claims expressed in this article are solely those of the authors and do not necessarily represent those of their affiliated organizations, or those of the publisher, the editors and the reviewers. Any product that may be evaluated in this article, or claim that may be made by its manufacturer, is not guaranteed or endorsed by the publisher.
Abate, G. T., Rashid, S., Borzaga, C., and Getnet, K. (2016). Rural Finance and Agricultural Technology Adoption in Ethiopia: Does the Institutional Design of Lending Organizations Matter? World Development 84, 235–253. doi:10.1016/j.worlddev.2016.03.003
Andrews, D., and Stock, J. H. (2007). Identification and Inference for Econometric Models[M]. Cambridge University Press. doi:10.1017/CBO9780511614491
Barnes, A. P., Soto, I., Eory, V., Beck, B., Balafoutis, A., Sánchez, B., et al. (2019). Exploring the Adoption of Precision Agricultural Technologies: a Cross Regional Study of EU Farmers. Land Use Policy 80, 163–174. doi:10.1016/j.landusepol.2018.10.004
Chen, L., Duan, Y., and Qian, W. (2021). Research on Coupling Coordination of Government Open Data Utilization Behavior Based on Coefficient of Variation Method. J. J. Inf. resource Manag. 11 (02), 109–118.
Fen, Li., Junbiao, Z., and Ke, H. (2019). Impact of Informal Institutions and Environmental Regulations on Farmers’ green Production Behavior: Based on Survey Data of 1105 Households in Hubei Province. [J]Resources Sci. 41 (07), 1227–1239.
Ge, J., Wei, Si., and Meng, T. (2021). Study on the Influence Mechanism of Environmental Regulation on enterprise Profit Margin -- Based on the Micro Data of Sugar Factory in Guangxi Zhuang Autonomous Region. J. Management Rev. 33 (08), 66–77.
Haghjou, M., Hayati, B., and Choleki, D. M. (2014). Identification of Factors Affecting Adoption of Soil Conservation Practices by Some Rainfed Farmers in Iran[J]. J. Agric. Sci. Technology 16 (5), 957–967.
Han, Li., Zhao, M., and Lu, Q. (2021a). Has the Livestock and Poultry Prohibition Zone Policy Reduced the Production Capacity of Pigs in China -- an Empirical Analysis Based on County Panel Data. J. Agric. Econ. Issues (08), 12–27.
Han, S., Jia, Xu., and Wang, Y. (2021b). The Impacts of Farmers’ Perceived Benefits on green Fertilization Behaviors under Environmental Regulations. J]Research Agric. Modernization 42 (05), 880–888.
Hou, L., Chen, X., Kuhn, L., and Huang, J. (2019). The Effectiveness of Regulations and Technologies on Sustainable Use of Crop Residue in Northeast China. Energ. Econ. 81, 519–527. doi:10.1016/j.eneco.2019.04.015
Hu, W. (2019). Zhang Jinhua, Chen Zhaojiu Small Farmers and Large-Scale Production: Agricultural Land Scale and Agricultural Capitalization——Taking Agricultural machinery operation service as an example. [J] Agric. Technol. economy (06), 82–96.
Huang, Z. H., Zhong, Y. Q., and Wang, X. L. (2016). Study on the impacts of government policy on farmers’pesticide application behavior. J. China Population, Resour. Environ. 26 (8), 148–155.
Jacquet, F., Butault, J.-P., and Guichard, L. (2011). An economic analysis of the possibility of reducing pesticides in French field crops. Ecol. Econ. 70 (9), 1638–1648. doi:10.1016/j.ecolecon.2011.04.003
Kolady, D. E., Van der Sluis, E., Uddin, M. M., and Deutz, A. P. (2021). Determinants of adoption and adoption intensity of precision agriculture technologies: Evidence from South Dakota. Precision Agric. 22 (3), 689–710. doi:10.1007/s11119-020-09750-2
Li, X. (2019). Financial Strategy Choice for Solving the Financing Dilemma of Pig Breeding. Beijing, China: University of international business and economics.
Man, M. J., Zhou, M. L., and Li, T. S. (2010). An analysis of the differences in farmers' households' behaviours of adoption of agricultural technology with different natures- based on survey to Shaanxi Province, Gansu Province and Ningxia Hui Autonomous Region[J]. Chin. Rural Economy (2), 68–78.
Mao, H. (2018). Research on Farmers' Technology Adoption Behavior from the Perspective of Contract Agriculture [D]. Nanjing, China: Nanjing Agricultural University.
Mao, H., Zhou, Li., and Ying, R. (2018). Farmers’ Risk Preferences and Their Technology Adoption Behavior: An,Examination from the Perspective of Contract Farming. J. Chin. Rural Economy (04), 74–89.
Mei, L. L., Liu, Z., and Qiang, D. (2018). Psychological cognition of pollution prevention of family-oriented scale pig breeders and environmental regulation influence effects. [J]Chinese J. Eco-Agriculture 26 (01), 156–166.
Moser, R., Pertot, I., Elad, Y., and Raffaelli, R. (2008). Farmers' attitudes toward the use of biocontrol agents in IPM strawberry production in three countries. Biol. Control. 47 (2), 125–132. doi:10.1016/j.biocontrol.2008.07.012
Mueller, W. (2013). The effectiveness of recycling policy options: waste diversion or just diversions? Waste Management 33 (3), 508–518. doi:10.1016/j.wasman.2012.12.007
Qian, Li., and Wang, Y. (2018). The choice of government behavior in the resource utilization of livestock and poultry waste -- incentive or Punishment. J. Rural Economy (09), 55–61.
Qiu, H., Fang, S. L., and Zhang, Yi. (2020). Risk Preference, Risk Perception and Farmers’Adoption of Conservation Tillage. [J] Chin. Rural Economy (07), 59–79.
Si, R., Lu, Q., and Zhang, S. (2020). Impact of environmental regulation on Farmers' resource treatment behavior of sick and dead pigs -- Based on the survey data of Hebei, Henan and Hubei. [J] Agric. Technol. economy (07), 47–60. doi:10.1177/0734242X19878496
Sun, H., Edziah, B. K., Kporsu, A. K., Sarkodie, S. A., and Taghizadeh-Hesary, F. (2021a). Energy efficiency: The role of technological innovation and knowledge spillover. Technol. Forecast. Soc. Change 167, 120659. doi:10.1016/j.techfore.2021.120659
Sun, H., Edziah, B. K., Sun, C., and Kporsu, A. K. (2021b). Institutional quality and its spatial spillover effects on energy efficiency. Socio-Economic Plann. Sci., 101023. doi:10.1016/j.seps.2021.101023
Sun, X., and Liu, Y. (2019). Can land trusteeship drive farmers' green production? [J]. China Rural Economy (10), 60–80.
Tian, C., and Chen, Y. U. (2021). Calculation and evaluation of China's inter provincial agricultural carbon emission level based on SIS. [J] J. Nat. Resour. 36 (02), 395–410. doi:10.31497/zrzyxb.20210210
Wei, Li. B., and Xu, X. (2017). Social networks, information flows and farmers' adoption of new technologies - A re-test of Granovetter's weak relationship hypothesis. [J]Journal Agrotechnical Econ. (12), 98–109.
Xiao, J., Chen, z., and Liu, Fei. (2020). Will participating in e-commerce promote the adoption of green production technology by Kiwi growers—— Counterfactual estimation based on propensity score matching. J. China Rural Economy (03), 118–135.
Xu, Li., Jin, W., and Chen, Ke. (2021). Breeding scale external restrictions and pig farmers’pro environmental behavior. [J] J. Arid Land Resour. Environ. 35 (04), 46–53.
Xu, Z. G., Zhang, J. Y., and Lv, K. Y. (2018). The scale of operation, term of land ownership and the adoption of inter- temporal agricultural technology: An example of“straw return to soil directly”[J]. China Rural Economy (3), 61–74.
Yin, X. (2019). Policies and practical examples of green transformation and development of animal husbandry in China. J. Chongqing Soc. Sci. (03), 18–30.
Yu, L., Zhang, W., and Xi, B. (2019). Research on the forced effect of environmental tax on enterprise green transformation. J. China Population, Resources Environment 29 (07), 112–120.
Zhang, Y., hong, Z., and Xiang, M. (2015). Study on the Influence of Family Endowments on the Environmental Behavior of Massive Pig Farmers Under the Situation of Ecological Compensation Policy: Based on the Survey of 248 Massive Pig Farmers in Hubei Province. [J] Issues Agric. Economy 36 (06), 82–91+112.
Zhang, Yu. (2016). An Empirical Study on the Relationship between Environmental Risk Perception. Wuhan, Hubei: environmental regulation and environmental behavior [D] Huazhong Agricultural University.
Keywords: environmental regulation, green technology adoption, pig breeding, economic incentive regulation, educational guidance regulation, command mandatory regulation
Citation: Lu Y, Tan Y and Wang H (2022) Impact of Environmental Regulation on Green Technology Adoption by Farmers Microscopic Investigation Evidence From Pig Breeding in China. Front. Environ. Sci. 10:885933. doi: 10.3389/fenvs.2022.885933
Received: 28 February 2022; Accepted: 21 March 2022;
Published: 08 April 2022.
Edited by:
Huaping Sun, Jiangsu University, ChinaReviewed by:
Ke He, Huazhong Agricultural University, ChinaCopyright © 2022 Lu, Tan and Wang. This is an open-access article distributed under the terms of the Creative Commons Attribution License (CC BY). The use, distribution or reproduction in other forums is permitted, provided the original author(s) and the copyright owner(s) are credited and that the original publication in this journal is cited, in accordance with accepted academic practice. No use, distribution or reproduction is permitted which does not comply with these terms.
*Correspondence: Hongmei Wang, d2htZWNvQG53c3VhZi5lZHUuY24=; Yongfeng Tan, dGFueW9uZ2Zlbmc0MjBAbndhZnUuZWR1LmNu
Disclaimer: All claims expressed in this article are solely those of the authors and do not necessarily represent those of their affiliated organizations, or those of the publisher, the editors and the reviewers. Any product that may be evaluated in this article or claim that may be made by its manufacturer is not guaranteed or endorsed by the publisher.
Research integrity at Frontiers
Learn more about the work of our research integrity team to safeguard the quality of each article we publish.