- 1Plant Stress Biology and Metabolomics Laboratory, Central Instrumentation Laboratory (CIL), Assam University, Silchar, India
- 2Department of Environmental Science, University of Kalyani, Kalyani, India
- 3ICAR−Indian Institute of Water Management, Bhubaneswar, India
- 4Department of Botany and Plant Physiology, Faculty of Agrobiology, Food, and Natural Resources, Czech University of Life Sciences Prague, Prague, Czechia
- 5ICAR-Directorate of Weed Research, Jabalpur, India
- 6Department of Zoology, Dhemaji College, Dhemaji, India
- 7Plant Stress Biology & Metabolomics Laboratory, Department of Life Science and Bioinformatics, Assam (Central) University, Silchar, India
- 8Department of Ecological Studies & International Centre for Ecological Engineering, University of Kalyani, Kalyani, India
- 9Department of Plant Physiology, Slovak University of Agriculture, Nitra, Slovak
- 10Department of Agronomy, Bangladesh Wheat and Maize Research Institute, Dinajpur, Bangladesh
Arsenic contamination in the rice agro-ecosystem, its consequent spread into the food chain, and its adverse impact are a global concern. However, assessment of the impact of arsenic contamination on the qualitative aspect of rice in the post-harvest phase has not been attempted. We laid down this particular experiment with the aim to assess how arsenic contamination influences the grain quality attributes and other elemental profiles of four popular rice varieties. The entire field study was conducted in the lower Indo-Gangetic plain from genotype (four) and environment (14 locations) interaction point of view, using the additive main effects and multiplicative interaction model. Our findings indicate that grain arsenic content can influence the grain quality attributes and other elemental profiles in a low to highly significant manner. Amylose content (r = 0.753), cooking time (r = 0.706), and gruel solid loss (r = 0.672, 0.721) were found to be positively correlated with grain arsenic content in high-yielding varieties. Noteworthy variations in micronutrient content like iron (6.63–9.23 mg kg−1), zinc (3.15–5.54 mg kg−1), and copper (2.04–3.86 mg kg−1) as well as soil properties are also visible. Moreover, indigenous rice varieties respond differently than high-yielding varieties. Besides these, a pronounced impact of gene/variety and environment interaction can be seen. The findings clearly indicate that the qualitative aspect of rice is also prone to As contamination. These outcomes will attract the attention of policy-makers and researchers to develop rice varieties that have desirable quality attributes appropriate for the arsenic-contaminated regions of the world for sustainable rice production.
Introduction
It is noteworthy that under business-as-usual situation, the world population is projected to reach a massive 9.7 billion by 2050 (United Nations, 2017), the majority of the increase of which will occur in developing countries. To feed the swelling population with rice as its staple food, rice production must increase proportionately in a sustainable manner. Approximately >100 countries are cultivating rice, but Asian countries are credited with producing as much as 90% of global rice production (Muthayya et al., 2014; Yu et al., 2020). During rice cultivation, encounters or interactions among environmental resources as well as contaminants with the genotypes are obvious. In order to enhance the productivity to feed the increasing population, farmers, as well as researchers, are required to modify the agronomic practices and associated arena (Ali et al., 2018).
Speaking of rice cultivation in Ganga–Meghna–Bramhaputra basin or GMB area (located in India and adjoining Bangladesh), which is regarded as one of the most productive rice-cultivating regions in the world, two distinct rice cultivation methods are in practice from an agronomic standpoint. Boro (winter rice) is entirely dependent upon groundwater irrigation, whereas kharif rice (monsoon rice cultivated from July to December), though dependent on available soil moisture, but with the irregularity of the rainfall pattern (as a result of climate fluctuation), requires occasional irrigation in order to maintain a suitable environment (Ghosh et al., 2020; Ghosh et al., 2022).
Arsenic (As) contamination in ground-water in large geographical areas of the world, irrigation with contaminated ground-water, its subsequent deposition in agricultural field soil used for rice cultivation, and later cooking with contaminated water (often As content beyond the permissible limit) and consumption of As-laden rice by the global population are visualized as a major disaster (Meharg, 2004).
Next to drinking As-contaminated water, numerous reports on concerns of rice cultivated in As-laden parts of the lower Indo-Gangetic plain in India and touching Bangladesh as well as around the world and on the transfer of As into the food chain have drawn global attention (Santra and Samal, 2013; Chowdhury et al., 2018; Chowdhury et al., 2020; Moulick et al., 2021; Moulick et al., 2016).
Season-wise, wide variations in grain As content of different rice varieties (cultivated in boro and kharif seasons) were reported from various rice fields of West Bengal (India) (Santra and Samal, 2013). Several authors have studied the influence of As content of irrigation water and baseline soil (Williams et al., 2006; Lu et al., 2009). Conversely, different physicochemical factors, such as pH, redox status (Eh), clay content, the existence of poorly crystalline iron oxide, and lastly organic matter content—the influences of macronutrient like nitrogen—of the soil, can influence the phyto-availability of As to rice plant (Bogdan and Schenk, 2009; Takahashi et al., 2004). Furthermore, Brammer (2009) revealed the influences of varied soil fractions on the phyto-availability of As based on their study on different scales from local to regional levels across Bangladesh. On the other hand, Norton et al. (2009b) studied the role of rice genetics on As accumulation, citing their findings based on an experiment conducted in Bangladesh. Later, upon the elaboration of this particular work conducted in various study sites located across India and China, the significant influence of agro-environment on grain As concentration, felt alongside rice genetics, was reported (Norton et al., 2009a). The findings of Yu et al. (2008) indicate that a great deal of discrepancy was noted in the Fe content (2.5–7.5 mg kg−1) among the genotypes investigated. In another investigation carried out by Huang et al. (2016), the elemental profile of 20 rice cultivars/varieties (analyzed for two successive seasons) was suggested, with noteworthy genotypic variations in seven studied elements whose content were measured (including Fe, Zn, and Cu). Zeng et al. (2019) observed that the greatest genotypic fluctuations were noted in terms of Cu content in brown rice (among 628 landraces). The variation is approximately 5.6 times among the genotypes with the highest and the lowest Cu content. Zeng et al. (2019) further added that the mean Cu concentration (13.6 mg kg−1) in the rice grains of these studied landraces also had a huge range (0.1–59.1 mg kg−1).
Furthermore, if we carefully observe the research inclination regarding the consequences of rice cultivation in an As-contaminated agroecosystem, this is scattered specifically around 1) biomonitoring of As accumulation in rice grain, 2) risk assessment of As from dietary exposure, 3) spatio-temporal variation of As in paddy field soil, 4) phytotoxicity of As, and 5) mitigation of As accumulation in rice grains, which are predominant in scientific literature at regular intervals. Though most research has focused on quantitative issues (like yield, As content, various agronomic parameters, etc.), very tiny attention has been given to assess the impact of As stress on the qualitative aspect of rice in the post-harvest phase, though several reports are addressing the adverse consequences of As contamination on rice plant’s life cycle and yield (Moulick et al., 2017; Moulick et al., 2018a; 2018b; 2018c; 2019a; 2019b; Saha et al., 2019) and the toxic health effects of As-rich rice on the consuming population (Upadhyay et al., 2019; Atiaga et al., 2020; Biswas et al., 2020; González et al., 2020; Menon et al., 2020). Moreover, a significant amount of research is also directed towards developing a wide range of mitigation options for various environmental odds, including As accumulation in rice plants as well as in the post-harvest phase, specially using rice parboiling (Hossain et al., 2021; Hossain et al., 2022; Choudhury et al., 2021a; Choudhury et al., 2021b, 2022; Saha et al., 2019; Sahoo et al., 2019).
Along with these, several articles are addressing the impact of various environmental odds (except for the As stress) on the grain quality traits available (Fahad et al., 2019; Yang and Wang, 2019; Zeng et al., 2019; Moulick et al., 2020a). However, no article illustrates the impact of arsenic contamination in the post-harvest phase, i.e., the qualitative aspect of rice grain. The current article presents, for the first time, how a hazardous element can influence the rice-based economy, which remains unanswered so far.
Among the various grain quality traits desired from the consumer/market point of view, head rice recovery percentage (HRR%) is the most important one. Besides appearance and shape, chemical and cooking characteristics (ECQ) also demand a great deal of attention (Aluko et al., 2004). Authors like Juliano (1972) and Fasahat et al. (2014) revealed that, among various chemical characteristics, amylose content significantly influences the cooking and eating characteristics. Rice varieties having a higher amylose content expand more upon cooking (i.e., volume expansion ratio) and have more flakiness, vice versa. As stress and its impact on rice grain quality attributes have been reported by Moulick et al. (2016, 2018a), but how the genotype and environment (As stress here) interaction influences the quality traits and mineralogical attributes has not been reported.
The multivariate genotypic responses to a wide range of environments are regarded as genotype–environment (GE) interaction, originally based on the classical work of Allard and Bradshaw (1964). Genotype by environment interaction effects are of particular curiosity to identify the most desirable genotypes (rice variety) with special reference to particular environments (such as the As-contaminated agro-ecosystem discussed here) symbolic to locations. In order to assess the GE interaction between genotype and environment, various authors have proposed the application of various statistical models—for example, Finlay and Wilkinson (1963) proposed linear regression models, while Zobel et al. (1988) advocated and supported the use of multivariate statistical procedures relative to the univariate regression method and introduced the additive main effects and multiplicative interaction model (AMMI) with emphasis on principal component analysis (PCA) to look into the GE interaction. Gauch and Zobel (1997) proposed the advantages of the first interaction of PCA above the linear regression to explain the GE variations. Gauch (2006) and Gauch et al. (2008) advocated in favor of the AMMI model based on its better performance than the formal univariate stability methods similar to linear regression model and some multivariate procedures such as GGE biplot, commonly known as genotype G × E interaction (Yan et al., 2000).
Here we try to evaluate the grain quality traits of four commonly cultivated rice varieties grown in As contaminated agroecosystem located in the Lower Indo-Gangetic Plain. In an attempt to bridge the existing knowledge gap regarding the interaction between genotype (G) and agricultural environment (E), the present study was conducted with the following objectives: 1) to assess the impact of the intensity of As contamination on grain As accumulation and selected quality traits and 2) to elucidate how the GE interaction can modulate the grain quality traits and elemental profile in rice varieties as well as their interrelationship.
Materials and Methods
Locations of the Study
In this study, we consider four different rice varieties. These were collected from 14 different sampling sites situated across three highly As-affected districts of West Bengal, located within the Lower Indo-Gangetic Plain of GMB. These are Murshidabad District (M1—Beldanga-I, M2—Beldanga-II, M3—Islampur, M4—Reginagar, and M5—Domkol), Nadia District (N1—North Chandamari, N2—PorarMath, N3—Bamonbelia, N4—Kurumbelia, and N5—Paschimer Math), and North 24 Pargana District (P1—Guma, P2—Bira, P3—Prithiba, and P4—Badarhat). These severely arsenic-affected districts are located on the eastern sides of Bhagirathi River, the major distributary of River Ganges and recognized as representative As hotspots in the Lower Indo-Gangetic Plain of the world’s largest delta (Ganges–Brahmaputra–Meghna deltaic alluvium, GBM system). The area has a thick alluvial deposition of the Quaternary age and is characterized by several geomorphological features, such as tidal swamps (permanently waterlogged areas rich in humus) and inter-distributary inland swamps (seasonally waterlogged areas with comparatively lesser organic matter containing silt and/or clay) (Bhowmick et al., 2013). Agricultural land represents the major land use, characterized by rice cultivation as the major agricultural practice. The climate is typically tropical, hot, and humid, with temperature that ranges between 16 and 42°C and average relative humidity of >65%. The annual rainfall ranges between 1,172 and 1,635 mm (mean: 1,436 mm), mostly concentrated during the monsoon (July–October) (Chatterjee et al., 2010). The sources of As are natural or man-made, emanating from anthropogenic activities such as intensive exploitation of groundwater and excessive application of fertilizers and insecticides, which result in elevated concentrations of As in the agricultural field soil. In the studied areas, underground water is used for Boro rice, and rainwater is usually used for Aman rice cultivations. The soil and crop of the rice fields in the GMB plain receive a huge quantity of arsenic through irrigation by arsenic-contaminated groundwater from shallow tube wells.
Experimental Details
Among the selected rice varieties, two are high yielding varieties (MTU-7029, popularly known as Swarna, with a short and bold grain morphology, non-aromatic variety; Satabdi—a medium and slender type grain and non-aromatic type) and two are indigenous aromatic varieties (Gobindabhog—having round and bold grain morphology; Bashkathi—long and slender grain morphology). All the studied varieties were cultivated during the rainy season (July to November 2017). These varieties were cultivated following the line sowing method with 15-cm hill-to-hill gap and 20-cm line-to-line gap at three seedlings per hill. During the first ploughing, 5.0 ton/hectare of farmyard manure, along with NPK at 60–30–30, was applied. Urea was divided into three splits, with a 20.0-kg dose applied at 20 and 40 days after transplanting, respectively. After 90% ripening (turns yellow) of grains took place, the mature rice plants were harvested and thrashed following the traditional method of lifting a paddy bundle and hitting on a wooden board.
Sample Collection
During harvesting, rice grains (1.0 kg) were collected along with the harvested paddy field soil. During the sampling process, the composite sampling method was followed. After that, all the grains (approximately 14.6 ± 0.3% moisture content) and the soil samples (collected from 15.0-cm depth) were sun-dried. During field sampling, the rice (n = 56) and soil samples (n = 56) were collected and analyzed in triplicate during analysis.
Soil Physicochemical and Textural Profile Analysis
The physicochemical properties, viz., pH, conductivity, available phosphate, available nitrate, and textural profile of As-contaminated paddy field were executed by adopting the methodology described by Jackson (2005) and Rayment and Lyons (2012), and organic matter content was determined according to the method described by Walkey and Black (1934) (details are available in Supplementary Table S1).
Sample Treatment for Elemental Analysis (Soil and Rice Grain)
The soil samples were kept in a hot-air oven at 72°C for 48 h, followed by grinding to fine powder, then passing through a 2.0-mm stainless steel sieve to get a homogenized mass, and packing in a pre-designated airtight zipper pouch. The rice grain samples were washed with double-distilled water to ensure the complete absence of soil deposition and sun-dried. A portion of the milled rice was washed thoroughly with double-distilled water to remove any unwanted deposition, then kept in a hot-air oven at 72°C for 48 h, ground to fine powder, and kept in marked plastic pouches for further analysis of the elemental profile (Moulick et al., 2018d).
Acid Digestion of Soil Samples
Soil samples were digested using the block digestion method using aqua regia mixture. The soil samples in the form of dried powder (0.5 g) were taken into clean digestion tubes along with blank reagent (5 ml of aqua regia only) and standard reference material (SRM) obtained from the National Institute of Science and Technology (NIST, United States) San Joaquin soil (item number 2709; see details in Supplementary Table S5) in triplicate; to each digestion tube, 5 ml of aqua regia mixture was added and left overnight without heating at room temperature on a sand bath. On the next morning, the tubes were heated at 110 ± 1.53°C until the content was transformed into a colorless solution (Bhatti et al., 2013). After cooling the tubes, double-distilled water was added to each tube, and the entire content was filtered using Whatman no. 41 filter paper. The final volume was adjusted to 25 ml and kept in pre-marked 60-ml polythene bottles (Supplementary Table S5).
Rice Cooking Method
In the case of cooked rice prior to acid digestion, surface moisture was abolished by keeping them in between two layers of filter paper, and these were kept inside a Petri plate in a hot-air oven at 72°C for 2 days. Then, the completely dried cooked rice was ground into fine powder and kept in separate pre-marked plastic pouches (Moulick et al., 2016).
Acid Digestion of Biological Samples (Rice Grain and Cooked Rice)
Milled rice and cooked rice samples were digested by adopting the methodology of Das et al. (2004). Precisely weighted 0.5-g samples were taken into clean digestion tubes along with SRM obtained from the National Institute of Science and Technology (NIST, United States) rice flour (item number 1568A; see details in Supplementary Table S5) and pure reagent blank in triplicates (prior to digestion, all the digestion tubes were rinsed with 2% nitric acid and dried). Next, 5 ml of tri-acid mixture of perchloric acid (70%), concentrated nitric acid, and sulphuric acid (all ACS grade MERCK) was mixed at 1:1.5:1 ratio and added to each tube, and these tubes were kept on a sand bath (without heating) overnight at room temperature. On the next morning, the mixtures were heated at 80 ± 1.3°C until the content was transformed into a colorless solution. Then, the tubes were allowed to cool at room temperature, followed by adding double-distilled water (As content <0.0003 mg kg−1) and filtering the solution using Whatman no. 42 filter paper. The volumes were adjusted to 25 ml and kept in pre-marked 60-ml polythene bottles.
Sample Analysis for Total As and Other Elements
The total As content and other elements of soil, irrigation water in different parts of the rice plant, and rice (both milled and cooked) were analyzed by using a flow injection hydride generation atomic absorption spectrophotometer (Perkin Elmer AAnalyst 400) and by adopting external calibration as per Koreňovská (2006) and Moulick et al. (2018c) (details are available in Supplementary Table S5).
Sample Preparation for Grain Quality Evaluation
Husk from the rice grains was removed by using Rubber Roll Paddy De-Husker Double—DMR 6 machine (model: DOUBLE DELUX 6″) manufactured by M.G Industries Ltd., Punjab. In order to obtain brown rice as milled rice, first milled and intact (whole) rice kernels (head rice here) were separated from broken rice kernels to further evaluate the physicochemical properties and cooking characteristics.
Rice Grain Quality Trait Evaluation Procedure
One thousand head rice kernels after milling were counted randomly in triplicate and weighed separately, and the mean of three replications was reported (Rao et al., 2013). Amylose content was measured in the rice flour by employing the colorimetric iodine-based method (Juliano, 1971); gel consistency was measured by adopting the methodology described by Cagampang et al. (1973) and based on the consistency of a cold 4.4% milled rice paste in 0.2 mol L−1 KOH. Minimum cooking time, gruel solid loss (GSL), and water uptake ratio (WUR) were measured following the methodology given by Yadav et al. (2007).
Statistical Analysis
Pearson correlation coefficient was obtained for studying the linear relationship between the As content present in the grains and all-grain quality parameters. The AMMI model was used to determine the G, E, and GE interaction effects (Gabriel, 1978). The AMMI model is commonly used to analyze the G × E interaction (Zobel et al., 1988). The model combines the analysis of variance for the genotype and environmental main effects and the PCA with multiplicative indices in a single analysis (Bocianowski et al., 2019). The AMMI model is a popular statistical tool and can easily explain the complicated interactions of genotypes and environments (Gauch, 2013).
The AMMI model for As content of the i-th variety in the j-th location is given by:
where
Results
Grain As and Other Micronutrients and Their Interrelationship
Variety- and location-wise, significant variations in As content (4.79–11.287 mg kg−1), soil pH (6.3–7.4), and other elemental contents in brown rice were observed. Significant (at p <0.05 level) variations in grain As accumulation can be seen in the range of Swarna (0.364 mg kg−1) to Bashkathi (0.195 mg kg−1). Compared to the two non-aromatic and high-yielding varieties, i.e., Swarna and Satabdi, the indigenous aromatic rice varieties, i.e., Gobindobhog and Bashkathi, contained 1.10- and 1.06-fold greater Fe content in brown rice, respectively, whereas the non-aromatic and high-yielding varieties had 11.98% greater Zn content than the indigenous aromatic varieties. The Gobindobhog and Bashkathi varieties had 1.01 and 1.03 times more Cu and Mn content in brown rice than the high-yielding varieties, respectively (Table 1; Supplementary Tables S1, S2).
Among the grain quality attributes considered here, the rice varieties exhibited noteworthy variations from location and varietal points of view. The physical grain quality traits, such as 1,000 grain weight and head rice recovery% (HRR), show that Swarna had the highest grain weight and HRR (67.24%) among the four selected rice varieties. The non-aromatic high-yielding rice varieties had greater grain weight and HRR by 5 and 6.64%, respectively, compared to the indigenous aromatic varieties. The amylose content (AC) and gel consistency (GC) values are the most crucial chemical parameters of rice grain quality attributes also considered here. The results suggest that the indigenous aromatic varieties had an edge over non-aromatic high-yielding rice varieties by 1.06- and 1.47-fold for AC and GC values, respectively. The findings from the current investigation indicated that the cooking times (CT) of the four studied varieties were in the range of 13.082–16.15 min. The WUR values were 2.49–2.84, and the GSL values were 5.85 to 11.70 g/g, respectively. The results also suggest that the non-aromatic high-yielding rice variety had higher scores for CT, WUR, and GSL over the indigenous aromatic varieties by 13.574, 4.304, and 18.901%, respectively (Supplementary Table S3).
Grain Quality, Elemental Profile, and Soil Quality Parameters
Best subset regression analysis was executed using grain As and other micronutrients as the dependent variables and grain quality traits and soil characteristics as the independent variables. The grain As content, explanatory variables, such as CT among grain quality traits, and Eh and NO3, as soil parameters, explained the highest variability (R2 = 0.677) in Satabdi variety. However, only CT significantly affect the As content in the grain at 5% level of significance, whereas in Bashkathi, grain quality traits such as CT and GW and soil parameters such as pH and PO4 explained the variability present in the As content in grain (R2 = 0.643). However, CT, GW, and PO4 significantly affect the As content in the grain at 5% level of significance. In Swarna, explanatory variables such as CT and WUR among grain quality traits and PO4 among soil characteristics explained the variability present in the As content in grain (R2 = 0.768). The other studied variety Gobindobhog revealed that explanatory variables such as GC and WUR explained the variability present in the Fe content in rice grain. Among these, only WUR significantly explained the variability in Fe content in rice. Similarly, in Bashkathi, AC and GSL and PO4, among soil parameters, explained the variability present in the Fe content in grain (R2 = 0.530). However, only AC explained the variability significantly at 5% level of significance, whereas in Swarna variety, the regression revealed that explanatory variable GW can significantly explain the variability present in the Fe content in rice grain (R2 = 0.427). Here the variables with variance inflation factor <5 is considered the criterion for the selection of explanatory variables in the model because it does not require any corrective measures for multicollinearity (Table 2; Supplementary Table S1).
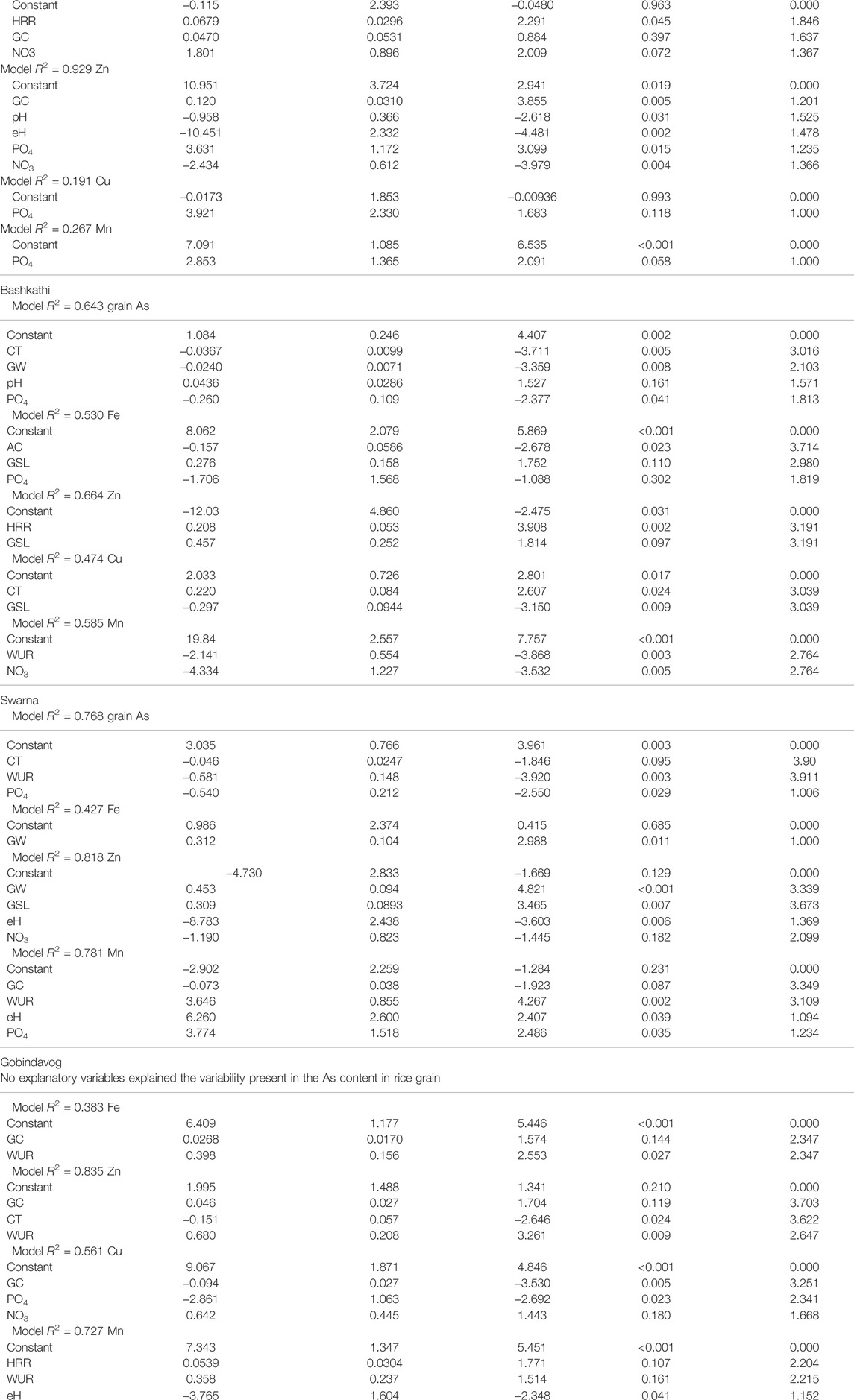
TABLE 2. Best subset regression among grain quality and soil parameters for rice variety(ies) with respect to studied As and other micronutrients.
Performance of Varieties, Location on As, and Other Micronutrients
The combined analysis of variance showed that the main effects of location and variety, as well as their interaction, were significant at 1% level of significance. The results also revealed that 19.8% of the total sum of squares was attributable to location effects, 52.4% to varietal effects, and 27.8% to interaction effects. The application of the AMMI model for partitioning the interaction effects revealed that PC1 and PC2 described 97.8% of the total sum of squares. However, PC3 contributed only 2.2% of the total sum of squares, which was again non-significant (p = 0.937) (Table 3).

TABLE 3. ANOVA for AMMI model and Gollob’s F-test for As content in grains for 4 varieties grown in 14 locations.
Table 4 reveals that the variety Bashkathi had a lower As content in all locations. The highest value of PC1 was observed in Swarna variety, which had the highest value of As in all the locations. Bira (P2), followed by Bamonbelia (N3), Prithiba (P3), and Paschimer Math (N5), had greater than average As content. On the other hand, locations like Reginagar (M4), Beldanga-I (M1), and Beldanga-II (M2) had lower As content in the grains.
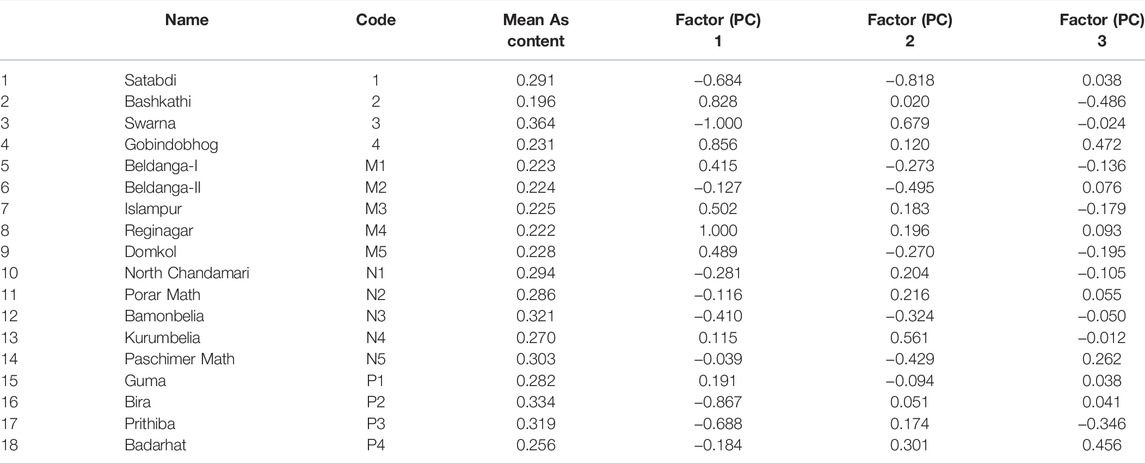
TABLE 4. Eigenvectors of factors in additive main effects and multiplicative interaction biplot analysis of As content in grains for 4 varieties grown in 14 locations.
The AMMI1 biplot depicts locations where the long arrow shows an interaction. In the case of AMMI1 for As content, Bira (P2), Prithiba (P3), and Reginagar (M4) showed a significant GE interaction. In the case of varieties, there appeared a strong interactive behavior. Varieties occurring close together on this plot show a similar pattern with respect to As content over the environments (Figures 1A–E). In the case of Cu, the variety Swarna (3), with its relative PC1 scores close to zero, had less response to the interaction and showed general adaptation to the locations.
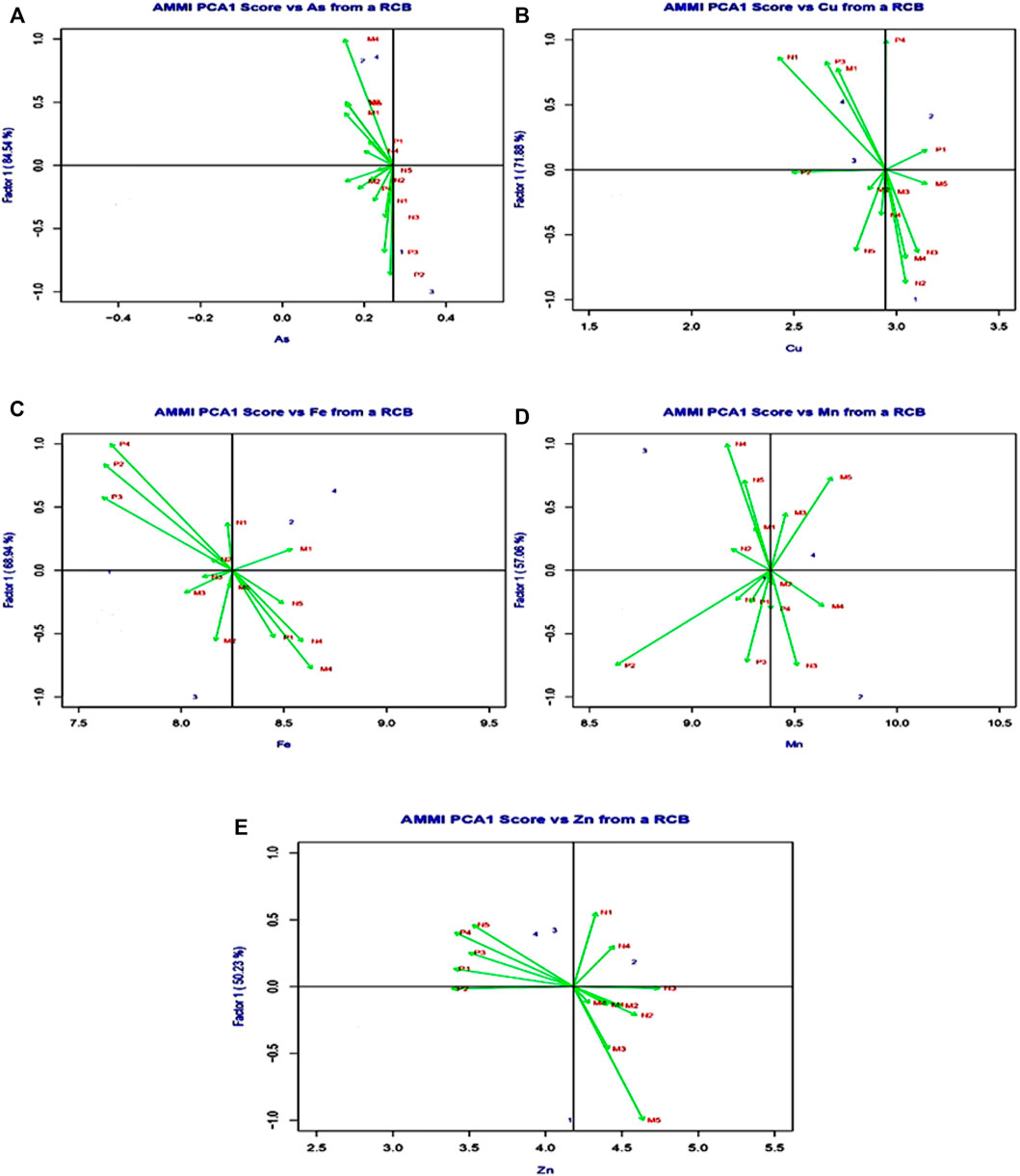
FIGURE 1. Additive main effects and multiplicative interaction 1 biplots for selected metal and metalloids (A) As, (B) Cu, (C) Fe, (D) Mn, and (E) Zn found in 4 varieties in 14 locations. M1, Beldanga-I; M2, Beldanga-II; M3, Islampur; M4, Reginagar; M5, Domkol; N1, North Chandamari; N2, Porar Math; N3, Bamonbelia; N4, Kurumbelia; N5, Paschimer Math; P1, Guma; P2, Bira; P3, Prithiba; P4, Badarhat.
The AMMI2 biplot was obtained by using the scores of the first two components (PC1 and PC2) to study the interaction pattern of four varieties cultivated in 14 locations (Figures 2A–E). The biplot for As content revealed that Satabdi (1) and Swarna (3) varieties expressed a high interactive behavior in either way and contributed more to the exhibited GE interaction. The graph also showed that other varieties, viz., Basakathi (2) and Gobindobhog (4), which are at the right side of the graph, are less affected by the GE interaction and are more or less similar to each other as far as As content is concerned. It can also be seen that variety Swarna (3) contains the highest value of As grown in North Chandamari (N1) and Bira (P2), followed by Prithiba (P3) and Bamonbelia (N3). Satabdi (1) was also observed to have higher As contents in these locations. On the other hand, the other two varieties, Bashkathi (2) and Gobindobhog (4), were found to be better adapted in Islampur (M3) and Reginagar (M4). These two indigenous varieties contain a lower amount of As in all the locations as compared to the other two high-yielding varieties. The Reginagar (M4) location contains higher As content compared to the average As concentration present in these two varieties (Bashkathi and Gobindobhog) (Figure 2).
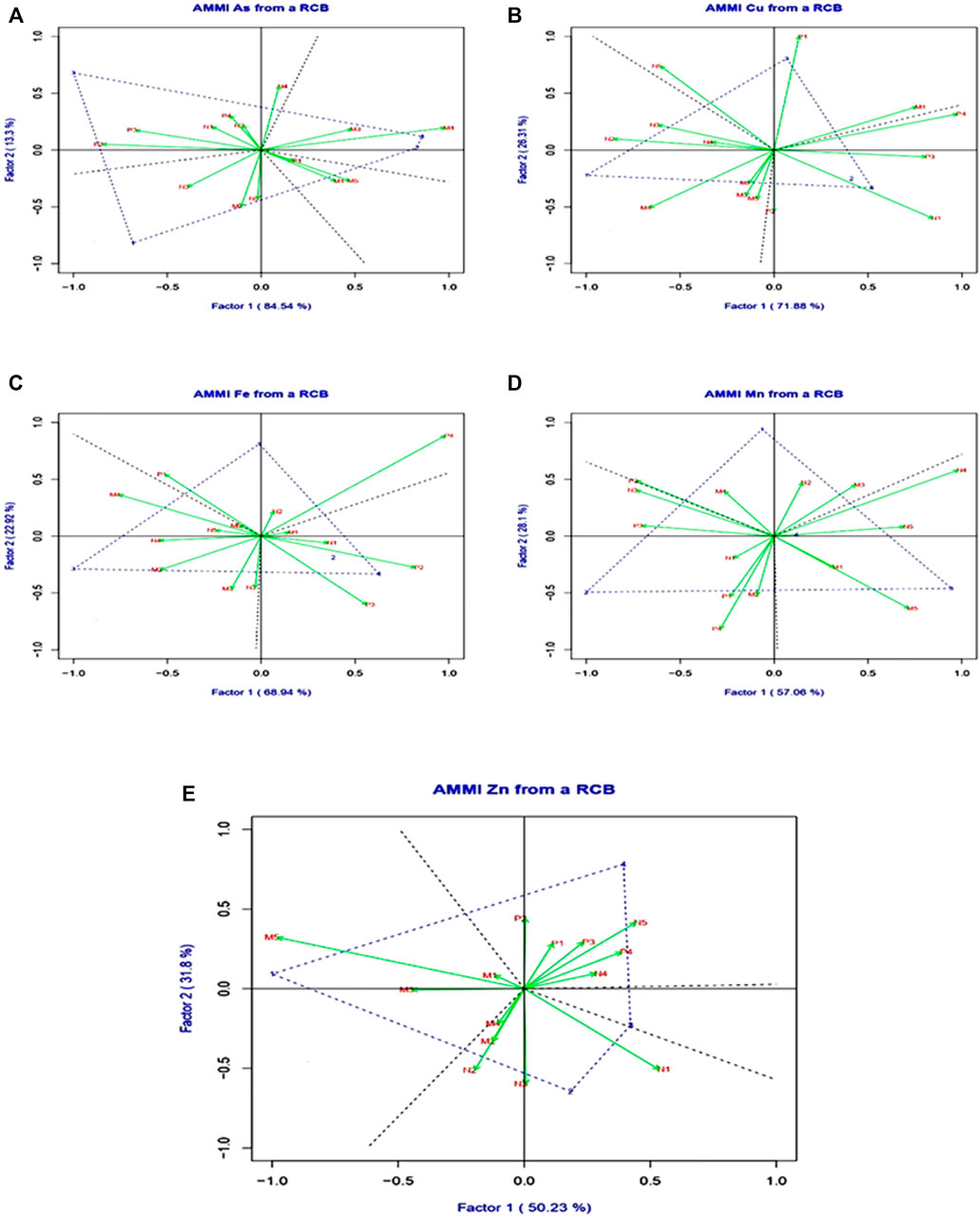
FIGURE 2. Additive main effects and multiplicative interaction 2 biplots for principal components 1 and 2 (factor 1 and factor 2) for selected metal and metalloids (A) As, (B) Cu, (C) Fe, (D) Mn, and (E) Zn. M1, Beldanga-I; M2, Beldanga-II; M3, Islampur; M4, Reginagar; M5, Domkol; N1, North Chandamari; N2, Porar Math; N3, Bamonbelia; N4, Kurumbelia; N5, Paschimer Math; P1, Guma; P2, Bira; P3, Prithiba; P4, Badarhat.
The AMMI2 biplot for Fe and Cu indicated that the varieties Satabdi, Swarna, and Gobindobhog contributed more to the GE interaction, among which Swarna (3) is more adopted to Beldanga-II (M2) and Kurumbelia (N4), while Gobindobhog (4) is more adapted to Bira (P2) and Prithiba (P3) as far as Fe is concerned. In the case of Cu, Swarna (3) is better adapted to Guma (P1), whereas Bashkathi (2) and Gobindobhog (4) are more adapted to North Chandamari (N1). In the case of Mn, the variety Gobindobhog (4) showed more stability against all the tested environments, while the other three varieties exhibited a high interactive behavior either positively or negatively (Table 4; Supplementary Table S4).
Discussion
Rice (Oryza sativa) is cultivated under a broad range of agro-ecosystem throughout the world. From an agro-ecosystem point of view, rice cultivation spans upland and irrigated agro-ecosystems to lowland and rain-fed situations. IRRI (2006) reported about their concern on developing high-yielding rice varieties that have desirable agronomic features (grain quality traits) for a wide range of ecosystems for sustainable production in the coming years (Demont et al., 2013).
Rice, being a field crop, has the credibility of feeding >50% of the global population not only as a generous amount of food but also as a reliable source of energy and nutrition. A significant amount (>40%) is consumed after cooking (Shinde et al., 2014). The grain quality attributes of rice is considered as a multi-layered feature comprising a number of key factors like amylose content, gelatinization temperature, and others (Custodio et al., 2019; Yan et al., 2014; Moulick et al., 2020b). According to Siddiqui et al. (2007), rice grain quality attributes incorporate the entirety of all characteristic features of rice grain as well as the rice products (brown rice here) that satisfy the consumers’ requirements and surprising partiality, too (Cuevas et al., 2017). The endosperm of rice mainly regulates the nutritional importance of its elements and other constituents like starches, proteins, etc. Upgrading of rice grain quality attributes is one of the main goals of the rice breeding program in order to release rice varieties with high-quality traits (Kaosa-ard and Juliano, 1992). Amylose, protein, and cooking behaviors are some of the vital factors that determine rice grain quality (Kang et al., 2011). Appearance, milling properties, nutritional value, and cooking quality of the rice grain are the four key quality traits generally employed to assess the grain quality (Yu et al., 2008).
Interrelationship Among As and Physical Grain Quality Attributes
The results suggest that the presence of As in grain are negatively correlated with the HRR% of Swarna, Satabdi, and Gobindobhog varieties, whereas the relationship among grain As content and HRR% are also positively correlated (r = 0.191) in the case of local landrace Bashkathi. Surprisingly, the impact of Zn had a positive role in modulating the HRR% in Bashkathi. Besides these, the roles of other elements like Fe and Zn (in Satabdi) and Mn (in Gobindobhog) also emerged as key factors in modulating the HRR%, apart from the impact of location(s) that was also evident on the HRR% of the studied varieties. A significantly negative correlation was revealed by the statistical analysis, carried out among 1,000/test—weight and grain As content in Satabdi (r = −0.682) and Swarna variety (r = −0.766). The regression analysis suggests the considerable involvement of Fe and Zn in modulating the 1,000 GW of Swarna variety, which was applicable to other varieties also (Figure 1; Tables 1, 2; Supplementary Table S3). During the course of the investigation, we have tried to illustrate the actual scenario in a holistic manner, so we analyze the soil quality attributes and so on. Apart from the As content, the soil physicochemical components do have an influential role in modulating the qualitative aspect in the post-harvest phase. Actually, the research on grain quality attributes is comparatively less when compared with the pre-harvest phase. In Table 2, it is suggested that soil components do have a key role in influencing the qualitative aspect. Soil pH and available phosphate (PO4) seem to have influenced the As content in an antagonistic manner in Bashkathi and Swarna, whereas soil conductivity and nitrate content can negatively modulate the As content in the Satabdi variety. A similar influence of soil physicochemical properties can also be seen in influencing other studied traits in the selected varieties (Supplementary Tables S1, S2).
The reduction in HRR% and 1,000 GW of rice variety(ies) cultivated in As-contaminated agro-environment is in good agreement with the findings of Moulick et al. (2016, 2018b). The physical traits (appearance) of the grain quality parameters are the primary features to be considered by the consumers. From a consumer’s point of view, milled rice having greater intact rice (kernel), i.e., higher HRR% and grain weight, are favored (Zhao and Fitzgerald, 2013; Moulick et al., 2020b). The quality of rice milling in the post-harvest phase is typically calculated by using head rice yield or HRR% (Saleh and Meullenet 2015). From farmers as well as consumers’ points of view, HRR% is the main factor for earning the market price. Rice with greater milling quality (i.e., HRR%) is obligatory for exporting to the global market. A satisfactory HRR% is also considered a valuable trait for nourishing inland milling industries. Irrespective of regional boundaries, rice varieties with higher HRR% are always preferred, as a variety with greater HRR% often fetches as much as double the market value compared to broken or brewer’s rice. Among the other field crops, paddy occupies a unique position, as its true economic potential has relayed on the HRR% after milling (Nelson et al., 2011). According to the reports/regulation imposed by USDA (2009) in addressing its standards, milled rice is categorized into six different classes. Though the HRR% data of milled rice have not been given in the mentioned standards, the important considerations incidentally specifying the milling quality of rice or importance of HRR% comprise kernels that got damaged from heating (singly or combined), chalkiness, brewer’s rice (or broken kernels), etc. (Bao, 2019; Li et al., 2014). Siebenmorgen and Lanning (2014) stated that the milling quality of rice can be significantly influenced by agro-environmental and genetic factors as well as post-harvest practices. Apart from these, Zheng et al. (2007) and Xie et al. (2013) found that the shape and the size of the paddy can also influence the HRR%. In the current investigation, variation in HRR% with the shape and the size can be easily observed, with pronounced impact of environmental factors like As and other micronutrients and their respective interactions with genotype (G × E) effects. The chalkiness of rice grain (though an unwanted trait) is also a decisive feature defining the degree of grain breakage after the milling operation. To be very precise, chalkiness is actually a key factor in determining the HRR% and brewer’s rice fraction, which, in turn, can modify the market value of a particular rice variety (Fitzgerald et al., 2009) (Tables 1, 2; Supplementary Table S3).
Authors like Zhao et al. (2016), Qiu et al. (2017), and Wang et al. (2017) observed that a large number of QTLs (scattered throughout the rice chromosomes) are found to be closely linked with the quality attributes. Morita (2000) and Wada et al. (2014) found that agro-environmental factors like elevated temperature and moisture content in the air were capable of modulating grain quality traits (Figures 1, 2).
Brown Rice As Content and ECQ
Rice endosperm (grain) comprises principally as much as ∼90% starch. Therefore, the characteristic features of the starch have a significant role in modulating the ECQ/eating and cooking quality (Zhou et al., 2002). During the course of rice grain formation and maturation, the biosynthesis of starch is catalyzed by sucrose synthase (by converting sucrose into glucose and fructose). The fluctuation in the agro-environment enhances the chalkiness due to a drop in starch deposition, which eventually affects the HRR% and other grain qualities (Fahad et al., 2019). Starch in the rice endosperm is deposited in two different forms, amylose and amylopectin. According to the findings reported by Bao (2012), rice starch and related features have a noteworthy influence in grain quality attributes such as AC, GC, and other components of the eating and cooking qualities (ECQ) of rice. The ECQ can be defined as the characteristic features of a cooking procedure as influenced by gel consistency and the amylose content of the grain concerned (Bao et al., 2002). A particular rice variety having greater gel consistency values signifies more softness and finer quality. In our study, a great deal of variation in GC values can be seen. Among the studied varieties, the GC values registered the following increasing order of variation among the rice varieties cultivated in 14 locations: 28.65–36.35 mm (Satabdi) < 42.29–56.11 mm (Swarna) < 44.86–54.10 mm (Gobindabhog) < 63.91–81.93 mm (Bashkathi). The findings indicate that the indigenous landraces, i.e., Bashkathi and Gobindabhog, had an edge over the other two HYVs studied.
Based on gel consistency values, a particular rice variety can be regarded as either soft (having a gel length of >61 mm), medium (41–60 mm), or hard (<40 mm), respectively (Graham, 2002). This gel consistency assessment was originally developed to categorize the cooked rice having a greater amylose content and widely employed by various breeding programs to ensure the textural property of rice variety (Juliano, 1985; Graham, 2002; Tran et al., 2011). The noteworthy intra- and inter-varietal variations in GC values in a location-specific manner may be attributed to either the creation of unbending rice gels due to the aggregation of starch polymers in the aqueous phase (Dea, 1989; Lii and Tsai, 2000), whereas the case of the intermediate nature of GC values may be due to the interactive effects of the minor genes (waxy, BEIII genes, pullulanase, etc.) or even with the environment (As here), which has yet to be confirmed convincingly (He et al., 2006; Ritika et al., 2010; Nguyen et al., 1998) (Figures 1, 2; Table 1; Supplementary Table S3).
The results suggest a situation where the high-yielding rice varieties behave differently from the indigenous aromatic landraces in terms of GSL and relationship with As. The high-yielding varieties share a significantly positive correlation (r = 0.672 and 0.721) in Satabdi and Swarna varieties, respectively, whereas a negative correlation is found in the case of indigenous races. The findings of Singh et al. (2005) and Singh et al. (2011) indicate that varieties having a greater amylose content usually have a greater GSL. Consumers generally give preference for polished grains due to their culinary aesthetic characteristics, such as the maximum cooking yield and minimum cooking time along with a softer texture (Puri et al., 2014) (Table 1).
Interrelationship Among As and Other Micronutrients
Along with starch, various mineral micronutrients, such as Fe, Zn, and Mn, also play a significant role from human health perspective and thus are considered as important ingredients for the nutritional assessment of rice grain. Speaking of brown rice, the existence of fiber (insoluble in nature) is an added advantage for the gastrointestinal tract and simultaneously promotes cardiovascular health. Authors like Meng et al. (2005) and Deepa et al. (2008) reported the analogous mineral content in brown rice, which is in good agreement with our findings. The deficiency of Fe and Zn, two key micronutrients, is distressing >2 billion people globally (Sandstead, 1991). The lack of Fe in diet results in anemia, whereas the deficiency of zinc results in stunted growth and lowered intelligence quotient (Umeta, et al., 2000). Traditionally, though rice is not regarded to be mineral-enriched, yet it can be a significant source of minerals for billions of people depending on the rice and rice-based products (Zhang et al., 2014). Thus, even a minor intensification in the mineral element content of rice grain, mainly in areas in developing countries (as well as As-contaminated areas), would be beneficial to bring high dividends from human health perspective (Supplementary Table S2).
The results also indicate that Cu and Mn share a negative correlation with grain As content, which is applicable to all the studied varieties irrespective of the location, whereas an interesting observation was made with respect to Fe content and grain As content and their varied statistical relationship in high-yielding varieties (Satabdi and Swarna had r = −0.576 and −0.629, respectively) as well as in indigenous varieties (i.e., Bashkathi and Gobindovog (r = 0.314 and 0.423), although non-significant in nature and yet sharing a positive correlation. The contrasting behavior of indigenous rice variety(ies) and high-yielding variety(ies) considered here in terms of Fe accumulation under As stress might be helpful in exploring indigenous rice variety(ies) for future breeding programs. The declining trend in trace element content in brown rice cultivated in As-contaminated soil of As-hotspot areas is corroborated by several research findings (Williams et al., 2009; Dwivedi et al., 2010; Norton et al., 2010). Under As stress conditions, it has been reported that As stress can significantly influence the flowering time and flag leaf area (Moulick et al., 2018a; 2018b). The different flowering time and interconnecting or interaction with site-specific (here in this study) environment during the grain filling period may be responsible for the varied trace element contents in rice grain (Norton et al., 2012; Norton et al., 2014). Genetic revelations through future investigations are necessary to give more insights into assessing the genetic gains for grains conditioned by environmental factors as well as elucidating the rice grain quality traits and mineralogical profile as a product of gene(s)–environment interactions, while genes act as a software and the environment (field conditions) as hardware to produce a specific phenotypic makeup of the grain (Figures 1, 2; Tables 3, 4; Supplementary Table S2). Apart from these, the uptake and the accumulation of various elements (ionome) in the grain that are also influenced by several QTLs are also found to be regulated by environmental factors (Du et al., 2013; Liu et al., 2021). Recently, Tan et al. (2020), after analyzing 11 mineral contents from 575 rice varieties, were able to identify 96 significant association loci (SALs). Upon drawing a correlation between mineral content and SALs, the authors have concluded that, along with the alleles in greater concentration, SALs can effectively influence the mineral content (including Fe, Mn, Zn and Cu) in rice grain, in both japonica and indica origin rice varieties.
The findings from the current investigation presented contrasting responses of rice varieties based on their grain morphology, origin (indigenous or high yielding), and aromaticity— grain quality attributes—as well as mineralogical profile—cultivated in an As-contaminated agro-ecosystem. Apart from these, the influences of other elements and soil components were also visible. At present, the main cultivar groups of rice, like “indica”, “japonica”, and “javanica”, have not been evaluated for their respective grain quality traits when grown neither under controlled nor even in field conditions. The huge numbers of rice germplasm (hybrid, high yielding, and indigenous) are undoubtedly a great challenge indeed for the researchers to document. Moreover, the documentation of the impact of single or multiple stress on the respective grain quality attributes and their subsequent correlation with the trace elemental profile is still in its infancy, though there are alternative sources (apart from rice) that have been constantly under evaluation for their potential to combat “hidden hunger” in a sustainable fashion globally. It is also an obvious fact that rice and rice-based products are feeding the ever-increasing world population as a wholesome serve to date.
Conclusion
The economic aspect of rice is determined in the post-harvest phase after assessing the grain quality attributes (without having a unanimously accepted standard) and governed by the location, consumer’s previous experience, socio-economic background, etc. In the coming decades apart from yield attributes, quality will also be taking its place in the priority list, albeit at a very slow pace. In the near future, the documentation of 1) quality traits under optimum conditions as well as under stressed conditions, 2) the creation of a global database of traits, 3) the screening of rice varieties based on consumers’ preference, 4) the development of rice varieties with desired traits (using conventional breeding, transgenic, CRISPR, etc.) will be expected to get prioritized. The actual goal of this particular investigation is to garner the attention of the scientific community and the policy-makers to afford adequate attention in developing a “rice variety with desirable quality attributes” to be incorporated in the concept of sustainable rice production in the coming years in the “local–regional–global” framework. To do so, a few necessary steps like 1) the development of a rapid quantification method (field-based), 2) documentation of consumers’ preferences, 3) speed-breeding of rice varieties that have desirable quality traits, and 4) multi-locational trials should be encouraged. The policy-making bodies, in various capacities, should encourage the farmers to cultivate the rice varieties with desired quality attributes as well as get feedback from the farmers and the consumers. Apart from these, an interdisciplinary research approach to address the qualitative aspect of rice in the near future should be taken; otherwise, the concept of grain quality attributes can be found in literature, not in reality.
Data Availability Statement
The original contributions presented in the study are included in the article/Supplementary Material, further inquiries can be directed to the corresponding authors.
Author Contributions
DM contributed to conception. DM and DG performed field sampling. DM, DG and MM contributed to analysis. DG and YG contributed to data analysis. DM, DG, MS, YG, MM, SC, JB, SS, MB, PV and AH wrote the manuscript. JB, SS, SC, MB, MS, PV and AH contributed to editing. SS, JB, and SC supervised the study. DM, DG, MS, YG, MM, SC, JB, SS, MB, PV and AH approved the manuscript.
Funding
This study was supported by an S-grant from the Ministry of Education, Youth, and Sports of the Czech Republic, and by the EU Project No. CZ.02.2.69/0.0/0.0/18_054/0014642.
Conflict of Interest
The authors declare that the research was conducted in the absence of any commercial or financial relationships that could be construed as a potential conflict of interest.
Publisher’s Note
All claims expressed in this article are solely those of the authors and do not necessarily represent those of their affiliated organizations or those of the publisher, the editors, and the reviewers. Any product that may be evaluated in this article or claim that may be made by its manufacturer is not guaranteed or endorsed by the publisher.
Acknowledgments
DM, JB, and SS thankfully acknowledge the Department of Environmental Science, University of Kalyani, West Bengal (India) for providing laboratory facilities. DG and YG are thankful to ICAR for the analysis. DM, MM and SC want to acknowledge Assam University. DM is thankful to the Department of Biotechnology, Government of India for providing the prestigious DBT—RA Award in Biotechnology and Life Science.
Supplementary Material
The Supplementary Material for this article can be found online at: https://www.frontiersin.org/articles/10.3389/fenvs.2022.857629/full#supplementary-material
References
Ali, J., Jewel, Z., Mahender, A., Anandan, A., Hernandez, J., and Li, Z. (2018). Molecular Genetics and Breeding for Nutrient Use Efficiency in rice. Ijms 19, 1762. doi:10.3390/ijms19061762
Allard, R. W., and Bradshaw, A. D. (1964). Implications of Genotype‐Environmental Interactions in Applied Plant Breeding 1. Crop Sci. 4, 503–508. doi:10.2135/cropsci1964.0011183x000400050021x
Aluko, G., Martinez, C., Tohme, J., Castano, C., Bergman, C., and Oard, J. H. (2004). QTL Mapping of Grain Quality Traits from the Interspecific Cross Oryza Sativa × O. Glaberrima. Theor. Appl. Genet. 109 (3), 630–639. doi:10.1007/s00122-004-1668-y
Atiaga, O., Nunes, L. M., and Otero, X. L. (2020). Effect of Cooking on Arsenic Concentration in rice. Environ. Sci. Pollut. Res. Int. 27, 10757–10765. doi:10.1007/s11356-019-07552-2
Bao, J. S., Sun, M., and Corke, H. (2002). Analysis of the Genetic Behavior of Some Starch Properties in Indica rice ( Oryza Sativa L.): thermal Properties, Gel Texture, Swelling Volume. Theor. Appl. Genet. 104 (2-3), 408–413. doi:10.1007/s001220100688
Bao, J. S. (2012). Toward Understanding the Genetic and Molecular Bases of the Eating and Cooking Qualities of rice. Cereal Foods World. 57 (4), 148–156. doi:10.1094/cfw-57-4-0148
Bhatti, S. M., Anderson, C. W. N., Stewart, R. B., and Robinson, B. H. (2013). Risk Assessment of Vegetables Irrigated with Arsenic-Contaminated Water. Environ. Sci. Process. Impacts 15, 1866–1875. doi:10.1039/c3em00218g
Bhowmick, S., Nath, B., Halder, D., Biswas, A., Majumder, S., Mondal, P., et al. (2013). Arsenic Mobilization in the Aquifers of Three Physiographic Settings of West Bengal, India: Understanding Geogenic and Anthropogenic Influences. J. Hazard. Mater. 262, 915–923. doi:10.1016/j.jhazmat.2012.07.014
Biswas, J. K., Warke, M., Datta, R., and Sarkar, D. (2020). Is Arsenic in Rice a Major Human Health Concern? Curr. Pollut. Rep 6 (2), 37–42. doi:10.1007/s40726-020-00148-2
Bocianowski, J., Warzecha, T., Nowosad, K., and Bathelt, R. (2019). Genotype by Environment Interaction Using AMMI Model and Estimation of Additive and Epistasis Gene Effects for 1000-kernel Weight in spring Barley (Hordeum Vulgare L.). J. Appl. Genet. 60, 127–135. doi:10.1007/s13353-019-00490-2
Bogdan, K., and Schenk, M. K. (2009). Evaluation of Soil Characteristics Potentially Affecting Arsenic Concentration in Paddy rice (Oryza Sativa L.). Environ. Pollut. 157 (10), 2617–2621. doi:10.1016/j.envpol.2009.05.008
Brammer, H. (2009). Mitigation of Arsenic Contamination in Irrigated Paddy Soils in South and South-East Asia. Environ. Int. 35 (6), 856–863. doi:10.1016/j.envint.2009.02.008
Cagampang, G. B., Perez, C. M., and Juliano, B. O. (1973). A Gel Consistency Test for Eating Quality of rice. J. Sci. Food Agric. 24, 1589–1594. doi:10.1002/jsfa.2740241214
Chatterjee, D., Halder, D., Majumder, S., Biswas, A., Nath, B., Bhattacharya, P., et al. (2010). Assessment of Arsenic Exposure from Groundwater and rice in Bengal Delta Region, West Bengal, India. Water Res. 44 (19), 5803–5812. doi:10.1016/j.watres.2010.04.007
Choudhury, S., Mazumder, M. K., Moulick, D., Sharma, P., Tata, S. K., Gosh, D., et al. (2022). A Computational Study of the Role of Secondary Metabolites for Mitigation of Acid Soil Stress in Cereals Using Dehydroascorbate and Mono-Dehydroascorbate Reductases. Antioxidants 11 (3), 458. doi:10.3390/antiox11030458
Choudhury, S., Moulick, D., and Mazumder, M. K. (2021a). Secondary Metabolites Protect against Metal and Metalloid Stress in rice: an In Silico Investigation Using Dehydroascorbate Reductase. Acta Physiol. Plant 43 (1), 1–10. doi:10.1007/s11738-020-03173-2
Choudhury, S., Sharma, P., Moulick, D., and Mazumder, M. K. (2021b). Unrevealing Metabolomics for Abiotic Stress Adaptation and Tolerance in Plants. J. Crop Sci. Biotechnol. 24, 479–493. doi:10.1007/s12892-021-00102-8
Chowdhury, N. R., Das, A., Joardar, M., De, A., Mridha, D., Das, R., et al. (2020). Flow of Arsenic between rice Grain and Water: Its Interaction, Accumulation and Distribution in Different Fractions of Cooked rice. Sci. Total Environ. 731, 138937. doi:10.1016/j.scitotenv.2020.138937
Cuevas, R. P., de Guia, A., and Demont, M. (2017). Developing a Framework of Gastronomic Systems Research to Unravel Drivers of Food Choice. Int. J. Gastronomy Food Sci. 9, 88–99. doi:10.1016/j.ijgfs.2017.06.001
Custodio, M. C., Cuevas, R. P., Ynion, J., Laborte, A. G., Velasco, M. L., and Demont, M. (2019). Rice Quality: How Is it Defined by Consumers, Industry, Food Scientists, and Geneticists? Trends Food Sci. Technol. 92, 122–137. doi:10.1016/j.tifs.2019.07.039
Das, H. K., Mitra, A. K., Sengupta, P. K., Hossain, A., Islam, F., and Rabbani, G. H. (2004). Arsenic Concentrations in rice, Vegetables, and Fish in Bangladesh: a Preliminary Study. Environ. Int. 30 (3), 383–387. doi:10.1016/j.envint.2003.09.005
Deepa, G., Singh, V., and Naidu, K. A. (2008). Nutrient Composition and Physicochemical Properties of Indian Medicinal rice - Njavara. Food Chem. 106, 165–171. doi:10.1016/j.foodchem.2007.05.062
Demont, M., Rutsaert, P., Ndour, M., and Verbeke, W. (2013). Reversing Urban Bias in African rice Markets: Evidence from Senegal. World Develop. 45, 63–74. doi:10.1016/j.worlddev.2012.11.011
Du, J., Zeng, D., Wang, B., Qian, Q., Zheng, S., and Ling, H.-Q. (2013). Environmental Effects on mineral Accumulation in rice Grains and Identification of Ecological Specific QTLs. Environ. Geochem. Health 35 (2), 161–170. doi:10.1007/s10653-012-9473-z
Dwivedi, S., Tripathi, R. D., Srivastava, S., Singh, R., Kumar, A., Tripathi, P., et al. (2010). Arsenic Affects mineral Nutrients in Grains of Various Indian rice (Oryza Sativa L.) Genotypes Grown on Arsenic-Contaminated Soils of West Bengal. Protoplasma 245 (1-4), 113–124. doi:10.1007/s00709-010-0151-7
Fahad, S., Noor, M., Adnan, M., Khan, M. A., Rahman, I. U., Alam, M., et al. (2019). “Abiotic Stress and rice Grain Quality,” in Advances in rice Research for Abiotic Stress Tolerance (Woodhead Publishing), 571–583.
Fasahat, P., Muhammad, K., Abdullah, A., Rahman, B. M., Siing, N. M., Gauch, J. H., et al. (2014). Genotype× Environment Assessment for Grain Quality Traits in rice. Commun. Biometry Crop Sci. 9 (2), 71–82.
Finlay, K., and Wilkinson, G. (1963). The Analysis of Adaptation in a Plant-Breeding Programme. Aust. J. Agric. Res. 14, 742–754. doi:10.1071/ar9630742
Fitzgerald, M. A., McCouch, S. R., and Hall, R. D. (2009). Not just a Grain of rice: The Quest for Quality. Trends Plant Sci. 14, 133–139. doi:10.1016/j.tplants.2008.12.004
Gabriel, K. R. (1978). Analysis of Meteorological Data by Means of Canonical Decomposition and Biplots. J. Appl. Meteorol. 11, 1072–1077.
Gauch, H. G. (2013). A Simple Protocol for AMMI Analysis of Yield Trials. Crop Sci. 53, 1860–1869. doi:10.2135/cropsci2013.04.0241
Gauch, H. G., Piepho, H.-P., and Annicchiarico, P. (2008). Statistical Analysis of Yield Trials by AMMI and GGE: Further Considerations. Crop Sci. 48 (3), 866–889. doi:10.2135/cropsci2007.09.0513
Gauch, H. G. (2006). Statistical Analysis of Yield Trials by AMMI and GGE. Crop Sci. 46 (4), 1488–1500. doi:10.2135/cropsci2005.07-0193
Gauch, H. G., and Zobel, R. W. (1997). Identifying Mega‐Environments and Targeting Genotypes. Crop Sci. 37, 311–326. doi:10.2135/cropsci1997.0011183x003700020002x
Ghosh, D., Brahmachari, K., Brestic, M., Ondrisik, P., Hossain, A., Skalicky, M., et al. (2020). Integrated Weed and Nutrient Management Improve Yield, Nutrient Uptake and Economics of Maize in the Rice-Maize Cropping System of Eastern India. Agronomy 10 (12), 1906. doi:10.3390/agronomy10121906
Ghosh, D., Brahmachari, K., Skalický, M., Roy, D., Das, A., Sarkar, S., et al. (2022). The Combination of Organic and Inorganic Fertilizers Influence the weed Growth, Productivity and Soil Fertility of Monsoon rice. PLoS ONE17 (1), e0262586. doi:10.1371/journal.pone.0262586
González, N., Calderón, J., Rúbies, A., Bosch, J., Timoner, I., Castell, V., et al. (2020). Dietary Exposure to Total and Inorganic Arsenic via rice and rice-based Products Consumption. Food Chem. Toxicol. 141, 111420. doi:10.1016/j.fct.2020.111420
Graham, R. (2002). A Proposal for IRRI to Establish a Grain Quality and Nutrition Research Centre. IRRI Discussion Paper Series No. 44. Baños, Philippines: International Rice Research Institute, 15. Available at: http://books.irri.org/DPS44_content.pdf [Accessed on: December 22, 2021].
He, Y., Han, Y., Jiang, L., Xu, C., Lu, J., and Xu, M. (2006). Functional Analysis of Starch-Synthesis Genes in Determining rice Eating and Cooking Qualities. Mol. Breed. 18 (4), 277–290. doi:10.1007/s11032-006-5505-7
Hossain, A., Ahmed, S., Ahmad, Z., Alam, M. J., Moulick, D., Saha, B., et al. (2021). “Next-generation Genetic Engineering Tools for Abiotic Stress Tolerance in Plants,” in Frontiers in Plant-Soil Interaction (Academic Press), 153–197.
Hossain, A., Maitra, S., Pramanick, B., Bhutia, K. L., Ahmad, Z., Moulik, D., et al. (2022). “Wild Relatives of Plants as Sources for the Development of Abiotic Stress Tolerance in Plants,” in Plant Perspectives to Global Climate Changes (Academic Press), 471–518. doi:10.1016/b978-0-323-85665-2.00011-x
Huang, Y., Tong, C., Xu, F., Chen, Y., Zhang, C., and Bao, J. (2016). Variation in Mineral Elements in Grains of 20 Brown Rice Accessions in Two Environments. Food Chem. 192, 873–878. doi:10.1016/j.foodchem.2015.07.087
Juliano, B. (1985). “Criteria and Test for rice Grain Quality,” in Rice Chemistry and Technology (Saint Paul: American Association of Cereal Chemists), 443–513.
Juliano, B. O. (1972). Physicochemical Properties of Starch and Protein in Relation to Grain Quality and Nutritional Value of rice. Rice Breed. 5, 389–405.
Kang, M.-Y., Rico, C. W., Kim, C.-E., and Lee, S.-C. (2011). Physicochemical Properties and Eating Qualities of Milled Rice from Different Korean Elite Rice Varieties. Int. J. Food Properties 14 (3), 640–653. doi:10.1080/10942910903312494
Kaosa-ard, M., and Juliano, B. O. (1992). “Assessing Quality Characteristics and price of rice in Selected International Markets,” in Consumer Demand for rice Grain Quality. Editors L. J. Unnevehr, B. Duff, and B. O. Juliano (Manila, Philippines: International Rice Research Institute), 201–219. Retrieved from: http://books.irri.org/9712200302_content.pdf.
Koreňovská, M. (2006). Determination of as, Antimony, and Selenium by FI-HG-AAS in Foods Consumed in Slovakia. J. Food Nutr. Res. 45, 84–88.
Li, Y., Fan, C., Xing, Y., Yun, P., Luo, L., Yan, B., et al. (2014). Chalk5 Encodes a Vacuolar H+-translocating Pyrophosphatase Influencing Grain Chalkiness in rice. Nat. Genet. 46, 398–404. doi:10.1038/ng.2923
Lii, C. Y., and Tsai, M. L. (2000). Effect of Hot-Water-Soluble Components on the Rheological Properties of rice Starch. Starch-Stärke 52 (2-3), 44–53. doi:10.1002/(sici)1521-379x(200004)52:2/3<44::aid-star44>3.0.co;2-t
Liu, H., Long, S. X., Pinson, S. R., Tang, Z., Guerinot, M. L., Salt, D. E., et al. (2021). Univariate and Multivariate QTL Analyses Reveal Covariance Among mineral Elements in the rice Ionome. Front. Genet. 12, 638555. doi:10.3389/fgene.2021.638555
Lu, Y., Adomako, E. E., Solaiman, A. R. M., Islam, M. R., Deacon, C., Williams, P. N., et al. (2009). Baseline Soil Variation Is a Major Factor in Arsenic Accumulation in Bengal Delta Paddy rice. Environ. Sci. Technol. 43, 1724–1729. doi:10.1021/es802794w
Meharg, A. A. (2004). Arsenic in rice - Understanding a New Disaster for South-East Asia. Trends Plant Sci. 9 (9), 415–417. doi:10.1016/j.tplants.2004.07.002
Meng, F., Wei, Y., and Yang, X. (2005). Iron Content and Bioavailability in rice. J. Trace Elem. Med. Biol. 18, 333–338. doi:10.1016/j.jtemb.2005.02.008
Menon, M., Sarkar, B., Hufton, J., Reynolds, C., Reina, S. V., and Young, S. (2020). Do arsenic Levels in rice Pose a Health Risk to the UK Population? Ecotoxicology Environ. Saf. 197, 110601. doi:10.1016/j.ecoenv.2020.110601
Morita, S. (2000). Effects of High Air Temperature on Ripening in Rice Plants. Analysis of Ripening Performance in Growth Chamber Experiments. Jpn. J. Crop Sci. 69 (3), 391–399. doi:10.1626/jcs.69.391
Moulick, D., Chowardhara, B., and Panda, S. K. (2019a). “Agroecotoxicological Aspect of Arsenic (As) and Cadmium (Cd) on Field Crops and its Mitigation: Current Status and Future Prospect,” in Plant-Metal Interactions (Cham: Springer), 217–246.
Moulick, D., Ghosh, D., and Santra, S. C. (2016). An Assessment of Some Physicochemical Properties and Cooking Characteristics of Milled rice and Associated Health Risk in Two rice Varieties of Arsenic Contaminated Areas of West Bengal, India. Int. J. Res. Agric. Food Sci. 6, 44–55.
Moulick, D., Samanta, S., Saha, B., Mazumder, M. K., Dogra, S., Panigrahi, K. C. S., et al. (2020a). “Salinity Stress Responses in Three Popular Field Crops Belonging to Fabaceae Family: Current Status and Future Prospect,” in The Plant Family Fabaceae (Singapore: Springer), 519–541. doi:10.1007/978-981-15-4752-2_20
Moulick, D., Samanta, S., Sarkar, S., Mukherjee, A., Pattnaik, B. K., Saha, S., et al. (2021). Arsenic Contamination, Impact and Mitigation Strategies in rice Agro-Environment: An Inclusive Insight. Sci. Total Environ. 800, 149477. doi:10.1016/j.scitotenv.2021.149477
Moulick, D., Santra, S. C., and Ghosh, D. (2018d). “Consequences of Paddy Cultivation in Arsenic-Contaminated Paddy fields of Lower Indo-Gangetic plain on Arsenic Accumulation Pattern and Selected Grain Quality Traits: A Preliminary Assessment,” in Mechanisms of Arsenic Toxicity and Tolerance in Plants 49-78 (Singapore: Springer). doi:10.1007/978-981-13-1292-2_3
Moulick, D., Santra, S. C., and Ghosh, D. (2018b). Rice Seed Priming with Se: a Novel Approach to Mitigate as Induced Adverse Consequences on Growth, Yield and as Load in Brown rice. J. Hazard. Mater. 355, 187–196. doi:10.1016/j.jhazmat.2018.05.017
Moulick, D., Santra, S. C., and Ghosh, D. (2018a). Effect of Selenium Induced Seed Priming on Arsenic Accumulation in rice Plant and Subsequent Transmission in Human Food Chain. Ecotoxicology Environ. Saf. 152, 67–77. doi:10.1016/j.ecoenv.2018.01.037
Moulick, D., Santra, S. C., Ghosh, D., and Panda, S. K. (2019b). “An Assessment of Efficiency of Zinc Priming in Rice (Cv. MTU-7029) during Germination and Early Seedling Growth,” in Priming and Pretreatment of Seeds and Seedlings (Singapore: Springer), 495–507.
Moulick, D., Santra, S. C., and Ghosh, D. (2017). Seed Priming with Se Alleviate as Induced Phytotoxicity during Germination and Seedling Growth by Restricting as Translocation in rice (Oryza Sativa L c.V. IET-4094). Ecotoxicology Environ. Saf. 145, 449–456. doi:10.1016/j.ecoenv.2017.07.060
Moulick, D., Santra, S. C., and Ghosh, D. (2018c). Seed Priming with Se Mitigates As-Induced Phytotoxicity in rice Seedlings by Enhancing Essential Micronutrient Uptake and Translocation and Reducing as Translocation. Environ. Sci. Pollut. Res. 25 (27), 26978–26991. doi:10.1007/s11356-018-2711-x
Moulick, D., Sarkar, S., Awasthi, J. P., Ghosh, D., Choudhury, S., Tata, S. K., et al. (2020b). “Rice Grain Quality Traits: Neglected or Less Addressed ?,” in Rice Research for Quality Improvement: Genomics and Genetic Engineering (Singapore: Springer), 729–745.
Muthayya, S., Sugimoto, J. D., Montgomery, S., and Maberly, G. F. (2014). An Overview of Global rice Production, Supply, Trade, and Consumption. Ann. N.Y. Acad. Sci. 1324 (1), 7–14. doi:10.1111/nyas.12540
Nelson, J. C., Mcclung, A. M., Fjellstrom, R. G., Moldenhauer, K. A. K., Boza, E., Jodari, F., et al. (2011). Mapping QTL Main and Interaction Influences on Milling Quality in Elite US rice Germplasm. Theor. Appl. Genet. 122, 291–309. doi:10.1007/s00122-010-1445-z
Nguyen, Q. D., Jensen, C. T. B., and Kristensen, P. G. (1998). Experimental and Modelling Studies of the Flow Properties of maize and Waxy maize Starch Pastes. Chem. Eng. Sci. 70, 2,165–171. doi:10.1016/s1385-8947(98)00081-3
Norton, G. J., Deacon, C. M., Xiong, L., Huang, S., Meharg, A. A., and Price, A. H. (2010). Genetic Mapping of the rice Ionome in Leaves and Grain: Identification of QTLs for 17 Elements Including Arsenic, Cadmium, Iron and Selenium. Plant Soil. 329 (1-2), 139–153. doi:10.1007/s11104-009-0141-8
Norton, G. J., Douglas, A., Lahner, B., Yakubova, E., Guerinot, M. L., Pinson, S. R. M., et al. (2014). Genome Wide Association Mapping of Grain Arsenic, Copper, Molybdenum and Zinc in Rice (Oryza Sativa L.) Grown at Four International Field Sites. PLoS One 9 (2), e89685. doi:10.1371/journal.pone.0089685
Norton, G. J., Duan, G., Dasgupta, T., Islam, M. R., Lei, M., Zhu, Y., et al. (2009a). Environmental and Genetic Control of Arsenic Accumulation and Speciation in rice Grain: Comparing a Range of Common Cultivars Grown in Contaminated Sites across Bangladesh, China, and India. Environ. Sci. Technol. 43 (21), 8381–8386. doi:10.1021/es901844q
Norton, G. J., Islam, M. R., Deacon, C. M., Zhao, F.-J., Stroud, J. L., McGrath, S. P., et al. (2009b). Identification of Low Inorganic and Total Grain Arsenic rice Cultivars from Bangladesh. Environ. Sci. Technol. 43 (15), 6070–6075. doi:10.1021/es901121j
Norton, G. J., Pinson, S. R. M., Alexander, J., Mckay, S., Hansen, H., Duan, G. L., et al. (2012). Variation in Grain Arsenic Assessed in a Diverse Panel of rice (Oryza Sativa) Grown in Multiple Sites. New Phytol. 193 (3), 650–664. doi:10.1111/j.1469-8137.2011.03983.x
Puri, S., Dhillon, B., and Sodhi, N. S. (2014). Effect of Degree of Milling (Dom) on Overall Quality of rice—A Review. Int. J. Adv. Biotechnol. Res. 5 (3), 474–489.
Qiu, X., Chen, K., Lv, W., Ou, X., Zhu, Y., Xing, D., et al. (2017). Examining Two Sets of Introgression Lines Reveals Background-independent and Stably Expressed QTL that Improve Grain Appearance Quality in rice (Oryza Sativa L.). Theor. Appl. Genet. 130 (5), 951–967. doi:10.1007/s00122-017-2862-z
Rao, P. S., Mishra, B., and Gupta, S. R. (2013). Effects of Soil Salinity and Alkalinity on Grain Quality of Tolerant, Semi-tolerant and Sensitive rice Genotypes. Rice Sci. 20 (4), 284–291. doi:10.1016/s1672-6308(13)60136-5
Rayment, G. E., and Lyons, D. J. (2012). New, Comprehensive Soil Chemical Methods Book for Australasia. Commun. Soil Sci. Plant Anal. 43 (1-2), 412–418. doi:10.1080/00103624.2012.641802
Ritika, B. Y., Khatkar, B. S., and Yadav, B. S. (2010). Physicochemical, Morphological, Thermal and Pasting Properties of Starches Isolated from Rice Cultivars Grown in India. Int. J. Food Properties 13 (6), 1339–1354. doi:10.1080/10942910903131407
Roy Chowdhury, N., Ghosh, S., Joardar, M., Kar, D., and Roychowdhury, T. (2018). Impact of Arsenic Contaminated Groundwater Used during Domestic Scale post Harvesting of Paddy Crop in West Bengal: Arsenic Partitioning in Raw and Parboiled Whole Grain. Chemosphere 211, 173–184. doi:10.1016/j.chemosphere.2018.07.128
Saha, B., Chowardhara, B., Kar, S., Devi, S. S., Awasthi, J. P., Moulick, D., et al. (2019). Advances in Heavy Metal-Induced Stress Alleviation with Respect to Exogenous Amendments in Crop Plants, 313–332.doi:10.1007/978-981-13-8625-1_15
Sahoo, S., Borgohain, P., Saha, B., Moulick, D., Tanti, B., and Panda, S. K. (2019). “Seed Priming and Seedling Pre-treatment Induced Tolerance to Drought and Salt Stress: Recent Advances,” in Priming and Pretreatment of Seeds and Seedlings. Editors M. Hasanuzzaman, and V. Fotopoulos. Singapore: Springer. doi:10.1007/978-981-13-8625-1_12
Saleh, M., and Meullenet, J.-F. (2015). Cooked rice Texture and rice Flour Pasting Properties; Impacted by rice Temperature during Milling. J. Food Sci. Technol. 52 (3), 1602–1609. doi:10.1007/s13197-013-1180-y
Sandstead, H. H. (1991). Zinc Deficiency. Am. J. Dis. Child. 145, 853–859. doi:10.1001/archpedi.1991.02160080029016
Santra, S. C., and Samal, A. C. (2013). Arsenic Scenario in Gangetic delta of West Bengal: Risk and Management. Development 25, 27.
Shinde, Y. H., Vijayadwhaja, A., Pandit, A. B., and Joshi, J. B. (2014). Kinetics of Cooking of rice: a Review. J. Food Eng. 1, 113–129. doi:10.1016/j.jfoodeng.2013.09.021
Siddiqui, S. U., Kumamaru, T., and Satoh, H. (2007). Pakistan rice Genetic Resources-I: Grain Morphological Diversity and its Distribution. Pak. J. Bot. 39 (3), 841–848.
Siebenmorgen, T. J., and Lanning, S. B. (2014). “Assessing rice Milling Quality,” in Rice Processing; the Comprehensive Guide to Global Rice Technology and Innovative Products. Editor J Sontag (Agrimedia, Germany: Chap), 141–153.
Singh, N., Kaur, L., Singh Sodhi, N., and Singh Sekhon, K. (2005). Physicochemical, Cooking and Textural Properties of Milled rice from Different Indian rice Cultivars. Food Chem. 89 (2), 253–259. doi:10.1016/j.foodchem.2004.02.032
Singh, N., Pal, N., Mahajan, G., Singh, S., and Shevkani, K. (2011). Rice Grain and Starch Properties: Effects of Nitrogen Fertilizer Application. Carbohydr. Polym. 86 (1), 219–225. doi:10.1016/j.carbpol.2011.04.039
Takahashi, Y., Minamikawa, R., Hattori, K. H., Kurishima, K., Kihou, N., and Yuita, K. (2004). Arsenic Behavior in Paddy fields during the Cycle of Flooded and Non-flooded Periods. Environ. Sci. Technol. 38 (4), 1038–1044. doi:10.1021/es034383n
Tan, Y., Sun, L., Song, Q., Mao, D., Zhou, J., Jiang, Y., et al. (2020). Genetic Architecture of Subspecies Divergence in Trace mineral Accumulation and Elemental Correlations in the rice Grain. Theor. Appl. Genet. 133 (2), 529–545. doi:10.1007/s00122-019-03485-z
Tran, N. A., Daygon, V. D., Resurreccion, A. P., Cuevas, R. P., Corpuz, H. M., and Fitzgerald, M. A. (2011). A Single Nucleotide Polymorphism in the Waxy Gene Explains a Significant Component of Gel Consistency. Theor. Appl. Genet. 123 (1234), 519–525. doi:10.1007/s00122-011-1604-x
Umeta, M., West, C. E., Haidar, J., Deurenberg, P., and Hautvast, J. G. (2000). Zinc Supplementation and Stunted Infants in Ethiopia: a Randomised Controlled Trial. The Lancet 355, 2021–2026. doi:10.1016/s0140-6736(00)02348-5
United Nations (2017) World Population Prospects: The 2017 Revision, Key Findings and Advance Tables”. Working Paper No.ESA/P/WP/248/2017, Department of Economic and SocialAffairs, Population Division. UN, New York
Upadhyay, M. K., Majumdar, A., Barla, A., Bose, S., and Srivastava, S. (2019). An Assessment of Arsenic hazard in Groundwater-Soil-rice System in Two Villages of Nadia District, West Bengal, India. Environ. Geochem. Health 41 (6), 2381–2395. doi:10.1007/s10653-019-00289-4
Wada, H., Masumoto-Kubo, C., Gholipour, Y., Nonami, H., Tanaka, F., Erra-Balsells, R., et al. (2014). Rice Chalky Ring Formation Caused by Temporal Reduction in Starch Biosynthesis during Osmotic Adjustment under Foehn-Induced Dry Wind. PLoS One 9 (10), e110374. doi:10.1371/journal.pone.0110374
Walkey, A., and Black, T. A. (1934). An Examination of the Dugtijaraff Method for Determining Soil Organic Matter and Proposed Modification of the Chronic and Titration Method. Soil Sci. 37, 23–38.
Wang, X., Pang, Y., Wang, C., Chen, K., Zhu, Y., Shen, C., et al. (2017). New Candidate Genes Affecting rice Grain Appearance and Milling Quality Detected by Genome-wide and Gene-Based Association Analyses. Front. Plant Sci. 7, 1998. doi:10.3389/fpls.2016.01998
Williams, P. N., Islam, M. R., Adomako, E. E., Raab, A., Hossain, S. A., Zhu, Y. G., et al. (2006). Increase in Rice Grain Arsenic for Regions of Bangladesh Irrigating Paddies with Elevated Arsenic in Groundwaters. Environ. Sci. Technol. 40 (16), 4903–4908. doi:10.1021/es060222i
Williams, P. N., Islam, S., Islam, R., Jahiruddin, M., Adomako, E., Soliaman, A. R. M., et al. (2009). Arsenic Limits Trace mineral Nutrition (Selenium, Zinc, and Nickel) in Bangladesh rice Grain. Environ. Sci. Technol. 43 (21), 8430–8436. doi:10.1021/es901825t
Xie, L., Tang, S., Chen, N., Luo, J., Jiao, G., Shao, G., et al. (2013). Rice Grain Morphological Characteristics Correlate with Grain Weight and Milling Quality. Cereal Chem. J. 90, 587–593. doi:10.1094/cchem-03-13-0055-r
Yadav, R. B., Khatkar, B. S., and Yadav, B. S. (2007). Morphological, Physicochemical and Cooking Properties of Some Indian rice (Oryzasativa L.) Cultivars. J. Agri. Technol. 3 (2), 203–210.
Yan, B., Tondi Yacouba, N., Chen, J., Wang, Y., Gao, G., Zhang, Q., et al. (2014). Analysis of Minor Quantitative Trait Loci for Eating and Cooking Quality Traits in rice Using a Recombinant Inbred Line Population Derived from Two Indica Cultivars with Similar Amylose Content. Mol. Breed. 34 (4), 2151–2163. doi:10.1007/s11032-014-0170-8
Yan, W., Hunt, L. A., Sheng, Q., and Szlavnics, Z. (2000). Cultivar Evaluation and Mega-Environment Investigation Based on the GGE Biplot. Crop Sci. 40 (3), 597–605. doi:10.2135/cropsci2000.403597x
Yang, L., and Wang, Y. (2019). “Impact of Climate Change on rice Grain Quality,” in Rice. Editor J. Bao (New Orleans, LA: Elsevier Inc.), 427–441. doi:10.1016/b978-0-12-811508-4.00013-7
Yu, S., Ali, J., Zhang, C., Li, Z., and Zhang, Q. (2020). Genomic Breeding of green Super rice Varieties and Their Deployment in Asia and Africa. Theor. Appl. Genet. 133 (5), 1427–1442. doi:10.1007/s00122-019-03516-9
Yu, T. Q., Jiang, W., Ham, T. H., Chu, S. H., Lestari, P., Lee, J. H., et al. (2008). Comparison of Grain Quality Traits between Japonica rice Cultivars from Korea and Yunnan Province of China. J. Crop Sci. Biotechnol. 11 (2), 135–140.
Zeng, Y., Tan, X., Zeng, Y., Xie, X., Pan, X., Shi, Q., et al. (2019). Changes in the rice Grain Quality of Different High-Quality rice Varieties Released in Southern China from 2007 to 2017. J. Cereal Sci. 87, 111–116. doi:10.1016/j.jcs.2019.03.015
Zhang, M., Pinson, S. R. M., Tarpley, L., Huang, X.-Y., Lahner, B., Yakubova, E., et al. (2014). Mapping and Validation of Quantitative Trait Loci Associated with Concentrations of 16 Elements in Unmilled rice Grain. Theor. Appl. Genet. 127, 137–165. doi:10.1007/s00122-013-2207-5
Zhao, X., and Fitzgerald, M. (2013). Climate Change: Implications for the Yield of Edible rice. PloS one. 8 (86), e66218. doi:10.1371/journal.pone.0066218
Zhao, X., Daygon, V. D., McNally, K. L., Hamilton, R. S., Xie, F., Reinke, R. F., et al. (2016). Identification of Stable QTLs Causing Chalk in rice Grains in Nine Environments. Theor. Appl. Genet. 129 (1), 141–153. doi:10.1007/s00122-015-2616-8
Zheng, T. Q., Xu, J. L., Li, Z. K., Zhai, H. Q., and Wan, J. M. (2007). Genomic Regions Associated with Milling Quality and Grain Shape Identified in a Set of Random Introgression Lines of rice (Oryza Sativa L.). Plant Breed. 126 (2), 158–163. doi:10.1111/j.1439-0523.2007.01357.x
Zhou, Z., Robards, K., Helliwell, S., and Blanchard, C. (2002). Composition and Functional Properties of rice. Int. J. Food Sci. Tech. 37 (8), 849–868. doi:10.1046/j.1365-2621.2002.00625.x
Keywords: rice, arsenic, grain quality traits, micronutrients, AMMI model, genotype, environment
Citation: Moulick D, Ghosh D, Skalicky M, Gharde Y, Mazumder MK, Choudhury S, Biswas JK, Santra SC, Brestic M, Vachova P and Hossain A (2022) Interrelationship Among Rice Grain Arsenic, Micronutrients Content and Grain Quality Attributes: An Investigation From Genotype × Environment Perspective. Front. Environ. Sci. 10:857629. doi: 10.3389/fenvs.2022.857629
Received: 18 January 2022; Accepted: 14 February 2022;
Published: 07 April 2022.
Edited by:
Hai Ocean Xu, Jiangsu University, ChinaReviewed by:
Munish Kumar Upadhyay, Banaras Hindu University, IndiaSuprasanna Penna, Bhabha Atomic Research Centre (BARC), India
Copyright © 2022 Moulick, Ghosh, Skalicky, Gharde, Mazumder, Choudhury, Biswas, Santra, Brestic, Vachova and Hossain. This is an open-access article distributed under the terms of the Creative Commons Attribution License (CC BY). The use, distribution or reproduction in other forums is permitted, provided the original author(s) and the copyright owner(s) are credited and that the original publication in this journal is cited, in accordance with accepted academic practice. No use, distribution or reproduction is permitted which does not comply with these terms.
*Correspondence: Milan Skalicky, skalicky@af.czu.cz; Akbar Hossain, akbar.hossain@bwmri.gov.bd
†These authors have contributed equally to this work