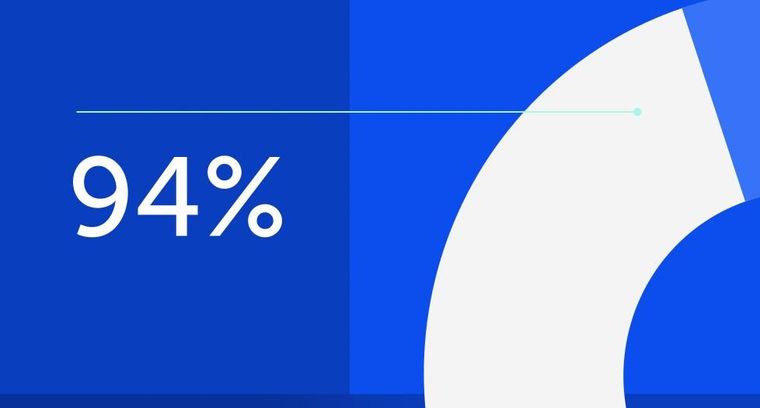
94% of researchers rate our articles as excellent or good
Learn more about the work of our research integrity team to safeguard the quality of each article we publish.
Find out more
ORIGINAL RESEARCH article
Front. Environ. Sci., 12 April 2022
Sec. Science and Environmental Communication
Volume 10 - 2022 | https://doi.org/10.3389/fenvs.2022.834760
This article is part of the Research TopicFood, Media, and the Environment: Cultures, Practices, and PoliciesView all 5 articles
The extension and diffusion of the green production mode in rural areas forms the micro basis for improve the ecological environment. While government has issued a series of policies to promote the green production mode, the effect depends on farmers’ behavior. Therefore, it is necessary to explore the causes and mechanisms underlying the influence of farmers’ behavior differences with regard to biopesticide application. Based on 1202 sampled rice farmers in China, this paper analyzes the impact of social network on their biological pesticide application behavior. Furthermore, the internal transmission mechanism of social network on biological pesticide application behavior of “ration-making” farmers (whose main purpose is to meet the demand for rations) and “profit-making” famers (whose main goal is to maximize their profit) is studied according to different production purposes. The results show that: 1) Social network has a significant positive role in promoting rice farmers’ biological pesticide application behavior. 2) Social network encourages rice farmers to apply biological pesticides by influencing both their behavioral attitude and behavioral efficacy toward green production. 3) The intermediary effect of behavioral efficacy exceeds that of behavioral attitude. 4) Compared with “profit-making” farmers, the biological pesticides application of “ration-making” farmers is stronger affected by their social network. Operation mode, operation scale, and educational level positively affect the pesticide application behavior of “profit-making” farmers, while age imposes a significantly negative influence. Biopesticide application of “ration-making” farmers is negatively affected by age and education level and positively affected by the mode of operation. 5) The behavioral attitude and behavioral efficacy of green production exert significant mediating effects on both types of farmers. Moreover, both intermediary variables exert stronger impacts on “profit-making” farmers. To a certain extent, this study expands the theoretical explanation of the “rational man” in neoclassical economics, and provides reliable evidence, both theoretical and empirical, for effective measures to stimulate green production in rice farmers.
For a long time, driven by accelerated industrialization and urbanization, obstacles imposed by the resource carrying capacity and the ecological environment capacity on China’s rural economic and social development have gradually become apparent. The green economy and green development have therefore received unprecedented attention (Acemoglu, 2008; Zhao, 2012). The extension and diffusion of the green production mode in rural areas forms the micro basis for the realization of a green development of agriculture. As a typical part of the green production mode, biological pesticides are of great significance to improve the ecological environment and ensure both the quality and safety of agricultural products (Hokkanen and Hajek, 2003; Huang et al., 2016). The Chinese government has issued a series of policies with the aim to lead farmers to the use of biological pesticides. This approach not only aims to promote the active participation of farmers in their application of biological pesticides, but also to accelerate the green transformation of agricultural production in general. These policies guide the application of biological pesticides at a governmental level. Since 2002, China has spent an average of nearly RMB 30 million per year on the promotion of green farming, and the relevant governmental departments have also adopted different forms of subsidies, tax exemptions, and other ways to encourage farmers to apply biological pesticides (or biopesticides) (Xie et al., 2018).
The promotion effect of biopesticides depends on farmers’ willingness to adopt them and its changing trend. In general, farmers tend to have a stronger willingness to adopt behaviors that indicates large expected earnings and high earnings certainty (Nofsinger, 2002). Therefore, a cost-benefit analysis is the main link that affects farmers’ decision-making, while material capital and human capital are regarded as key factors (Moran et al., 2011; He et al., 2013; Li et al., 2019). In fact, the application of biopesticides is beneficial for the promotion of public welfare, which has a certain “altruistic” nature. At the same time, the green transformation of the agricultural production mode is a public problem that requires the participation of a large number of farmers to achieve a substantive effect, i.e., it has a “collective action” nature (Xu et al., 2013). Therefore, it is necessary to consider the influence of social capital factors, such as the relationship among farmers, and the relationship between farmers and relevant organizations. As a key representation of social capital, the social network effectively improves the ability of individuals to obtain information, as well as decreases the cost of the adoption of new technologies, and thus affects the technology adoption decision of individuals (Isham et al., 2002). This effect is often more pronounced in the rural areas of China, which show obvious characteristics of a “nepotist society”. Also, farmers’ adoption of biopesticides is closely related to their production purposes. With increasing attention to food safety, with their dual identity of producer and consumer, farmers are increasingly inclined to adopt different production methods for the agricultural products they eat and for those they sell (Xu et al., 2013). Previous studies have shown that, compared with the “production for market” mode, when farmers produce for their own consumption (i.e., “production for life”), farmers tend to have a stronger willingness to apply biopesticides (Lu and Hu, 2015; Huang and Luo, 2018).
Therefore, the question is, under the background of different production purposes, what effect does the social network have on farmers’ adoption of biopesticides? How does the internal mechanism function? How can the positive impact of social networks be effectively used to encourage farmers to use biopesticides? The answers to these questions not only expand the theoretical explanation of economic preferences in neoclassical economics, but also deepens the understanding of social network internalization and enhances the understanding of how the “economic rationality” affects decision-making.
At present, scholars have conducted considerable and creative research on farmers’ willingness to adopt and behavior of green production in China as well as other countries, the main differences are the choice of variables and the processing of data. Fufa et al. (2016) used the random panel probit model to analyze the influencing factors of farmers’ green production from the aspects of their education level, farm scale, number of plots, and distance from homestead. Lee (2017) suggested that guidance system conditions and cognitive concepts are very important for farmers to participate in the production of agricultural green vegetables. Shiferaw and Holden (2015) indicated that the control effect on farmers’ green production behavior is limited by completely relying on policy guidance. However, most of the existing research, which focused on farmers’ behavior, discussed effective measures to encourage farmers’ green production on the basis of behavioral characteristics and the willingness of farmers to participate, while the impact of external factors such as social network and policy background has been less addressed. In fact, choices of actors are typically affected by changes of the external environment. Several researchers have noticed and investigated the relationship between social network and farmers’ behavior. Based on a comprehensive analysis of the existing research, two directions are worthy of further investigation: First, the available literature mainly focused on the direct impact of social networks on farmers’ behavior, while little consideration has been given to differences in production purposes of farmers. Second, further discussion on the impact mechanism was rarely reported. It is important to test the relationship between social network and farmers’ green production behavior; however, revealing the internal mechanism with which the social network influences different types of farmers forms the basis for the exploration of the most effective path for the green transformation of the agricultural production mode.
With this background and based on 1202 survey samples from Hubei Province, the main rice producing area of China, this paper empirically studies the impact of the social network on rice farmers’ biopesticide application behavior. The differences in rice farmers’ production purposes are fully considered, and an intermediary effect model is built to explore the causes and mechanisms underlying the influence of rice farmers’ behavior differences with regard to biopesticide application. This study aims to further expand research on the differences of Chinese farmers’ green production behavior, and provide reliable theoretical and empirical evidence for the formulation of effective and relevant policies and measures proposed by the Chinese government with the goal to encourage rice farmers to adopt green production.
In the context of this study, the application of biopesticides is essentially an agricultural management behavior, driven by the motivation of rice farmers to maximize their own utility (Schultz, 1964). As “rational economic people”, rice farmers always adopt the mode that yields the highest utility. Compared with traditional pesticides, biological pesticides offer the advantages of environmental friendliness, less resistance, and long-lasting effects. All of these advantages can effectively improve the quality and efficiency of agricultural production, and thus also enhance the income expectations of farmers. However, this benefit also has the characteristics of a long return period and may be difficult to detect (Xu and Yao, 2016). Therefore, the analysis of farmers’ behavior cannot be limited to a “cost-benefit” analysis in neoclassical economics. First, farmers are bounded rational. Because of the limitation imposed by insufficient knowledge structure and information, farmers cannot form an accurate understanding and evaluation of a new production mode within a short period of time. The “rational choice” about whether to adopt this mode will be promoted via “learning” (Hodgson, 2012). Second, individual farmers are “heterogeneous”. In the equilibrium analysis employed by neoclassical economics, the assumption of “homogeneity” is typically made for individuals. In fact, in production, farmers frequently interact in the local geographical space, which forms a certain relationship network. Different farmers have different positions in this network, and the heterogeneity of individual farmers will also affect their behavior. Third, the innovation of the production mode has the characteristics of “complex” and “dynamic”. Changing minor conditions may have unpredictable impacts on the future, which will inevitably impose a greater impact on farmers’ adoption of a new production mode. As a key representation of social capital, based on the social relationships among individuals, the social network constitutes a relatively stable system with high density and short communication paths, which can provide an effective guarantee for individual behavior (Wellman, 1988). The social network can provide three advantages for individual farmers: resource advantage, knowledge advantage, and confidence advantage, all of which can promote the application of biopesticides.
Specifically, a good and wide social network can improve the ability of individual farmers to access resources. Individual farmers can become acquainted and even intimate through social networks, and may gradually apply a mutual trust mechanism with their families, friends, and neighbors, thus reducing the transaction cost of economic activities. Farmers with large social networks are more likely to access resources, including capital, materials, and human resources, which is conducive to the transformation of the production mode. Second, social networks can promote the diffusion of knowledge or information. Communication and learning based on social networks can effectively reduce information asymmetry (Besley, 1993). Through social networks, farmers can exchange their experience of technology adoption, which can enrich both technical knowledge and information, further improve the behavioral efficacy of information diffusion, and expand the “bounded rationality” of individual farmers (Oriana and Imran, 2006). The learning and driving effects that are active in social networks can significantly stimulate the adoption of biopesticides (Hodgson, 2012). Third, social networks can improve farmers’ confidence in biopesticide application. A social network is constructed by various social connections among individuals. Individuals perceive norms within social groups through the behavior of other closely related individuals (Zhu, 2016). Leading farmers provide an example for other farmers to follow, and followers are more likely to imitate their behavioral choices. Under the influence of group norms, farmers within the same social network have more confidence in their behavioral choices and are therefore more likely to adopt new production methods.
Based on the above inference, this paper introduces research hypothesis 1:
Hypothesis 1: The social network has a positive impact on rice farmers’ application of biopesticides.
In addition to the direct impact, social networks may also affect farmers’ adoption of biopesticides indirectly through a series of intermediary mechanisms. In general, the farmer’s choice of a certain economic behavior depends on two aspects: The first aspect is whether he has a positive attitude toward this behavior, expressed as “Do we want to do it?”. The second aspect is the ability to take relevant actions, expressed as “Can we do it?”. The theory of behavioral economics emphasizes that the behavioral decision-making of the actor includes subjective initiative, and has the space of choice, internalization, and implementation of his economic behavior (Zheng et al., 2017). The expansion of the social network needs to stimulate farmers’ subjective initiative, and internalize it into a positive attitude and behavioral efficacy, to further affect their green production behavior. This paper explains the relationship between social network and farmers’ green production behavior from two aspects: behavioral attitude and behavioral efficacy.
First, the social network enhances farmers’ behavioral attitude by influencing their value perception. On the one hand, social networks affect the value perception of the actor, which is conducive to the understanding of biopesticides, the acceptance of their benefits, and their ease of use (Foster and Rosenzweig, 1995). Farmers with rich social network resources can obtain more technical information and have more opportunities for interchange, and can thus expand their originally limited understanding (Oriana and Imran, 2006). Under the influence of the social network, the behavioral subject is more likely to perceive the benefits of biopesticide application, then internalize its value, and form a beneficial value orientation and stable individual behavior. On the other hand, through their social network, farmers can also observe the successful experiences of other members of the network and rely on the “learning by watching”. Farmers who have achieved good results will enhance their expectations of the benefits of biopesticide application, and then become their own motivation to continue to apply biopesticides.
Second, the social network enhances the behavioral efficacy by improving the ability of resource acquisition. It has been shown that the ability of farmers to access resources is closely linked to their decision-making on production behavior (Bradford et al., 2004). The expansion of their social network can effectively improve their ability to access capital, technology, information, and other necessary resources. Through a gradually established mutual benefit and mutual trust mechanism, the transaction cost in economic activities is lowered. This is helpful for farmers to obtain the information, funds, and other resources needed to change their applied production mode, and thus to enhance the ability of farmers to apply biopesticides. In addition, their social network can effectively reduce the time cost farmers have to exert to obtain technical information. The cost of time and energy generated by farmers to learn and apply new production methods can be reduced by the spread of technical information by “model households” within social networks (Rogers, 1995). This is not only conducive for farmers to obtain more production resources, but also effectively enhances farmers’ long-term participation in the application of biopesticides. Therefore, this paper proposes the following hypothesis:
Hypothesis 2: The social network positively affects rice farmers’ green production behavior by improving their behavioral attitude and efficacy. The value perception and resource acquisition ability of rice farmers form an important internal transmission mechanism with which social networks promote biopesticide application.
According to their different production purposes, farmers can be divided into “ration-making farmers” (whose main purpose is to meet the demand for rations), and “profit-making farmers” (whose main goal is to maximize their profit) (Huang and Luo 2018). The product commercialization rate of “ration-making” farmers is relatively low, and their focal point is food safety; however, “profit-making” farmers have a higher rate of product commercialization, and they are more focused on the market profit of their products. Because of the differences in the utility goals of these two types of farmers, their behavioral decision-making toward applying biological pesticides also differs. This provides the possibility to explore the effect of the social network on farmers’ adoption of biopesticides through different intermediaries produced by different production purposes.
The positive behavioral attitude exerts different degrees of direct impact on these two types of farmers. The products of “ration-making” farmers are mainly for their own consumption, and the information is completely symmetrical. The pursuit of food safety is directly reflected in the application of biopesticides. Even if “ration-making” farmers do not have a positive behavioral attitude, the probability of their passive application of biopesticides is still high under the rigid impetus of food safety pressure (Bell et al., 2016). On the contrary, “profit-making” farmers, who are highly dependent on the market, use the market principle of high quality and good price as the main basis for their decision-making on whether to apply biopesticides. Their aim is the pursuit of profit maximization. In market transactions, information asymmetry is widespread. Whether “profit-making” farmers use biopesticides is more affected by (and sensitive to) their attitudes. This shows that their positive attitude has a relatively small impact on “ration-making” farmers, but has a stronger impact on “profit-making” farmers.
The behavioral efficacy also exerts different degrees of direct impact on these two types of farmers. Generally, the production scale of “ration-making” farmers is smaller; their products are mainly used to meet their own consumption needs. Therefore, relatively little consideration is given to output and revenue. Thus, the demand for capital, market information, and other resources is relatively low. In contrast, “profit-making” farmers, with their main goal to obtain the maximum profit, usually have a larger production scale and require a keen grasp of market information. They also have a relatively higher demand for various resources in the production. A broad social network can help farmers to obtain key resources and increase the probability that “profit-making” farmers conduct green production. Compared with “ration-making” farmers, behavioral efficacy exerts a stronger impact on “profit-making” farmers. In conclusion, the following assumptions are proposed:
Hypothesis 3: Behavioral attitude and behavioral efficacy impose a stronger positive impact on biopesticide application for “profit-making” farmers than for “ration-making” farmers; compared with “ration-making” farmers, behavioral attitude and behavioral efficacy have a stronger intermediary role in promoting the application of biopesticides by “profit-making” farmers.
Figure 1 shows the intermediary effect model proposed in this paper, including the direct effect (Hypothesis 1), intermediary effect (Hypothesis 2), and production purpose difference (Hypothesis 3). Moreover, this paper also controls for the possible influence of other related factors.
The data used in this paper originates from data of a household survey conducted by this research group in Hubei Province in 2018. Hubei Province is the main food-producing province in China. The west, north, and east of Hubei are surrounded by mountains as well as their foothills. The Jianghan plain in the south-central part is connected with the Dongting Lake in Hunan Province. According to the topographic characteristics and agricultural economic development level of Hubei Province, the four cities of Wuhan, Jingzhou, Suizhou, and Enshi were selected as research areas. In terms of topography, Wuhan belongs to the hilly terrain of Eastern Hubei, Jingzhou is located on the Jianghan Plain, Suizhou belongs to the hilly terrain of Northern Hubei, and Enshi belongs to the mountainous terrain of Western Hubei. In terms of their economic development, Wuhan and Jingzhou are better developed than the other two cities, and their proportion of agricultural output value exceeds 10%. Suizhou and Enshi have medium and poor agricultural economic development, respectively.
According to the principle of stratified sampling, two to four towns were selected from each city, and two to four villages were selected from each town. A total of 1296 questionnaires were collected from 36 villages in 12 towns. The investigators were mainly graduate students of agricultural colleges and universities. Before the start of the survey, they have been trained for about a week. The actual survey was conducted as a “one-to-one” interview. The specific content of the questionnaire includes three parts: 1) basic information of rice farmers (i.e., individual characteristics, family income and expenditure, agricultural operation status, etc.); 2) rice farmers’ current adoption of biopesticides; 3) value cognition of agricultural green production. The research object was limited to rice farmers in this paper. The reasons are as follows: 1) rice is a commonly planted crop in the survey area, which will improve the universality of the survey object without targeting specific farmers; 2) pesticides need to be applied multiple times at different stages of rice culture. The market for biopesticides suitable for rice is relatively mature, and rice farmers can easily choose pesticide types. Therefore, research based on the willingness of rice-growing farmers to apply biopesticides has a high representativeness. After screening and sorting, 1202 effective samples were obtained, and the effective recovery rate was 92.75%.
To set the explained variable “whether to apply biopesticide”, this paper uses the logistic regression model to estimate the impact path of the social network on biopesticide application (as shown in Figure 1). Then, the intermediary effect was tested according to the step-by-step regression method of Baron and Kenny (Baron and Kenny, 1986). Furthermore, using the bootstrap method, the marginal probability product statistic was constructed to test the significance of the intermediary effect, and to enhance the robustness of the test results (Besley, 1993). In the estimation of each part, this paper always takes the individual characteristics and family management characteristics of respondents as control variables, and dummy variables are added to control for the influence of resource endowment conditions. The specific treatment is as follows:
According to Hypothesis 1, firstly, the impact of the social network on biopesticide application was investigated. The binary selection model of “whether to use biological pesticide” is defined as follows:
where, Y represents the application of biopesticide, X represents the social network variable, K represents the control variable, β and γ are the coefficients to be estimated, and ε is the random disturbance term.
The social network affects rice farmers’ application of biopesticides through two mechanisms: behavioral attitude and behavioral efficacy. First, the logistic model 1) was used to test whether the main effect of the social network on the application of biopesticide is significant; second, the impact of the social network on mediators was estimated. Therefore, the following binary selection model is set:
where, M is the intermediary variable, and the interpretation of other variables is the same as in model (1). If β1 is significant, M is a reasonable intermediate variable. The third step is to estimate the effect of both the social network and mediators on the application of biopesticides. The model is as follows:
The interpretation of variables is the same as that of models (1) and (2). In the fourth step, the bootstrap method was used to test the intermediary effect, thus enhancing the robustness of the research results.
1) Rice farmers’ application of biopesticides. Biopesticides have the characteristics of high selectivity, low pollution, and low resistance (Qiu, 2015). In the questionnaire, the respondents answered “Have you ever used biopesticides in grain production?” and the binary selection variable y is defined as, Yes = 1 for applying biopesticides; No = 0 for not applying biopesticides.
2) Social networks. In reference to relevant research (Magnan et al., 2015) and the available data, the mobile communication cost was chosen as a proxy variable of the social network. The underlying assumption was that a higher cost of mobile communication indicates a closer relationship between rice farmers and other members of the social network, and consequently, the more support they can obtain from the social network. In the questionnaire, the respondents answered “How much is your monthly average mobile communication cost?” (unit: RMB yuan).
3) Production purpose. The commercialization rate of rice reflects the different production purposes of rice farmers. In the questionnaire, the answer “the proportion of rice you sell to the total output of the year” (%) is an indication of the commercialization rate. Rice farmers whose proportion is less than 50% were defined as “ration-making” farmers, otherwise, they were defined as “profit-making” farmers.
4) Mediating variables: Behavioral attitude and behavioral efficacy.
Firstly, the behavioral attitude of green production is measured by the rice farmers’ value perception of biopesticide application. The goal of rice farmers’ behavioral decision-making is to maximize utility. In the questionnaire, “Do you think the application of biopesticide is conducive to improving the quality of agricultural products” (Yes = 1; No = 0) was set to reflect the respondents’ behavioral attitude of applying biopesticides M1.
Secondly, the behavioral ability of biopesticide application is measured by the ability of rice farmers to obtain resources. Knowledge and skills are important resources when considering biopesticide application. In the questionnaire, “Do you have the skills, knowledge and experience required for the application of biopesticide” (Yes = 1; No = 0) was set to reflect the interviewee’s behavioral efficacy M2.
5) Control variables: Based on an analysis of reality and related literature, this paper selects a group of indicators as control variables, specifically including:
① Family agricultural management characteristics. Variables such as labor force structure, cultivated land area, and management mode have been proved to exert an important impact on the decision-making of rice farmers’ production behavior (Foster and Rosenzweig, 1995). Therefore, the variables selected in this paper include: the quantity of the agricultural labor force K1 (unit: person), the mode of agricultural operation K2 (purchasing productive service = 1, not purchasing productive service = 0), and the cultivated land area K3 (unit: hm2).
② Individual characteristics. It has been confirmed that age, education, and personal experience of rice farmers are important factors that affect their behavioral decision-making (Fufa et al., 2016). Therefore, the individual characteristic variables selected in this paper include: age K4 (unit: year), education K5 (unit: year), and personal experience K6 (assuming village cadres = 1, not assuming village cadres = 0).
③ Regional control variables. To distinguish external influences such as natural conditions, economic level, policy differences, crop varieties, and crop systems, this paper introduces regional virtual variables to control the influence of regional differences.
Supplementary Table S1 shows that the average age of respondents is 59.35 years, and respondents have an average education period of 8.36 years 11.15% of rice farmers have experience of village cadres, which reflects that the main body of agricultural management is affected by serious aging and their level of education is low. In terms of agricultural operation, the operating area is about 1.17 hm2, and the number of family members working as agricultural labor force is mainly 1-2. Most of the rice farmers have experience of purchasing production services (71.21%), with an average commercialization rate of 65.17%. This reflects the small scale of agricultural operation and agricultural labor force in the survey area, and diversified operation modes. Among the samples, 343 households apply biopesticides, while 859 households do not apply biopesticides. The average monthly mobile communication cost of the respondents is RMB 47.45 yuan. 55.74% of the respondents believe that pesticide application is conducive to improving the quality of agricultural products. 45.51% of the respondents have the skills, knowledge, and experience required for the application of biopesticides.
To better understand the current characteristics of rice farmers’ biopesticides application behaviors in response to different social network intensities and production purposes, this paper divides the samples. A cross contingency table analysis is conducted (Supplementary Table S2) based on whether the mobile communication cost exceeds the sample average (RMB 47.45 yuan) and whether the rice commercialization rate (R) exceeds 50%. From the perspective of horizontal comparison, with the enhancement of the social network, the proportion of “ration-making” farmers and “profit-making” farmers who use biopesticides increased from 22.05 to 19.65%–39.08 and 35.28%, respectively.
According to a vertical comparison, independent of how strong the social network is, the proportion of rice farmers whose rice commercialization rate exceeds 50% and who apply biological pesticides is lower than that of rice farmers whose commercialization rate is less than 50%. This shows that compared with “profit-making” farmers, “ration-making” farmers have a stronger willingness to apply biological pesticides, which is consistent with existing research results (Huang and Luo 2018; Huang, Zhong and Wang, 2016).
First, the multicollinearity test was conducted. According to the results of the single factor method, the total variance explained by the first principal component factor is only 17.23%. Moreover, the fitting results of each index are poor, indicating that the problem of homologous deviation of the sample data is not serious. The value of variance inflation factor (VIF) among the variables does not exceed 2.45, indicating that the model test results are less likely to be affected by multicollinearity.
Supplementary Table S3 shows the estimation results without mediators. To avoid possible influences of heteroscedasticity and outliers, the robustness test has been conducted for all regression models in this study. The statistical results show that the Wald values of each regression model are large, and statistically significant at the 1% level. This indicates that the model has a high degree of fitting, and that the parameter estimation results are relatively stable.
Supplementary Table S3 also shows that the estimation coefficient of the social network variables is positive and statistically significant at the 1% level, thus verifying Hypothesis 1. According to the estimation results of control variables, the number of labor force, operation mode, and operational scale exert statistically significant positive effects on rice farmers’ biopesticide application. This indicates that the larger the agricultural labor force, the more diversified the operation mode, and the larger the operation scale, the higher the willingness of farmers to apply biopesticides for production. The negative effect of age on biopesticide application behavior indicates that the tendency of biopesticide application weakens with increasing age of rice farmers. The coefficients of the education level and experience of village cadres are all positive, but they are not statistically significant, which shows that farmers with high education level or experience of cadres are not showing a stronger willingness to use biopesticides.
Supplementary Table S3 report the estimation results after adding mediators one by one. The social network and the mediators all passed the statistical test at the 1% level, indicating that the conclusion that the social network exerts a positive effect on rice farmers’ biopesticide application behavior is still supported. After adding the mediators, the coefficient of social network variables becomes larger and the significance is enhanced. This shows that the mediators strengthen part of the impact of the social network on the application of biopesticides, which is in line with the previous inference.
The test results show that the two mediators of behavioral attitude and behavioral efficacy in biopesticide application play a positive role in the effect the social network has on rice farmers’ biopesticide application behaviors, and Hypothesis 2 is also tested. In control variables, the mode of operation still significantly affects the application of biopesticides after adding mediators; however, the significance is weakened. The number of labor force, the scale of operation, and the age factors are no longer significant, while the significant strength of education and the experience of village cadres remained unchanged.
Supplementary Table S4 shows the binary logistic estimation model of the influence social networks exert on mediators. As shown in Supplementary Table S4, the coefficient estimates of the social network on rice farmers’ value perception and resource acquisition ability are significantly positive. This indicates that the larger social network can significantly improve rice farmers’ behavioral attitudes and abilities to use biopesticides, and again verifies Hypothesis 2.
Based on these results, the regression coefficient of the impact of social networks on rice farmers’ behavioral attitudes and behavioral efficacy is significant at the 1% level. According to the principle of testing in turn, according to the intermediary effect model (Bradford et al., 2004), social networks affect the application of biopesticides through two mediators. Rice farmers’ attitude and ability exert a significant mediating role in the impact of social networks on the application of biopesticides, which verifies Hypothesis 2.
To enhance the robustness of the intermediary effect test, this study uses the method described above to calculate the intermediary effect, and the results are shown in Supplementary Table S5. Consistent with the step-by-step test results, the bootstrap test results also showed that the intermediary effects of behavioral attitude and behavioral efficacy were both significant at the 1% level, further verifying Hypothesis 2. From the perspective of horizontal comparison, this effect shows that the intermediary effect of green production capacity is stronger than the behavioral attitude. This indicates that the enhancement of the capacity induced by the expansion of the social network can effectively motivate rice farmers to apply biopesticide. This is also in line with the current situation that rice farmers’ adoption of new technologies is often limited by their capacity of technology use.
Supplementary Table S6 shows the results of multiple logistic estimates for the biopesticide application of both types of farmers when the mediators are not entered into the model. Social networks exert a significant positive impact on both ration-making and profit-making farmers at the 1% level, and the Wald test rejects the original hypothesis at the 1% significance level. This shows that the total impact of social networks on the two types of farmers is positive and statistically significant. This forms the premise for the test of the intermediary effect.
From the coefficient estimation of the control variables, the effect of age on both types of farmers’ biopesticide application behaviors is negative, which is consistent with the above conclusions. The coefficients of management mode and management scale are significantly positive in the biopesticide application behavior of profit-making farmers, which indicates that profit-making farmers with multiple management modes and large management scale use more biopesticides. The most obvious difference is that the variable of the education level has a significant negative impact on ration-making farmers’ biopesticide application behavior, while it has a significant positive impact on profit-making farmers. This indicates that the improvement of the education level plays an important role in promoting the use of biopesticides by profit-making farmers. Accordingly, the main groups that apply biopesticides can be identified as: (for ration-making farmers) young and middle-aged farmers, farmers with low education background, and farmers with small scale of cultivated land; (for profit-making farmers) young farmers, highly educated farmers, and farmers with diversified and large-scale cultivated land.
Supplementary Table S6 lists the estimation results of multiple logistic models after addition of intermediary variables. The social network has a significant positive impact on biopesticide application, but the degree of impact is weak. The results of bootstrap testing are consistent with the step-by-step test, indicating that Hypothesis 3 is verified.
Furthermore, the strength of the mediation effect was analyzed. First, chi square tests were conducted for both types of intermediary effects of “ration-making” farmers and “profit-making” farmers. If the difference between the two types of farmers’ intermediary variable coefficients is significantly different from zero, this indicates that the intermediary effect is different in the two types of farmers. Supplementary Table S7 shows that the difference of coefficients between the two types of farmers is significantly greater than zero at the 1% level, and the difference of intermediary effect is also significantly different. Moreover, the overall roles of intermediary variables were tested in both categories of farmers. The Chi square test statistic value with coefficient difference of zero is 27.38, which is also significant at the 1% level. This indicates that the intermediary effect of behavioral attitude and behavioral efficacy among “profit-making” farmers is stronger than that of “ration-making” farmers, indicating that Hypothesis 3 is fully verified.
At the theoretical level, this paper explains differences in the decision-making with regard to biopesticide application caused by social networks. Moreover, it presents an analysis of the behavioral differences among different types of farmers from the perspectives of social preference and production purpose. This enriches the theoretical explanation of the “rational man” in neoclassical economics to some extent. On this basis, this paper further verifies that there are two ways to transfer the impact of social networks on rice farmers’ biopesticide application, i.e., to let potential participants perceive the feasibility and desirability of the application of biopesticides. This deepens the understanding of social network internalization, and also enhances the understanding of the influence of “economic rationality” on individual behavioral decision-making.
This paper explores the impact of social networks on the biopesticide application of rice farmers. Based on the perspective of a differentiation between farmer types and the different production purposes, this paper presents an in-depth analysis of the effects and mechanisms of social networks on rice farmers’ biopesticide application. This analysis is based on 1202 sample survey data from Hubei Province, China. The main conclusions are as follows:
Social networks exert a significant positive impact on the use of biopesticides; after controlling factors such as management characteristics, individual characteristics, time, and regional resource endowment, social networks still significantly impact the use of biopesticides. Factors such as the number of labor force, operation mode, and scale of operation exert positive and significant effects on biopesticide application. The effect of age is significantly negative, and the effects of education level and village cadre experience are not obvious. Therefore, the impact path of social networks on rice farmers’ biopesticide application should be clarified. The influence of social networks on rice farmers’ biopesticide application can be strengthened by the expansion of information channels, the improvement of the means of strengthening and benefiting agriculture, and the improvement of the ability to acquire resources.
The internal mechanism is reflected by the further effect of social networks on rice farmers’ biopesticide application behaviors through both their behavioral attitude and behavioral efficacy. The behavioral attitude and behavioral efficacy of potential biopesticide users play important mediating roles in the influence social networks exert on biopesticide application. The results of the bootstrap test also support the conclusion that social networks can encourage rice farmers to use biopesticides by influencing both their attitude and ability. Among these, the intermediary effect of their behavioral ability is stronger than that of their behavioral attitude. Therefore, corresponding policies should be issued according to the status of rice farmers within a social network when encouraging rice farmers to use biopesticides or other green production behaviors. Exemplary households should be seen as the main direction, so that all farmers can benefit from their example as much as possible. Moreover, the farmers’ value cognition can be improved by expanding publicity and demonstration effect.
Compared with “profit-making” farmers, the biological pesticides application of “ration-making” farmers is stronger affected by their social network. Operation mode, operation scale and education level positively affect the pesticide application behavior of “profit-making” farmers, and the influence of age is significantly negative. Biopesticide application of “ration-making” farmers is negatively affected by age and education level and positively affected by the operation mode. The behavioral attitude and behavioral efficacy of green production exert significant mediating effects on both types of farmers. Both intermediary variables have greater impacts on the “profit-making” farmers. Therefore, the impact of the production purpose on rice farmers’ green production decision-making should be fully considered. Better institutional arrangements should be made at the policy level according to individual differences, and social networks should be effectively used to promote the use of biopesticides, to achieve an overall improvement of the agricultural green development level.
The raw data supporting the conclusion of this article will be made available by the authors, without undue reservation.
All authors listed have made a substantial, direct, and intellectual contribution to the work and approved it for publication.
This work was supported by the National Natural Science Foundation of China (71803145, 71933004, and 71963005), and Youth Program for Social Science of Department of Education of Hubei Province(21Q026).
The authors declare that the research was conducted in the absence of any commercial or financial relationships that could be construed as a potential conflict of interest.
All claims expressed in this article are solely those of the authors and do not necessarily represent those of their affiliated organizations, or those of the publisher, the editors and the reviewers. Any product that may be evaluated in this article, or claim that may be made by its manufacturer, is not guaranteed or endorsed by the publisher.
The Supplementary Material for this article can be found online at: https://www.frontiersin.org/articles/10.3389/fenvs.2022.834760/full#supplementary-material
Abdollahzadeh, G., Sharifzadeh, M. S., and Damalas, C. A. (2015). Perceptions of the Beneficial and Harmful Effects of Pesticides Among Iranian Rice Farmers Influence the Adoption of Biological Control. Crop Prot. 75, 124–131. doi:10.1016/j.cropro.2015.05.018
Acemoglu, D. (2008). Introduction to Modern Economic Growth. New Jersey: Princeton University Press.
Baron, R. M., and Kenny, D. A. (1986). The Moderator-Mediator Variable Distinction in Social Psychological Research: Conceptual, Strategic, and Statistical Considerations. J. Personal. Soc. Psychol. 51 (6), 1173–1182. doi:10.1037/0022-3514.51.6.1173
Bell, A., Zhang, W., and Nou, K. (2016). Pesticide Use and Cooperative Management of Natural Enemy Habitat in a Framed Field experiment. Agric. Syst. 143, 1–13. doi:10.1016/j.agsy.2015.11.012
Besley, T. (1993). Property Rights and Investment Incentives: Theory and Micro-evidence from Ghana. J. Polit. Economy 103 (5), 903–937. doi:10.2307/2234340
Bradford, L., BarhamJeremy, D., Foltz, D., Jackson-Smith, S., and Moon, (2004). The Dynamics of Agricultural Biotechnology Adoption: Lessons from Series rBST Use in Wisconsin, 1994–2001. Am. J. Agric. Econ. 86 (1), 61–72.
Foster, A. D., and Rosenzweig, M. R. (1995). Learning by Doing and Learning from Others: Human Capital and Technical Change in Agriculture. J. Polit. Economy 103 (6), 1176–1209. doi:10.1086/601447
He, Ke., Zhang, Junbiao., and Tian, Yun. (2013). Influencing Factors and Differences in Farmer Willingness to Pay for Ecological Compensation of Agricultural Waste Utilization:Based on Rural Areas of Hubei. Resour. Sci. 35 (3), 627–637.
Hokkanen, M. T., and Hajek, A. (2003). Environmental Impacts of Microbial Insecticides. Kluwer: Academic Publishers.
Huang, Y., and Luo, X. (2018). Both to Eat and Sell": An Analysis of Biological Pesticides Application Behaviours of Different Rice Farmers. Chin. Rural Economy 7, 63–78.
Huang, Z., Zhong, Y., and Wang, X. (2016). Study on the Impacts of Government Policy on Farmers' Pesticide Application Behavior. China Popul. Resour. Environ. 26 (8), 148–155.
Isham, J., Kähkönen, S., and Satu, (2002). Institutional Determinants of the Impact ofCommunity‐Based Water Services:Evidence from Sri Lanka and India. Econ. Develop. Cult. Change 50 (3), 667–691. doi:10.1086/342357
Li, Z., Luo, X., and Qiu, W. (2019). Land Scale, Tenure Security and Adoption of Organic Fertilizer of Farmer: A Mediation and Moderated Model. esources Environ. Yangtze Basin 28 (8), 1918–1928.
Lu, H., and Hu, H. (2015). Analysis of the Impact of Land Fragmentation and Plant Diversification on Agricultural Production Profit and Efficiency. J. Agrotechnical Econ. 7, 4–15.
Magnan, N., Spielman, D. J., Gulati, K., and Lybbert, T. J. (2015). Information Networks Among Women and Men and the Demand for an Agricultural Technology in India. Social Science Electronic Publishing.
Moran, D., Macleod, M., Wall, E., Eory, V., Mcvittie, A., Barnes, A., et al. (2011). Marginal Abatement Cost Curves for UK Agricultural Greenhouse Gas Emissions. J. Agric. Econ. 62 (1), 93–118. doi:10.1111/j.1477-9552.2010.00268.x
Oriana, B., and Imran, R. (2006). Social Networks and Technology Adoption in Northern Mozambique. Econ. J. 116 (514), 869–902.
Qiu, D. (2015). Analysis of the Development Situation and Trends of Biological Pesticides in China. Chin. J. Biol. Control. 31 (5), 679–684.
Rogers, E. M. (1995). Diffusion of Innovations. 4th enlarged edition4th. New York: Free PressFree Press.
Wellman, B. (1988). The Healing Web: Social Networks and Human Survival.Marc Pilisuk , Susan Hillier ParksThe Healing Web: Social Networks and Human Survival.by Marc Pilisuk; Susan Hillier Parks. Am. J. Sociol. 93 (4), 1006–1008. doi:10.1086/228852
Xie, W., Chen, T., and Liu, G. (2018). Analysis on the Adoption Differences of Farmers' Cultivated Land Quality Protection Technology under the Background of Rural Revitalization. Probe 11, 117–129.
Xu, L., Zhou, L., and Pan, S. (2013). One Family, Two Ways”: Social Self-Production under the Threat of Food Safety. Chin. Rural Economy 5, 32–44.
Zhao, D. (2012). Research on the Dynamic Mechanism and Institutional Change of Green Agriculture Development in China. Issues Agric. Economy 11, 72–79.
Zheng, H., Zhou, X., and Zhang, L. (2017). Why Does College Education Matter? Unveiling the Contributions of Selection Factors. Soc. Sci. Res. 68 (11), 59–73. doi:10.1016/j.ssresearch.2017.09.003
Keywords: social network, production purpose, biological pesticide application, intermediary effect, China
Citation: Li Z, Zhu M and Zuo Q (2022) Social Network, Production Purpose, and Biological Pesticide Application Behavior of Rice Farmers. Front. Environ. Sci. 10:834760. doi: 10.3389/fenvs.2022.834760
Received: 15 December 2021; Accepted: 21 March 2022;
Published: 12 April 2022.
Edited by:
Anna Maria Jönsson, Södertörn University, SwedenReviewed by:
Luigi Aldieri, University of Salerno, ItalyCopyright © 2022 Li, Zhu and Zuo. This is an open-access article distributed under the terms of the Creative Commons Attribution License (CC BY). The use, distribution or reproduction in other forums is permitted, provided the original author(s) and the copyright owner(s) are credited and that the original publication in this journal is cited, in accordance with accepted academic practice. No use, distribution or reproduction is permitted which does not comply with these terms.
*Correspondence: Mande Zhu, bWR6aHVAZ3p1LmVkdS5jbg==
†These authors have contributed equally to this work
Disclaimer: All claims expressed in this article are solely those of the authors and do not necessarily represent those of their affiliated organizations, or those of the publisher, the editors and the reviewers. Any product that may be evaluated in this article or claim that may be made by its manufacturer is not guaranteed or endorsed by the publisher.
Research integrity at Frontiers
Learn more about the work of our research integrity team to safeguard the quality of each article we publish.