- 1 School of Management, Guangzhou University, Guangzhou, China
- 2 School of Business, Hunan University of Technology, Zhuzhou, China
- 3 Durham University Business School, Durham University, Durham, United Kingdom
- 4 Business School, Shanghai University of Finance and Economics, Shanghai, China
- 5 Department of Civil, Environmental and Architectural Engineering (DICEA), University of Padua, Padua, Italy
- 6 Graduate School, Northern Arizona University, Flagstaff, AZ, United States
The digital divide between urban and rural communities has substantially narrowed as information and communication technology has evolved, enabling increasingly more interactions between urban and rural areas. Rural areas now have the foundation and conditions to take advantage of e-commerce opportunities, which is no longer exclusively a city-centric economic mode. Taking advantage of the Internet’s vast resources, many villagers jumped at the opportunity to launch rural e-commerce businesses. Rural inhabitants, however, face several challenges when it comes to starting their own e-commerce enterprises. Meanwhile, local governments and rural e-commerce platform providers, find it difficult to provide accurate help to practitioners and entrepreneurs. To this end, a system of indicators based on a model of entrepreneurial events was developed to identify e-commerce entrepreneurs. And the main objective of this paper is to explore the factors influencing the abilities and expectations of rural residents to set up their own e-commerce businesses in order to provide them with tailored support. Survey data from 162 rural e-commerce practitioners were analyzed using a deep neural network in R. The results reveal that the index system developed in this paper has a good level of reliability and validity, and the prediction approach has a high degree of precision (over 90%), indicating that it can successfully identify rural e-commerce entrepreneurs. Based on the findings of this study, we recommend that local governments and e-commerce businesses work together to address the practical issues of perceived feasibility and desirability for rural e-commerce practitioners. Residents in rural areas who want to start their own businesses can take advantage of the development opportunities provided by the information and communication technology, while local governments should keep up with the speed of digitization and informatization to better manage rural economic growth.
1 Introduction
Labor, capital, market, information, and other resources have primarily flowed to cities since China’s reform and opening-up (Li, 2008). Due to their distance from cities and the difficulties in accessing varied resources, rural areas were formerly far behind metropolitan areas in terms of development (including business, education, science and technology). As a result, the phenomenon of “dual structure” has emerged, characterized by a significant development gap between urban and rural areas (Li, 2008). Cities with a thriving economy are the first to experiment with new business and management strategies. For example, in the early twenty-first century, metropolitan regions were the birthplace of China’s e-commerce, as represented by Dangdang.com, Taobao.com, and Jingdong Multimedia (Huang and Zhou, 2015). E-commerce has risen quickly in China’s urban areas since then, with the digital divide between urban and rural areas widening further (Guo et al., 2017).
Nevertheless, since 2013, e-commerce expansion was no longer restricted to metropolitan areas, and several new trends emerged (Sohu net, 2021). With the advancement of information and communication technology, as well as the widespread usage of mobile smart phones, e-commerce has gradually penetrated rural areas (Zhou et al., 2021), supporting the development of interactions between urban and rural areas. In comparison to the oversaturated metropolitan markets, the internet retail sector in rural areas has arguably more room for development (Zhou et al., 2021). While urban e-commerce is still expanding slowly, online businesses in rural China produced more than 700 billion yuan in sales and created more than 6.83 million employment positions from 1 July 2018 to 30 June 2019 (Zhou et al., 2021). Therefore, promoting e-commerce in rural regions not only offers rural inhabitants with a wide range of commodity options, but it also creates a large number of job opportunities for people in the local communities (Chao et al., 2021). Many studies have indicated that the growth of rural e-commerce has boosted rural women’s inventiveness and entrepreneurship, as well as contributed to the alleviation of rural poverty (e.g., Yu and Cui, 2019; Abbas et al., 2019; Ge et al., 2022). More importantly, widespread access to and use of mobile Internet in rural regions has reduced and eliminated market information asymmetry (Leng et al., 2020). Hence, rural inhabitants have the opportunity to use the Internet and e-commerce platforms to sell local agricultural and ancillary goods and create rural e-commerce enterprises (Gao and Liu, 2020). Rural e-commerce, in the setting of COVID-19, enabling start-ups to improve their performance and product competitiveness (Liu et al., 2021a).
The development of scientific and technology information services in rural areas is inextricably linked to the advent of rural e-commerce (Wen et al., 2012). From an economic point of view, the development of rural e-commerce is due to the progressive expansion of e-commerce in China’s rural areas from a single point to several places, finally transforming into a tailored network information platform for farmers (Shen et al., 2018). Rural e-commerce entrepreneurship is defined as rural inhabitants creating their own enterprises and selling their agricultural and sideline items online (Shen et al., 2018). Many academics (e.g., Gao and Liu, 2020; Mei et al., 2020; Ma et al., 2020) have concentrated on the factors driving and influencing rural e-commerce in recent years, since the emergence of rural e-commerce entrepreneurship. These studies contribute to better understanding the potential formation and operation processes behind rural e-commerce (Liu, 2018; Gao and Liu, 2020; Mei et al., 2020), as well as the differences in influencing variables in various scenarios.
Previous research however, has mostly relied on case studies (e.g., Yu and Cui, 2019; Gao and Liu, 2020; Cui et al., 2019), and only a few empirical studies exist on this topic (e.g., Mei et al., 2020; Ma et al., 2020). These studies generally explore the various elements influencing rural e-commerce in order to assess the factors that influence their importance. There is currently limited research however, on how to determine if a person is a rural e-commerce entrepreneur based on a number of specific characteristics or influencing factors. Therefore, this article is to answer the following question: what factors determine whether rural e-commerce practitioners are entrepreneurs? Through a literature analysis, this research develops an index system for identifying and predicting rural e-commerce entrepreneurs based on the entrepreneurial event model. The acquired data used and tested by employing a deep neural network approach, yielding a prediction model with excellent accuracy and stability. Our research adds to the body of knowledge by suggesting an approach to identifying rural e-commerce entrepreneurs and offers a practical tool for rural e-commerce platforms, businesses, and governments. More importantly, identifying rural e-commerce entrepreneurs facilitates the speedy matching of entrepreneurial resources and precise delivery of market information, thereby promoting rural e-commerce entrepreneurs’ sustainable entrepreneurship.
The rest of the paper is organized as follows: the next section reviews the existing literature to identify the relevant factors and develop the index system, while the third section presents the study methodology and findings; the fourth section discusses the study findings and proposes theoretical and practical implications, while the final section presents conclusions, limitations, and future prospects.
2 Theoretical Background and Index System Construction
2.1 Influencing Factors of Rural E-Commerce Entrepreneurship
Many researchers have used case studies and empirical research to investigate the factors that influence rural residents’ e-commerce entrepreneurship. Mei et al. (2020), for example, discovered that rural e-commerce clusters, local resource endowment, entrepreneurial atmosphere, entrepreneurial threshold, and government regulations all influence rural people’ entrepreneurial activity. Furthermore, the existence of seed entrepreneurs and information and communication technology, according to Gao and Liu (2020), have a significant influence on rural people’ e-commerce entrepreneurship. Similarly, Ma et al. (2020) found that Internet availability and use increased the likelihood of farmers embracing e-commerce by 20%. Furthermore, Sousa et al. (2020) thought that the last mile of rural logistics and distribution convenience influenced the growth and entrepreneurship of rural e-commerce. Rural e-commerce is flourishing in China’s vast rural areas, thanks to its considerable benefits in lowering capital entry and removing information asymmetry (Lin et al., 2016). Therefore, e-commerce is considered an essential means to provide digital benefits to the rural people in the long run (Li et al., 2021a).
In summary, recent research on the characteristics that influence rural people’ e-commerce entrepreneurship (see Table 1) provides a strong foundation for this paper. As can be seen in our Table, the main areas of interest are related to: 1) what factors drive rural residents to start e-commerce businesses; 2) how are rural e-commerce entrepreneurial modes and mechanisms formed under the effect of certain factors and 3) what benefits might rural e-commerce entrepreneurship provide. These studies, however, are primarily concerned with the impact of various factors. To-date there has been limited research on how to identify rural e-commerce entrepreneurs when a number of characteristics or contributing factors are known. Following the identification of the probable contributing factors, this study classified and ordered them according to the entrepreneurial event model, and built an index system for the identification and prediction of rural e-commerce entrepreneurs.
2.2 Entrepreneurial Event Model
In their work, Shapero and Sokol (Shapero and Sokol, 1982) proposed the entrepreneurial event model. The authors argue that entrepreneurial intents stem from perceptions of desirability and feasibility, as well as a propensity to act on opportunities (Shapero and Sokol, 1982). According to Shapero’s entrepreneurial paradigm, starting a firm necessitates two factors (Krueger, 1993): 1) entrepreneurs should intend to do so; 2) entrepreneurship necessitates some type of triggering event. Shapero has claimed that information on perceived feasibility and perceived desirability is the main information required to determine entrepreneurial intentions (Shapero, 1985). Therefore, in this paper, the influencing factors of rural e-commerce entrepreneurship are separated into features relating to perceived desirability and perceived feasibility.
It is worth noting that if individuals in rural areas are interested in creating an e-commerce firm, this can lead to one of two outcomes: 1) individuals may have only expressed an interest in starting a business but have yet to engage in entrepreneurial activity; or 2) entrepreneurial actions may have been triggered by their intentions. It is conceivable that the two outcomes are due to differing attitudes or levels of recognition toward various contributing factors. As a result, we use this theory to determine if individuals in rural areas who exhibit certain traits of entrepreneurial intent are actually rural e-commerce entrepreneurs. Therefore, in our study, we try to identify rural e-commerce entrepreneurs through the detailed assessment of a number of given factors.
2.3 Index System Construction
Following Shapero and Sokol’s (Shapero and Sokol, 1982) entrepreneurship event model, we split the influencing factors of rural e-commerce entrepreneurship into two groups: perceived desirability and perceived feasibility. Perceived desirability refers to factors that influence a person’s perception of the attractiveness of entrepreneurial opportunities, such as future prospects and digital dividends, while perceived feasibility refers to factors that influence a person’s belief in his or her own entrepreneurial ability, such as familiarity with online technologies, funds available for rural e-commerce, smart phone proficiency (Shapero and Sokol, 1982; Shapero, 1985; Krueger, 1993). The information in Table 1 was used to generate these factors. Table 2 summarizes the factors as categorized for this study. Perceived desirability include degree of identity for development prospects, expectations for digital dividends, the convenience of express delivery, and local resource endowments. All of these variables are outside factors that might have an impact on the entrepreneurial intentions of rural e-commerce practitioners to a certain degree. Perceived feasibility include length of online purchasing, smart phone proficiency, mastery of professional knowledge, and funds available for rural e-commerce. These are internal elements that might have an effect on rural e-commerce practitioners’ abilities to conduct entrepreneurial operations.
It is worth noting that factors identified by earlier academics have been modified to fit more accurately the context of the current study. For example, in this paper, factors such as “information and communication technology” and the “Internet” have been interpreted as: length of online purchasing (the number of years an individual has utilized online shopping), smart phone proficiency, and level of professional knowledge. Locals may utilize smart phones to purchase online, and they can also gain professional expertise about rural e-commerce operations online, thanks to the promotion of Internet and information and communication technology in rural regions (Gao and Liu, 2020). We also use the degree of identity of development prospects to represent the benefits of rural e-commerce in terms of reducing capital entry and eliminating information asymmetry, as well as rural e-commerce clusters, entrepreneurial atmosphere, entrepreneurial threshold, government policies, and seed entrepreneurs. This is because improving these characteristics has a beneficial impact on the growth of rural e-commerce prospects. For example, enhancing the entrepreneurial atmosphere, lowering the entry barrier to entrepreneurship, and promoting positive government policies may all help to create a favorable environment for rural e-commerce development, enticing locals to participate in rural e-commerce entrepreneurship.
3 Research Methods and Results
Our objective is to use eight independent variables (each of the two types, perceived desirability and perceived feasibility, has four variables) to create a multi-layer deep neural network that can predict a dependent variable, in our case rural e-commerce entrepreneurship. To achieve that, the research process diagram in Figure 1 has been developed, based on the identification of influencing factors and theoretical models which enabled the construction of an index system. Our research process also comprises data collection, reliability and validity tests, and the identification of rural e-commerce entrepreneurs, in addition to the index system construction done in the previous section. We’ll go over the precise study steps and results of the remaining three parts in depth below.
3.1 Data Collection
The research data have been collected through a questionnaire survey of rural e-commerce workers in Guangdong, Guangzhou’s Conghua district. Figure 2 depicts its geographical location. Conghua is a Guangzhou suburb located near the Tropic of Cancer, with a moderate temperature and it is well-known for its production of litchi, longan, and other fruits (Baidu Encyclopedia, 2021). In addition to the above, Conghua District has grabbed the opportunities created by the fast growth of rural e-commerce. According to data from Alibaba’s rural-based Taobao platform, there were more than 60 Taobao village service stations in the Conghua district alone in 2016, and as many as 1,000 villagers sold lychees through e-commerce platforms (The Southern Plus, 2017). Most importantly, despite being the outer suburb of Guangzhou and on the intersection of many rural areas, Conghua district is highly developed in comparison to other“typical” rural areas in the area. According to Huang et al. (Huang et al., 2021), when the local economic environment changes, the role of the residents in the area may shift from “consumers” to “partners,” with a slew of new “entrepreneurs” emerging. Similarly, rural e-commerce practitioners in Conghua district are currently in the stages of becoming “partners” and “entrepreneurs.” Hence, there are a disproportionately large number of rural e-commerce practitioners in this region, compared to other rural regions, which provides the necessary basis and environment for rural e-commerce entrepreneurship. Our research team traveled to Conghua District in 2018 to conduct a survey on litchi e-commerce sales and formed strong bonds with local businesses and rural e-commerce associations, which supported the development of this research.
We monitored the survey respondents’ details closely in order to identify appropriate participants for this study as accurately as feasible. Our sampling frame comprises those who have: 1) worked in rural e-commerce for the last year; 2) resided in Conghua District for roughly a year; 3) know how to use a smart phone; and 4) have experience with online purchasing. Gender, age, and educational background were also included in the background information, which served as the paper’s control variables. The second section of the questionnaire was created using our index system, which included one dependent variable and eight independent variables. Table 3 shows the unit and numerical measurement of each variable. In order to identify rural e-commerce entrepreneurs, we used two criteria: 1) enterprises or individual industrial/commercial businesses registered with the rural e-commerce operation authority; 2) since the enterprise or individual industrial and commercial business registration, the enterprise or individual industrial and commercial business has operated for at least one year.
Our investigation ran from June 2021 to September 2021, and we received 162 valid questionnaires. The information for the questionnaire was gathered mostly through an online survey and structured telephone interviews. It is a more secure method of obtaining data in the midst of the COVID-19 pandemic (Liu et al., 2021b; Su et al., 2021). Table 4 displays the questionnaire’s descriptive statistical results. The proportion of male and female respondents was comparable, according to the statistics, and the respondents were mostly between the ages of 26 and 45, with high school and junior college degrees.
3.2. Reliability and Validity Test
In order to assure the reliability and validity of the questionnaire we created (Heale and Twycross, 2015; Huang et al., 2017), using RStudio (Diederich and Milton, 1988), we first standardized the gathered effective data. The reliability, validity, and collinearity of normalized data were then tested using SPSS software (Rockwell, 1975).
3.2.1 Reliability Test
As demonstrated in Table 5, the Cronbach’s Alpha value is more than 0.8, indicating that the scale we created is very reliable (Heale and Twycross, 2015; Zhang et al., 2020; Aman and Shi, 2021; Mubeen et al., 2021).
3.2.2 Validity Test
The value of KMO is larger than 0.8, as shown in Table 6, and the Chi-square value of the Bartlett sphericity test findings is 730.237, which is a significant value with a p ≤ 0.001 (Raza et al., 2019; Li et al., 2021b).
We also ran a collinearity test on the scale data, which generated the findings given in Table 7. The data revealed that Tolerance levels were all more than 0.1 and VIF values were all less than 10. This shows that our scale has no collinearity issues (Rockwell, 1975; Norušis, 1982). We may infer that the scale in this work has excellent validity based on the results of the KMO and Bartlett’s tests as well as the collinearity test.
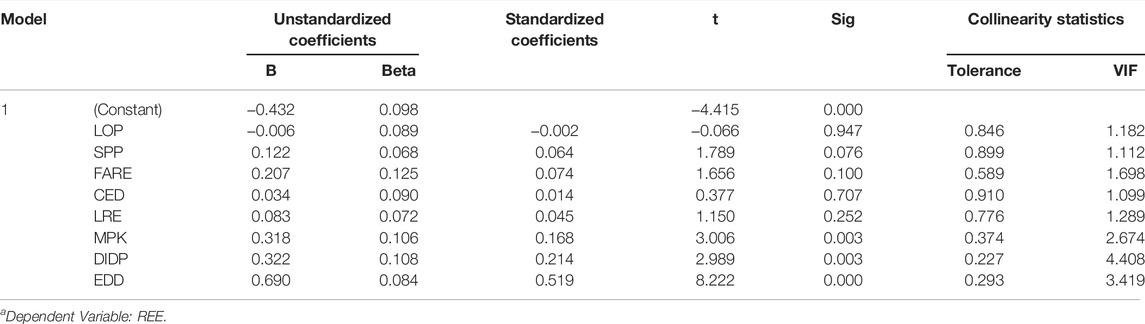
TABLE 7. Coefficients a .
3.3 Identification of Rural E-Commerce Entrepreneurs
Based on the data of 8 independent factors collected, this paper uses the deep neural network approach (Liu et al., 2017) to predict rural e-commerce entrepreneurship as the dependent variable. The deep neural network can cope with complicated nonlinear issues in data by constructing several hidden layers in the neural network (Ben-Bassat et al., 2018). The deep neural network, similar to other machine learning algorithms, must go through three steps: training set construction, learning algorithm, and prediction (Tirdad et al., 2021).
The deep neural network, as a way to enhance the specialization of models of training data, has been employed in recent years by researchers in various areas, such as medical diagnosis (Mukherjee et al., 2021), natural language processing (Li et al., 2021c), and computer vision (Rajvanshi and Pramod, 2021), following the gorowing popularity of machine learning. We use this technique to identify rural e-commerce entrepreneurs because of the benefits of deep neural network in dealing with nonlinear issues, great data analysis capacity, and extensive application in real life.
This paper’s deep neural network data processing is performed using the R programming environment. R program is simple to use and includes a number of neural network augmentation packages (Peng et al., 2021). For our data analysis, we largely employed the R software’s Neuralnet add-on package. The neural network, using specific techniques, allocates a set of starting weights (different every time) at the start of each training, and then uses this initial weight as the basis for the traiing (Canziani et al., 2016; Liu et al., 2017). Therefore, it converges to a new local optimum solution virtually every time, at which point the training is complete. As a result, the accuracy of each prediction is likely to vary (Canziani et al., 2016; Liu et al., 2017). We made several predictions for the different partition ratio of training sets and the diverse number of neurons in hidden layers in order to mitigate the disadvantages caused by the aforesaid difficulties as much as possible. Table 8 shows the anticipated outcomes. The findings demonstrate that a deep neural network can be used to construct a rural e-commerce entrepreneurship identification model, and that a good result can be produced based on the gathered data of 8 factors, with a prediction accuracy of higher than 90%. Admittedly, this may be a tentative finding. Because under the model constituted of diverse index system, the final calculation result may create variance. In addition, the sample size of our research is restricted (162 samples), and there may be inconsistencies in the measurement results of various sample groups, and there may also be discrepancies in the findings after expanding the sample size. But the upside is that we have presented an effective method to identify rural e-commerce entrepreneurs, and the results are acceptable in small sample testing.
4 Discussion
Based on a thorough literature review and the entrepreneurial event model, in the current study we develop an index system for predicting and identifying rural e-commerce entrepreneurs. The index system develop consists of eight elements that are used to explore perceived desirability and perceived feasibility. The obtained data was analyzed using a deep neural network, and the findings reveal that the resulting prediction technique has an accuracy of greater than 90%. We apply machine learning’s deep neural network to the field of rural e-commerce entrepreneurship, resulting in a novel and effective method for identifying rural e-commerce entrepreneurs. We propose the following theoretical and practical implications based on our findings.
4.1 Theoretical Implications
First, this paper adds to the growing body of knowledge about rural e-commerce business. Rural e-commerce entrepreneurship has become a popular study issue, particularly in terms of evaluating the factors that influence it (e.g., Gao and Liu, 2020; Mei et al., 2020; Ma et al., 2020). However, few researchers have looked at developing an index system to identify rural e-commerce entrepreneurship. From the standpoint of a rural e-commerce practitioner or an individual with entrepreneurial ambitions to become a rural e-commerce entrepreneur in the future, a variety of factors should be considered by rural residents. This study focuses on identifying rural e-commerce entrepreneurs from factors in the aspects of perceived feasibility and perceived desirability, which may be useful for local governments and rural e-commerce businesses when developing their strategies including offering incentives and training programs.
Second, this paper develops an index system for identifying rural e-commerce entrepreneurs. The development of this index system is based on factors related to perceived desirability and feasibility as described in the entrepreneurial event model (Shapero and Sokol, 1982; Krueger, 1993). The specific factors at play have been adapted from prior studies (e.g., Sousa et al., 2020; Ma et al., 2020; Lin et al., 2016; Li et al., 2021a). Our index system for identifying rural e-commerce entrepreneurs may be utilized as a theoretical foundation for deep neural network prediction, laying the groundwork for the development of deep neural network identification models.
Third, this paper applies the deep neural network from the field of machine learning to the subject of rural e-commerce entrepreneurship, broadening the applicability of the approach [i.e., beyond its current use in medical diagnosis (Mukherjee et al., 2021), natural language processing (Li et al., 2021c) and computer vision (Rajvanshi and Pramod, 2021)]. Furthermore, our prediction results demonstrate that the proposed method has a high accuracy in prediction (identification) (more than 90 percent). To some extent, this also adds to the methodological literature in the subject of rural e-commerce entrepreneurship.
Finally, our topic differs from typical entrepreneurship research in that it focuses on identifying rural e-commerce entrepreneurs. According to previous research on entrepreneurship, rural locations may suffer from poor communication and information infrastructure, high transit costs (Korsgaard et al., 2015), and a scarcity of money, labor, and resources (Lionais, 2004). Nevertheless, rural e-commerce business may emerge and thrive when communication (Gao and Liu, 2020; Ma et al., 2020) and logistical (Sousa et al., 2020) infrastructure is supported. This has increased the country’s and government’s investment and development in rural areas. Furthermore, the cost of starting a rural e-commerce business can be a lot lower (compared to urban areas), while the demand for labor is also relatively low (Gao and Liu, 2020; Li et al., 2021a). Most importantly, as an online platfotm, e-commerce may be applied to a variety of businesses and products in rural areas, and it has the potential for widespread use and promotion (Gao and Liu, 2020). Hence, this paper describes a developing entrepreneurial phenomena in rural areas and presents a method for identifying entrepreneurs in this setting.
4.2 Practical Implications
Local governments, e-commerce businesses, and rural e-commerce practitioners (including rural e-commerce entrepreneurs) can benefit from the findings of our research. First, local governments and e-commerce businesses should address practical issues relating to the rural areas and the local residents’ perceptions of feasibility and desirability for e-commerce entrepreneurship. Table 8 indicates that, using the index system we developed, a deep neural network may well be utilized to identify rural e-commerce entrepreneurs with an accuracy of more than 90%. This implies that we should focus on the measurement variables stated in the index system. Furthermore, Table 7 data reveal that the regression coefficients of MPK, DIDP, and EDD are significant (independent variable MPK belongs to the perceived feasibility, while DIDP and EDD belong to the perceived desirability). Therefore, offering training for locals who want to create their own rural e-commerce firms, provide opportunities for professional knowledge learning, improve entrepreneurial environment for rural e-commerce, and narrow the digital divide between rural and urban locations can support the development of more rural e-commerce businesses (Huang et al., 2021). Simultaneously, residents can be offered business visits and staff exchange opportunities so they can fully comprehend the potential that e-commerce has to transform and develop rural areas (Leng et al., 2020) and the role of rural e-commerce in enhancing rural resilience (Steiner and Atterton, 2015). These measures have the potential to boost practitioners’ expectations for the development of rural e-commerce in the future.
Second, rural e-commerce practitioners should seize the development opportunity of information and communication technology and use it to improve their professional knowledge and entrepreneurial abilities (Gao and Liu, 2020), so that they can help transform themselves into entrepreneurs and share the dividends of the digital economy’s development.
Finally, local administrations should keep up with the pace of digitization and informatization in order to better manage rural economic development. We present a deep neural network approach to identify rural e-commerce entrepreneurs in this work. This technique can assist local governments in making reasonable and efficient use of available data and resources in order to mine information that can be used to improve management methods and concepts (Hong and Wang, 2021) and give data support for rural economic development. In general, a practitioner who is motivated to launch a business in rural e-commerce but has not yet done so would face different challenges and obstacles than an entrepreneur who has already started a rural e-commerce firm. Different categories of rural e-merchants may be promptly and correctly identified using a deep neural network approach, allowing local governments to provide more targeted and effective support. Therefore, this study approach contributes to enhancing the current rural e-commerce entrepreneurship environment.
5 Conclusion
As information communication technology has progressed, the digital barrier between urban and rural communities has effectively narrowed, and urban-rural interactions are slowly gaining more traction. E-commerce is no longer a big-city business sector, as rural regions also have the basis and conditions for e-commerce development. Recently, several rural areas took advantage of the opportunities created by the increasing popularity and accessibility of the Internet and the online services, to launch rural e-commerce businesses. Rural residents, however, face several challenges when it comes to starting their own e-commerce enterprises, and local governments and rural e-commerce platform businesses find it difficult to provide accurate help to rural practitioners and entrepreneurs. To address this issue and promote sustainable entrepreneurship in rural e-commerce, we first created an index system based on a model of entrepreneurial events that may be used to identify rural e-commerce entrepreneurs. Eight factors influence rural inhabitants’ ability and expectation to create their own e-commerce enterprises, according to the index system. Following, we used a deep neural network approach to identify rural e-commerce entrepreneurs based on the data from these eight variables. The findings reveal that the index system we created has excellent reliability and validity, and the prediction method’s accuracy is greater than 90%.
Like any other study, there are certain limitations in this research. First and foremost, our research sample is modest and comes from a specific area, i.e., the Conghua District, Guangzhou, Guangdong Province. Therefore, our findings are limited to a specific region and may not be universal or deterministic. As such, further research can expand and include more geographic regions, while a comparison between our findings with finding of similar studies in other areas will allow researchers to see if comparable results can be reached for different parts of China or even in other countries. Second, this research developed an index system for identifying rural e-commerce entrepreneurs based on a literature review and the factors described as part of the entrepreneurial event model (perceived desirability and perceived feasibility). However, the index system’s list of potential criteria is not exhaustive. Factors such as personality and socio-demographic characteristics, psychological variables, educational and employment background (used as control variables in this study) and leadership capacity of an entrepreneur may have an impact on the individual’s potential to become a rural e-commerce entrepreneur. Our index system is focused on information from a specific body of literature and a single, albeit solid, theoretical framework. Therefore, researchers in the future may consider modifying the index system against the backdrop of various ideas and different bodies of literature. Third, the intelligence of deep neural networks makes identifying rural e-commerce businesses a viable option. However, it is worth investigating the potential of different approaches to identify rural e-commerce entrepreneurship compared to the one described in this work. Finally, the identification of rural e-commerce entrepreneurs is merely one of the steps required for information technology to empower rural economic growth. Future research can focus on themes such as the link between rural e-commerce entrepreneurship and poverty, the factors influencing the rural e-commerce entrepreneurship ecosystem, as well as the realization paths of sustainable entrepreneurship in rural e-commerce.
Data Availability Statement
The datasets presented in this study can be found in online repositories. The names of the repository/repositories and accession number(s) can be found below: https://github.com/96Guojie/data-and-code.
Ethics Statement
Written informed consent was obtained from the individual(s) for the publication of any potentially identifiable images or data included in this article.
Author Contributions
Conceptualization, GX and LH; methodology, GX; software, GX; validation, GX, LH and BH; formal analysis, LH; investigation, GX and LH; resources, LH and HB; data curation, GX; writing—original draft preparation, GX; writing—review and editing, GX, CA, YJ, GL and WC; visualization, GX; supervision, LH and HB; project administration, LH and HB; funding acquisition, LH and HB. All authors have read and agreed to the published version of the manuscript.
Funding
This research was funded by the National Social Science Foundation Of China, grant number 21BGL032, and 19BGL177; the MOE (Ministry of Education in China) Project of Humanity and Social Science Foundation, grant number 18YJA630001; 2021 Guangdong Province’s Colleges and Universities’ Typical Innovation Projects, grant number 2021WTSCX070; 2021 Hunan Province’s Scientific Research Project From Education Department, grant number 21A0348.
Conflict of Interest
The authors declare that the research was conducted in the absence of any commercial or financial relationships that could be construed as a potential conflict of interest.
Publisher’s Note
All claims expressed in this article are solely those of the authors and do not necessarily represent those of their affiliated organizations, or those of the publisher, the editors and the reviewers. Any product that may be evaluated in this article, or claim that may be made by its manufacturer, is not guaranteed or endorsed by the publisher.
Acknowledgments
The authors thank the editor and reviewers for their numerous constructive comments and encouragement that have improved our paper greatly. We are also grateful for the support of 2019 Guangdong Provincial Higher Education Teaching Reform Project (project title: exploration and practice of cross-border e-commerce talent training mode in universities under the initiative of “One Belt, One Road”), and the Comprehensive Educational Reform Project of E-commerce Major Teaching Steering Committee of Guangdong Undergraduate Universities (project title: mode and practice exploration of rural e-commerce innovation and entrepreneurship talent cultivation in the era of digital economy).
References
Abbas, J., Aqeel, M., Abbas, J., Shaher, B., Jaffar, A, Sundas, J., et al. (2019). The Moderating Role of Social Support for Marital Adjustment, Depression, Anxiety, and Stress: Evidence from Pakistani Working and Nonworking Women. J. Affect Disord. 244, 231–238. doi:10.1016/j.jad.2018.07.071
Aman, J., and Shi, G. (2021). Community Wellbeing under China-Pakistan Economic Corridor: Role of Social, Economic, Cultural, and Educational Factors in Improving Residents' Quality of Life. Front. Psychol. 12, 816592. doi:10.3389/fpsyg.2021.816592
Baidu encyclopedia (2021). Brief Introduction of Conghua District. Guangzhou. Available online: https://baike.baidu.com/item/%E4%BB%8E%E5%8C%96%E5%8C%BA/13020007?fr=aladdin (accessed on August 16, 2021).
Ben-Bassat, I., Chor, B., and Orenstein, Y. (2018). A Deep Neural Network Approach for Learning Intrinsic Protein-RNA Binding Preferences. Bioinformatics 34 (17), i638–i646. doi:10.1093/bioinformatics/bty600
Canziani, A., Paszke, A., and Culurciello, E. 2016. An Analysis of Deep Neural Network Models for Practical Applications. arXiv preprint arXiv:1605.07678.
Chao, P., Biao, M. A., and Zhang, C. (2021). Poverty Alleviation through E-Commerce: Village Involvement and Demonstration Policies in Rural China. J. Integr. Agric. 20 (4), 998–1011. doi:10.1016/S2095-3119(20)63422-0
Cui, M., Pan, S. L., and Cui, L. (2019). Developing Community Capability for E‐commerce Development in Rural China: A Resource Orchestration Perspective. Inf. Syst. J. 29 (4), 953–988. doi:10.1111/isj.12241
Diederich, J., and Milton, J. (1988). New Methods and Fast Algorithms for Database Normalization. ACM Trans. Database Syst. (Tods) 13 (3), 339–365. doi:10.1145/44498.44499
Gao, P., and Liu, Y. (2020). Endogenous Inclusive Development of E‐commerce in Rural China: A Case Study. Growth and Change 51 (4), 1611–1630. doi:10.1111/grow.12436
Ge, T., Azhar, A., Ullah, R., Sadiq, I., and Zhang, R. (2022). Women's Entrepreneurial Contribution to Family Income: Innovative Technologies Promote Females' Entrepreneurship amid COVID-19 Crisis. Front. Psychol. 12, 828040. doi:10.3389/fpsyg.2022.828040
Guo, M. R., Li, J., and Feng, X. (2017). Research on Integrated Urban and Rural Development Modes under the Circumstances of “Internet +”. China Soft Sci. (09), 10–17. Chinese.
Heale, R., and Twycross, A. (2015). Validity and Reliability in Quantitative Studies. Evid Based. Nurs. 18 (3), 66–67. doi:10.1136/eb-2015-102129
Hong, M., and Wang, H. (2021). Research on Customer Opinion Summarization Using Topic Mining and Deep Neural Network. Mathematics Comput. Simulation 185, 88–114. doi:10.1016/j.matcom.2020.12.009
Huang, L., Xie, G., Huang, R., Li, G., Cai, W., and Apostolidis, C. (2021). Electronic Commerce for Sustainable Rural Development: Exploring the Factors Influencing BoPs’ Entrepreneurial Intention. Sustainability 13, 10604. doi:10.3390/su131910604
Huang, L., Zhao, W., and Xie, G. (2017). Evaluating and Optimizing City E-Commerce Competitiveness Based on FA-CA Model: A Case from 32 Cities in China. Revista de la Facultad de Ingenieria 32, 298–308.
Huang, X., and Zhou, Y. G. (2015). History, Actuality and Development of China’s E-Commerce from the Perspective of Alibaba Listing. Commercial Res. (12), 11–17. Chinese.
Korsgaard, S., Ferguson, R., and Gaddefors, J. (2015). The Best of Both Worlds: How Rural Entrepreneurs Use Placial Embeddedness and Strategic Networks to Create Opportunities. Entrepreneurship Reg. Dev. (9-10), 574–598. doi:10.1080/08985626.2015.1085100
Krueger, N. (1993). The Impact of Prior Entrepreneurial Exposure on Perceptions of New Venture Feasibility and Desirability. Entrepreneurship Theor. Pract. 18 (1), 5–21. doi:10.1177/104225879301800101
Leng, C., Ma, W., Tang, J., and Zhu, Z. (2020). ICT Adoption and Income Diversification Among Rural Households in China. Appl. Econ. 52, 3614–3628. doi:10.1080/00036846.2020.1715338
Li, J., Liu, X., Yin, W., Yang, M., Ma, L., and Jin, Y. (2021). Empirical Evaluation of Multi-Task Learning in Deep Neural Networks for Natural Language Processing. Neural Comput. Appl. 33 (9), 4417–4428. doi:10.1007/s00521-020-05268-w
Li, J., Wang, D., Duan, K., and Mubeen, R. (2021). Tourists' Health Risk Threats amid COVID-19 Era: Role of Technology Innovation, Transformation, and Recovery Implications for Sustainable Tourism. Front. Psychol. 12, 769175. doi:10.3389/fpsyg.2021.769175
Li, L., Zeng, Y., Ye, Z., and Guo, H. (2021). E‐commerce Development and Urban‐rural Income gap: Evidence from Zhejiang Province, China. Pap. Reg. Sci. 100 (2), 475–494. doi:10.1111/pirs.12571
Li, Y. N. (2008). The Reform of the Rural-Urban Dualism. J. Peking University(Philosophy Soc. Sciences) (02), 5–11.
Lin, G., Xie, X., and Lv, Z. (2016). Taobao Practices, Everyday Life and Emerging Hybrid Rurality in Contemporary China. J. Rural Stud. 47, 514–523. doi:10.1016/j.jrurstud.2016.05.012
Lionais, D. (2004). Depleted Communities and Community Business Entrepreneurship: Revaluing Space through Place. Entrepreneurship Reg. Dev. 16 (3), 217–233. doi:10.1080/0898562042000197117
Liu, F., Wang, D., Duan, K., and Mubeen, R. (2021). Social media Efficacy in Crisis Management: Effectiveness of Non-pharmaceutical Interventions to Manage the COVID-19 Challenges. Front. Psychiatry 12 (1099), 626134. doi:10.3389/fpsyt.2021.626134
Liu, Q., Qu, X., Wang, D., and Mubeen, R. (2021). Product Market Competition and Firm Performance: Business Survival through Innovation and Entrepreneurial Orientation amid COVID-19 Financial Crisis. Front. Psychol. 12 (4910), 790923. doi:10.3389/fpsyg.2021.790923
Liu, W., Wang, Z., Liu, X., Zeng, N., and Liu, Y., (2017). A Survey of Deep Neural Network Architectures and Their Applications. Neurocomputing 234, 11–26. doi:10.1016/j.neucom.2016.12.038
Liu, Y. J. (2018). Double-Case Study on Endogenous Inclusive Growth Promoted by Internet Enabling and Bottom-Of-The-Pyramid Entrepreneurship. Chin. J. Manag. 15, 28–38. Chinese.
Ma, W., Zhou, X., and Liu, M. (2020). What Drives Farmers’ Willingness to Adopt E‐commerce in Rural China? the Role of Internet Use. Agribusiness 36 (1), 159–163. doi:10.1002/agr.21624
Mei, Y., Mao, D., Lu, Y., and Chu, W. (2020). Effects and Mechanisms of Rural E‐commerce Clusters on Households' Entrepreneurship Behavior in China. Growth and Change 51 (4), 1588–1610. doi:10.1111/grow.12424
Mubeen, R., Han, D., Abbas, J., Álvarez-Otero, S., and Sial, M. S. (2021). The Relationship between CEO Duality and Business Firms' Performance: The Moderating Role of Firm Size and Corporate Social Responsibility. Front. Psychol. 12, 4817. doi:10.3389/fpsyg.2021.669715
Mukherjee, H., Ghosh, S., Dhar, A., Obaidullah, S. M., Santosh, K. C., and Roy, K. (2021). Deep Neural Network to Detect COVID-19: One Architecture for Both CT Scans and Chest X-Rays. Appl. Intelligence 51 (5), 2777–2789. doi:10.1007/s10489-020-01943-6
Peng, Y., Peng, Z., and Lan, T. (2021). Neural Network Based Inverse Kinematics Solution for 6-R Robot Implement Using R Package Neuralnet. 2021 5th International Conference on Robotics and Automation Sciences (ICRAS). IEEE, 65–69. doi:10.1109/icras52289.2021.9476364
Rajvanshi, A., and Pramod, D. (2021). Queue Time Estimation in Checkout Counters Using Computer Vision and Deep Neural Network. J. Phys. Conf. Ser. IOP Publishing (6), 062108. doi:10.1088/1742-6596/1964/6/062108
Raza, S., Nurunnabi, M., Minai, M. S., and Bano, S. (2019). The Impact of Entrepreneurial Business Networks on Firms' Performance through a Mediating Role of Dynamic Capabilities. Sustainability 11 (11), 3006. doi:10.3390/su11113006
Rockwell, R. C. (1975). Assessment of Multicollinearity: The Haitovsky Test of the Determinant. Sociological Methods Res. 3 (3), 308–320. doi:10.1177/004912417500300304
Shapero, A., and Sokol, L.The Social Dimensions of Entrepreneurship (1982). University of Illinois at Urbana-Champaign's Academy for Entrepreneurial Leadership Historical Research Reference in Entrepreneurship. Available at SSRN: https://ssrn.com/abstract=1497759.
Shapero, A. (1985). Why Entrepreneurship? A Worldwide Perspective. J. Small Business Manag. (pre-1986) 23 (000004), 1.
Shen, K. N., Lindsay, V., and Xu, Y. (2018). Digital Entrepreneurship. Inf. Syst. J. 28 (6), 1125–1128. doi:10.1111/isj.12219
Sohu net (2021) Study on Evolution Process and Path of Rural E-Commerce. Available online: https://www.sohu.com/a/358974694_796374 (accessed on July 5, 2021).
Sousa, R., Horta, C., Ribeiro, R., and Rabinovich, E. (2020). How to Serve Online Consumers in Rural Markets: Evidence-Based Recommendations. Business Horizons 63 (3), 351–362. doi:10.1016/j.bushor.2020.01.007
Steiner, A., and Atterton, J. (2015). Exploring the Contribution of Rural Enterprises to Local Resilience. J. Rural Stud. 40, 30–45. doi:10.1016/j.jrurstud.2015.05.004
Su, Z., McDonnell, D., Wen, J., Kozak, M., Šegalo, S., and Xiang, Y.-T. (2021). Mental Health Consequences of COVID-19 media Coverage: the Need for Effective Crisis Communication Practices. Globalization and Health 17 (1), 4. doi:10.1186/s12992-020-00654-4
The Southern Plus (2021). The First Half of the Year Had a Turnover of More Than 100 Million Yuan! Agricultural Products from Conghua Are Flying All over the Country. Available online: http://static.nfapp.southcn.com/content/201709/21/c690073.html (accessed on August 16, 2021).
Tirdad, K., Cruz, A. D., Sadeghian, A., and Cusimano, M. (2021). A Deep Neural Network Approach for Sentiment Analysis of Medically Related Texts: an Analysis of Tweets Related to Concussions in Sports. Brain Inform. 8 (1), 1–17. doi:10.1186/s40708-021-00134-4
Wen, Y. G., Kang, Y. N., and Zang, W. H. (2012). The Study on Chinese Agricultural E-Commerce Development. Software Engineering and Knowledge Engineering: Theory and Practice. Berlin, Heidelberg: Springer.
Yu, H., and Cui, L. (2019). China's E-Commerce: Empowering Rural Women? China Q. 238, 418–437. doi:10.1017/s0305741018001819
Zhang, Q., Hussain, I., Akram, S., Afaq, A., and Shad, M. A. (2020). Sustainable Innovation in Small Medium Enterprises: The Impact of Knowledge Management on Organizational Innovation through a Mediation Analysis by Using SEM Approach. Sustainability 12 (6), 2407. doi:10.3390/su12062407
Keywords: rural e-commerce, entrepreneurs, sustainable entrepreneurship, entrepreneurship event model, neural networks
Citation: Xie G, Huang L, Bin H, Apostolidis C, Jiang Y, Li G and Cai W (2022) Sustainable Entrepreneurship in Rural E-Commerce: Identifying Entrepreneurs in Practitioners by Using Deep Neural Networks Approach. Front. Environ. Sci. 10:840479. doi: 10.3389/fenvs.2022.840479
Received: 21 December 2021; Accepted: 21 March 2022;
Published: 05 May 2022.
Edited by:
Maria Urbaniec, Kraków University of Economics, PolandReviewed by:
J. Abbas, Shanghai Jiao Tong University, ChinaNorziani Dahalan, University of Science Malaysia, Malaysia
Copyright © 2022 Xie, Huang, Bin, Apostolidis, Jiang, Li and Cai. This is an open-access article distributed under the terms of the Creative Commons Attribution License (CC BY). The use, distribution or reproduction in other forums is permitted, provided the original author(s) and the copyright owner(s) are credited and that the original publication in this journal is cited, in accordance with accepted academic practice. No use, distribution or reproduction is permitted which does not comply with these terms.
*Correspondence: Lijuan Huang, c29taHVhbmdsakBnemh1LmVkdS5jbg==; Hou Bin, Ymh2MTY4QGhudS5lZHUuY24=