- 1Guangzhou Institute of International Finance, Guangzhou University, Guangzhou, China
- 2School of Economics and Statistics, Guangzhou University, Guangzhou, China
The spatial relationship between high-tech industrial agglomeration and urban ecological efficiency has been a widespread concern. This study empirically analyzed the bidirectional influence relationship between high-tech industrial agglomeration and urban ecological efficiency and its spatial spillover effect by constructing a spatial simultaneous equation model, and based on the classification of urban resource endowment, this paper studies the heterogeneity of spatial spillover effects between high-tech industrial agglomeration and urban ecological efficiency based on the sample data of 285 cities in China from 2004 to 2017. The empirical results reveal the following: first, there is a two-way promoting effect between high-tech industrial agglomeration and urban ecological efficiency; second, there is a significant spatial spillover effect of urban ecological efficiency, that is, the urban ecological efficiency in this region is significantly positively correlated with the urban ecological efficiency in neighboring regions; third, there is a significant difference in the regional interaction between high-tech industry agglomeration and urban eco-efficiency, that is, the urban eco-efficiency in the neighboring areas has a significant negative impact on the regional high-tech industry agglomeration, while the high-tech industry agglomeration in the neighboring areas has a negative but not significant impact on the regional urban eco-efficiency; fourth, the spatial interaction spillover effects between high-tech industrial agglomeration and urban ecological efficiency are heterogeneous in terms of regional resources, and the results of regional interaction and spatial spillover effects are better in the sample study.
1 Introduction
Eco-efficiency not only plays an important role in the global climate but is also the key to facilitating the transition from high-speed to high-quality economic development. However, high energy consumption, high emission, high pollution, and other extensive development modes have not been fundamentally changed, which has brought many environmental pollution and ecological damage problems. With the increasingly sharp contradiction between economic development and environmental resources, ecological efficiency problems have attracted wide attention from all directions. The coordinated development of economic development and environmental resources is an important prerequisite for the construction of ecological civilization. The essence of achieving high-quality economic development lies in technological progress. It is of great significance to promote the transformation of social development mode through high-tech development and replace the traditional high energy consumption and high input production mode.
Ecological efficiency emphasizes the coordinated development of the economy and environment and takes the minimum input of resources and environment consumption as the core to achieve the maximum economic and social benefits. Ecological efficiency mainly reflects the impact of resources and environment on economic activities, and ecological efficiency evaluations reflect the regional ecological status and the level of input and output, which is conducive to solving the problems of ecological environment governance. Existing literature mainly focuses on the research of ecological efficiency in micro-enterprises and industries (Dyckhoff and Allen, 2001), and studies on regional and urban ecological efficiency also have important value and practical significance (Hur et al., 2004; Seppala et al., 2005). Due to the differences in development modes and resource endowments, the development of ecological efficiency between different cities is not the same. Therefore, it is of great significance to analyze the differences in urban ecological efficiency among different regions.
Many scholars have researched the influencing factors of ecological efficiency to alleviate the downward pressure of the economy and environment. At present, the relevant literature on the influencing factors of ecological efficiency mainly focuses on the following aspects: first, the relationship between ecological efficiency and technological innovation. Liu et al. (2019) used the Boostrap regression model to analyze the influencing factors of urban ecological efficiency and found that technological progress was the main driving force for the improvement of urban ecological efficiency in Henan Province. Song et al. (2020b) found that pilot low-carbon city policies of China improve urban ecological efficiency through technological innovation paths. Technological progress improves energy efficiency and thus reduces emissions, which can make up for the loss of ecological efficiency to some extent, and also improves the dynamic ecological efficiency of urban agglomeration (Li T. et al., 2021b; Li Z. et al., 2021a). In addition, the public budgets for energy RD&D also affect air pollution levels, and the impact of technological innovation on eco-efficiency is significantly positive at both national and regional levels (Balsalobre et al., 2015; Yasmeen et al., 2020; Kassouri, 2022). The second is the relationship between ecological efficiency and industrial structure. Industrial structure has a positive effect on ecological efficiency, and the sustainable development of the ecosystem can be realized by transforming resource-intensive industries into technology-intensive industries (Zhao et al., 2020). Wang et al. (2020a) found that urban agglomeration development is conducive to improving regional ecological efficiency. With the improvement of the efficiency of urban agglomeration, the gap of eco-efficiency between cities continues to expand, and industrial structure and innovation intensity have a positive impact on eco-efficiency (Xu and Wang, 2020). The third is the relationship between ecological efficiency and behavioral consciousness. Zhang et al. (2020) consider that improving education levels can enhance people’s awareness of environmental protection and conservation, thus improving ecological efficiency. Population agglomeration and economic development have a significant negative impact on urban ecological efficiency, and the development of higher education and medical services significantly promote the improvement of urban ecological efficiency (Zhang et al., 2020; Shen et al., 2021; Si et al., 2021). Financial behavior has a heterogeneous influence on total factor green productivity and promotes coordinated development of the economy and environment (Liu et al., 2020; Li Z. et al., 2021b; Li Z. et al., 2021c). Financial development alleviates environmental pressure through innovation and technology introduction (Haas et al., 2021; Sardo and Serrasqueiro, 2021; Wang M. et al., 2021; Yao et al., 2021). Through literature review, it is found that scholars have studied the influencing factors of ecological efficiency from different perspectives, and technological progress is closely related to ecological efficiency. Technological progress reduces pollutant emission and energy consumption through optimizing industrial structures.
Ecological efficiency varies across different locations and timelines. Ecological efficiency and technological progress form a certain degree of spatial differentiation and agglomeration. There are some differences in resource endowment and environmental conditions in different regions, which leads to unbalanced economic development among regions, and also leads to the spatial diversity and difference of urban ecological efficiency among regions. There is a serious imbalance in ecological efficiency between regions, and ecological efficiency has an obvious spatial aggregation effect (Ma et al., 2018; Li, 2019; Shi and Huang, 2019). Wang Z. et al. (2021) empirically analyzed the spatial and temporal dynamic evolution of urban ecological efficiency and its driving factors in the Yellow River Basin and found that the overall spatial pattern of urban ecological efficiency in the middle reaches was higher than that in the upper and lower reaches. The spatial agglomeration of high-tech industries has an impact on urban ecological efficiency. The impact of industrial agglomeration on eco-efficiency is mainly reflected in two aspects. On the one hand, with its own scale effect, industrial agglomeration promotes the improvement of energy efficiency through knowledge spillover and high-tech diffusion, which is conducive to alleviating environmental pressure (Liu J. et al., 2017). On the other hand, industrial agglomeration is often accompanied by the expansion of city scale and the increase of population density, which leads to the increase in energy consumption and regional environmental pollution (Liu S. et al., 2017). In the past, the one-way impact of technological progress on ecological efficiency was mainly studied, and there was a lack of literature on the relationship between the two. Based on the above analysis, it is preliminarily predicted that there is a bidirectional influence between high-tech industrial agglomeration and urban ecological efficiency. However, ecological efficiency has spatio-temporal differentiation, and high-tech industries form agglomeration in space. It is of practical significance to study the spatial impact and spillover effect of high-tech industry agglomeration and urban ecological efficiency. The relationship between high-tech industry agglomeration and urban ecological efficiency should be empirically concluded through relevant models.
This paper focuses on the spatial spillover effects between high-tech industrial agglomeration and urban ecological efficiency. The marginal contribution is mainly as follows: First, the interaction between high-tech industrial agglomeration and urban ecological efficiency is studied. Through the empirical test, there is a two-way promoting effect between high-tech industrial agglomeration and urban ecological efficiency. Second, the spatial simultaneous equation model combined with the three-stage least square method is used to test the spillover effect between regions under the geographical distance space matrix and economic-geographical space weight matrix respectively. Third, considering the regional interaction between high-tech industrial agglomeration and urban ecological efficiency, the significant difference between them is studied. Fourth, all samples are divided into resource-based cities and non-resource-based cities according to different resource endowments, and the heterogeneity of spatial interaction spillover effect between high-tech industry agglomeration and urban eco-efficiency is studied.
The rest of this paper is structured as follows: the second part is the theoretical analysis part of the paper, and puts forward the research hypothesis. The third part sets up the research model and gives the calculation method of ecological efficiency. The fourth part tests the interaction between high-tech industrial agglomeration and urban ecological efficiency. The fifth part further discusses the resource heterogeneity of different cities and tests the stability of the results. The sixth part summarizes the research conclusions and gives some enlightenment.
2 Theoretical Analysis and Research Hypothesis
2.1 Two-Way Influence Mechanism of High-Tech Industrial Agglomeration and Urban Ecological Efficiency
The agglomeration effect and innovation effect of high-tech industry agglomeration have a certain influence on urban ecological efficiency. Zeng and Zhao (2009) found that manufacturing agglomeration mitigated the pollution effect, which passed the test of a spatial economic model in two countries and two sectors. Innovation promotes economic development, agglomeration is conducive to knowledge spillover, labor market sharing, and technology expansion. Industrial agglomeration reduces environmental pollution and improves energy efficiency through energy sharing. Yin and Guo (2021) consider that high-tech industrial agglomeration improves industrial productivity through scale effect and agglomeration effect, promotes technological progress, and optimizes industrial structure to protect the environment. As knowledge-intensive and technology-intensive industries, high-tech industries form clusters of talents and technologies with a high degree of agglomeration. The competition formed by agglomeration is the main source of innovation, which is beneficial to the enhancement of independent innovation ability within the region. High-tech industry agglomeration promotes urban ecological efficiency through the agglomeration effect and innovation effect.
Urban eco-efficiency also has an impact on high-tech industrial agglomeration. The improvement of urban ecological efficiency means that the input of resources and environmental factors has achieved greater social and economic benefits. The ecological industry is the key to realizing the green transformation, and the development of ecological industry is beneficial to realize the coordinated development of the economy, society, and environment. Song and Zhou (2021) found that the establishment of national eco-industrial parks promotes industrial agglomeration, and the use of ecological processes to improve energy efficiency and reduce pollution emissions has a long-term, though delayed, impact. The improvement of ecological efficiency promotes the development of a circular economy, which is conducive to the upgrading of industrial structure and sustainable development. Industrial agglomeration is a kind of economic growth model, the improvement of urban ecological efficiency can not only promote high-tech industrial agglomeration by realizing the sustainable development of the industry, but also promote the development of the circular economy of high-tech industry. Based on this, this paper puts forward the first hypothesis.
Hypothesis 1. There is a two-way promoting effect between high-tech industrial agglomeration and urban ecological efficiency.
2.2 Spatial Spillover Effects of High-Tech Industrial Agglomeration and Urban Ecological Efficiency
While agglomeration promotes the technological progress of the industrial chain within the region, its externality has a certain influence on industrial agglomeration in surrounding areas. There is a spatial spillover effect of urban ecological efficiency, that is, the agglomeration of high-tech industries in neighboring areas has an impact on the agglomeration of high-tech industries in the region. Yin and Guo (2021) found that high-tech industrial agglomeration promotes industrial agglomeration in adjacent areas by forming innovative knowledge networks and technology spillover. The agglomeration reflects the convenience of space. The agglomeration of high-tech industries plays a demonstration role to some extent through the spillover of knowledge and technology and promotes the agglomeration of high-tech industries between regions. At the same time, inter-regional industrial agglomeration has the risk of a crowding effect, which leads to the scramble for resources and the negative spatial spillover effect of industrial agglomeration through the “siphon effect.”
The development of urban ecological efficiency is uneven in space, and there are great differences in urban ecological efficiency. There is a spatial spillover effect of urban ecological efficiency, that is, the urban ecological efficiency in neighboring areas has an impact on the regional urban ecological efficiency. Chen et al. (2020) found that industrial agglomeration and ecological efficiency both have spatial spillover effects. The improvement of urban ecological efficiency aims to realize the coordinated development of the economy and environment. The development of ecological efficiency in neighboring cities improves the ecological efficiency of local cities through the demonstration effect. However, environmental regulations between regions lead to the transfer of polluting industries (D'Agostino, 2015; Wang S. et al., 2021). Due to resource consumption and waste gas emissions, the ecological efficiency of cities in neighboring regions hinders the improvement of the ecological efficiency of cities in the region, resulting in the phenomenon of “broken cans.” The spatial spillover effect of high-tech industrial agglomeration and urban ecological efficiency is affected by many factors and shows complexity. Therefore, this paper proposes the second hypothesis.
Hypothesis 2. Both high-tech industrial agglomeration and urban ecological efficiency have a spatial spillover effect.
2.3 Regional Interaction Between High-Tech Industrial Agglomeration and Urban Ecological Efficiency
High-tech industry agglomeration and urban ecological efficiency affect each other between regions. High-tech industry agglomeration in neighboring regions has an impact on the urban ecological efficiency of the region, while the urban ecological efficiency in neighboring regions has an impact on the local High-tech industry agglomeration. Yin and Guo (2021) used the spatial Dubin model to demonstrate the positive spatial correlation between high-tech industrial agglomeration and industrial environmental efficiency. Wang J. et al. (2019) found that technology spillover effectively reduces pollutant emission intensity in neighboring areas using the spatial Dubin model, while agglomeration produces negative environmental effects on neighboring areas through social and spatial networks. On the one hand, the agglomeration of high-tech industries in neighboring areas promotes the improvement of urban ecological efficiency through technological diffusion and plays an exemplary role when technological innovation breakthroughs are achieved in neighboring areas. The local area can learn and imitate the development of local high-tech industries through information exchange, so as to improve urban ecological efficiency. On the other hand, in the process of developing high-tech industries, local cities actively introduce economic, technological, and human resources from neighboring cities. While promoting economic development, it brings serious environmental pollution, which is not conducive to the development of urban ecological efficiency. Urban ecological efficiency in neighboring areas promotes the development of a circular economy through pollution prevention and control and helps to promote the innovative development of high-tech industries. The demand for innovation drives the formation of cross-regional industrial agglomeration, and further enhances the clustering degree of high-tech industries in this region and neighboring regions. At the same time, ecological efficiency and environmental regulation complement each other, and environmental regulation has a direct driving influence on ecological efficiency (Song et al., 2020a), environmental regulation affects the regional environmental pollution level by promoting the trans-regional transfer of polluting industries (D'Agostino, 2015), the development of urban ecological efficiency in neighboring areas inhibits the agglomeration of high-tech industries in this region. Therefore, this paper proposes the third hypothesis.
Hypothesis 3. There is a regional interaction between high-tech industrial agglomeration and urban ecological efficiency.
2.4 Resource Heterogeneity of the Interaction Between High-Tech Industrial Agglomeration and Urban Ecological Efficiency
The agglomeration degree of high-tech industries and urban ecological efficiency is different due to the different modes of industrial production and development among regions, as well as the existence of factors such as different urban locations and resources. Heterogeneity analysis mainly studies the relationship between things from different perspectives and various aspects and studies the heterogeneous characteristics of influencing factors (Li T. et al., 2021a; Li and Ma, 2021). Yu et al. (2018) found that there was regional heterogeneity in ecological efficiency, so as to study the dynamic impact of industrial agglomeration on ecological efficiency. Chen et al. (2020) researched the urban heterogeneity of the impact of industrial agglomeration on ecological efficiency in eastern, central, and western China. The impact of industrial agglomeration on ecological efficiency shows obvious heterogeneity in the scale of cities, so as to effectively improve the coordination between economy and environment. Song et al. (2020b) studied the impact of low-carbon city construction on ecological efficiency in terms of urban heterogeneity. Ecological efficiency is resource-dependent, and cities with a strong dependence on resources are relatively low in ecological efficiency, while cities with less dependence on resources are relatively high in ecological efficiency. Considering the difference in urban resource endowment, cities are divided into resource-based cities and non-resource-based cities. The spatial spillover effect and regional interaction effect of high-tech industry agglomeration and urban ecological efficiency will show different results in different types of city samples. Therefore, this paper proposes the fourth hypothesis.
Hypothesis 4. Spatial spillover effect and regional interaction between high-tech industrial agglomeration and urban ecological efficiency are heterogeneous in terms of regional resources.
3 Research Model and Method Design
3.1 Spatial Simultaneous Equation Model
According to the above analysis, there is a spatial relationship between high-tech industrial agglomeration and urban ecological efficiency. Based on this, this paper adopts the spatial simultaneous equation model and the generalized three-stage spatial least square estimation method to empirically study the spatial spillover effect and regional interaction between high-tech industrial agglomeration and urban ecological efficiency, and to verify the hypothesis mentioned above. The spatial simultaneous equation model is as follows:
In Eqs 1, 2,
In Eq. 3,
3.2 Measurement of Urban Ecological Efficiency
The evaluation of ecological environment quality involves many aspects of research, including ecological footprint in addition to ecological efficiency (Kassouri, 2021). Ecological footprint mainly refers to the impact of human activities on the ecosystem, and its measurement indicators and data are huge and involve a wide range. In this paper, urban ecological efficiency is studied to reflect the quality of the ecological environment, and the relatively mature research method of energy efficiency is used to analyze ecological efficiency, that is, the total factor productivity method based on the Malmquist index. The change of ecological efficiency is divided into total factor productivity change and factor substitution change. The change of total factor productivity is equivalent to technological progress, and the replacement of factors depends more on the change of relative prices among factors, which intuitively reflects the impact on ecological efficiency.
Ecological efficiency emphasizes the coordinated development of the economy and resources, and it is the input-output efficiency that takes into account all kinds of resource inputs and pays attention to economic output as well as the undesired output of environmental pollution. This paper uses the DEA-Malmquist index method to measure urban ecological efficiency and take the environment into account as an undesired output. DEA-Malmquist index method not only considers labor, capital, and resource input, but also pays attention to expected output and non-expected output, which is the combination of data envelopment analysis method and Malmquist index method.
3.2.1 Date Envelopment Analysis
The data envelopment analysis method is a non-parametric estimation method to study multiple inputs and outputs. It mainly evaluates and observes the dynamic change of subject efficiency through a decision-making unit, which avoids the interference of subjective factors, so as to effectively evaluate the resource allocation efficiency (Shakouri et al., 2019). The BBC model is adopted in this paper and its expression is as follows:
The constraints are
In Eqs 4–6,
3.2.2 Malmquist Index Method
The Malmquist index, or total factor productivity (TFP). This index mainly studies the dynamic change process and analyzes the dynamic efficiency change of DMUs from
Eq. 9 is the expression of total factor productivity.
It can be seen from Eq. 10 that, assuming that return to scale remains unchanged, from
In Eq. 11, when returns to scale change, technical efficiency change (
4 Metrological Test of the Interaction Between High-Tech Industrial Agglomeration and Urban Ecological Efficiency
4.1 Variables and Data
Urban ecological efficiency, this paper adopts the DEA-Malmquist index method to measure urban ecological efficiency. Labor, capital, energy, and land are taken as input variables, and real GDP and emissions of “three wastes” are taken as expected output and unexpected output, respectively. Among them, labor input is measured by the number of employees in units of municipal districts at the end of the year; the perpetual inventory method is used to estimate the capital stock, according to the calculation formula:
High-tech industry agglomeration, this paper selects the employment density of high-tech industry to measure. According to the calculation formula:
This paper selects a series of control variables that affect high-tech industrial agglomeration and urban ecological efficiency. Economic development level, measured by per capita GDP of municipal districts, the city with better economic conditions is more conducive to the inflow of talents, which will improve the innovation level and facilitate the agglomeration of high-tech industries. With economic development, the increase of energy consumption and emission inevitably brings environmental pollution and affects ecological efficiency. Population density is measured by the number of people per square kilometer of the municipal district. An increasing population provides more labor and brings environmental pressure. Upgrade of industrial structure is measured by the proportion of the added value of the secondary and tertiary industries in GDP. The optimization and upgrading of industrial structure mean the reduction of traditional production mode, and the development of modern manufacturing and service industry promotes the improvement of urban ecological efficiency. Science and technology, expressed by the proportion of science and technology expenditure in general government expenditure, represents the impact of science and technology on high-tech industrial agglomeration and urban ecological efficiency. The high-tech industrial agglomeration and other control variables selected in this paper are shown in Table 2.
The data samples of this study are 285 cities above the prefecture-level in China from 2004 to 2017, and the time-frequency of the data is set as annual. Unfortunately, the “three wastes” emissions in the China city statistical yearbook data are incomplete. In other words, the data of industrial wastewater discharge, industrial sulfur dioxide discharge, and industrial soot discharge published by the China Urban Statistical Yearbook in the past 2 years are missing significant data. In order to ensure the reliability of the research results, the sample data of the study is finally up 2017. The data are mainly from the “China Urban Statistical Yearbook” and EPS database. The fixed asset investment data in 2017 are from the annual statistical bulletin of each city, and some missing data are supplemented by the interpolation method.
Descriptive statistics were made for the main research variables according to the collected data, and the data characteristics of the variables were analyzed. The results were shown in Table 3.
As can be seen from Table 3, there is a great difference in the degree of change among the variables. Table 3 describes the basic characteristics of the data of 285 Cities in China from 2004 to 2017 and analyzes the variables through the mean value, standard deviation, minimum value, and maximum value of the data. According to the data of high-tech industry agglomeration, there is a large gap between the maximum value of 51.619 and the minimum value of 0.006, which indicates that there is a significant hierarchical drop in the level of high-tech industry agglomeration among regions. In terms of standard deviation, upgrading of industrial structure has the largest standard deviation, followed by high-tech industrial agglomeration and economic development level. The large standard deviation of the upgrading of the industrial structure indicates that there are great differences in economic development modes among cities. Due to the differences in labor and resource endowment between regions, high-tech industries are concentrated in major regions, resulting in uneven economic development among cities.
4.2 Results and Empirical Analysis
Considering the spatial correlation between high-tech industrial agglomeration and urban ecological efficiency, this paper uses the spatial simultaneous equation model to test and analyze. The VIF test values of variance inflation factors of all variables in this paper are below 5 and close to 1, indicating that there is no serious multicollinearity among explanatory variables. In order to solve the endogenous problem of high-tech industrial agglomeration and urban ecological efficiency variables, generalized three-stage spatial least squares (GS3SLS) were used to estimate. The estimated results of all urban samples are shown in Table 4.
According to the empirical results in Table 4, based on the estimation results of the geospatial weight matrix, high-tech industrial agglomeration and urban ecological efficiency have a two-way promotion effect, which verifies Hypothesis 1. The impact coefficient of local city ecological efficiency on local high-tech industrial agglomeration is 19.0274, and the impact coefficient of local high-tech industrial agglomeration on local city ecological efficiency is 0.0525, both of which pass the significance level test of 1%. This finding is consistent with previous research suggesting that technological innovation has a positive impact on eco-efficiency (Wang D. et al., 2019). There is an interactive effect between high-tech industry agglomeration and urban ecological efficiency, that is, high-tech industry agglomeration can promote the improvement of urban ecological efficiency, and urban ecological efficiency can also promote high-tech industry agglomeration, and the marginal effect of urban ecological efficiency on high-tech industry agglomeration is greater. Industrial agglomeration has strong environmental externalities, and enterprises in the agglomeration area can reduce the cost of pollution reduction of large-scale enterprises by sharing clean technology spillover and professional pollution prevention facilities (Glaeser and Kahn, 2010). By promoting the upgrading and optimization of industrial structure, various regions can enhance industrial competitiveness, promote the development of high-tech industries and form certain agglomeration, so as to promote the development of urban ecological efficiency.
There is a significant spatial spillover effect of urban eco-efficiency, that is, the urban eco-efficiency in this region has a significant positive correlation with the urban eco-efficiency in neighboring regions, which verifies Hypothesis 2. The estimation results of spatial lag items show that the urban ecological efficiency of the neighboring region has a significant promotion effect on the urban ecological efficiency of the region, while the influence coefficient of the high-tech industrial agglomeration of the neighboring region is positive but not significant. The spatial spillover effects between high-tech industrial agglomeration and urban ecological efficiency are basically consistent with the conclusions of related studies (Su et al., 2021). The inter-regional urban ecological efficiency has a positive spillover effect, and the improvement of ecological efficiency plays a demonstration role among cities, so as to promote the development of urban ecological efficiency in local and neighboring regions. Because of the siphon effect, high-tech industrial agglomeration causes certain mobility of human resources, and the promotion effect between regions is not significant.
There is a significant difference in the regional interaction between high-tech industry agglomeration and urban eco-efficiency, that is, the impact of urban eco-efficiency in the neighboring region on high-tech industry agglomeration is significantly negative, while the impact of high-tech industry agglomeration in the neighboring region on local urban eco-efficiency is negative but not significant, which verifies Hypothesis 3. The spatial lag item of urban ecological efficiency is significantly negative to the estimation coefficient of high-tech industrial agglomeration, while the spatial lag item of high-tech industrial agglomeration is negative but not significant to the estimation coefficient of urban ecological efficiency. Some studies have found that there is a nonlinear relation between industrial agglomeration and environmental pollution, including inverted U shape (Yu et al., 2018; Chen et al., 2020). the increase of industrial agglomeration in neighboring areascauses pressure on local resources, and the rapid development of the industrial economy cannot give consideration to environmental protection, thus affecting the green development of the region. However, the improvement of adjacent ecological efficiency will cause downward pressure on the transfer of polluting industries, which is not conducive to the agglomeration and development of high-tech industries in this region.
Among the control variables, the impact of economic development level on urban ecological efficiency is significantly positive. The improvement of the economic development level means that the financing constraints of urban innovation subjects are less, making it easier to apply resources reasonably and effectively, thus increasing the stability of urban ecological efficiency. The research on coordinated development of economic growth and ecological efficiency is consistent with the conclusion of this paper (Wang et al., 2020b). The impact of economic development level on high-tech industry agglomeration is significantly negative, which may be due to the existence of unbalanced patterns such as resource mismatch, which weakens the impact of economic development level on high-tech industry agglomeration. Population density has a significant positive impact on urban ecological efficiency, but a significant negative impact on high-tech industry agglomeration. The impact of industrial structure upgrading and science and technology on urban ecological efficiency is significantly negative, but the impact on high-tech industrial agglomeration is significantly positive. On the one hand, innovation and R&D are conducive to the transfer of knowledge, and the growth of regional innovation human resources and innovation efficiency promotes the upgrading of industrial structure, which helps optimize the high-tech industrial ecosystem by absorbing richer resources to expand market potential and promote the agglomeration of high-tech industries. On the other hand, in the process of industrial upgrading, regional policies and resources are inclined to other key industries and the unreasonable input of factors affect the improvement of urban ecological efficiency.
The estimation results based on an economic-geospatial weight matrix show that high-tech industrial agglomeration and urban ecological efficiency are mutually reinforcing. The estimated result of a spatial lag item of high-tech industrial agglomeration shows that the influence coefficient of high-tech industrial agglomeration in this region is 0.6032, which is smaller than that of the geographical distance matrix, and the influence coefficient of urban ecological efficiency in this region is −0.0879, which is larger than the negative influence under geographical distance space weight matrix. The small economic gap between neighboring cities strengthens the competition effect and makes the exploitation of resources more brutal. The estimation results of the spatial lag item of urban eco-efficiency show that the influence coefficient of high-tech industrial agglomeration in this region is −3.9767, and the influence coefficient of urban eco-efficiency in this region is 0.5776. The absolute values of the estimated coefficients of both are smaller than the estimated results under the weight matrix of geographical distance space. Small economic gaps between neighboring cities promote spillover effects of innovative technologies. The reduction of industrial structure differences between regions weakens the inhibition effect of urban ecological efficiency in neighboring regions on high-tech industrial agglomeration to some extent.
5. Further Discussion on Heterogeneity of Urban Resources
5.1 Heterogeneity Analysis of Urban Resources
To further analyze the heterogeneity of spatial impact between high-tech industrial agglomeration and urban eco-efficiency, based on the difference of resource endowment between regions, the sample was divided into resource-based cities and non-resource-based cities. According to the list of national resource-based cities contained in “Notice of The State Council on Printing and Distributing the Sustainable Development Plan of National Resource-Based Cities (2013-2020),” 115 resource-based cities and 170 non-resource-based cities were identified. Tables 5, 6 show the estimation results of resource-based cities and non-resource-based cities under two different matrices, respectively.
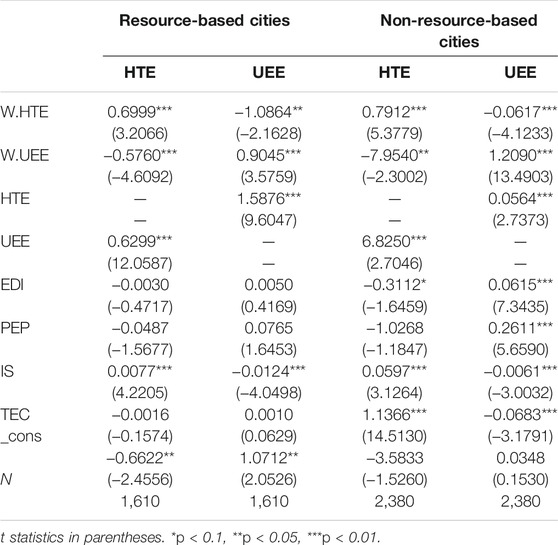
TABLE 5. Estimation results of cities by resource Endowment (geographical distance spatial weight matrix).
According to the empirical results in Tables 5, 6, the spillover effect of spatial interaction between high-tech industrial agglomeration and urban ecological efficiency is heterogeneous on regional resources, which verifies Hypothesis 4. Under the weight matrix of geographical distance space, the estimated coefficients of the impact of high-tech industrial agglomeration on urban ecological efficiency in resource-based cities and non-resource-based cities are 1.5876 and 0.0564 respectively, and the impact coefficients of urban ecological efficiency on high-tech industrial agglomeration are 0.6299 and 6.8250 respectively. Cities with higher resource endowments have richer resource allocation. Accordingly, the more developed urban innovation technology is, the greater the effective promotion effect on ecological efficiency is. The influence coefficient of high-tech industry agglomeration in neighboring areas is 0.6999 in resource-based cities and 0.7912 in non-resource-based cities, respectively. The spillover effect of high-tech industry agglomeration in resource-based cities is better, and resource-based cities have a better resource base, which is conducive to the expansion of industrial scale. In resource-based cities and non-resource-based cities, the influence coefficient of high-tech industry agglomeration in adjacent areas is -1.0864 and -0.0617 respectively, and the negative impact of high-tech industry agglomeration in adjacent areas of resource-based cities is greater. The impact coefficient of the urban eco-efficiency in neighboring areas on the regional urban eco-efficiency is 0.9045 in resource-based cities and 1.2090 in non-resource-based cities, respectively. The eco-efficiency in non-resource-based cities has a stronger spillover effect. In resource-based cities and non-resource-based cities, the influencing coefficient of eco-efficiency of adjacent areas on local high-tech industrial agglomeration is −0.576 and −7.954, respectively. Based on the study of resource-based cities and non-resource-based cities, the empirical results of spatial spillover effect and regional interaction between high-tech industrial agglomeration and urban ecological efficiency are significant. This indicates that among cities with small differences in resource endowments, high-tech industries are more likely to form agglomeration through technology and knowledge spillover, play the driving role in industrial agglomeration, and promote high-tech industrial agglomeration across regions. Eco-efficiency promotes the green development of the urban economy between regions through demonstration.
Under the economic-geospatial weight matrix, there is a significant spatial spillover effect between high-tech industrial agglomeration and urban ecological efficiency in resource-based cities, and the regional interaction between high-tech industrial agglomeration and urban ecological efficiency is significantly positive. The spatial spillover of high-tech industrial agglomeration in non-resource-based cities is significantly positive, while the regional interaction between high-tech industrial agglomeration and urban ecological efficiency is not significant. Under the small economic gap among cities, the interaction effect between resource-based cities is strong. Therefore, when developing high-tech industries, resource-based cities can not only form industrial agglomeration and play the cooperation effect of regional industrial agglomeration, but also improve urban ecological efficiency.
5.2 Robustness Test
In this paper, both the geographical distance space weight matrix and the economic-geographic space weight matrix are used for estimation. The estimation results under different spatial weight matrices show that the direction and significance of the estimated coefficients of each variable are basically the same, indicating that the estimation results are robust to a certain extent. In order to test the robustness of the estimation results, this paper selected the method of changing the number of samples and removed the sample data of 2004 and 2017 to re-estimate. The estimated results are shown in Table 7.
According to the estimation results of the robustness test, the coefficient symbols of the estimation results of the core variables are consistent with those mentioned above, with only a small difference in significance level. It indicates that there is a stable spatial relationship between high-tech industrial agglomeration and urban ecological efficiency, which means that the regression results of this paper are robust.
6 Conclusion
Ecological efficiency is of great significance to the sustainable economic development of a country or region. By constructing a spatial simultaneous equation model and using the generalized three-stage spatial least squares estimation method, this paper examines the spatial spillovers and regional interactions of high-tech industrial agglomeration and urban ecological efficiency in 285 cities above the prefecture-level in China and analyzes their heterogeneity. The conclusions are as follows:
First, there is a two-way promoting effect between high-tech industrial agglomeration and urban ecological efficiency. High-tech industrial agglomeration and urban ecological efficiency promote each other. High-tech industrial agglomeration reduces emissions and environmental pollution through innovative technologies and promotes the improvement of urban ecological efficiency. Urban ecological efficiency promotes the formation of innovation-oriented high-tech industry agglomeration through incentives. Therefore, the development of high-tech industry promotes the improvement of ecological efficiency, which is of great significance to the development of circular economy and other long-term construction.
Second, there is a significant spatial spillover effect of urban eco-efficiency, that is, the urban eco-efficiency in this region has a significant positive correlation with the urban eco-efficiency in neighboring regions. The urban ecological efficiency of the neighboring region promotes the improvement of the urban ecological efficiency of the region through the spillover effect, and the improvement of the urban ecological efficiency of the neighboring region plays a demonstrative role, driving the regional ecological efficiency to follow. The high technology industry agglomeration forms a competition effect and there also exists a siphon effect, and the spillover effect between regions is not obvious.
Third, there is a significant difference in the interaction between high-tech industrial agglomeration and urban ecological efficiency, that is, the impact of urban ecological efficiency in neighboring areas on local high-tech industry agglomeration is significantly negative, while the impact of high-tech industry agglomeration in neighboring areas on local urban ecological efficiency is negative but not significant. The rapid development of economic industries such as high-tech industries ignores the synchronous improvement of ecological efficiency, while the construction of ecological efficiency accelerates the transfer of polluting industries, which is not conducive to the agglomeration of high-tech industries in the surrounding areas.
Fourth, the spatial spillover effects of high-tech industrial agglomeration and urban ecological efficiency are heterogeneous in terms of regional resources, and it is better to study the regional interaction and spatial spillover effect by sample analysis. The spatial spillover effect of high-tech industrial agglomeration in resource-based cities is stronger than that in non-resource-based cities, and resource-based cities have better resource allocation, which is conducive to the spillover of knowledge and technology. However, non-resource-based cities have more advantages in ecological efficiency. Comparatively speaking, non-resource-based cities have less resource consumption and environmental pollution.
Data Availability Statement
The original contributions presented in the study are included in the article/Supplementary Material, further inquiries can be directed to the corresponding author.
Author Contributions
MW and GL contributed to all aspects of this work; YL mainly completed data collection and manuscript writing. All authors reviewed the manuscript. All authors have read and agreed to the published version of the manuscript.
Funding
This research was funded by the Chinese National Funding of Social Sciences, grant number 18ATJ002 and 17BTJ016, and the 13th Five-year Plan of Guangzhou Social Science, grant number 2018GZYB129.
Conflict of Interest
The authors declare that the research was conducted in the absence of any commercial or financial relationships that could be construed as a potential conflict of interest.
Publisher’s Note
All claims expressed in this article are solely those of the authors and do not necessarily represent those of their affiliated organizations, or those of the publisher, the editors, and the reviewers. Any product that may be evaluated in this article, or claim that may be made by its manufacturer, is not guaranteed or endorsed by the publisher.
Supplementary Material
The Supplementary Material for this article can be found online at: https://www.frontiersin.org/articles/10.3389/fenvs.2022.829851/full#supplementary-material
References
Balsalobre, D., Álvarez, A., and Cantos, J. M. (2015). Public Budgets for Energy RD&D and the Effects on Energy Intensity and Pollution Levels. Environ. Sci. Pollut. Res. 22, 4881–4892. doi:10.1007/s11356-014-3121-3
Chen, C., Sun, Y., Lan, Q., and Jiang, F. (2020). Impacts of Industrial Agglomeration on Pollution and Ecological Efficiency-A Spatial Econometric Analysis Based on a Big Panel Dataset of China's 259 Cities. J. Clean. Prod. 258, 120721. doi:10.1016/j.jclepro.2020.120721
D’Agostino, L. M. (2015). How MNEs Respond to Environmental Regulation: Integrating the Porter Hypothesis and the Pollution haven Hypothesis. Econ. Polit. 32, 245–269. doi:10.1007/s40888-015-0010-2
Dyckhoff, H., and Allen, K. (2001). Measuring Ecological Efficiency with Data Envelopment Analysis (DEA). Eur. J. Oper. Res. 132, 312–325. doi:10.1016/s0377-2217(00)00154-5
Glaeser, E. L., and Kahn, M. E. (2010). The Greenness of Cities: Carbon Dioxide Emissions and Urban Development. J. Urban Econ. 67, 404–418. doi:10.1016/j.jue.2009.11.006
Haas, R., Ajanovic, A., Ajanovic, A., Ramsebner, J., Perger, T., Knápek, J., et al. (2021). Financing the Future Infrastructure of Sustainable Energy Systems. Green. Financ. 3, 90–118. doi:10.3934/gf.2021006
Hur, T., Kim, I., and Yamamoto, R. (2004). Measurement of green Productivity and its Improvement. J. Clean. Prod. 12, 673–683. doi:10.1016/j.jclepro.2003.08.004
Kassouri, Y. (2022). Fiscal Decentralization and Public Budgets for Energy RD&D: A Race to the Bottom? Energy Policy 161, 112761. doi:10.1016/j.enpol.2021.112761
Kassouri, Y. (2021). Monitoring the Spatial Spillover Effects of Urbanization on Water, Built-Up Land and Ecological Footprints in Sub-saharan Africa. J. Environ. Manage. 300, 113690. doi:10.1016/j.jenvman.2021.113690
Li, G. D. (2019). Spatiotemporal Dynamics of Ecological Total-Factor Energy Efficiency and Their Drivers in China at the Prefecture Level. Ijerph 16, 3480. doi:10.3390/ijerph16183480
Li, T., Li, X., Li, X., and Albitar, K. (2021a). Threshold Effects of Financialization on enterprise R & D Innovation: a Comparison Research on Heterogeneity. Quantitative Finance Econ. 5, 496–515. doi:10.3934/qfe.2021022
Li, T., Li, X., and Liao, G. (2021b). Business Cycles and Energy Intensity. Evidence from Emerging Economies. Borsa Istanbul Rev. doi:10.1016/j.bir.2021.07.005
Li, T., Ma, J., and Ma, J. (2021). Does Digital Finance Benefit the Income of Rural Residents? A Case Study on China. Qfe 5, 664–688. doi:10.3934/qfe.2021030
Li, Z., Ao, Z., and Mo, B. (2021b). Revisiting the Valuable Roles of Global Financial Assets for International Stock Markets: Quantile Coherence and Causality-In-Quantiles Approaches. Mathematics 9, 1750. doi:10.3390/math9151750
Li, Z., Zou, F., and Mo, B. (2021a). Does Mandatory CSR Disclosure Affect enterprise Total Factor Productivity? Econ. Research-Ekonomska Istraživanja, 1–20. doi:10.1080/1331677x.2021.2019596
Li, Z., Zou, F., Tan, Y., and Zhu, J. (2021c). Does Financial Excess Support Land Urbanization-An Empirical Study of Cities in China. Land 10, 635. doi:10.3390/land10060635
Liu, J., Cheng, Z., and Zhang, H. (2017). Does Industrial Agglomeration Promote the Increase of Energy Efficiency in China? J. Clean. Prod. 164, 30–37. doi:10.1016/j.jclepro.2017.06.179
Liu, S., Zhu, Y., and Du, K. (2017). The Impact of Industrial Agglomeration on Industrial Pollutant Emission: Evidence from China under New Normal. Clean. Techn Environ. Pol. 19, 2327–2334. doi:10.1007/s10098-017-1407-0
Liu, T., Li, J., Chen, J., and Yang, S. (2019). Urban Ecological Efficiency and its Influencing Factors-A Case Study in Henan Province, China. Sustainability 11, 5048. doi:10.3390/su11185048
Liu, Y., Li, Z., and Xu, M. (2020). The Influential Factors of Financial Cycle Spillover: Evidence from China. Emerging Markets Finance and Trade 56, 1336–1350. doi:10.1080/1540496x.2019.1658076
Ma, X., Wang, C., Yu, Y., Li, Y., Dong, B., Zhang, X., et al. (2018). Ecological Efficiency in China and its Influencing Factors-A Super-efficient SBM Metafrontier-Malmquist-Tobit Model Study. Environ. Sci. Pollut. Res. 25, 20880–20898. doi:10.1007/s11356-018-1949-7
Sardo, F., Serrasqueiro, Z., and Serrasqueiro, Z. (2021). Intellectual Capital and High-Tech Firms' Financing Choices in the European Context: a Panel Data Analysis. Quantitative Finance Econ. 5, 1–18. doi:10.3934/qfe.2021001
Seppäläa, J., Melanen, M., Mäenpää, I., Koskela, S., Tenhunen, J., and Hiltunen, M.-R. (2005). How Can the Eco-Efficiency of a Region Be Measured and Monitored? J. Ind. Ecol. 9, 117–130. doi:10.1162/108819805775247972
Shakouri, R., Salahi, M., Salahi, M., and Kordrostami, S. (2019). Stochastic P-Robust Approach to Two-Stage Network DEA Model. Quantitative Finance Econ. 3, 315–346. doi:10.3934/qfe.2019.2.315
Shen, Y. C., Sun, X. L., and Fu, Y. Y. (2021). The Spatial Network and its Driving Factors for Sustainable Total-Factor Ecology Efficiency: the Case of China. Environ. Sci. Pollut. Res. 28 (48), 68930–68945. doi:10.1007/s11356-021-15456-3
Shi, J., and Huang, Y. L. (2019). [Spatial and Temporal Differences and Influencing Factors of Ecological Capital Efficiency in Northeast China]. Ying Yong Sheng Tai Xue Bao 30, 3527–3534. doi:10.13287/j.1001-9332.201910.012
Si, L., Wang, J., Yang, S., Yang, Y., and Zhang, J. (2021). Urban Green Development towards Sustainability in Northwest China: Efficiency Assessment, Spatial-Temporal Differentiation Characters, and Influencing Factors. Complexity. 2021, 1–19. doi:10.1155/2021/6630904
Song, L., and Zhou, X. (2021). Does the Green Industry Policy Reduce Industrial Pollution Emissions?-Evidence from China's National Eco-Industrial Park. Sustainability. 13, 6343. doi:10.3390/su13116343
Song, M., Zhao, X., Shang, Y., and Chen, B. (2020a). Realization of green Transition Based on the Anti-driving Mechanism: An Analysis of Environmental Regulation from the Perspective of Resource Dependence in China. Sci. Total Environ. 698, 134317. doi:10.1016/j.scitotenv.2019.134317
Song, M., Zhao, X., and Shang, Y. (2020b). The Impact of Low-Carbon City Construction on Ecological Efficiency: Empirical Evidence from Quasi-Natural Experiments. Resour. Conservation Recycling 157, 104777. doi:10.1016/j.resconrec.2020.104777
Su, Y., Li, Z., and Yang, C. (2021). Spatial Interaction Spillover Effects between Digital Financial Technology and Urban Ecological Efficiency in China: An Empirical Study Based on Spatial Simultaneous Equations. Ijerph 18, 8535. doi:10.3390/ijerph18168535
Wang, D., Wan, K., and Yang, J. (2019). Ecological Efficiency of Coal Cities in China: Evaluation and Influence Factors. Nat. Hazards 95, 363–379. doi:10.1007/s11069-018-3441-6
Wang, J., Ye, X., and Wei, Y. (2019). Effects of Agglomeration, Environmental Regulations, and Technology on Pollutant Emissions in China: Integrating Spatial, Social, and Economic Network Analyses. Sustainability. 11, 363. doi:10.3390/su11020363
Wang, M., Gu, R., Gu, R., Wang, M., and Zhang, J. (2021). Research on the Impact of Finance on Promoting Technological Innovation Based on the State-Space Model. Green. Financ. 3, 119–137. doi:10.3934/gf.2021007
Wang, S., Hua, G., and Yang, L. (2020b). Coordinated Development of Economic Growth and Ecological Efficiency in Jiangsu, China. Environ. Sci. Pollut. Res. 27, 36664–36676. doi:10.1007/s11356-020-09297-9
Wang, S., Jia, M., Zhou, Y., and Fan, F. (2020a). Impacts of Changing Urban Form on Ecological Efficiency in China: a Comparison between Urban Agglomerations and Administrative Areas. J. Environ. Plann. Manage. 63, 1834–1856. doi:10.1080/09640568.2019.1689932
Wang, S., Sun, X., and Song, M. (2021). Environmental Regulation, Resource Misallocation, and Ecological Efficiency. Emerging Markets Finance and Trade 57, 410–429. doi:10.1080/1540496x.2018.1529560
Wang, Z., Liu, B., Wang, L., and Shao, Q. (2021). Measurement and Temporal & Spatial Variation of Urban Eco-Efficiency in the Yellow River Basin. Phys. Chem. Earth, Parts A/B/C 122, 102981. doi:10.1016/j.pce.2021.102981
Xu, R., and Wang, W. (2020). Spatiotemporal Evolution and Impacts of Water Ecological Efficiency of Zhongyuan Urban Agglomeration from the Perspective of Social Network. J. Coastal Res. 104, 54–57. doi:10.2112/jcr-si104-009.1
Yao, Y., Hu, D., Hu, D., Yang, C., and Tan, Y. (2021). The Impact and Mechanism of Fintech on green Total Factor Productivity. Green. Financ. 3, 198–221. doi:10.3934/gf.2021011
Yasmeen, H., Tan, Q., Zameer, H., Tan, J., and Nawaz, K. (2020). Exploring the Impact of Technological Innovation, Environmental Regulations and Urbanization on Ecological Efficiency of China in the Context of COP21. J. Environ. Manage. 274, 111210. doi:10.1016/j.jenvman.2020.111210
Yin, X., and Guo, L. (2021). Industrial Efficiency Analysis Based on the Spatial Panel Model. J. Wireless Com Netw. 2021. doi:10.1186/s13638-021-01907-5
Yu, Y., Zhang, Y., and Miao, X. (2018). Impacts of Dynamic Agglomeration Externalities on Eco-Efficiency: Empirical Evidence from China. Ijerph 15, 2304. doi:10.3390/ijerph15102304
Zeng, D.-Z., and Zhao, L. (2009). Pollution Havens and Industrial Agglomeration. J. Environ. Econ. Manage. 58, 141–153. doi:10.1016/j.jeem.2008.09.003
Zhang, J. (2008). Estimation of China's Provincial Capital Stock (1952-2004) with Applications. J. Chin. Econ. Business Stud. 6, 177–196. doi:10.1080/14765280802028302
Zhang, Y., Chen, S., and Wang, N. (2020). The Impact of Educational Level on Ecological Efficiency: An Empirical Analysis in the Middle Reaches of Yangtze River. J. Coastal Res. 106, 388–395. doi:10.2112/si106-089.1
Keywords: high-tech industry agglomeration, urban ecological efficiency, spatial spillover effect, regional interaction, spatial simultaneous equation model
Citation: Wang M, Li Y and Liao G (2022) Spatial Spillover and Interaction Between High-Tech Industrial Agglomeration and Urban Ecological Efficiency. Front. Environ. Sci. 10:829851. doi: 10.3389/fenvs.2022.829851
Received: 06 December 2021; Accepted: 27 January 2022;
Published: 21 March 2022.
Edited by:
Kai Fang, Zhejiang University, ChinaReviewed by:
Yacouba Kassouri, Leipzig University, GermanyLuigi Aldieri, University of Salerno, Italy
Copyright © 2022 Wang, Li and Liao. This is an open-access article distributed under the terms of the Creative Commons Attribution License (CC BY). The use, distribution or reproduction in other forums is permitted, provided the original author(s) and the copyright owner(s) are credited and that the original publication in this journal is cited, in accordance with accepted academic practice. No use, distribution or reproduction is permitted which does not comply with these terms.
*Correspondence: Yanling Li, MjExMjA2NDEwMEBlLmd6aHUuZWR1LmNu