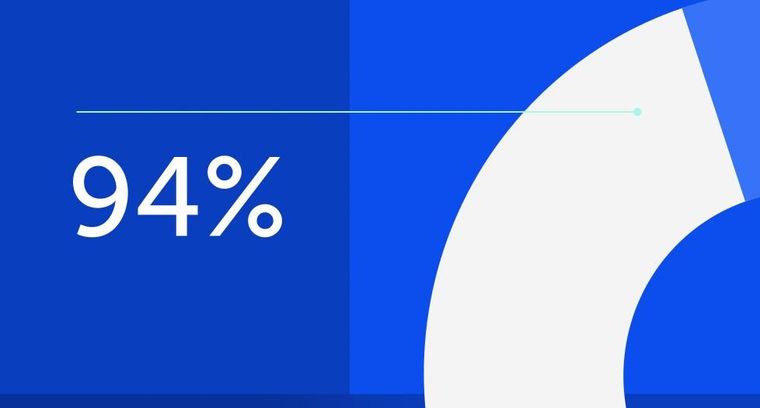
94% of researchers rate our articles as excellent or good
Learn more about the work of our research integrity team to safeguard the quality of each article we publish.
Find out more
ORIGINAL RESEARCH article
Front. Environ. Sci., 16 June 2022
Sec. Environmental Economics and Management
Volume 10 - 2022 | https://doi.org/10.3389/fenvs.2022.884965
This article is part of the Research TopicThe Nexus Between the Transportation Sector and Sustainable Development Goals: Theoretical and Practical ImplicationsView all 9 articles
Under the background of the rapid development of high-speed rail (HSR), the urban spatial distance has been significantly compressed. Exploring the impact of high-speed railways on urban consumption from a spatial perspective is conducive to further understanding and clarifying the spatial economic effects caused by the opening of high-speed railways and providing a scientific basis for the future spatial layout of high-speed railways. Based on the panel data of prefecture-level cities from 2008 to 2018, the spatial spillover effect of high-speed railways on urban consumption is explored and decomposed by building a spatial econometric model. The results show that urban consumption shows significant spatial correlation, and the conclusion is still valid under three different forms of spatial weight matrices. In addition, the high-speed railway network has a positive effect on local urban consumption and acts on cities in neighboring areas through a spatial spillover effect. Further analysis shows that the spillover effect has an effective radius of 100–500 km, which is inverted U-shaped with distance, and 300 km from the central city is the optimal radius.
For the most part, in the new economic geography theory, the development of traffic infrastructure is considered one of the critical drivers of economic development (Wang et al., 2020). Under the influence of the idea of “building roads before getting rich,” many people believe that transportation infrastructure, especially modern highways and high-speed rails, is always beneficial to all aspects of regional economic development. In terms of rail construction, since the first high-speed rail opened in 2008, China’s high-speed rail construction has shown a “blowout” of growth. By the end of 2020, China’s operational mileage of high-speed rails has reached 38,000 km, more than 70% of the “eight vertical and eight horizontal” main high-speed rail network skeletons have been built, and the national high-speed rail network has covered 94.7% of cities with a population of more than 1 million. China has become the country with the longest operational mileage and the largest construction scale of high-speed rail all around the world, entering the “high-speed rail era.”
The relationship between transportation infrastructure and economic growth has been confirmed by numerous theoretical and empirical studies (Straub, 2011; Magazzino and Mele, 2021). As a large-scale inter-regional transportation infrastructure, the massive investment, construction, and operation of high-speed rail have not only changed passengers’ travel choices and transportation patterns (Chen, 2012) but also reshaped China’s spatial economic layout and accelerated the pace of urbanization in an unprecedented way (Li et al., 2016). The launch of HSR has made cities more accessible (Levinson, 2012) and expanded the space for the agglomeration and diffusion of economic factors (Banerjee et al., 2020). However, according to existing study evidences, the impact of HSR on the economic growth of cities still does not seem to reach a certain conclusion. Some studies have found that the accessibility and lower transportation costs brought by HSR can stimulate regional economic growth (Ahlfeldt and Feddersen, 2018; Shirley and Winston, 2004; Zhang et al., 2019). While some other studies found that HSR is expected to have different impacts on core cities and surrounding cities, especially while promoting economic growth in core cities, it may lead to a negative economic impact on surrounding cities (Garmendia et al., 2012; Cao et al., 2013; Faber, 2014; Qin, 2017).
Although the present article focuses primarily on the economic impact and social impact of HSR, research on the direct impact on consumption is still lacking. Consumption is inextricably related to regional economic development, but it is not the same as the regional economy. It is an important link in regional economic development and a key factor in promoting regional economic development (Zhang et al., 2021). The launch of HSR has greatly improved the convenience of travel conditions for passengers and reduced inter-city transportation costs of persons and goods (Hong et al., 2011). Urban residents’ consumption is also affected by the launch of HSR, especially in industries closely related to transportation infrastructure, such as tourism, catering, and service industries (Chen et al., 2016; Delaplace et al., 2014). Our study will focus on the consumption impact of the launch of HSR. There is also a substantial body of literature that has verified that transportation infrastructure has significant spatial spillover effects (Hulten et al., 2006; Yu et al., 2013). Based on these studies, our research will answer the following questions: Can the launch of HSR bring a spatial increase in urban consumption? Does this consumption effect have a differential impact on local-adjacent cities? Is this spatial spillover effect related to geographical distance?
Our study will systematically and scientifically analyze the spatial spillover effect of high-speed rail on urban consumption. The findings of this study can not only deepen the research on local urban consumption but also help analyze the effect of its influence on urban consumption in the adjacent areas, so as to better play the driving role of the central city, narrow the consumption gap in the surrounding cities, and change the spatial structure of the region. At the same time, our study will provide valuable experience for the construction of high-speed railways in other countries and regions, so that the spatial pattern of urban consumption as well as the regional economy can be planned more rationally.
There are three possible contributions to the previous literature. First, many articles have mainly focused on the effect of HSR on regional economies, but ignored the exploration of urban consumption. We separate urban consumption from regional economic research and consider the uniqueness of urban consumption. By applying panel data to prefecture-level cities from 2008 to 2018, we empirically explore the spatial effect of HSR on urban consumption with three different types of spatial matrices. Second, differing from existing studies that focus only on the local urban consumption effects of high-speed rail networks, we examine the urban consumption effects of high-speed rail networks in adjacent cities and examine the spatial role characteristics of urban consumption effects in adjacent cities on this basis, which enriches the relevant studies on the effects of HSR on urban consumption. Third, according to the law of spatial distance decay effect, this study tries to explore the effective radius of the impact of HSR on urban consumption, which enables to provide a scientific support for urban consumption development and decision-making.
The rest of the article is organized as follows: Section 2 provides theoretical analysis and hypotheses. Section 3 describes the data and spatial model. Section 4 presents the results and analysis. Section 5 discusses further study to explore the effective impact radius. The implications and conclusion are in Section 6. Limitations and future research are in Section 7.
The positive spatial spillover effects of transportation infrastructure on the regional economy have been widely confirmed (Yu et al., 2013; Liu et al., 2019; Magazzino and Giolli, 2021). As a large-scale transportation infrastructure, high-speed rail has had an unprecedented impact on people’s travel and life. During the long-time form establishing to opening, HSR have contribute to increase a large amount of job opportunities (Hiramatsu, 2018), which will attract more labor people. Undoubtedly, labor growth will lead to an increase in the total population and then boost total consumption. Urban residents’ income and consumption levels are also promoted by HSR, which has significantly reduced geographical distance and economic distance. Hiramatsu. (2018) pointed out that opening HSR and lowering the travel cost may lead to population migration, changing the spatial distribution of population. Several empirical studies have demonstrated that transportation infrastructure plays an important role in realizing urbanization and regional integration (Atack et al., 2010; Verma et al., 2013). On the other hand, some cities in China choose to establish HSR stations in the suburbs or new districts. The areas near the stations are often where public service facilities gather. Therefore, the area around high-speed rail stations will gradually develop into a new economic growth point in cities, which will reshape the urban economic spatial pattern.
The spatial effect of opening HSR has exerted an agglomeration force and a dispersion force on urban space at the same time (Holl, 2004). Under the combined influence of the two forces, two spatial patterns will take shape, i.e., the “core-edge” spatial pattern and the “multi-center” pattern. On the one hand, HSR lines have significantly enhanced the accessibility of cities. The reduction of inter-city trade costs may make the agglomeration force bigger than the dispersion force, resulting in the spatial competition and disparity between core cities and surrounding cities (Zhang et al., 2019). Because of location advantages and city development levels, core cities have natural advantages in transportation, manpower, knowledge, and information compared with surrounding cities (Guirao et al., 2017). On the other hand, with the increase of population density in core cities, the limited land resources will become tense, the land price will rise and the cost of commercial housing and industrial land will also be raised. At the same time, the production and labor costs of enterprises will also increase, and the production and living cost of core cities will rise accordingly. Zheng and Kahn. (2013) found that the HSR promotes cooperation and exchange between the surrounding cities and the core cities. The high housing prices and relatively lower quality of life in big cities make many families choose to work in big cities and live in nearby small cities to save costs. The launch of HSR has also influenced enterprises’ locational decisions (Urena et al., 2009). Some consumption-related companies have been attracted to move to surrounding cities to save labor costs, which will further influence the relative location and the distribution of consumption resources, narrowing the economic gap between surrounding cities and core cities. The high-speed rail made cities connected with a railway network, which brought accessibility to shift production market and labor structure (Cheng et al., 2015).
The impact of the high-speed rail network on urban consumption is complex and multifaceted. Previous studies have shown that the opening of high-speed rail can significantly improve the level of urban consumption (Zhang et al., 2021). The temporal and spatial distance between cities is compressed, and the high-density high-speed rail network makes the effect of urbanization and regional integration more obvious. The realization of 1-h, 2-h and 3–5-h high-speed rail traffic circles centered on the core cities allows cross-regional mobility consumption such as working and living in two places; cross-city consumption; medical treatment in different places gradually changes to normalization. On the other hand, the convergence effect of the opening of high-speed rail on the regional spatial pattern has promoted the rapid flow and redistribution of urban human capital, information resources, knowledge, and technology in the region. The boundaries of urban administration have gradually become blurred, the administrative boundaries of production factors flow have been broken, more and more public infrastructure and service functions have been shared, which drives the flow and reorganization of elements and resources in a wider space to achieve a closer economic space community (Vickerman, 2018). The optimization of consumption space enables enterprises to obtain production factors in a larger market geographical space, and consumers can also purchase higher-quality services and heterogeneous goods. According to the theory of spatial externality, within a certain spatial scope, the economic development of a certain region will be affected not only by its own development conditions and capital investment, but also by the surrounding regions, and it will also have an economic impact on the surrounding regions (Long et al., 2018). Therefore, while promoting the overall improvement of consumption in high-speed rail core cities, high-speed rail will also have a spatial spillover effect on urban consumption in adjacent areas (Liang et al., 2020). High-speed rail relies on its own advantages to drive the circulation of factors between regions, connect economic activities, and produce positive spatial spillover effects. That is to say, the high-speed rail makes the cross-regional flow of production factors such as knowledge, information, capital, and technology a reality, which will further drive the consumption level of surrounding cities and show the positive spatial correlation of urban consumption (Ortega et al., 2012; Zheng et al., 2019).
Therefore, based on the aforementioned analysis, this study proposes the following hypotheses:
Hypothesis 1. HSR network has a positive effect on local urban consumption, and it also acts on adjacent consumption through a spatial spillover effect.The “First Law of Geography” holds that everything has a certain spatial dependence (Campante and Yanagizawa-Drott, 2018), and this dependence will show different strengths depending on the distance. The closer the distance is, the more significant the effect will be (Anselin, 1988). However, following the geographic distance decay characteristics, with the increase of geographic distance, the rise of transportation costs and time costs will suppress the possibility of spillover to a certain extent. Based on spatial measurement methods, most scholars have also verified that the spillover effect of regional economic growth is affected by geographical distance to a certain extent. The opening of high-speed rail has greatly reduced the temporal and spatial distances between cities, but the influence of distance shortening on core cities and surrounding cities is different (Monzón et al., 2013). For core cities, the shortening of city distance promotes the flow of production factors, expands the scope of consumption, and increases the trading space for high-quality commodities, which has a positive effect on promoting urban consumption. On the other hand, due to core cities have natural economic advantages, the opening of the high-speed rail has consolidated their central advantages and accelerated the speed and frequency of resources flowing into core cities, thereby promoting consumption in core cities (Wang et al., 2018). For the surrounding cities, the opening of high-speed rail reduces the distance between the surrounding cities and the central city and increases the economic resources, human capital, knowledge, technology, information, and other elements that the surrounding cities obtain from the central city, thereby driving the improvement of urban consumption levels (Babalik-Sutcliffe, 2016); on the one hand, the opening of high-speed rail has accelerated the flow of resources from surrounding cities to core cities, and the convenience of residents in surrounding cities to consume in core cities, which also led to the loss of consumption and inhibited urban consumption. Therefore, distance has two sides to the city. There are two directions of action, “the agglomeration force” and “the dispersion force.” Under the dual action of these two forces, the spatial spillover effect of high-speed rail on urban consumption may have an optimal radius. Considering that the spatial spillover effect has obvious geographical attenuation characteristics, the expansion of distance between cities will significantly weaken the spillover effect (Ahlfeldt and Feddersen, 2018). Between nearby cities, the construction of high-speed rail provides convenient channels for urban consumption, expands the spatial scope of consumption, promotes the flow of production factors and technical information among cities through the high-speed rail network, and accelerates the circulation and sharing of goods and services within the region, which is helpful to promote the spatial spillover of urban consumption power and improve the consumption level of regional cities (Diao, 2018). However, between cities with farther distances, the time cost and economic cost of consumers taking high-speed rail will increase significantly with the increase of distance. The increase in cost due to distance changes will lead to a weakening of the effect of high-speed rails on urban consumption in adjacent areas.Therefore, based on the aforementioned analysis, this study proposes the following hypotheses:
Hypothesis 2. The spillover range of the impact of high-speed rail on urban consumption is limited, that is, there is an effective radius.
Considering the variability of urban consumption levels in various regions of China, this article adopts a spatial autocorrelation test and establishes a spatial econometric model to empirically analyze the impact of high-speed rail on urban consumption levels, with a view to provide useful references for promoting the improvement of urban consumption levels.
Spatial autocorrelation is a valid measure of the degree of aggregation of spatial unit attributes. It is called “Tobler’s first law of geography” and includes two types of indicators: global autocorrelation and local autocorrelation (LeSage, 2014).
In econometrics, it leads to the use of the global Moran’s index, which is more widely applicable and stable, to measure the overall spatial global correlation of the region. Eq. 1 is shown as follows:
where
In order to analyze the spatial differences of different regions within the study area, we introduced a local spatial autocorrelation model, which is calculated as (2):
where
In addition, Moran scatter plots are often used to observe local spatial correlation characteristics, which can divide the whole space into four quadrants to correspond to four local spatial correlation patterns (Table 1).
Cities located in quadrant I and quadrant III indicate a strong positive spatial correlation in consumption levels, while cities located in quadrant II and quadrant IV have a strong negative spatial correlation in consumption.
The spatial spillover effect can be accurately measured by setting a reasonable spatial weight matrix, which is the fundamental work for conducting spatial econometric analysis. Given all possible spatial weight matrices, we will select three spatial weight matrices to test the local-adjacent city effect of a high-speed rail network.
(1) Adjacent spatial weight matrix (W1)
The geographical adjacency space matrix is selected to measure the spatial effect between cities; that is, if there are geographically connected edges or points between geospatial unit i and geospatial unit j, it is considered that there is a spatial adjacency relationship. On the contrary, if there are no geographically connected edges or points between geospatial unit i and geospatial unit j, it is considered that there is no spatial adjacency relationship. The specific publicity expression is as follows:
Standardized processing is adopted to reflect the spatial weighted average value of other adjacent geospatial units to this geospatial unit more reasonably. Specifically, each element in the matrix is divided by the sum of elements in the row at the same time, so as to ensure that the sum of elements in each row is 1,
(2) Geography distance spatial weight matrix (W2)
The adjacent spatial weight matrix is only based on whether different geographical spatial units are geographically connected, but there is a deviation from the objective reality in practical application, so it is necessary to further use geographical distance to construct spatial weight indicators. Common geographical distance spatial weight matrices are as follows:
In Eq. 5,
(3) Economy distance spatial weight matrix (W3)
The absolute or relative position of geographical units in space is not the only factor that influences spatial correlation. Economic activities such as trade exchanges and financial transactions between regions will also have an effect on spatial units. Cities with different economic levels have different impacts on neighboring cities, so the spatial weight matrix can be constructed according to the reciprocal of the per capita GDP gap. The specific publicity expression is as follows:
where
Spatial econometric models can effectively analyze the spatial effects among elements, especially when there are spatial autocorrelations among the studied elements, and the estimation by spatial econometric models can make the research results more accurate. Spatial lag model (SLM), spatial error model (SEM) and spatial Durbin model (SDM) are three common spatial econometric models. Their models are constructed as follows:
where
For studying different issues and spatial effects, it is extremely important to choose the appropriate spatial econometric model. The steps of the determination are: first, the Lagrange multiplier test (LM) and robust Lagrange multiplier test (Robust LM) are selected to determine the model. Compared with the Wald test and LR test, the LM test is more suitable for the spatial econometric model. The suitable spatial econometric model can be selected and determined by referring to the results of the spatial interaction effects shown by the LM test. If there is only spatial error in the results, the SEM is more suitable; if there is only a spatial lag effect in the results, the SLM is more suitable; if both effects are present, the SDM is more suitable; if neither effect is present, the general econometric model is chosen. The LM test results are listed in Table 2.
The results show that: both the SEM and the SLM results are significant (p < 0.01), rejecting the original hypothesis, and the SEM has better results compared to the SLM. Next, to test whether the SDM can be reduced to a spatial lag model, the Wald test and the LR test are performed, and the results are shown in Table 3.
The results show that the Wald test and LR test results pass the 10% significance test for both the SLM and the SEM, rejecting the original hypothesis that the SDM simplifies to a SLM, and the optimal choice is a two-way fixed SDM according to the spatial model discriminant criterion proposed by Elhorst (2010).
The focus of this article is on the impact of high-speed rail on “local-adjacent” urban consumption, and the preliminary spatial econometric model is constructed because of the possible spatial dependence between regions, as shown in Eq. 7:
where
The impact effects generated by high-speed rail need enough time to manifest their effects, and a data set covering a sufficiently long period of time is necessary to properly assess the impact of high-speed rail on urban consumption (Ciccarelli, Magazzino, & Marcucci, 2021). Therefore, based on the perspective of the “local-neighborhood” effect, this article empirically analyzes the spatial spillover effects of high-speed rail networks on urban consumption in prefecture-level and above cities in China from 2008 to 2018.
(1) Explained variable: urban consumption level (CONS). The retail sales of social consumer goods are the most direct data of urban consumption demand, which reflects the scale of the retail market, commodity purchasing power, and the material and cultural living conditions of urban residents in a certain period. This article selects the total retail sales of social consumer goods per capita in each prefecture-level city to objectively measure the urban consumption level.
(2) Explanatory variables: the high-speed rail network centrality (HDC) index is used to characterize high-speed rail network variables. The interaction term of the dummy variable before and after the opening of the high-speed rail and the degree centrality are used. The dummy variable of the year before the opening of high-speed rail is taken as 0, and the dummy variable of the year after the opening of high-speed rail is taken as 1. Referring to the practice of Arafat and Bressan, (2017), the high-speed rail network is defined using the undirected and weightless form of P space, that is, if the same high-speed rail line passes through two cities, it is assigned a value of 1; otherwise, it is assigned a value of 0. If there are different routes passing through the same two cities, the value is still 1. The specific calculation method is as follows:
where k represents the number of cities connected with city i in the high-speed rail network consisting of n-node cities. Degree centrality measures the degree of direct connection between a node and other nodes and reflects the importance of the node in the network. The larger the index is, the more associated cities the node city has, which means the network connection breadth is stronger.
(3) Control variables: There are many factors that affect the level of urban consumption. Drawing on the existing relevant research on urban consumption and considering the availability of data, this article selects the following control variables: 1) economic level (PerGDP), which measures the degree of urban economic development with per capita GDP; 2) income, whether it is the absolute income hypothesis, the relative income hypothesis, the life cycle theory or the permanent income hypothesis, all demonstrate the decisive role of income on consumption, and the average wage is used to measure the income level of residents; 3) industrial structure (Ind), expressed by the ratio of the added value of the tertiary industry to GDP; 4) government intervention degree (Gov), calculated by the ratio of local fiscal budgetary expenditure to GDP; and 5) information level (Inform), which is expressed by the ratio of per capita total post and telecommunications business to per capita GDP. In order to eliminate the influence of data heteroscedasticity, this article takes the logarithm of some economic variables, and the descriptive statistical results of each variable are shown in Table 4.
(4) Data Sources: The economic data for this study covers information on prefecture-level cities from 2008 to 2018, sourced from the statistical yearbooks of each province and city, the China City Statistical Yearbook, and the CSMAR database. The data of the China’s high-speed rail network comes from the high-speed rail route data (CRAD) from China Research Data Service Platform (CNRDS), and the high-speed rail network data of each year is obtained by matching the three data sets of high-speed rail train information, high-speed rail line information, and high-speed rail station opening times in CRAD. We match the high-speed rail line information with the high-speed rail station opening time and exclude the freight and stop lines to get all the station names belonging to the high-speed rail network from 2008 to 2018 and then match them with the high-speed rail train information data to get the high-speed rail network from 2008 to 2018.
We used three spatial weight matrices and Stata15 software to examine global Moran’s I for the urban consumption of 285 prefecture-level cities in China from 2008 to 2018 (Table 5).
It can be seen from the results that the Moran’s I index of the urban consumption level from 2008 to 2018 is all positive and passes the test at the level of 1% significance, indicating that there is a positive spatial dependence of the urban consumption level, that is, a city with a higher consumption level often has a higher consumption level in its surrounding cities, and vice versa. Under the W1 weight matrix, the maximum value of Moran'I is 0.347, the minimum value is 0.176, and the p-value is 0.000, all at the 1% significance level. Under the W2 weight matrix, the maximum value is 0.118, the minimum value is 0.076, and the p-value is 0.000, all at the 1% significance level. Under the W3 weight matrix, the maximum value is 0.347, the minimum value is 0.237, and the p-value is 0.000, all at 1% level of significance. Under the W2 weight matrix, the maximum value is 0.246, the minimum value is 0.176, and the p-value is 0.000, all at the 1% significance level. This indicates a clear global spatial agglomeration characteristic of urban consumption, and the spatial correlation showed an upward trend year by year.
Figure 1 shows selected Moran’s I scatter plots for 2005, 2008, 2013, and 2018, respectively. It can be seen that Moran’s I index of urban consumption at the national level is mainly distributed in the first and the third quadrant, which indicates that urban consumption presents a high–high (H-H) and low–low (L-L) spatial agglomeration state, and there is a significant positive correlation with spatiality.
From a regional perspective (Figures 2–5), the consumption level of the southeastern coastal cities dominated by the Yangtze River Delta and the Pearl River Delta shows a high–high agglomeration feature, indicating that these cities have a high consumption level, and the consumption level of the surrounding cities is also high, with little difference. However, the local Moran’s index of the consumption level of cities, mainly in the western region and the northeast region, shows the characteristics of a low–low agglomeration, indicating that the consumption level of these cities is low, and the consumption level of the surrounding cities is also low. Overall, China’s urban consumption level still shows obvious regional differences and gradient distribution characteristics, which also shows the rationality of using spatial econometric models for research.
Table 6 reports the benchmark regression results based on the SDM (Eq. 7). It can be seen from the results in Table 6 that, under the assumptions of three different spatial weight matrices, the general regression coefficient and spatial regression coefficient of the HSR network variables are both greater than zero and pass the 1% significance level test, which shows that the improvement of the high-speed rail network also plays a positive role in promoting the local urban consumption effect under the action of different spatial weight matrices. Compared with the spatial weight matrix of adjacent and economic distance, urban consumption under the spatial weight matrix of geographical distance shows a weaker positive spatial spillover effect, and it can be seen that the administrative division is one of the hindering factors affecting economic spatial activities.
We believe that the relocation of labor and economic factors of production between high-speed rail cities and non-high-speed rail cities by means of high-speed rail will bring about changes in urban consumption levels. Under the conditions of high-speed rail travel, the transportation time and transportation costs of production factors are significantly reduced. Consumers are also able to purchase local quality services and goods from geographically distant cities or businesses, and the availability of heterogeneous goods is significantly enhanced. In China, cross-regional consumption such as cross-province travel, cross-city medical treatment, and cross-city shopping has already become common (Shao et al., 2017). With the launch of HSR, the city’s location advantage will be enhanced, which will attract more talents and enterprises to gather. The improvement of enterprise efficiency and the increase of jobs will attract more individuals to participate in labor, and personal income will gradually increase (Jia et al., 2017). With the increase of per capita income, the consumption ability will be enhanced, providing stronger growth impetus for the increase of total consumption.
The model introduces control variables such as urban economic level, residents’ income, industrial structure, government behavior, and information technology. The effects of urban economic development (PerGDP) and industrial structure (Ind) on urban consumption are significantly positive, which indicates that the improvement of urban economic development and the optimization of industrial structure can significantly increase the consumption level of urban residents. The results of Wang et al. (2020) are consistent with the results reported in this article. HSR improves regional accessibility and significantly reduces transportation, information, and transaction costs, thereby increasing market potential and expanding the spatial scope of transactions. As a passenger-only railroad, high-speed railways have a significant impact on the flow of people, increasing the concentration density of the service industry, which in turn increases the value added and share of the tertiary industry (Wang et al., 2020). Income has the most significant effect on consumption, and the results are consistent with the income determination theory, i.e., residents’ current income has an absolute effect on consumption expenditure (Viceira, 2001; Akerlof, 2007).
According to the New Economy Geography theory introduced by Krugman (1991), there are two economic forces that make the flow of economic activities occur in space: the agglomeration force and the dispersion force. The agglomeration force is conducive to gathering economic activities and factors around large core cities, gradually reinforcing the siphon effect and thereby expanding the spatial disparity. At the same time, the dispersion force is conducive to spreading economic activities and factors to different locations, balancing the amount of economic activities between core cities and surrounding small cities. Chen and Hall (2012) pointed out that HSR’s effects are differentially on different kinds of cites; large cities will be reinforced, but smaller cities will not. In other words, the effects of HSR may eventually reshape the spatial pattern of the regional economy.
Therefore, we further try to decompose the spatial spillover effect into direct and indirect effects, and the results are shown in Table 7. It can be seen that under the assumptions of three different spatial weight matrices, the direct effect, indirect effect, and total effect of the high-speed rail network on urban consumption are all positive and passed the significant test. This indicates that not only the opening and development of HSR in the local region will promote the increase of consumption in the local region, but also the construction of high-speed rail in other regions adjacent to the region will help to enhance local consumption. In other words, HSR development will not only enhance urban consumption in local regions but also help enhance urban consumption in adjacent regions, i.e., there is a spatial spillover effect of the high-speed railroads. Wang et al. (2019) also reached the same conclusion. Numerically, compared with the indirect effect, the direct effect of the high-speed rail network is more significant, indicating that the opening of the high-speed rail and the improvement of the network will first promote the consumption level of the cities in the local region and then drive the consumption growth of adjacent cities, resulting in a superimposed linkage effect. The H1 hypothesis is verified.
In the above empirical results, under three different spatial weight matrices, the spatial spillover estimation results of the high-speed rail network on urban consumption have shown that the results remain robust. However, considering the multi-angle characterization of high-speed rail variables, this article selects the dummy variable of high-speed rail opening and the number of high-speed rail lines as replacement variables to test the robustness again. The results are shown in Table 8. Both the dummy variable of the opening of the high-speed rail and the variable of the high-speed rail line have a significant positive spillover effect on urban consumption, which is basically consistent with the benchmark regression results, indicating that the aforementioned results are robust.
From the aforementioned regression results, we can see that the neighborhood consumption effect of the HSR network is consistent with the local effect, i.e., HSR helps to increase the consumption level of adjacent cities. Then, what is the effective spatial radius of this adjacent consumption effect? What is the optimal radius of the high-speed rail network for the consumption level of the adjacent cities? To answer these questions, we constructed spatial weight matrices with different distance thresholds using 100, 200, 300, 400, and 500 km as thresholds, to examine the spatial characteristics of the effect of high-speed rail on the local-adjacent urban consumption effect. The results are shown in Table 9.
It can be seen from Table 9 that the spatial spillover effect of high-speed rail on urban consumption has different significance under different spatial distance radii. The regression coefficient within 100 km from the central city is not significant, which indicates that there is a dividend gap in cities within 100 km from the central city, which means that the opening of high-speed rail has a significant siphon phenomenon for these cities, so that the consumption effect of these cities is not significant. Within 100–500 km from the central city, the regression coefficient is positive and all pass the significance test, but the regression coefficient is not significant in the spatial radius range above 500 km. The spillover effect results of high-speed rail opening variables and high-speed rail network variables are consistent, which shows that the spillover range of the impact of high-speed rail on urban consumption is limited, with an effective radius of 500 km, and the H2 hypothesis is verified.
Figure 6 shows the variation curves of coefficient values of different radius estimation results. It can be clearly seen from the figure that 100–500 km away from the central city is the effective range of high-speed rail, and the regression coefficient shows a trend of first rising and then falling, indicating that the effectiveness of high-speed rail shows the characteristics of an “inverted U,” and 300 km away from the central city is the effect optimal radius. The results are consistent with the current situation, and similar results with the same characteristics were obtained in the study by Huang and Wang (2020).
Since 2008, when China’s first high-speed railway was opened to traffic, a boom in high-speed railway construction has subsequently emerged across the country, driving China’s high-speed railway network to continue to expand. We collected the data from “cities with high-speed rail,” constructed three different spatial weight matrices, and combined them with the spatial Dubin model to explore and test the spatial spillover effect of high-speed rail on urban consumption. The following findings were obtained in this study: first, there is a positive spatial dependence on China’s urban consumption level, and the spatial correlation shows an upward trend year by year. Second, the high-speed rail network has a positive effect on local urban consumption, and at the same time, it affects urban consumption in adjacent areas through the spatial spillover effect. In the robustness test, the counterfactual test was performed by replacing the measurement of HSR, and consistent results were obtained. Third, the spillover range of the impact of high-speed rail on urban consumption is limited. The effective radius of the adjacent area of high-speed rail is 100–500 km away from the central city, and the optimal effect occurs at 300 km. Cities within 100 km away from the central city will have no significant consumption effect due to the siphon phenomenon. If the distance from the central city exceeds 500 km, the driving effect of high-speed rail on urban consumption will be weakened due to the long distance. This also shows that being too close or too far is not conducive to the role of high-speed rails.
According to the research results of this article, the following insights are obtained: the government should give full play to the consumption effect of high-speed railway networks on local and adjacent cities, enhance the consumption level of the city itself and neighboring cities, and gradually form a balanced development trend of regional consumption. The construction of urban high-speed railroad networks provides convenient channels for inter-regional flows of people, information, and capital, promotes the exchange and reintegration of factors, drives the overflow of technology and products to neighboring cities, and thus promotes the improvement of consumption levels in neighboring cities. Therefore, local governments should continue to promote the construction of high-speed rail stations not only in cities that have not opened high-speed rail but also in cities that have opened high-speed rail and should continue to increase the number of open lines and strengthen the density of the existing high-speed rail network. At the same time, they should break the barriers to the flow of production factors between regions within urban clusters, optimize the institutional mechanism for the movement of people, and design sufficiently attractive talent introduction programs to promote the inflow of people, so as to more effectively exploit the consumption spillover effect of high-speed rail networks and thus promote the increase in consumption levels in urban cluster cities.
In recent decades, China’s high-speed rail has been at the forefront of the world. The impact on China’s economic development status has been increasing. With the increase in the number of high-speed rail lines, new consumer growth points have emerged. However, the impact of high-speed rail on urban consumption is a complex system project. Although this study adopts the degree centrality, which can reflect the importance of the high-speed rail network in which the node cities are located, as an indicator of high-speed rail, the frequency and passenger volume data of high-speed lines in China are difficult to obtain and apply due to the limitation of data availability, which may lead to the lack of more detailed impact analysis and empirical tests.
Considering the vast territory of China, the obvious differences and uniqueness of city locations and their own economies, and the differences in the frequency of opening high-speed railroad lines in different cities, future research can select one or more high-speed railroad lines as research objects and further analyze the impact mechanism of specific high-speed railroad lines on the consumption of cities along the routes and surrounding cities, thus providing practical case support for the economic development of more cities and the promotion of high-speed railway construction. Future studies should also focus on the micro-level and discuss how the opening of high-speed railroads affects the segmented consumption structures of residents, thus providing a new research perspective to promote the upgrading of consumption structures and the increase of consumption rates.
The raw data supporting the conclusions of this article will be made available by the authors, without undue reservation.
WC is the first author, contributed to most of the work in this manuscript. ZW is the second author, contributed to guidance of the manuscript. YL is the corresponding author, contributed to the interpretation part.
The authors declare that the research was conducted in the absence of any commercial or financial relationships that could be construed as a potential conflict of interest.
All claims expressed in this article are solely those of the authors and do not necessarily represent those of their affiliated organizations, or those of the publisher, the editors, and the reviewers. Any product that may be evaluated in this article, or claim that may be made by its manufacturer, is not guaranteed or endorsed by the publisher.
Ahlfeldt, G. M., and Feddersen, A. (2018). From Periphery to Core: Measuring Agglomeration Effects Using High-Speed Rail. J. Econ. Geogr. 18 (2), 355–390. doi:10.1093/jeg/lbx005
Akerlof, G. A. (2007). The Missing Motivation in Macroeconomics. Am. Econ. Rev. 97 (1), 5–36. doi:10.1257/aer.97.1.5
Anselin, L. (1988). Spatial Econometrics: Methods and Models. Dordrecht: Springer Science and Business Media.
Arafat, N. A., and Bressan, S. (2017). “Hypergraph Drawing by Force-Directed Placement,” in Database and Expert Systems Applications. DEXA 2017. Lecture Notes in Computer Science. Editors D. Benslimane, E. Damiani, W. Grosky, A. Hameurlain, A. Sheth, and R. Wagner (Cham: Springer), 387–394. doi:10.1007/978-3-319-64471-4_31
Atack, J., Bateman, F., Haines, M., and Margo, R. A. (2010). Did Railroads Induce or Follow Economic Growth? Soc. Sci. Hist. 34 (2), 171–197. doi:10.1215/01455532-2009-02410.1017/s0145553200011202
Babalık-Sutcliffe, E. (2016). Urban Rail Operators in Turkey: Organisational Reform in Transit Service Provision and the Impact on Planning, Operation and System Performance. J. Transp. Geogr. 54, 464–475. doi:10.1016/j.jtrangeo.2015.08.008
Banerjee, A., Duflo, E., and Qian, N. (2020). On the Road: Access to Transportation Infrastructure and Economic Growth in China. J. Dev. Econ. 145, 102442. doi:10.1016/j.jdeveco.2020.102442
Campante, F., and Yanagizawa-Drott, D. (2018). Long-Range Growth: Economic Development in the Global Network of Air Links. Q. J. Econ. 133 (3), 1395–1458. doi:10.1093/qje/qjx050
Cao, J., Liu, X. C., Wang, Y., and Li, Q. (2013). Accessibility Impacts of China's High-Speed Rail Network. J. Transp. Geogr. 28, 12–21. doi:10.1016/j.jtrangeo.2012.10.008
Chen, C.-L., and Hall, P. (2012). The Wider Spatial-Economic Impacts of High-Speed Trains: a Comparative Case Study of Manchester and Lille Sub-regions. J. Transp. Geogr. 24, 89–110. doi:10.1016/j.jtrangeo.2011.09.002
Chen, C.-L. (2012). Reshaping Chinese Space-Economy through High-Speed Trains: Opportunities and Challenges. J. Transp. Geogr. 22, 312–316. doi:10.1016/j.jtrangeo.2012.01.028
Chen, H., Sun, D., Zhu, Z., and Zeng, J. (2016). The Impact of High-Speed Rail on Residents’ Travel Behavior and Household Mobility: A Case Study of the Beijing-Shanghai Line, China. Sustainability 8 (11), 1187. doi:10.3390/su8111187
Cheng, Y.-s., Loo, B. P. Y., and Vickerman, R. (2015). High-speed Rail Networks, Economic Integration and Regional Specialisation in China and Europe. Travel Behav. Soc. 2 (1), 1–14. doi:10.1016/j.tbs.2014.07.002
Ciccarelli, C., Magazzino, C., and Marcucci, E. (2021). Early Development of Italian Railways and Industrial Growth: A Regional Analysis. Res. Transp. Econ. 88, 100916. doi:10.1016/j.retrec.2020.100916
Delaplace, M., Pagliara, F., Perrin, J., and Mermet, S. (2014). Can High Speed Rail Foster the Choice of Destination for Tourism Purpose? Procedia - Soc. Behav. Sci. 111, 166–175. doi:10.1016/j.sbspro.2014.01.049
Diao, M. (2018). Does Growth Follow the Rail? the Potential Impact of High-Speed Rail on the Economic Geography of China. Transp. Res. Part A Policy Pract. 113, 279–290. doi:10.1016/j.tra.2018.04.024
Faber, B. (2014). Trade Integration, Market Size, and Industrialization: Evidence from China's National Trunk Highway System. Rev. Econ. Stud. 81 (3), 1046–1070. doi:10.1093/restud/rdu010
Garmendia, M., Ribalaygua, C., and Ureña, J. M. (2012). High Speed Rail: Implication for Cities. Cities 29, S26–S31. doi:10.1016/j.cities.2012.06.005
Guirao, B., Lara-Galera, A., and Campa, J. L. (2017). High Speed Rail Commuting Impacts on Labour Migration: The Case of the Concentration of Metropolis in the Madrid Functional Area. Land Use Policy 66, 131–140. doi:10.1016/j.landusepol.2017.04.035
Hiramatsu, T. (2018). Job and Population Location Choices and Economic Scale as Effects of High Speed Rail: Simulation Analysis of Shinkansen in Kyushu, Japan. Res. Transp. Econ. 72, 15–26. doi:10.1016/j.retrec.2018.06.007
Holl, A. (2004). Transport Infrastructure, Agglomeration Economies, and Firm Birth: Empirical Evidence from Portugal*. J. Reg. Sci. 44 (4), 693–712. doi:10.1111/j.0022-4146.2004.00354.x
Hong, J., Chu, Z., and Wang, Q. (2011). Transport Infrastructure and Regional Economic Growth: Evidence from China. Transportation 38 (5), 737–752. doi:10.1007/s11116-011-9349-6
Huang, Y., and Wang, Y. (2020). How Does High-Speed Railway Affect Green Innovation Efficiency? A Perspective of Innovation Factor Mobility. J. Clean. Prod. 265, 121623. doi:10.1016/j.jclepro.2020.121623
Hulten, C. R., Bennathan, E., and Srinivasan, S. (2006). Infrastructure, Externalities, and Economic Development: A Study of the Indian Manufacturing Industry. World Bank. Econ. Rev. 20 (2), 291–308. doi:10.1093/wber/lhj007
Jia, S., Zhou, C., and Qin, C. (2017). No Difference in Effect of High-Speed Rail on Regional Economic Growth Based on Match Effect Perspective? Transp. Res. Part A Policy Pract. 106, 144–157. doi:10.1016/j.tra.2017.08.011
Krugman, P. (1991). Increasing Returns and Economic Geography. J. Political Econ. 99 (3), 483–499. doi:10.1086/261763
LeSage, J. P. (2014). Spatial Econometric Panel Data Model Specification: A Bayesian Approach. Spat. Stat. 9, 122–145. doi:10.1016/j.spasta.2014.02.002
Levinson, D. M. (2012). Accessibility Impacts of High-Speed Rail. J. Transp. Geogr. 22, 288–291. doi:10.1016/j.jtrangeo.2012.01.029
Li, X., Huang, B., Li, R., and Zhang, Y. (2016). Exploring the Impact of High Speed Railways on the Spatial Redistribution of Economic Activities - Yangtze River Delta Urban Agglomeration as a Case Study. J. Transp. Geogr. 57, 194–206. doi:10.1016/j.jtrangeo.2016.10.011
Liang, Y., Zhou, K., Li, X., Zhou, Z., Sun, W., and Zeng, J. (2020). Effectiveness of High-Speed Railway on Regional Economic Growth for Less Developed Areas. J. Transp. Geogr. 82, 102621. doi:10.1016/j.jtrangeo.2019.102621
Liu, S., Wan, Y., Ha, H.-K., Yoshida, Y., and Zhang, A. (2019). Impact of High-Speed Rail Network Development on Airport Traffic and Traffic Distribution: Evidence from China and Japan. Transp. Res. Part A Policy Pract. 127, 115–135. doi:10.1016/j.tra.2019.07.015
Long, F., Zheng, L., and Song, Z. (2018). High-speed Rail and Urban Expansion: An Empirical Study Using a Time Series of Nighttime Light Satellite Data in China. J. Transp. Geogr. 72, 106–118. doi:10.1016/j.jtrangeo.2018.08.011
Magazzino, C., and Giolli, L. (2021). The Relationship Among Railway Networks, Energy Consumption, and Real Added Value in Italy. Evidence Form ARDL and Wavelet Analysis. Res. Transp. Econ. 90, 101126. doi:10.1016/j.retrec.2021.101126
Magazzino, C., and Mele, M. (2021). On the Relationship between Transportation Infrastructure and Economic Development in China. Res. Transp. Econ. 88, 100947. doi:10.1016/j.retrec.2020.100947
Monzón, A., Ortega, E., and López, E. (2013). Efficiency and Spatial Equity Impacts of High-Speed Rail Extensions in Urban Areas. Cities 30, 18–30. doi:10.1016/j.cities.2011.11.002
Ortega, E., López, E., and Monzón, A. (2012). Territorial Cohesion Impacts of High-Speed Rail at Different Planning Levels. J. Transp. Geogr. 24, 130–141. doi:10.1016/j.jtrangeo.2011.10.008
Qin, Y. (2017). 'No County Left behind?' the Distributional Impact of High-Speed Rail Upgrades in China. J. Econ. Geogr. 17 (3), lbw013–520. doi:10.1093/jeg/lbw013
Shao, S., Tian, Z., and Yang, L. (2017). High Speed Rail and Urban Service Industry Agglomeration: Evidence from China's Yangtze River Delta Region. J. Transp. Geogr. 64, 174–183. doi:10.1016/j.jtrangeo.2017.08.019
Shirley, C., and Winston, C. (2004). Firm Inventory Behavior and the Returns from Highway Infrastructure Investments. J. Urban Econ. 55 (2), 398–415. doi:10.1016/j.jue.2003.11.001
Straub, S. (2011). Infrastructure and Development: A Critical Appraisal of the Macro-Level Literature. J. Dev. Stud. 47 (5), 683–708. doi:10.1080/00220388.2010.509785
Ureña, J. M., Menerault, P., and Garmendia, M. (2009). The High-Speed Rail Challenge for Big Intermediate Cities: A National, Regional and Local Perspective. Cities 26 (5), 266–279. doi:10.1016/j.cities.2009.07.001
Verma, A., Sudhira, H. S., Rathi, S., King, R., and Dash, N. (2013). Sustainable Urbanization Using High Speed Rail (HSR) in Karnataka, India. Res. Transp. Econ. 38 (1), 67–77. doi:10.1016/j.retrec.2012.05.013
Viceira, L. M. (2001). Optimal Portfolio Choice for Long-Horizon Investors with Nontradable Labor Income. J. Finance 56 (2), 433–470. doi:10.1111/0022-1082.00333
Vickerman, R. (2018). Can High-Speed Rail Have a Transformative Effect on the Economy? Transp. Policy 62, 31–37. doi:10.1016/j.tranpol.2017.03.008
Wang, C., Meng, W., and Hou, X. (2020). The Impact of High-Speed Rails on Urban Economy: An Investigation Using Night Lighting Data of Chinese Cities. Res. Transp. Econ. 80, 100819. doi:10.1016/j.retrec.2020.100819
Wang, D.-g., Niu, Y., and Qian, J. (2018). Evolution and Optimization of China's Urban Tourism Spatial Structure: A High Speed Rail Perspective. Tour. Manag. 64, 218–232. doi:10.1016/j.tourman.2017.08.010
Wang, F., Wei, X., Liu, J., He, L., and Gao, M. (2019). Impact of High-Speed Rail on Population Mobility and Urbanisation: A Case Study on Yangtze River Delta Urban Agglomeration, China. Transp. Res. Part A Policy Pract. 127, 99–114. doi:10.1016/j.tra.2019.06.018
Yu, N., de Jong, M., Storm, S., and Mi, J. (2013). Spatial Spillover Effects of Transport Infrastructure: Evidence from Chinese Regions. J. Transp. Geogr. 28, 56–66. doi:10.1016/j.jtrangeo.2012.10.009
Zhang, A., Wan, Y., and Yang, H. (2019). Impacts of High-Speed Rail on Airlines, Airports and Regional Economies: A Survey of Recent Research. Transp. Policy 81, A1–A19. doi:10.1016/j.tranpol.2019.06.010
Zhang, Y., Ma, W., Yang, H., and Wang, Q. (2021). Impact of High-Speed Rail on Urban Residents' Consumption in China-from a Spatial Perspective. Transp. Policy 106, 1–10. doi:10.1016/j.tranpol.2021.03.017
Zheng, L., Long, F., Chang, Z., and Ye, J. (2019). Ghost Town or City of Hope? the Spatial Spillover Effects of High-Speed Railway Stations in China. Transp. Policy 81, 230–241. doi:10.1016/j.tranpol.2019.07.005
Keywords: high-speed rail, urban consumption, spatial metrology, effective radius, impact analysis
Citation: Cai W, Wu Z and Lu Y (2022) The Impact of High-Speed Rails on Urban Consumption—From the Perspective of “Local-Adjacent” Effect. Front. Environ. Sci. 10:884965. doi: 10.3389/fenvs.2022.884965
Received: 27 February 2022; Accepted: 10 May 2022;
Published: 16 June 2022.
Edited by:
Cosimo Magazzino, Roma Tre University, ItalyReviewed by:
Jun Yang, Northeastern University, ChinaCopyright © 2022 Cai, Wu and Lu. This is an open-access article distributed under the terms of the Creative Commons Attribution License (CC BY). The use, distribution or reproduction in other forums is permitted, provided the original author(s) and the copyright owner(s) are credited and that the original publication in this journal is cited, in accordance with accepted academic practice. No use, distribution or reproduction is permitted which does not comply with these terms.
*Correspondence: Yurong Lu, bHV5dXJvbmcxOTkxQDE2My5jb20=
Disclaimer: All claims expressed in this article are solely those of the authors and do not necessarily represent those of their affiliated organizations, or those of the publisher, the editors and the reviewers. Any product that may be evaluated in this article or claim that may be made by its manufacturer is not guaranteed or endorsed by the publisher.
Research integrity at Frontiers
Learn more about the work of our research integrity team to safeguard the quality of each article we publish.