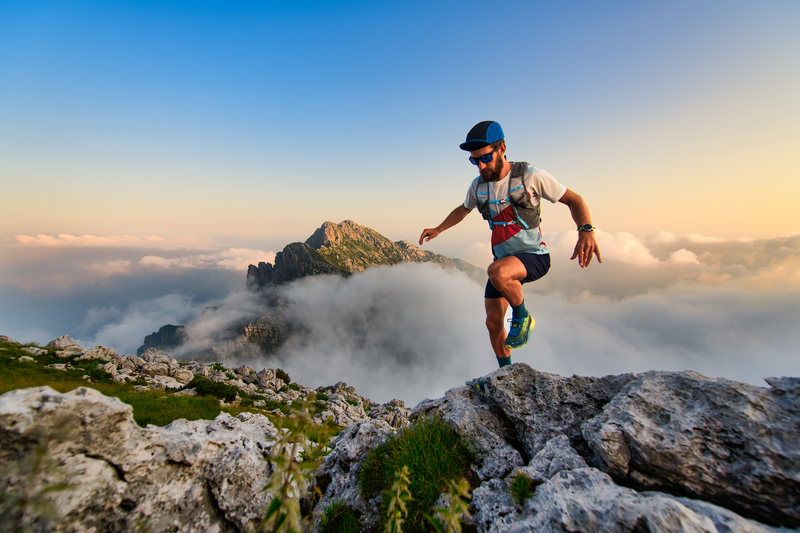
95% of researchers rate our articles as excellent or good
Learn more about the work of our research integrity team to safeguard the quality of each article we publish.
Find out more
ORIGINAL RESEARCH article
Front. Environ. Sci. , 28 April 2022
Sec. Environmental Economics and Management
Volume 10 - 2022 | https://doi.org/10.3389/fenvs.2022.878060
Using China’s provincial panel data from 2006 to 2016, this paper develops a dynamic panel data model to investigate the impact and mechanism of green credit on carbon emissions at the national and regional levels. According to the findings, green credit significantly reduces carbon emissions, with the eastern region having the greatest reduction effect. Green credit, with the exception of the western region, has a strong positive impact on disruptive low-carbon innovation. When green credit is combined with disruptive low-carbon innovation, both can significantly reduce carbon emissions; however, the green credit impact diminishes. It can be concluded that disruptive low-carbon innovation has a mediation effect on green credit’s contribution to carbon emission reduction. As a result, China should broaden the scope of green credit, concentrate on providing high-quality low-carbon patented technology to science and technology enterprises, and alleviate their financing constraints. Furthermore, these enterprises should increase their output of disruptive low-carbon innovation while lowering carbon emissions through technological innovation.
China’s rapid industrialization has resulted in massive environmental pollution, which has had a negative impact both on human health and environmental quality (Xu and Lin, 2017; Hollingsworth et al., 2021; Wang et al., 2021) Climate change, particularly carbon dioxide emissions, has emerged as a critical issue inextricably linked to economic growth and social development (Kang et al., 2020; Sherafati et al., 2020). The main source of environmental pollution is industrial pollution, and one important feature of industrial production is that it consumes natural resources and causes environmental damage. Infrastructure construction and heavy chemical industry consume massive amounts of mineral resources and fossil fuels as part of China’s industrialization process. As a result, China’s environmental pollution is directly related to a resource-intensive industrial structure, as well as the investment-driven growth model (Kemp, 1980). Many factors influence carbon emissions, including technological level, energy structure, economic structure, population structure, economic globalization, economic growth, energy structure and technological innovation, and industrial structure (Zhang et al., 2016; Khan et al., 2019). Shen and Gong (2011) believe that technological innovation is one of the most important factors influencing energy consumption, carbon emissions, and environmental pollution.
To combat climate change, nearly 200 countries, including China, signed the Paris Climate Agreement, which came into effect in November 2016. According to the agreement, China’s CO2 emissions per unit of GDP will be reduced by 60–65% by 2030 compared to 2005 levels. To meet the expected CO2 emissions target and achieve economic growth through the conversion from old to the new growth drivers, President Xi Jinping, the general secretary of the Communist Party of China (CPC), clearly stated his commitment to establishing and perfecting the green, low-carbon, and circular economic system, and stressed the need to construct a market-oriented system of innovation for green technology and to develop green finance, as well as energy conservation and environmental protection industries, clean production industries, and clean energy industries in a report delivered at the 19th National Congress of the Communist Party of China (Xu, 2021).
In the modern economic development process, credit funds are an important driving force for economic development. Green credit was born after the publication of the Equator Principles in 2002. Green credit is a component of the green financial system that stems from green finance, whereas green finance stems from the emergence and development of the concept of sustainable development. Green credit refers to the practice of banks taking into account not only economic benefit indicators, but also environmental factors in the credit issuance process, and then making appropriate loan decisions (Thompson and Cowton, 2004; Xiao et al., 2022).
Green credit policies have a direct impact on the financing capacity of heavily polluted enterprises, thereby influencing credit resource allocation (Liu et al., 2019). The outflow of traditional credit capital from financial institutions has facilitated the growth of capital-intensive, energy-intensive, and high-value industries, thus aggravating energy consumption and environmental pollution (Wen and Liu, 2019), and has resulted in a significant positive correlation between credit resource allocation and pollution emissions (Minghua, 2015). Green finance can, to some extent, encourage financial institutions to take more responsibility for environmental protection, thereby contributing to regional energy conservation and emission reduction (Dong et al., 2020).
Green credit is an important tool of green finance, with the primary function of reducing carbon emissions by optimizing resource allocation and directing capital to industries with low energy consumption and low pollution. According to the statistics from the China Banking and Insurance Regulatory Commission, the green credit balance of 21 leading Chinese banks has been growing continuously, rising from 4.85 trillion yuan at the end of June 2013 to 8.3 trillion yuan at the end of June 2017.
At the same time, CO2 emission reduction has increased from 71,900 tons in 2013 to 490 million tons in 2017. Green credit at the national level, in general, aids in the reduction of carbon emissions. Thus, it is worthwhile exploring whether green credit promotes the reduction of carbon emissions at the provincial and regional levels, as well as its operating mechanism. The literature on the effect of financial factors on carbon emission reduction is primarily concerned with financial development, with little attention paid to green credit. This paper uses a dynamic panel model to examine green credit in the financial sector at the provincial and regional levels. Subsequently, green credit is investigated as a means of promoting the operating mechanism of carbon emission reduction.
The driving factors that contribute to carbon emissions have long been the subject of research. According to the literature, financial development and technological innovation, as well as economic growth, urbanization, and other factors, are important factors influencing carbon emissions.
Financial development has a multifaceted influence on carbon emissions. On one hand, financial development has attracted foreign direct investment (FDI) to advance the technology, disperse risks, and lower financing costs, thereby encouraging businesses to reduce carbon emissions through technological innovation and increased environmental awareness (Shahbaz et al., 2013). Previous research has found that financial development has had an inhibitory effect on carbon emissions using a variety of methods based on data from various countries (Jalil and Feridun, 2011; Köksal et al., 2021; Tamazian et al., 2009). Financial development has been found to moderate the effect of real output on carbon emissions (in the short term, negatively), whereas financial development has been found to moderate the effect of real output on carbon emissions in the long term in Turkey (Katircioğlu and Taşpinar, 2017; Memduh Eren et al., 2022). Financial development, on the other hand, increases carbon emissions by accelerating economic growth and energy consumption, resulting in an inverted U-shaped relationship (Charfeddine and Ben Khediri, 2016; Dogan and Seker, 2016; Fan et al., 2022; Salahuddin et al., 2015). Financial development has been studied from various perspectives, as has its impact on carbon emissions (Xing et al., 2017). The relationship between the credit scale and China’s CO2 intensity has been found to be an inverted U-shaped, whereas the relationship between the FDI scale and China’s CO2 intensity has been found to be U-shaped (Chengliang et al., 2016). Several factors, such as financing scale, competition in the financial industry, and the marketization of credit capital allocation, have a negative effect on China’s CO2 intensity.
Technological progress has the potential to improve resource utilization efficiency and promote the innovation of management systems and institutional mechanisms in the process of economic operation, as well as energy conservation and emission reduction (Cai et al., 2021; Wen et al., 2021). After investigating the relationship among technological progress, industrial structure, and carbon emissions using a spatial econometric model, it has been discovered that technological progress, as well as the interaction between technological progress and industrial structure, have an inhibitory effect on carbon emissions (Li et al., 2017). Other studies, however, have found that the effect of technological progress on economic growth is greater than the effect of technological progress on emission reduction, whereas the effect of innovation on CO2 emission reduction is limited, with significant regional and time differences (Albino et al., 2014; Pei-Zhen et al., 2014; Mensah et al., 2018). Technological progress reduces carbon emissions in the long run, but not in the short run (Li and Qu, 2012). In recent years, some researchers have used empirical methods to demonstrate the impact of low-carbon technology innovation on carbon emissions. When investigating the relationship between China’s energy technology and carbon emissions, researchers have discovered that carbon-free energy technology reduces CO2 emissions more effectively than fossil fuel technology (Wang et al., 2012). Carbon sequestration technology advancement is critical in achieving carbon emission reductions in the face of global climate change (Huaman and Jun 2014). While researching the inhibiting effect of low-carbon technology innovation on carbon emissions, it was discovered (Wang et al., 2018) that there is a threshold effect of carbon reduction effect. Using spatial panel data, it was discovered that disruptive low-carbon innovation has a significant inhibitory effect on China’s carbon emissions in both the short and long term (Wilson, 2018).
Although financial development boosts overall innovation, the effects of the stock and credit markets on innovation vary. The financial market has the potential to reduce a company’s external financing costs, optimize resource allocation, and promote research and development (R&D) and innovation (Hall and Lerner, 2010; Hwang et al., 2010). Based on data from 51 countries and EU members from 1993 to 2008, researchers discovered that financial development had a significant positive effect on innovation and technological progress (Bayar, 2015; Meierrieks, 2014). The effects on innovation are heterogeneous when financial development is measured by the stock market and bank credit. The stock market encourages innovation in technology-intensive industries, whereas the credit market discourages it (Hsu et al., 2014). Zhong and Wang (2017) used data from China to measure financial development along three dimensions (stock market scale, banking scale, and banking marketization), suggesting that the stock market had a significantly greater influence on high-quality invention patents than the banking industry, while banking marketization did not promote the company’s patent output (Zhong and Wang, 2017).
Financial development has a significant impact on carbon emissions (Amin et al., 2022). When financial development is excluded as a cause of carbon emissions, significant variable loss may result (Shahbaz et al., 2013). Currently, the scale and level of financial development are used to represent financial development, with only a few exceptions using bank credit. China has been implementing a green credit policy since 2007. Green credit, as opposed to general credit, is the primary source of funding for emerging strategic industries, such as energy conservation and environmental protection, new energy, and new energy vehicles, the majority of which are in the clean technology sector. Theoretically, green credit will help strategic emerging industries overcome financing constraints, improve innovation output, and thus promote carbon reduction. Based on the non-linear threshold panel model, Xiu et al. found that green credit regulation was beneficial for energy conservation and emission reduction (Xiu et al., 2015). Liu et al. (2017)created the financial CGE model to investigate the systematic impact of green credit policies, and their findings revealed that green credit policies were effective in discouraging investment in energy-intensive industries. Furthermore, some scholars have investigated the industrial upgrading effect of green credit, its impact on bank operating efficiency, and its relationship with commercial bank competitiveness (Sheng et al., 2018; Liao et al., 2019). Despite the fact that relevant research on green credit has been steadily increasing in recent years, few studies have been conducted on the impact of green credit on carbon emissions, resulting in a lack of in-depth discussion of the impact mechanism of green credit on carbon emissions. Based on the dynamic panel data model and the perspective of green credit, this paper attempts to answer two questions (whether green credit will promote carbon emission reduction and how to promote it) in order to supplement the theoretical findings of relevant studies and provide a scientific foundation for the development of green credit policies.
As illustrated in Figure 1, this paper investigates the relationship between green credit and carbon reduction in the context both of the internal and external environment.
According to the Cobb–Douglas (CD) production function, enterprise output is an increasing function of human capital and fund capital. According to the China Banking Regulatory Commission (CBRC), the key areas supported by green credit are low-carbon industries, including energy conservation, environmental protection, new energy, and new energy vehicles. Green credit can help alleviate enterprise financing constraints while encouraging enterprise development in order to provide more low-carbon products to society and reduce carbon emissions. According to CBRC information disclosure, from 2013 to 2017, the outstanding green credit business loans of 21 major Chinese banks increased from 36,853.49 billion yuan to 65,312.63 billion yuan, while energy conservation and emission reductions increased from 4,790.27 tons to 4,905.64 tons of CO2 equivalent. On the other hand, green credit policy has cut off a portion of the capital sources for the “two high” enterprises, i.e., those with high pollution and carbon emissions. Thus, the goal of enterprises expanding their production scale is stymied, and the reduction in output leads to a reduction in carbon emissions. Enterprises will reduce their energy consumption per unit of output by adjusting their future development strategy, upgrading technology, and innovating the original products. Therefore, the following research hypothesis is proposed:
H1: Green credit contributes to lower CO2 emissions.
Enterprises require a significant amount of money for R&D. Equity and debt financing are two types of enterprise financing. Equity financing entails raising funds in the capital market through the issuance of stocks and bonds, whereas debt financing entails borrowing money from banks. It is difficult for new small and medium-sized high-tech enterprises to raise capital in China’s capital market due to certain thresholds. Enterprises in the energy-saving and environmental protection industry, new energy industry, and new energy vehicle industry in accordance with national industrial policies, particularly those in strategic emerging industries, can obtain credit funds through green credit to carry out low-carbon innovation. In the literature, there are three main measures of technological innovation: input method; output method; and efficient method. The output method is mainly measured by patents. Based on the innovation content, patents can be classified as incremental innovation or radical innovation (Luna et al., 2019). The latter is also known as subversive innovation (Zhong and Wang, 2017). Incremental innovation is the use of current technology to make minor improvements to existing products, whereas disruptive innovation is a fundamental change in new products that represents a technological revolution (Dewar and Dutton, 1986). In the field of low-carbon technology, disruptive low-carbon innovation has a greater impact on carbon emissions than incremental low-carbon innovation. Solar photovoltaic and Tesla electric vehicles, for instance, are part of the new energy and energy vehicle industries, respectively. They are typical examples of disruptive low-carbon innovation, potentially shifting the entire industry’s technological trajectory. Since commercial banks are risk-averse, enterprises with disruptive low-carbon innovation will be preferred when banks select and screen green credit customers. As a result, the following research hypothesis is proposed:
H2: Green credit has a positive impact on disruptive low-carbon innovation.
Low-carbon technologies can reduce the intensity of energy use and greenhouse gas emissions (Fisher-Vanden and Sue Wing, 2008). By focusing on the R&D of key industries and technologies, China’s low-carbon transformation strategy has yielded preliminary results and laid a relatively solid technological foundation for meeting the target of carbon emission reduction. Disruptive low-carbon innovation has a significant inhibitory effect on local carbon emissions (Luna et al., 2019). Globally, low-carbon technological innovation improves energy efficiency while limiting the carbon intensity of energy structure. Therefore, the following research hypothesis is proposed:
H3: The impact of green credit on carbon emissions is transmitted through the mediation effect of disruptive low-carbon innovation.
Green credit alleviates enterprises’ investment constraints (Fengrong and Kangshi, 2018), has an incentive effect on the investment and financing activities of environmentally friendly enterprises, and promotes technological innovation in enterprises, which encourages enterprises to conserve energy and reduce emissions (Guo et al., 2015; Kang et al., 2020).
Given that carbon emissions have path dependence and inertia, current carbon emissions will be influenced by previous emissions, and there may be endogenies among carbon emissions, green credits, and disruptive low-carbon innovation; therefore, system generalized method of moments (GMM) is used for modeling. In three steps, the paper examines the impact of green credit on carbon emissions as well as the intermediary effect of disruptive low-carbon innovation. Based on the hierarchical regression analysis and judgment criteria, the paper examines the impact of green credit on carbon emissions as well as the mediation effect of disruptive low-carbon innovation in three steps (Baron and Kenny, 1986; Wen et al., 2005).
Model I is created to test H1. If the coefficient
Model II is constructed to test H2. If the coefficient
Model III is built to test H3. If
Carbon intensity is the ratio of carbon emissions to real GDP, representing the carbon emissions associated with each unit of economic output. When compared to total or average carbon emissions, the index of carbon intensity is more comparable to samples from different economic scales. Carbon intensity is treated with a logarithm as the response variable in this paper, and ln(C/GDP) represents the logarithm of this variable. China’s provincial carbon emissions are calculated using the carbon emission methodology proposed by the Intergovernmental Panel on Climate Change (IPCC). The formula is as follows:
where C is carbon emission; En is the consumption of nth energy;
The majority of existing green credit statistics come from commercial banks, with data derived from the banks’ social responsibility reports. There is no unified measurement method for the level of green credit across provinces. The CBRC disclosed green credit data from 21 major banks in 2018, but there is a lack of provincial green credit data. In the Green Finance Research Report of China 2020, the indicator of green credit refers to the proportion of interest expenditure in high energy-consuming industries, indicating that the data are shifting toward green credit. According to the 2020 National Economic and Social Development issued by the National Bureau of Statistics, six major energy-intensive industries are classified: the chemical raw materials and chemical products manufacturing industry; the non-metallic mineral products industry; the black metal smelting and rolling processing industry; the non-ferrous metal smelting and rolling processing industry; the oil processing and coking and nuclear fuel processing industry; and the production and supply of electric power and heat power industry. To calculate the green credit in this paper, the logarithm of the difference between the total interest expenditure of industrial industries in each province and the interest expenditure of the six energy-intensive industries is used. ln(GCL) denotes calculating the logarithm of green credit. Because interest payments reflect the size of credit, these six industries account for the majority of CO2 emissions. In this paper, the logarithm of the difference between the total interest expenditure of industrial industries in each province and the interest expenditure of the six energy-intensive industries is selected to measure the green credit. Because interest payments reflect the size of credit, these six industries are the main sources of carbon dioxide emissions.
According to Luna et al. (2019) the number of patent citations is a key indicator for the quality of patients. The number of Y02 classified patent applications in the Cooperative Patent Classification (CPC), a cooperative patent classification system jointly developed by the US and the United Kingdom, was used to assess low-carbon technology innovation. The top 1% of patents cited in each technology field each year were then defined as disruptive innovation, and then the number of annual disruptive low-carbon innovations for each province was calculated by combining all fields.
The rate of marketization is represented by the variable MAR. ln(MAR) are logarithms of this variable. The scale of resource-based industries and low-value-added industries is constrained in regions with a high level of marketization. For example, China’s first-tier cities have a high degree of marketization, with a significantly higher proportion of high-end manufacturing and service industries than other cities. The marketization rate index is calculated using the method of measuring marketization level proposed by (Fan et al., 2010).
Industrial structure (STR) is a ratio of the secondary industry output to GDP. The primary source of carbon emissions in China’s secondary industry is the energy consumption of coal and fossil fuels, and the acceleration of industrialization leads to much higher energy consumption in the industrial sector than in other industries.
R&D input (RD) is represented by log(RD). Capital investment is one of the important components of innovation activities, and R&D investment will promote low-carbon innovation to a certain extent.
The log of the number of people in each province is used to reflect population (PEO). Human activity emits a significant amount of carbon dioxide, and the more populous the province, the more carbon it emits.
In this paper, the research interval is from 2006 to 2016, and the research objects are 23 provinces (Gansu, Qinghai, Ningxia, Guizhou, Xizang, and Shanxi were omitted due to a lack of data on disruptive innovation). The Y02 patent data for each province were obtained from the Incopat patent database, while the data on energy consumption, discount coal coefficient, and carbon emission coefficient were obtained from the China Energy Statistical Yearbook. The China Statistical Yearbook provides data on R&D investment, industrial structure, and population. Economic variables are treated at constant prices in the base period of 2006 to eliminate the impact of price fluctuations.
The descriptive results of each variable are shown in Table 1, indicating that the standard deviation of carbon emission intensity (logarithm) is 0.913, with a maximum of 11.885 and a minimum of 4.959. Green credit has a larger provincial difference than carbon emission, with a standard deviation of 1.108, a maximum value of 6.801, and a minimum value of 1.803. The provincial difference in disruptive innovation is greater than the provincial difference in carbon emission and green credit, with a standard of 1.280, a maximum value of 4.736, and a minimum value of 0.
The system GMM method is used to estimate Eq. 4 using provincial panel data, and the results are shown in the first column of Table 2. Sargan statistics and Hansen statistics differ in their ability to test the over-identifying restrictions of instrumental variables. The former, while not robust, is unaffected by a large number of instrumental variables, whereas the latter is robust but may fail due to a large number of instrumental variables. In this paper, Sargan statistics are used to test the over-identifying restrictions of instrumental variables. At the provincial level, the p-value of the Sargan test in model I was 0.094, which was greater than 0.05, indicating that the null hypothesis that “all instrumental variables are valid” could not be rejected at the 5% significance level, i.e., the selected instrumental variables were all valid instrumental variables. The disturbance terms were subjected to a sequence correlation test, and the corresponding p-value revealed that the model’s disturbance terms lacked sequence correlation. Therefore, modeling with the system GMM makes sense.
According to the provincial model I in Table 2, the first-order lag term of carbon intensity has a significantly positive impact on current carbon intensity with a 10% significant level, implying that carbon emission is viscous. Under the same conditions, the higher the previous period’s carbon intensity, the higher the current period. Green credit has a significant negative impact on carbon emission intensity at a significant level of 1%. Every 1% increase in green credit reduces the intensity of carbon emissions by 0.441%, indicating that green credit has the potential to reduce carbon emissions. Furthermore, the proportion of secondary industry and the population of the province have a significant positive impact on carbon emissions.
Furthermore, the country is divided into three regions based on its level of economic development: the eastern region; the central region; and the western region. Based on the regional panel data, the system GMM is used for modeling. The results are shown in columns 3–5 in Table 2. According to the p-values of the Sargan test and the autocorrelation test of the interference item, it is reasonable to use system GMM for modeling at the significance level of 5%. The impact of green credit on carbon emissions varies; the eastern region has the greatest impact, while the central and western districts have nearly identical effects. A 1% increase in green credit [ln(GCL)] reduces carbon intensity by 0.532%, 0.170%, and 0.169% in the eastern, central, and western regions, respectively. On the one hand, carbon emissions are relatively high in the central and western regions. The average intensity of carbon emissions in the eastern, central, and western regions is 7.043, 7.721, and 7.498, respectively. Carbon emission intensity in the central and western regions, on the other hand, has a high viscosity. The coefficient of the variable ln(C/GDP) (–1) in model I in the eastern region is not significant at the 1% significance level, but the coefficient of ln(C/GDP) in the central and western regions is significantly positive, weakening the carbon emission reduction effect of green credit.
The system GMM model was used to model the panel data at the provincial and regional levels to further investigate the impact of green credit on disruptive low-carbon innovation. Table 3 displays the results of the model II parameter estimation. It is reasonable to use system GMM modeling based on the p-values of the Sargan test and AR(2) in Table 3. Green credit significantly promotes disruptive low-carbon innovation at a significant level of 5% at the provincial level, as well as in eastern and western provinces, but the intensity of action varies. The disruptive low-carbon innovation that lags one period has no significant impact on the current period at the provincial level. The disruptive low-carbon innovation will increase by 0.491% for every 1% increase in green credit. The impact coefficient of disruptive low-carbon innovation lagging one period is 0.278 in the eastern region, reflecting the stickiness of disruptive low-carbon innovation. The disruptive low-carbon innovation will increase by 0.348% for every 1% increase in green credit. The impact of disruptive low-carbon innovation lagging one period on the current period is negative but not significant in the central region. Disruptive low-carbon innovation increases by 1.027% for every 1% increase in green credit, and the positive effect of green credit on disruptive innovation is not significant in the western region. One possible explanation is that the western region is lacking in disruptive low-carbon innovation, as evidenced by the low number of patents. Some provinces have had no disruptive innovation in some years, with an average of 4.026, which is lower than the eastern region (M = 4.900) and the central region (M = 4.422).
The system GMM model is used to model the panel data at the provincial and regional levels, taking into account the impact of green credit and disruptive innovation on carbon emissions. Table 4 displays the parameter estimation results for model III. The system GMM method is appropriate for estimation based on the p-values of the Sargan test and AR(2) in Table 4. Based on provincial and regional panel data, both green credit and disruptive low-carbon innovation can significantly reduce carbon emissions at a significant level of 5%. Furthermore, when compared to the green credit coefficient [ln(GCL)] in Table 2, the green credit coefficient in Table 4 decreases to varying degrees. At a significance level of 10%, the coefficient of the variable ln(DIS) is significant, indicating that disruptive low-carbon innovation can significantly reduce carbon emissions at the provincial level, as well as in the eastern, central, and western regions. The coefficients of ln(GCL) in models I and II are significant for provincial, eastern, and central regions, and the coefficients of ln(GCL) and ln(DIS) in model III are also significant. The mediation effect of variable ln(DIS) is significant, as per the mediation effect judgment criteria of (Baron and Kenny, 1986; Sobel, 1987). Since the coefficient of ln(GCL) in model II is not significant for the western region, the Sobel test is required, and the test results show that ln(DIS) has a significant mediation effect (Hsu et al., 2014). It is clear that ln(DIS) is a mediating variable, and disruptive innovation mediates the impact of green credit on carbon emission reduction, implying that green credit can promote disruptive innovation and thus reduce carbon emissions.
TABLE 4. Results of system GMM on the impact of green credit and disruptive innovation on carbon emission.
Because of its insignificance in Tables 2, 4, ln(MAR) was eliminated, and industrial structure (STR) was replaced by urbanization rate (UR). On the basis of provincial panel data, the control variables were replaced and robustness tests were run on models I, II, and III. According to the findings shown in Table 5, green credit has a direct inhibitory effect on carbon emissions and significantly promotes disruptive low-carbon innovation. Both green credit and disruptive low-carbon innovation significantly reduce carbon emissions. Thus, disruptive low-carbon innovation mediates the impact of green credit on reducing carbon emissions. Models I, II, and III show a high level of robustness.
Based on panel data from 23 provinces, municipalities, and autonomous regions from 2006 to 2016, and using a dynamic panel data model to investigate the effect of China’s green credit on carbon emissions and its mechanism, the following conclusions were reached:
1. Green credit significantly reduces the intensity of carbon emissions at the provincial level, as well as in eastern, central, or western regions, with the eastern region having the greatest carbon reduction effect.
2. The facilitation effect of green credit on disruptive low-carbon innovation varies by region. Green credit has a significant positive effect on disruptive low-carbon innovation at the provincial, eastern, and central regional levels, but not at the regional level.
3. Taking green credit and disruptive low-carbon innovation into account, both significantly reduce the intensity of carbon emissions at the provincial level, as well as eastern, central, and western regions.
4. When only green credit is considered, the coefficient of green credit decreases. As a result, disruptive low-carbon innovation acts as a mediator, i.e., green credit reduces carbon emissions by influencing disruptive low-carbon innovation.
The findings of this study are critical for the relevant government departments, financial institutions such as the banking industry, and managers of heavily polluting enterprises.
First, the government should broaden its green credit coverage, promote green credit business to more commercial banks, establish “green branches,” and accelerate the development of green credit business.
Second, to promote the development of green finance, the government should encourage more private capital to invest in green projects, make green risk investments, and build a multi-level green financial system.
First, banks should expand the credit business and continue to support three emerging major strategic industries (energy conservation and environmental protection, new energy, and new energy vehicles), as well as energy conservation and environmental protection programs and services. Banks should look into developing a green credit retail channel, such as a green credit card or a green loan, to promote low-carbon products and services, as well as the green consumption concept.
Second, to alleviate financing constraints of innovative enterprises, banks should implement differentiated green credit policies, prioritize lending to innovative enterprises with high-quality low-carbon patents, and innovate mortgages by using high-quality low-carbon patents as collateral.
Third, banks should investigate issuing green municipal bonds and implementing environmental rights pledges, as well as establishing green finance evaluation criteria based on existing green credit, green bonds, and green funds.
First, the implementation of green credit not only exacerbates the credit constraints of heavily polluting enterprises, but also reduces access to commercial credit financing, thus inhibiting technological innovation. Heavy polluting enterprises should place a high value on the direct and indirect effects of green credit policies and vigorously promote green transformation and development in order to attract more external financial support and foster enterprise technological innovation.
Second, enterprises should improve their green management, raise awareness about environmental protection, enhance their environmental information disclosure system, and reduce information asymmetry between banks and enterprises. Furthermore, enterprises should strengthen their own financing capacity in order to avoid the long-term punishment mechanism of green credit, which might cause them to fall into financing difficulties, resulting in insufficient capital investment and deterioration of operating conditions.
This study used empirical evidence to demonstrate that green credit reduces carbon emissions by encouraging disruptive low-carbon innovation. The following aspects should be the focus of future research. First, the green credit studied in this paper is only a small part of the green financial system, and it is insufficient to thoroughly investigate the role of the green financial system in economic development. Second, while this paper develops the model for theoretical analysis, it falls short of covering a wide range of economic sectors, including banking, financial markets, finance, etc.
All claims expressed in this article are solely those of the authors and do not necessarily represent those of their affiliated organizations, or those of the publisher, the editors and the reviewers. Any product that may be evaluated in this article, or claim that may be made by its manufacturer, is not guaranteed or endorsed by the publisher.
The raw data supporting the conclusion of this article will be made available by the authors, without undue reservation.
All authors listed have made a substantial, direct, and intellectual contribution to the work and approved it for publication.
The authors declare that the research was conducted in the absence of any commercial or financial relationships that could be construed as a potential conflict of interest.
All claims expressed in this article are solely those of the authors and do not necessarily represent those of their affiliated organizations, or those of the publisher, the editors, and the reviewers. Any product that may be evaluated in this article, or claim that may be made by its manufacturer, is not guaranteed or endorsed by the publisher.
Albino, V., Ardito, L., Dangelico, R. M., and Messeni Petruzzelli, A. (2014). Understanding the Development Trends of Low-Carbon Energy Technologies: A Patent Analysis. Appl. Energy 135, 836–854. doi:10.1016/j.apenergy.2014.08.012
Amin, A., Ameer, W., Yousaf, H., and Akbar, M. (2022). Financial Development, Institutional Quality, and the Influence of Various Environmental Factors on Carbon Dioxide Emissions: Exploring the Nexus in China. Front. Environ. Sci. 9. doi:10.3389/fenvs.2021.838714
Baron, R. M., and Kenny, D. A. (1986). The Moderator-Mediator Variable Distinction in Social Psychological Research: Conceptual, Strategic, and Statistical Considerations. J. personality Soc. Psychol. 51 (6), 1173–1182. doi:10.1037/0022-3514.51.6.1173
Bayar, Y. (2015). Macroeconomic Determinants of Technological Progress in Major Eurozone Member Countries. Int. J. Econ. Pract. Theor. 5 (5), 420–427.
Cai, A., Zheng, S., Cai, L., Yang, H., and Comite, U. (2021). How Does Green Technology Innovation Affect Carbon Emissions? A Spatial Econometric Analysis of China’s Provincial Panel Data. Front. Environ. Sci. 9. doi:10.3389/fenvs.2021.813811
Charfeddine, L., and Ben Khediri, K. (2016). Financial Development and Environmental Quality in UAE: Cointegration with Structural Breaks. Renew. Sustain. Energy Rev. 55, 1322–1335. doi:10.1016/j.rser.2015.07.059
Chengliang, Y., Tao, L., and Wei, L. (2016). Financial Development Innovation and Carbon Dioxide Emissions. Financial Res. 427 (1), 14–30.
Dewar, R. D., and Dutton, J. E. (1986). The Adoption of Radical and Incremental Innovations: An Empirical Analysis. Manag. Sci. 32 (11), 1422–1433. doi:10.1287/mnsc.32.11.1422
Dogan, E., and Seker, F. (2016). The Influence of Real Output, Renewable and Non-renewable Energy, Trade and Financial Development on Carbon Emissions in the Top Renewable Energy Countries. Renew. Sustain. Energy Rev. 60, 1074–1085. doi:10.1016/j.rser.2016.02.006
Dong, Q., Wen, S., and Liu, X. (2020). Credit Allocation, Pollution, and Sustainable Growth: Theory and Evidence from China. Emerg. Mark. Finance Trade 56 (12), 2793–2811. doi:10.1080/1540496X.2018.1528869
Fan, G., Wang, X., and Zhu, H. (2010). China’s Marketization Index. Beijing: Economic Science Press.
Fan, M., Khalique, A., Qalati, S. A., Gillal, F. G., and Gillal, R. G. (2022). Antecedents of Sustainable E-Waste Disposal Behavior: the Moderating Role of Gender. Environ. Sci. Pollut. Res. 29 (14), 20878–20891. doi:10.1007/s11356-021-17275-y
Fengrong, W., and Kangshi, W. (2018). Green Policy and Allocative Efficiency of Green Finance: Evidence from Chinese Manufacturing Listed Enterprises. Finance Econ. 5, 1–14.
Fisher-Vanden, K., and Sue Wing, I. (2008). Accounting for Quality: Issues with Modeling the Impact of R&D on Economic Growth and Carbon Emissions in Developing Economies. Energy Econ. 30 (6), 2771–2784. doi:10.1016/j.eneco.2007.04.002
Guo, C.-x., Liu, Y.-h., and Yang, X.-y. (2015). Investment and Financing on China’s Environmental Protection Industry: Problems and Solutions. China Popul. Resour. Environ. 8, 92–99.
Hall, B. H., and Lerner, J. (2010). “The Financing of R&D and Innovation,” in Handbook of the Economics of Innovation. Editors B. H. Hall,, and N. Rosenberg (North-Holland), 1, 609–639. doi:10.1016/s0169-7218(10)01014-2
Hollingsworth, A. J., Konisky, D. M., and Zirogiannis, N. (2021). The Health Consequences of Excess Emissions: Evidence from Texas. J. Environ. Econ. Manag. 108, 102449. doi:10.1016/j.jeem.2021.102449
Hsu, P.-H., Tian, X., and Xu, Y. (2014). Financial Development and Innovation: Cross-Country Evidence. J. Financial Econ. 112 (1), 116–135. doi:10.1016/j.jfineco.2013.12.002
Huaman, R. T. E., and Jun, T. X. (2014). Energy Related CO2 Emissions and the Progress on CCS Projects: A Review. Renew. Sustain. Energy Rev. 31, 368–385. doi:10.1016/j.rser.2013.12.002
Hwang, Y.-S., Min, H.-G., and Han, S.-H. (2010). The Influence of Financial Development on R&D Activity: Cross-Country Evidence. Rev. Pac. Basin Finan. Mark. Pol. 13 (03), 381–401. doi:10.1142/S0219091510001998
Jalil, A., and Feridun, M. (2011). The Impact of Growth, Energy and Financial Development on the Environment in China: A Cointegration Analysis. Energy Econ. 33 (2), 284–291. doi:10.1016/j.eneco.2010.10.003
Kang, H., Jung, S.-Y., and Lee, H. (2020). The Impact of Green Credit Policy on Manufacturers' Efforts to Reduce Suppliers' Pollution. J. Clean. Prod. 248, 119271. doi:10.1016/j.jclepro.2019.119271
Katircioğlu, S. T., and Taşpinar, N. (2017). Testing the Moderating Role of Financial Development in an Environmental Kuznets Curve: Empirical Evidence from Turkey. Renew. Sustain. Energy Rev. 68, 572–586. doi:10.1016/j.rser.2016.09.127
Khan, M. K., Teng, J.-Z., Khan, M. I., and Khan, M. O. (2019). Impact of Globalization, Economic Factors and Energy Consumption on CO2 Emissions in Pakistan. Sci. Total Environ. 688, 424–436. doi:10.1016/j.scitotenv.2019.06.065
Köksal, C., Katircioglu, S., and Katircioglu, S. (2021). The Role of Financial Efficiency in Renewable Energy Demand: Evidence from OECD Countries. J. Environ. Manag. 285, 112122. doi:10.1016/j.jenvman.2021.112122
Li, K., and Qu, R. (2012). The Impact of Technological Progress on China’s Carbon Emissions—An Empirical Study Based on Vector Error Correction Model. China Soft Sci. 6, 51–58.
Li, W., Wang, W., Wang, Y., and Qin, Y. (2017). Industrial Structure, Technological Progress and CO2 Emissions in China: Analysis Based on the STIRPAT Framework. Nat. Hazards 88 (3), 1545–1564. doi:10.1007/s11069-017-2932-1
Liao, Y., Hu, W., and Yang, D. (2019). Dynamic Analysis of the Impact of Green Credit on Bank Operating Efficiency: Based on Panel VAR Model. Finance Econ. Rev. 2, 57–64.
Liu, J.-Y., Xia, Y., Fan, Y., Lin, S.-M., and Wu, J. (2017). Assessment of a Green Credit Policy Aimed at Energy-Intensive Industries in China Based on a Financial CGE Model. J. Clean. Prod. 163, 293–302. doi:10.1016/j.jclepro.2015.10.111
Liu, X., Wang, E., and Cai, D. (2019). Green Credit Policy, Property Rights and Debt Financing: Quasi-Natural Experimental Evidence from China. Finance Res. Lett. 29, 129–135. doi:10.1016/j.frl.2019.03.014
Luna, , , Wang, W., Wang, M., et al. (2019). Disruptive Low-Carbon Innovation and Carbon Emission: Direct Impact and Space Spillover. China Popul. Resour. Environ. 29, 95–103.
Meierrieks, D. (2014). Financial Development and Innovation: Is There Evidence of a Schumpeterian Finance-Innovation Nexus? Ann. Econ. Finance 15 (2), 61–81.
Memduh Eren, B., Katircioglu, S., and Gokmenoglu, K. K. (2022). The Moderating Role of Informal Economy on Financial Development Induced Ekc Hypothesis in turkey. Energy & Environ., 0958305X2110707. doi:10.1177/0958305X211070775
Mensah, C. N., Long, X., Boamah, K. B., Bediako, I. A., Dauda, L., and Salman, M. (2018). The Effect of Innovation on CO2 Emissions of OCED Countries from 1990 to 2014. Environ. Sci. Pollut. Res. 25 (29), 29678–29698. doi:10.1007/s11356-018-2968-0
Minghua, Z. (2015). Financial Frictions, Bank Lending Channel under Monetary Policy and Misallocation in Industry of Credit Resources. J. Financial Res. 5, 1–17.
Pei-Zhen, J., Ya-Bin, Z., and Xing, P. (2014). The Double-Edged Effect of Technological Progress in Carbon Dioxide Emissions Reduction: Empirical Evidence from 35 Sub-industrial Sectors in China. Stud. Sci. Sci. 32 (5), 706.
Salahuddin, M., Gow, J., and Ozturk, I. (2015). Is the Long-Run Relationship between Economic Growth, Electricity Consumption, Carbon Dioxide Emissions and Financial Development in Gulf Cooperation Council Countries Robust? Renew. Sustain. Energy Rev. 51, 317–326. doi:10.1016/j.rser.2015.06.005
Shahbaz, M., Solarin, S. A., Mahmood, H., and Arouri, M. (2013). Does Financial Development Reduce CO2 Emissions in Malaysian Economy? A Time Series Analysis. Econ. Model. 35, 145–152. doi:10.1016/j.econmod.2013.06.037
Shen, K., and Gong, J. (2011). Environmental Pollution, Technical Progress, and Productivity Growth of Energy-Intensive Industries in China—Empirical Study Based on ETFP. China Ind. Econ. 12, 25–34.
Sheng, X., Xinxin, Z., and Shuang, Y. (2018). Analysis on the Effect of Green Credit on the Upgrading of Industrial Structure. J. Shanghai Univ. Finance Econ. 20 (02), 59–72.
Sherafati, M., Bashiri, M., Tavakkoli-Moghaddam, R., and Pishvaee, M. S. (2020). Achieving Sustainable Development of Supply Chain by Incorporating Various Carbon Regulatory Mechanisms. Transp. Res. Part D Transp. Environ. 81, 102253. doi:10.1016/j.trd.2020.102253
Sobel, M. E. (1987). Direct and Indirect Effects in Linear Structural Equation Models. Sociol. Methods & Res. 16 (1), 155–176. doi:10.1177/0049124187016001006
Tamazian, A., Chousa, J. P., and Vadlamannati, K. C. (2009). Does Higher Economic and Financial Development Lead to Environmental Degradation: Evidence from BRIC Countries. Energy Policy 37 (1), 246–253. doi:10.1016/j.enpol.2008.08.025
Thompson, P., and Cowton, C. J. (2004). Bringing the Environment into Bank Lending: Implications for Environmental Reporting. Br. Account. Rev. 36 (2), 197–218. doi:10.1016/j.bar.2003.11.005
Wang, W., Lu, N., and Zhang, C. (2018). Low-carbon Technology Innovation Responding to Climate Change from the Perspective of Spatial Spillover Effects. Chin. J. Popul. Resour. Environ. 16 (2), 120–130. doi:10.1080/10042857.2018.1480689
Wang, Y., Lei, X., Zhao, D., Long, R., and Wu, M. (2021). The Dual Impacts of Green Credit on Economy and Environment: Evidence from China. Sustainability 13 (8), 4574. doi:10.3390/su13084574
Wang, Z., Yang, Z., Zhang, Y., and Yin, J. (2012). Energy Technology patents-CO2 Emissions Nexus: An Empirical Analysis from China. Energy Policy 42, 248–260. doi:10.1016/j.enpol.2011.11.082
Wen, S., Lin, B., and Zhou, Y. (2021). Does Financial Structure Promote Energy Conservation and Emission Reduction? Evidence from China. Int. Rev. Econ. Finance 76, 755–766. doi:10.1016/j.iref.2021.06.018
Wen, S., and Liu, X. (2019). Financial Misallocation, Pollution and Sustainable Growth. Res. Econ. Manag. 3, 3–20.
Wen, Z., Hou, T., and Zhang, L. (2005). Comparing and Applying of Mediating Effect and Interaction Effects. J. Psychol. 37 (2), 268–274.
Wilson, C. (2018). Disruptive Low-Carbon Innovations. Energy Res. Soc. Sci. 37, 216–223. doi:10.1016/j.erss.2017.10.053
Xiao, Z., Yu, L., Liu, Y., Bu, X., and Yin, Z. (2022). Does Green Credit Policy Move the Industrial Firms toward a Greener Future? Evidence from a Quasi-Natural Experiment in China. Front. Environ. Sci. 9. doi:10.3389/fenvs.2021.810305
Xing, T., Jiang, Q., and Ma, X. (2017). To Facilitate or Curb? the Role of Financial Development in China's Carbon Emissions Reduction Process: A Novel Approach. Ijerph 14 (10), 1222. doi:10.3390/ijerph14101222
Xiu, J., Liu, H., and Zang, X. (2015). The Industrial Growth and Prediction under the Background of Green Credit and Energy Savingand Emission Reduction. Model Econ. Sci. 37, 55–62.
Xu, R., and Lin, B. (2017). Why Are There Large Regional Differences in CO 2 Emissions? Evidence from China's Manufacturing Industry. J. Clean. Prod. 140, 1330–1343. doi:10.1016/j.jclepro.2016.10.019
Xu, W. (2021). The Impact and Influencing Path of the Pilot Carbon Emission Trading Market--Evidence from China. Front. Environ. Sci. 9. doi:10.3389/fenvs.2021.787655
Zhang, K., Wang, D., and Zhou, H. (2016). Regional Endogenetic Strategic Interaction of Environmental Protection Investment and Emission. China Ind. Econ. 2, 68–82.
Keywords: green credit, disruptive low-carbon innovation, carbon reduction, mediation effect, generalized method of moments (GMM)
Citation: Lyu B, Da J, Ostic D and Yu H (2022) How Does Green Credit Promote Carbon Reduction? A Mediated Model. Front. Environ. Sci. 10:878060. doi: 10.3389/fenvs.2022.878060
Received: 18 February 2022; Accepted: 13 April 2022;
Published: 28 April 2022.
Edited by:
Chien-Chiang Lee, Nanchang University, ChinaReviewed by:
Muntasir Murshed, North South University, BangladeshCopyright © 2022 Lyu, Da, Ostic and Yu. This is an open-access article distributed under the terms of the Creative Commons Attribution License (CC BY). The use, distribution or reproduction in other forums is permitted, provided the original author(s) and the copyright owner(s) are credited and that the original publication in this journal is cited, in accordance with accepted academic practice. No use, distribution or reproduction is permitted which does not comply with these terms.
*Correspondence: Dragana Ostic, ZG9zdGljQHVqcy5lZHUuY24=
Disclaimer: All claims expressed in this article are solely those of the authors and do not necessarily represent those of their affiliated organizations, or those of the publisher, the editors and the reviewers. Any product that may be evaluated in this article or claim that may be made by its manufacturer is not guaranteed or endorsed by the publisher.
Research integrity at Frontiers
Learn more about the work of our research integrity team to safeguard the quality of each article we publish.