- 1Department of Animal Ecology and Systematics, Justus Liebig University Giessen, Giessen, Germany
- 2Department of Landscape Ecology and Resource Management, Justus Liebig University Giessen, Giessen, Germany
- 3Naturalis Biodiversity Center, Leiden, Netherlands
- 4Centre for International Development and Environmental Research (ZEU), Justus Liebig University Giessen, Giessen, Germany
- 5Limnology Research Unit, Ghent University, Ghent, Belgium
- 6Department of Biology, Royal Museum for Central Africa, Tervuren, Belgium
Schistosomiasis affects over 700 million people globally. 90% of the infected live in sub-Saharan Africa, where the trematode species Schistosoma mansoni and S. haematobium transmitted by intermediate hosts (IH) of the gastropod genera Biomphalaria and Bulinus are the major cause of the human disease burden. Understanding the factors influencing the distribution of the IH is vital towards the control of human schistosomiasis. We explored the applicability of a machine learning algorithm, random forest, to determine significant predictors of IH distribution and their variation across different geographic scales in crater lakes in western Uganda. We found distinct variation in the potential controls of IH snail distribution among the two snail genera as well as across different geographic scales. On the larger scale, geography, diversity of the associated mollusk fauna and climate are important predictors for the presence of Biomphalaria, whereas mollusk diversity, water chemistry and geography mainly control the occurrence of Bulinus. Mollusk diversity and geography are relevant for the presence of both genera combined. On the scale of an individual crater lake field, Biomphalaria is solely controlled by geography, while mollusk diversity is most relevant for the presence of Bulinus. Our study demonstrates the importance of combining a comprehensive set of predictor variables, a method that allows for variable selection and a differentiated assessment of different host genera and geographic scale to reveal relevant predictors of distribution. The results of our study contribute to making realistic predictions of IH snail distribution and schistosomiasis prevalence and can help in supporting strategies towards controlling the disease.
Introduction
Human schistosomiasis (bilharzia) is the second most important tropical parasitic disease after malaria (World Health Organization, 2016) and ranked the most important water-borne disease (Steinmann et al., 2006). It poses a global burden to humankind with over 700 million individuals in 78 countries at risk of infection, claiming over 200,000 lives annually (World Health Organization, 2016). In addition, more than 240 million people are infected worldwide, predominantly in sub-Saharan Africa (World Health Organization, 2016), where the disease burden is up to 90% of the global infections due to poor standards of living (Bergquist et al., 2017). Thus, schistosomiasis is commonly referred to as “the disease of the poor”. Countries in sub-Saharan Africa face a challenge of high population growth, and most people live in rural or semi-rural settings associated with poverty, poor sanitation and no access to clean water (Gray et al., 2010; King, 2010; Payne and Fitchett, 2010). In such geographical settings, people might continuously be in contact with water contaminated with schistosome eggs (Stothard et al., 2005), and a large part of the population is at risk of infection.
Despite schistosomiasis being one of the most prevalent tropical diseases (Steinmann et al., 2006), it is also probably the most neglected and was given little priority by the funding bodies compared to HIV/AIDS, malaria and tuberculosis (Hotez et al., 2007; Utzinger et al., 2009). Nevertheless, a recent growing interest in neglected tropical diseases including schistosomiasis has been observed over the last decade (World Health Organization, 2012; Shiff, 2017; King et al., 2020).
So far, strategies to control the spread of the disease via the provision of schistosomicides and/or WASH (water, sanitation, hygiene) programmes have shown limited effectiveness, and were consequently leading to disease re-emergences in spite of the interventions (Gryseels and Polderman, 1991; Chitsulo et al., 2000; Fenwick et al., 2009). Schistosomiasis is caused by trematode worms of the genus Schistosoma being transmitted through intermediate host (IH) snails. The reproductive cycle of Schistosoma trematodes starts with parasitic eggs released into freshwater through faeces and urine by infected humans. Eventually, motile larvae called miracidia hatch from the eggs and swim in search of snails to infect as intermediate host. The parasite then reproduces asexually within the snail, before shedding to the water as cercariae, larvae that penetrate the skin of the human host to complete the cycle and eventually cause the disease (Colley et al., 2014).
Sustainable vector snail control has been suggested as a more reliable approach to the schistosomiasis problem (Gryseels et al., 2006; Steinmann et al., 2006; Wang et al., 2008; Colley et al., 2014). The control aims at interrupting the transmission and stopping the spread of infection (Rollinson et al., 2013; Walz et al., 2015; Sokolow et al., 2016), by interrupting the Schistosoma life cycle through eliminating potential host snails from local habitats (King and Bertsch, 2015). Yet, this approach relies on the availability of high-quality snail distribution data, which represents a major knowledge gap in most developing countries in sub-Saharan Africa.
In sub-Saharan Africa, Schistosoma mansoni and S. haematobium are the major cause of the human disease burden in Africa (Chitsulo et al., 2000; Gryseels et al., 2006). Schistosoma mansoni is transmitted by snails of the genus Biomphalaria (Planorbidae) and causes human intestinal schistosomiasis. In contrast, S. haematobium is transmitted by species of Bulinus (Bulinidae) and cause human urogenital schistosomiasis (Wang et al., 2008; Colley et al., 2014). Schistosoma mansoni and S. haematobium are mainly distributed in and around a variety of freshwater habitats such as dams, lakes and rivers (Brown, 1994; Steinmann et al., 2006; Appleton and Madsen, 2012). Bulinus species in particular can live in permanent or seasonal pools, rice fields and ditches. In addition, there are several other species of Schistosoma that are of significant veterinary importance causing schistosomiasis in livestock. They are either hosted by Bulinus species (S. bovis) or selected species of the genus (S. magrebowiei) (Standley et al., 2012).
Previous studies dealing with the factors that influence the distribution of Schistosoma IH snails included water depth (Prah and James, 1978; Utzinger and Tanner, 2000; Boelee and Laamrani, 2004), altitude (John et al., 2008; Stanton et al., 2017), temperature, precipitation, pH level, forest cover and distance to nearest water bodies (Stensgaard et al., 2013), heat waves, droughts and floods (McCreesh and Booth, 2013; Pedersen et al., 2014), calcium and salinity (Hoverman et al., 2011; Maes et al., 2021). Although the relationships with these potential predictors have been examined and tested individually, they were not quantitatively assessed for their combined importance. The contribution of these factors to the distribution of IH snails and the prevalence of schistosomiasis accordingly across different regions is far from understood.
This study focuses on two main goals: 1) to assess the significance of extrinsic (geographical, hydrological, climatic, faunistic and anthropogenic) factors for the distribution of intermediate IH snails of the genera Bulinus and Biomphalaria, being the major causes of human infections in Africa, 2) to test for differences of potential predictors and their varying impact across different geographical scales. To do so, we used a model system of 56 crater lakes in western Uganda that variably contain IH species of the two genera, along with a diverse set of geographical, climatic, hydrological, faunistic and anthropogenic parameters. We used a machine learning approach (random forest, RF) to assess the importance of the individual parameters and how they differ across the study area. Considering the geographic variation of population density (Gelorini et al., 2012; Hartter et al., 2012), land use intensity (Hartter et al., 2015), and limnological characteristics of the lakes (De Crop and Verschuren, 2019), we hypothesize that a different set of parameters is relevant in the different regions examined. Moreover, we expect that different parameters control the distributions of the two genera, since they do have different dispersal and drought resistance capacities (Brown, 1994).
Materials and Methods
Study Area
The study area is located on the hilly uplands (>1,600 m a.s.l.) of the Edward-George branch of the East African Rift valley in western Uganda (Figure 1). It is bordered by the Rwenzori Mountain range in the North-West, Lake Albert in the North and Queen Elizabeth National Park in the South. It contains over 90 small crater lakes, most of which formed between 4,000 and 10,000 years ago as a result of faulting and volcanic eruptions (Vinogradov, 1980; Schumann et al., 2015). Lake Ntambi, which formed c. 50,000 years ago is an exception, (Dirk Verschuren, pers. comm.).
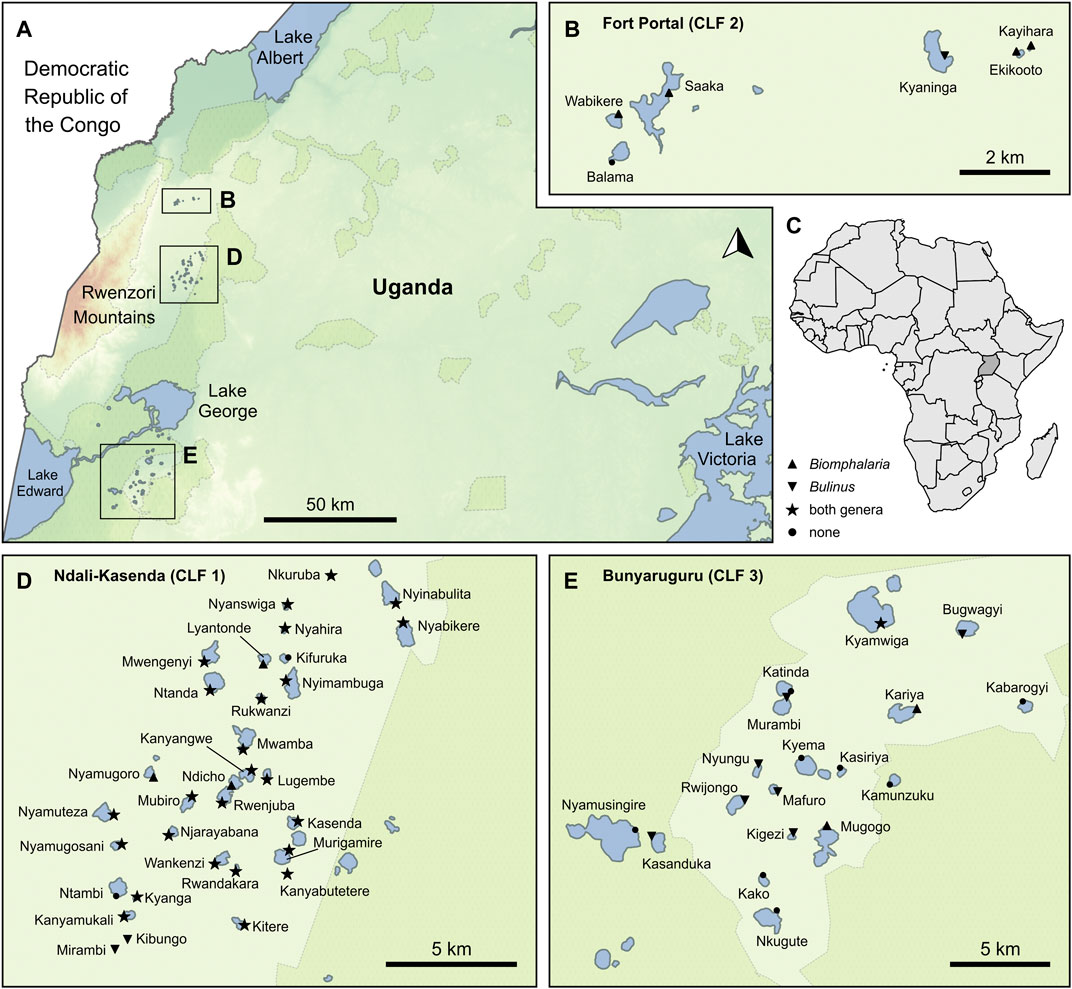
FIGURE 1. Study area and occurrences of intermediate host (IH) snails. Three crater lake fields (CLF1–3) (B,D,E) in western Uganda (A) in Africa (C) were studied. Symbols at sampled lakes indicate the presence of Biomphalaria (triangle), Bulinus (inverted triangle), and both genera combined (dark star), whereas lakes marked with a small circle indicate localities where no IH snail was found.
The crater lakes of western Uganda have been repeatedly promoted as an ideal model system for studying large environmental gradients in limnological characteristics in a setting that allows meaningful comparisons due to shared geological history (e.g. Melack, 1978; Mills and Ryves, 2012; Saulnier-Talbot and Lavoie, 2018). The crater lakes region is one of the most densely populated rural areas in sub-Saharan Africa (Hartter et al., 2012), with a population growth rate of 3.3% annually (Gelorini et al., 2012). The population growth is coupled with increasing human impact through settlement, fishing, agriculture on the fertile volcano soils, swimming and water extraction for irrigation and domestic use. As a result of the high population density, the prevalence of (human) schistosomiasis in the region has dramatically increased (Kabatereine et al., 2004; Kabatereine et al., 2006; John et al., 2008; Stanton et al., 2017). In 2014, the crater lakes region covered parts of the 73 out of 112 districts of Uganda with prevalence of schistosomiasis (Loewenberg, 2014). Nationwide, four million people are estimated to be infected and almost 20 million are at risk of infection (Loewenberg, 2014). The only preventative measures in the area are massive drug administration while environmental transmission interruption is rarely emphasized (Loewenberg, 2014).
Melack (1978) classified the region into four crater lake fields (CLFs, Figure 1). The Ndali-Kasenda field is locatedin the central part of the region, ∼20 km from Fort Portal field in the North and ∼65 km from Bunyaruguru field in the South. The fourth field of Katwe-Kikorongo contains lakes with saline waters (Rumes et al., 2011), unsuitable for mollusks (Tumwebaze et al., 2019).
Our dataset combines information from a total of 56 crater lakes in the three fields, including 32 lakes of the Ndali-Kasenda field (CLF 1), six lakes of the Fort Portal field (CLF 2) and 18 lakes of the Bunyaruguru field (CLF three; see Supplementary Table S1).
Data Collection
Malacological Field Data
Snails were sampled across the three regions in random months between 2010 and 2019 to account for a range of weather conditions, but mostly in dry seasons at normal or low water levels between 2010 and 2019 (Figure 1). Field work periods were aligned to times when highest population densities of the intermediate host snails were expected. Confounding effects by flooding and restricted accessibility during rainy seasons were avoided. Due to the steep and slippery escarpments of the crater lakes, sampling in the wet season was also avoided. Sampling methods involved dredging and/or scoop netting up to a maximum depth of 1.5 m or hand-picking snails found attached to shoreline vegetation and any solid substrates. The sampling time per lake was 40 min, and snails were collected in one to two localities (depending on the size of the respective lake). In some cases, lakes were visited more than once to ensure comprehensive representation of the local fauna. At each locality, we identified and counted the IH snails as well as the associated mollusk fauna on the genus level. The survey also revealed the presence of seven other non-host snail genera (Radix, Gabbiella, Pila, Melanoides, Segmentorbis, Afrogyrorbis and Gyraulus) as well as the bivalve genus Sphaerium.
Climatic and Environmental Data
We included air temperature and precipitation as proxies, because they have previously been shown to influence the distribution of freshwater mollusks (e.g., Hauffe et al., 2016a; Georgopoulou et al., 2016) and particularly those of IH snails (Appleton, 1978; Rowel et al., 2015). Specifically, temperature influences the survival and reproduction rates of snails (Paull and Johnson, 2011; McCreesh et al., 2014; Kalinda et al., 2017). Precipitation is associated with organic matter input and nutrient supply, which affects snail growth and fecundity (Madsen et al., 1987; Camara et al., 2012; Nyström Sandman et al., 2013). We retrieved the climatic data (averaged for the period 1970–2000) from the WorldClim two global database (Fick and Hijmans, 2017). We used mean annual temperature (BIO1), temperature of warmest month (BIO5), temperature of the coldest month (BIO6), annual precipitation (BIO12), precipitation of the wettest month (BIO13) and precipitation of the driest month (BIO14) to account for potential selectivity of the IH snail species to climatic fluctuations. Since the different temperature and precipitation parameters showed a similar range of variation, we calculated principal component analyses and used the first principal component for each of the two sets.
Water chemistry also plays a vital role for the occurrence and abundance of freshwater gastropods. This concerns pH, oxygen, conductivity, surface water temperature, magnesium and calcium (Rumes et al., 2011; Marie et al., 2015; Mahmoud et al., 2019; Alhassan et al., 2020; Olkeba et al., 2020). Surface water temperature, dissolved oxygen, pH and conductivity were measured using a handheld multi-meter probe. Calcium and magnesium data were retrieved from Rumes et al. (2011) and Nankabirwa et al. (2019).
Previous studies have shown the relevance of depth (both absolute lake depth and Secchi depth, i.e., a measure of water transparency) for the occurrence of both Bulinus and Biomphalaria. Absolute depth was retrieved from De Crop and Verschuren (2019), and water transparency (i.e., Secchi depth) was measured at the sampling points using a Secchi disk.
The crater lakes are characterized by seasonal fluctuations in water levels, and some lakes occasionally dry out (e.g., Lake Mirambi and Lake Kibungo). We included lake surface area and surface area variance over time as parameters in this study. The lake surface area was retrieved from satellite images from Google Earth Pro v. 7.3 taken in 2019. The lake area variance was calculated as the variance of four time slots of lake surface areas traced from satellite images taken in 2003, 2008, 2013 and 2018. Information for a few satellite images was missing, because either some lakes dried out or no records were captured in Google Earth. In such cases, we used the image of the closest time prior or after a given time slot to retrieve lake surface area.
Geographical Data
Longitude and latitude of the sampling sites were included as variables to account for potential variation in the geographical distribution of the IH snails. Altitude has been also proved relevant in the occurrence of snail hosts (John et al., 2008; Stanton et al., 2017) and was therefore considered in our study. Longitude, latitude and altitude were measured with a handheld Garmin GPS eTrex 20 device.
We used two measures for geographical distance to serve as proxies of biogeographical isolation, which might impact colonization and thus the IH presence in the area: 1) distance from a crater lake to the nearest other crater lake, and 2) distance from a crater lake to the nearest larger lake in the surrounding (i.e., lakes Victoria, Edward, Albert and George; Figure 1). All distances were measured “as the crow flies” in Google Earth.
Human Impact
To obtain a measure of human impact, we distinguished and quantified the proportion of land use. Since no data are available from online databases, we used the total percentage of cultivatable fields (cropland, fallow land and plantations) as a proxy and visualized a square of 0.0625 km2 (0.25 × 0.25 km) around each crater lake in Google Earth (centered around the lake centroid). Final percentages were arcsine/square-root transformed according to Warton and Hui (2011) to limit the influence of outliers.
Additionally, the number of people living in the surroundings of a lake directly relates to the risk of schistosomiasis infection. Due to the lack of population census records in the region, we counted the number of houses in a standardized area of 0.25 km2 (0.5 × 0.5 km) around each lake using satellite images from Google Earth taken in 2019 as a measure of population density.
A total of 20 predictor variables belonging to five categories were used in our study, 15 of which were retrieved in the course of the present survey, two were obtained from online databases and three were taken from the literature (Table 1, Supplementary Table S1).
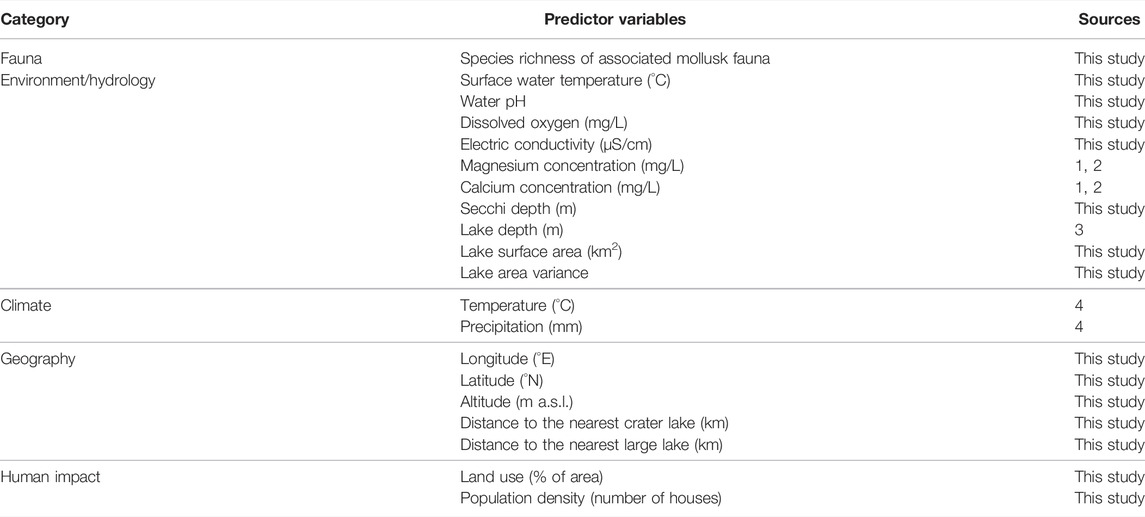
TABLE 1. Predictor variables used in this study. Note that temperature and precipitation each represent the first principal component calculated from three climate parameters; see text for details. Sources: 1—Rumes et al. (2011), 2—Nankabirwa et al. (2019), 3—De Crop and Verschuren (2019), 4—Fick and Hijmans (2017).
Data Analysis
We applied a machine learning approach, i.e., random forest (RF; Breiman, 2001), to assess the combined impact of the chosen set of predictors on the distribution of IH snails. Machine learning approaches, and particularly RFs, have gained prominence in classification and regression analyses across various fields of science in recent years (e.g., Huang and Boutros, 2016; Pang et al., 2017; Schonlau and Zou, 2020; Collin et al., 2021; Georganos et al., 2021; Ruiz-Aĺvarez et al., 2021). In classification problems, RFs have been demonstrated to give more accurate predictions than other approaches, such as logistic regression (Boulesteix et al., 2012; Bunyamin and Tunys, 2016; Couronné et al., 2018; Xia et al., 2019; Zhang et al., 2020). Since it is a non-parametric technique, the RF algorithm is not affected by multicollinearity among the predictor variables (Boonprong et al., 2018), which is a common problem in ecology. Also, many RF software packages come with convenient solutions to deal with missing values (Brieuc et al., 2018).
We conducted separate RF analyses to variably predict the presence of Bulinus, Biomphalaria and both genera combined. To assess variation of the potential predictors across different geographical scales, at a larger geographical extent, we ran analyses for the overall and complete dataset combining data from all the three crater lake fields. On the scale of individual crater lakes regions, we ran analyses for two subsets of CLF 1 and CLF 3. The analysis for Fort Portal field (CLF 2 subset) was not performed because it contains only six lakes. All analyses were done in the R statistical environment v. 4.0.3 (R Core Team, 2020), using the packages randomForest v. 4.6-14 (Liaw and Wiener, 2002), rfUtilities v. 2.1-5 (Evans and Murphy, 2019) and rfPermute v. 2.1.81 (Archer, 2020).
We performed imputation to fill missing data prior to further data analyses, using the function “rfImpute” in the package randomForest, which uses the RF algorithm to obtain weighted averages of the available observations. This was done for the predictors; calcium, magnesium and water depth, for which no data were available for 18, 16 and 16 lakes, respectively. Overall, missing data added up 4.7%. The “rfPermute” algorithm was used to assess variable importance in each RF model via permutation. The algorithm creates decision trees from the original dataset by random sampling of rows (i.e., lakes) without replacement. At each node, two-thirds of the rows are taken as training data to create the model, the remaining one-third is taken as so-called out-of-bag (OOB) sample and is used to make predictions and test for the performance of the model (Breiman, 1996; Breiman, 2001). Variables were permuted 100 times over 1,000 decision trees.
Model performance was additionally assessed via cross validation. This approach was chosen over the standard train–test data procedure because of the comparably low number of lakes in the dataset. Cross validation is a commonly used resampling method to assess the generalization potential of a model and to avoid overfitting (Berrar, 2019). We report here the kappa coefficient K, which determines the model’s predictive accuracy, i.e., it gives the percentage of the data that is in agreement with the model (and is thus the opposite of the validation error). We adopted the suggestion of Viera and Garrett (2005), who regarded kappa values of 0.81-1 as excellent and 0.61–0.80 as substantial agreement, to evaluate our model performances.
The relevance of individual parameters to the overall RF models was assessed using two importance metrics, i.e., the mean decrease in accuracy (MDA) and the mean decrease Gini (MDG) (Calle and Urrea, 2011; Huang and Boutros, 2016). Due to discrepancies in ranking results between MDA and MDG, the results of a single metric are not completely exhaustive (Strobl et al., 2007; Liu et al., 2011). Therefore, we included both metrics but discussed in detail only those parameters that are found significant by both MDA and MDG. We used partial dependence plots to visualize the relationships and marginal effects of individual predictor variables (Friedman, 2001; Evans et al., 2011).
Results
Models converged and were stable across all datasets, with those for Biomphalaria always performing better than those for Bulinus or both genera combined (Supplementary Figure S1). The RF models resulted in excellent to substantial classification successes for the presences of Biomphalaria and Bulinus in relation to the chosen set of predictor variables (Table 2). Classification errors were generally higher for false negatives compared to false positives, which is probably a result of the low number of absences in all datasets. Nonetheless, the cross validation showed that the classification agreements ranged from substantial (CLF subsets) to excellent (total dataset; Table 2).
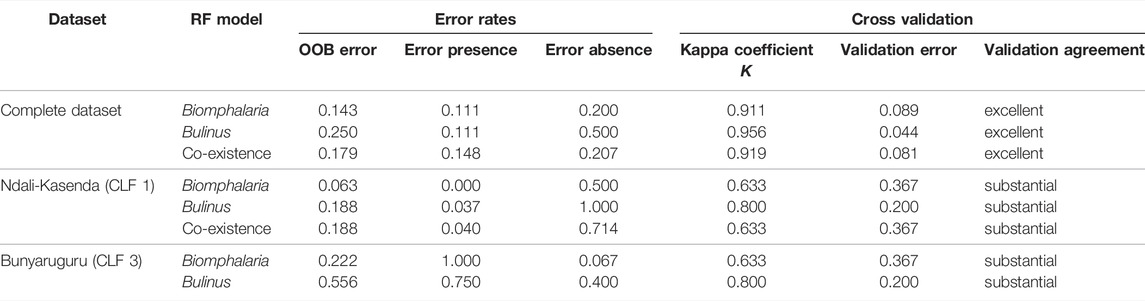
TABLE 2. Error rates and results of cross validation for all runs of the random forest (RF) models. Validation agreement was evaluated in accordance with Viera and Garrett (2005). The co-existence model for CLF three had insufficient data and is not included here. OOB, out-of-bag error.
For the overall and complete dataset, geographical, water chemistry and biotic parameters were the most important predictors, but their relative importance and contributions varied across the three RF models, i.e., Biomphalaria vs. Bulinus vs. both genera combined (Figure 2). The distribution of Biomphalaria was mainly controlled by latitude, longitude, diversity of the associated mollusk fauna and distance to large lake, as well as by precipitation to some extent; oxygen was only found significant by MDA. For Bulinus, the diversity of the associated mollusk fauna, water conductivity and latitude were most important, whereas only MDG identified altitude as a significant predictor. For the combined model, the diversity of the associated mollusk fauna, latitude, longitude and distance to large lake were found relevant. Distance to the next crater lake, magnesium and calcium played a minor role and were only found significant by MDA. Other predictors such as human impact, water pH, surface area and temperature had very little effect and were not significantly impacting the IH species distribution in the region (Figure 2).
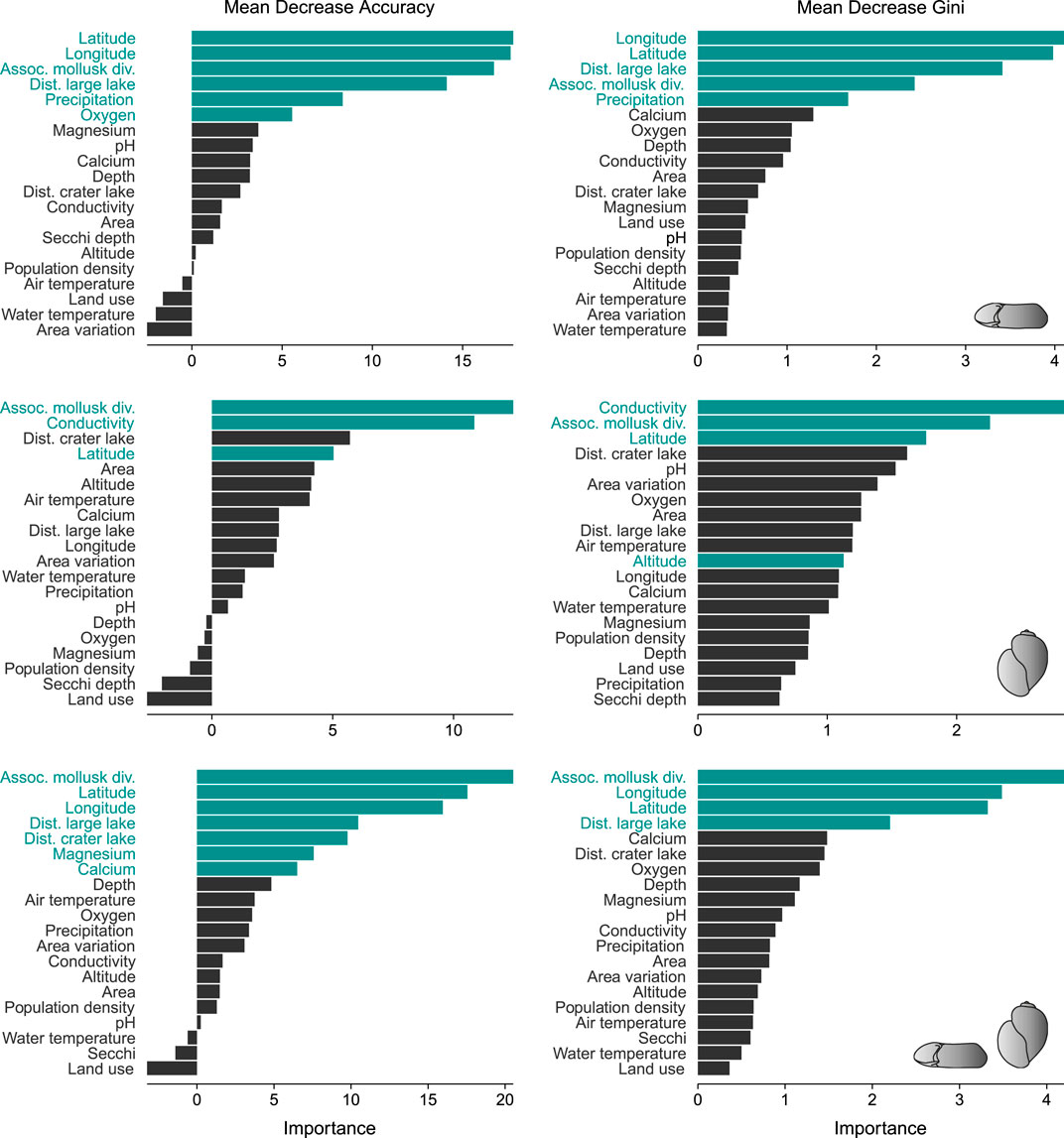
FIGURE 2. Importance scores of predictor variables for Biomphalaria (upper panels), Bulinus (middle panels) and both genera combined (lower panels) for the complete dataset. The green bars indicate the significant predictors as determined via permutation tests. See Supplementary Figure S2 for the importance scores of the subset CLF 1.
A different set of parameters was found to be important for the presence of IH snails in individual crater lake fields. For CLF 1, the distance to the next crater lake was the sole important parameter for the distribution of Biomphalaria (Supplementary Figure S2). In turn, the diversity of the associated mollusk fauna seemed to be the most relevant factor shaping the distribution of Bulinus, in addition to distance to the next large lake and lake surface area (MDA only). The co-existence of both genera was controlled by the diversity of the associated mollusk fauna and distance from the next crater lake (Supplementary Figure S2). The model for CLF three yielded comparably high error rates, concerning both overall errors as well as classification errors for presences and absences (Table 2). Consequently, any association found for CLF 3 with individual parameters is unreliable and will not be discussed further.
The predicted probabilities for the occurrence of IH snails show non-linear relationships with individual predictor variables, with a generally positive trend being apparent in many cases (Figure 3). For Biomphalaria, the predicted probabilities of its occurrence increases with a rise in latitude, longitude, distance to the large lake (with a slight increase at the end), diversity of the associated mollusk fauna and precipitation. Increasing mollusk diversity also links with an increased probability to encounter Bulinus. The parameter conductivity shows a more complex relationship, featuring a steep probability increase up to ∼400 μS/cm followed by a weakly, more or less gradually declining trend towards higher values (Figure 3). For both genera combined, similar positive trends are revealed for the relationship with the diversity of the associated mollusk fauna, latitude and longitude. The association with distance to the nearest large lake is more complex but indicates an increasing probability to encounter both genera above a distance of ∼35 km.
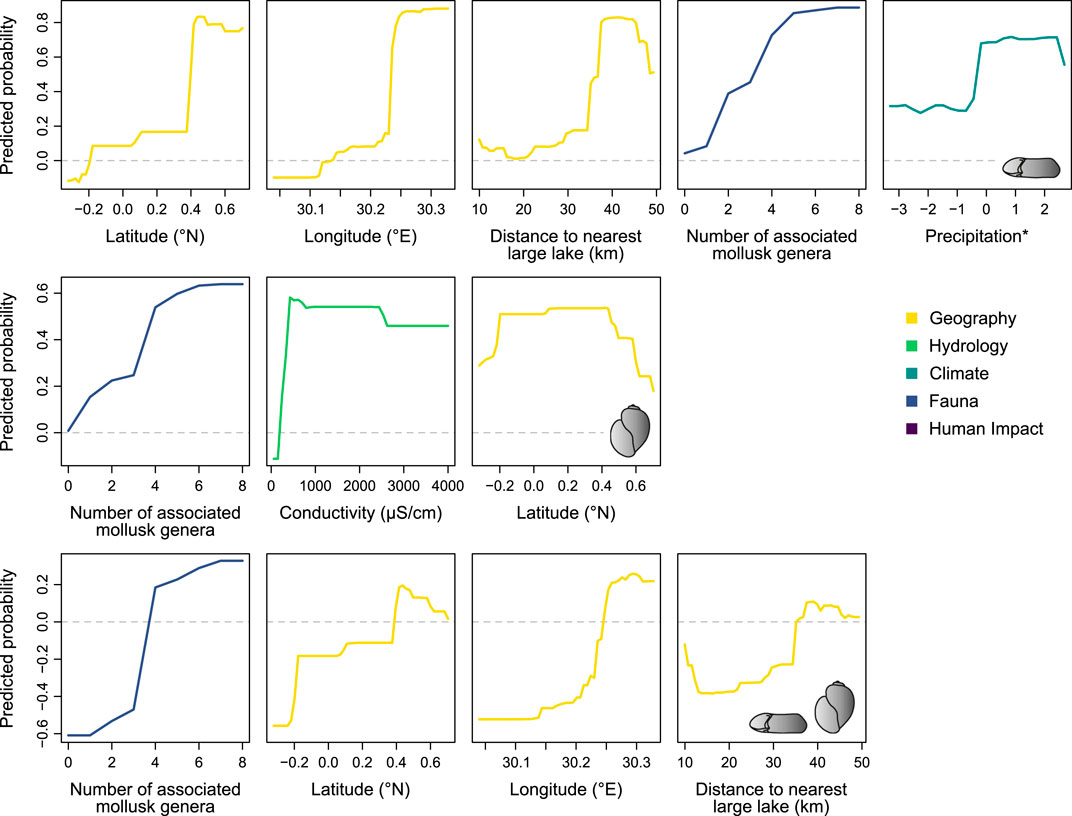
FIGURE 3. Predicted probabilities for the presence of Biomphalaria (upper panels), Bulinus (middle panels) and both genera combined (lower panels) in relation to selected predictor variables from each dataset. Only the contributors that were found significant by both importance metrics (MDA and MDG) for the entire dataset are shown (compare Figure 2; see Supplementary Figures S3–S8 for the remaining predictors as well as for the predicted probabilities for CLF 1). The asterisk denotes scaled parameters, i.e., the x-axis does not denote the original extent of the variable.
Discussion
Understanding the factors influencing the distribution of snails acting as IH for Schistosoma is crucial for the control of human schistosomiasis. In this study, we explored the applicability of a machine learning algorithm to determine significant predictors of IH distribution and whether they differ among different Ugandan crater lakes. We found distinct variation in the potential controls of IH snail distribution. A different set of parameters is found to influence the distribution of the different genera and across different geographical scales. On the larger scale, geography, diversity of the associated mollusk fauna and climate are important predictors for the presence of Biomphalaria, whereas mollusk diversity and water chemistry control the occurrence of Bulinus. Finally, mollusk diversity and geography are relevant for the presence of both genera. On the scale of an individual crater lake field (CLF1) geography (yet with a different variable) and mollusk diversity are relevant for the presence of Bulinus and both genera while Biomphalaria is solely controlled by geography. These results confirm our hypothesis that a different set of parameters is relevant on different geographical scales.
In the following sections, we discuss the relationships between IH snail occurrence and individual factors and groups of factors (geography, hydrology/water chemistry, climate, biotic and human impact) in an ecological context. Specifically, we discuss the distribution of the two genera with respect to metacommunity assembly processes, i.e., dispersal limitation, environmental filtering and biotic interactions, which limit the successful establishment of species in an ecosystem (see, e.g., Hauffe et al., 2016b for another freshwater gastropod example).
Geography: A Driver on Various Spatial Scales
Geographical variables were found to be among the most important controls for IH snail distribution, but the individual parameters depend on the scale of observation and the taxon in question. Latitude, longitude and distance to the nearest large lake are relevant for the occurrence of Biomphalaria as well as both genera combined. In contrast, Bulinus is much less influenced by geographical variables. Although latitude and altitude were identified significant, no positive relationship was found for the first (Figure 3), whereas the latter was of comparatively low importance (Figure 2). A similar trend is found in the Ndali-Kasenda crater lake field (CLF 1), where the distribution of Biomphalaria is strongly shaped by the distance to the nearest crater lake, whereas the presence of Bulinus is mostly related to the diversity of the associated mollusk fauna (Supplementary Figure S2).
The strong positive relationship between the presence of Biomphalaria and latitude as well as longitude reflects the uneven occurrence of the genus across the study area (Figure 1). Particularly the rarity of the genus in the Bunyaruguru crater lake field (CLF 3) indicates that dispersal limitations and/or environmental filtering (e.g., Hauffe et al., 2016b) might constrain its distribution.
The generally positive association between the occurrence of Biomphalaria and distance to the nearest large lake is surprising at first, considering that distance is related to biogeographical isolation and colonization potential (Covich, 2010). Generally, these snails have a high passive dispersal capacity, a high reproduction rate and short generation times (Brown 1994). This combination is pronounced particularly in pulmonate snails such as Biomphalaria and makes them prime colonizers (Kappes and Haase, 2012; Kappes et al., 2014). The crater lakes are hydrologically disconnected, the possible longitudinal dispersal means are natural vectors such as birds (Kappes and Haase, 2012) and humans, through the attachment to fishing nets and/or boats.
A possible explanation for the positive association with distance concerns the taxonomic resolution. Here, we investigated the presence of genera, which may overprint patterns of dispersal and colonization of individual species of Bulinus and Biomphalaria. Also, Euclidean shore-to-shore distances might not reflect real dispersal means or pathways, because hydrological connectivity varies considerably between the large lakes and the crater lake fields. For example, the Fort Portal field (CLF 2) is connected to the Lake George–Lake Edward system via the Mpanga River but not to the geographically closer Lake Albert. The pattern may also results from choosing only four major lakes but disregarding smaller ones and river systems. Finally, in colonization processes, stochastic components might play an important role. For instance, regular episodes of shifting mixing regimes in some crater lakes (De Crop and Verschuren, 2021) could lead to low dissolved oxygen and consequently to the demise of most aquatic life. Based on genetic relationships of Bulinus, a previous study suggested that the large lakes acted as potential sources for (re)-colonization of the crater lakes (Tumwebaze et al., 2019), a scenario that cannot be tested here given the lack of genetic data. Fast re-appearance or aestivation in fluctuating environments is an intrinsic ecological feature of many Bulinus species, including the ones found in the study area (Watson, 1958; Brown, 1994). As such, the colonization and re-colonization patterns might be more complex and faster than previously anticipated.
Altitude was found to have limited influence on the distribution of IH snails. Several previous studies have indicated a shift in recent years of intestinal human schistosomiasis transmission towards higher altitudes (Kabatereine et al., 2004; John et al., 2008; Stanton et al., 2017). This is also supported by our findings showing that IH snails occur up to approx. 1,600 m a.s.l., and thus considerably higher than the previously presumed threshold of 1,400 m a.s.l. (Kabatereine et al., 2004). Future studies will have to establish an upper limit for both forms of schistosomiasis, because both IH snail genera have been found at altitudes above 2,000 m a.s.l. in Uganda (Stanton et al., 2017, unpubl. data).
Associated Mollusk Diversity
A strong control for the distribution of both Bulinus and Biomphalaria is the diversity of the associated mollusk fauna. The strong positive relationships in all three models (Figure 3) indicate that both genera are more likely encountered in generally diverse systems. One may expect the opposite, that a higher number of species (especially in a small crater lake) results in higher competition (e.g. Svanbäck and Bolnick, 2007; Hauffe et al., 2016b) and thus a lower chance of successful establishment. Instead, the positive association with diversity indicates that the IH snail genera are present in environments that provide favorable conditions for mollusks generally.
The associated mollusk fauna consists predominantly of pulmonates (Radix natalensis and several species of planorbids other than Biomphalaria), which are good colonizers in general and are characterized by a high productivity and shorter generation times (Kappes et al., 2014). Malacological surveys showed the presence of Biomphalaria sudanica, Biomphalaria pfeifferi, Bulinus forskalii, Bulinus globosus, and Bulinus tropicus (Tumwebaze et al., 2019; Tumwebaze et al., unpubl. data). All Biomphalaria species in the region are regarded susceptible to S. mansoni. Only B. forskalii and B. globosus were identified as host species for human schistosomes and the majority of Bulinus in the crater lakes region were B. tropicus, which is an important IH snail for livestock schistosomes. The non-pulmonate species are common and widespread regional species with no particular ecological requirements (Brown, 1994). More specialized taxa such as Bellamya or Cleopatra or unionid bivalves were not found in the crater lakes. The mollusk association in the crater lakes could still be seen as depauperate. The most obvious variation in habitat conditions and thus potentially determining mollusk associations is in the limnological characteristics of the crater lakes. Although we gathered a variety of different parameters concerning water chemistry and climatic conditions, the associations with individual parameters do not allow drawing a multifactorial picture. Moreover, the parameters measured for each crater lake do not cover seasonal variability (except for climate and lake area variation).
Hydrology and Water Chemistry
Only selected hydrochemical variables had an influence on the distribution of IH snails in our study region. Water conductivity was a significant driver across the entire study region and in CLF one for Bulinus. For Biomphalaria, oxygen was found a significant driver by one metric (MDA), but its relative importance was low (Figure 2). Similarly, MDA found the presence of both genera linked to magnesium and calcium, but again with low importance values.
Water conductivity determines the ionic strength of the concentration of dissolved solids including calcium and magnesium (Cormier et al., 2013). An expanding distribution with increasing conductivity was also suggested by Camara et al. (2012). This might reflect the presence of dissolved ions (e.g., calcium), which stimulate shell development for snail species (Dillon, 2000; see also below). The non-linear relationship with a sharp increase around ∼400 μS/cm followed by a nearly steady but weak decline indicates that a certain threshold must be met to allow the establishment of Bulinus in a lake. Higher electric conductivity is, in turn, less favorable. Conductivity as a complex factor integrating several chemical components has been identified as a determinant for Bulinus mortality and thus poses an important constraint on its occurrence (Brown, 1994; Marie et al., 2015).
The predicted probabilities for the presence of both genera in relation to magnesium and calcium concentrations, which were, however, only found significant by one importance metric (MDA), demonstrate that a certain threshold must be surpassed in both factors to promote the establishment of IH snails. Especially the relationship with calcium is not surprising, considering that a low concentration would constrain snail growth, fecundity, survival rate and reproduction, which, in turn, limit snail distribution (Dillon, 2000; Brodersen and Madsen, 2003). The concentration of calcium and magnesium and their ratio in the water both affect the presence and life cycle performances in southern African streams with thresholds at the lower and higher ends of concentrations (Brown, 1994).
Climate
Climatic factors seem to have surprisingly little effect on the distribution of IH snails in Ugandan crater lakes. Precipitation was found a significant but rather weak predictor in the model with Biomphalaria, whereas air and water temperature do not appear influential. Generally, climatic conditions and climate change are known to be important predictors for mollusk species distribution on larger geographical scales (Marcogliese, 2008; Stensgaard et al., 2019) and were also found to be relevant for IH snails. Temperature influences the survival and reproduction rates of the snails (Paull and Johnson, 2011; McCreesh et al., 2014; Kalinda et al., 2017). Higher rainfall causes more runoff into freshwater ecosystems increasing the supply of organic matter serving as food for the snails, which, in turn, promotes growth and fecundity (Madsen et al., 1987; Camara et al., 2012; Nyström Sandman et al., 2013; but see also discussion in David et al., 2018 for opposite associations). This may also explain the relationship with precipitation in our case. However, the lack of a (strong) association in our models is likely owed to the constrained geographical scale of our observations. The crater lakes are located in the same climatic zone in western Uganda, which is why precipitation and air temperature vary only little across the study region. Whereas air temperature seems to be irrelevant on that scale, the relationship with precipitation indicates that even small variations might have a significant impact on the IH snail distribution.
Non-Significant Drivers
A series of parameters was found to be non-significant in any of our models. Here, we briefly discuss the potential reasons for these findings, especially in the light of conflicting results reported in the literature. In contrast, previous studies found relationships between the distribution of Biomphalaria and water pH, whereas both oxygen and water pH have been suggested to determine the occurrence of Bulinus (Yirenya-Tawiah et al., 2011; Stensgaard et al., 2013; Marie et al., 2015; Mahmoud et al., 2019; Alhassan et al., 2020). However, no such influences were noticed despite of the influential factors of high oxygen concentration and water pH of the lakes studied (Supplementary Table S1). Possibly, the high carbon dioxide emission from decomposing submerged vegetation and organic matter, together with the presence of other dissolved ions could have indirectly affected such relationships (Tchakonté et al., 2014). Lake area variation, being a measure of ecosystem stability, seems also of little importance. Apparently, most of the lakes show minimal fluctuations through time, and the few that occasionally dry up could not influence the distribution or recolonization, which is facilitated by the short distances between the crater lakes. The mollusk associations of mostly opportunistic species found is also supporting this interpretation (see above).
Human impact, quantified by both land use and population density around the crater lakes, has apparently no impact on snail distribution. This result is rather unexpected, because humans are often involved in introduction of snails into new environments as passive dispersal vectors (Kappes and Haase, 2012). In the Ugandan crater lake fields, extensive anthropogenic activities (e.g., multipurpose water fetching, watering for livestock, littering and pollution) are limited to lower, relatively flat and thus more easily accessible shores of most of the crater lakes. As such, one may have expected an impact on snail occurrences. Moreover, only about 26% of the crater lakes are located in national parks (Queen Elizabeth NP and Kibale NP), thus three-quarter of the lakes are accessible and utilizable by humans. Despite these constraints, some reserves and parks like Kibale NP increasingly face migration of settlers and extensive cultivation (Hartter et al., 2015). Perhaps the prevailing population pressure affects the prevalence of infected snails, but it does not influence their presence in general. Follow-up studies should focus specifically on this aspect for mollusks communities and the prevalence of schistosomiasis. Interestingly, in other non-gastropod taxa such as cladocerans (Rumes et al., 2011) and fungi communities (Gelorini et al., 2012), negative effects of land use change and anthropogenic pressure have already been demonstrated for the crater lakes region. With increasing human activities in the Ugandan crater lakes region (including tourism), we expect further changes in biological communities of the lakes as well as increasing cases of human schistosomiasis among both local communities and visiting travelers (Lachish et al., 2013) in the near future.
Methodological Implications and Limitations
Despite the numerous advantages machine learning approaches like RFs offer, one disadvantage concerns the non-linearity of the approach, resulting in a limited prediction power outside the data range. Therefore, we cannot extrapolate our findings to other datasets or regions. Furthermore, small datasets (e.g. CLF 2, Biomphalaria subset with CLF3), decisively limit RF classification due to insufficient data. Optimally, future studies need to include data from a larger geographical scale, involving a greater variation in the ranges of predictor variables, to provide more general predictions for the controls of IH snail distributions. Using machine-learning algorithms like RFs on a comprehensive dataset will eventually facilitate more general conclusions about the importance of individual predictors (or sets of predictors) for the presence of IH snails. Future studies may focus on applying this approach to map infection risk areas, especially in comparison to areas with actual prevalence records and those where preventative measures are in place.
Conclusion
The results indicate that Biomphalaria is mainly controlled by geography, associated mollusk diversity and climate, while fauna, hydrology and to some extent geography controlled the presence of Bulinus. Geography (Biomphalaria) and mollusk diversity (Bulinus) were the only significant predictors on the scale of an individual crater lake field. The intricate relationship between IH snail distribution and geography likely reflects dispersal limitations and/or environmental filtering on the hand and a complex pattern of (re-)colonization on the other hand. The positive association with the diversity of accompanying mollusks, as well as the relationship with water conductivity, indicates that IH snails are common in ecosystems offering favorable conditions for mollusks in general.
Our machine learning approach helped disentangling relevant factors in IH snail distribution. The results of this study provide baseline data that assist future research towards controlling schistosomiasis.
Data Availability Statement
All data used in the study is available in the paper or the Supplementary Material.
Author Contributions
CA, ZT, and IT conceived the study. CA and IT conducted field work. ZT and TN performed the analyses. ZT wrote the manuscript with contributions from TN and CA. TN, BS, and CH prepared the figures. LB and CA supervised and critically revised the study. All authors reviewed the manuscript and approved the final version.
Funding
Research was partly supported by German Academic Exchange Service (DAAD) in support of a PhD scholarship for ZT (Grant No. 57507871) and German Science Foundation (DFG, Grant Nos. SCHR352/9-1, AL1076/5-2 and AL1076/6-2).
Conflict of Interest
The authors declare no conflict of interest. Any suggestions, results, discussions and conclusions or recommendations presented in this study are those of the authors and do not express the views of the funding agencies.
Publisher’s Note
All claims expressed in this article are solely those of the authors and do not necessarily represent those of their affiliated organizations, or those of the publisher, the editors and the reviewers. Any product that may be evaluated in this article, or claim that may be made by its manufacturer, is not guaranteed or endorsed by the publisher.
Acknowledgments
We thank Julius Tumusiime and Naboth Ainomugisha (Mbarara University of Science and Technology), Marie Claire Dusabe (formerly at Rwanda Wildlife Conservation Association, Kigali, Rwanda), Thies Geertz, Daniel Engelhard, and Roland Schultheiß (formerly JLU Giessen) for assistance during field work between 2010 and 2019. We also acknowledge the National Council for Science of Uganda together with Uganda Wildlife Authority granting us permits to conduct field work. We are moreover grateful to Klemens Ekschmitt (JLU Giessen) for fruitful discussions concerning classification problems and to Catharina Clewing (JLU Giessen) for creating snail reconstructions for the figures. Two reviewers are thanked for their constructive comments.
Supplementary Material
The Supplementary Material for this article can be found online at: https://www.frontiersin.org/articles/10.3389/fenvs.2022.871735/full#supplementary-material
References
Alhassan, A., Abidemi, A., Gadzama, I., Shaaba, R., Wada, Y., and Kelassanthodi, R. (2020). Distribution and Diversity of Freshwater Snails of Public Health Importance in Kubanni Reservoir and Weir/sediment Trap, Zaria, Nigeria. J. Environ. Occup. Health 10 (1), 1–9. doi:10.5455/jeoh.20190704093531
Appleton, C. C., and Madsen, H. (2012). Human Schistosomiasis in Wetlands in Southern Africa. Wetlands Ecol. Manage. 20 (3), 253–269. doi:10.1007/s11273-012-9266-2
Appleton, C. C. (1978). Review of Literature on Abiotic Factors Influencing the Distribution and Life Cycles of Bilharziosis Intermediate Host Snails. Malacol. Rev. 11, 1–25.
Archer, E. (2020). rfPermute: Estimate Permutation P-Values for Random Forest Importance Metrics. R Package Version 2.1.81. Available at: https://cran.r-project.org/package=rfPermute. (Accessed July 1, 2021).
Bergquist, R., Zhou, X.-N., Rollinson, D., Reinhard-Rupp, J., and Klohe, K. (2017). Elimination of Schistosomiasis: the Tools Required. Infect. Dis. Poverty 6 (1), 1–9. doi:10.1186/s40249-017-0370-7
Berrar, D. (2019). “Cross-Validation,” in Encyclopedia of Bioinformatics and Computational Biology. Editors S. Ranganathan, M. Gribskov, K. Nakai, and C. Schönbach (Oxford: Academic Press), 542–545. doi:10.1016/B978-0-12-809633-8.20349-X
Boelee, E., and Laamrani, H. (2004). Environmental Control of Schistosomiasis through Community Participation in a Moroccan Oasis. Trop. Med. Int. Health 9 (9), 997–1004. doi:10.1111/j.1365-3156.2004.01301.x
Boonprong, S., Cao, C., Chen, W., and Bao, S. (2018). Random Forest Variable Importance Spectral Indices Scheme for Burnt Forest Recovery Monitoring-Multilevel RF-VIMP. Remote Sensing 10 (6), 807. doi:10.3390/rs10060807
Boulesteix, A.-L., Janitza, S., Kruppa, J., and König, I. R. (2012). Overview of Random forest Methodology and Practical Guidance with Emphasis on Computational Biology and Bioinformatics. WIREs Data Mining Knowl. Discov. 2 (6), 493–507. doi:10.1002/widm.1072
Brieuc, M. S. O., Waters, C. D., Drinan, D. P., and Naish, K. A. (2018). A Practical Introduction to Random Forest for Genetic Association Studies in Ecology and Evolution. Mol. Ecol. Resour. 18 (4), 755–766. doi:10.1111/1755-0998.12773
Brodersen, J., and Madsen, H. (2003). The Effect of Calcium Concentration on the Crushing Resistance, Weight and Size of Biomphalaria sudanica (Gastropoda: Planorbidae). Hydrobiologia 490 (1), 181–186. doi:10.1023/a:1023495326473
Brown, D. S. (1994). Freshwater Snails of Africa and Their Medical Importance. Second edition. London: Taylor & Francis, 609.
Bunyamin, H., and Tunys, T. (2016). A Comparison of Retweet Prediction Approaches: the Superiority of Random Forest Learning Method. Telkomnika 14 (3), 1052–1058. doi:10.12928/TELKOMNIKA.v14i3.3150
Calle, M. L., and Urrea, V. (2011). Letter to the Editor: Stability of Random forest Importance Measures. Brief. Bioinform. 12 (1), 86–89. doi:10.1093/bib/bbq011
Camara, I. A., Bony, Y. K., Diomandé, D., Edia, O. E., Konan, F. K., Kouassi, C. N., et al. (2012). Freshwater Snail Distribution Related to Environmental Factors in Banco National Park, an Urban reserve in the Ivory Coast (West Africa). Afr. Zoolog. 47 (1), 160–168. doi:10.1080/15627020.2012.11407534
Chitsulo, L., Engels, D., Montresor, A., and Savioli, L. (2000). The Global Status of Schistosomiasis and its Control. Acta Tropica 77 (1), 41–51. doi:10.1016/S0001-706X(00)00122-4
Colley, D. G., Bustinduy, A. L., Secor, W. E., and King, C. H. (2014). Human Schistosomiasis. Lancet 383 (9936), 2253–2264. doi:10.1016/S0140-6736(13)61949-2
Collin, F. D., Durif, G., Raynal, L., Lombaert, E., Gautier, M., Vitalis, R., et al. (2021). Extending Approximate Bayesian Computation with Supervised Machine Learning to Infer Demographic History from Genetic Polymorphisms Using DIYABC Random Forest. Mol. Ecol. Resour. 21 (8), 2598–2613. doi:10.1111/1755-0998.13413
Cormier, S. M., Suter, G. W., Zheng, L., and Pond, G. J. (2013). Assessing Causation of the Extirpation of Stream Macroinvertebrates by a Mixture of Ions. Environ. Toxicol. Chem. 32 (2), 277–287. doi:10.1002/etc.2059
Couronné, R., Probst, P., and Boulesteix, A.-L. (2018). Random forest versus Logistic Regression: a Large-Scale Benchmark experiment. BMC Bioinformatics 19 (1), 1–14. doi:10.1186/s12859-018-2264-5
Covich, A. P. (2010). Winning the Biodiversity Arms Race Among Freshwater Gastropods: Competition and Coexistence through Shell Variability and Predator Avoidance. Hydrobiologia 653 (1), 191–215. doi:10.1007/s10750-010-0354-0
David, N. F., Cantanhede, S. P. D., Monroe, N. B., Pereira, L. P. L. A., Silva-Souza, N., Abreu-Silva, A. L., et al. (2018). Spatial Distribution and Seasonality of Biomphalaria Spp. In São Luís (Maranhão, Brazil). Parasitol. Res. 117 (5), 1495–1502. doi:10.1007/s00436-018-5810-1
De Crop, W., and Verschuren, D. (2019). Determining Patterns of Stratification and Mixing in Tropical Crater Lakes through Intermittent Water-Column Profiling: A Case Study in Western Uganda. J. Afr. Earth Sci. 153, 17–30. doi:10.1016/j.jafrearsci.2019.02.019
De Crop, W., and Verschuren, D. (2021). Mixing Regimes in the Equatorial Crater Lakes of Western Uganda. Limnologica 90, 125891. doi:10.1016/j.limno.2021.125891
Evans, J. S., and Murphy, M. A. (2019). rfUtilities: Random Forests Model Selection and Performance Evaluation. R Package Version 2.1-5. Available at: https://cran.r-project.org/package=rfUtilities. (Accessed July 1, 2021).
Evans, J. S., Murphy, M. A., Holden, Z. A., and Cushman, S. A. (2011). “Modeling Species Distribution and Change Using Random forest,” in Predictive Species and Habitat Modeling in Landscape Ecology (New York, NY: Springer), 139–159. doi:10.1007/978-1-4419-7390-0_8
Fenwick, A., Webster, J. P., Bosque-Oliva, E., Blair, L., Fleming, F. M., Zhang, Y., et al. (2009). The Schistosomiasis Control Initiative (SCI): Rationale, Development and Implementation from 2002-2008. Parasitology 136 (13), 1719–1730. doi:10.1017/S0031182009990400
Fick, S. E., and Hijmans, R. J. (2017). WorldClim 2: New 1‐km Spatial Resolution Climate Surfaces for Global Land Areas. Int. J. Climatol 37 (12), 4302–4315. doi:10.1002/joc.5086
Friedman, J. H. (2001). Greedy Function Approximation: a Gradient Boosting Machine. Ann. Stat. 29 (5), 1189–1232. doi:10.1214/aos/1013203451
Gelorini, V., Verbeken, A., Lens, L., Eggermont, H., Odgaard, B. V., and Verschuren, D. (2012). Effects of Land Use on the Fungal Spore Richness in Small Crater-lake Basins of Western Uganda. Fungal Divers. 55 (1), 125–142. doi:10.1007/s13225-012-0155-z
Georganos, S., Grippa, T., Niang Gadiaga, A., Linard, C., Lennert, M., Vanhuysse, S., et al. (2021). Geographical Random Forests: a Spatial Extension of the Random forest Algorithm to Address Spatial Heterogeneity in Remote Sensing and Population Modelling. Geocarto Int. 36 (2), 121–136. doi:10.1080/10106049.2019.1595177
Georgopoulou, E., Neubauer, T. A., Harzhauser, M., Kroh, A., and Mandic, O. (2016). Distribution Patterns of European Lacustrine Gastropods: a Result of Environmental Factors and Deglaciation History. Hydrobiologia 775 (1), 69–82. doi:10.1007/s10750-016-2713-y
Gray, D. J., McManus, D. P., Li, Y., Williams, G. M., Bergquist, R., and Ross, A. G. (2010). Schistosomiasis Elimination: Lessons from the Past Guide the Future. Lancet Infect. Dis. 10 (10), 733–736. doi:10.1016/S1473-3099(10)70099-2
Gryseels, B., and Polderman, A. M. (1991). Morbidity, Due to Schistosomiasis Mansoni, and its Control in Subsaharan Africa. Parasitol. Today 7 (9), 244–248. doi:10.1016/0169-4758(91)90238-J
Gryseels, B., Polman, K., Clerinx, J., and Kestens, L. (2006). Human Schistosomiasis. Lancet 368 (9541), 1106–1118. doi:10.1016/S0140-6736(06)69440-3
Hartter, J., Stampone, M. D., Ryan, S. J., Kirner, K., Chapman, C. A., and Goldman, A. (2012). Patterns and Perceptions of Climate Change in a Biodiversity Conservation Hotspot. Plos One 7 (2), e32408. doi:10.1371/journal.pone.0032408
Hartter, J., Ryan, S. J., MacKenzie, C. A., Goldman, A., Dowhaniuk, N., Palace, M., et al. (2015). Now There Is No Land: a story of Ethnic Migration in a Protected Area Landscape in Western Uganda. Popul. Environ. 36 (4), 452–479. doi:10.1007/s11111-014-0227-y
Hauffe, T., Schultheiß, R., Van Bocxlaer, B., Prömmel, K., and Albrecht, C. (2016a). Environmental Heterogeneity Predicts Species Richness of Freshwater Mollusks in Sub-Saharan Africa. Int. J. Earth Sci. (Geol Rundsch) 105 (106), 1795–1810. doi:10.1007/s00531-014-1109-3
Hauffe, T., Albrecht, C., and Wilke, T. (2016b). Assembly Processes of Gastropod Community Change with Horizontal and Vertical Zonation in Ancient Lake Ohrid: a Metacommunity Speciation Perspective. Biogeosciences 13, 2901–2911. doi:10.5194/bg-13-2901-2016
Hotez, P. J., Molyneux, D. H., Fenwick, A., Kumaresan, J., Sachs, S. E., Sachs, J. D., et al. (2007). Control of Neglected Tropical Diseases. N. Engl. J. Med. 357 (10), 1018–1027. doi:10.1056/NEJMra064142
Hoverman, J. T., Davis, C. J., Werner, E. E., Skelly, D. K., Relyea, R. A., and Yurewicz, K. L. (2011). Environmental Gradients and the Structure of Freshwater Snail Communities. Ecography 34 (6), 1049–1058. doi:10.1111/j.1600-0587.2011.06856.x
Huang, B. F. F., and Boutros, P. C. (2016). The Parameter Sensitivity of Random Forests. BMC Bioinformatics 17 (1), 1–13. doi:10.1186/s12859-016-1228-x
John, R., Ezekiel, M., Philbert, C., and Andrew, A. (2008). Schistosomiasis Transmission at High Altitude Crater Lakes in Western Uganda. BMC Infect. Dis. 8 (1), 1–6. doi:10.1186/1471-2334-8-110
Kabatereine, N. B., Brooker, S., Tukahebwa, E. M., Kazibwe, F., and Onapa, A. W. (2004). Epidemiology and Geography of Schistosoma Mansoni in Uganda: Implications for Planning Control. Trop. Med. Int. Health 9 (3), 372–380. doi:10.1046/j.1365-3156.2003.01176.x
Kabatereine, N. B., Tukahebwa, E., Kazibwe, F., Namwangye, H., Zaramba, S., Brooker, S., et al. (2006). Progress towards Countrywide Control of Schistosomiasis and Soil-Transmitted Helminthiasis in Uganda. Trans. R. Soc. Trop. Med. Hyg. 100 (3), 208–215. doi:10.1016/j.trstmh.2005.03.015
Kalinda, C., Chimbari, M. J., and Mukaratirwa, S. (2017). Effect of Temperature on the Bulinus globosus - Schistosoma haematobium System. Infect. Dis. Poverty 6 (1), 1–7. doi:10.1186/s40249-017-0260-z
Kappes, H., and Haase, P. (2012). Slow, but Steady: Dispersal of Freshwater Molluscs. Aquat. Sci. 74 (1), 1–14. doi:10.1007/s00027-011-0187-6
Kappes, H., Tackenberg, O., and Haase, P. (2014). Differences in Dispersal- and Colonization-Related Traits between Taxa from the Freshwater and the Terrestrial Realm. Aquat. Ecol. 48 (1), 73–83. doi:10.1007/s10452-013-9467-7
King, C. H., and Bertsch, D. (2015). Historical Perspective: Snail Control to Prevent Schistosomiasis. PLOS Negl. Trop. Dis. 9 (4), e0003657. doi:10.1371/journal.pntd.0003657
King, C. H., Yoon, N., Wang, X., Lo, N. C., Alsallaq, R., Ndeffo-Mbah, M., et al. (2020). Application of Schistosomiasis Consortium for Operational Research and Evaluation Study Findings to Refine Predictive Modeling of Schistosoma mansoni and Schistosoma haematobium Control in Sub-Saharan Africa. J. Trop. Med. Hyg. 103 (1 Suppl. l), 97–104. doi:10.4269/ajtmh.19-0852
King, C. H. (2010). Parasites and Poverty: the Case of Schistosomiasis. Acta Tropica 113, 95–104. doi:10.1016/j.actatropica.2009.11.012
Lachish, T., Tandlich, M., Grossman, T., and Schwartz, E. (2013). High Rate of Schistosomiasis in Travelers after a Brief Exposure to the High-Altitude Nyinambuga Crater lake, Uganda. Clin. Infect. Dis. 57 (10), 1461–1464. doi:10.1093/cid/cit559
Liaw, A., and Wiener, M. (2002). Classification and Regression by randomForest. R. News 2 (3), 18–22.
Liu, C., Ackerman, H. H., and Carulli, J. P. (2011). A Genome-wide Screen of Gene-Gene Interactions for Rheumatoid Arthritis Susceptibility. Hum. Genet. 129 (5), 473–485. doi:10.1007/s00439-010-0943-z
Loewenberg, S. (2014). Uganda's Struggle with Schistosomiasis. Lancet 383 (9930), 1707–1708. doi:10.1016/S0140-6736(14)60817-5
Madsen, H., Coulibaly, G., and Furu, P. (1987). Distribution of Freshwater Snails in the River Niger basin in Mali with Special Reference to the Intermediate Hosts of Schistosomes. Hydrobiologia 146 (1), 77–88. doi:10.1007/bf00007580
Maes, T., Hammoud, C., Volckaert, F. A. M., and Huyse, T. (2021). A Call for Standardised Snail Ecological Studies to Support Schistosomiasis Risk Assessment and Snail Control Efforts. Hydrobiologia 848 (8), 1773–1793. doi:10.1007/s10750-021-04547-4
Mahmoud, A., Fangary, H., Hussein, M., and Obuid-Allah, A. (2019). Population Dynamics of Freshwater Snails (Mollusca: Gastropoda) at Qena Governorate, Upper Egypt. Egypt. Acad. J. Biol. Sci. 3 (1), 11–22. doi:10.21608/eajbsz.2011.14309
Marcogliese, D. J. (2008). The Impact of Climate Change on the Parasites and Infectious Diseases of Aquatic Animals. Rev. Sci. Tech. 27 (2), 467–484. doi:10.20506/rst.27.2.1820
Marie, M. A. S., El-Deeb, F. A. A., Hasheesh, W. S., Mohamed, R. A., and Sayed, S. S. M. (2015). Impact of Seasonal Water Quality and Trophic Levels on the Distribution of Various Freshwater Snails in Four Egyptian Governorates. Appl. Ecol. Environ. Res. 3 (4), 117–126. doi:10.12691/aees-3-4-4
McCreesh, N., and Booth, M. (2013). Challenges in Predicting the Effects of Climate Change on Schistosoma mansoni and Schistosoma haematobium Transmission Potential. Trends Parasitol. 29 (11), 548–555. doi:10.1016/j.pt.2013.08.007
McCreesh, N., Arinaitwe, M., Arineitwe, W., Tukahebwa, E. M., and Booth, M. (2014). Effect of Water Temperature and Population Density on the Population Dynamics of Schistosoma Mansoni Intermediate Host Snails. Parasites Vectors 7 (1), 1–9. doi:10.1186/s13071-014-0503-9
Melack, J. M. (1978). Morphometric, Physical and Chemical Features of the Volcanic Crater Lakes of Western Uganda. Arch. Hydrobiol. 84, 430–453.
Mills, K., and Ryves, D. B. (2012). Diatom-based Models for Inferring Past Water Chemistry in Western Ugandan Crater Lakes. J. Paleolimnol. 48 (2), 383–399. doi:10.1007/s10933-012-9609-2
Nankabirwa, A., De Crop, W., Van der Meeren, T., Cocquyt, C., Plisnier, P.-D., Balirwa, J., et al. (2019). Phytoplankton Communities in the Crater Lakes of Western Uganda, and Their Indicator Species in Relation to lake Trophic Status. Ecol. Indicators 107, 105563. doi:10.1016/j.ecolind.2019.105563
Nyström Sandman, A., Wikström, S. A., Blomqvist, M., Kautsky, H., and Isaeus, M. (2013). Scale-dependent Influence of Environmental Variables on Species Distribution: a Case Study on Five Coastal Benthic Species in the Baltic Sea. Ecography 36 (3), 354–363. doi:10.1111/j.1600-0587.2012.07053.x
Olkeba, B. K., Boets, P., Mereta, S. T., Yeshigeta, M., Akessa, G. M., Ambelu, A., et al. (2020). Environmental and Biotic Factors Affecting Freshwater Snail Intermediate Hosts in the Ethiopian Rift Valley Region. Parasites Vectors 13, 1–13. doi:10.1186/s13071-020-04163-6
Pang, B., Yue, J., Zhao, G., and Xu, Z. (2017). Statistical Downscaling of Temperature with the Random forest Model. Adv. Meteorology 2017, 1–11. doi:10.1155/2017/7265178
Paull, S. H., and Johnson, P. T. J. (2011). High Temperature Enhances Host Pathology in a Snail-Trematode System: Possible Consequences of Climate Change for the Emergence of Disease. Freshw. Biol. 56 (4), 767–778. doi:10.1111/j.1365-2427.2010.02547.x
Payne, L., and Fitchett, J. R. (2010). Bringing Neglected Tropical Diseases into the Spotlight. Trends Parasitol. 26 (9), 421–423. doi:10.1016/j.pt.2010.06.002
Pedersen, U. B., Midzi, N., Mduluza, T., Soko, W., Stensgaard, A.-S., Vennervald, B. J., et al. (2014). Modelling Spatial Distribution of Snails Transmitting Parasitic Worms with Importance to Human and Animal Health and Analysis of Distributional Changes in Relation to Climate. Geospat. Health 8, 335–343. doi:10.4081/gh.2014.23
Prah, S. K., and James, C. (1978). The Influence of Physical Factors on the Behaviour and Infectivity of Miracidia of Schistosoma mansoni and S. haematobium II. Effect of Light and Depth. J. Helminthol. 52 (2), 115–120. doi:10.1017/S0022149X00005228
R Core Team (2020). R: A Language and Environment for Statistical Computing. Version 4.0.3. Vienna: R Foundation for Statistical Computing. Available at: http://www.R-project.org.
Rollinson, D., Knopp, S., Levitz, S., Stothard, J. R., Tchuem Tchuenté, L.-A., Garba, A., et al. (2013). Time to Set the Agenda for Schistosomiasis Elimination. Acta Tropica 128 (2), 423–440. doi:10.1016/j.actatropica.2012.04.013
Rowel, C., Fred, B., Betson, M., Sousa-Figueiredo, J. C., Kabatereine, N. B., and Stothard, J. R. (20152015). Environmental Epidemiology of Intestinal Schistosomiasis in Uganda: Population Dynamics of Biomphalaria (Gastropoda: Planorbidae) in Lake Albert and Lake Victoria with Observations on Natural Infections with Digenetic Trematodes. Biomed. Res. Int. 2015, 1–11. doi:10.1155/2015/717261
Ruiz-Aĺvarez, M., Gomariz-Castillo, F., and Alonso-Sarría, F. (2021). Evapotranspiration Response to Climate Change in Semi-arid Areas: Using Random forest as Multi-Model Ensemble Method. Water 13 (2), 222. doi:10.3390/w13020222
Rumes, B., Eggermont, H., and Verschuren, D. (2011). Distribution and Faunal Richness of Cladocera in Western Uganda Crater Lakes. Hydrobiologia 676 (1), 39–56. doi:10.1007/s10750-011-0829-7
Saulnier-Talbot, É., and Lavoie, I. (2018). Uncharted Waters: the Rise of Human-Made Aquatic Environments in the Age of the "Anthropocene". Anthropocene 23, 29–42. doi:10.1016/j.ancene.2018.07.003
Schonlau, M., and Zou, R. Y. (2020). The Random forest Algorithm for Statistical Learning. Stata J. 20 (1), 3–29. doi:10.1177/1536867X20909688
Schumann, A., Muwanga, A., Lehto, T., Staudt, M., Schlüter, T., Kato, V., et al. (2015). Ugandan Geosites. Geology. Today 31 (2), 59–67. doi:10.1111/gto.12089
Shiff, C. (2017). Why Reinvent the Wheel? Lessons in Schistosomiasis Control from the Past. PLOS Negl. Trop. Dis. 11 (10), e0005812. doi:10.1371/journal.pntd.0005812
Sokolow, S. H., Wood, C. L., Jones, I. J., Swartz, S. J., Lopez, M., Hsieh, M. H., et al. (2016). Global Assessment of Schistosomiasis Control over the Past century Shows Targeting the Snail Intermediate Host Works Best. PLOS Negl. Trop. Dis. 10 (7), e0004794. doi:10.1371/journal.pntd.0004794
Standley, C. J., Dobson, A. P., and Stothard, J. R. (2012). “Out of Animals and Back Again: Schistosomiasis as a Zoonosis in Africa,” in Schistosomiasis (London: Intech), 209–230.
Stanton, M. C., Adriko, M., Arinaitwe, M., Howell, A., Davies, J., Allison, G., et al. (2017). Intestinal Schistosomiasis in Uganda at High Altitude (>1400 M): Malacological and Epidemiological Surveys on Mount Elgon and in Fort Portal Crater Lakes Reveal Extra Preventive Chemotherapy Needs. Infect. Dis. Poverty 6 (1), 34. doi:10.1186/s40249-017-0248-8
Steinmann, P., Keiser, J., Bos, R., Tanner, M., and Utzinger, J. (2006). Schistosomiasis and Water Resources Development: Systematic Review, Meta-Analysis, and Estimates of People at Risk. Lancet Infect. Dis. 6 (7), 411–425. doi:10.1016/S1473-3099(06)70521-7
Stensgaard, A.-S., Utzinger, J., Vounatsou, P., Hürlimann, E., Schur, N., Saarnak, C. F. L., et al. (2013). Large-scale Determinants of Intestinal Schistosomiasis and Intermediate Host Snail Distribution across Africa: Does Climate Matter? Acta Tropica 128 (2), 378–390. doi:10.1016/j.actatropica.2011.11.010
Stensgaard, A.-S., Vounatsou, P., Sengupta, M. E., and Utzinger, J. (2019). Schistosomes, Snails and Climate Change: Current Trends and Future Expectations. Acta Tropica 190, 257–268. doi:10.1016/j.actatropica.2018.09.013
Stothard, J. R., Mathieson, W., Webster, J. P., Fenwick, A., Tukahebwa, E. M., Kazibwe, F., et al. (2005). Field Evaluation of the Meade Readiview Handheld Microscope for Diagnosis of Intestinal Schistosomiasis in Ugandan School Children. J. Trop. Med. Hyg. 73 (5), 949–955. doi:10.4269/ajtmh.2005.73.949
Strobl, C., Boulesteix, A.-L., Zeileis, A., and Hothorn, T. (2007). Bias in Random forest Variable Importance Measures: Illustrations, Sources and a Solution. BMC Bioinformatics 8 (1), 1–21. doi:10.1186/1471-2105-8-25
Svanbäck, R., and Bolnick, D. I. (2007). Intraspecific Competition Drives Increased Resource Use Diversity within a Natural Population. Proc. R. Soc. B. 274 (1611), 839–844. doi:10.1098/rspb.2006.0198
Tchakonté, S., Ajeagah, G. A., Diomandé, D., Camara, A. I., and Ngassam, P. (2014). Diversity, Dynamic and Ecology of Freshwater Snails Related to Environmental Factors in Urban and Suburban Streams in Douala–Cameroon (Central Africa). Aquat. Ecol. 48 (4), 379–395. doi:10.1007/s10452-014-9491-2
Tumwebaze, I., Clewing, C., Dusabe, M. C., Tumusiime, J., Kagoro-Rugunda, G., Hammoud, C., et al. (2019). Molecular Identification of Bulinus spp. Intermediate Host Snails of Schistosoma spp. In Crater Lakes of Western Uganda with Implications for the Transmission of the Schistosoma haematobium Group Parasites. Parasites Vectors 12 (1), 1–23. doi:10.1186/s13071-019-3811-2
Viera, A. J., and Garrett, J. M. (2005). Understanding interobserver agreement: the kappa statistic. Fam med. 37 (5), 360–363.
Utzinger, J., and Tanner, M. (2000). Microhabitat Preferences of Biomphalaria Pfeifferi and Lymnaea Natalensis in a Natural and a Man-Made Habitat in southeastern Tanzania. Mem. Inst. Oswaldo Cruz 95, 287–294. doi:10.1590/s0074-02762000000300002
Utzinger, J., Raso, G., Brooker, S., De Savigny, D., Tanner, M., Ørnbjerg, N., et al. (2009). Schistosomiasis and Neglected Tropical Diseases: towards Integrated and Sustainable Control and a Word of Caution. Parasitology 136 (13), 1859–1874. doi:10.1017/s0031182009991600
Vinogradov, V. I. (1980). C13/C12 and O18/O16 Ratios and C14 Concentration in Carbonatites of the Kaliango Volcano (East Africa). Int. Geol. Rev. 22 (1), 51–57. doi:10.1080/00206818209466862
Walz, Y., Wegmann, M., Dech, S., Vounatsou, P., Poda, J.-N., N'Goran, E. K., et al. (2015). Modeling and Validation of Environmental Suitability for Schistosomiasis Transmission Using Remote Sensing. PLOS Negl. Trop. Dis. 9 (11), e0004217. doi:10.1371/journal.pntd.0004217
Wang, L., Utzinger, J., and Zhou, X.-N. (2008). Schistosomiasis Control: Experiences and Lessons from China. Lancet 372 (9652), 1793–1795. doi:10.1016/S0140-6736(08)61358-6
Warton, D. I., and Hui, F. K. C. (2011). The Arcsine is Asinine: the Analysis of Proportions in Ecology. Ecology 92 (1), 3–10. doi:10.1890/10-0340.1
Watson, J. M. (1958). Ecology and Distribution of Bulinus truncatus in the Middle East; with Comments on the Effect of Some Human Activities in Their Relationship to the Snail Host on the Incidence of Bilharziasis Haematobia in the Middle East and Africa. Bull. World Health Organ. 18 (5-6), 833–894.
World Health Organization (2012). Elimination of Schistosomiasis. Sixty-fifth World Health Assembly, WHA65. 21, Agenda item 13.11, 26 May 2012. Available at: http://apps.who.int/gb/ebwha/pdf_files/WHA65/A65_R21-en.pdf (Accessed July, , 2021).
World Health Organization (2016). Schistosomiasis. Available at: https://www.who.int/features/factfiles/schistosomiasis/en/(Accessed July, 2021).
Xia, C., Hu, Y., Ward, M. P., Lynn, H., Li, S., Zhang, J., et al. (2019). Identification of High-Risk Habitats of Oncomelania Hupensis, the Intermediate Host of Schistosoma Japonium in the Poyang Lake Region, China: A Spatial and Ecological Analysis. PLOS Negl. Trop. Dis. 13 (6), e0007386. doi:10.1371/journal.pntd.0007386
Yirenya-Tawiah, D., Abdul Rashid, A., Futagbi, G., Aboagye, I., and Dade, M. (2011). Prevalence of Snail Vectors of Schistosomiasis in the Kpong Head Pond, Ghana. West. Afr. J. Appl. Ecol. 18, 39–45. doi:10.4314/wajae.v18i1.70310
Keywords: schistosomisis, biotic and abiotic predictors, mollusks, random forest, Africa
Citation: Tabo Z, Neubauer TA, Tumwebaze I, Stelbrink B, Breuer L, Hammoud C and Albrecht C (2022) Factors Controlling the Distribution of Intermediate Host Snails of Schistosoma in Crater Lakes in Uganda: A Machine Learning Approach. Front. Environ. Sci. 10:871735. doi: 10.3389/fenvs.2022.871735
Received: 08 February 2022; Accepted: 15 March 2022;
Published: 14 April 2022.
Edited by:
Tatenda Dalu, University of Mpumalanga, South AfricaReviewed by:
Nelson A. F. Miranda, Nelson Mandela University, South AfricaRoss Cuthbert, Queen’s University Belfast, United Kingdom
Copyright © 2022 Tabo, Neubauer, Tumwebaze, Stelbrink, Breuer, Hammoud and Albrecht. This is an open-access article distributed under the terms of the Creative Commons Attribution License (CC BY). The use, distribution or reproduction in other forums is permitted, provided the original author(s) and the copyright owner(s) are credited and that the original publication in this journal is cited, in accordance with accepted academic practice. No use, distribution or reproduction is permitted which does not comply with these terms.
*Correspondence: Zadoki Tabo, tabozac@gmail.com
†Present Address: Björn Stelbrink, Museum für Naturkunde ‐ Leibniz Institute for Evolution and Biodiversity Science, Berlin, Germany