- 1School of Geographical Sciences/Hebei Key Laboratory of Environmental Change and Ecological Construction, Hebei Normal University, Shijiazhuang, China
- 2Institute of Geographical Sciences, Hebei Academy Sciences/Hebei Engineering Research Center for Geographic Information Application, Shijiazhuang, China
The Agro-Pastoral Ecotone of Northern China (APEC) is a transitional area suffering from severe wind erosion. The wind data used in wind erosion modeling generally have different temporal resolutions and spatial station distributions. Previous studies have suggested that the temporal wind speed resolution influences the prediction of wind erosion events at the field scale. To date, no studies have been conducted to assess the impact of the type of wind data on regional wind erosion estimation. In this study, the Revised Wind Erosion Equation (RWEQ) and the Integrated Wind Erosion Modeling System (IWEMS) were used to evaluate the regional potential wind erosion in the Agro-Pastoral Ecotone of Northern China (APEC) during 2000 and 2012 based on four wind data type scenarios, including basic weather stations with daily wind statistics, basic weather stations with four wind speed measurements per day, reference climatological stations with daily wind statistics, and reference climatological stations with four wind speed measurements per day. The principal results reveal that the potential wind erosion estimates evaluated using the two models are closely correlated with the measured wind erosion data reported in the published literature, but the predicted values are generally lower than the observed values for the different scenarios. The magnitudes of the mean potential wind erosion ranged from 15.73 to 27.33 t ha−1 a−1 by RWEQ and changed between 61.77 and 98.54 t ha−1 a−1 by IWEMS for different scenarios. The spatial distribution and temporal trends of the annual or seasonal potential wind erosion obtained using the two models were similar for the different scenarios. This study revealed that wind speed is the most sensitive input, and hourly wind speed generated by the different temporal interpolation can significantly affect regional wind erosion estimation. Some studies involving precise regional wind erosion estimation, such as the impacts of landscape changes (land use/cover) on wind erosion, ecosystem service evaluation of reducing soil erosion, soil carbon sequestration and emissions through wind erosion, and wind erosion induced surface soil nutrient loss (e.g., nitrogen and phosphorus), may have been influenced by conducting regional wind erosion modeling based on different types of wind data. The users need to calibrate and validate the selected models for precise wind erosion prediction.
Introduction
Land degradation due to wind-induced soil erosion is an important surface process in arid and semi-arid regions (Dong and wang, 2000; Song et al., 2005; Guo et al., 2014; Webb et al., 2020; Borrelli et al., 2021). In China, according to the First National Bulletin on Water and Soil Conservation in the National Water Resources Survey (MWRPRC, 2013), the total land area affected by wind erosion is approximately 165.59 × 104 km2, accounting for 17.24% of the national territory. Wind erosion has a significant impact on agricultural activities and human beings (Zobeck et al., 2000). Therefore, determining a method of reducing the damage caused by wind erosion is an important challenge for governments in arid and semi-arid areas (O’Loingsigh et al., 2014; Du et al., 2015a).
Many wind erosion models have been developed to quantify wind erosion since the 1960s. These models mainly include the Wind Erosion Equation (WEQ) (Woodruff and Siddoway, 1965), the Revised Wind Erosion Equation (RWEQ) (Fryrear et al., 2000), the Wind Erosion Prediction System (WEPS) (Hagen, 1991), the Texas Tech Erosion Analysis Model (TEAM) (Gregory et al., 2004), the Wind Erosion on European Light Soil (WEELS) (Böhner et al., 2003), and the Wind Erosion Stochastic Simulator (WESS) (Potter et al., 1998) at the field scale, and the Integrated Wind-Erosion Modeling System (IWEMS) (Lu and Shao, 2001; Shao, 2001) and the AUStralian Land Erodibility Model (AUSLEM) (Webb et al., 2009) at a regional scale. Basically, these models include empirical (e.g., WEQ, RWEQ) or physical scheme (e.g., WEPS, IWEMS). In China, several studies have been conducted to build wind erosion models for different target regions at various scales since the 1990s (e.g., Dong, 1998; Zhao et al., 2011; Zou et al., 2015). To meet the demand of the first national wind erosion survey of China, the National Wind Erosion Survey Model of China (NWESMC) was developed in 2012 (Li et al., 2013). Wind erosion models play an important role in evaluating regional on-site wind erosion and off-site dust emissions. The inputs of a wind erosion model include soil properties (e.g., soil texture and moisture), meteorological factors (e.g., wind speed and precipitation), ground surface characteristics (e.g., roughness and vegetation cover), and anthropogenic activities (e.g., land management) (Wang et al., 2018; Fattahi et al., 2020; Zhang et al., 2020; Liu et al., 2021). Wind data (speed, direction, and turbulence) are generally considered to be the most sensitive parameters in wind erosion modeling (Lin et al., 2020; Webb et al., 2020).
In China, there are 2425 meteorological observing stations (reference and basic stations), of which 756 are national reference climatological stations (reference stations) (MBPRC, 2019). Correspondingly, previous wind erosion modeling studies have used either the wind data from the basic (e.g., Chi et al., 2019; Wang et al., 2020) or reference (e.g., Guo et al., 2013; Du et al., 2015a; Du et al., 2018) stations. Evaluations of regional wind erosion require a detailed representation of the wind data. However, only limited wind data (e.g., daily wind statistics) are available for many locations where wind erosion is occurring (van Donk et al., 2008; Guo et al., 2012; Liu et al., 2013). Consequently, the daily wind statistics and four wind records per day documented at meteorological observing stations have been extensively used in regional wind erosion research. For instance, the NWESMC and RWEQ were used to estimate the potential wind erosion in the Agro-Pastoral Ecotone of Northern China (APEC) using four wind records per day (Guo et al., 2013; Wang et al., 2020). The average and maximum wind speeds per day were used to predict the soil loss due to wind in Northern China (Du et al., 2015a; Du et al., 2018). The daily average wind speed was used to assess the wind erosion in Inner Mongolia, China (Lyu et al., 2021). To improve the accuracy of wind erosion modeling, several methods have been developed to generate detailed wind data (e.g., hourly wind speeds) from limited wind data (e.g., daily wind statistics) (e.g., Skidmore and Tatarko, 1990; van Donk et al., 2008; Donatelli et al., 2009; Yuan et al., 2018). Further studies have also revealed that the averaging time has a significant impact on wind erosion estimations, and it may influence single-event and period (e.g., seasonal and annual) wind erosion evaluations (van Donk et al., 2005; Panebianco and Buschiazzo, 2013; Guo et al., 2016; Yizhaq et al., 2020). Generally, the effect of the type of wind data on regional wind erosion modelling has been neglected. In this study, the widely-used empirical RWEQ and process-based IWEMS were selected to explore how the type of wind data (basic weather/reference climatological stations and wind data with different temporal resolutions) influences regional potential wind erosion estimation.
Materials and Methods
Study Area
The APEC is located in the transition zone between the monsoon region in eastern China and the arid and semi-arid regions in northwestern China (Guo et al., 2013). The APEC includes parts of Inner Mongolia, Liaoning, Jilin, Hebei, Shanxi, Shaanxi, and Ningxia Provinces (36°30′–46°42′N, 106°16′–124°51′E) (Figure 1B). Most of the APEC is semi-arid. The annual precipitation distribution is uneven and is concentrated in summer and autumn. The average annual precipitation is 300–450 mm and the annual average temperature is 2.4–11.5°C. Strong winds are frequent and mainly occur in spring (March–April–May). The annual average wind speed ranges from 1.3 to 3.9 m s−1, with an average maximum wind speed of 16–24 m s−1. The land uses are diverse and the sandy land (including the Mu Us Sandy Land, Hunshan Dake Sandy Land, and Horqin Sandy Land), grassland, and farmland have staggered distributions in the APEC. In addition, agricultural and animal husbandry activities are still intensive in some region with a fragile ecology, and the human activities and severe wind erosion continuously decrease the local soil productivity in these areas.
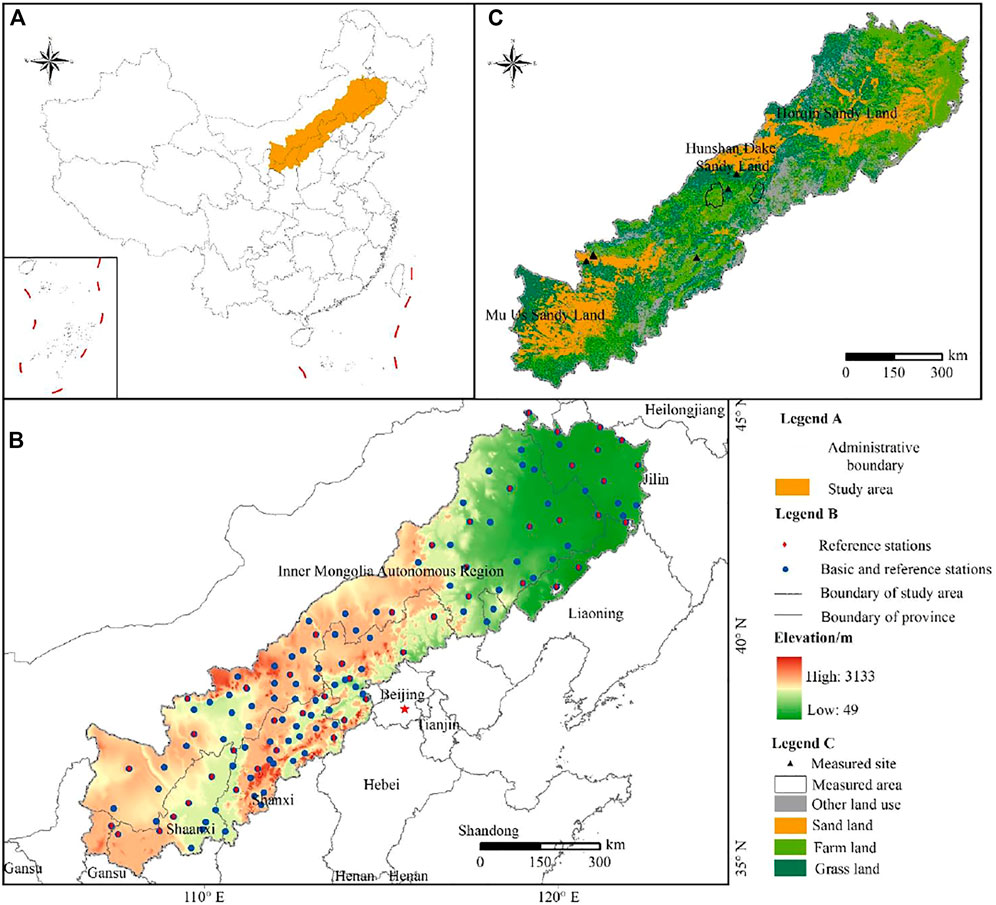
FIGURE 1. (A) Location of the Agricultural Pastoral Ecotone in northern China (APEC); (B) Distribution of the reference climatological stations and basic weather stations; and (C) Distribution of the measured wind erosion sites from published documents.
Data Preparation
In this study, we collected meteorological data, vegetation data, land uses/cover data, Digital Elevation Model (DEM) data, and soil data to calculate the wind erosion in the APEC (Table 1). The data from all of the meteorological stations from 2000 to 2012 were obtained from the China Meteorological Science Data Sharing Service Network (http://data.cma.cn). These meteorological data included wind speed, temperature, precipitation, sunshine duration, and other variables. Two wind databases are available, one dataset contains four wind records per day (UTC+8 2:00, UTC+8 8:00, UTC+8 14:00, and UTC+8 20:00), and the other contains two wind records (average and maximum). The hourly wind speed data were generated from the four wind records per day using the linear interpolation method (Li et al., 2013) and from the two wind records per day using WINDGEN (Skidmore and Tatarko, 1990). The Normalized Difference Vegetation Index (NDVI) data were derived from Moderate Resolution Imaging Spectroradiometer (MODIS) data products obtained from the United States Geological Survey’s website (https://www.usgs.gov), and the MOD13A2, MOD11A2, and MOD09A1 products were mainly used. The 1 km resolution land use/cover data of 2010 was obtained from the Resource and Environment Data Center, Chinese Academy of Sciences (http://www.resdc.cn). The DEM data (China 1 km Low Range Model Data Set) were provided by the Science Data Centre for Cold and Arid Areas (http://westdc.westgis.ac.cn). The soil data were mainly obtained from the world soil database (HWSD) and were provided by the Scientific Data Center (http://westdc.westgis.ac.cn) for Cold and Arid Regions. These data were used to calculate the surface soil factors in the different regions. Farmland, grassland and sands (or desert) were selected to evaluate regional potential wind erosion.
Model Description
RWEQ Model
The RWEQ was proposed by the United States Department of Agriculture (USDA) to estimate soil wind erosion in farmland in the United States (Fryrear et al., 1998). The RWEQ is an empirical wind model that has been widely used to estimate wind erosion at the field scale. The main factors include climatic parameters, soil properties, surface roughness, and vegetation cover (Fryrear et al., 2000; Fryrear et al., 2001). The principal equations of the RWEQ are (Fryrear et al., 2000)
where
The weather factor (WF) can be calculated using:
where U2 is the wind speed (m s-1) at a height of 2 m, and it can be converted from the wind speed observed at standard anemometer heights using Elliot’s method (Elliot, 1979). Ut is the threshold wind speed (m s-1) at a height of 2 m, and the threshold wind speed is assumed to be 5 m s-1 in the RWEQ (Fryrear et al., 2000). N is the number of wind speed observations during the period; Nd is the number of observation days in the study; g is the acceleration of gravity (m s-2); SW is the soil moisture factor (dimensionless); and SD is the snow-cover factor (dimensionless).
The soil erodible factor (EF) and the soil crust factor (SCF) are calculated using Eqs 5, 6:
where Sa is the sand content of the soil (%), Si is the silt content of the soil (%), Sa/Cl is the ratio of the sand to clay contents of the soil (%), OM is the organic-matter content of the soil (%), and CaCO3 is the calcium carbonate content of the soil (%).
The combined vegetation factor (C) is the product of three factors: the crop canopy (SLRc), flat-residues factor (SLRf), and standing residues factor (SLRs). However, the crop residues are normally used and most of the agricultural land is exposed in the research area (Guo et al., 2013). Therefore, the combined vegetation factor (C) was adjusted and is expressed as follows:
where cc is the growing vegetation cover.
The surface roughness factors include the directional roughness and random roughness. Tillage measures produce roughness, while climatic factors such as rainfall gradually reduce the roughness factors. The surface roughness factor is calculated as follows (Shen et al., 2016):
where α is the slope of the terrain, which was extracted from DEM data (Table 1) using ArcGIS 10.2.
IWEMS Model
The Integrated Wind Erosion Modelling System (IWEMS) was proposed by Shao (2001). This model has been widely used to estimate the wind erosion in China (Song, 2004; Du et al., 2018). The horizontal saltation flux
where Ac is the fraction of the erodible area, and
The threshold fraction velocity
where u*t (ds;λ;θ) (m s−1) is the threshold friction velocity of sand particles with diameter
The
where ρa and ρp are the densities of the air and sand particles, 1.29 and 2,600 kg m−3, respectively; g is the acceleration of gravity (9.8 m s−2);
The roughness element correction function
where
The soil moisture correction function fω (θ) was calculated using the simple method proposed by Fecan et al. (1998):
where
The typical value of
where
Sensitivity Analysis
Sensitivity analysis is generally used to determine the main sensitive factors of a wind and water erosion model (Hagen et al., 1999; Feng and Sharratt, 2005). The sensitivity parameter, SS, is calculated by:
where I1 and I2 are the least and greatest values of input parameters; O1 and O2 are the output values correspond to inputs values; I12 and O12 are the average of input and output values.
Temporal Interpolation of Wind Speed
WINDGEN (Wind-generator) is a subsystem of WEPS developed by United States Department of Agriculture (Skidmore and Tatarko, 1990). WINDGEN is a sub-daily wind speed generator and can reproduce the hourly wind speed. Wind speed for any hour of the day u(i) can be simulated by:
where u(i) is the wind speed for any hour (i) of the day; urep is the daily mean representative wind speed; umax and umin are the maximum and minimum wind speed for the day; hmax is the hour of the day when wind speed is maximum.
Linear interpolation method refers to the wind speed estimation data obtained 24 times per day by calculating the wind speed at 2 adjacent integer points, and the equation is expressed as (Li et al., 2013):
where t1 and t2 are the hours of two adjacent wind speed in four wind speeds on day (02:00, 08:00, 14:00 and 20:00); ti is the hour between t1 and t2; uti, ut1 and ut2 are the wind speed correspond to ti, t1 and t2.
Scenario
In the APEC, there are 130 meteorological observing stations (reference and basic stations), of which 47 are reference climatological stations (reference stations). The four wind speeds per day (UTC+8 2:00, UTC+8 8:00, UTC+8 14:00, and UTC+8 20:00) or the daily wind statistics (average and maximum) per day are generally available in China. Based on the characteristics of the available wind data, four scenarios regarding the selection of the type of wind data were used to model the regional potential wind erosion (Table 2). In Scenario 1, 130 meteorological observing stations (reference and basic stations) with daily average and maximum wind speed data were used. In Scenario 2, 130 meteorological observing stations (reference and basic stations) with four wind records per day were used. In Scenario 3, 47 reference climatological stations (reference stations) with daily average and maximum wind speed data were used. In Scenario 4, 47 reference climatological stations (reference stations) with four wind records per day were used. The “Standards for Classification and Gradation of Soil Erosion” were used to classify the potential wind erosion hazard (weak, slight, moderate, severe, very severe, and catastrophic) (MWRPRC, 2007).
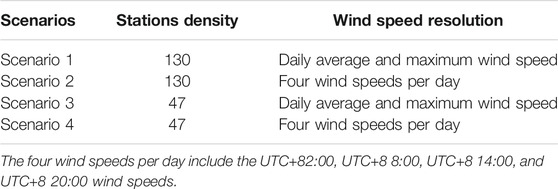
TABLE 2. The spatial resolution of the climatological stations and temporal resolution of the wind speed for different scenarios.
Results
Sensitivity Analysis of Model Parameters
The parameters testing, the base values, the input values, and the sensitivity values are presented in Table 3. Overall, wind speed is the most sensitive parameters of the two models. Generally, wind erosion intensity is remarkably affected by threshold wind speed. The wind speed data with high spatial and temporal resolution is thus important for regional wind erosion simulation. Besides wind speed, for RWEQ, snow cover and vegetation cover can effectively control wind erosion, and the climatic factors have apparent seasonality. Several soil properties, such as the clay content and soil moisture can also reduce the wind erosion. However, the least sensitivity was observed for the inputs of temperature, precipitation days and sunshine time. For IWEMS, the wind speed is also the most sensitive inputs for wind erosion modeling.
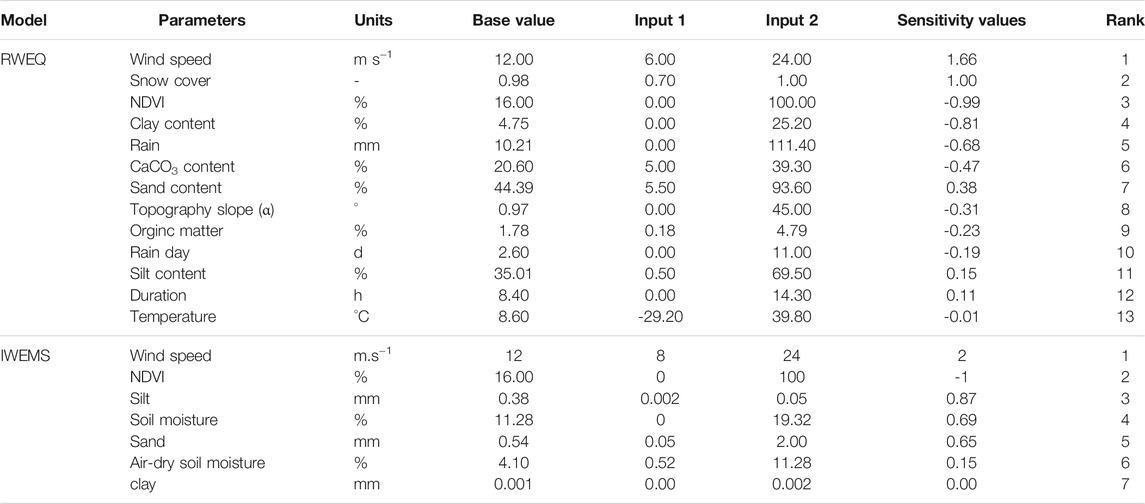
TABLE 3. The values of testing parameters, base values, input values and sensitivity values in RWEQ and IWEMS.
Potential Wind Erosion for Different Scenarios
The average potential wind erosion values from 2000 to 2012 for the different scenarios are presented in Figure 2A. For the RWEQ, the highest average potential wind erosion values were 27.33, 22.12, 19.34, and 15.73 t hm−2 a−1 for scenarios 1, 2, 3, and 4, respectively. For the IWEMS, the average annual potential wind erosion values were 98.54, 84.73, 69.46, and 61.77 t hm−2 a−1 for scenarios 1, 2, 3, and 4, respectively. As is shown in Figure 2B, most of the severe wind erosion occurred in spring and the wind erosion was the lowest in summer. Table 4 presents the potential wind erosion with different erosion hazards from 2000 to 2012 for the different scenarios. For the RWEQ, the percentage of the area with weak and slight erosion hazards accounted about 80% of the total area. The percentages of the areas with weak erosion hazards were 27.52, 41.10, 39.43, and 47.82% for scenarios 1, 2, 3, and 4, respectively, and the percentages of the areas with slight erosion hazards were 50.75, 39.21, 45.36, and 38.97%, respectively. For the IWEMS, the areas with weak and slight erosion hazards were remarkably smaller than those for the RWEQ, and the weak and slight erosion hazard areas occupied the large proportion (∼50%) of the total area. In contrast, the percentages of the areas with severe, very severe, and catastrophic erosion hazards were high for all of the scenarios, i.e., 41.37, 32.82, 33.07, and 25.83% for scenarios 1, 2, 3, and 4, respectively. The areas with severe, very severe, and catastrophic erosion hazards decreased and the areas with weak and slight erosion hazards increased from scenario 1 to scenario 4. In summary, weak and slight erosion hazards were predominant in the APEC. Moreover, the potential wind erosion estimated using the IWEMS model is higher than that estimated using the RWEQ, and the four scenarios produce different results.
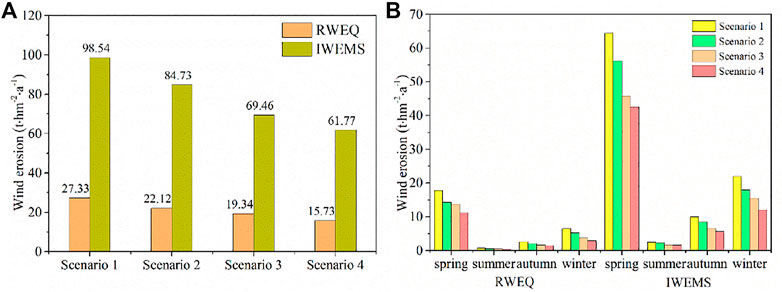
FIGURE 2. (A) Average potential wind erosion estimated using the RWEQ and IWEMS for different scenarios. (B) Seasonal distribution of the average potential wind erosion estimated using the RWEQ and IWEMS for different scenarios.
Spatiotemporal Distribution of Potential Wind Erosion
Figure 3 shows the spatial patterns of the potential wind erosion estimated using the RWEQ and IWEMS for the different scenarios. For the RWEQ, the area of very severe and catastrophic erosion hazards markedly decreased from scenarios 1 to 4 in the Horqin Sandy Land and the Hunshan Dake Sandy Land, and no catastrophic erosion hazards were scattered in the Hunshan Dake Sandy Land for scenario 4. Moderate erosion hazards dominated the Mu Us Sandy Land for all of the scenarios, and severe erosion hazards occurred in small regions for scenarios 1 and 2. For the IWEMS, the Horqin Sandy Land, the Hunshan Dake Sandy Land, and the Mu Us Sandy Land exhibited very severe and catastrophic erosion hazards for all of the scenarios. The very severe and catastrophic erosion hazards decreased remarkably from scenarios 1 to 4 in the Horqin Sandy Land and the Hunshan Dake Sandy Land. In summary, the geographic distributions of the potential wind erosion were similar, and the wind erosion hotspots were mainly located in the Horqin Sandy Land, the Hunshan Dake Sandy Land, and the Mu Us Sandy Land.
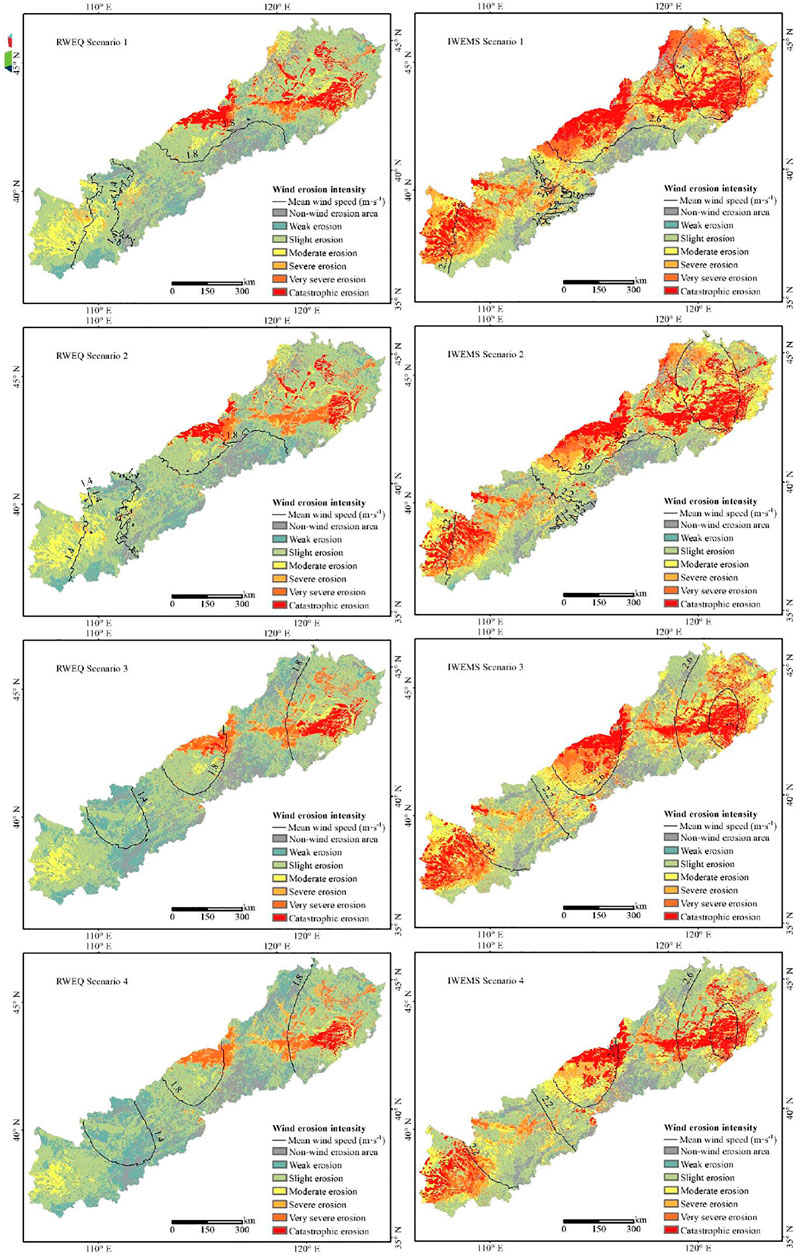
FIGURE 3. Spatial distribution of the wind erosion hazards for the different scenarios. Note: Mean wind speed in RWEQ at 2 m height and in IWEMS at 10 m height.
The results show that there were seasonal variations and annual fluctuations in the potential wind erosion. Figure 2B shows the average seasonal potential wind erosion in the APEC. Wind erosion occurred during all of the seasons, and the seasonal variation of the potential wind erosion was as follows for all of the scenarios: spring > winter > autumn > summer. The potential wind erosion exhibited obvious annual fluctuations from 2000 to 2012 (Figure 4). The trends of the inter-annual variations in the average potential wind erosion were similar between 2000 and 2012 for all of the scenarios. The average potential wind erosion was the highest in 2001 for all of the scenarios; and it was the lowest in 2011, except for scenarios 3 and 4 for the IWEMS, in which they occurred in 2005 and 2008, respectively. Overall, the potential wind erosion significantly decreased from 2000 to 2012.
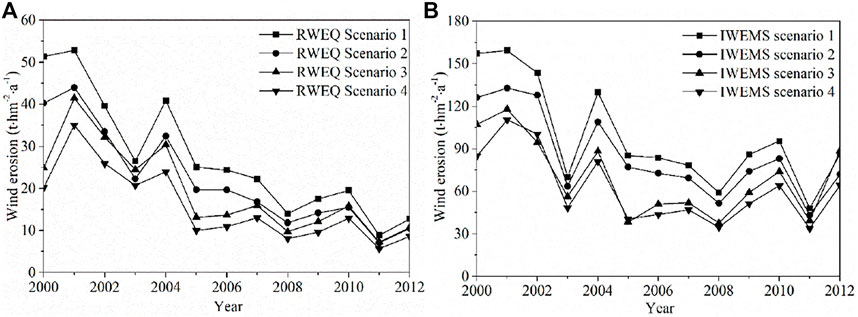
FIGURE 4. Inter-annual variations in the average potential wind erosion obtained using (A) the RWEQ and (B) IWEMS for the different scenarios.
Discussion
Model Applicability and Verification
Several methods often employed in soil wind erosion evaluation, particularly the common radioisotope 137Cs monitoring method have high degree of precision for wind erosion monitoring in field surface experiments and observations in different model (Gharibreza et al., 2020). The verification of the models was investigated based on collected measured wind erosion data. Here, the seven sites and two regional areas of measured wind erosion were obtained using radioisotope 137Cs method from the literatures (Tables 5, 6). The locations of the observation are presented in Figure 1C. We compared our results in all scenarios with previous findings by using radioisotope 137Cs method. From Table 5, the simulated results were generally in agreement with the wind erosion sites reported in the literature in two models. For all the sites, the results of simulated wind erosion are usually over the measured wind erosion in IWEMS, except for Datong county and Dalad Banner in scenario 2 and scenario 4, respectively. However, RWEQ modeling results generally larger than previous measured values using 137Cs in scenario 1 and scenario 2, meanwhile, simulated results less than the measured in scenario 3 and scenario 4. We also compared our results in regional range with previous study of radioisotope 137Cs in Kangbao and Fengning, Hebei of china (Table 6). On the whole, simulated results with IWEMS larger than RWEQ, particularly in Kangbao county. And maximum values of the simulated over the maximum from observed values.
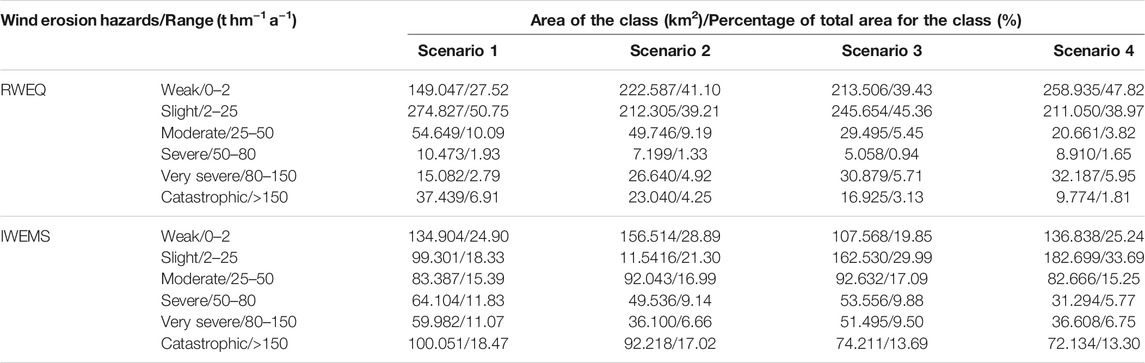
TABLE 6. The area and percentage of the wind erosion hazards determined using the RWEQ and IWEMS for different scenarios.
Influence of the Type of Wind Data on Regional Potential Wind Erosion Evaluation
Herein, the average potential wind erosion was found to be significantly lower for scenarios 1 to 4 for both models. For the same basic spatial meteorological data (scenarios 1 and 2 and scenarios 3 and 4), the average potential wind erosion estimated from the daily average and maximum wind speeds was higher than that calculated using four wind speeds per day (Figure 2). This is due to the fact that the methods of generating the hourly wind speed were different. WINDGEN was used to reproduce the hourly wind speed based on the daily average and maximum wind speeds, whereas the four wind speed measurements per day were interpolated using the linear interpolation method to obtain the hourly wind speed Wagner et al., 1992. Table 7 presents the average wind speed and the percentage of erosive wind (>5 m s−1 wind speed at a height of 2 m). Clearly, the average wind speed and the percentage of erosive wind generated using WINDGEN are greater than those generated using the linear interpolation method. High temporal resolution wind speeds are generally required to generate a precise wind erosion model (van Donk et al., 2008; Guo et al., 2012; Panebianco and Buschiazzo, 2013). Since detailed wind data may be unavailable for some regions, several temporal wind data interpolation methods have been developed (Donatelli et al., 2009). It is suggested that the users need to access the prediction errors of the different temporal interpolation methods when modeling regional wind erosion.

TABLE 7. Mean wind speed and percentage of wind speeds greater than the threshold wind speed (5 m s−1) estimated by the WINDGEN and Linear interpolation methods.
For the same temporal meteorological data (scenarios 1 and 3 and scenarios 2 and 4), the average regional wind erosion obtained from the 130 meteorological stations was higher than that obtained from the 47 meteorological stations (Figure 2). Point meteorological data are generally interpolated into regional raster data for regional geographical and environmental modeling (Mauger et al., 2013; Li and Heap, 2014). Mathematically, the factors affecting the performances of spatial interpolation methods include the sampling design, the spatial distribution of the samples, the nature and quality of the data, the correlation between the primary and secondary variables, and the interactions among the factors (Shepard, 1968; Li and Heap, 2014). Here the kriging method was used to upscale the wind data. As the number of meteorological stations and the measured accuracy increase, the performance of the kriging interpolation tends to increase (MacEachren and Davidson, 1987; Luo et al., 2008; Martin et al., 2016). In addition, the locations and distribution of the meteorological stations within the study area also affect the accuracy of the kriging interpolation (MacEachren and Davidson, 1987; Keskin et al., 2015; Ozturk and Kilic, 2016).
Implications for the Impacts of Climate Change on Wind Erosion
Climate change is an important contributor to wind and water erosion. It can accelerate or decelerate the rates of wind and water erosion and can further alter the soil’s health, productivity, and surface cover (Gao et al., 2002; Sharratt et al., 2015; Webb et al., 2020). The influences of historical climate changes on wind erosion have been extensively studied. The variations in the historical wind speed, precipitation, and temperature have generally been summarized as the main factors affecting temporal wind erosion trends (e.g., Haiyan Zhang et al., 2018; Edwards et al., 2019; Lin et al., 2020). Recently, the effects of future climate change scenarios on wind erosion have also been explored. For example, Sharratt et al. (2015) reported that the soil and PM10 losses via wind erosion will be 25–84% lower under the mid-21st century climate (2035–2064) on the Columbian Plateau, USA, compared with the baseline scenario (1970–1999). Li et al. (2020) reported that projected climate changes (2006–2099) will decrease the regional wind erosion by 10.71–33.74% in Central Asia. These studies generally used different types of wind data (e.g., daily or hourly data) and various wind erosion models (e.g., the RWEQ and WEPS). Mathematically, the magnitude of the wind erosion variations due to climate change may be similar to the effects of using different types of wind data (e.g., Munson et al., 2011; Zhang et al., 2019). The discrepancy in the wind erosion modeling caused by using different types of wind data or different wind erosion models may interfere with characterizing the effect of climate change on wind erosion. Therefore, determining a method of selecting a type of wind data in terms of the temporal resolution (daily or hourly data) and spatial network (basic or reference stations) is vital to quantifying the effects of historical or future climate change on regional wind erosion.
Moreover, many studies involving correlations with wind erosion, such as the impacts of landscape changes (land use/cover) on wind erosion (e.g., Chunlai Zhang et al., 2018; Chi et al., 2019), ecosystem services evaluation of reducing soil erosion (e.g., Hao et al., 2017; Zhao et al., 2017), soil CO2 sequestration and emissions due to wind erosion (e.g., Webb et al., 2012; Chappell et al., 2019), and wind erosion-induced surface soil nutrient loss (e.g., nitrogen and phosphorus) (e.g., Du et al., 2019; Song et al., 2019), have generally neglected the effect of the type of wind data on precise wind erosion modeling.
Limitations and Future Perspectives
Soil loss due to wind erosion at the field or regional scales can be simulated by wind erosion models. However, the models’ predictions are widely underestimated or overestimated (Pi et al., 2017). For instance, Buschiazzo and Zobeck (2008) found that the RWEQ and WEPS underestimated the soil mass transport by 45 and 40%, respectively, in a bare agriculture field. Several studies have demonstrated the underestimation of the maximum sediment transport (Qmax) and field soil loss (SL) and the overestimation of the critical field length (S) when using the RWEQ model at multiple sites (Zobeck et al., 2001; VanPelt et al., 2004).
To improve the accuracy of wind erosion models, calibration of the sensitive inputs of the models is necessary. At the field scale, measured wind erosion data can be relatively easily determined (Jarrah et al., 2020). Several studies have concluded that the prediction accuracy of wind erosion models (e.g., the RWEQ and WEPS) can be remarkably improved by calibrating some of the key parameters (Visser et al., 2005; Fryrear et al., 2008; Youssef et al., 2012; Xing et al., 2018). At the regional scale, the calibration of wind erosion models requires more standard long-term observation data (Jarrah et al., 2020). Du et al. (2018) calibrated the IWEMS model to enhance its performance using data from 452 passive sand traps from 2009 to 2011. Song et al. (2019) predicted the soil organic carbon and nutrient losses resulting from aeolian dust emissions after calibrating the IWEMS using data from 293 field experimental sites from 2014 to 2015. The RWEQ was upscaled to the regional scale and calibrated using measured wind erosion data, and then, it was further used to access the wind erosion risk in the Yellow River watershed, China (Du et al., 2015a; Du et al., 2015b). Several other studies have focused on validating wind erosion models. The soil loss through wind erosion determined using the 137Cs method was used to validate the upscaled RWEQ (e.g., Chunlai Zhang et al., 2018; Chi et al., 2019; Wu et al., 2021). Published wind erosion data have also been used to verify wind erosion models (e.g., Wang et al., 2020; Zhou et al., 2020). Nevertheless, many studies have directly assessed regional wind erosion, but no calibration or verification of the wind erosion models were conducted (e.g., Feng and Sharratt, 2005; Guo et al., 2013; Borrelli et al., 2017; Fenta et al., 2020; Lin et al., 2020). Our study indicates that the type of wind data has a significant influence on the potential wind erosion estimation obtained using the RWEQ and IWEMS. Therefore, it is necessary to systematically calibrate and validate these models to achieve precise wind erosion modeling.
Conclusion
To quantitatively determine the effect of wind data with different temporal resolutions or spatial station distributions on regional wind erosion modeling, the Revised Wind Erosion Equation and the Integrated Wind Erosion Modeling System were used to evaluate the regional potential wind erosion in the APEC during 2000–2012 based on four scenarios with different types of wind data (Table 2). The principal conclusions of this study are as follows.
1) The potential wind erosion evaluated using the two models are closely correlated with the measured wind erosion documented in the literature, but the observed values were generally lower than the predicted values for all four scenarios.
2) The magnitudes of the mean potential wind erosion ranged from 15.73 to 27.33 t ha−1 a−1 by RWEQ and changed between 61.77 and 98.54 t ha−1 a−1 by IWEMS, but the spatial distributions and temporal trends of the annual and seasonal potential wind erosion for the two models were similar for the different scenarios.
3) The impacts of landscape changes (land use/cover) on wind erosion, ecosystem service evaluation of reducing soil erosion, CO2 soil sequestration and emissions through wind erosion, and wind erosion induced surface soil nutrient loss (e.g., nitrogen and phosphorus) may be influenced by regional wind erosion modeling based on different types of wind data (Shepard, 1968; Wagner et al., 1992).
In this study, it was determined that the type of wind data can significantly affect regional wind erosion estimation. Users need to calibrate and validate their selected model to achieve precise wind erosion prediction.
Data Availability Statement
The original contributions presented in the study are included in the article/Supplementary Material, further inquiries can be directed to the corresponding authors.
Author Contributions
ZG and JL initialed and designed the work. LZ and ZG wrote the original draft. CC, RW, and QL reviewed and edited the manuscript.
Funding
This research was funded by the National Science Foundation of China (41877066, 41901001), Natural Science Foundation of Hebei Province (D2018205192, D2018205212), and Young Talents Foundation in Hebei Province (13505197).
Conflict of Interest
The authors declare that the research was conducted in the absence of any commercial or financial relationships that could be construed as a potential conflict of interest.
Publisher’s Note
All claims expressed in this article are solely those of the authors and do not necessarily represent those of their affiliated organizations, or those of the publisher, the editors, and the reviewers. Any product that may be evaluated in this article, or claim that may be made by its manufacturer, is not guaranteed or endorsed by the publisher.
Acknowledgments
The authors thank reviewers and the editors for their suggestions on improving the manuscript.
References
Bagnold, R. A. (1941). The Physics of Blown Sand and Desert Dunes. London: Chapman & Hall. doi:10.1038/148480a0
Böhner, J., Schäfer, W., Conrad, O., Gross, J., and Ringeler, A. (2003). The WEELS Model: Methods, Results and Limitations. Catena 52 (3-4), 289–308. doi:10.1016/S0341-8162(03)00019-5
Borrelli, P., Alewell, C., Alvarez, P., Anache, J. A. A., Baartman, J., Ballabio, C., et al. (2021). Soil Erosion Modelling: A Global Review and Statistical Analysis. Sci. total Environ. 780, 146494. doi:10.1016/j.scitotenv.2021.146494
Borrelli, P., Lugato, E., Montanarella, L., and Panagos, P. (2017). A New Assessment of Soil Loss Due to Wind Erosion in European Agricultural Soils Using a Quantitative Spatially Distributed Modelling Approach. Land Degrad. Develop. 28 (1), 335–344. doi:10.1002/ldr.2588
Buschiazzo, D. E., and Zobeck, T. M. (2008). Validation of WEQ, RWEQ and WEPS Wind Erosion for Different Arable Land Management Systems in the Argentinean Pampas. Earth Surf. Process. Landforms 33 (12), 1839–1850. doi:10.1002/esp.1738
Chappell, A., Webb, N. P., Leys, J. F., Waters, C. M., Orgill, S., and Eyres, M. J. (2019). Minimising Soil Organic Carbon Erosion by Wind Is Critical for Land Degradation Neutrality. Environ. Sci. Pol. 93, 43–52. doi:10.1016/j.envsci.2018.12.020
Chi, W., Zhao, Y., Kuang, W., and He, H. (2019). Impacts of Anthropogenic Land Use/cover Changes on Soil Wind Erosion in China. Sci. total Environ. 668, 204–215. doi:10.1016/j.scitotenv.2019.03.015
Chunlai Zhang, C., Song, C., Wang, Z., Zou, X., and Wang, X. (2018). Review and prospect of the Study on Soil Wind Erosion Process. Adv. Earth Sci. 33, 27–41. doi:10.11867/j.issn.1001-8166.2018.01.0027
Donatelli, M., Bellocchi, G., Habyarimana, E., Confalonieri, R., and Micale, F. (2009). An Extensible Model Library for Generating Wind Speed Data. Comput. Elect. Agric. 69 (2), 165–170. doi:10.1016/j.compag.2009.07.022
Dong, Z. (1998). Establishing Statistic Model of Wind Erosion on Small Watershed Basis. Bull. Soil Water Conservation 18, 55–62. doi:10.3969/j.issn.1000-288X.1998.05.013
Dong, Z., Wang, X. M., and Liu, L. Y. (2000). Wind Erosion in Arid and Semiarid China: an Overview. Soil & Water Conservation 55 (4), 439–444. doi:10.3321/j.issn:1000-694X.2000.02.007
Du, H., Wang, T., Xue, X., and Li, S. (2018). Modelling of Sand/dust Emission in Northern China from 2001 to 2014. Geoderma 330, 162–176. doi:10.1016/j.geoderma.2018.05.038
Du, H., Wang, T., Xue, X., and Li, S. (2019). Estimation of Soil Organic Carbon, Nitrogen, and Phosphorus Losses Induced by Wind Erosion in Northern China. Land Degrad. Dev. 30 (8), 1006–1022. doi:10.1002/ldr.3288
Du, H., Xue, X., Wang, T., and Deng, X. (2015a). Assessment of Wind-Erosion Risk in the Watershed of the Ningxia-Inner Mongolia Reach of the Yellow River, Northern China. Aeolian Res. 17, 193–204. doi:10.1016/j.aeolia.2015.04.003
Du, H., Xue, X., Wang, T., and Deng, X. (2015b). Wind Erosion Modulus and Quantity Evaluation of Aeolian Sediment Feed into River in Watershed of Ningxia-Inner Mongolia Reach of Yellow River from 1986 to 2013. Trans. Chin. Soc. Agric. Eng. 31, 142–151. doi:10.11975/j.issn.1002-6819.2015.10.019
Edwards, B. L., Webb, N. P., Brown, D. P., Elias, E., Peck, D. E., Pierson, F. B., et al. (2019). Climate Change Impacts on Wind and Water Erosion on US Rangelands. J. Soil Water Conservation 74 (4), 405–418. doi:10.2489/jswc.74.4.405
Elliot, D. L. (1979). “Adjustment and Analysis of Data for Regional Wind Energy Assessments,” in Paper Presented at the Workshop on Wind Climate (Asheville, NC, 121–213.
Fattahi, S. M., Soroush, A., and Huang, N. (2020). Wind Erosion Control Using Inoculation of Aeolian Sand with Cyanobacteria. Land Degrad. Dev. 31, 2104–2116. doi:10.1002/ldr.3590
Fécan, F., Marticorena, B., and Bergametti, G. (1998). Parametrization of the Increase of the Aeolian Erosion Threshold Wind Friction Velocity Due to Soil Moisture for Arid and Semi-arid Areas. Ann. Geophys. 17 (1), 149–157. doi:10.1007/s00585-999-0149-7
Feng, G., and B. Sharratt, B. S. (2005). Sensitivity Analysis of Soil and PM10 Loss in WEPS Using the LHS-OAT Method. Trans. ASABE 48 (4), 1409–1420. doi:10.13031/2013.19198
Fenta, A. A., Tsunekawa, A., Haregeweyn, N., Poesen, J., Tsubo, M., Borrelli, P., et al. (2020). Land Susceptibility to Water and Wind Erosion Risks in the East Africa Region. Sci. Total Environ. 703, 135016. doi:10.1016/j.scitotenv.2019.135016
Fryrear, D. W., Saleh, A., Bilbro, J. D., Schomberg, H. M., Stout, J. E., and Zobeck, T. M. (1998). “Revised Wind Erosion Equation (RWEQ). Wind Erosion and Water Conservation Research Unit,” in USDA-ARS, Southern Plains Area Cropping Systems Reseach Laboratory (Lubbock: Technical Bulletin No. 1).
Fryrear, D. W., Bilbro, J. D., Saleh, A., Schomberg, H. M., Stout, J. E., and Zobeck, T. M. (2000). RWEQ: Improved Wind Erosion Technology. Soil and Water Conservation 55 (2), 183–189.
Fryrear, D. W., Sutherland, P. L., Davis, G., Hardee, G., and Dollar, M. (2001). Wind Erosion Estimates with RWEQ and WEQ. CO KS Assembly St. 67401, 760–765.
Fryrear, D. W., Wassif, M. M., Tadrus, S. F., and Ali, A. A. (2008). Dust Measurements in the Egyptian Northwest Coastal Zone. Trans. ASABE 51 (4), 1255–1262. doi:10.13031/2013.25242
Gao, Q., Ci, L., and Yu, M. (2002). Modeling Wind and Water Erosion in Northern China under Climate and Land Use Changes. Soil & Water Conservation 57 (1), 46–55.
Gharibreza, M., Zaman, M., Porto, P., Fulajtar, E., Parsaei, L., and Eisaei, H. (2020). Assessment of Deforestation Impact on Soil Erosion in Loess Formation Using 137Cs Method (Case Study: Golestan Province, Iran). Int. Soil Water Conservation Res. 8 (4), 393–405. doi:10.1016/j.iswcr.2020.07.006
Gregory, J., Wilson, G., Singh, U., and Darwish, M. (2004). TEAM: Integrated, Process-Based Wind-Erosion Model. Environ. Model. Softw. 19 (2), 205–215. doi:10.1016/S1364-8152(03)00124-5
Guo, Z., Zobeck, T. M., Stout, J. E., and Zhang, K. (2012). The Effect of Wind Averaging Time on Wind Erosivity Estimation. Earth Surf. Process. Landforms 37 (7), 797–802. doi:10.1002/esp.3222
Guo, Z., Zobeck, T. M., Zhang, K., and Li, F. (2013). Estimating Potential Wind Erosion of Agricultural Lands in Northern China Using the Revised Wind Erosion Equation and Geographic Information Systems. J. Soil Water Conservation 68 (1), 13–21. doi:10.2489/jswc.68.1.13
Guo, Z., Huang, N., Dong, Z., Van Pelt, R., and Zobeck, T. (2014). Wind Erosion Induced Soil Degradation in Northern China: Status, Measures and Perspective. Sustainability 6 (12), 8951–8966. doi:10.3390/su6128951
Guo, Z., Chang, C., and Wang, R. (2016). A Novel Method to Downscale Daily Wind Statistics to Hourly Wind Data for Wind Erosion Modelling. Geo-Informatics Resource Manag. Sust. Ecosystem 569, 611–619. doi:10.1007/978-3-662-49155-3_64
Hagen, L. J., Wagner, L. E., and Skidmore, E. L. (1999). Analytical Solutions and Sensitivity Analyses for Sediment Transport in Weps. Anal. solutions sensitivity analyses sediment transport WEPS.Transactions Asae 42 (6), 1715–1722. doi:10.13031/2013.13334
Hagen, L. J. (1991). A Wind Erosion Prediction System to Meet User Needs. J. Soil Water Conservation 46 (2), 106–111.
Haiyan Zhang, H., Fan, J., Cao, W., Harris, W., Li, Y., Chi, W., et al. (2018). Response of Wind Erosion Dynamics to Climate Change and Human Activity in Inner Mongolia, China during 1990 to 2015. Sci. Total Environ. 639, 1038–1050. doi:10.1016/j.scitotenv.2018.05.082
Hao, R., Yu, D., Liu, Y., Liu, Y., Qiao, J., Wang, X., et al. (2017). Impacts of Changes in Climate and Landscape Pattern on Ecosystem Services. Sci. Total Environ. 579, 718–728. doi:10.1016/j.scitotenv.2016.11.036
Hu, Y., Liu, J., Zhuang, D., Cao, H., Yan, H., and Yang, F. (2005). Distribution Characteristics of 137Cs in Wind-Eroded Soil Profile and its Use in Estimating Wind Erosion Modulus. Chin. Sci Bull 50 (11), 1155–1159. doi:10.1360/04wd0312
Jarrah, M., Mayel, S., Tatarko, J., Funk, R., and Kuka, K. (2020). A Review of Wind Erosion Models: Data Requirements, Processes, and Validity. Catena 187, 104388. doi:10.1016/j.catena.2019.104388
Jiang, H. (2010). Study on Soil Erosion in Agro Pastoral Ecotone of Northern China Based on 137Cs Technology. Huhehaote: Inner Mongolia Normal University.
Keskin, M., Dogru, A. O., Balcik, F. B., Cigdem, G., Ulugtekin, N., and Sozen, S. (2015). Comparing spatial interpolation methods for mapping meteorological data in Turkey. Energy Syst. Manage. 26, 33–42. doi:10.1007/978-3-319-16024-5_3
Li, J., and Heap, A. D. (2014). Spatial Interpolation Methods Applied in the Environmental Sciences: A Review. Environ. Model. Softw. 53, 173–189. doi:10.1016/j.envsoft.2013.12.008
Li, Z., Zou, X., and Cheng, H. (2013). Method of Wind Erosion Sampling Survey in China. Sci. Soil Water Conservation 11 (4), 17–21. doi:10.3969/j.issn.1672-3007.2013.04.003
Li, M., Yao, W., Shen, Z., Yang, J., and Yang, E. (2016). Erosion Rates of Different Land Uses and Sediment Sources in a Watershed Using the 137Cs Tracing Method: Field Studies in the Loess Plateau of China. Environ. Earth Sci. 75 (7), 591. doi:10.1007/s12665-015-5225-6
Li, J., Ma, X., and Zhang, C. (2020). Predicting the Spatiotemporal Variation in Soil Wind Erosion across Central Asia in Response to Climate Change in the 21st century. Sci. Total Environ. 709, 136060. doi:10.1016/j.scitotenv.2019.136060
Lin, J., Guan, Q., Pan, N., Zhao, R., Yang, L., and Xu, C. (2020). Spatiotemporal Variations and Driving Factors of the Potential Wind Erosion Rate in the Hexi Region, PR China. Land Degrad. Dev. 32 (1), 139–157. doi:10.1002/ldr.3702
Liu, J., Qi, Y., Shi, H., Zhuang, D., and Hu, Y. (2008). Estimation of Wind Erosion Rates by Using 137Cs Tracing Technique: A Case Study in Tariat-Xilin Gol Transect, Mongolian Plateau. Chin. Sci. Bull. 53, 751–758. doi:10.1007/s11434-008-0007-0
Liu, B., Qu, J., and Wagner, L. E. (2013). Building Chinese Wind Data for Wind Erosion Prediction System Using Surrogate US Data. J. Soil Water Conservation 68 (4), 104A–107A. doi:10.2489/jswc.68.4.104A
Liu, X. M., Song, H. Q., Lei, T. J., Liu, P. F., Xu, C. D., Wang, D., et al. (2021). Effects of natural and anthropogenic factors and their interactions on dust events in Northern China. Catena 196, 104919. doi:10.1016/j.catena.2020.104919
Lu, H., and Shao, Y. (2001). Toward Quantitative Prediction of Dust Storms: an Integrated Wind Erosion Modelling System and its Applications. Environ. Model. Softw. 16 (3), 233–249. doi:10.1016/S1364-8152(00)00083-9
Luo, W., Taylor, M. C., and Parker, S. R. (2008). A Comparison of Spatial Interpolation Methods to Estimate Continuous Wind Speed Surfaces Using Irregularly Distributed Data from England and Wales. Int. J. Climatol. 28 (7), 947–959. doi:10.1002/joc.1583
Lyu, X., Li, X., Wang, H., Gong, J., Li, S., Dou, H., et al. (2021). Soil Wind Erosion Evaluation and Sustainable Management of Typical Steppe in Inner Mongolia, China. J. Environ. Manage. 277, 111488. doi:10.1016/j.jenvman.2020.111488
MacEachren, A. M., and Davidson, J. V. (1987). Sampling and Isometric Mapping of Continuous Geographic Surfaces. The Am. Cartographer 14 (4), 299–320. doi:10.1559/152304087783875723
Martin, R., Aler, R., Valls, J. M., and Galvan, I. M. (2016). Machine Learning Techniques for Daily Solar Energy Prediction and Interpolation Using Numerical Weather Models. Concurrency Computat.: Pract. Exper. 28 (4), 1261–1274. doi:10.1002/cpe.3631
Mauger, G. S., Bumbaco, K. A., Hakim, G. J., and Mote, P. W. (2013). Optimal Design of a Climatological Network: beyond Practical Considerations. Geosci. Instrum. Method. Data Syst. 2 (2), 199–212. doi:10.5194/gi-2-199-2013
Meteorological Bureau of the People's Republic of China (MBPRC) (2019). Classification and Rules of Nomenclature for Meteorological Observing Stations. Beijing, China: China Meteorological Press.
Ministry of Water Resources of the People's Republic of China (MWRPRC) (2007). Standards for Classification and Gradation of Soil Erosion. Beijing, China: China Water Power Press.
Ministry of Water Resources of the People’s Republic of China (MWRPRC) (2013). The First National Bulletin on Water and Soil Conservation in the National Water Resources Survey. Beijing, China: Soil and Water Conservation in China.
Munson, S. M., Belnap, J., and Okin, G. S. (2011). Responses of Wind Erosion to Climate-Induced Vegetation Changes on the Colorado Plateau. Proc. Natl. Acad. Sci. 108 (10), 3854–3859. doi:10.1073/pnas.1014947108
O’Loingsigh, T., McTainsh, G. H., Tews, E. K., Strong, C. L., Leys, J. F., Shinkfield, P., et al. (2014). The Dust Storm Index (DSI): A Method for Monitoring Broadscale Wind Erosion Using Meteorological Records. Aeolian Res. 12, 29–40. doi:10.1016/j.aeolia.2013.10.004
Owen, P. R. (1964). Saltation of Uniform Grains in Air. J. Fluid Mech. 20 (02), 225–242. doi:10.1017/S0022112064001173
Ozturk, D., and Kilic, F. (2016). Geostatistical Approach for Spatial Interpolation of Meteorological Data. Acad. Bras. Ciênc. 88 (4), 2121–2136. doi:10.1590/0001-3765201620150103
Panebianco, J., and Buschiazzo, D. (2013). Effect of Temporal Resolution of Wind Data on Wind Erosion Prediction with the Revised Wind Erosion Equation (RWEQ). Ciencia Del. Suelo 31 (2), 189–199.
Pi, H., Sharratt, B., Feng, G., and Lei, J. (2017). Evaluation of Two Empirical Wind Erosion Models in Arid and Semi-arid Regions of China and the USA. Environ. Model. Softw. 91 (MAY), 28–46. doi:10.1016/j.envsoft.2017.01.013
Potter, K. N., Williams, J. R., Larney, F. J., and Bullock, M. S. (1998). Evaluation of EPIC's Wind Erosion Submodel Using Data from Southern Alberta. Can. J. Soil Sci. 78 (3), 485–492. doi:10.4141/S97-091
Raupach, M. R., Gillette, D. A., and Leys, J. F. (1993). The Effect of Roughness Elements on Wind Erosion Threshold. J. Geophys. Res. 98 (D2), 3023–3029. doi:10.1029/92JD01922
Shao, Y. (2001). A Model for mineral Dust Emission. J. Geophys. Res. 106 (D17), 20239–20254. doi:10.1029/2001JD900171
Sharratt, B. S., Tatarko, J., Abatzoglou, J. T., Fox, F. A., and Huggins, D. (2015). Implications of Climate Change on Wind Erosion of Agricultural Lands in the Columbia Plateau. Weather Clim. Extremes 10 (PA), 20–31. doi:10.1016/j.wace.2015.06.001
Sheikh, V., Visser, S., and Stroosnijder, L. (2009). A Simple Model to Predict Soil Moisture: Bridging Event and Continuous Hydrological (BEACH) Modelling. Environ. Model. Softw. 24 (4), 542–556. doi:10.1016/j.envsoft.2008.10.005
Shen, L., Tian, M., and Gao, J. (2016). Analysis on Wind Erosion and Main Factors in Desertification Control Ecologincal Function Area of Hunshandake Using the Revised Wind Erosio Equation Model. Res. Soil Water Conservation 23 (06), 90–97. doi:10.13869/j.cnki.rswc.2016.06.010
Shepard, D. (1968). “A Two-Dimensional Interpolation Function for Irregularly-Spaced Data,” in Proceedings of the 1968 23rd ACM national conference on, January 1968, 517–524. doi:10.1145/800186.810616
Skidmore, E. L., and Tatarko, J. (1990). Stochastic Wind Simulation for Erosion Modeling. Trans. Asae Am. Soc. Agric. Eng. 33 (6), 1893–1899. doi:10.13031/2013.31555
Song, Y., Liu, L., Yan, P., and Cao, T. (2005). A Review of Soil Erodibility in Water and Wind Erosion Research. J. Geogr. Sci. 15 (2), 167–176. doi:10.1007/BF02872682
Song, H., Zhang, K., Piao, S., Liu, L., Wang, Y.-P., Chen, Y., et al. (2019). Soil Organic Carbon and Nutrient Losses Resulted from spring Dust Emissions in Northern China. Atmos. Environ. 213, 585–596. doi:10.1016/j.atmosenv.2019.06.043
Song, Z. (2004). A Numerical Simulation of Dust Storms in China. Environ. Model. Softw. 19 (2), 141–151. doi:10.1016/S1364-8152(03)00116-6
van Donk, S. J., Wagner, L. E., Skidmore, L. E., and Tatarko, J. (2005). Comparison of the Weibull Model with Measured Wind Speed Distributions for Stochastic Wind Generation. Soil Water Division. ASABE. 48, 503–510. doi:10.13031/2013.18324
van Donk, S. J., Liao, C., and Skidmore, E. L. (2008). Using Temporally Limited Wind Data in the Wind Erosion Prediction System. ASABE 51 (5), 1585–1590. doi:10.13031/2013.25315
Vanpelt, R., Potter, K., Stout, J., Popham, T., and Zobeck, T. (2004). Validation of the Wind Erosion Stochastic Simulator (WESS) and the Revised Wind Erosion Equation (RWEQ) for Single Events. In Proceedings of the International Symposium on SoilErosion Research for the 21th Century, Honolulu, January 3-5, 471–474. doi:10.1016/S1364-8152(03)00122-1
Visser, S. M., Sterk, G., and Karssenberg, D. (2005). Wind Erosion Modelling in a Sahelian Environment. Environ. Model. Softw. 20 (1), 69–84. doi:10.1016/j.envsoft.2003.12.010
Wagner, L. E., Tatarko, J., and Skidmore, E. L. (1992). WIND-GEN: A Statistical Database and Generator for Wind Data. Paper-American Society of Agricultural Engineers USA.
Wang, X., Guo, Z., Chang, C., Wang, R., Li, J., Li, Q., et al. (2020). Spatiotemporal Distribution of Soil Wind Erosion Modulus in the Agro-Pastoral Ecotone of north China. J. Desert Res. 40 (1), 12–22. doi:10.7522/j.issn.1000-694X.2019.00030
Wang, X., Liu, J., Che, H., Ji, F., and Liu, J. (2018). Spatial and Temporal Evolution of Natural and Anthropogenic Dust Events over Northern China. Sci. Rep. 8 (5), 241–257. doi:10.1038/s41598-018-20382-5
Webb, N. P., McGowan, H. A., Phinn, S. R., Leys, J. F., and McTainsh, G. H. (2009). A Model to Predict Land Susceptibility to Wind Erosion in Western Queensland, Australia. Environ. Model. Softw. 24 (2), 214–227. doi:10.1016/j.envsoft.2008.06.006
Webb, N. P., Chappell, A., Strong, C. L., Marx, S. K., and McTainsh, G. H. (2012). The Significance of Carbon-Enriched Dust for Global Carbon Accounting. Glob. Change Biol. 18 (11), 3275–3278. doi:10.1111/j.1365-2486.2012.02780.x
Webb, N. P., Kachergis, E., Miller, S. W., McCord, S. E., Bestelmeyer, B. T., Brown, J. R., et al. (2020). Indicators and Benchmarks for Wind Erosion Monitoring, Assessment and Management. Ecol. indicators 110, 105881. doi:10.1016/j.ecolind.2019.105881
Woodruff, N. P., and Siddoway, F. H. (1965). A Wind Erosion Equation1. Proc. Soil ence Soc. America 29 (5), 602–608. doi:10.2136/sssaj1965.03615995002900050035x
Wu, X., Fan, J., Sun, L., Zhang, H., Xu, Y., Yao, Y., et al. (2021). Wind Erosion and its Ecological Effects on Soil in the Northern piedmont of the Yinshan Mountains. Ecol. Indicators 128, 107825. doi:10.1016/j.ecolind.2021.107825
Xing, C., Guo, Z., Chang, C., Wang, R., and Zhang, Z. (2018). Validation of RWEQ Model in the Bashang Area, Hebei, china. J. Desert Res. 38 (06), 1180–1192. doi:10.7522/j.issn.1000-694X.2018.00014
Yizhaq, H., Xu, Z., and Ashkenazy, Y. (2020). The Effect of Wind Speed Averaging Time on the Calculation of Sand Drift Potential: New Scaling Laws. Earth Planet. Sci. Lett. 544, 116373. doi:10.1016/j.epsl.2020.116373
Youssef, F., Visser, S., Karssenberg, D., Bruggeman, A., and Erpul, G. (2012). Calibration of RWEQ in a Patchy Landscape; a First Step towards a Regional Scale Wind Erosion Model. Aeolian Res. 3 (4), 467–476. doi:10.1016/j.aeolia.2011.03.009
Yuan, Y., Yin, S., Xie, Y., and Guo, Z. (2018). Temporal and Spatial Characteristics of Diurnal Variations of Wind Speed in Wind Erosion Areas over China. Arid Land Geogr. 41 (3), 480–487. doi:10.13826/j.cnki.cn65-1103/x.2018.03.006
Zhang, J., Zou, X., Zhang, C., Yang, S., Pan, X., and Liu, Y. (2010). Evaluating farmland Soil Erosion by Wind in Bashang of Zhangjiakou with 137Cs as a Tracer, 46. Natural Science: Journal of Beijing Normal University, 724–728.
Zhang, G., Azorin-Molina, C., Shi, P., Lin, D., Guijarro, J. A., Kong, F., et al. (2019). Impact of Near-Surface Wind Speed Variability on Wind Erosion in the Eastern Agro-Pastoral Transitional Zone of Northern China, 1982-2016. Agric. For. Meteorology 271, 102–115. doi:10.1016/j.agrformet.2019.02.039
Zhang, D., Liang, P., Yang, X., and Li, H. (2020). The Control of Wind Strength on the Barchan to Parabolic Dune Transition. Earth Surf. Process. Landforms 45 (10), 2300–2313. doi:10.1002/esp.4881
Zhao, Y., Yue, J., Xu, C., Du, C., and Chang, Y. (2005). Application of 137Cs Tracer Technique to Estimate the Wind Erosion Rate of Castanozem in Luanhe River Source Area. Acta Scientiae Circumstantiae 2005 (4), 562–566. doi:10.3321/j.issn:0253-2468.2005.04.024
Zhao, Y., Pei, Y. S., and Zhai, Z. J. (2011). Distributed Simulation of Soil Wind Erosion and its Application to the Tuhaimajia River basin. Hydraulic Eng. 42 (05), 554–562. doi:10.13243/j.cnki.slxb.2011.05.009
Zhao, Y., Wu, J., He, C., and Ding, G. (2017). Linking Wind Erosion to Ecosystem Services in Drylands: a Landscape Ecological Approach. Landscape Ecol. 32 (12), 2399–2417. doi:10.1007/s10980-017-0585-9
Zhou, Z., Zhang, Z., Zou, X., Zhang, K., and Zhang, W. (2020). Quantifying Wind Erosion at Landscape Scale in a Temperate Grassland: Nonignorable Influence of Topography. Geomorphology 370, 107401. doi:10.1016/j.geomorph.2020.107401
Zobeck, T. M., Parker, N. C., Haskell, S., and Guoding, K. (2000). Scaling up from Field to Region for Wind Erosion Prediction Using a Field-Scale Wind Erosion Model and GIS. Agriculture. Ecosyst. Environ. 82 (1-3), 247–259. doi:10.1016/S0167-8809(00)00229-2
Zobeck, T. M., Van Pelt, S., Stout, J. E., and Popham, T. W. (2001). Validation of the Revised Wind Erosion Equation (RWEQ) for Single Events and Discrete Periods. Soil Erosion, 471–474. doi:10.13031/2013.4579
Keywords: wind data, wind erosion modeling, potential wind erosion, RWEQ, IWEMS
Citation: Zhang L, Guo Z, Li J, Chang C, Wang R and Li Q (2022) Effect of the Type of Wind Data on Regional Potential Wind Erosion Estimation. Front. Environ. Sci. 10:847128. doi: 10.3389/fenvs.2022.847128
Received: 01 January 2022; Accepted: 02 February 2022;
Published: 04 March 2022.
Edited by:
Guang-Lei Gao, Beijing Forestry University, ChinaReviewed by:
Yuanyuan Zhao, Beijing Forestry University, ChinaDongwei Liu, Inner Mongolia University, China
Heqiang Du, Northwest Institute of Eco-Environment and Resources (CAS), China
Copyright © 2022 Zhang, Guo, Li, Chang, Wang and Li. This is an open-access article distributed under the terms of the Creative Commons Attribution License (CC BY). The use, distribution or reproduction in other forums is permitted, provided the original author(s) and the copyright owner(s) are credited and that the original publication in this journal is cited, in accordance with accepted academic practice. No use, distribution or reproduction is permitted which does not comply with these terms.
*Correspondence: Zhongling Guo, Z3psZGhyQGhlYnR1LmVkdS5jbg==; Jifeng Li, bGpmZ2lzQDE2My5jb20=