- 1Shaanxi Key Laboratory of Land Consolidation, School of Land Engineering, Chang’an University, Xi’an, China
- 2School of Earth Science and Resources, Chang’an University, Xi’an, China
- 3China Center for Resources Satellite Data, Beijing, China
- 4Qinba Ecological Protection Center, Hanzhong, China
Based on the maximum entropy modeling (Maxent) and ArcGIS tool, this study assessed the potential of marginal land and analyzed the impact of environmental variables for Jerusalem artichoke (Helianthus tuberosus L.) in Shaanxi Province, China. The results showed that the dominant land type used for the growth of Jerusalem artichoke was moderately dense grassland. Additionally, significant environmental variables of Jerusalem artichoke and their suitable range in Shaanxi Province were average slope (SLP, 0–5°C), average soil depth (DPT, 1.50–1.60 m), max temperature of the warmest month (Bio5, 30–31°C), annual mean temperature (Bio1, 16.5–18.0°C), precipitation of the wettest quarter (Bio16, 0.01–0.02 m), July solar radiation (SR7, 1.66–1.67 × 107 W/m2), precipitation seasonality (Bio15, 50–60%), precipitation of the driest quarter (Bio17, 0–0.005 m), and isothermality (Bio3, 265–275). Furthermore, the suitable area was mainly distributed in southern (mainly Hanzhong, Ankang, and Shangluo) and northern (mainly Yan’an and Yulin) parts of Shaanxi Province, covering around 8.81 × 1010 m2 and accounting for 42.8% of the total area of the Shaanxi Province. This study can provide a reference for the rational planting of Jerusalem artichoke in Shaanxi Province.
1 Introduction
Climate change has garnered huge attention worldwide, and rising greenhouse gas emissions are the primary drivers of climate change (such as carbon dioxide and methane) (Jhariya et al., 2019; Jhariya et al., 2021a; Jhariya et al., 2021c). The massive usage of fossil fuels has led to a rapid increase in greenhouse gas emissions (Foster, 2019; Jhariya et al., 2019). The United Nations Framework Convention on Climate Change (UNFCCC) was conducted to control carbon dioxide and other greenhouse gas emissions (Jhariya et al., 2019). Replacing or reducing the usage of fossil fuels with renewable energy is an effective way to reduce carbon emission (Maddalwar et al., 2021; Zhang B. et al., 2021). As one of the largest consumers of fossil energy and carbon emitter globally (Mi et al., 2017; Shi et al., 2022), the Chinese government actively responded to international carbon neutral actions (Nie et al., 2022), and it urgently needs to explore economical and environmentally friendly renewable energy sources to achieve carbon emission reduction targets.
Bioenergy, as one of the renewable energy sources (RES), is affianced with renewability, cleanliness (Li X. et al., 2020), and ecological friendliness (Paul et al., 2020) and has proven to be the most promising renewable resource in the future (Bauer et al., 2020; Cao et al., 2022). On the one hand, China has a vast potential for bioenergy production (Zhao and Liu, 2014). On the other hand, energy plant growth necessitates substantial land resources (Xue et al., 2020), putting land for energy plants in direct or indirect competition with land for food crops (Leppäkoski et al., 2021; Jhariya et al., 2021a). One feasible approach is growing energy plants on marginal lands (Jiang et al., 2018; Mehmood et al., 2019), which not only helps to alleviate the energy crisis (Liu et al., 2015) but also reduces soil erosion and improves marginal soil quality without interfering with food production (Bogucka and Jankowski, 2020; Qaseem and Wu, 2020). Besides, the marginal land resources have huge development potential in China. Xu et al. (2013) estimated that suitable marginal land for planting energy plants was approximately 17.6 × 1011 m2, accounting for 1.82% of the total area of China.
Ideal energy crops for marginal lands were occupied with a long growing season, well-developed canopy, and fewer reproductive structures (Jones et al., 2015). After 2007, the grains and green grain crops as raw materials for biomass energy were banned in China (Xue et al., 2016; Qaseem and Wu, 2021), so the non-food biomass feedstock is the first choice of bioenergy production in China (Qaseem and Wu, 2021). FAO experts have dubbed Jerusalem artichoke (Helianthus tuberosus L.) a “21st-century human livestock crop” because of its high tolerance to environmental challenges such as soil salinity, drought, and plant diseases (Long et al., 2016; Krivorotova and Sereikaite, 2018), making it ideal for marginal lands. Moreover, with rapid growth and low production cost (such as fewer pesticides input, fertilizers, and water) (Bogucka et al., 2021), as well as high biomass (Bogucka and Jankowski, 2020), Jerusalem artichoke is regarded as one of the most promising bioenergy raw material sources (Epie et al., 2018).
Assessing the distribution of energy plants and habitat suitability is a prerequisite for their rational planting and development. Many models are being used to predict the species geographic distributions, such as the generalized linear model (GLM), boosted regression trees (BRT), support vector machine (SVM), multifactor-integrated assessment method (Fukuda et al., 2013; Yin et al., 2018; Jiang et al., 2019; Ghareghan et al., 2020), and maximum entropy modeling (Maxent). In comparison, Maxent is a widely recognized model used in geographical distribution of species (Phillips et al., 2006; Li J. et al., 2020) due to its good performance, especially in cases where species’ distribution information is incomplete (Li J. et al., 2020; Zhang J.-M. et al., 2021). Most of the existing literature used the Maxent model to predict the potential distribution of energy plants (Wen et al., 2016; Li et al., 2021; Cao et al., 2022). But these studies lack further analysis of species suitability on the marginal land.
Shaanxi Province is one of the target areas for the development of bioenergy (Council, 2018). According to the investigation, Jerusalem artichoke has been widely planted in Yan’an and Shangluo. However, large-scale rational planting that is essential for the development of bioenergy has not been realized, and the marginal land resources suitable for Jerusalem artichoke planting are ambiguous. This study adopts the Maxent model and ArcGIS tools to assess the potential of marginal land suitable for Jerusalem artichoke and to analyze the impact of environmental variables for Jerusalem artichoke in Shaanxi Province. The main objectives of this study are as follows: 1) to determine the distribution of Jerusalem artichoke in Shaanxi Province; 2) to identify the most critical environmental variables for Jerusalem artichoke and determine their effects on the spread of Jerusalem artichoke; and 3) to access the potential marginal land resources suitable for planting Jerusalem artichoke in Shaanxi Province, and the habitat suitability of Jerusalem artichoke. For the first time, the Maxent model is used with ArcGIS tools to assess Jerusalem artichoke’s marginal land resource potential in Shaanxi Province. It can provide a reference for the rational planting of Jerusalem artichoke and can contribute to relevant policy-making in Shaanxi Province.
2 Materials and Methods
2.1 Study Area
Shaanxi Province is located in the northwestern part of China (105°29′E-111°15′E and 31°42′N-39°35′N). The area is 2.06 × 1011 m2, where the Loess Plateau accounts for 40% of the whole land area of Shaanxi Province. The topography is low in the middle and high in the south and north. The northern part is the Loess Plateau, most of which belongs to the warm temperate monsoon climate, and the northern part of Shaanxi along the Great Wall belongs to the middle temperate monsoon climate; the precipitation varies from 0.40 to 0.60 m. The central part is the Guanzhong Plain, which belongs to the warm temperate monsoon climate, and the rainfall ranges from 0.50 to 0.70 m. The southern part is the Qinling Mountains, which belong to the north subtropical monsoon climate, and the precipitation varies from 0.70 to 0.90 m. Shaanxi Province spans from subtropical, warm temperate, and temperate zones from south to north, with significant climate differences.
2.2 Marginal Land
The marginal land is highly variable due to its various definitions (Richards et al., 2014; Jiang et al., 2018). Generally, the land unsuitable for food production, ambiguous lower quality land, and economically marginal land (Shortall, 2013) are considered marginal land. According to the method of Yin et al. (2018), this study selected seven types of land (including shrubland (SHL), sparse forest land (SFL), dense grassland (DG), moderately dense grassland (MDG), sparsely dense grassland (SDG), saline land (SAL), and bare land (BL)) as marginal land based on the land use data in 2018 (Xu et al., 2018). Eventually, the total area of marginal land in Shaanxi Province is 1.07 × 1011 m2, accounting for 51.9% of the total area of Shaanxi Province.
2.3 Datasets
2.3.1 Occurrence Data
In this study, the occurrence data were mainly obtained by retrieving the specimen database, consulting the data and field investigation. Specimen databases include the public repository of the Global Biodiversity Information Facility (https://www.gbif.org/) and the platform of teaching specimen standardization and resource sharing (http://mnh.scu.edu.cn/). A Garmin global positioning system receiver (accuracy 5 m) was used for detecting positions in the field survey. Google Earth software was used to fill in the missing geographic coordinates in the acquired data. A total of 28 Jerusalem artichoke sites were selected. The Krasovsky 1940 Albers projected coordinate system was used, and the data were stored in the csv format.
2.3.2 Environmental Variables
Natural conditions (such as soil quality, precipitation, topography, accumulated temperature, and solar radiation) significantly impact the growth of Jerusalem artichoke (Zorić et al., 2016). Therefore, 35 environmental variables affecting Jerusalem artichoke were chosen, including climate (19 variables), solar radiation (12 variables), soil conditions (2 variables), and terrain (2 variables) (Supplementary Appendix A).
The following is an overview of the data and its source.
Meteorology: The CHELSA version 1.2 (http://chelsa-climate.org/) climate dataset for earth land surface areas was adopted in this study, which is licensed under a Creative Commons Attribution 4.0 International License. It has high resolution (30 arc-second resolution). It included monthly and annual mean temperature and precipitation patterns incorporated with topological climate (such as orographic rainfall and wind fields) from 1979 to 2013.
Topography: The ASTER Digital Elevation Model (DEM) version 3 and slope version 3 datasets with 30 m resolution were obtained from the National Earth System Science Data Center, National Science and Technology Infrastructure of China (http://www.geodata.cn).
Solar radiation: The monthly Global-Extra Terrestrial Solar Radiation data (12 months, 30 arc-second resolution) was derived from the Consortium for Spatial Information (https://cgiarcsi.community/). The unit of the extraterrestrial solar radiation is W/m2.
Soil: The National Secondary Soil Survey dataset was used in the analysis. Soil depth and organic matter content were used in the study, and an ordinary kriging interpolation algorithm obtained China’s spatial continuous soil data.
Land use: The dataset was collected from the Data Center for Resources and Environmental Sciences, Chinese Academy of Sciences (RESDC) (http://www.resdc.cn).
After unifying the projected coordinate system (Krasovsky 1940 Albers), all environmental layers were trimmed and resampled to the exact geographic boundaries with a pixel size (about 100 m × 100 m) and recorded in an ASCII grid (.asc) format.
2.4 Modeling
The Maxent model, based on statistics and machine learning, is a popular software for predicting species distribution (Phillips et al., 2006), and it is available for free (https://biodiversityinformatics.amnh.org/open_source/maxent/). A model was built to forecast species distribution based on environmental layers for a group of grid cells and a collection of observed species sample locations (Phillips et al., 2004). The model’s appropriateness was represented by the function of its environment variables in each grid cell. The higher the value of the function of a particular grid cell, the more suitable the conditions are for the species. The model was a probability distribution of all grid cells, and the selected distribution is the one with maximum entropy (Merow et al., 2013).
The percent contribution and the jackknife test are common indicators to evaluate the importance of environmental predictors in the model (Zhang J.-M. et al., 2021). The percent contribution is only heuristically defined as a function of the particular path of the optimal solution of the Maxent model (Phillips, 2017). The jackknife test creates a model in three ways: (a) removing one variable at a time and relying on the remaining variables; (b) each variable is used individually; and (c) using all variables (Charrua et al., 2020). The response curves depict the Maxent model, which was generated by using only the provided variables (Phillips, 2017), which showed the quantitative relationship between environmental variables and predicted sustainability (Yi et al., 2016; Zhang et al., 2019).
2.4.1 Selection of Environmental Variables
A preliminary Maxent model screened the variables that substantially impacted Jerusalem artichoke. As mentioned in Section 2.4.3, keeping the same settings, 28 occurrence points data of Jerusalem artichoke and 35 environmental variables were imported in the Maxent model. Then the environmental variables with a percent contribution greater than 1.5 as important variables on Jerusalem artichoke were chosen. 15 environmental variables (mentioned in bold letters in Supplementary Appendix A) with substantial impacts on the distribution of Jerusalem artichoke were selected by preliminary Maxent model, including average slope (SLP), digital elevation model (DEM), soil depth (DPT), May solar radiation (SR5), July solar radiation (SR7), mean temperature of the wettest quarter (Bio8), mean temperature of coldest quarter (Bio11), annual mean temperature (Bio1), max temperature of the warmest month (Bio5), min temperature of the coldest month (Bio6), precipitation of the wettest quarter (Bio16), precipitation of the driest quarter (Bio17), precipitation seasonality (Bio15), isothermality (Bio3), and organic matter (OM). Besides, highly correlated environmental variables demonstrated interference with the result of the Maxent model (Sillero and Barbosa, 2020); as a result, Pearson’s correlation analysis was used to screen these 15 environmental factors, with 0.8 chosen as the correlation threshold (Supplementary Appendix B) (Yang et al., 2013), and nine significant environmental variables were selected for further study, including SLP, DPT, Bio5, Bio1, Bio16, SR7, Bio15, Bio17, and Bio3, and their descriptive statistics results are shown in Table 1.
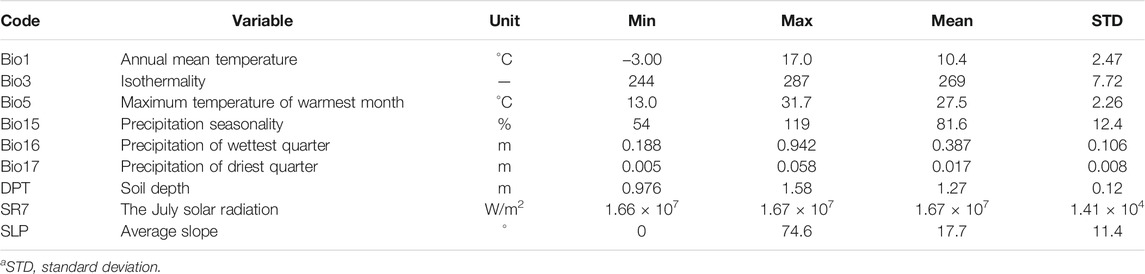
TABLE 1. Statistics of nine significant environmental variables for modeling the habitat suitability distribution of Jerusalem artichoke.
2.4.2 Importing Data
In Maxent, 28 occurrence point data of Jerusalem artichoke and nine significant environmental variables were imported.
2.4.3 Parameter Setting
The replicates option was set to 10. The feature type of response curve selected was “hinge features,” and the items checked “create response curves” and “do jackknife to measure variable importance.” The bootstrap verification method was utilized, with 25% of random presence points reserved in each study phase. The rest of the parameters were left at their default settings.
2.4.4 Model Evaluation
The area under the receiver operating characteristic (AUC) curve is used to evaluate the model’s accuracy. In general, the higher the AUC value, the stronger the correlation between the relevant environmental variables and the predicted geographical distribution of species, and the greater the accuracy of the model prediction results. The performance of the model is classified into five cases according to the value of AUC (Miguel et al., 2005): (1) fail model (AUC<0.5); (2) poor model (0.5 ≤ AUC<0.7); (3) fair model (0.7 ≤ AUC<0.8); (4) good model (0.8 ≤ AUC<0.9); and (5) excellent model (0.9 ≤ AUC<1).
2.4.5 Output Data
The output format of Maxent was set to the default output (“cloglog”) that is the easiest way to conceptualize and give estimate values between 0 and 1 of the probability of presence.
2.4.6 Results Processing
By superimposing Shaanxi Province marginal land data on the Maxent result, the distribution of marginal land suitable for Jerusalem artichoke was eventually produced. To conceptualize the results according to Kumar (2012), the probability of existence ranged from 0 to 1 was reclassified and divided into four classes in ArcGIS 10.2: (1) unsuitable area (<0.08); (2) moderate potential area (0.08–0.28); (3) good potential area (0.28–0.58); and (4) high potential area (>0.58).
3 Results
3.1 Modeling Precision
Because the results of the same species distribution predicted by this model somewhat varied each time (Cullen, 2017), the average results of 10 model runs were used in this study. The mean standard deviation of the model after 10 replicates was 0.08. The AUC values of all models performed well in the training data, ranging from 0.86 to 0.94 with a mean of 0.90. While in the test data, the AUC values ranged from 0.65 to 0.94 with a mean of 0.79. The area under the receiver operating characteristic curves with error bars and the average AUC across models (Figure 1) show an excellent model. The mean results of the model after 10 replicates calculations are used to ensure the reliability of the model results. Therefore, the model successfully predicted the distribution of Jerusalem artichoke in theory.
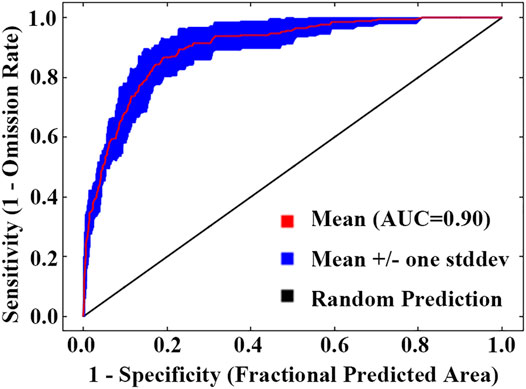
FIGURE 1. ROC curves with error bars and average AUC across models (the red curve shows the mean of AUC of the Maxent model, the blue margins indicated the mean of +/− one standard deviation of the Maxent model, and the black line shows the random prediction calculated in 10 replicates respectively).
3.2 Response of Significant Environmental Variables to Jerusalem Artichoke Species Distribution
Percentage contributions of the variables in the Maxent model are shown in Figure 2A with a descending order of SLP, DPT, Bio5, Bio1, Bio16, SR7, Bio15, Bio17, and Bio3. Figure 2B illustrates the jackknife test of regularized training gain of variable importance, which shows the variables SLP, Bio5, Bio1, Bio16, DPT, Bio15, SR7, Bio17, and Bio3 in a descending order. SLP and DPT were the most potent predictors of Jerusalem artichoke distribution since SLP and DPT variables contained more information than other factors. The training gain was dramatically reduced when the SLP and DPT variables were excluded. When Bio3 and Bio17 were employed in isolation, they had a minor influence on calculating the spread of Jerusalem artichoke (Figure 2B).
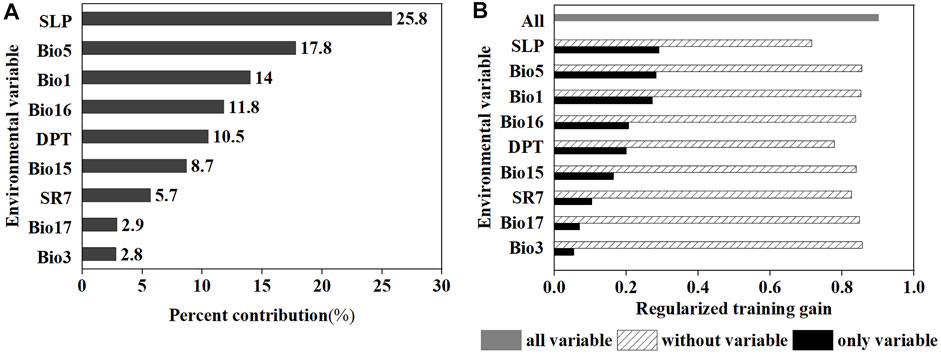
FIGURE 2. Evaluation of the significant environmental predictors: (A) the estimates of percent contributions of the environmental variables to the Maxent model; (B) jackknife test evaluating the significant environmental variables on the distribution of Jerusalem artichoke.
Figure 3 depicts the response curves of the nine significant environmental variables on the distribution of Jerusalem artichoke. It also indicates that the Jerusalem artichoke–favored areas in Shaanxi Province are places where the annual mean temperature (Bio1) is 16.5–18.0°C, the isothermality (Bio3) is 265–275, the max temperature of the warmest month (Bio5) is 30–31°C, the precipitation seasonality (Bio15) is 50–60%, the precipitation of wettest quarter (Bio16) is 0.01–0.02 m, the precipitation of the driest quarter (Bio17) is 0–0.005 m, the soil depth (DPT) is 1.50–1.60 m, the average slope (SLP) is 0–5°, and the July solar radiation (SR7) is 1.66–1.67 × 107 W/m2.
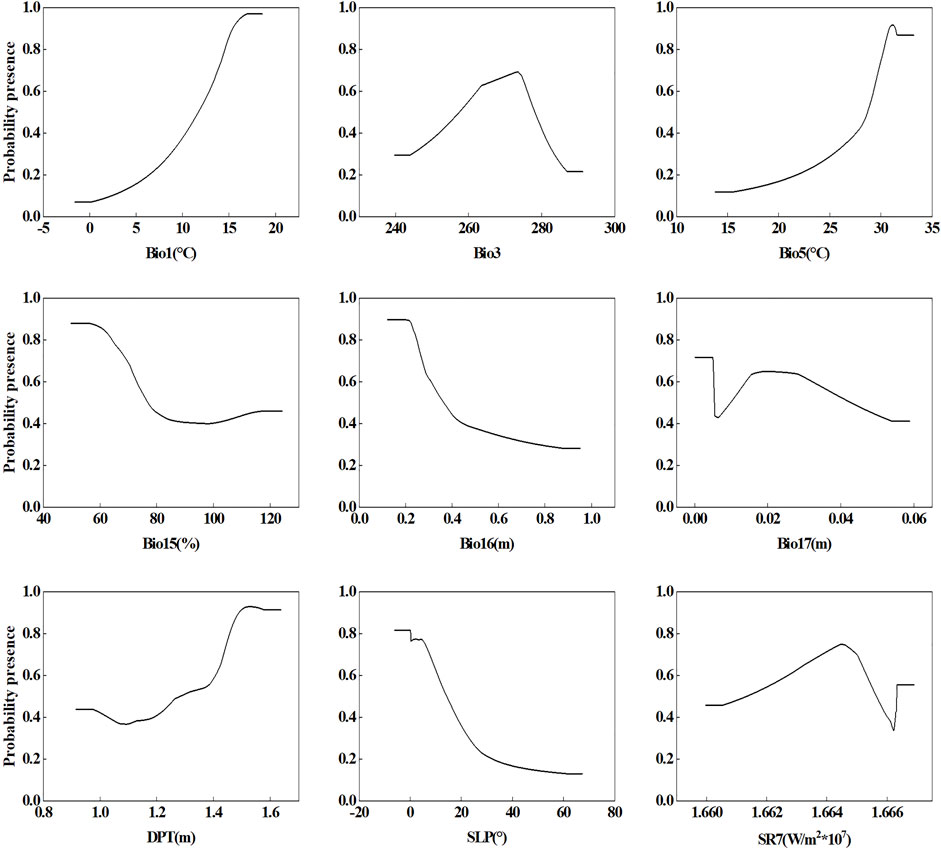
FIGURE 3. The mean response curves of the 9 significant environment variables of the Maxent model calculated in 10 replicates.
3.3 Species Distribution and Habitat Suitability on Marginal Land
The prediction of the Maxent model is shown in Figure 4A, which indicates that the suitable areas for growing Jerusalem artichoke in the Guanzhong Plain (Xianyang, Baoji, Weinan, and Hanzhong) and the Southern Shaanxi (mainly Ankang and Shangluo). Superimposing Figure 4A and the marginal land of Shaanxi Province (Figure 4B) in ArcGIS 10.2 yielded the suitable marginal land for growing Jerusalem artichoke (Figure 4C), which revealed that moderately dense grassland was the dominating land type used for growing Jerusalem artichoke. The classification result of marginal land is shown in Figure 4D, which indicates that the areas suitable for Jerusalem artichoke are mainly in northern and southern parts of Shaanxi Province.
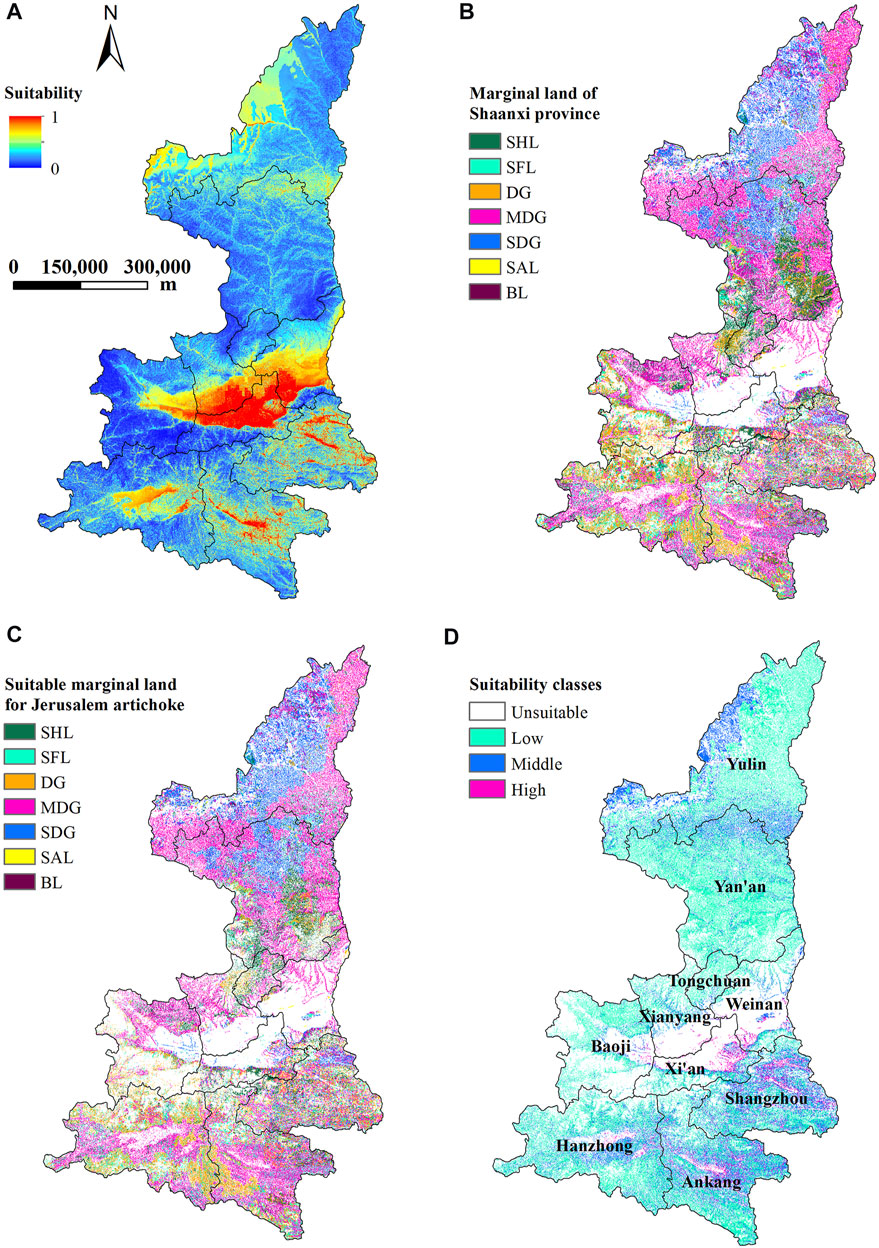
FIGURE 4. The area suitable for planting Jerusalem artichoke in Shaanxi Province: (A) spatial distribution of the pre-diction of the Maxent model (different colors indicate the prediction probability of different suitable conditions, and the red color (the value close to 1) means more suitable for planting, and the blue color (the value close to 0) means less suitable for planting of Jerusalem artichoke); (B) spatial distribution of marginal land of Shaanxi Province (SHL: shrubland, SFL: sparse forest land, DG: dense grassland, MDG: moderate dense grassland, SDG: sparse dense grassland, SAL: saline land, BL: bare land); (C) spatial distribution of marginal land suitable for Jerusalem artichoke in Shaanxi Province; (D) spatial distribution of classification results of marginal land suitable for Jerusalem artichoke in Shaanxi Province.
The results of the statistics in Figure 4C are shown in Table 2. The results show that the suitable area of shrubland, sparse forest land, dense grassland, moderately dense grassland, sparsely dense grassland, saline land, and bare land are 1.06 × 1010 m2, 9.94 × 109 m2, 1.46 × 1010 m2, 4.00 × 1010 m2, 1.26 × 1010 m2, 1.37 × 108 m2, and 1.51 × 108 m2, respectively. Accounting for 99.7% of the total appropriate area in the southern (Hanzhong, Ankang, and Shangluo) and northern (Yan’an and Yulin) regions of Shaanxi Province, shrubland, sparse forest land, and dense, sparsely dense, and moderately dense grasslands are best for cultivating Jerusalem artichokes.
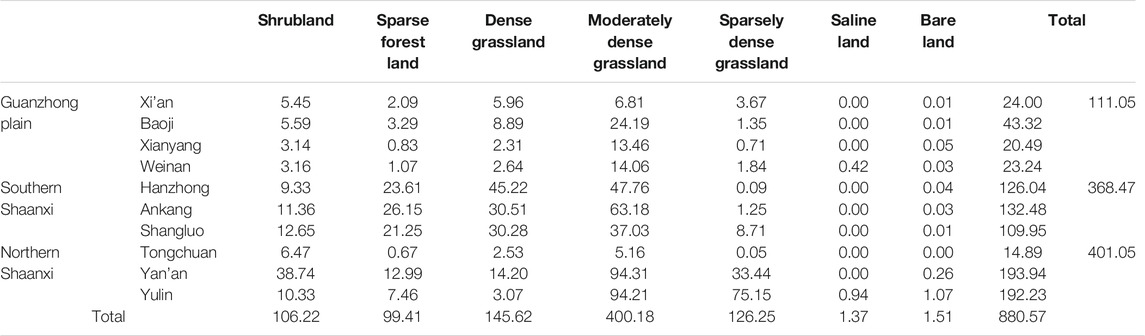
TABLE 2. Statistical results of marginal land suitable for growing Jerusalem artichoke in each city of Shaanxi Province with the unit 1.00 × 108 m2.
The results of the statistics in Figure 4D indicated that incompetent, moderate potential, good potential, and high potential areas for growing Jerusalem artichoke are approximately 1.89 × 1010 m2, 6.29 × 1010 m2, 2.19 × 1010 m2, and 3.19 × 1010 m2, respectively (Table 3). The total suitable area (moderate potential, good potential, and high potential) for growing Jerusalem artichoke in Shaanxi Province is approximately 8.81 × 1010 m2, with the southern (Hanzhong, Ankang, and Shangluo) and northern (Yan’an and Yulin) regions accounting for 42.8% of the total area of Shaanxi Province.
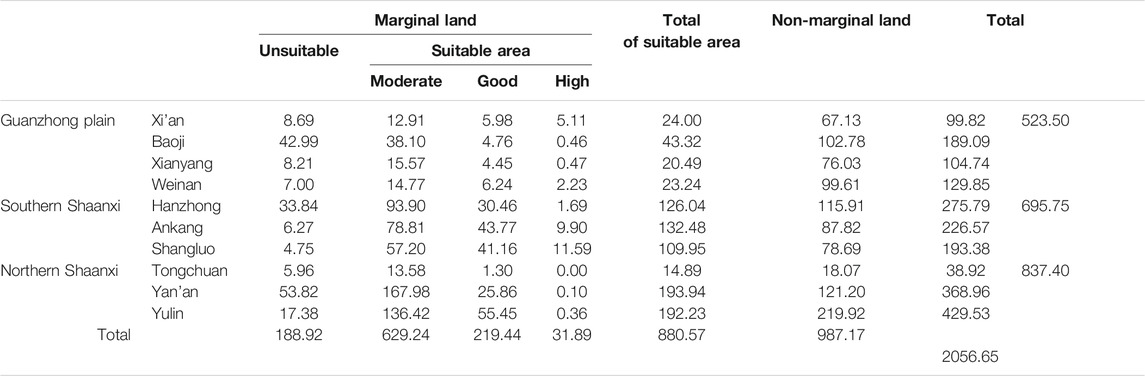
TABLE 3. Statistical results of classification of marginal lands suitable for planting Jerusalem artichoke in Shaanxi Province with the unit 1.00 × 108 m2.
4 Discussion
4.1 Reliability of the Model
Studies have shown that soil, topography, and solar radiation are also important factors affecting the growth of Jerusalem artichoke (Zorić et al., 2016). Unlike previous studies that were limited to the effects of climatic factors on species distribution (Zhang et al., 2020; Tarnian et al., 2021), this study considered the effects of soil depth, topography, land use, and solar radiation on the distribution of Jerusalem artichoke. This multifactorial pattern explained and predicted species distribution better than a temperature-based pattern, and it was more in line with plant growth needs.
Furthermore, the preliminary Maxent model (Supplementary Appendix A) revealed that not all environmental variables substantially impact Jerusalem artichoke growth. A previous research has shown that modeling with strongly correlated variables might give unexpected results (Sillero and Barbosa, 2020). Moreover, compared with the multifactor-integrated assessment method, the Maxent model can obtain the contribution of environmental factors for species and respond to the impact of each environmental factor on the species. To improve the calculation efficiency of the model, this study screened 15 environmental variables that have a significant effect on the distribution of Jerusalem artichoke in combination with the percent contribution of the preliminary Maxent model, and then selected nine important environmental factors by Pearson’s correlation analysis, excluding covariance among environmental variables. Then to make the model more resilient, the mean results from running the Maxent model 10 times are used, and each model has good accuracy.
Meanwhile, the distribution of Jerusalem artichoke in this study is consistent with previous research (Xu et al., 2013). The total area suitable for the growth of Jerusalem artichoke in this study is approximately 8.81 × 1010 m2, which is less than 11.0 × 1010 m2 given in Liu’s study (Liu, 2011) owing to differences in research methods, environmental factors selection, classification criteria, and land use data. The results of the model, as stated previously, are credible.
4.2 Argumentation of the Response Results of Environmental Variables
Because of differences in evaluation methods, the jackknife and percent contribution results had minor differences in terms of variable orders. Still, the variables that significantly affected the Jerusalem artichoke were consistent, confirming that SLP and DPT were the main environmental variables affecting the distribution of Jerusalem artichoke. They were also compatible with the percent contribution of the preliminary model (Supplementary Appendix A). These findings revealed that topography and soil conditions were the most relevant variables for the Jerusalem artichoke species being modeled.
Furthermore, Jerusalem artichoke is a long day plant that requires a short photoperiod to flower, grows best at 18–26°C (Zhuang et al., 2011), needs abundant rainfall (>0.15 m) (Swanton et al., 1992), and it can survive in both flat and mountainous areas (Wang et al., 2019). The results of this study are compatible with these conditions. Moreover, the findings demonstrated that seasonality significantly impacted Jerusalem artichoke growth, consistent with earlier studies (Zorić et al., 2016; Krivorotova and Sereikaite, 2018).
4.3 Practical Implications of the Study
According to studies, Jerusalem artichoke has a huge potential for energy plant development in the arid and semi-arid areas in the western part of China (Liu et al., 2011). Simultaneously, the development and utilization of Jerusalem artichoke are of great practical significance to assist rural communities in overcoming poverty (Nie et al., 2022). According to the investigation, most marginal land areas are underutilized, and large-scale rational planting has yet to be realized. Jerusalem artichoke only grows sporadically on roadsides and wastelands in some regions. Thus, this study selected the Jerusalem artichoke as an energy plant and researched its potential on marginal lands that can guide the rational planting of Jerusalem artichoke, which is in line with the practical requirements.
Furthermore, the area suited for Jerusalem artichoke differed from Figure 4A, owing to the perfect environmental conditions for cultivating Jerusalem artichoke in Xi’an, Baoji, Xianyang, and Weinan. As shown in Figure 4D, the marginal land ideal for the growth of Jerusalem artichoke in Guanzhong only accounted for 5.40% of Shaanxi Province, indicating that industrialization and urbanization significantly impact the distribution of Jerusalem artichoke species. Furthermore, when the Guanzhong Plain (Xi’an, Baoji, Xianyang, and Weinan) was excluded, the results in Figure 4D showed that the area suitable for planting Jerusalem artichoke in Northern Shaanxi was greater than that in Southern Shaanxi. But, the good potential and high potential locations more ideal for producing Jerusalem artichoke in Northern Shaanxi were less than those in Southern Shaanxi. The differences between Southern and Northern Shaanxi in terms of nine environmental variables are shown in Figure 5, and this result shows significant differences between Southern and Northern Shaanxi at Bio1, Bio15, Bio16, Bio17, and SR7. Thus, these environmental variables make Southern Shaanxi more suitable for planting Jerusalem artichoke than Northern Shaanxi in terms of quality. It can be seen that temperature, precipitation, and solar radiation are important factors affecting the classification of Jerusalem artichoke suitability. Thus, this study’s findings have greater practical implications and can guide smart Jerusalem artichoke planning.
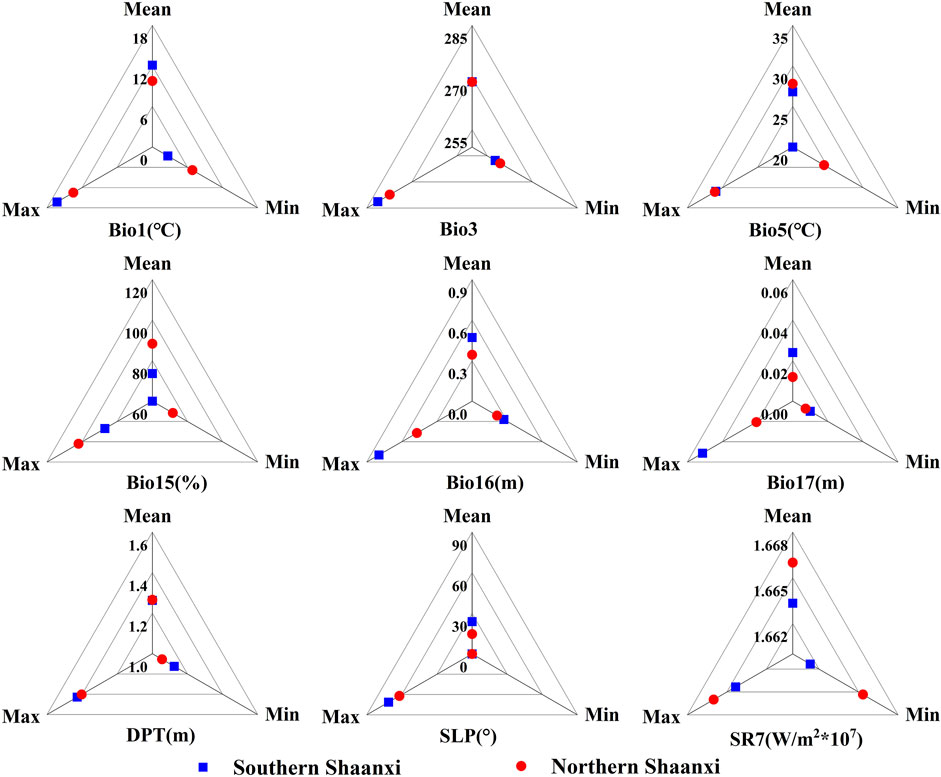
FIGURE 5. Mean, maximum and minimum values of 9 significant environment variables in Southern and Northern Shaanxi.
5 Conclusion
This study used the Maxent model and ArcGIS tool to predict the distribution of Jerusalem artichoke on the marginal land in Shaanxi Province. Not only was the potential distribution of Jerusalem artichoke determined but also the main influencing factors and regional differences affecting the distribution of Jerusalem artichoke in Shaanxi Province were also discussed. Finally, the marginal land resource potential and habitat suitability classification for planting Jerusalem artichoke were evaluated:
a) The significant environmental variables affecting Jerusalem artichoke were SLP, DPT, Bio5, Bio1, Bio16, SR7, Bio15, Bio17, and Bio3. The suitable ranges of these environmental variables were 0–5°C, 1.50–1.60 m, 30–31°C, 16.5–18.0°C, 0.01–0.02 m, 1.66–1.67 × 107 W/m2, 50–60%, 0–0.005 m, and 265–275, respectively. Additionally, the percent contribution and the jackknife test results showed that SLP and DPT were the strongest predictors affecting the distribution of Jerusalem artichoke species. In other words, the variables of topography and soil conditions have the most critical impact on species distribution.
(b) Shrubland, sparse forest land, dense grassland, moderately dense grassland, and sparsely dense grassland were the peripheral land use types more favorable for growing Jerusalem artichoke, accounting for 99.7% of the total suitable area for growing Jerusalem artichoke. Among them, moderately dense grassland was the dominant land use type for the growth of Jerusalem artichoke.
(c) The total area of Shaanxi Province suitable for cultivating Jerusalem artichoke was roughly 8.81 × 1010 m2, accounting for 42.7% of the total area of Shaanxi Province. The area suitable for planting Jerusalem artichoke in Northern Shaanxi was more than Southern Shaanxi in terms of quantity. But, Hanzhong, Ankang, Shangluo, Yan’an, and Yulin areas of Southern Shaanxi were ideal for planting Jerusalem artichoke than Northern Shaanxi in terms of quality.
Data Availability Statement
The raw data supporting the conclusion of this article will be made available by the authors, without undue reservation.
Author Contributions
FY contributed to conceptualization. DZ, TA, and LY helped with data curation. FY and DZ performed formal analysis. DZ, ZY, and LY assisted with investigation. DZ and FY framed the methodology. FY contributed to project administration. DZ, FY, and LY helped with resources. DZ assisted with software. FY contributed to supervision. DZ assisted with writing—original draft. TA and FY helped with writing—review and editing.
Funding
The research was funded by the National Natural Science Foundation of China (42071258), Fund Project of Shaanxi Key Laboratory of Land Consolidation (2018-ZY04), and the Special Fund for Basic Scientific Research of Central Colleges (Nos. 300102270204, 300102278303, and 300102280401), Chang’an University, China.
Conflict of Interest
The authors declare that the research was conducted in the absence of any commercial or financial relationships that could be construed as a potential conflict of interest.
Publisher’s Note
All claims expressed in this article are solely those of the authors and do not necessarily represent those of their affiliated organizations, or those of the publisher, the editors, and the reviewers. Any product that may be evaluated in this article, or claim that may be made by its manufacturer, is not guaranteed or endorsed by the publisher.
Supplementary Material
The Supplementary Material for this article can be found online at: https://www.frontiersin.org/articles/10.3389/fenvs.2022.837947/full#supplementary-material
References
Bauer, N., Klein, D., Humpenöder, F., Kriegler, E., Luderer, G., Popp, A., et al. (2020). Bio-energy and CO2 Emission Reductions: an Integrated Land-Use and Energy Sector Perspective. Climatic Change 163 (3), 1675–1693. doi:10.1007/s10584-020-02895-z
Bogucka, B., and Jankowski, K. (2020). Jerusalem Artichoke: Quality Response to Potassium Fertilization and Irrigation in Poland. Agronomy 10 (10), 1518. doi:10.3390/agronomy10101518
Bogucka, B., Pszczółkowska, A., Okorski, A., and Jankowski, K. (2021). The Effects of Potassium Fertilization and Irrigation on the Yield and Health Status of Jerusalem Artichoke (Helianthus Tuberosus L.). Agronomy 11 (2), 234. doi:10.3390/agronomy11020234
Cao, B., Bai, C., Zhang, M., Lu, Y., Gao, P., Yang, J., et al. (2022). Future Landscape of Renewable Fuel Resources: Current and Future Conservation and Utilization of Main Biofuel Crops in China. Sci. Total Environ. 806, 150946. doi:10.1016/j.scitotenv.2021.150946
Charrua, A. B., Bandeira, S. O., Catarino, S., Cabral, P., and Romeiras, M. M. (2020). Assessment of the Vulnerability of Coastal Mangrove Ecosystems in Mozambique. Ocean Coastal Manag. 189, 105145. doi:10.1016/j.ocecoaman.2020.105145
Council, S. (2018). The State Council Issued a Notice on the Three-Year Action Plan to Win the Blue Sky Defense War, Issued by the State Council. Available at: http://www.gov.cn/zhengce/content/2018-07/03/content_5303158.htm (Accessed October 3, 2021).
Cullen, R. (2017). Evaluating Renewable Energy Policies. Aust. J. Agric. Resour. Econ. 61 (1), 1–18. doi:10.1111/1467-8489.12175
Epie, K. E., Santanen, A., Makela, P. S. A., and Stoddard, F. L. (2018). Fertilizer and Intercropped Legumes as Nitrogen Source for Jerusalem Artichoke (Helianthus Tuberosus L.) Tops for Bioenergy. Agric. Food Sci. 27 (3), 199–205. doi:10.23986/afsci.70110
Foster, G. (2019). Low-Carbon Futures for Bioethylene in the United States. Energies 12 (10), 1958. doi:10.3390/en12101958
Fukuda, S., De Baets, B., Waegeman, W., Verwaeren, J., and Mouton, A. M. (2013). Habitat Prediction and Knowledge Extraction for Spawning European grayling (Thymallus thymallus L.) Using a Broad Range of Species Distribution Models. Environ. Model. Softw. 47 (-), 1–6. doi:10.1016/j.envsoft.2013.04.005
Ghareghan, F., Ghanbarian, G., Pourghasemi, H. R., and Safaeian, R. (2020). Prediction of Habitat Suitability of Morina Persica L. Species Using Artificial Intelligence Techniques. Ecol. Indicators 112, 106096. doi:10.1016/j.ecolind.2020.106096
Jhariya, M., Banerjee, A., Meena, R., Kumar, S., and Raj, A. (2021c). Sustainable Intensification for Agroecosystem Services and Management. Springer Nature Singapore.
Jhariya, M., Banerjee, A., Meena, R., and Yadav, D. (2019). Sustainable Agriculture, Forest and Environmental Management. Springer Nature Singapore.
Jhariya, M., Meena, R., and Banerjee, A. (2021a). Ecological Intensification of Natural Resources for Sustainable Agriculture. Springer Nature Singapore.
Jiang, D., Wang, Q., Ding, F., Fu, J., and Hao, M. (2019). Potential Marginal Land Resources of Cassava Worldwide: A Data-Driven Analysis. Renew. Sust. Energ. Rev. 104 (APR), 167–173. doi:10.1016/j.rser.2019.01.024
Jiang, W., Jacobson, M. G., and Langholtz, M. H. (2018). A Sustainability Framework for Assessing Studies about Marginal Lands for Planting Perennial Energy Crops. Biofuels, Bioprod. Bioref. 13 (1), 228–240. doi:10.1002/bbb.1948
Jones, M. B., Finnan, J., and Hodkinson, T. R. (2015). Morphological and Physiological Traits for Higher Biomass Production in Perennial Rhizomatous Grasses Grown on Marginal Land. GCB Bioenergy 7 (2), 375–385. doi:10.1111/gcbb.12203
Krivorotova, T., and Sereikaite, J. (2018). Correlation between Fructan Exohydrolase Activity and the Quality of Helianthus Tuberosus L. Tubers. Agronomy 8 (9), 184. doi:10.3390/agronomy8090184
Kumar, P. (2012). Assessment of Impact of Climate Change on Rhododendrons in Sikkim Himalayas Using Maxent Modelling: Limitations and Challenges. Biodivers Conserv 21 (5), 1251–1266. doi:10.1007/s10531-012-0279-1
Liu, L. (2011). The Potential and Impacts of Biofuel Development for the Five Provinces in Southwest China. Beijing: dissertation, University of Chinese Academy of Sciences.
Leppäkoski, L., Marttila, M. P., Uusitalo, V., Levänen, J., Halonen, V., and Mikkilä, M. H. (2021). Assessing the Carbon Footprint of Biochar from Willow Grown on Marginal Lands in Finland. Sustainability 13 (18), 10097. doi:10.3390/su131810097
Li, J., Fan, G., and He, Y. (2020a). Predicting the Current and Future Distribution of Three Coptis Herbs in China under Climate Change Conditions, Using the MaxEnt Model and Chemical Analysis. Sci. Total Environ. 698, 134141. doi:10.1016/j.scitotenv.2019.134141
Li, X., Cen, N., Liu, L., Chen, Y., Yang, X., Yu, K., et al. (2020b). Collagen Peptide Provides Saccharomyces cerevisiae with Robust Stress Tolerance for Enhanced Bioethanol Production. ACS Appl. Mater. Inter. 12 (48), 53879–53890. doi:10.1021/acsami.0c18919
Li, Y., Shao, W., and Jiang, J. (2021). Predicting the Potential Global Distribution of Sapindus Mukorossi under Climate Change Based on MaxEnt Modelling. Environ. Sci. Pollut. Res. 18. doi:10.1007/s11356-021-17294-9
Liu, Z., Han, L., Yosef, S., and Guang, h. (2011). Genetic Variation and Yield Performance of Jerusalem Artichoke Germplasm Collected in China. Agricultural Sciences in China. doi:10.1016/s1671-2927(11)60049-7
Liu, Z. X., Steinberger, Y., Chen, X., Wang, J. S., and Xie, G. H. (2015). Chemical Composition and Potential Ethanol Yield of Jerusalem Artichoke in a Semi-arid Region of China. Ital. J. Agron. 10 (1), 34. doi:10.4081/ija.2015.603
Long, X., Shao, H., Liu, L., Liu, L., and Liu, Z. (2016). Jerusalem Artichoke: A Sustainable Biomass Feedstock for Biorefinery. Renew. Sust. Energ. Rev. 54, 1382–1388. doi:10.1016/j.rser.2015.10.063
Maddalwar, S., Kumar Nayak, K., Kumar, M., and Singh, L. (2021). Plant Microbial Fuel Cell: Opportunities, Challenges, and Prospects. Bioresour. Tech. 341, 125772. doi:10.1016/j.biortech.2021.125772
Mehmood, M. A., Ahmad, M. S., Liu, Q., Liu, C.-G., Tahir, M. H., Aloqbi, A. A., et al. (2019). Helianthus Tuberosus as a Promising Feedstock for Bioenergy and Chemicals Appraised through Pyrolysis, Kinetics, and TG-FTIR-MS Based Study. Energ. Convers. Manag. 194, 37–45. doi:10.1016/j.enconman.2019.04.076
Merow, C., Smith, M. J., and Silander, J. A. (2013). A Practical Guide to MaxEnt for Modeling Species' Distributions: what it Does, and Why Inputs and Settings Matter. Ecography 36 (10), 1058–1069. doi:10.1111/j.1600-0587.2013.07872.x
Mi, Z., Meng, J., Guan, D., Shan, Y., Song, M., Wei, Y.-M., et al. (2017). Chinese CO2 Emission Flows Have Reversed since the Global Financial Crisis. Nat. Commun. 8, 10. doi:10.1038/s41467-017-01820-w
Miguel, B. A., Pearson, Richard. G., Thuiller, Wilfried., and Erhard, Markus. (2005). Validation of Species-Climate Impact Models under Climate Change. Glob. Change Biol. 11 (9). doi:10.1111/j.1365-2486.2005.001000.x
Nie, Y., Li, J., Wang, C., Huang, G., Fu, J., Chang, S., et al. (2022). A fine-resolution Estimation of the Biomass Resource Potential across China from 2020 to 2100. Resour. Conservation Recycling 176, 105944. doi:10.1016/j.resconrec.2021.105944
Paul, S., Bhagobaty, R. K., Nihalani, M. C., and Joshi, S. R. (2020). Characterization of Oleaginous Endophytic Fungi of Biodiesel Plants as Potential Biofuel Minifactories. Biomass and Bioenergy 142, 105750. doi:10.1016/j.biombioe.2020.105750
Phillips, S. J. (2017). A Brief Tutorial on Maxent. Available at: http://biodiversityinformatics.amnh.org/open_source/maxent/(Accessed October 5, 2021).
Phillips, S. J., Dudík , , and Schapire, R. E. (2004). A Maximum Entropy Approach to Species Distribution Modeling, Proceedings of the twenty-first international conference on Machine learning, Banff, Alberta, July 4–8, 2004. Machine learning. doi:10.1145/1015330.1015412
Phillips, S. J., Anderson, R. P., and Schapire, R. E. (2006). Maximum Entropy Modeling of Species Geographic Distributions. Ecol. Model. 190 (3-4), 231–259. doi:10.1016/j.ecolmodel.2005.03.026
Qaseem, M. F., and Wu, A.-M. (2020). Marginal Lands for Bioenergy in China; an Outlook in Status, Potential and Management. GCB Bioenergy 13 (1), 21–44. doi:10.1111/gcbb.12770
Qaseem, M. F., and Wu, A.-M. (2021). Marginal Lands for Bioenergy in China; an Outlook in Status, Potential and Management. GCB Bioenergy 13 (1), 21–44. doi:10.1111/gcbb.12770
Richards, B. K., Stoof, C. R., Cary, I. J., and Woodbury, P. B. (2014). Reporting on Marginal Lands for Bioenergy Feedstock Production: a Modest Proposal. Bioenerg. Res. 7 (3), 1060–1062. doi:10.1007/s12155-014-9408-x
Shi, H., Chai, J., Lu, Q., Zheng, J., and Wang, S. (2022). The Impact of China's Low-Carbon Transition on Economy, Society and Energy in 2030 Based on CO2 Emissions Drivers. Energy 239, 122336. doi:10.1016/j.energy.2021.122336
Shortall, O. K. (2013). “Marginal Land” for Energy Crops: Exploring Definitions and Embedded Assumptions. Energy Policy 62, 19–27. doi:10.1016/j.enpol.2013.07.048
Sillero, N., and Barbosa, A. M. (2020). Common Mistakes in Ecological Niche Models. Int. J. Geographical Inf. Sci. 35 (2), 213–226. doi:10.1080/13658816.2020.1798968
Swanton, C. J., Clements, D. R., Moore, M. J., and Cavers, P. B. (1992). The Biology of Canadian Weeds. 101. Helianthus Tuberosus L. Can. J. Plant Sci. 72 (72), 1367–1382.
Tarnian, F., Kumar, S., Azarnivand, H., Chahouki, M. A. Z., and Mossivand, A. M. (2021). Assessing the Effects of Climate Change on the Distribution of Daphne Mucronata in Iran. Environ. Monit. Assess. 193 (9), 1–19. doi:10.1007/s10661-021-09311-8
Wang, L., Sun, X., Tan, L., and Yi, L. I. (2019). Study on Accumulation and Dynamic Changes of Three Carbohydrates in Jerusalem Artichoke in Natural Habitats. Agricultutural Blotechnology 8 (3), 7.
Wen, J., Song, J., Xie, C., Zhang, Q., Zeng, F., and Zhang, Y. (2016). Identification of Potential Distribution Areas for Energy Plant Jatropha Curcas L. Using the Maxent Entropy Model. Plant Sci. J. 34 (6), 849–856.
Xu, X., Li, S., Fu, Y., and Zhuang, D. (2013). An Analysis of the Geographic Distribution of Energy Crops and Their Potential for Bioenergy Production. Biomass and Bioenergy 59, 325–335. doi:10.1016/j.biombioe.2013.08.036
Xu, X., Liu, J., Zhang, S., Li, R., Yan, C., and Wu, S. (2018). China Multi-Period Land Use Land Cover Remote Sensing Monitoring Data Set(CNLUCC). Beijing: Data Registration and Publishing System of the Resource and Environmental Science Data Center of the Chinese Academy of Sciences. [DB/OL].
Xue, S., Guo, M., Iqbal, Y., Liao, J., Yang, S., Xiao, L., et al. (2020). Mapping Current Distribution and Genetic Diversity of the Native Miscanthus Lutarioriparius across China. Renew. Sust. Energ. Rev. 134, 110386. doi:10.1016/j.rser.2020.110386
Xue, S., Lewandowski, I., Wang, X., and Yi, Z. (2016). Assessment of the Production Potentials of Miscanthus on Marginal Land in China. Renew. Sust. Energ. Rev. 54, 932–943. doi:10.1016/j.rser.2015.10.040
Yang, X., Kushwaha, S. P. S., Saran, S., Xu, J., and Roy, P. S. (2013). Maxent Modeling for Predicting the Potential Distribution of Medicinal Plant, Justicia Adhatoda L. In Lesser Himalayan Foothills. Ecol. Eng. 51, 83–87. doi:10.1016/j.ecoleng.2012.12.004
Yi, Y. J., Cheng, X., Yang, Z. F., and Zhang, S. H. (2016). Maxent Modeling for Predicting the Potential Distribution of Endangered Medicinal Plant (H. riparia Lour) in Yunnan, China. Ecol. Eng. 92, 260–269. doi:10.1016/j.ecoleng.2016.04.010
Yin, F., Yang, X., Wang, H., Liu, L., Meng, X., and Li, S. (2018). Evaluation of the Potential Land for Biofuel Plant Development in the Shaanxi Province, China. Geol. J. 53, 332–341. doi:10.1002/gj.3120
Zhang, B., Yang, J., and Cao, Y. (2021a). Assessing Potential Bioenergy Production on Urban Marginal Land in 20 Major Cities of China by the Use of Multi-View High-Resolution Remote Sensing Data. Sustainability 13 (13), 7291. doi:10.3390/su13137291
Zhang, J. M., Song, M. L., Li, Z. J., Peng, X. Y., Su, S., Li, B., et al. (2021b). Effects of Climate Change on the Distribution of Akebia Quinata. Front. Ecol. Evol. 9, 11. doi:10.3389/fevo.2021.752682
Zhang, K., Zhang, Y., Jia, D., and Tao, J. (2020). Species Distribution Modeling of Sassafras Tzumu and Implications for Forest Management. Sustainability 12 (10), 4132. doi:10.3390/su12104132
Zhang, L., Jing, Z., Li, Z., Liu, Y., and Fang, S. (2019). Predictive Modeling of Suitable Habitats for Cinnamomum Camphora (L.) Presl Using Maxent Model under Climate Change in China. Ijerph 16 (17), 3185. doi:10.3390/ijerph16173185
Zhao, X., and Liu, P. (2014). Focus on Bioenergy Industry Development and Energy Security in China. Renew. Sust. Energ. Rev. 32, 302–312. doi:10.1016/j.rser.2014.01.011
Zhuang, D., Jiang, D., Liu, L., and Huang, Y. (2011). Assessment of Bioenergy Potential on Marginal Land in China. Renew. Sust. Energ. Rev. 15 (2), 1050–1056. doi:10.1016/j.rser.2010.11.041
Keywords: marginal land resources, energy plant, Maxent model, environmental variables, habitat suitability, Shaanxi Province
Citation: Zhao D, Yin F, Ashraf T, Yuan Z and Ye L (2022) Evaluation of Marginal Land Potential and Analysis of Environmental Variables of Jerusalem Artichoke in Shaanxi Province, China. Front. Environ. Sci. 10:837947. doi: 10.3389/fenvs.2022.837947
Received: 17 December 2021; Accepted: 17 January 2022;
Published: 11 February 2022.
Edited by:
Ayman Elshkaki, Institute of Geographic Sciences and Natural Resources Research (CAS), ChinaReviewed by:
Manoj Kumar Jhariya, Sant Gahira Guru Vishwavidyalaya, IndiaLal Singh, National Environmental Engineering Research Institute (CSIR), India
Copyright © 2022 Zhao, Yin, Ashraf, Yuan and Ye. This is an open-access article distributed under the terms of the Creative Commons Attribution License (CC BY). The use, distribution or reproduction in other forums is permitted, provided the original author(s) and the copyright owner(s) are credited and that the original publication in this journal is cited, in accordance with accepted academic practice. No use, distribution or reproduction is permitted which does not comply with these terms.
*Correspondence: Fang Yin, eWluZkBjaGQuZWR1LmNu