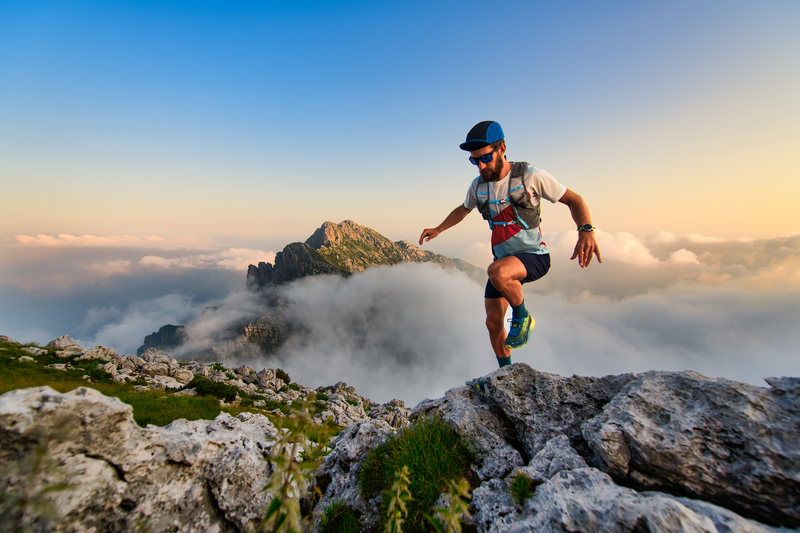
95% of researchers rate our articles as excellent or good
Learn more about the work of our research integrity team to safeguard the quality of each article we publish.
Find out more
ORIGINAL RESEARCH article
Front. Environ. Sci. , 25 May 2022
Sec. Environmental Economics and Management
Volume 10 - 2022 | https://doi.org/10.3389/fenvs.2022.868740
This study aims to demonstrate the impact of economic growth and energy consumption on environmental degradation in China, the top country that produced the highest carbon dioxide (CO2) emissions, by considering that environmental degradation is one of the extreme challenges that the world and China have been facing. Parallel to this aim, this study uses dynamic ARDL (DYNARDL) simulations to investigate the long-run and short-run cointegration amongst the selected parameters from 1979 to 2019. The results of the long-run and short-run simulations illustrate that 1) economic growth increases environmental degradation; 2) growth in energy consumption worsens the environmental degradation situation; 3) urbanization improves the environmental situation in the long run, whereas growth in urban population increases CO2 emissions in the short-run. The research argues that improved energy production and management should be included in economic policy planning and the government should invest more in renewable energy to prevent environmental degradation.
One of the most important issues affecting the modern world is environmental degradation (Li et al., 2021; Liu et al., 2021). This is because it has negative consequences for human health, biodiversity, the ozone layer, quality of air, natural resources (e.g., water, soil, and forest), and the overall economy (Rehman et al., 2021b). High CO2 emissions have been influencing both developed and developing countries throughout the world. CO2 emissions are one of the main factors that cause environmental degradation (Adebayo et al., 2021; Satrovic et al., 2021). Despite international organizations’ efforts to mitigate its negative impact on the environment and formulate measures to reduce CO2 emissions, still global energy-related
Energy consumption, particularly from oil, gas, and coal sources, is the primary cause of
Environmental degradation is generally caused by several factors. Human-related factors like energy consumption and economic growth are among the leading causes of environmental degradation (Guo et al., 2022b). Energy consumption is a vital component of economic growth in most developing countries since it supports a wide range of economic activities (Nathaniel and Bekun, 2021). Although energy consumption, overall, stimulates economic growth (Koengkan and Fuinhas, 2020), the type of energy resource utilized determines the environmental quality (Shahzad et al., 2021).
The cointegration between economic growth and environmental quality was deeply examined under the conceptual framework of the Environmental Kuznets Curve (EKC) hypothesis (Kuznets, 1955; Grossman and Krueger, 1995), which states that a country may boost environmental degradation with economic growth, but that as economic growth increases, the level of environmental degradation decreases (Rothman, 1998). The EKC implies an inverted U-shaped nexus between economic growth and pollution (Kuznets, 1955). Some studies support the existence of EKC (Ali et al., 2020; Ulucak et al., 2020), whereas others do not confirm EKC (Rahman et al., 2020; Pata and Isik, 2021). This ambiguity in the literature comes from factors such as the selection of countries, period, and difference of parameters in the model, selection of quadratic or cubic EKC model, socioeconomic characteristics of the examined country, and selection of econometric methodologies. Even in some cases when the EKC is evaluated for the same country, different findings are obtained (Mehmood and Tariq, 2020).
CO2 emissions have caught the interest of researchers, with evidence indicating that energy consumption (Nurgazina et al., 2021), population (Dong et al., 2018), human capital (Bano et al., 2018), urbanization (Wang et al., 2016), financial development (Khan et al., 2022), research and development (Danish et al., 2018), trade openness (Kwakwa et al., 2018), use of natural resources (Umar et al., 2020), and globalization (Pata, 2021) among other factors, are important determinants of CO2 emissions.
Several studies (Liu et al., 2020; Nathaniel et al., 2020) discover that economic growth, use of the natural resource, urbanization, and globalization are responsible for the increase in
FDI inflows are the most important accelerator of economic growth because they facilitate the transfer of capital and technology to developing countries (Murshed, 2021). Moreover, FDI inflows assist the host economy in obtaining the advantages of the latest technology, management, and communication systems, resulting in increased output and economies of scale inside the country. FDI, on the other hand, has the potential to harm the environment (Canh et al., 2020). The results of the nexus between FDI and
This study aims to observe the impact of economic growth and energy consumption in the presence of financial development, urbanization, and FDI inflow for China. According to 2019 data from the Global Carbon Project, China produced the most
The main originality of the study is that any study applies the DYNARDL simulations to examine the China case by including the factors in this study and using data between 1979 and 2019. This research contributes to clarifying the impacts of energy consumption, economic growth, financial development, FDI, and urbanization on CO2 emissions in the literature. This study is critical because China intends to achieve carbon neutrality by 2060. Furthermore, the goal of this study is to fill a substantial knowledge gap, notably in China, and to assist policymakers in developing policies to achieve carbon neutrality in the next 40 years.
After the introduction part, the second part reviews the literature. The third part explains the data and methodology. The fourth part presents the empirical results and discussion. Finally, the fifth part concludes and tells about policy implications.
Numerous researchers in various countries or regions have observed the nexus between economic growth and the environment. The results differ based on the size of the sample and the studied period (Koengkan et al., 2019a; Chishti et al., 2021; Qin et al., 2021). A large number of researchers have used the EKC hypothesis to study the nexus between economic growth and environmental quality (Yilanci and Pata, 2020). The validity of the theory is proved in various countries like the US (Atasoy, 2017), Pakistan (Rehman et al., 2021a), Malaysia (Nurgazina et al., 2021), China (Pata and Caglar, 2021), OECD (Cao et al., 2022).
On the other hand, some studies cannot find the nexus between economic growth and environmental degradation. For instance, Zambrano-Monserrate et al. (2018) analyze the nexus in Peru and find that the results do not support the EKC hypothesis. Another research on South Korea by Koc and Bulus (2020) discovers evidence of an N-shaped link between economic growth and environmental degradation that invalidates the EKC theory. The EKC hypothesis is also invalid in Pakistan according to the findings of Ahmed et al. (2020), where an increase in wealth boosts
The EKC theory, on the other hand, has been supported by multiple studies. For example, Katrakilidis et al. (2016) indicated a positive nexus between economic growth and environmental degradation in Greece. Rauf et al. (2018) revealed in research on the Belt and Road Initiative (BRI) countries that the EKC hypothesis fits all regional panels of the BRI countries. Furthermore, Işık et al. (2019) confirmed the EKC theory in the context of ten states across the United States and concluded that economic growth first increased
A variety of studies have examined the nexus between energy usage and environmental degradation, especially
Omri (2013) establishes the presence of positive unidirectional causation between energy consumption and environmental degradation in 14 MENA countries. Furthermore, Ahmed et al. (2017), Aye and Edoja (2017), and Musah et al. (2021) discover energy consumption to be a key booster of
On the other hand, Al-Mulali et al. (2015) show that energy usage does not influence
Despite the importance of the topic, there is still a research gap, because numerous studies (Magazzino et al., 2020b; Koengkan and Fuinhas, 2021a; Guo et al., 2022a) in the energy and environmental economics literature apply panel data and time-series analyses to investigate the long-run short-run relation between different variables, however, this research applies the DYNARDL simulations model established by Jordan and Philips (2018) in terms of carbon neutrality in China. This study uses the DYNARDL model to analyze the actual change in the dependent variable in the long and short term by introducing 100% negative shock from explanatory variables. The DYNARDL simulations have the capacity to solve the data’s existence issues and interpret the results of the standard ARDL model. While the remaining variables are kept constant, the DYNARDL simulations will approximate and reflect the predictions of an actual change in the independent variable (Jordan and Philips, 2018).
To study the essential variables’ influence on environmental degradation, the study contributes to the literature by including energy consumption, economic growth, financial development, FDI, and urbanization in the CO2 emission equation. Furthermore, no other researcher has conducted similar research for the China case by using the same factors and for the same period. Therefore, this study can be evaluated as pioneering and significant. Also, this study contributes to the existing literature by providing a clear route for scholars to understand the nexus between selected factors. Moreover, this study assists policymakers of China in developing and implementing strategies to reduce CO2 emissions to meet the carbon neutrality objective by 2060.
This study analyses the time series annual data set from 1979 to 2019 for China. Summary statistics are presented in Table 1. CO2 emissions (metric tons per capita) are used as a reference for the environmental degradation in this study. Furthermore, the study employs GDP per capita (constant 2010 US dollars) for the economic growth (EG), energy consumption (EC) is measured in kilograms of oil equivalent per capita, domestic credit to the private sector (% of GDP) represent financial development (FD), urbanization (UR) is measured in % of the total population, FDI shows the net foreign direct investment inflow (% of GDP). The data for the independent and dependent variables are obtained from the World Bank (WorldBank, 2021).
There are five stages for the evaluation of the impact of economic growth and energy consumption in the context of financial development, urbanization, and FDI inflow for China as indicated in Figure 1.
In the first step, PP and ADF tests are used to identify the order of variable integration. In the second step, the PSS bound test is performed to confirm the presence of long-run cointegration among the variables. In the third step, the ARDL model is employed to identify the short-run and long-run associations between variables. In the fourth step, DYNARDL simulations are estimated. In the fifth step, Kernel-based regularized least squares (KRLS) are utilized to identify the causal association between the variables.
The environmental degradation function for this study is expressed as:
where,
where,
where,
where
The study also performs DYNARDL simulations by Jordan and Philips (2018) to evaluate the counterfactual shock of one factor whereas the others are kept fixed on the dependent variable. Because of the dynamic nature of the data, the model simulation is qualified to assess the impact of positive or negative changes on the independent variables (Sarkodie and Owusu, 2020). The DYARDL model fits an ARDL model in error-correction form and is presented in Eq. 5.
The DYNARDL model is based on -100%
Further, the nexus is investigated using KRLS which employs the pointwise derivatives (Hainmueller and Hazlett, 2014). By eliminating parametric assumptions and enabling a flexible hypothesis, the KRLS model exceeds classic regression analysis and classification issues (Hainmueller and Hazlett, 2014; Ferwerda et al., 2015). As a result, the KRLS model makes it possible to detect potential nonlinearities, interactions, and heterogeneous effects that result in detailed interpretations (Hainmueller and Hazlett, 2014; Hipp et al., 2017).
Preliminary estimates, including the unit root test, are essential to assess the data’s stationarity status to avoid inaccurate findings during analysis. The variables in the ARDL model must be stationary at level I (0), first difference I (1), or a mix of both (Pesaran et al., 2001). The DYNARDL simulations, on the other hand, necessitate the dependent parameter’s rigorous first difference stationarity (Jordan and Philips, 2018). The independent variables can be integrated at either level I (0) or first difference I (1), but not higher than I (1).
After satisfying the criteria of rigorous first difference stationarity of the dependent variable, the optimal lag for the proposed model is defined. The cointegration is evaluated using the Pesaran et al. (2001) bounds test with novel Kripfganz and Schneider (2020) critical values and approximate p-values using the optimal lag.
The stability of the models is verified by analysis for serial correlation, normality, heteroscedasticity, and structural breaks. To assess for autocorrelation in the estimated model’s residuals, the Breusch-Godfrey LM test is employed. Cameron and Trivedi’s decomposition of the IM-test is used to determine residual heteroskedasticity. Skewness/Kurtosis tests are used to determine the independence of residuals. Additionally, both standardized normal probability plots and quantiles of residuals against quantiles of normal distribution estimates support the existence of a normal distribution. Using the cumulative sum test for the stability of variables, possible structural breaks are evaluated.
To establish the order of integration and produce comprehensive results, the unit root tests are employed for checking the characteristics of the parameters by utilizing PP and ADF tests (Dickey and Fuller, 1981; Perron, 1989). The findings of the unit root tests are shown in Table 2.
According to the test results, the ARDL and DYNARDL models can be utilized with the studied variables because the dependent variable is integrated at the I (1) and independent parameters are stationary and integrated at the order I (0) and I (1).
The lag range (LR) test, final perdition error (FPE), Akaike Information Criteria (AIC), Hannan-Quinn Information Criteria (HQIC), and Schwarz’s Bayesian information criterion (SBIC) is used to select the optimal lag for further analysis. The results reveal that the suitable lag is lag 2 (Appendix 1).
The results of the ARDL (1, 2, 0, 1, 1, 2) regression are presented in Figure 2 with its empirical results presented in Table 3.
FIGURE 2. Parameter estimates of the ARDL model. Notes: the estimate in a log-log model is shown by the blue (•), the reference line is represented by the brown teal dash-dot, and the marron-spike represents the lower and upper 95% confidence limit, respectively.
The result of the analysis discloses that economic growth and energy consumption are found to increase environmental degradation in both short-run and long-run analyses. This output aligns with the studies of Fuinhas et al. (2017), Fuinhas et al. (2021), Nawaz et al. (2021), who find the same nexus between energy consumption and
Furthermore, the error correction term (ECT) is negative and significant at less than 5%, indicating that the adjustment speed to the long-run equilibrium will take more than 5 years. Moreover, the
To examine the long-run cointegration relationship, the ARDL bounds cointegration test (Pesaran et al., 2001) is evaluated by utilizing the novel Kripfganz and Schneider (2020) critical values and approximate p-values. The bounds test’s results are shown in Table 4.
Table 4 illustrates that the F-statistic value of the proposed model is higher than the critical value of the upper bound (4.54) at a 1% level of significance thus there are long-term relationships among variables. Several tests checking autocorrelation, heteroskedasticity normality, and structural breaks are done to ensure the stability of the ARDL model. It can be observed from the result of the Breusch Godfrey LM test that the hypothesis of no serial correlation among variables is accepted at a 5% significance level, which means that residuals are free of serial correlation. Cameron and Trivedi’s decomposition of the IM-test shows that the homoscedasticity null hypothesis is accepted at a 5% level of significance, thus the residuals are free from heteroscedasticity. The Skewness/Kurtosis normality tests disclose that the residuals are normally distributed within the mean.
A standardized normal probability plot (Figure 3A) and quantiles of residuals against quantiles of normal distribution (Figure 3B) are used to further examine the validity of the normality assumption determined by the Skewness/Kurtosis tests.
FIGURE 3. (A) Standardized normal probability plot. (B) Quantiles of residuals against quantiles of normal distribution.
The residuals based on the ARDL model are normally distributed in both Figures 3A,B. Furthermore, the cumulative sum test is used to analyze potential structural breaks that are presented in Figure 4.
Figure 4 shows that the assessed t-statistic is within the 95% confidence interval which means the calculated coefficients are stable through the years.
Figure 5 shows the variable graph of the DYNARDL, while Table 5 shows its empirical estimation.
FIGURE 5. Parameter estimates of DYNARDL simulations. Notes: the estimate in a log-log model is shown by the blue (•), the reference line is represented by the brown teal dash-dot, and the marron-spike represents the lower and upper 95% confidence limit, respectively.
The results of the long and short-run simulations illustrate that economic growth will increase
Moreover, the estimated ECT of -0.484, which is significant at a 5% level indicates the long-run cointegration between economic growth, energy consumption, financial development, urbanization, FDI inflow, and environmental degradation. Moreover, the
In general, both the ARDL and DYNARDL estimates suggest that China’s economic development and energy consumption have a detrimental impact on the environment. The DYNARDL simulation is based on carbon neutrality by 2060 (Mallapaty, 2020; Hepburn et al., 2021; Ren et al., 2021). The simulation results are presented in Figure 6.
FIGURE 6. Representation of counterfactual shock in forecast variables employing the DYNARDL model: (A) economic growth: (B) energy consumption. Note: dark navy dot (•) represent the forecast emissions by −100% shocks in a log-log model; navy teal, bright blue, and light-blue spikes show 75%, 90%, and 95% confidence bands.
The plots presented in Figure 6 expose that -100% of shocks in the estimated economic growth do not affect environmental degradation, while the same shocks in the calculated energy consumption boost
A machine learning KRLS approach is employed to check and identify the relationships among the variables to additional enhance the results of this study and the results are presented in Table 6.
Table 6 shows that the general model’s predictive power is 0.997 meaning that descriptive factors explain 99.7% of the variation in
FIGURE 7. Representation of Pointwise marginal effect: (A) of economic growth; (B) of energy consumption.
The marginal impact of economic growth and energy consumption on environmental degradation represented in Figure 6 shows that the increasing level of economic growth and energy consumption raise
This study examines the impact of economic growth and energy consumption on the
Our findings have far-reaching ramifications. Firstly, to address environmental issues, the monitoring and control of carbon emissions should be strengthened, and multiple solutions, such as accelerating economic reconstruction, reducing fossil energy consumption, and encouraging environment-friendly energy consumption, should be designed to address carbon emissions and the resulting problems. In addition, renewable energy should be used to minimize dependency on insecure energy infrastructure and maintain energy security in specific high-energy-consumption industries such as manufacturing, transportation, housing, and others. Furthermore, authorities must establish tax exemptions for renewable energy so that companies may quickly switch from fossil fuel to renewable energy.
Secondly, to slow climate change and reduce the adverse effects of carbon emissions on China’s economy, the government should rigorously implement the low carbon emission reduction policy and speed up the economic transition to an environmentally friendly growth pattern.
Moreover, to support the environment, the government must focus on increasing the environmental effect of ecological innovation. As a result, authorities should make a concerted effort to encourage environmental innovations to promote green policies. Environmental and social challenges must be addressed while encouraging long-term economic growth via green innovation and technology policy. Setting standards to identify environmental requirements for technology that might enhance environmental quality is also essential. Environmental innovation creates a platform that allows businesses to exchange innovative technologies and benefits while also encouraging cooperation.
Even though this study determines a link between energy consumption, economic growth, FDI, urbanization, and CO2 emissions, it also has several shortcomings. The findings of this study also indicate that more investigation using various statistical models is required. This research only focused on energy consumption and economic growth, leaving out renewable and nonrenewable energy consumption as well as green technologies. As a result, it is important to include these variables in future studies because renewable energy consumption and green technologies can assist to cut carbon emissions and attain carbon neutrality.
The original contributions presented in the study are included in the article/Supplementary Materials, further inquiries can be directed to the corresponding author.
ZN: Conceptualization, Data curation, Formal analysis, Methodology, Writing—original draft; QG: Funding acquisition, Project administration, Supervision; UA: Writing—review and editing; MTK: Writing—review and editing; AU: Writing—review and editing; ZAK: Writing—review and editing.
This work was supported by Hainan Provincial Natural Science Foundation of China (grant number: 722RC637) and the Research Startup Fund of Hainan University (grant number: kyqdsk201903).
The authors declare that the research was conducted in the absence of any commercial or financial relationships that could be construed as a potential conflict of interest.
All claims expressed in this article are solely those of the authors and do not necessarily represent those of their affiliated organizations, or those of the publisher, the editors and the reviewers. Any product that may be evaluated in this article, or claim that may be made by its manufacturer, is not guaranteed or endorsed by the publisher.
Lag Length Selection Criteria
Adebayo, T. S., and Akinsola, G. D. (2021). Investigating the Causal Linkage Among Economic Growth, Energy Consumption and CO 2 Emissions in Thailand: An Application of the Wavelet Coherence Approach. Int. J. Renew. Energy Dev. 10 (1), 17–26. doi:10.14710/ijred.2021.32233
Adebayo, T. S., Udemba, E. N., Ahmed, Z., and Kirikkaleli, D. (2021). Determinants of Consumption-Based Carbon Emissions in Chile: an Application of Non-linear ARDL. Environ. Sci. Pollut. Res. 28, 1–15. doi:10.1007/s11356-021-13830-9
Adebayo, T. S. (2021). Testing the EKC Hypothesis in Indonesia: Empirical Evidence from the ARDL-Based Bounds and Wavelet Coherence Approaches. Appl. Econ. J. 28 (1), 78–100. doi:10.1007/s11356-020-11442-3
Ahmad, M., Khattak, S. I., Khan, A., and Rahman, Z. U. (2020). Innovation, Foreign Direct Investment (FDI), and the Energy-Pollution-Growth Nexus in OECD Region: a Simultaneous Equation Modeling Approach. Environ. Ecol. Stat. 27 (2), 203–232. doi:10.1007/s10651-020-00442-8
Ahmad, M., Ahmed, Z., Majeed, A., and Huang, B. (2021). An Environmental Impact Assessment of Economic Complexity and Energy Consumption: Does Institutional Quality Make a Difference? Environ. Impact Assess. Rev. 89, 106603. doi:10.1016/j.eiar.2021.106603
Ahmed, K., Rehman, M. U., and Ozturk, I. (2017). What Drives Carbon Dioxide Emissions in the Long-Run? Evidence from Selected South Asian Countries. Renew. Sustain. Energy Rev. 70, 1142–1153. doi:10.1016/j.rser.2016.12.018
Ahmed, Z., Zafar, M. W., and Mansoor, S. (2020). Analyzing the Linkage between Military Spending, Economic Growth, and Ecological Footprint in Pakistan: Evidence from Cointegration and Bootstrap Causality. Environ. Sci. Pollut. Res. 27 (33), 41551–41567. doi:10.1007/s11356-020-10076-9
Ali, H. S., Nathaniel, S. P., Uzuner, G., Bekun, F. V., and Sarkodie, S. A. (2020). Trivariate Modelling of the Nexus between Electricity Consumption, Urbanization and Economic Growth in Nigeria: Fresh Insights from Maki Cointegration and Causality Tests. Heliyon 6 (2), e03400. doi:10.1016/j.heliyon.2020.e03400
Ali, M. U., Gong, Z., Ali, M. U., Wu, X., and Yao, C. (2021). Fossil Energy Consumption, Economic Development, Inward FDI Impact on CO2 Emissions in Pakistan: Testing EKC Hypothesis through ARDL Model. Int. J. Fin. Econ. 26 (3), 3210–3221. doi:10.1002/ijfe.1958
Al-Mulali, U., Tang, C. F., and Ozturk, I. (2015). Estimating the Environment Kuznets Curve Hypothesis: Evidence from Latin America and the Caribbean Countries. Renew. Sustain. Energy Rev. 50, 918–924. doi:10.1016/j.rser.2015.05.017
Anwar, A., Younis, M., and Ullah, I. (2020). Impact of Urbanization and Economic Growth on CO2 Emission: A Case of Far East Asian Countries. Int. J. Environ. Res. Public Health 17 (7), 2531. doi:10.3390/ijerph17072531
Atasoy, B. S. (2017). Testing the Environmental Kuznets Curve Hypothesis across the U.S.: Evidence from Panel Mean Group Estimators. Renew. Sustain. Energy Rev. 77, 731–747. doi:10.1016/j.rser.2017.04.050
Aye, G. C., and Edoja, P. E. (2017). Effect of Economic Growth on CO2 Emission in Developing Countries: Evidence from a Dynamic Panel Threshold Model. Cogent Econ. Finance 5 (1), 1379239. doi:10.1080/23322039.2017.1379239
Bano, S., Zhao, Y., Ahmad, A., Wang, S., and Liu, Y. (2018). Identifying the Impacts of Human Capital on Carbon Emissions in Pakistan. J. Clean. Prod. 183, 1082–1092. doi:10.1016/j.jclepro.2018.02.008
Bashir, M. F., Ma, B. J., Bilal, B., Komal, B., Bashir, M. A., Farooq, T. H., et al. (2020). Correlation between Environmental Pollution Indicators and COVID-19 Pandemic: A Brief Study in Californian Context. Environ. Res. 187, 109652. doi:10.1016/j.envres.2020.109652
Bildirici, M., and Gokmenoglu, S. M. (2020). The Impact of Terrorism and FDI on Environmental Pollution: Evidence from Afghanistan, Iraq, Nigeria, Pakistan, Philippines, Syria, Somalia, Thailand and Yemen. Environ. Impact Assess. Rev. 81, 106340. doi:10.1016/j.eiar.2019.106340
Can, M., Ahmed, Z., Mercan, M., and Kalugina, O. A. (2021). The Role of Trading Environment-Friendly Goods in Environmental Sustainability: Does Green Openness Matter for OECD Countries? J. Environ. Manag. 295, 113038. doi:10.1016/j.jenvman.2021.113038
Canh, N. P., Binh, N. T., Thanh, S. D., and Schinckus, C. (2020). Determinants of Foreign Direct Investment Inflows: The Role of Economic Policy Uncertainty. Int. Econ. 161, 159–172. doi:10.1016/j.inteco.2019.11.012
Cao, H., Khan, M. K., Rehman, A., Dagar, V., Oryani, B., and Tanveer, A. (2022). Impact of Globalization, Institutional Quality, Economic Growth, Electricity and Renewable Energy Consumption on Carbon Dioxide Emission in OECD Countries. Environ. Sci. Pollut. Res. 29 (16), 24191–24202. doi:10.1007/s11356-021-17076-3
Chishti, M. Z., Ahmed, Z., Murshed, M., Namkambe, H. H., and Ulucak, R. (2021). The Asymmetric Associations between Foreign Direct Investment Inflows, Terrorism, CO2 Emissions, and Economic Growth: A Tale of Two Shocks. Environ. Sci. Pollut. Res. 28, 1–19. doi:10.1007/s11356-021-15188-4
Chopra, R., Magazzino, C., Shah, M. I., Sharma, G. D., Rao, A., and Shahzad, U. (2022). The Role of Renewable Energy and Natural Resources for Sustainable Agriculture in ASEAN Countries: Do Carbon Emissions and Deforestation Affect Agriculture Productivity? Resour. Policy 76, 102578. doi:10.1016/j.resourpol.2022.102578
Danish, , , Khan, N., Baloch, M. A., Saud, S., and Fatima, T. (2018). The Effect of ICT on CO2 Emissions in Emerging Economies: Does the Level of Income Matters? Environ. Sci. Pollut. Res. Int. 25 (23), 22850–22860. doi:10.1007/s11356-018-2379-2
Dickey, D. A., and Fuller, W. A. (1981). Likelihood Ratio Statistics for Autoregressive Time Series with a Unit Root. Econometrica 49, 1057–1072. doi:10.2307/1912517
Dong, K., Hochman, G., Zhang, Y., Sun, R., Li, H., and Liao, H. (2018). CO2 Emissions, Economic and Population Growth, and Renewable Energy: Empirical Evidence across Regions. Energy Econ. 75, 180–192. doi:10.1016/j.eneco.2018.08.017
Fan, F., and Zhang, X. (2021). Transformation Effect of Resource-Based Cities Based on PSM-DID Model: An Empirical Analysis from China. Environ. Impact Assess. Rev. 91, 106648. doi:10.1016/j.eiar.2021.106648
Fan, F., Lian, H., and Wang, S. (2020). Can Regional Collaborative Innovation Improve Innovation Efficiency? An Empirical Study of Chinese Cities. Growth Change 51 (1), 440–463. doi:10.1111/grow.12346
Ferwerda, J., Hainmueller, J., and Hazlett, C. (2015). KRLS: A Stata Package for Kernel-Based Regularized Least Squares. J. Stat. Softw. 79 (3), 1–26. doi:10.2139/ssrn.2325523
Fuinhas, J. A., Marques, A. C., and Koengkan, M. (2017). Are Renewable Energy Policies Upsetting Carbon Dioxide Emissions? The Case of Latin America Countries. Environ. Sci. Pollut. Res. 24 (17), 15044–15054. doi:10.1007/s11356-017-9109-z
Fuinhas, J. A., Koengkan, M., Leitão, N. C., Nwani, C., Uzuner, G., Dehdar, F., et al. (2021). Effect of Battery Electric Vehicles on Greenhouse Gas Emissions in 29 European Union Countries. Sustainability 13 (24), 13611. doi:10.3390/su132413611
Grossman, G. M., and Krueger, A. B. (1995). Economic Growth and the Environment. Q. J. Econ. 110 (2), 353–377. doi:10.2307/2118443
Guo, Q., Wang, Y., and Dong, X. (2022a). Effects of Smart City Construction on Energy Saving and CO2 Emission Reduction: Evidence from China. Appl. Energy 313, 118879. doi:10.1016/j.apenergy.2022.118879
Guo, Q., Wang, Y., Zhang, Y., Yi, M., and Zhang, T. (2022b). Environmental Migration Effects of Air Pollution: Micro-level Evidence from China. Environ. Pollut. 292, 118263. doi:10.1016/j.envpol.2021.118263
Hainmueller, J., and Hazlett, C. (2014). Kernel Regularized Least Squares: Reducing Misspecification Bias with a Flexible and Interpretable Machine Learning Approach. Polit. Anal. 22 (2), 143–168. doi:10.1093/pan/mpt019
Haseeb, A., Xia, E., Danish, M. A., Baloch, M. A., and Abbas, K. (2018). Financial Development, Globalization, and CO2 Emission in the Presence of EKC: Evidence from BRICS Countries. Environ. Sci. Pollut. Res. 25 (31), 31283–31296. doi:10.1007/s11356-018-3034-7
Hepburn, C., Qi, Y., Stern, N., Ward, B., Xie, C., and Zenghelis, D. (2021). Towards Carbon Neutrality and China's 14th Five-Year Plan: Clean Energy Transition, Sustainable Urban Development, and Investment Priorities. Environ. Sci. Ecotechn. 8, 100130. doi:10.1016/j.ese.2021.100130
Hipp, J. R., Kane, K., and Kim, J. H. (2017). Recipes for Neighborhood Development: A Machine Learning Approach toward Understanding the Impact of Mixing in Neighborhoods. Landsc. Urban Plan. 164, 1–12. doi:10.1016/j.landurbplan.2017.03.006
Işık, C., Ongan, S., and Özdemir, D. (2019). Testing the EKC Hypothesis for Ten US States: An Application of Heterogeneous Panel Estimation Method. Environ. Sci. Pollut. Res. Int. 26 (11), 10846–10853. doi:10.1007/s11356-019-04514-6
Jordan, S., and Philips, A. Q. (2018). Cointegration Testing and Dynamic Simulations of Autoregressive Distributed Lag Models. Stata J. 18 (4), 902–923. doi:10.1177/1536867x1801800409
Katrakilidis, C., Kyritsis, I., and Patsika, V. (2016). The Dynamic Linkages between Economic Growth, Environmental Quality and Health in Greece. Appl. Econ. Lett. 23 (3), 217–221. doi:10.1080/13504851.2015.1066482
Khan, M. K., Khan, M. I., and Rehan, M. (2020). The Relationship between Energy Consumption, Economic Growth and Carbon Dioxide Emissions in Pakistan. Financ. Innov. 6 (1), 1–13. doi:10.1186/s40854-019-0162-0
Khan, I., Hou, F., and Le, H. P. (2021). The Impact of Natural Resources, Energy Consumption, and Population Growth on Environmental Quality: Fresh Evidence from the United States of America. Sci. Total Environ. 754, 142222. doi:10.1016/j.scitotenv.2020.142222
Khan, M. K., Babar, S. F., Oryani, B., Dagar, V., Rehman, A., Zakari, A., et al. (2022). Role of Financial Development, Environmental-Related Technologies, Research and Development, Energy Intensity, Natural Resource Depletion, and Temperature in Sustainable Environment in Canada. Environ. Sci. Pollut. Res. 29 (1), 622–638. doi:10.1007/s11356-021-15421-0
Koc, S., and Bulus, G. C. (2020). Testing Validity of the EKC Hypothesis in South Korea: Role of Renewable Energy and Trade Openness. Environ. Sci. Pollut. Res. 27 (23), 29043–29054. doi:10.1007/s11356-020-09172-7
Koengkan, M., and Fuinhas, J. A. (2020). Exploring the Effect of the Renewable Energy Transition on CO2 Emissions of Latin American & Caribbean Countries. Int. J. Sustain. Energy 39 (6), 515–538. doi:10.1080/14786451.2020.1731511
Koengkan, M., and Fuinhas, J. A. (2021a). Does the Overweight Epidemic Cause Energy Consumption? A Piece of Empirical Evidence from the European Region. Energy 216, 119297. doi:10.1016/j.energy.2020.119297
Koengkan, M., and Fuinhas, J. A. (2021b). Is Gender Inequality an Essential Driver in Explaining Environmental Degradation? Some Empirical Answers from the CO2 Emissions in European Union Countries. Environ. Impact Assess. Rev. 90, 106619. doi:10.1016/j.eiar.2021.106619
Koengkan, M., Losekann, L. D., and Fuinhas, J. A. (2019a). The Relationship between Economic Growth, Consumption of Energy, and Environmental Degradation: Renewed Evidence from Andean Community Nations. Environ. Syst. Decis. 39 (1), 95–107. doi:10.1007/s10669-018-9698-1
Koengkan, M., Santiago, R., Fuinhas, J. A., and Marques, A. C. (2019b). Does Financial Openness Cause the Intensification of Environmental Degradation? New Evidence from Latin American and Caribbean Countries. Environ. Econ. Policy Stud. 21 (4), 507–532. doi:10.1007/s10018-019-00240-y
Kripfganz, S., and Schneider, D. C. (2020). Response Surface Regressions for Critical Value Bounds and Approximate P‐values in Equilibrium Correction Models. Oxf. Bull. Econ. Stat. 82 (6), 1456–1481. doi:10.1111/obes.12377
Kwakwa, P. A., Adu, G., and Osei-Fosu, A. K. (2018). A Time Series Analysis of Fossil Fuel Consumption in Sub-saharan Africa: Evidence from Ghana, Kenya and South Africa. Int. J. Sustain. Energy Plan. Manag. 17, 31–44. doi:10.5278/ijsepm.2018.17.4
Le, T.-H., Chang, Y., and Park, D. (2020). Renewable and Nonrenewable Energy Consumption, Economic Growth, and Emissions: International Evidence. Energy J. 41 (2). doi:10.5547/01956574.41.2.thle
Li, Z.-Z., Li, R. Y. M., Malik, M. Y., Murshed, M., Khan, Z., and Umar, M. (2021). Determinants of Carbon Emission in China: How Good is Green Investment? Sustain. Prod. Consum. 27, 392–401. doi:10.1016/j.spc.2020.11.008
Liu, Z., Ciais, P., Deng, Z., Lei, R., Davis, S. J., Feng, S., et al. (2020). Near-real-time Monitoring of Global CO2 Emissions Reveals the Effects of the COVID-19 Pandemic. Nat. Commun. 11 (1), 5172–5212. doi:10.1038/s41467-020-18922-7
Liu, J., Murshed, M., Chen, F., Shahbaz, M., Kirikkaleli, D., and Khan, Z. (2021). An Empirical Analysis of the Household Consumption-Induced Carbon Emissions in China. Sustain. Prod. Consum. 26, 943–957. doi:10.1016/j.spc.2021.01.006
Magazzino, C., Mele, M., and Schneider, N. (2020a). A Machine Learning Approach on the Relationship Among Solar and Wind Energy Production, Coal Consumption, GDP, and CO2 Emissions. Renew. Energy 151, 829–836. doi:10.1016/j.renene.2020.11.050
Magazzino, C., Udemba, E. N., and Bekun, F. (2020b). Modeling the Nexus between Pollutant Emission, Energy Consumption, Foreign Direct Investment, and Economic Growth: New Insights from China. Environ. Sci. Pollut. Res. Int. 27, 17831–17842. doi:10.1007/s11356-020-08180-x
Malik, M. Y., Latif, K., Khan, Z., Butt, H. D., Hussain, M., and Nadeem, M. A. (2020). Symmetric and Asymmetric Impact of Oil Price, FDI and Economic Growth on Carbon Emission in Pakistan: Evidence from ARDL and Non-linear ARDL Approach. Sci. Total Environ. 726, 138421. doi:10.1016/j.scitotenv.2020.138421
Mallapaty, S. (2020). How China Could Be Carbon Neutral by Mid-century. Nature 586 (7830), 482–483. doi:10.1038/d41586-020-02927-9
Martins, T., Barreto, A. C., Souza, F. M., and Souza, A. M. (2021). Fossil Fuels Consumption and Carbon Dioxide Emissions in G7 Countries: Empirical Evidence from ARDL Bounds Testing Approach. Environ. Pollut. 291, 118093. doi:10.1016/j.envpol.2021.118093
Mehmood, U., and Tariq, S. (2020). Globalization and CO2 Emissions Nexus: Evidence from the EKC Hypothesis in South Asian Countries. Environ. Sci. Pollut. Res. 27 (29), 37044–37056. doi:10.1007/s11356-020-09774-1
Mele, M., and Magazzino, C. (2020). A Machine Learning Analysis of the Relationship Among Iron and Steel Industries, Air Pollution, and Economic Growth in China. J. Clean. Prod. 277, 123293. doi:10.1016/j.jclepro.2020.123293
Muhammad, B. (2019). Energy Consumption, CO2 Emissions and Economic Growth in Developed, Emerging and Middle East and North Africa Countries. Energy 179, 232–245. doi:10.1016/j.energy.2019.03.126
Murshed, M., Alam, R., and Ansarin, A. (2021). The Environmental Kuznets Curve Hypothesis for Bangladesh: The Importance of Natural Gas, Liquefied Petroleum Gas, and Hydropower Consumption. Environ. Sci. Pollut. Res. 28 (14), 17208–17227. doi:10.1007/s11356-020-11976-6
Murshed, M. (2021). Can Regional Trade Integration Facilitate Renewable Energy Transition to Ensure Energy Sustainability in South Asia? Energy Rep. 7, 808–821. doi:10.1016/j.egyr.2021.01.038
Musah, M., Kong, Y., Mensah, I. A., Antwi, S. K., Osei, A. A., and Donkor, M. (2021). Modelling the Connection between Energy Consumption and Carbon Emissions in North Africa: Evidence from Panel Models Robust to Cross-Sectional Dependence and Slope Heterogeneity. Environ. Dev. Sustain. 23, 1–15. doi:10.1007/s10668-021-01294-3
Nathaniel, S. P., and Bekun, F. V. (2021). Electricity Consumption, Urbanization, and Economic Growth in Nigeria: New Insights from Combined Cointegration amidst Structural Breaks. J. Public Aff. 21 (1), e2102. doi:10.1002/pa.2102
Nathaniel, S., Anyanwu, O., and Shah, M. (2020). Renewable Energy, Urbanization, and Ecological Footprint in the Middle East and North Africa Region. Environ. Sci. Pollut. Res. Int. 27, 14601–14613. doi:10.1007/s11356-020-08017-7
Nawaz, M. A., Hussain, M. S., Kamran, H. W., Ehsanullah, S., Maheen, R., and Shair, F. (2021). Trilemma Association of Energy Consumption, Carbon Emission, and Economic Growth of BRICS and OECD Regions: Quantile Regression Estimation. Environ. Sci. Pollut. Res. 28 (13), 16014–16028. doi:10.1007/s11356-020-11823-8
Nurgazina, Z., Ullah, A., Ali, U., Koondhar, M. A., and Lu, Q. (2021). The Impact of Economic Growth, Energy Consumption, Trade Openness, and Financial Development on Carbon Emissions: Empirical Evidence from Malaysia. Environ. Sci. Pollut. Res. Int. 28, 60195–60208. doi:10.1007/s11356-021-14930-2
Omri, A. (2013). CO2 Emissions, Energy Consumption and Economic Growth Nexus in MENA Countries: Evidence from Simultaneous Equations Models. Energy Econ. 40, 657–664. doi:10.1016/j.eneco.2013.09.003
Pao, H.-T., Yu, H.-C., and Yang, Y.-H. (2011). Modeling the CO2 Emissions, Energy Use, and Economic Growth in Russia. Energy 36 (8), 5094–5100. doi:10.1016/j.energy.2011.06.004
Pata, U. K., and Caglar, A. E. (2021). Investigating the EKC Hypothesis with Renewable Energy Consumption, Human Capital, Globalization and Trade Openness for China: Evidence from Augmented ARDL Approach with a Structural Break. Energy 216, 119220. doi:10.1016/j.energy.2020.119220
Pata, U. K., and Isik, C. (2021). Determinants of the Load Capacity Factor in China: A Novel Dynamic ARDL Approach for Ecological Footprint Accounting. Resour. Policy 74, 102313. doi:10.1016/j.resourpol.2021.102313
Pata, U. K., and Kumar, A. (2021). The Influence of Hydropower and Coal Consumption on Greenhouse Gas Emissions: A Comparison between China and India. Water 13 (10), 1387. doi:10.3390/w13101387
Pata, U. K. (2021). Linking Renewable Energy, Globalization, Agriculture, CO2 Emissions and Ecological Footprint in BRIC Countries: A Sustainability Perspective. Renew. Energy 173, 197–208. doi:10.1016/j.renene.2021.03.125
Perron, P. (1989). The Great Crash, the Oil Price Shock, and the Unit Root Hypothesis. Econometrica 57, 1361–1401. doi:10.2307/1913712
Pesaran, M. H., Shin, Y., and Smith, R. J. (2001). Bounds Testing Approaches to the Analysis of Level Relationships. J. Appl. Econ. 16 (3), 289–326. doi:10.1002/jae.616
Qin, L., Raheem, S., Murshed, M., Miao, X., Khan, Z., and Kirikkaleli, D. (2021). Does Financial Inclusion Limit Carbon Dioxide Emissions? Analyzing the Role of Globalization and Renewable Electricity Output. Sustain. Dev. 29, 1138. doi:10.1002/sd.2208
Rahman, M. M., Saidi, K., and Mbarek, M. B. (2020). Economic Growth in South Asia: the Role of CO2 Emissions, Population Density and Trade Openness. Heliyon 6 (5), e03903. doi:10.1016/j.heliyon.2020.e03903
Rahman, M. M., Nepal, R., and Alam, K. (2021). Impacts of Human Capital, Exports, Economic Growth and Energy Consumption on CO2 Emissions of a Cross-Sectionally Dependent Panel: Evidence from the Newly Industrialized Countries (NICs). Environ. Sci. Policy 121, 24–36. doi:10.1016/j.envsci.2021.03.017
Rauf, A., Liu, X., Amin, W., Ozturk, I., Rehman, O. U., and Hafeez, M. (2018). Testing EKC Hypothesis with Energy and Sustainable Development Challenges: a Fresh Evidence from Belt and Road Initiative Economies. Environ. Sci. Pollut. Res. 25 (32), 32066–32080. doi:10.1007/s11356-018-3052-5
Rehman, A., Ma, H., Ozturk, I., Murshed, M., and Dagar, V. (2021a). The Dynamic Impacts of CO2 Emissions from Different Sources on Pakistan's Economic Progress: A Roadmap to Sustainable Development. Environ. Dev. Sustain. 23 (12), 17857–17880. doi:10.1007/s10668-021-01418-9
Rehman, A., Ulucak, R., Murshed, M., Ma, H., and Işık, C. (2021b). Carbonization and Atmospheric Pollution in China: The Asymmetric Impacts of Forests, Livestock Production, and Economic Progress on CO2 Emissions. J. Environ. Manag. 294, 113059. doi:10.1016/j.jenvman.2021.113059
Rehman, A., Ma, H., Ozturk, I., and Ulucak, R. (2022). Sustainable Development and Pollution: the Effects of CO2 Emission on Population Growth, Food Production, Economic Development, and Energy Consumption in Pakistan. Environ. Sci. Pollut. Res. 29 (12), 17319–17330. doi:10.1007/s11356-021-16998-2
Ren, L., Zhou, S., Peng, T., and Ou, X. (2021). A Review of CO2 Emissions Reduction Technologies and Low-Carbon Development in the Iron and Steel Industry Focusing on China. Renew. Sustain. Energy Rev. 143, 110846. doi:10.1016/j.rser.2021.110846
Rothman, D. S. (1998). Environmental Kuznets Curves-Real Progress or Passing the Buck? A Case for Consumption-Based Approaches. Ecol. Econ. 25 (2), 177–194. doi:10.1016/s0921-8009(97)00179-1
Sahoo, M., and Sahoo, J. (2020). Effects of Renewable and Non‐renewable Energy Consumption on CO2 Emissions in India: Empirical Evidence from Disaggregated Data Analysis. J. Public Aff. 22, e2307. doi:10.1002/pa.2307
Saint Akadiri, S., Alola, A. A., Akadiri, A. C., and Alola, U. V. (2019). Renewable Energy Consumption in EU-28 Countries: Policy toward Pollution Mitigation and Economic Sustainability. Energy Policy 132, 803–810. doi:10.1016/j.enpol.2019.06.040
Sarkodie, S. A., and Owusu, P. A. (2020). How to Apply the Novel Dynamic ARDL Simulations (Dynardl) and Kernel-Based Regularized Least Squares (krls). MethodsX 7, 101160. doi:10.1016/j.mex.2020.101160
Satrovic, E., Ahmad, M., and Muslija, A. (2021). Does Democracy Improve Environmental Quality of GCC Region? Analysis Robust to Cross-Section Dependence and Slope Heterogeneity. Environ. Sci. Pollut. Res. 28, 1–16. doi:10.1007/s11356-021-15020-z
Shahzad, U., Fareed, Z., Shahzad, F., and Shahzad, K. (2021). Investigating the Nexus between Economic Complexity, Energy Consumption and Ecological Footprint for the United States: New Insights from Quantile Methods. J. Clean. Prod. 279, 123806. doi:10.1016/j.jclepro.2020.123806
Talbi, B., Jebli, M. B., Bashir, M. F., and Shahzad, U. (2020). Does Economic Progress and Electricity Price Induce Electricity Demand: A New Appraisal in Context of Tunisia. J. Public Aff. 22, e2379. doi:10.1002/pa.2379
Ulucak, Z. Ş., İlkay, S. Ç., Özcan, B., and Gedikli, A. (2020). Financial Globalization and Environmental Degradation Nexus: Evidence from Emerging Economies. Resour. Policy 67, 101698. doi:10.1016/j.resourpol.2020.101698
Umar, M., Ji, X., Kirikkaleli, D., Shahbaz, M., and Zhou, X. (2020). Environmental Cost of Natural Resources Utilization and Economic Growth: Can China Shift Some Burden through Globalization for Sustainable Development? Sustain. Dev. 28 (6), 1678–1688. doi:10.1002/sd.2116
Ummalla, M., and Goyari, P. (2021). The Impact of Clean Energy Consumption on Economic Growth and CO2 Emissions in BRICS Countries: Does the Environmental Kuznets Curve Exist? J. Public Aff. 21 (1), e2126. doi:10.1002/pa.2126
Wang, Y., Li, L., Kubota, J., Han, R., Zhu, X., and Lu, G. (2016). Does Urbanization Lead to More Carbon Emission? Evidence from a Panel of BRICS Countries. Appl. Energy 168, 375–380. doi:10.1016/j.apenergy.2016.01.105
Wasti, S. K. A., and Zaidi, S. W. (2020). An Empirical Investigation between CO2 Emission, Energy Consumption, Trade Liberalization and Economic Growth: A Case of Kuwait. J. Build. Eng. 28, 101104. doi:10.1016/j.jobe.2019.101104
WorldBank (2021). The World Bank Data. Available at: https://data.worldbank.org/.
Yilanci, V., and Pata, U. K. (2020). Investigating the EKC Hypothesis for China: The Role of Economic Complexity on Ecological Footprint. Environ. Sci. Pollut. Res. 27 (26), 32683–32694. doi:10.1007/s11356-020-09434-4
Zambrano-Monserrate, M. A., Silva-Zambrano, C. A., Davalos-Penafiel, J. L., Zambrano-Monserrate, A., and Ruano, M. A. (2018). Testing Environmental Kuznets Curve Hypothesis in Peru: The Role of Renewable Electricity, Petroleum and Dry Natural Gas. Renew. Sustain. Energy Rev. 82, 4170–4178. doi:10.1016/j.rser.2017.11.005
Zeraibi, A., Balsalobre-Lorente, D., and Murshed, M. (2021). The Influences of Renewable Electricity Generation, Technological Innovation, Financial Development, and Economic Growth on Ecological Footprints in ASEAN-5 Countries. Environ. Sci. Pollut. Res. 28, 1–19. doi:10.1007/s11356-021-14301-x
Zhang, L., Godil, D. I., Bibi, M., Khan, M. K., Sarwat, S., and Anser, M. K. (2021). Caring for the Environment: How Human Capital, Natural Resources, and Economic Growth Interact with Environmental Degradation in Pakistan? A Dynamic ARDL Approach. Sci. Total Environ. 774, 145553. doi:10.1016/j.scitotenv.2021.145553
Zhu, L., Hao, Y., Lu, Z.-N., Wu, H., and Ran, Q. (2019). Do economic Activities Cause Air Pollution? Evidence from China's Major Cities. Sustain. Cities Soc. 49, 101593. doi:10.1016/j.scs.2019.101593
Keywords: DYNARDL model, environmental degradation, economic growth, energy consumption, urbanization
Citation: Nurgazina Z, Guo Q, Ali U, Kartal MT, Ullah A and Khan ZA (2022) Retesting the Influences on CO2 Emissions in China: Evidence From Dynamic ARDL Approach. Front. Environ. Sci. 10:868740. doi: 10.3389/fenvs.2022.868740
Received: 03 February 2022; Accepted: 19 April 2022;
Published: 25 May 2022.
Edited by:
Cosimo Magazzino, Roma Tre University, ItalyReviewed by:
Matheus Koengkan, University of Evora, PortugalCopyright © 2022 Nurgazina, Guo, Ali, Kartal, Ullah and Khan. This is an open-access article distributed under the terms of the Creative Commons Attribution License (CC BY). The use, distribution or reproduction in other forums is permitted, provided the original author(s) and the copyright owner(s) are credited and that the original publication in this journal is cited, in accordance with accepted academic practice. No use, distribution or reproduction is permitted which does not comply with these terms.
*Correspondence: Qingbin Guo, Z3FiQGhhaW5hbnUuZWR1LmNu
Disclaimer: All claims expressed in this article are solely those of the authors and do not necessarily represent those of their affiliated organizations, or those of the publisher, the editors and the reviewers. Any product that may be evaluated in this article or claim that may be made by its manufacturer is not guaranteed or endorsed by the publisher.
Research integrity at Frontiers
Learn more about the work of our research integrity team to safeguard the quality of each article we publish.