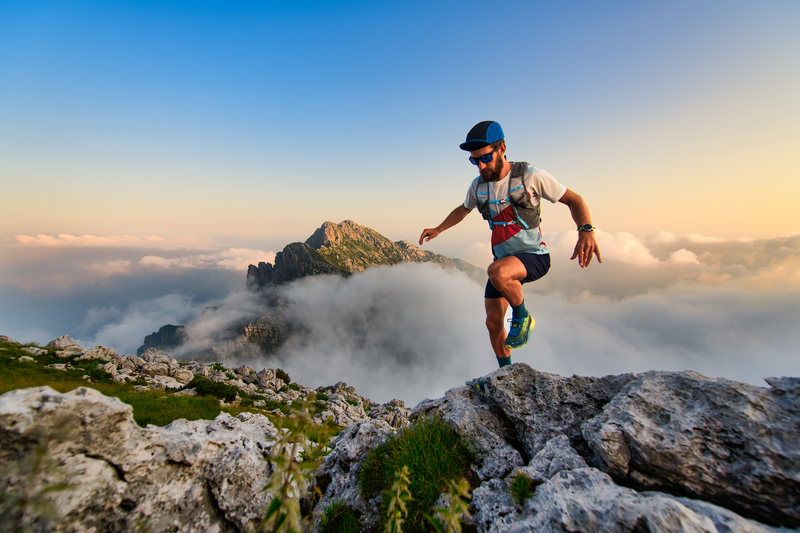
94% of researchers rate our articles as excellent or good
Learn more about the work of our research integrity team to safeguard the quality of each article we publish.
Find out more
ORIGINAL RESEARCH article
Front. Environ. Sci. , 26 January 2022
Sec. Water and Wastewater Management
Volume 10 - 2022 | https://doi.org/10.3389/fenvs.2022.838883
This article is part of the Research Topic Flood Management: Multi-Disciplinary Approaches for Data Observation, Analysis, Forecasting, and Management View all 4 articles
Lakes are of significant importance in regulating floods and providing water sources. The seasonal water storage variations for the plain lake group in the Yangtze–Huai River Basin (YHRB) are significant for alleviating flood pressure and regulating runoff. However, to date, the seasonal amplitude of lake water storage variations and its capacity of buffering floodwater in the YHRB is not quantified well and remains to be investigated comprehensively. To advance the understanding of such a critical scientific issue, the water level data of the plain lake group (area>100 km2, 29 lakes) in the YHRB is collected from multi-source data between 1990 and 2020. Using lake inundation area obtained from Global Surface Water and water level variations, water storage dynamics for the plain lake group are quantified. Furthermore, this study also uses the Gravity Recovery and Climate Experiment (GRACE) products to analyze the terrestrial water storage anomalies (TWSA) in the whole basin. The results indicate that the seasonal amplitude of water level change and water storage variation of the plain lake group are 2.80 ± 0.71 m and 37.38 ± 14.19 Gt, respectively. Poyang and Dongting Lakes, two lakes that maintain the natural connection with the Yangtze River, have the most substantial seasonal amplitude in the hydrological situation. The amplitude in water level and water storage in Poyang Lake is 9.53 ± 2.02 m and 14.13 ± 5.54 Gt respectively, and that in Dongting Lake is 7.39 ± 1.29 m and 7.31 ± 3.42 Gt respectively. The contribution of seasonal variation of water storage for large plain lakes to TWSA in the YHRB is approximately 33.25%, fully reflecting these lake’s imperative position in the YHRB. This study is expected to enhance the scientific understanding of the seasonal hydrologic regime for the large lakes in the YHRB and contribute to the management of flood risks and water resources in East China.
As a proportion of the earth’s hydrosphere, lakes play an imperative role in maintaining ecological balance, providing freshwater resources, and preventing floods (Verpoorter et al., 2014; Yang et al., 2015; Wang et al., 2018; Zhu et al., 2020; Cooley et al., 2021). With the significant climate change and increased anthropogenic intervention in the past few decades, extreme natural disasters have occurred frequently (e.g., floods or droughts) (Messager et al., 2016; Marsooli et al., 2019; Bloschl et al., 2020). The most serious floods in the world usually occur near rivers and coastal regions (such as China, the United States, and India) (Xie et al., 2018; Marsooli et al., 2019; Yang et al., 2021). According to statistics, from 2000 to 2019, the losses caused by global flood disasters reached about 651 billion (USD) dollars, with the proportion of the world’s population exposed to flooding increasing (UN Office for Disaster Risk Reduction, 2020; Tellman et al., 2021). Therefore, it is necessary to accurately quantify and assess the flood storage capacity of lakes for water resources management.
The Yangtze–Huai River Basin (YHRB) is located in East China, with flat terrain, interlaced rivers, and numerous lakes (Liu, 2018; Yao et al., 2020). On account of the impact of the East Asian summer monsoon, the YHRB suffers frequent rainfalls from June to August, which makes it vulnerable to form flood disasters (Ye and Glantz, 2005; Liu & Li, 2014; Xie et al., 2018; Wang et al., 2021b). The seasonal amplitude of water storage, which reflects the seasonal variation of the hydrological regime of lakes, is an imperative element in the regional water budget (Ye and Glantz, 2005; Klein et al., 2021). The water storage variation of lakes in the YHRB has vast seasonal fluctuations, which is significant for regulating river runoff, reducing flood disasters, and maintaining human wealth and biodiversity.
Given the ecological and socioeconomic importance of the lake, several studies have been carried out on the lake water dynamics in the YHRB. For instance, the inter-annual and seasonal fluctuation in the water area and water level of certain large lakes in the YHRB have been estimated using middle and high-resolution satellite image and altimetry satellite data. These lakes are mainly targeted in the top largest lakes, such as Poyang Lake (Song and Ke, 2014; Mei et al., 2015; Zeng et al., 2017; Wang et al., 2019b; Mu et al., 2020), Dongting Lake (Huang et al., 2011; Hu et al., 2015; Xing et al., 2018; Long et al., 2019; Wang et al., 2021a), Taihu Lake (Hu and Wang, 2009; Zhao et al., 2012; Wang et al., 2019c; Xu et al., 2020b), Hongze Lake (Yin et al., 2013; Cai et al., 2020; Mei et al., 2021) and Chaohu Lake (Chen et al., 2013; Lin et al., 2021), which have a significant impact on the surrounding ecological environment. At present, research related to water storage mostly exists in Poyang Lake (the largest freshwater lake in China) (Liu H. et al., 2020; Xu et al., 2020a; Song et al., 2021), while the estimate on seasonal water storage changes of other lakes in the YHRB still remains poorly quantified. In addition, most of the existing researches also have concentrated on monitoring the lake water dynamics in the sub-basins of the YHRB [including the Middle and Lower Reaches of Yangtze River Basin (MLRYRB) and the Huai River Basin (HRB)] (Sun et al., 2014; Wang et al., 2014; Li L. et al., 2015; Cai et al., 2016; Ye et al., 2017; Xia et al., 2019; Li P. et al., 2020). For instance, Cai et al. (2016) utilized area-based water storage estimation models to quantify the water storage dynamics of large lakes and reservoirs in the Yangtze River Basin from 2000 to 2014. Various efforts have improved our understanding of the lake’s hydrologic budget in the YHRB. However, comprehensive quantification of the seasonal amplitude of water storage variations in the YHRB plain lake group has not yet been well addressed due to the difficulty of obtaining the full-covered water level records or lake bathymetry on a regional scale.
Stimulated by the urgent requirement for precise information about seasonal lake water storage variations in the YHRB, the primary objective aim of this research is to quantify the seasonal amplitude of water storage variations in the YHRB plain lake group for better understanding their flood regulation and storage capacity. Here, we quantify the lake water storage variations using the lake area change based on the Global Surface Water (GSW) datasets and multi-source water level data. In addition, this study also analyzes the seasonal amplitude of terrestrial water storage (TWS) derived from the Gravity Recovery and Climate Experiment (GRACE) and the contribution of lakes to TWS in the YHRB. A comprehensive investigation of lake flood regulation and control capacity is expected to inform scientific guidance and policy initiatives for flood regulation and water resources management in East China.
The YHRB includes the Middle and Lower Reaches of the Yangtze River basin (MLRYRB) and the Huai River Basin (HRB), which roughly covers an area of 1,064,156 km2 (28°–35°N, 111°–121°E, presented in Figure 1). The YHRB is a low-lying alluvial plain generally below 50 m in elevation and is composed of abundant lakes and rivers (Liu, 2018; Li P. et al., 2020). From June to August, the YHRB experiences frequent rainfall because of the affection of East Asian summer monsoon, called “plum rain” or “Mei-yu” season. The average annual precipitation in the YHRB is between 900 and 1,400 mm, and 50–75% of the rainfall is concentrated in the East Asian summer monsoon season (Wang et al., 2016; Song et al., 2020). Therefore, the plain lake group consisting of 29 lakes with an inundation area of more than 100 km2 is selected in the YHRB. Among these lakes, China’s five largest freshwater lakes (including Poyang, Dongting, Taihu, Hongze, and Chaohu Lakes) are all located in this basin, which constitutes an essential proportion of the YHRB lake system. Lakes in the YHRB are the vital water sources in China and play a critical part in various economic and ecological functions, such as irrigation, hydropower, and flood storage.
FIGURE 1. The geographic distribution of the plain lake group (29 studied lakes) in the Yangtze–Huai River Basin (YHRB).
The Global Surface Water (GSW, https://global-surface-water.appspot.com/) dataset generated from Landsat 5, 7, and 8 scenarios (4,453,989 images) with a high spatial resolution of 30 m, used to depict the dynamics of global surface water (Pekel et al., 2016). The dataset applies a dedicated expert system for water extraction and detection, continuously updated to the present (since 16 March 1984). The surface water occurrence map (varying from 0 to 100%) refers to the frequency of surface water in each pixel. The GSW surface water occurrence data is used for this study. The lake water bodies with water frequency (WF) less than or equal 10, 25, 50, 75, and 90% are extracted to calculate the lake area in different wet-to-dry periods. We need to convert the vector data into equal-area projection before calculating the areas.
The water levels of the targeted lakes are derived from five different data sources, including the satellite altimetry data accessed in the Hydroweb from the Laboratoire d'Etudes en Géophysique et Océanographie Spatiales (LEGOS) and the database for Hydrological Time Series of Inland Waters (DAHITI), and gauging station data from hydrological station websites, literature, and government public report. Many lakes have hydrological stations around them, but obtaining water level data from in situ records is a challenging task. In this research, the in-situ measured daily water level time series of Poyang Lake (1990–2017) and Taihu Lake (1990–2020) are obtained from Jiangxi Provincial Hydrology Monitoring Center (http://www.jxssw.gov.cn/) and Taihu Lake Basin Administration bureau of the Ministry of Water Resources (http://www.tba.gov.cn/), respectively. In addition, Hydroweb (Crétaux et al., 2011) and DAHITI (Schwatke et al., 2015) provide multi-mission satellite altimetry of water level time series for lakes, which has been broadly utilized in hydrological research (Liu et al., 2019; Zhan et al., 2020; Zhang X. et al., 2021; Fan et al., 2021). The lake water level records are provided by combining several altimetry satellite products, including Ocean Topography Experiment/Poseidon Mission (TOPEX/Poseidon), European Remote-Sensing Satellite (ERS), Jason-1/2/3 Ocean Surface Topography Mission, Cryosphere Satellite (CryoSat), Environmental Satellite (ENVISAT), Satellite for ARgos and ALtika (SARAL), and Sentinel-3 Satellite. The water level products of three lakes (Dongting, Hongze, and Hong Lakes) are gained from the Hydroweb (http://hydroweb.theia-land.fr/). Water level time series of four lakes (Poyang, Dongting, Chaohu, and Junshan Lakes) are attained from the DAHITI (https://dahiti.dgfi.tum.de/en/). The water level time series of 16 lakes are collected from different types of literature (shown in Table 1).
TABLE 1. Geographic location and water level data source of 29 lakes in the YHRB (rank lakes by lake water area, NWL indicates the normal water level, WWL indicates the warning water level, Min_WLC indicates the annual minimum water level change, and Max_WLC indicates the maximum water level change).
In addition, ten lakes failed to gather the time series of water levels. According to the statistical data of the local water resources bureau, the difference between the normal water level (NWL) and the warning water level (WWL) is considered as the annual water level change for seven lakes (Wabu, Nanyi, Nvshan, Futou, Baima, Chengxi, and Xiliang Lakes). The average of the annual minimum water level change (Min_WLC) and maximum water level change (Max_WLC) is regarded as the annual water level change for three lakes (Luoma, Shijiu, and Yangcheng Lakes). The seasonal water level amplitude uncertainty for these lakes is replaced by the average standard deviation of lake water level change in the sub-basins. The specific data sources for each lake are described in Table 1.
In order to evaluate the accuracy of lake water level data, this paper cross-validates the lake water level data that can be obtained from multi-source data. Due to data limitations, only the water level data of Poyang, Dongting, and Hong Lakes are verified in this paper (the results are presented in Figure 2). Correlation analysis shows that the fitting relationship between water levels from different sources of Poyang, Dongting, and Hong Lakes are significant (p < 0.01), the R2 is 0.97, 0.98, and 0.84, respectively. To reduce the error between water level data obtained from different data sources for the lakes (including, Dongting, Hongze, Longgan, Chaohu, and Hong Lakes), the average value of water level variation calculated by each water level data source is used as the seasonal amplitude of water level change.
FIGURE 2. Scatter plots of water level obtained from Hydroweb or DAHITI against in-situ gauge measurements. (A) Poyang Lake; (B) Dongting Lake; and (C) Hong Lake.
The seasonal amplitude of water storage variations of 27 lakes is estimated by combining the lake area and water level (except Poyang and Dongting Lakes). This paper uses the empirical formula (1) to estimate water storage change (Crétaux et al., 2016; Zhang et al., 2019; Luo et al., 2021).
where ΔV represents the water storage variation; H1, H2 (m) mean the water levels at different times, respectively; A1, A2 (km2) represent the lake areas at the corresponding stages. Multiply by a factor of 0.001 to convert the unit of the output result to km3. It should be noted that 1 km3 of water has a mass of one Gigatonne (1 Gt), so this article uses Gt as the ΔV unit.
In our study, the intra-annual water level variation is used as the fluctuation from H1 to H2 to estimate the storage variations. We divided the WF fluctuation into three combinations of 10–90%, 25–75% as the lake area change to quantify the seasonal amplitudes of water storage variation at different stages. The lake water body area with WF less than or equal to 50% is calculated as the average lake area. This variability in water level may be converted to an approximate storage change by multiplying the height change by the average lake area.
In addition, combined with the monthly water level data of Poyang and Dongting Lakes, the seasonal amplitude of water storage variations in Poyang and Dongting Lakes is estimated respectively by using the hypsometric curve between water level and water storage proposed by Li et al. (2019) and Kameyama (2004), respectively. Furthermore, we collected bathymetric DEM of Poyang Lake (the Hydrological Bureau of Jiangxi Province, http://www.jxssw.gov.cn/) and Dongting Lake (the Hydrological Bureau of Hunan Province, http://slt.hunan.gov.cn/hnsw/), which is applied to display their complex terrain and lake forms.
The GRACE and GRACE-FO satellites, which are designed to measure the gravity field over time, are used to evaluate spatial-temporal variability in TWS (including surface water, snow water, groundwater, soil moisture, and biomass) (Song et al., 2015; Yin et al., 2020; Sun et al., 2021). The service period of the GRACE satellites was from March 2002 to June 2017. Its follow-on satellite is GRACE-FO, launched in May 2018 and continues to collect data until now. GRACE satellites consisted of two satellites with a distance of 220 km and an orbital altitude of 300–500 km. GRACE-FO satellites operate at lower orbits and shorter inter-satellite distances than GRACE satellites, improving the resolution accuracy of global gravity field products. The official GRACE products are managed and released by three data processing agencies, namely, the Centre for Space Research (CSR), GeoForschungsZentrum Potsdam (GFZ), and Jet Propulsion Laboratory (JPL). The current Release-06 monthly gridded GRACE product (RL06) has consisted of a set of the spherical harmonic coefficients (SHC) data and mass concentration (mascon) data.
To minimize the uncertainty in solving the original data, we averaged the three RL06 spherical harmonic solutions from CSR, JPL, and GFZ for this study, with a spatial resolution of 1 × 1°. Calculate the standard deviation of the three solutions to represent the uncertainty in the different GRACE products. Utilizing the same equal-area projection, the equivalent water thickness (averaged_SH/cm) is transformed into water mass change (GRACE_SHC/Gt) to compare better the difference between TWS changes and water storage variation from water level and area. All monthly TWS anomalies (TWSA) data are displayed as anomalies relative to the time-mean baseline from January 2004 to December 2009 (Tapley et al., 2004; Dahle, 2018; Kornfeld et al., 2019). Due to satellite sensors and other aspects, data of some months are missing. The missing data are filled with linear interpolation using observation month data before and after the missing month. The method of using linear interpolation to fill the gap of GRACE products has also been widely applied in other studies (Ramillien et al., 2006; Long et al., 2015; Song et al., 2015). We derived the seasonal amplitudes of GRACE TWS for each grid square in the YHRB by calculating the multi-year average of time-series annual difference of the maximum and minimum monthly TWS values within each year during 2003–2020 (except for 2017 and 2018). Besides, the monthly time series of TWSA for the two units HRB and MLRYRB were calculated by spatial averaging of the GRACE grids within the basin extent.
The correlation of water level data from the different sources is evaluated with the determinate coefficient R2 (R2) value. In the unary regression analysis, the range of R2 values is both [−1, 1]. For the R2 value, the value of 1 figures the perfect fit (Fan et al., 2021). Besides, the standard deviation (Std) is utilized to quantify the fluctuation range in the lakes’ water level and storage variations. Specifically, the formula of R2 and Std are shown as follows:
where, yi and fi are the ith observed and predicted value, respectively; ‾y is the mean value of y; n represents the total number of observations.
Based on water levels from the hydrological station and multi-source altimetry data, the water level changes in the YHRB for the last 30 years are analyzed. The result of the lake level changes for the plain lake group is shown in Figure 3. The seasonal variation amplitude of lake level in the YHRB presents a heterogeneous pattern. The seasonal water level changes are significantly more substantial for lakes directly connected with the mainstreams of the Yangtze River or Huai River than closed lakes. The mean seasonal amplitude of water level variations for the plain lake group is approximately 2.80 ± 0.71 m. There are 12 lakes with seasonal water level change exceeding the average amplitude, accounting for 41% of the total examined lakes. Poyang and Dongting Lakes are still naturally connected with the Yangtze River (Zhang et al., 2014; Zhang C. et al., 2021), with complex river-lake interaction and considerable seasonal amplitude in the water level cycle, which are 9.53 ± 2.02 m and 7.39 ± 1.29 m, respectively. On the other hand, Taihu Lake has the slightest seasonal dynamic change of water level (0.76 ± 0.42 m), mainly caused by artificial management such as the construction of embankments and dams (Wang J. et al., 2019; Wang et al., 2019c).
FIGURE 3. The spatial distribution of seasonal amplitudes of lake water level variations in the YHRB.
The variation of lake areas varies with different water frequencies, and the three scenarios of WF changes (water area of 10–90%, 25–75%, and 50% WF) for 27 lakes are indicated in Figure 4C. In this section, the lake surface water area estimated at 50% WF is selected as the average area to quantify the seasonal amplitude of water storage variation of 27 lakes, except for Poyang and Dongting Lakes. Poyang and Dongting Lakes show too strong spatial heterogeneity in inundation area dynamics and thus are estimated directly according to the hypsometric curve. The total water storage regulation value of the plain lake group in the YHRB is approximately 37.38 ± 14.19 Gt. The water storage variation of twenty lakes in the MLRYRB is 31.01 ± 13.07 Gt, 4.87 times that of the nine lakes in the HRB (6.37 ± 1.12 Gt). Figure 4A and Figure 4B display the lake bathymetry of Poyang and Dongting Lakes, respectively. Poyang and Dongting Lakes have considerable water storage changes, which are 14.13 ± 5.54 Gt and 7.31 ± 3.42 Gt, respectively, accounting for 57% of the seasonal amplitude of water storage variations in the YHRB. Other large lakes, including Taihu, Hongze, Gaoyou, and Chaohu Lakes, which all exceed 800 km2, have seasonal storage variations of 1.80 ± 0.98 Gt, 1.56 ± 0.30 Gt, 0.85 ± 0.31 Gt, and 1.23 ± 0.81 Gt, respectively.
FIGURE 4. The seasonal amplitude of water storage variations for the plain lake group. (A) Bathymetry of Poyang Lake; (B) Bathymetry of Dongting Lake; and (C) The seasonal amplitude of water storage variations for 27 lakes (ranking by lake water inundation area, with the exclusion of Poyang and Dongting Lakes).
Poyang and Dongting Lakes are directly connected with the mainstream of Yangtze River with large seasonal fluctuations, effectively alleviating the flood threat in the surrounding areas (Yao et al., 2018; Wang et al., 2019b; Li et al., 2021). Figure 5 shows the time series of the water storage and the relative change percentage of water storage (RCPWS) for Poyang and Dongting Lakes spanning 1990 to 2020. The RCPWS is calculated with the long-term mean value as the reference, and the long-term mean values of Poyang Lake and Dongting Lake are 6.00 Gt and 5.82 Gt respectively. The water storage of Poyang and Dongting Lakes has substantial inter-annual and seasonal amplitudes. The strongest seasonal fluctuations in water storage can be observed in 1998 and 2020 (as the extreme flood year), the water storage variations in Poyang Lake are 26.80 Gt and 24.35 Gt in 1998 and 2020, respectively, and that in Dongting Lake is 12.96 Gt and 11.04 Gt. Compared with the seasonal amplitude of multi-year average water storage variations, the water storage variations in Poyang and Dongting Lakes increased by approximately 81 and 68%, respectively. In actual situations, the surrounding areas of Poyang and Dongting Lakes have been damaged by floods (Zong and Chen, 2000; Ye and Glantz, 2005; Wang et al., 2021b; Chen et al., 2021). Besides, Taihu, Gaoyou, Hongze, Chaohu, and other lakes are related to the Yangtze River through tributaries, and their outlet is far from the Yangtze River, resulting in small seasonal fluctuations and relatively weaker flood regulation capacity. Owing to the scarcity of long-time water level data for other lakes, this section only makes a specific analysis of the long-term water storage changes of Poyang and Dongting Lakes from 1990 to 2020.
FIGURE 5. Time series of the water storage and the relative change percentage of water storage (RCPWS) for Poyang and Dongting Lakes during 1990–2020.
Figure 6 illustrates the spatial-temporal patterns of seasonal amplitude and the linear trends for TWS in the YHRB derived from the GRACE observations between 2003 and 2020, except for 2017 and 2018. The seasonal variation amplitude of TWS in the YHRB exhibits an uneven distribution, and the variation range of TWS in the MLRYRB (mostly blue) is significantly greater than that in the HRB (mainly orange). The seasonal TWS amplitudes of the MLRYRB and HRB are 90.47 ± 21.95 Gt and 27.42 ± 6.40 Gt, respectively. The GRACE TWSA increased significantly in the MLRYRB, with a change rate of 2.66 ± 0.46 Gt/yr, p < 0.01. The annual TWSA in the MLRYRB showed a noticeable periodic fluctuation, mostly reaching the peak in summer due to the influence of monsoonal rain. The seasonal amplitude for TWS in HRB also has obvious periodic changes (mostly reaches the peak in autumn) and shows a distinct downward trend (with a change rate of −1.72 ± 0.14 Gt/yr, p < 0.01), which is mainly due to the continuous improvement of urbanization level and the increase of groundwater consumption in the HRB (Yi et al., 2016; Su et al., 2020). The seasonal water storage changes of the plain lake group contributed approximately 33.25% to the TWSA (in mass) in the YHRB, which perfectly reflects the important position of these lakes in the YHRB.
FIGURE 6. Spatial-temporal patterns of seasonal amplitude and the linear trends for TWS in the YHRB derived from the GRACE observations between 2003 and 2020, except for 2017 and 2018. (A) Spatial distribution of the seasonal amplitude of TWS in the YHRB; (B) Long-term variation characteristics of GRACE TWSA in the HRB; and (C) Long-term variation characteristics of GRACE TWSA in the MLRYRB.
One hundred forty-four lakes with an area between 10 and 100 km2 are distributed in the YHRB, with a gross surface water area of 4,060.74 km2. In compliance with the lake WF extracted from GSW, most of these lakes are distributed near the Yangtze River and Huai River, as shown in Figure 7. Since these lakes are relatively small, it is challenging to obtain time series of water level data. Thus, the annual water level change amplitude of YHRB large lakes (area>100 km2) estimated in Analysis of Seasonal Amplitude of Water Level Variations for the Plain Lake Group is used to estimate these unrecorded lake’s seasonal amplitude roughly. This section uses the area with the WF equaling 50% to calculate lake water storage change. To gain the water storage variation of lakes (area>10 km2), the water storage changes of lakes with an area of 10–100 km2 are deduced based on the variation formula of water storage mentioned in Estimation of the Seasonal Amplitude of Water Storage Variations. As a result, the total annual water storage change of all lakes is 44.81 ± 16.01 Gt, of which the total water storage change of the 144 unrecorded lakes is 7.43 ± 1.82 Gt, accounting for approximately 17% of the water storage variation in the YHRB. Limited by the scope of lake water area, these small lakes are not as good as YHRB large lakes in terms of water storage regulation. Nevertheless, they remain critical players in local biophysical environments and socioeconomic development.
Ideally, the seasonal amplitude of lake water storage variations should be quantified using their bathymetry data (Cai et al., 2016; Jiang et al., 2017; Schwatke et al., 2020). However, it is problematic to acquire the lake underwater topography for most lakes in the YHRB, which hinders error-free estimation of water storage variation. As a compromise, this study integrates the water area extracted from GSW and the water level data collected from multi-source altimetry or in-situ observations to quantify lake water storage variation. The low revisit period (16 days) and high cloud coverage probability of Landsat images make it hard to identify the monthly lake inundation area in the YHRB precisely. Therefore, using the surface water occurrence of GSW to extract lake area changes at different stages can better represent the variations of lake water bodies over the years. Previous studies have also demonstrated that surface water occurrence can be used to characterize changes in water area (Pekel et al., 2016; Fang et al., 2019; Luo et al., 2019). According to the variation of water area with WF fluctuation of 10–90% and 25–75%, the seasonal amplitude of water storage variations of 27 large lakes (except Poyang and Dongting Lakes) is 14.10 ± 4.74 Gt and 15.42 ± 5.12 Gt, respectively. The relative deviations between them and the estimated water storage variations from the average area (15.85 ± 4.89 Gt) are 6 and 2%, respectively. The exceptional case could be Chengxi Lake due to the severe reclamation (De, 2016), and the relative deviation between the seasonal amplitude of water storage variations estimated by different water area fluctuation is the largest, which is 31.28 and 12.64%, respectively. In addition, the water level data applied in this study are collected from various data sources. We made strict quality control and inspection on the data source authority before processing and analyses.
On the other hand, the lake’s main function is to absorb excess floodwater during the flood season in the YHRB, but these floods usually bring in many sediments. Sedimentation may lead to the siltation of these lakes and ultimately reduce their storage capacity. Yang et al. (2015) pointed out that the dramatic decreases in sediment discharge from the Yangtze River are attributed to decreased precipitation and the construction of reservoirs (e.g., Three Gorges Dam). Poyang and Dongting Lakes are the only two lakes that are still naturally connected to the Yangtze River, which are the most important regulation and storage lakes in the YHRB. Therefore, the sediment deposition in these two lakes is probably more obvious compared to other lakes. Ye et al. (2019) indicated a total volume of 0.096 Gt/yr sediment in a net change of lake bottom topography during 2000–2011, which did not suffer radical changes for Poyang Lake. Previous studies have also shown that Poyang Lake bathymetry in most areas is relatively stable, and the storage estimation bias caused by deposition processes is significantly less than 1% except for the dry season (Li Y. et al., 2020; Yuan et al., 2021). Li et al. (2008) showed that the average annual deposition of Dongting Lake is about 0.10 Gt, and the overall sediment transport showed a decreasing trend. In terms of the seasonal variation of siltation, siltation mainly occurred in wet season, while the lake area was scoured in dry season.
Large lakes in the YHRB play a crucial part in the hydrologic cycle, freshwater provision, and flood prevention. However, comprehensive quantification of the seasonal amplitude of water storage change in the plain lake group in the YHRB remains unexplored. Thus, this study combined water area gained from Global Surface Water and water level data collected from satellite altimetry and in-situ observations of multiple sources, including Hydroweb, DAHITI, hydrological station, literature, and government statistics, to characterize the seasonal water level and storage variations of the plain lake group (29 lakes >100 km2) from 1990 to 2020, and estimates the seasonal amplitude of TWS in the YHRB with GRACE products.
This study quantified the seasonal amplitude of water storage variations of the plain lake group in the YHRB and analyzed their water storage potential in extreme flood years. The seasonal amplitude of water storage variations for the plain lake group in the YHRB is estimated using the empirical formula of water storage change by combining the lake area and water level changes. The results show that the average intra-annual water level variation of the plain lake group in the YHRB is 2.81 ± 0.69 m. The water level change of 12 lakes exceeded the annual average water level change, accounting for 41% of the total observed lakes. Poyang and Dongting Lakes with complex river-lake interactions are the two lakes with enormous seasonal water level changes, 9.53 ± 2.02 m and 7.39 ± 1.29 m, respectively. The annual cycle of water level change of Taihu Lake is the smallest (0.76 ± 0.42 m) due to artificial management. The plain lake group’s total water storage regulation capacity is about 37.38 ± 14.19 Gt. The seasonal amplitude of water storage variations in the twenty lakes in the MLRYRB (31.01 ± 13.07 Gt) is 4.87 times that of the nine lakes in the HRB (6.37 ± 1.12 Gt). The seasonal water storage changes of Poyang and Dongting Lakes are 14.25 ± 5.40 Gt and 7.31 ± 3.42 Gt, respectively, accounting for 57% of that of the YHRB. The two lakes still maintain the natural connection with the Yangtze River and play a crucial role in mitigating the flood during the flood period. The seasonal amplitude of water storage variation in large plain lakes contributes about 33.25% to the TWSA in the YHRB, indicating that these lakes play a crucial role in TWS in the YHRB.
The seasonal amplitude of water storage variations of large lakes is contributed to a critical reference for future flood control and water resources management in the YHRB and East China. Future research will focus on combining multi-source altimetry satellite missions to estimate water storage changes for more small lakes and further improve the accuracy of the research results.
The original contributions presented in the study are included in the article/supplementary material, further inquiries can be directed to the corresponding author.
LS and CS developed the methodology, collected and analyzed the data, led the writing of the manuscript. LS and PZ provided advice and background on GRACE products analysis. TC, KL, and HJ helped to organize the paper and edit the text. HJ provided substantive comments, corrections and valuable suggestions for improving the manuscript. All authors contributed to the article and approved the submitted version.
This work was supported by the National Key Research and Development Program of China (Grant No. 2018YFD1100101 and 2018YFD0900804), the Strategic Priority Research Program of the Chinese Academy of Sciences (Grant No. XDA23100102), National Natural Science Foundation of China (Grant Nos. 41971403 and 42101402), and Natural Science Foundation of Jiangsu Province (Grant No. BK20210990).
The authors declare that the research was conducted in the absence of any commercial or financial relationships that could be construed as a potential conflict of interest.
All claims expressed in this article are solely those of the authors and do not necessarily represent those of their affiliated organizations, or those of the publisher, the editors and the reviewers. Any product that may be evaluated in this article, or claim that may be made by its manufacturer, is not guaranteed or endorsed by the publisher.
We are grateful to the Laboratoire d'Etudes en Géophysique et Océanographie Spatiales (LEGOS) and the Hydrological Time Series of Inland Waters (DAHITI) for providing satellite altimetry data for this study. In addition, the authors express their gratitude to the Global Surface Water data (https://global-surface-water.appspot.com/) generated by Joint Research Centre (JRC). Finally, we are grateful to the scientific editor and the reviewers for their careful review of the manuscript used to improve the study.
An, L., Liao, K., Zhu, L., and Zhou, B. (2021). Influence of River-lake Isolation on the Water Level Variations of Caizi Lake, Lower Reach of the Yangtze River. J. Geogr. Sci. 31 (4), 551–564. doi:10.1007/s11442-021-1858-4
Blöschl, G., Kiss, A., Viglione, A., Barriendos, M., Böhm, O., Brázdil, R., et al. (2020). Current European Flood-Rich Period Exceptional Compared with Past 500 Years. Nature 583 (7817), 560–566. doi:10.1038/s41586-020-2478-3
Cai, X., Feng, L., Hou, X., and Chen, X. (2016). Remote Sensing of the Water Storage Dynamics of Large Lakes and Reservoirs in the Yangtze River Basin from 2000 to 2014. Sci. Rep. 6, 36405. doi:10.1038/srep36405
Cai, Y., Ke, C.-Q., and Shen, X. (2020). Variations in Water Level, Area and Volume of Hongze Lake, China from 2003 to 2018. J. Great Lakes Res. 46 (6), 1511–1520. doi:10.1016/j.jglr.2020.08.024
Chen, J., and Liao, J. (2020). Monitoring lake Level Changes in China Using Multi-Altimeter Data (2016-2019). J. Hydrol. 590, 125544. doi:10.1016/j.jhydrol.2020.125544
Chen, T., Song, C., Zhan, P., Yao, J., Li, Y., and Zhu, J. (2022). Remote Sensing Estimation of the Flood Storage Capacity of basin-scale Lakes and Reservoirs at High Spatial and Temporal Resolutions. Sci. Total Environ. 807 (Pt 1), 150772. doi:10.1016/j.scitotenv.2021.150772
Chen, X., Yang, X., Dong, X., and Liu, E. (2013). Environmental Changes in Chaohu Lake (Southeast, China) since the Mid 20th century: The Interactive Impacts of Nutrients, Hydrology and Climate. Limnologica 43 (1), 10–17. doi:10.1016/j.limno.2012.03.002
Chen, Y., Guan, Y., Miao, J., and Zhang, D. (2017). Determination of the Ecological Water-Level and Assuring Degree in the Lake Gaoyou, Northern Jiangsu with Long-Term Hydrological Alteration. J. Lake Sci. 29 (002), 398–408. (in Chinese). doi:10.18307/2017.0216
Cooley, S. W., Ryan, J. C., and Smith, L. C. (2021). Human Alteration of Global Surface Water Storage Variability. Nature 591 (7848), 78–81. doi:10.1038/s41586-021-03262-3
Crétaux, J.-F., Abarca-del-Río, R., Bergé-Nguyen, M., Arsen, A., Drolon, V., Clos, G., et al. (2016). Lake Volume Monitoring from Space. Surv. Geophys. 37 (2), 269–305. doi:10.1007/s10712-016-9362-6
Crétaux, J.-F., Arsen, A., Calmant, S., Kouraev, A., Vuglinski, V., Bergé-Nguyen, M., et al. (2011). SOLS: A lake Database to Monitor in the Near Real Time Water Level and Storage Variations from Remote Sensing Data. Adv. Space Res. 47 (9), 1497–1507. doi:10.1016/j.asr.2011.01.004
Dahle, C. (2018). GRACE 327-743 (Gravity Recovery and Climate Experiment): GFZ Level-2 Processing Standards Document for Level-2 Product Release 06 Rev. 1.0, October 26, 2018) .
De, Z. (2016). The Research of Flood Resources Utilization in ChengXihu Flood Storagemaster Thesis. Hefei University of Technology.
Deng, Y., Zhou, S., Yang, C., Xia, Z., and Liu, K. (2020). Analysis of Drought and Flood Change Based on Standard Precipitation Index(SPI) and its Impact on lake Water Level of Honghu lake. Hubei Agric. Sci. 59 (10), 32–37. (in Chinese). doi:10.14088/j.cnki.issn0439-8114.2020.10.007
Fan, C., Song, C., Liu, K., Ke, L., Xue, B., Chen, T., et al. (2021). Century‐Scale Reconstruction of Water Storage Changes of the Largest Lake in the Inner Mongolia Plateau Using a Machine Learning Approach. Water Res. 57 (2). doi:10.1029/2020wr028831
Fang, L., Wan, Z., and Wang, H. (2019). Assessment of Water Storage Change in China's Lakes and Reservoirs over the Last Three Decades. Remote Sensing 11 (12), 1467. doi:10.3390/rs11121467
Fu, Y. (2020). Community Composition and Distribution Pattern of Aquatic Plants in Changdanghu Lake. Wetland Sci. Manage. 16 (04), 27–31. (in Chinese). doi:10.3969/j.issn.1673-3290.2020.04.07
Han, Q., Zhang, S., Huang, G., and Zhang, R. (2016). Analysis of Long-Term Water Level Variation in Dongting Lake, China. Water 8 (7), 306. doi:10.3390/w8070306
Hu, Q.-f., and Wang, Y.-t. (2009). Impact Assessment of Climate Change and Human Activities on Annual Highest Water Level of Taihu Lake. Water Sci. Eng. 2 (1), 1–15. doi:10.3882/j.issn.1674-2370.2009.01.001
Hu, Y., Huang, J., Du, Y., Han, P., and Huang, W. (2015). Monitoring Spatial and Temporal Dynamics of Flood Regimes and Their Relation to Wetland Landscape Patterns in Dongting Lake from MODIS Time-Series Imagery. Remote Sensing 7 (6), 7494–7520. doi:10.3390/rs70607494
Huang, S., Li, J., and Xu, M. (2011). Water Surface Variations Monitoring and Flood hazard Analysis in Dongting Lake Area Using Long-Term Terra/MODIS Data Time Series. Nat. Hazards 62 (1), 93–100. doi:10.1007/s11069-011-9921-6
Ji, H., Zhang, X., Wang, S., and Li, J. (2021). Study on Water Quantity Guarantee and Water Quality Safety of Changzhou reserve Water Source—Take Ge Lake for Example. Resour. Economization Environ. Prot. (04), 13–15. (in Chinese). doi:10.16317/j.cnki.12-1377/x.2021.04.009
Jiang, L., Nielsen, K., Andersen, O. B., and Bauer-Gottwein, P. (2017). CryoSat-2 Radar Altimetry for Monitoring Freshwater Resources of China. Remote Sensing Environ. 200, 125–139. doi:10.1016/j.rse.2017.08.015
Kameyama, S. (2004). An Approach to Estimate the Water Ievel and Volume of Dongting Lake Using Terra/MODIS Data. Acta Geographica Sinica (01), 88–94. (in Chinese).
Klein, I., Mayr, S., Gessner, U., Hirner, A., and Kuenzer, C. (2021). Water and Hydropower Reservoirs: High Temporal Resolution Time Series Derived from MODIS Data to Characterize Seasonality and Variability. Remote Sensing Environ. 253, 112207. doi:10.1016/j.rse.2020.112207
Kornfeld, R. P., Arnold, B. W., Gross, M. A., Dahya, N. T., Klipstein, W. M., Gath, P. F., et al. (2019). GRACE-FO: The Gravity Recovery and Climate Experiment Follow-On Mission. J. Spacecraft Rockets 56 (3), 931–951. doi:10.2514/1.A34326
Li, C., Yang, Y., Wang, Z., Yang, L., Zhang, D., and Zhou, L. (2018). The Relationship between Seasonal Water Level Fluctuation and Habitat Availability for Wintering Waterbirds at Shengjin Lake, China. Bird Conservation Int. 29 (1), 100–114. doi:10.1017/s0959270918000035
Li, C., Zhang, C., Ke, B., and Zhu, J. (2015a). The Level Variation Monitoring of Chaohu Lake by Cryosat-2 Satellite Altimetry Data. Sci. Surv. Mapp. 40 (12), 12–15. (in Chinese). doi:10.16251/j.cnki.1009-2307.2015.12.003
Li, J., Wang, C., Xu, L., Wu, F., Zhang, H., and Zhang, B. (2021). Multitemporal Water Extraction of Dongting Lake and Poyang Lake Based on an Automatic Water Extraction and Dynamic Monitoring Framework. Remote Sensing 13 (5), 865. doi:10.3390/rs13050865
Li, J., Yin, H., Lu, C., Mao, D., and Zhou, H. (2008). Effects of Sediment Deposition in Dongting Lake Area. Acta Geographica Sinica (05), 487–496. (in Chinese).
Li, L., Xia, H., Li, Z., and Zhang, Z. (2015b). Temporal-Spatial Evolution Analysis of Lake Size-Distribution in the Middle and Lower Yangtze River Basin Using Landsat Imagery Data. Remote Sensing 7 (8), 10364–10384. doi:10.3390/rs70810364
Li, P., Li, H., Chen, F., and Cai, X. (2020a). Monitoring Long-Term Lake Level Variations in Middle and Lower Yangtze Basin over 2002-2017 through Integration of Multiple Satellite Altimetry Datasets. Remote Sensing 12 (9), 1448. doi:10.3390/rs12091448
Li, Y., Zhang, Q., Tan, Z., and Yao, J. (2020b). On the Hydrodynamic Behavior of Floodplain Vegetation in a Flood-Pulse-Influenced River-lake System (Poyang Lake, China). J. Hydrol. 585, 124852. doi:10.1016/j.jhydrol.2020.124852
Li, Y., Zhang, Q., Yao, J., Tan, Z., and Liu, X. (2019). Assessment of Water Storage Response to Surface Hydrological Connectivity in a Large Floodplain System (Poyang Lake, China) Using Hydrodynamic and Geostatistical Analysis. Stoch Environ. Res. Risk Assess. 33 (11-12), 2071–2088. doi:10.1007/s00477-019-01740-9
Lin, Y., Zhang, T., Ye, Q., Cai, J., Wu, C., Khirni Syed, A., et al. (2021). Long-term Remote Sensing Monitoring on LUCC Around Chaohu Lake with New Information of Algal Bloom and Flood Submerging. Int. J. Appl. Earth Observation Geoinformation 102, 102413. doi:10.1016/j.jag.2021.102413
Liu, H.-B. (2018). Dominant Variation Modes Associated with Yangtze-Huai River Basin Summer Heavy Rainfall Events. Atmos. Oceanic Sci. Lett. 11 (6), 464–471. doi:10.1080/16742834.2018.1526620
Liu, H., Zheng, L., Jiang, L., and Liao, M. (2020a). Forty-year Water Body Changes in Poyang Lake and the Ecological Impacts Based on Landsat and HJ-1 A/B Observations. J. Hydrol. 589, 125161. doi:10.1016/j.jhydrol.2020.125161
Liu, N., and Li, S. (2014). Predicting Summer Rainfall over the Yangtze-Huai Region Based on Time-Scale Decomposition Statistical Downscaling. Weather Forecast. 29 (1), 162–176. doi:10.1175/waf-d-13-00045.1
Liu, Y., Yang, S.-Q., Jiang, C., Long, Y., Deng, B., and Yan, S. (2020b). Hydrological Drought in Dongting Lake Area (China) after the Running of Three Gorges Dam and a Possible Solution. Water 12 (10), 2713. doi:10.3390/w12102713
Liu, Z., Yao, Z., and Wang, R. (2019). Evaluation and Validation of CryoSat-2-Derived Water Levels Using In Situ Lake Data from China. Remote Sensing 11 (8), 899. doi:10.3390/rs11080899
Long, D., Longuevergne, L., and Scanlon, B. R. (2015). Global Analysis of Approaches for Deriving Total Water Storage Changes from GRACE Satellites. Water Resour. Res. 51 (4), 2574–2594. doi:10.1002/2014wr016853
Long, Y., Tang, R., Wu, C., Jiang, C., and Hu, S. (2019). Estimating Real-Time Water Area of Dongting Lake Using Water Level Information. Water 11 (6), 1240. doi:10.3390/w11061240
Luo, S., Song, C., Liu, K., Ke, L., and Ma, R. (2019). An Effective Low-Cost Remote Sensing Approach to Reconstruct the Long-Term and Dense Time Series of Area and Storage Variations for Large Lakes. Sensors 19 (19), 4247. doi:10.3390/s19194247
Luo, S., Song, C., Zhan, P., Liu, K., Chen, T., Li, W., et al. (2021). Refined Estimation of lake Water Level and Storage Changes on the Tibetan Plateau from ICESat/ICESat-2. Catena 200, 105177. doi:10.1016/j.catena.2021.105177
Marsooli, R., Lin, N., Emanuel, K., and Feng, K. (2019). Climate Change Exacerbates hurricane Flood Hazards along US Atlantic and Gulf Coasts in Spatially Varying Patterns. Nat. Commun. 10 (1), 3785. doi:10.1038/s41467-019-11755-z
Mei, H., Wang, Z., Liu, M., and Zhou, J. (2021). Characteristic Water Levels of Hongze Lake in the Past Five Decades: Variation Rules and Influencing Factors. J. Yangtze River Scientific Res. Inst. 38 (01), 35–40. (in Chinese). doi:10.11988/ckyyb.20191272
Mei, X., Dai, Z., Du, J., and Chen, J. (2015). Linkage between Three Gorges Dam Impacts and the Dramatic Recessions in China's Largest Freshwater lake, Poyang Lake. Sci. Rep. 5, 18197. doi:10.1038/srep18197
Messager, M. L., Lehner, B., Grill, G., Nedeva, I., and Schmitt, O. (2016). Estimating the Volume and Age of Water Stored in Global Lakes Using a Geo-Statistical Approach. Nat. Commun. 7 (1), 13603. doi:10.1038/ncomms13603
Mu, S., Li, B., Yao, J., Yang, G., Wan, R., and Xu, X. (2020). Monitoring the Spatio-Temporal Dynamics of the Wetland Vegetation in Poyang Lake by Landsat and MODIS Observations. Sci. Total Environ. 725, 138096. doi:10.1016/j.scitotenv.2020.138096
Ni, P. (2018). Research on Water Environment Capacity and the Lowest Ecological Water Level, Changhu Lake. Master Thesis. Wuhan University.
Pekel, J.-F., Cottam, A., Gorelick, N., and Belward, A. S. (2016). High-resolution Mapping of Global Surface Water and its Long-Term Changes. Nature 540 (7633), 418–422. doi:10.1038/nature20584
Ramillien, G., Frappart, F., Güntner, A., Ngo-Duc, T., Cazenave, A., and Laval, K. (2006). Time Variations of the Regional Evapotranspiration Rate from Gravity Recovery and Climate Experiment (GRACE) Satellite Gravimetry. Water Resour. Res. 42 (10). doi:10.1029/2005wr004331
Schwatke, C., Dettmering, D., Bosch, W., and Seitz, F. (2015). DAHITI - an Innovative Approach for Estimating Water Level Time Series over Inland Waters Using Multi-mission Satellite Altimetry. Hydrol. Earth Syst. Sci. 19 (10), 4345–4364. doi:10.5194/hess-19-4345-2015
Schwatke, C., Dettmering, D., and Seitz, F. (2020). Volume Variations of Small Inland Water Bodies from a Combination of Satellite Altimetry and Optical Imagery. Remote Sensing 12 (10), 1606. doi:10.3390/rs12101606
Song, C., Ke, L., Huang, B., and Richards, K. S. (2015). Can Mountain Glacier Melting Explains the GRACE-observed Mass Loss in the Southeast Tibetan Plateau: From a Climate Perspective. Glob. Planet. Change 124, 1–9. doi:10.1016/j.gloplacha.2014.11.001
Song, C., and Ke, L. (2014). Recent Dramatic Variations of China's Two Largest Freshwater Lakes: Natural Process or Influenced by the Three Gorges Dam. Environ. Sci. Technol. 48 (3), 2086–2087. doi:10.1021/es405500s
Song, L., Song, C., Luo, S., Chen, T., Liu, K., Li, Y., et al. (2021). Refining and Densifying the Water Inundation Area and Storage Estimates of Poyang Lake by Integrating Sentinel-1/2 and Bathymetry Data. Int. J. Appl. Earth Observation Geoinformation 105, 102601. doi:10.1016/j.jag.2021.102601
Song, Z., Xia, J., She, D., Zhang, L., Hu, C., and Zhao, L. (2020). The Development of a Nonstationary Standardized Precipitation Index Using Climate Covariates: A Case Study in the Middle and Lower Reaches of Yangtze River Basin, China. J. Hydrol. 588, 125115. doi:10.1016/j.jhydrol.2020.125115
Su, Y., Guo, B., Zhou, Z., Zhong, Y., and Min, L. (2020). Spatio-Temporal Variations in Groundwater Revealed by GRACE and its Driving Factors in the Huang-Huai-Hai Plain, China. Sensors 20 (3), 922. doi:10.3390/s20030922
Sun, A. Y., Scanlon, B. R., Save, H., and Rateb, A. (2021). Reconstruction of GRACE Total Water Storage through Automated Machine Learning. Water Res. 57 (2). doi:10.1029/2020wr028666
Sun, F., Zhao, Y., Gong, P., Ma, R., and Dai, Y. (2014). Monitoring Dynamic Changes of Global Land Cover Types: Fluctuations of Major Lakes in China Every 8 Days during 2000-2010. Chin. Sci. Bull. 59 (2), 171–189. doi:10.1007/s11434-013-0045-0
Tan, W., Xing, J., Yang, S., Yu, G., Sun, P., and Jiang, Y. (2020). Long Term Aquatic Vegetation Dynamics in Longgan Lake Using Landsat Time Series and Their Responses to Water Level Fluctuation. Water 12 (8), 2178. doi:10.3390/w12082178
Tapley, B. D., Bettadpur, S., Watkins, M., and Reigber, C. (2004). The Gravity Recovery and Climate experiment: Mission Overview and Early Results. Geophys. Res. Lett. 31 (9), n/a. doi:10.1029/2004gl019920
Tellman, B., Sullivan, J. A., Kuhn, C., Kettner, A. J., Doyle, C. S., Brakenridge, G. R., et al. (2021). Satellite Imaging Reveals Increased Proportion of Population Exposed to Floods. Nature 596 (7870), 80–86. doi:10.1038/s41586-021-03695-w
UN Office for Disaster Risk Reduction. (2020). UN Office for Disaster Risk Reduction. E. coli. Available at: https://reliefweb.int/report/world/human-cost-disasters-overview-last-20-years-2000-2019. [Accessed March 15, 2020].
Verpoorter, C., Kutser, T., Seekell, D. A., and Tranvik, L. J. (2014). A Global Inventory of Lakes Based on High-Resolution Satellite Imagery. Geophys. Res. Lett. 41 (18), 6396–6402. doi:10.1002/2014GL060641
Wang, C., Jiang, W., Deng, Y., Ling, Z., and Deng, Y. (2022a). Long Time Series Water Extent Analysis for SDG 6.6.1 Based on the GEE Platform: A Case Study of Dongting Lake. IEEE J. Sel. Top. Appl. Earth Observations Remote Sensing 15, 490–503. doi:10.1109/JSTARS.2021.3088127
Wang, H. L., Zhang, X. K., Jin, B. S., Wang, M. D., Chen, W. X., and Liu, D. (2020). Water Level Fluctuations Determine the Spatial and Temporal Distribution of Manchurian Wild Rice (Zizania Latifolia) in Six Yangtze River Floodplain Lakes, China. Appl. Ecol. Env. Res. 18 (4), 5491–5503. doi:10.15666/aeer/1804_54915503
Wang, H., Sun, J., Fu, S., and Zhang, Y. (2021b). Typical Circulation Patterns and Associated Mechanisms for Persistent Heavy Rainfall Events over Yangtze-Huaihe River Valley during 1981-2020. Adv. Atmos. Sci. 38 (12), 2167–2182. doi:10.1007/s00376-021-1194-8
Wang, J., Sheng, Y., and Tong, T. S. D. (2014). Monitoring Decadal lake Dynamics across the Yangtze Basin Downstream of Three Gorges Dam. Remote Sensing Environ. 152, 251–269. doi:10.1016/j.rse.2014.06.004
Wang, J., Song, C., Reager, J. T., Yao, F., Famiglietti, J. S., Sheng, Y., et al. (2018). Recent Global Decline in Endorheic basin Water Storages. Nat. Geosci 11, 926–932. doi:10.1038/s41561-018-0265-7
Wang, J., Xu, Y., Wang, Y., Yuan, J., Wang, Q., and Xiang, J. (2019a). Non-stationarity Analysis of Extreme Water Level in Response to Climate Change and Urbanization in the Taihu Basin, China. Stoch Environ. Res. Risk Assess. 33 (3), 891–904. doi:10.1007/s00477-019-01666-2
Wang, Y., Ma, J., Xiao, X., Wang, X., Dai, S., and Zhao, B. (2019b). Long-Term Dynamic of Poyang Lake Surface Water: A Mapping Work Based on the Google Earth Engine Cloud Platform. Remote Sensing 11 (3), 313. doi:10.3390/rs11030313
Wang, Y., Tabari, H., Xu, Y., Xu, Y., and Wang, Q. (2019c). Unraveling the Role of Human Activities and Climate Variability in Water Level Changes in the Taihu Plain Using Artificial Neural Network. Water 11 (4), 720. doi:10.3390/w11040720
Wang, Y., Zhang, Q., and Singh, V. P. (2016). Spatiotemporal Patterns of Precipitation Regimes in the Huai River basin, China, and Possible Relations with ENSO Events. Nat. Hazards 82 (3), 2167–2185. doi:10.1007/s11069-016-2303-3
Xia, H., Zhao, J., Qin, Y., Yang, J., Cui, Y., Song, H., et al. (2019). Changes in Water Surface Area during 1989-2017 in the Huai River Basin Using Landsat Data and Google Earth Engine. Remote Sensing 11 (15), 1824. doi:10.3390/rs11151824
Xie, Z., Du, Y., Zeng, Y., and Miao, Q. (2018). Classification of Yearly Extreme Precipitation Events and Associated Flood Risk in the Yangtze-Huaihe River Valley. Sci. China Earth Sci. 61 (9), 1341–1356. doi:10.1007/s11430-017-9212-8
Xing, L., Tang, X., Wang, H., Fan, W., and Wang, G. (2018). Monitoring Monthly Surface Water Dynamics of Dongting Lake Using Sentinal-1 Data at 10 M. PeerJ 6, e4992. doi:10.7717/peerj.4992
Xu, X., Huang, X., Zhang, Y., and Yu, D. (2018). Long-Term Changes in Water Clarity in Lake Liangzi Determined by Remote Sensing. Remote Sensing 10 (9), 1441. doi:10.3390/rs10091441
Xu, Y., Li, J., Wang, J., Chen, J., Liu, Y., Ni, S., et al. (2020a). Assessing Water Storage Changes of Lake Poyang from Multi-mission Satellite Data and Hydrological Models. J. Hydrol. 590, 125229. doi:10.1016/j.jhydrol.2020.125229
Xu, Y., Xu, Y., and Wang, Q. (2020b). Evolution Trends in Water Levels and Their Causes in the Taihu Basin, China. Hydrological Sci. J. 65 (13), 2296–2308. doi:10.1080/02626667.2020.1802026
Yang, P., Xia, J., Luo, X., Meng, L., Zhang, S., Cai, W., et al. (2021). Impacts of Climate Change-Related Flood Events in the Yangtze River Basin Based on Multi-Source Data. Atmos. Res. 263, 105819. doi:10.1016/j.atmosres.2021.105819
Yang, S. L., Xu, K. H., Milliman, J. D., Yang, H. F., and Wu, C. S. (2015). Decline of Yangtze River Water and Sediment Discharge: Impact from Natural and Anthropogenic Changes. Sci. Rep. 5, 12581. doi:10.1038/srep12581
Yao, J., Zhang, Q., Ye, X., Zhang, D., and Bai, P. (2018). Quantifying the Impact of Bathymetric Changes on the Hydrological Regimes in a Large Floodplain lake: Poyang Lake. J. Hydrol. 561, 711–723. doi:10.1016/j.jhydrol.2018.04.035
Yao, X., Ma, J., Zhang, D.-L., and Yan, L. (2020). A 33-yr Mei-Yu-Season Climatology of Shear Lines over the Yangtze-Huai River Basin in Eastern China. J. Appl. Meteorology Climatology 59 (6), 1125–1137. doi:10.1175/jamc-d-19-0229.1
Ye, Q., and Glantz, M. H. (2005). The 1998 Yangtze Floods: The Use of Short-Term Forecasts in the Context of Seasonal to Interannual Water Resource Management. Mitig Adapt Strat Glob. Change 10 (1), 159–182. doi:10.1007/s11027-005-7838-7
Ye, X., Guo, Q., Zhang, Z., and Xu, C. (2019). Assessing Hydrological and Sedimentation Effects from Bottom Topography Change in a Complex River-Lake System of Poyang Lake, China. Water 11 (7), 1489. doi:10.3390/w11071489
Ye, X., Xu, C.-Y., Li, Y., Li, X., and Zhang, Q. (2017). Change of Annual Extreme Water Levels and Correlation with River Discharges in the Middle-Lower Yangtze River: Characteristics and Possible Affecting Factors. Chin. Geogr. Sci. 27 (2), 325–336. doi:10.1007/s11769-017-0866-x
Yi, S., Wang, Q., and Sun, W. (2016). Basin Mass Dynamic Changes in China from GRACE Based on a Multibasin Inversion Method. J. Geophys. Res. Solid Earth 121 (5), 3782–3803. doi:10.1002/2015JB012608
Yin, W., Han, S.-C., Zheng, W., Yeo, I.-Y., Hu, L., Tangdamrongsub, N., et al. (2020). Improved Water Storage Estimates within the North China Plain by Assimilating GRACE Data into the CABLE Model. J. Hydrol. 590, 125348. doi:10.1016/j.jhydrol.2020.125348
Yin, Y., Chen, Y., Yu, S., Xu, W., Wang, W., and Xu, Y. (2013). Maximum Water Level of Hongze Lake and its Relationship with Natural Changes and Human Activities from 1736 to 2005. Quat. Int. 304, 85–94. doi:10.1016/j.quaint.2012.12.042
Yuan, S., Tang, H., Li, K., Xu, L., Xiao, Y., Gualtieri, C., et al. (2021). Hydrodynamics, Sediment Transport and Morphological Features at the Confluence between the Yangtze River and the Poyang Lake. Water Res. 57 (3). doi:10.1029/2020wr028284
Zeng, K., Wang, J., Zhang, Y., Zhang, L., and Yang, Q. (2020). Influence of Water Level Fluctuation on Water Quality of Huayang River and lake Group. J. Yangtze River Scientific Res. Inst. 37 (08), 49–53+68. (in Chinese). doi:10.11988/ckyyb.20190419
Zeng, L., Schmitt, M., Li, L., and Zhu, X. X. (2017). Analysing Changes of the Poyang Lake Water Area Using Sentinel-1 Synthetic Aperture Radar Imagery. Int. J. Remote Sensing 38 (23), 7041–7069. doi:10.1080/01431161.2017.1370151
Zhan, P., Song, C., Wang, J., Li, W., Ke, L., Liu, K., et al. (2020). Recent Abnormal Hydrologic Behavior of Tibetan Lakes Observed by Multi-mission Altimeters. Remote Sensing 12 (18), 2986. doi:10.3390/RS12182986
Zhang, C., Ji, C., Wang, Y., and Xiao, Q. (2021a). Flood Hydrograph Coincidence Analysis of the Upper Yangtze River and Dongting Lake, China. Nat. Hazards. doi:10.1007/s11069-021-04993-2
Zhang, G., Chen, W., and Xie, H. (2019). Tibetan Plateau's Lake Level and Volume Changes from NASA's ICESat/ICESat‐2 and Landsat Missions. Geophys. Res. Lett. 46 (22), 13107–13118. doi:10.1029/2019gl085032
Zhang, Q., Ye, X.-c., Werner, A. D., Li, Y.-l., Yao, J., Li, X.-h., et al. (2014). An Investigation of Enhanced Recessions in Poyang Lake: Comparison of Yangtze River and Local Catchment Impacts. J. Hydrol. 517, 425–434. doi:10.1016/j.jhydrol.2014.05.051
Zhang, X., Kurbaniyazov, A., and Kirillin, G. (2021b). Changing Pattern of Water Level Trends in Eurasian Endorheic Lakes as a Response to the Recent Climate Variability. Remote Sensing 13 (18), 3705. doi:10.3390/rs13183705
Zhao, D., Jiang, H., Cai, Y., and An, S. (2012). Artificial Regulation of Water Level and its Effect on Aquatic Macrophyte Distribution in Taihu Lake. PLoS One 7 (9), e44836. doi:10.1371/journal.pone.0044836
Zhu, J., Song, C., Wang, J., and Ke, L. (2020). China's Inland Water Dynamics: The Significance of Water Body Types. Proc. Natl. Acad. Sci. USA 117 (25), 13876–13878. doi:10.1073/pnas.2005584117
Keywords: lakes, seasonality, water level, water storage, flood regulation, Yangtze-Huai River Basin
Citation: Song L, Song C, Zhan P, Chen T, Liu K and Jing H (2022) Seasonal Amplitude of Water Storage Variations of the Yangtze–Huai Plain Lake Group: Implicaion for Floodwater Storage Capacity. Front. Environ. Sci. 10:838883. doi: 10.3389/fenvs.2022.838883
Received: 18 December 2021; Accepted: 12 January 2022;
Published: 26 January 2022.
Edited by:
Nagesh Kumar D, Indian Institute of Science (IISc), IndiaReviewed by:
Priyank J. Sharma, Indian Institute of Technology Indore, IndiaCopyright © 2022 Song, Song, Zhan, Chen, Liu and Jing. This is an open-access article distributed under the terms of the Creative Commons Attribution License (CC BY). The use, distribution or reproduction in other forums is permitted, provided the original author(s) and the copyright owner(s) are credited and that the original publication in this journal is cited, in accordance with accepted academic practice. No use, distribution or reproduction is permitted which does not comply with these terms.
*Correspondence: Chunqiao Song, Y3Fzb25nQG5pZ2xhcy5hYy5jbg==
Disclaimer: All claims expressed in this article are solely those of the authors and do not necessarily represent those of their affiliated organizations, or those of the publisher, the editors and the reviewers. Any product that may be evaluated in this article or claim that may be made by its manufacturer is not guaranteed or endorsed by the publisher.
Research integrity at Frontiers
Learn more about the work of our research integrity team to safeguard the quality of each article we publish.