- 1Institute of Marine Development, Ocean University of China, Qingdao, China
- 2School of Economics, Ocean University of China, Qingdao, China
The green and coordinated development of the Yangtze River Economic Belt (YREB) is of great significance to the overall high-quality growth of the Chinese economy. Targeting green developmental issues and regional disparities in the YREB, this article proposes a method that combines the Super-EBM and Malmquist–Luenberger indexes to measure the green economic efficiency (GEE) of 108 cities from 2003 to 2018. Moreover, we adopted the dynamic spatial Durbin panel model to conduct a convergence test including economic linkage, capital liquidity, talent mobility, and information sharing. The outcomes pointed out that 1) the green economy shows a volatile growing trend, and technological progress is the main source of the YREB’s GEE; 2) we have noticed the formation of spatial differentiation in the three basins, especially in the central areas of big cities such as Shanghai, Hangzhou, Wuhan, and Chongqing; 3) the GEE follows a global β-convergence trend that narrows the gap in green economic efficiency. More specifically, the downstream (11.48%) has the fastest convergence rate, followed by the midstream (8.09%) and upstream (7.97%); 4) capital liquidity, governance, and economic openness contribute a lot to maintaining the convergence trend. The primary contributions of this study are the policy recommendations to promote green development and narrow the gap in the YREB to ultimately achieve effective coordinated development.
1 Introduction
Green economy takes efficiency, harmony, and sustainability as the development goal and is a matter of global consensus in the 21st century. Targeting an effective development direction, many countries and international organizations have been placing greater importance on the green economy (OECD, 2012; World Bank, 2012; Aguiar et al., 2018), which is currently regarded as a means to solve the worldwide crisis and guarantee human survival. Over the past decades, resource depletion and environmental pollution have also become serious constraints for China’s sustainable development (Ahmed et al., 2020; Lin et al., 2021). Since 2017, the country has implemented high-quality developmental initiatives (Zhou et al., 2020) and adopted low-carbon development strategies (Han et al., 2021), putting its national ecological environment in a crucial position.
The Yangtze River traverses the east, the middle, and the west of China and connects the eastern coast and the vast inland of the country. It has unique advantages and great potential to build a new economic belt through the river’s golden waterway (Chen et al., 2018). However, high-intensity exploitation and unreasonable resource utilization have caused degradation of the local ecosystem functions, shedding light on ecological and environmental issues (Zhang et al., 2019). The Plan for Conservation of Aquatic Biodiversity in Key River Basins issued in 2018 reported that the number of threatened fish species of Yangtze upstream accounted for 27.6% of the total. At the same time, Baiji, white sturgeon, and shad became functionally extinct, and the Yangtze finless porpoise and Chinese sturgeon became critically endangered species. Moreover, according to the Announcement on Soil Conservation in the Yangtze River Basin (2020), soil erosion in the YREB reached 389,000 square kilometers in 2020, accounting for 18.88% of the total land area. While China has already started implementing the Yangtze River Protection Law to protect the environment since March 2021, more practical efforts are still needed to administer the other potential issues, for example, pollution, biological protection, and soil conservation (Tickner et al., 2017).
Meanwhile, the deterioration of the ecological environment has deepened the developmental gap between the regions of the YREB. The upstream’s location, traffic, and social resources are inherently poor. In addition to that, the deteriorating environment makes it more difficult to attract funds and talents, exacerbating its backwardness. According to 2021 China Statistical Yearbook, the upstream, midstream, and downstream per capita GDP were about 58,635 CNY/person, 64,737 CNY/person, and 110,261 CNY/person, respectively. The official report also highlighted that the downstream per capita GDP was nearly twice as high as that of the upstream. However, due to the fragile environment in the upstream, this gap may continue to widen. Although the upstream ecological protection ensures the safety and benefits of the midstream and downstream, the upstream is not financially compensated. In short, environmental protection is closely related to narrowing the developmental gap. Particularly in the YREB, the green development and coordinated development are inseparable. Both the aspects are the central topics of this article that focuses on studying means to achieve coordinated development in the area, strengthening its green economy.
This article contributes to promoting coordinated and sustainable development in the Yangtze River Economic Belt. First, the green economic efficiency indicators are constructed from considering the region’s development quality. These indicators are used to measure the green economy in the YREB from dynamic and static perspectives and outline the characteristics of spatial–temporal evolution and the sources of economic growth. Second, due to the impact of environmental protection on coordinated development, it is necessary to include the regional differences and the changes they promote in order to successfully analyze the factors that narrow the green developmental gap. A spatial convergence test is conducted, including economic linkage, capital liquidity, talent mobility, and information sharing as variables. Finally, based on the results, we are able to provide policy recommendations for improving the green, harmonious development in the YREB.
The other sections of this article are structured as follows. Literature Review reviews the literature and proposes contribution margins. Materials and Methods constructs the GEE’s evaluation index system and introduces the measurement and convergence test methods. Results measures the GEE, explores the growth sources, and analyzes the convergence trend and influencing factors. Discussion and Conclusion discusses, concludes, and puts forward policy recommendations.
2 Literature Review
Affected by global warming and harsh ecological environment, the green economy became a scholarly hot topic that has been explained, analyzed, and studied by many authors worldwide (Bina, 2013; Loiseau et al., 2016; Buseth, 2017; Maran and Nedelea, 2017). The most recognized definition of “green economy” comes from United Nations Environment Programme (UNEP) which defines it as an economy that contributes to improving human well-being and social equity and significantly reducing environmental risks and ecological scarcity. Additionally, the concept of green economy also embraces some mandatory features such as being low-carbon, resource-efficient, and society-inclusive (UNEP, 2011). Moreover, the green economic efficiency (GEE) is an indicator that meets the sustainable development need, and comprehensively considers resource and environmental costs (Yang and Wen, 2017). Specifically in the YREB, improving the efficiency of the green economy is an important way to build an ecological civilization and promote economic transformation and development in the region (Che et al., 2018).
Modern research on GEE mainly focuses on the measurement analysis of regional differences and influencing factors. Some of the most commonly used methods of measuring efficiency are the index system evaluation (Gao et al., 2013), data envelopment analysis (DEA) (Tong et al., 2019), and stochastic frontier analysis (Zhang and Yang., 2020). Integrating multiple inputs (including capital, labor, and energy) into the evaluation of GEE is currently a widespread practice (Young, 2003; Tang and Qin, 2021). The DEA methodology considers the substitution effects between the various elements, relying on the radial and non-radial directional distance functions to evaluate the GEE (Liu and Shang, 2020). Meanwhile, many scholars have used ARCGIS software to exhibit the spatial–temporal dynamic evolution characteristics of the GEE (Lin et al., 2017; Li et al., 2021). The research on GEE’s temporal and spatial differences in the YREB found a leading stage in the east, followed by the west, and collapsing in the middle, and a registered cascading rise from 2005 to 2016 (Lu et al., 2017). Significant spatial spillover effects were also found in YREB’s green economy (Bai and Xia, 2019). Scholars have also been systematically analyzing the influencing factors in the region, which mainly includes industrialization, urbanization, regional integration, government investments, fossil fuel energy use, and financial development (Hao et al., 2018; Zheng et al., 2018; Wang et al., 2019; Dmuchowski et al., 2021; Wu et al., 2021; Wu and Wu, 2021).
The convergence trend of the green economy is an important prerequisite and realistic basis for the regional, sustainable, and coordinated development, and it is widely adopted in research (Yang and Hu, 2010; Kinfemichael and Morshed, 2019; Zhu et al., 2020; Blampied, 2021; Desli and Gkoulgkoutsika, 2021). The existing literature can be divided into two categories based on whether or not spatial factors have been considered. Some studies have used the traditional convergence test to testify the existence of convergence in the YREB (Li and Tu, 2017; Chen et al., 2020). However, some scholars’ estimation results may be biased due to the neglect of spatial effects. Other scientists have relied on the spatial convergence test, which takes into account the spatial correlation of economic growth in different regions, so their estimation results are more credible. Anselin (1988) attested that the spatial correlation between regions had an important impact on the convergence of the regional economy. Similarly, Barthélemy (2011) also found that the structure and the evolution of spatial networks were significantly linked to urbanization and regional development. However, many modern studies only considered proximity or geographic distance to confirm the existence of convergence (Qian and Liu, 2014; Xu and Zhao, 2015), leaving behind the flow and impact factors of this dynamic.
To fill this literature gap, this study constructs spatial correlation networks from four dimensions: economic linkage, capital liquidity, talent mobility, and information sharing. This methodology is suitable for the goals of this research because economic linkage and interregional economic relevance are regarded as the main channels of spatial overflow, which means that the economic volume can represent the size of overflow (Zhang and Zhou, 2012). Second, human resources are the most important resources for talent mobility in the 21st century. Rational workers would move to those regions with better employment conditions, more opportunities, and more generous benefits to maximize their interests. This dynamic leads to knowledge spillover and imitation learning among different regions (Shao and Su, 2017). Regarding capital liquidity, capital is an important means of production, which expands production scale, attracts more talents, and introduces advanced production equipment. This indicator is mainly made of deposits and loans from financial institutions. Capital is also allocated in areas with higher yields and lower risks and dynamically flows across regions (Liu and Jia, 2019a). Finally, for information sharing, if companies grasp information early, that is, government policies, consumer demand changes, and market news, they can seize opportunities and take initiatives to gain advantages among competitors. In this context, the virtual network breaks through the geographical restrictions, making information transfer easier. Therefore, information sharing increases opportunities for cooperation and regional development (Li and Wang, 2014).
In summary, previous studies on GEE mainly relied on the traditional DEA model, and their single indicators were simple, which led to similar and unreliable results in different regions. This study measures GEE by defining a novel, comprehensive evaluation index system, which takes the main features of the YREB into account, and combines the Super-EBM index and the ML index into a new model. Therefore, it enriches the index system and calculation methods already available in the field, boosting the production of more reliable results and the identification of GEE’s growth sources (Tone and Tsutsui, 2010). In addition, most of the studies on convergence have been developed based on traditional convergence tests. We have constructed new spatial correlation networks that comprise multiple factors such as economic linkage, capital liquidity, talent mobility, and information sharing, making the convergence test results more credible. Finally, we also identified that previous studies looked at GEE from a provincial or municipal level and paid less attention to specific areas similar to a certain watershed. One of the novelties of this study is the discussion of GEE in the YREB and the region’s green growing trends taken from a spatial perspective. Ultimately, the outcomes presented here enrich the research on the sustainable development of the Yangtze River.
3 Materials and Methods
3.1 Study Area
Relying on the geographical advantages of the Yangtze River waterway, the YREB has been developed with a high degree of economic openness, a large concentration of outstanding talents, complete modern industrial systems, and robust economic growth. The area became an important engine to promote the high-quality development of China’s economy (Zhang et al., 2021). With dense cities and concentrated economic activities, the YREB is an inland river economic belt area with one of the world’s largest exploitations. With the rapid development of resources and population growth, the ecological environment of YREB cities has been under great pressure in response to human activities. According to the National Bureau of Statistics, the YREB is about 2.05 million square kilometers, accounting for 21.4% of China’s total area. The region also concentrates more than 42% of China’s population, accounting for 46% of the country’s GDP in 2019. Based on the aforementioned features, we chose 108 cities as the study domain to research the green and coordinated development in the YREB (shown in Figure 1).
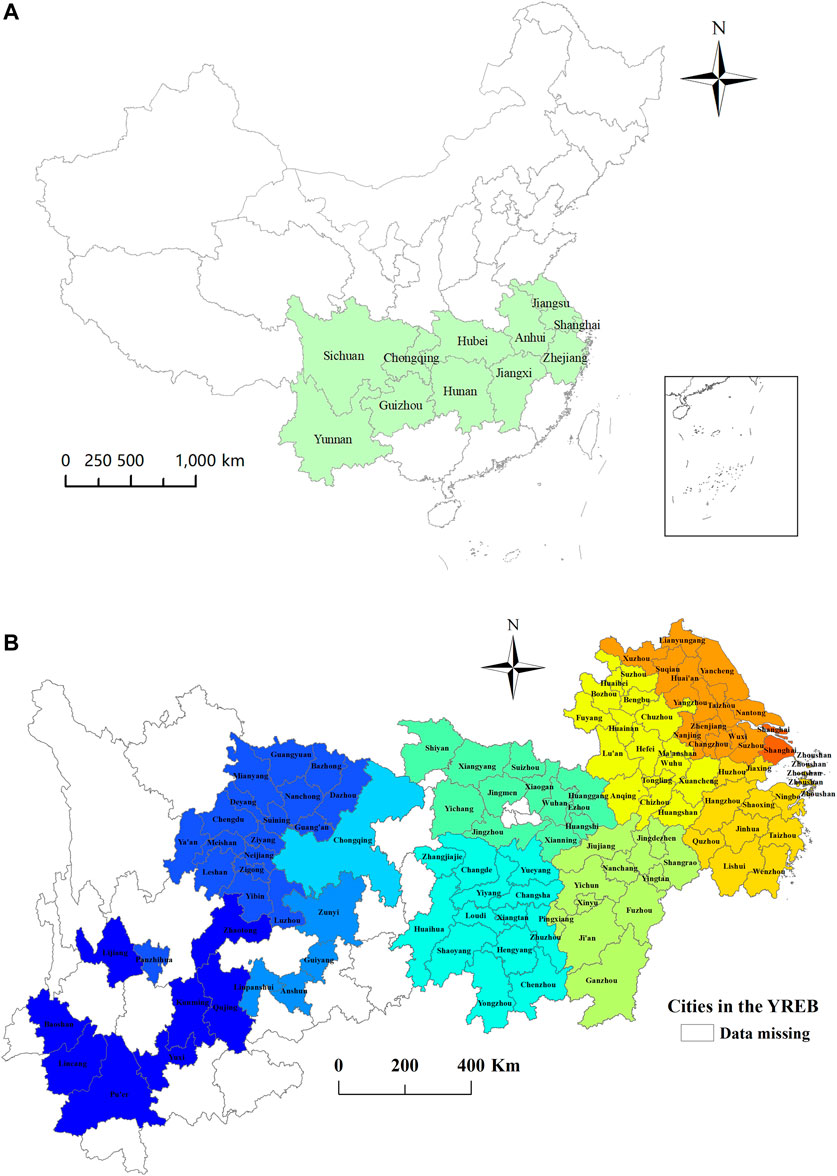
FIGURE 1. (A) represents the schematic of the locations of the 11 surveyed provinces—Shanghai, Jiangsu, Zhejiang, Anhui, Jiangxi, Hubei, Hunan, Chongqing, Guizhou, Sichuan, and Yunnan in the Yangtze River Economic Belt, China. (B) shows the 108 cities, including two municipalities, five sub-provincial cities, and 101 prefecture-level cities.
3.2 Construction of Indicator and Data Sources
We have defined an input–output indicator system fully considering the resources and environmental factors according to the connotation of GEE and existing research (Yang and Wen, 2017; Chen et al., 2020). This system reflects the variables urban economic growth, social benefits, resource conservation, and environmental protection (refer to Table 1). We have considered information from 2003 to 2018 after examining data availability and comprehensiveness. The relevant data included in this study were extracted from China City Statistical Yearbook and local statistical bulletins. The forward (or backward) interpolation method was used to fill in any missing data.
3.3 Methods
First, we referred to the methodology adopted in previous studies to measure the green economic efficiency in the YREB and constructed a novel input–output indicator, combining the Super-EBM and ML index models (Chen et al., 2020). Second, to analyze GEE’s gap trends and factors, we built spatial correlation networks and a spatial Durbin model for convergence based on Liu and Jia’s (2019b) and Qian and Liu’s (2014) outcome. In addition, to ensure the robustness of the spatial effects, Moran’s I index was also used to test the spatial autocorrelation effects (Moran, 1948).
3.3.1 Super EBM-ML Index
Tone and Tsutsui (2010) put forward an EBM model that simultaneously takes into account the advantages of radial and non-radial DEA models to evaluate the efficiency of the decision-making unit (DMU). The decision-making unit is the object of efficiency evaluation, which can be understood as the entity that converts “input” into “output.” Following this trend, Andersen and Petersen (1993) proposed a super-efficiency DEA model to make coherent comparisons between the effective DMUs. Based on the definition of GEE, this study adopts a Super-EBM model with unexpected outputs, which can be expressed as follows:
where
The dynamic GEE changes cannot be accurately measured only with the Super-EBM model. Färe et al. (1992) were the first to propose the calculation of the Malmquist index using the DEA model and further decomposed it into technical efficiency change (EC) and technological change (TC) to reflect the frontier shift in the DEA. A few years later, Chung et al. (1997) introduced the directional distance function into the Malmquist index to deal with the problem of undesired output and officially named it Malmquist–Luenberger (ML) index. The ML productivity index of period t+1 with period t as the base period is calculated as follows:
where
3.3.2 Convergence Model
This study introduces a conditional β-convergence test to examine whether the GEE of cities in the YREB has convergence characteristics. Conditional β-convergence represents the trend of approaching a steady state, considering the different characteristics and conditions of each area. This method takes into account regional differences to accurately test the convergence trend and can also outline the factors that affect convergence. According to Islam (1998), the conditional β-convergence of regional GEE can be expressed as follows:
where
Affected by the gradient development model and spatial strategy, the economic efficiency of various regions is spatially linked through the “polarization effect” and “trickle-down effect” (Lv and Yu, 2009). These two effects explain the economic interaction and influence between economically developed regions and economically underdeveloped regions. According to Peng et al. (2020), regional energy eco-efficiency also has spatial correlations. Therefore, the geographic spatial dependence must be taken into account to obtain a more credible estimation result. Our dynamic spatial Durbin panel model is constructed to test the convergence trend of GEE, based on Yu and Lee’s (2012) outcomes:
where
3.3.3 Spatial Weight Setting
Social gravity law widely exists in human travel, population migration, commodity trade, information communication, scientific collaboration, and so on (Wang et al., 2021). There are spatial interactions between the regions within a certain range, which forms an increasingly complex network (Li et al., 2014). Many scholars have applied the gravity model to the quantitative analysis of the interaction between different regions (Ramajo et al., 2008; Hou et al., 2009; Zhang and Zhou, 2012; Li and Wang, 2014; Shao and Su, 2017; Liu and Jia, 2019a). The spatial interaction of regional economic growth can be expressed as follows:
where
The weight matrix under the spatial network connections is introduced from the dimensions of economic linkage, capital liquidity, talent mobility, and information sharing. First, per capita GDP was used to measure economic growth since more economically developed regions generally have more economic linkage with other regions. Second, the total number of employees in urban was used to measure the talent concentration and labor. A higher number of employees represent a greater likelihood of talent mobility. Third, the balance of deposits and loans in financial institutions was used to measure capital. The more the capital, the more active it is, and the greater is the scale of capital liquidity. Finally, the number of Internet users is used to measure the accessibility of information. The more the Internet users, the greater the possibility of information sharing.
The adjacent weight and geographic distance weight were also used to compare the differences in convergence with the traditional weights. The adjacent weight is based on whether the two regions are adjacent or not. If the areas i and j are adjacent,
3.3.4 Global Spatial Autocorrelation
The data must first be verified for spatial dependencies when determining whether the spatial econometric model should be used as a part of the evaluation process. Spatial autocorrelation is usually measured by Moran’s I index (Moran, 1948), Geary’s C index (Geary, 1954), and Getis-Ord’s G index (Getis and Ord, 1992). Although Moran’s I index was proposed earlier, it is still widely used now (Ganegoda et al., 2021; Cai et al., 2022) because it is more sensitive to spatial correlation and is suitable for the nonbinary nested spatial matrices. It is noteworthy that the test here only provides preliminary evidence, and a more in-depth analysis depends on the following multiple regression model. Moran’s I index can be expressed as follows:
where S2 is the sample variance and
4 Results
4.1 Measurement of the Green Economic Efficiency
The results are calculated by MaxDEA Professional Edition and are illustrated in detail in Figure 2. Three indexes show the features of rising volatility around 1, indicating that the overall level of green economic development in the YREB has improved from 2004 to 2018. From the perspective of the sources of GEE, the growth of the GEE in the YREB mainly relied on the improvement of technological progress that contributed to 0.24% of the GEE. Meanwhile, the contribution of green economy technological efficiency to the GEE was relatively small, about 0.1%. This is mainly because the YREB has greatly improved its green technology in new energy and pollution control fields. The area has received increasing financial subsidies, preferential development treatment, and talent introduction. The improvement of green technology efficiency is mainly based on optimizing the rational allocation of resources and improving management, and its effects on GEE are relatively moderate. Therefore, continuing to promote green technical innovation, improving the market-oriented operating mechanism, and optimizing the business environment will further boost the green development of the YREB.
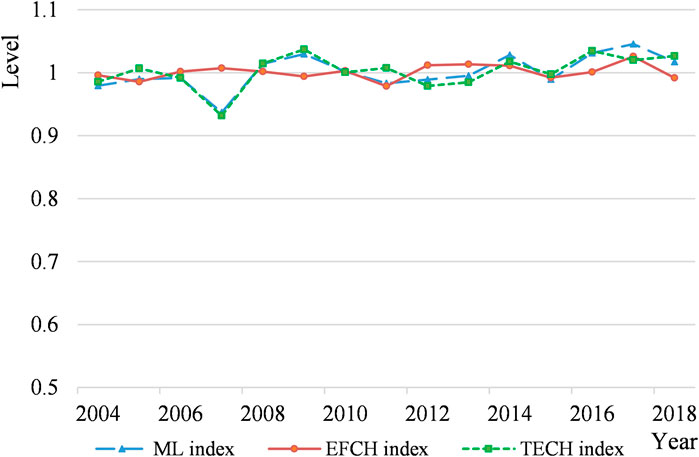
FIGURE 2. Annual change of the ML index, EFCH index, and TECH index in the YREB over 2004–2018. The ML index, EFCH index, and TECH index represent the GEE’s change, technical efficiency change, and technological change, respectively. Meanwhile, the ML index is the product of the EFCH index and TECH index.
Figure 3 shows the spatial–temporal characteristics of GEE in the YREB and presents significant spatial differentiation in 2003, 2008, 2013, and 2018. As illustrated, the GEE has formed the spatial differentiation in the upstream, midstream, and downstream, and high-efficiency regions are concentrated in big cities, such as Shanghai, Hangzhou, Wuhan, and Chongqing. From 2003 to 2018, cities with rising and falling GEE had clear and distinct regional characteristics. The areas where GEE increases are mainly concentrated in the upstream are Chongqing, Zunyi, Zigong, Neijiang, Ziyang, Nanchong, and Bazhong. Due to economic progress, the GEE has been rising and progressing in the overall level of the YREB. These areas are relatively fragile in ecology, but the country’s poverty alleviation policy brings them development opportunities. These regions achieve economic growth and ecological protection, ultimately improving their GEE. The areas where GEE declines are mainly located in Jiangxi Province (in the midstream) include Ji’an, Fuzhou, Yingtan, Yichun, and Xinyu. This is because in that region, the primary economic drivers are agriculture and industries such as nonferrous metallurgy, coal, steel, machinery manufacturing, and fertilizers. These heavy industries cannot be easily transformed, and the development of new energy and new materials industries requires more time. In addition, these cities cannot compete with hubs like Shanghai and Changsha for talents and capital to develop high-tech and modern service industries, so they are in a backward position in the development process.
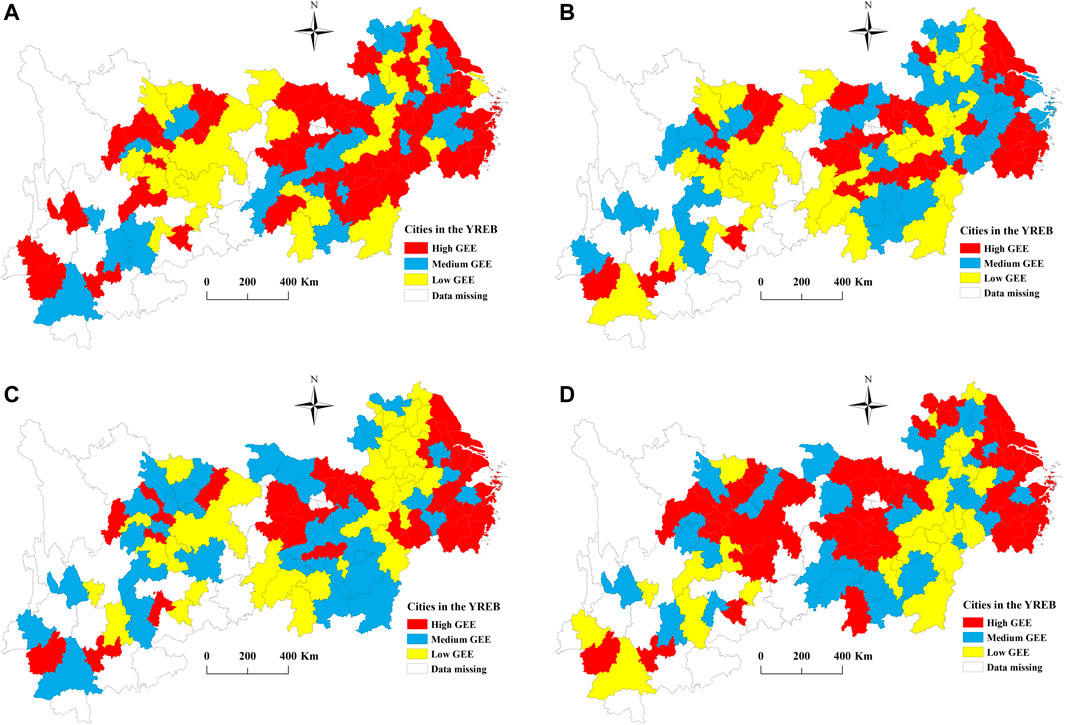
FIGURE 3. Distribution of low (yellow), medium (blue), and high (red) grades of GEE in the Yangtze River Economic Belt. It is made by Arcgis 10.6 and shown in (A) 2003, (B) 2008, (C) 2013, and (D) 2018, respectively.
In total, 26 cities, including Huai’an, Quzhou, Yichang, and Bengbu, lagged in GEE in 2003. The cities with a medium degree of GEE were Xuzhou, Yangzhou, and 27 other regions. The remaining cities mostly had a relatively higher level of GEE, and many of them were in backward economic development stages with weak industrial sectors. These cities entered a period of accelerated economic development after 2003.
In 2008, the relative differences of GEE significantly narrowed; 38 cities were in the medium GEE development period, and 33 cities, most of which were in the midstream of YREB in 2008, lagged in GEE. The remaining regions, including Wuxi, Taizhou, and Nanchang, also advanced in GEE. Based on the GEE results between 2003 and 2008, it can be concluded that most cities were paying more attention to the harmonious development of economic and ecological systems, consequently narrowing regional differences. From a spatial perspective, downstream cities such as Wuxi and Taizhou improved considerably, while midstream cities showed insignificant improvement.
Overall, 33 cities, mostly agglomerated in Anhui Province, in the midstream of YREB, lagged in GEE after 2008. 40 cities (including Xinyu, Yichun et al.) remained in the medium development period around the same time. The remaining 35 cities, including 16 regions in the downstream, 11 cities in the midstream, and eight cities in the upstream, also elevated their GEE level. Based on the GEE rates from 2008 to 2013, it can be concluded that Anhui Province and its upstream cities developed slowly in GEE, limited by the economic crisis since 2008. Midstream cities still need time to upgrade their heavy chemical industry.
In 2018, 24 cities, including Wuhu and Chuzhou, lagged in GEE. The cities with a medium degree of GEE were Huai’an, Shaoxing, and 36 other locations. The remaining 48 cities had a relatively higher level of GEE, many of them being big hubs and middle-sized areas. Based on the GEE results from 2013 to 2018, it can be concluded that spatial characteristics significantly changed during this period. From a spatial perspective, big cities such as Shanghai and Chongqing improved preferentially, tightly followed by medium-size cities. On the other hand, small-sized cities showed minor improvements. Finally, urban agglomerations in the three basins were constantly forming and strengthening.
4.2 Spatial Correlation Test of the Green Economic Efficiency
To gain insights into how these differences develop with economic growth, we conducted a convergence test on the GEE. It was necessary to clarify the spatial correlation of GEE to make the procedure more accurate. From Table 2, it was concluded that Moran’s I index of GEE in the YREB was greater than 0, and most of Moran’s I index passed the 5% significance level test. The results showed that there was a strong spatial autocorrelation in the YREB and proved the existence of typical spatial positive correlation distribution characteristics of the high–high and low–low agglomeration. The test could verify that the spatial interaction between regions played an important role in the green economic growth of the YREB and the correctness of our methodology using the dynamic spatial Durbin panel model. Moran’s I index was the highest under the information sharing weight matrix and the lowest under the capital liquidity weight matrix. Furthermore, the green economic growth of the YREB under the information exchange weight matrix showed more significant spatial agglomeration and spatial dependence characteristics.
4.3 Spatial Convergence of the Green Economic Efficiency
Table 3 reports the convergence test results of the GEE in the YREB under full samples. Columns 1 and 2 are regressions based on Eq. 3 without considering spatial effects, and based on the traditional ordinary least square (OLS) estimation method. Columns 3–8 are the regressions based on Eq. 5 considering spatial effects, and columns 3 and 4 are regressions under the adjacency weight and geographic distance weight matrices. Columns 5 to 8 are regressions under the weights of economic linkage, capital liquidity, talent mobility, and information sharing. For Eq. 5, when the spatial term was included, the estimator obtained using OLS was biased and inconsistent. Therefore, we chose maximum likelihood estimation (MLE) to estimate the model and introduce the lag period of the variables, effectively solving the issue with missing variables and endogeneity in the model.
According to column 1 of Table 3, when the spatial effect is not considered, the estimated value of the regression coefficient in an absolute convergence test model is less than 0, so it passed the 1% level of significance tests. This shows that from 2003 to 2018, there was a global convergence in the GEE of YREB, and the overall convergence rate reached 6.34%, with a half-life cycle of about 11 years. The regression results obtained by the dynamic panel data model also support the existence of GEE’s global convergence in the YREB. However, the overall convergence rate has accelerated to 8.56%, and the corresponding half-life cycle has been shortened to about 8 years. According to columns 3–8, when considering spatial effects, the regression coefficients of the spatial terms in the model under the spatial weight matrix passed the 1% level of significance tests. This shows that the spatial measurement model is more suitable than the traditional one. The regression results under the spatial network weight matrix show that the spatial interaction between different regions has strengthened the communication and connection between each other. It promoted the green economic growth rate of the backward areas and made it faster than that of the rich regions.
In terms of convergence speed, the model regression results under different spatial networks show slight differences. The regression result of the model based on the capital liquidity weight matrix reached the highest convergence rate of 8.28%, with a half-life cycle of about 8.5 years. The convergence rate of the model regression results under the weights of economic linkage, talent mobility, and information sharing were roughly the same: 7.46, 7.76, and 7.73%, respectively, with half-life cycles of about 9.5, 9, and 9 years. The reason for this phenomenon is that different spatial network weights represent different ways of spatial interaction. Although there are solid developmental differences between regions, the three basins of the YREB are highly integrated and correlated. In this context, economic development has led to a strong convergence trend of green economic growth in the form of spatial interaction. In addition, the different convergence speeds of regional economic growth under different weights are also related to the strength of interregional interaction. Therefore, we could further examine the convergence trend of economic growth among subregions.
Regarding the control variables, the coefficients of government governance and openness are negative and pass the 1% significance test, showing that they have a positive impact on convergence. This is mainly due to the Chinese government’s targeted poverty alleviation and counterpart assistance policies. These policies have promoted the development of underdeveloped areas, for example, Chinese photovoltaic poverty alleviation projects (Han et al., 2020), and the opening up initiatives have increased resource sharing, for example, capital-intensive land investments in Africa (Bergius et al., 2018). These movements are conducive to the green development of undeveloped regions. However, the coefficient of industrial upgrading is positive and also passes the 1% significance test, indicating that it has a negative impact on convergence. In this case, the impact of economic growth is not evident. Industrial upgrades in developed regions adopt low-carbon and environmentally friendly technologies that can increase productivity, save resources, and reduce pollution. However, environmental pollution caused by economic growth affects the development of the green economy, and the effect of this context on convergence remains uncertain.
4.4 Regional Spatial Convergence of Green Economic Efficiency
According to Table 4, the three basins in four weight matrices all support the conditional β-convergence trend of the green economic growth. The downstream had the fastest convergence rate, with an average of 11.48%. The midstream and upstream had roughly the same convergence rate, 8.09 and 7.97%, respectively. The rapid convergence rate of the downstream was mainly due to the similar degree of urban economic development. The small overall gap, complete traffic network, strong industrial relevance, close regional economic ties, and a high degree of integration most likely led to the positive spatial spillover effects. However, only the spatial regression coefficients of the explained variables in the downstream were significant at the 1% statistical level, while the other regression coefficients were not significant. This shows that the convergence trend in the three basins was not linked to the spatial correlation between club members, indicating that the correlation between cities is weak.
Furthermore, the coefficients of control variables in the three basins are considerably different. For the upstream and midstream cities, the coefficient of opening up is negative and has passed the 1% significance test, showing that opening up is conducive to narrowing the gap and promoting convergence in the two basins. For the downstream cities, the coefficient of government governance is negative and has also passed the 1% significance test. This means that local governments can further improve the governance effectiveness, strengthen interconnection, and narrow the gap between central and fringe cities.
5 Discussion and Conclusion
5.1 Discussion
First, we measured the GEE in the YREB and studied regional differences and sources of the region’s GEE. The results confirmed that GEE declined from the central to marginal cities while forming three high-efficiency green economic zones in the upstream, midstream, and downstream, respectively. Our conclusion is consistent with the observations made by Ye et al. (2021), who reported that the YREB presented “block” green development characteristics characterized by urban agglomerations. In addition, we could also verify that the contribution of technological progress to green growth was greater than technological efficiency. These results were consistent with conclusions presented by He et al. (2021) and Albort-Morant et al. (2016), who claimed that green technological innovation plays an essential role in reducing or avoiding the destruction of the ecological environment, further helping improve the green economy. This provides a theoretical basis for green development through promoting technological progress.
Second, we observed that GEE in the YREB follows a global convergence trend, in which the average convergence rate is 7.81%. This outcome is consistent with the economic growth convergence theory of neoclassical economics (Solow, 1956). Chen et al. (2020) also proved that the YREB had prominent β-convergence characteristics overall, which means that the green economy in poor areas was growing faster than in wealthy areas. The speed of convergence we identified was faster than the calculation results of Liu and Jia (2019a), who calculated the convergence rate of China’s regional economic growth as ranging between 0.4 and 2.3%. Moreover, the speed detected by our methodology was also faster than the results of Barro et al. (1991), who calculated the convergence rate of the traditional economic growth as 2%. There are mainly two reasons why our results are not consistent with those reported in previous studies. On the one hand, the research scope of our study is the Yangtze River Economic Belt, and its regional gap is smaller than that of China. On the other hand, existing studies have neglected the resource and environment sacrificed by developed regions for economic growth. However, resource and environmental issues cannot be ignored in this context because they also affect the gap between developed and underdeveloped regions. Furthermore, from the perspective of spatial connection, GEE had the fastest convergence rate in capital liquidity connection. This result was consistent with that of Liu and Jia (2019a), who believed that capital liquidity was an important transmission channel for economic spillover effects.
5.2 Conclusions
Taking into account the ecological protection, sustainability of economic growth is the general trend of regional development. Through the combination of the super-efficiency EBM and the Malmquist–Luenberger index model, this study proposes a novel methodology to accurately measure GEE and its decomposition components in the YREB. Subsequently, we verified the regional convergence of GEE from four spatial correlation networks and explored its main factors. Our results show that the main source of GEE is technological progress. On average, technological progress contributed 0.24% to GEE, triggering the formation of spatial differentiation in the upstream, midstream, and downstream. Convergence analysis shows that economic linkage, capital liquidity, talent mobility, and information sharing can promote GEE’s convergence. A subsequential heterogeneous analysis showed that GEE has a stronger convergence trend in the downstream, where the linkage is stronger. Finally, capital liquidity presented as an important transmission channel for the GEE’s convergence and an analysis of influencing factors showed that government intervention and opening up are equally important in this context. However, economic growth and industrial structure upgrading may restrain this process.
Based on the conclusions previously presented, we outline some policy recommendations that may contribute to the green and coordinated development. First, under the background of accelerating technological change, the Chinese authorities should focus on initiatives that can promote technological progress. According to the findings of our study, technological progress promotes green development. Local construction should be guided by innovation and the gradual introduction of new, innovative protection policies. Preferential policies should also be under constant discussion, for example, fiscal subsidies, tax reductions, and exemptions to innovative enterprises. At the same time, the Chinese government should support and encourage the development of green technologies, promote cleaner production, and strengthen legislation and policy guarantees, especially low-carbon technologies. It is equally important to make full use of modern information technologies, that is, communication technology and Internet sharing platform to reduce the threshold of green innovation and promote the transformation of green scientific and technological achievements. Moreover, the government should attract modern high-tech industries and offer possibilities for the green transformation of key industries and fields. Finally, there is the need to highlight the improvement of technical efficiency, management, and business environment and give a solid space to the decisive role of the market in resource allocation.
Second, it is necessary to strengthen the coordination and linkage between cities in the YREB. On the one hand, our analysis of influencing factors showed that government intervention and openness could promote GEE convergence, so the role of public authorities in coordinating all parties is essential in this context. The Chinese government should speed up the transformation and upgrading of the industrial structure and the rational allocation of resources while adjusting the environmental regulation and investment structure. This can be possible by setting up special institutions to uniformly arrange and coordinate water resources distribution, river regulation, and other affairs. At last, the authorities in the midstream and downstream should give appropriate compensation to the upstream, such as financial subsidies, talent training, and paired assistance for their expenses, to protect the ecological environment. Once the local YREB governance realizes the complementarity of functions and industrial fit between cities, it will be possible to further promote the interactive growth of urban green development and coordinated development.
Third, relying on the spillover effect under the spatial connection network, local authorities should give full play to the important role of economic connection, capital liquidity, talent mobility, and information sharing. These features are available to establish a shared mechanism, acknowledge official documents among local governments, and improve traffic accessibility to facilitate the flow of elements. On the one hand, regions should improve the overall level of development in the establishment of closer ties. In terms of technology and talents, it is of great significance to ensure the cultivation and supply of research talents in the frontiers of technology and basic science. It is also important to encourage investment attraction and opening-up focusing on green innovation. Capital flows are essential channels to obtain financial support by establishing a green financial system that enables green investment, leveraging the role of private capital. On the other hand, backward regions need to be vigilant that developed regions are exploiting talents, capital, industries, and other elements. This disadvantage requires them to develop and expand featured industries based on competitive elements and endowments and make efforts to optimize their business and ecological environment and improve their infrastructure.
This study extensively measures the GEE and explores the convergence of GEE in the YREB based on economic linkage, capital liquidity, talent mobility, and information sharing. The results show the existence of convergence trends, which means that the green economy in poor areas is growing faster than in rich areas and the gap between regions will gradually narrow. Meanwhile, there are factors such as government governance and opening-up that are conducive to narrowing the gap. The outcomes of our research pointed out to three directions that deserved to be investigated by complementary studies. First, we empirically verified the convergence of GEE under different spatial correlation networks, but we did not analyze the dynamic spatial correlation networks that are changing over time. Therefore, further study can incorporate dynamic spatial correlation networks into research to explore the impact of changes in the mobility of different factors on regional development gaps. Second, this study investigates the measurement and convergence of GEE. More in-depth analyses can be useful to measure and compare convergence in the inclusive green economic efficiency, emphasizing equal opportunities and social equity, as well as efficient and affordable green growth. Finally, considering the goals of “carbon peaking” and “carbon neutrality” that were put forward in 2020, reducing carbon emissions is a very urgent task. Further research can strengthen the knowledge on carbon emission policies, rights trading, and targets affecting the green economic efficiency and promote green development from the perspective of carbon reduction.
Data Availability Statement
The raw data supporting the conclusion of this article will be made available by the authors, without undue reservation.
Author Contributions
Conceptualization: SL; methodology: LS; software: LS; validation: YH; formal analysis: LS; investigation: LS; resources: YH; writing—original draft preparation: LS; writing—review and editing: YH and LS; supervision: SL; project administration: SL; and funding acquisition: SL. All authors have read and agreed to the published version of the manuscript.
Funding
This study was funded by the National Social Science Foundation of China (No. 15ZDB170) and Key Research Program of National Social Science Fund of China (No. 18VSJ067).
Conflict of Interest
The authors declare that the research was conducted in the absence of any commercial or financial relationships that could be construed as a potential conflict of interest.
Publisher’s Note
All claims expressed in this article are solely those of the authors and do not necessarily represent those of their affiliated organizations, or those of the publisher, the editors, and the reviewers. Any product that may be evaluated in this article, or claim that may be made by its manufacturer, is not guaranteed or endorsed by the publisher.
Acknowledgments
The authors would like to thank reviewers who provided many helpful suggestions for improvements. The authors would also like to thank the editor for editing and comments.
References
Aguiar, F. C., Bentz, J., Silva, J. M. N., Fonseca, A. L., Swart, R., Santos, F. D., et al. (2018). Adaptation to Climate Change at Local Level in Europe: An Overview. Environ. Sci. Pol. 86, 38–63. doi:10.1016/j.envsci.2018.04.010
Ahmed, Z., Asghar, M. M., Malik, M. N., and Nawaz, K. (2020). Moving towards a Sustainable Environment: The Dynamic Linkage between Natural Resources, Human Capital, Urbanization, Economic Growth, and Ecological Footprint in China. Resour. Pol. 67, 101677. doi:10.1016/j.resourpol.2020.101677
Albort-Morant, G., Leal-Millán, A., and Cepeda-Carrión, G. (2016). The Antecedents of green Innovation Performance: A Model of Learning and Capabilities. J. Business Res. 69, 4912–4917. doi:10.1016/j.jbusres.2016.04.052
Andersen, P., and Petersen, N. C. (1993). A Procedure for Ranking Efficient Units in Data Envelopment Analysis. Manage. Sci. 39, 1261–1264. doi:10.1287/mnsc.39.10.1261
Anselin, L. (1988). Lagrange Multiplier Test Diagnostics for Spatial Dependence and Spatial Heterogeneity. Geogr. Anal. 20, 1–17. doi:10.1111/j.1538-4632.1988.tb00159.x
Bai, J., and Xia, K. Y. (2019). Government Intervention, Regional Differences and green Economy Efficiency Measurement-Based on Data from 107 Cities at the Prefecture Level and above in the Yangtze River Economic Belt. Jianghan Tribune 62, 21–27. doi:10.3969/j.issn.1003-854X.2019.07.003
Barro, R. J., Sala-I-Martin, X., Blanchard, O. J., and Hall, R. E. (1991). Convergence across States and Regions. Brookings Pap. Econ. Activity 1991, 107–182. doi:10.2307/2534639
Bergius, M., Benjaminsen, T. A., and Widgren, M. (2018). Green Economy, Scandinavian Investments and Agricultural Modernization in Tanzania. J. Peasant Stud. 45, 825–852. doi:10.1080/03066150.2016.1260554
Bina, O. (2013). The green Economy and Sustainable Development: An Uneasy Balance? Environ. Plann. C Gov Pol. 31, 1023–1047. doi:10.1068/c1310j
Blampied, N. (2021). Economic Growth, Environmental Constraints and Convergence: The Declining Growth Premium for Developing Economies. Ecol. Econ. 181, 106919. doi:10.1016/j.ecolecon.2020.106919
Buseth, J. T. (2017). The Green Economy in Tanzania: From Global Discourses to Institutionalization. Geoforum 86, 42–52. doi:10.1016/j.geoforum.2017.08.015
Cai, X. W., Wang, W. J., Rao, A., Rahim, S., and Zhao, X. (2022). Regional Sustainable Development and Spatial Effects from the Perspective of Renewable Energy. Front. Environ. Sci. 10, 859523. doi:10.3389/fenvs.2022.859523
Che, L., Bai, Y. P., Zhou, L., Wang, F., Ji, X. P., and Qiao, F. W. (2018). Spatial Pattern and Spillover Effects of Green Development Efficiency in China. Sci. Geogr. Sin. 38, 1788–1798. doi:10.13249/j.cnki.sgs.2018.11.006
Chen, G. N., Yang, Z. H., and Chen, S. H. (2020). Measurement and Convergence Analysis of Inclusive Green Growth in the Yangtze River Economic Belt Cities. Sustainability 12, 2356. doi:10.3390/su12062356
Chen, Z., Kahn, M. E., Liu, Y., and Wang, Z. (2018). The Consequences of Spatially Differentiated Water Pollution Regulation in China. J. Environ. Econ. Manage. 88, 468–485. doi:10.1016/j.jeem.2018.01.010
Chung, Y. H., Färe, R., and Grosskopf, S. (1997). Productivity and Undesirable Outputs: A Directional Distance Function Approach. J. Environ. Manage. 51, 229–240. doi:10.1006/jema.1997.0146
Desli, E., and Gkoulgkoutsika, A. (2021). Economic Convergence Among the World's Top-Income Economies. Q. Rev. Econ. Financ. 80, 841–853. doi:10.1016/j.qref.2019.03.001
Dmuchowski, P., Dmuchowski, W., Baczewska-Dabrowska, A. H., and Gworek, B. (2021). Green Economy - Growth and Maintenance of the Conditions of Green Growth at the Level of Polish Local Authorities. J. Clean. Prod. 301, 126975. doi:10.1016/j.jclepro.2021.126975
Färe, R., Grosskopf, S., Lindgren, B., and Roos, P. (1992). Productivity Changes in Swedish Pharamacies 1980-1989: A Non-Parametric Malmquist Approach. Int. Appl. Prod. Effic. Anal. 3, 81–97. doi:10.1007/978-94-017-1923-0_6
Ganegoda, N. C., Wijaya, K. P., Amadi, M., Erandi, K. K. W. H., and Aldila, D. (2021). Interrelationship between Daily COVID-19 Cases and Average Temperature as Well as Relative Humidity in Germany. Sci. Rep. 11, 11302. doi:10.1038/s41598-021-90873-5
Gao, Y. Y., Xu, X. Y., Wang, H. R., Gao, X., and Yin, X. L. (2013). New Model for Water Use Efficiency Evaluation of China and its Application. Syst. Eng. 33, 776–784. doi:10.12011/1000-6788(2013)3-776
Geary, R. C. (1954). The Contiguity Ratio and Statistical Mapping. The Incorporated Statistician 5, 115–145. doi:10.2307/2986645
Getis, A., and Ord, J. K. (1992). The Analysis of Spatial Association by Use of Distance Statistics. Geographical Anal. 24, 189–206. doi:10.1111/j.1538-4632.1992.tb00261.x
Han, M. Y., Xiong, J., Wang, S. Y., and Yang, Y. (2020). Chinese Photovoltaic Poverty Alleviation: Geographic Distribution, Economic Benefits and Emission Mitigation. Energy Policy 144, 111685. doi:10.1016/j.enpol.2020.111685
Han, M. Y., Liu, W. D., Xie, Y. T., and Jiang, W. B. (2021). Regional Disparity and Decoupling Evolution of China's Carbon Emissions by Province. Resour. Sci. 43, 710–721. doi:10.18402/resci.2021.04.06
Hao, G. C., Xu, Y. L., Zhang, X. M., and Chen, M. H. (2018). The Spillover Effect and Decomposition of Urban Green Economic Performance in the Yangtze River Economic Belt. China Pop. Resour. Environ. 28, 75–83. doi:10.12062/cpre.20171204
He, J. X., Wang, L. X., and Tang, D. C. (2021). Research on Green Total Factor Productivity of Yangtze River Economic Belt Based on Environmental Regulation. Int J Environ. Res. Public Health 18, 12242. doi:10.3390/ijerph182212242
Hou, Z. H., Liu, Z. B., and Yue, Z. G. (2009). Social Network Analysis over the Process of Economic Integration in the Yangtze River Delta. China Soft Sci. 24, 90–101. doi:10.3969/j.issn.1002-9753.2009.12.012
Islam, N. (1998). Growth Empirics: A Panel Data Approach--A Reply. Q. J. Econ. 113, 325–329. doi:10.1162/003355398555513
Kinfemichael, B., and Morshed, A. K. M. M. (2019). Convergence of Labor Productivity across the US States. Econ. Model. 76, 270–280. doi:10.1016/j.econmod.2018.08.008
Li, G., Zhou, Y., Liu, F., and Wang, T. (2021). Regional Differences of Manufacturing green Development Efficiency Considering Undesirable Outputs in the Yangtze River Economic Belt Based on Super-SBM and WSR System Methodology. Front. Environ. Sci. 8, 631911. doi:10.3389/fenvs.2020.631911
Li, H., and Wang, Y. X. (2014). Financial Agglomeration, Spatial Spillover, and Urban Economic Growth: An Empirical Study Based on the Dubin Model of 286 Urban Space Panel. Studi. Int. Financ. 31, 89–96.
Li, J., Chen, P., Wan, G. H., and Fu, C. M. (2014). Study on the Spatial Correlation and Explanation of Regional Economic Growth in China——Based on Analytic Network Process. Econ. Res. 49, 4–16.
Li, J. L., and Xu, B. (2018). "Curse" or "Gospel": How Does the Abundance of Resources Affect China's green Economic Growth? Econ. Res. 53, 151–167.
Li, W. B., and Tu, L. (2017). Spatial Difference and Convergence of Green Total Factor Productivity in Chinese Cities. Urban Probl. 36, 55–63. doi:10.13239/j.bjsshkxy.cswt.170908
Lin, B. Q. (2003). Electricity Consumption and China's Economic Growth: A Study Based on Production Function. Manage. World 19, 18–27. doi:10.19744/j.cnki.11-1235/f.2003.11.003
Lin, X., Xu, W., Yang, F., and Zhao, L. (2017). Spatio-Temporal Characteristics and Driving Forces of green Economic Efficiency in Old Industrial Base of Northeastern China: A Case Study of Liaoning Province. Econ. Geogra. 37, 125–132. doi:10.15957/j.cnki.jjdl.2017.05.017
Lin, Y. T., Huang, R. T., and Yao, X. (2021). Air Pollution and Environmental Information Disclosure: An Empirical Study Based on Heavy Polluting Industries. J. Clean. Prod. 278, 124313. doi:10.1016/j.jclepro.2020.124313
Liu, H. J., and Jia, W. X. (2019a). Convergence Test and Coordinated Development of China’s Regional Economic Growth under the Background of Different Spatial Network. Nankai Econ. Stud. 35, 104–124. doi:10.14116/j.nkes.2019.03.006
Liu, H. J., and Jia, W. X. (2019b). Spatial Network Correlation and Convergence Test of Regional Economic Growth in China. Sci. Geogra. Sin. 39, 726–733. doi:10.13249/j.cnki.sgs.2019.05.003
Liu, S. G., and Shang, Y. S. (2020). Green Development Efficiency Evaluation and Obstacle Factor Analysis of Coastal City Groups in east China. Urban Probl. 39, 73–80. doi:10.13239/j.bjsshkxy.cswt.200108
Loiseau, E., Saikku, L., Antikainen, R., Droste, N., Hansjürgens, B., Pitkänen, K., et al. (2016). Green Economy and Related Concepts: An Overview. J. Clean. Prod. 139, 361–371. doi:10.1016/j.jclepro.2016.08.024
Lu, W. L., Song, D. Y., and Huang, C. (2017). Measurement on the Green Total Factor Productivity of the Yangtze River Economic Belt: Taking 108 Cities for Example. Urban Probl. 36, 61–67. doi:10.13239/j.bjsshkxy.cswt.170108
Lv, B. Y., and Yu, D. L. (2009). Improving Economic Efficiency within the Framework of China's Tiered Development Model——An analysis from a Spatial Perspective. Soc. Sci. China. 29, 60–72.
Mankiw, N. G., Romer, D., and Weil, D. N. (1992). A Contribution to the Empirics of Economic Growth. Q. J. Econ. 107, 407–437. doi:10.2307/2118477
Maran, R. M., and Nedelea, A. M. (2017). Green Economy: Challenges and Opportunities. EcoForum 6, 1–4.
Moran, P. A. P. (1948). The Interpretation on Statistical Maps. J. R. Stat. Soc. B. 10, 243–251. doi:10.1111/j.2517-6161.1948.tb00012.x
Peng, B. H., Wang, Y. Y., and Wei, G. (2020). Energy Eco-Efficiency: Is There Any Spatial Correlation Between Different Regions? Energy Policy 140, 111404. doi:10.1016/j.enpol.2020.111404
Qian, Z. M., and Liu, X. C. (2014). The Spatial Evolution of Green Economic Efficiency with Resource and Environmental Constraint. J. Jilin Univ. 54, 31–39. doi:10.15939/j.jujsse.2014.05.001
Ramajo, J., Márquez, M. A., Hewings, G. J. D., and Salinas, M. M. (2008). Spatial Heterogeneity and Interregional Spillovers in the European Union: Do Cohesion Policies Encourage Convergence across Regions? Eur. Econ. Rev. 52, 551–567. doi:10.1016/j.euroecorev.2007.05.006
Shao, C. D., and Su, D. N. (2017). The Spatial Spillover Effect of Global Value Chain on Productivity. China Ind. Econ. 35, 94–114. doi:10.19581/j.cnki.ciejournal.2017.04.006
Solow, R. M. (1956). A Contribution to the Theory of Economic Growth. Q. J. Econ. 70, 65–94. doi:10.2307/1884513
Tang, J., and Qin, F. M. (2021). Analyzing the Effect of Local Government Competition on Green Total Factor Productivity From the Market Segmentation Perspective in China-Evidence From a Three-Stage DEA Model. Front. Environ. Sci. 9, 763945. doi:10.3389/fenvs.2021.763945
Tickner, D., Parker, H., Moncrieff, C. R., Oates, N. E. M., Ludi, E., and Acreman, M. (2017). Managing Rivers for Multiple Benefits-A Coherent Approach to Research, Policy and Planning. Front. Environ. Sci. 5, 4. doi:10.3389/fenvs.2017.00004
Tone, K., and Tsutsui, M. (2010). An Epsilon-Based Measure of Efficiency in DEA - A Third Pole of Technical Efficiency. Eur. J. Oper. Res. 207, 1554–1563. doi:10.1016/j.ejor.2010.07.014
Tong, Q. Y., Sun, B., Dong, X., and Gao, J. (2019). Statistical Assessment and Determinant Analysis of the Pollutant Removal Efficiencies of Wastewater Treatment Plants. China Pop. Resour. Environ. 29, 49–57. doi:10.12062/cpre.20181007
UNEP (2011). Towards a Green Economy: Pathways to Sustainable Development and Poverty Eradication. Nairobi, Kenya: UNEP.
Wang, H., Yan, X. Y., and Wu, J. S. (2021). Free Utility Model for Explaining the Social Gravity Law. J. Stat. Mech. 2021, 033418. doi:10.1088/1742-5468/abee18
Wang, Z. B., Wang, X. M., and Liang, L. W. (2019). Green Economic Efficiency in the Yangtze River Delta: Spatio-Temporal Evolution and Influencing Factors. Ecosyst. Health Sust. 5, 20–35. doi:10.1080/20964129.2018.1559000
World Bank (2012). Inclusive Green Growth: The Pathway to Sustainable Development. Washington D.C: World Bank.
Wu, Y., Cui, Z. X., and Hu, C. (2021). Does the New Urbanization Influence Air Quality in China? Front. Environ. Sci. 9, 645010. doi:10.3389/fenvs.2021.645010
Wu, Z. J., and Wu, N. J. (2021). Research on the Measurement, Decomposition and Influencing Factors of Green Economic Efficiency in the Yangtze River Economic Belt: Based on Super-Efficiency SBM-ML-Tobit Model. Urban Probl. 40, 52–62+89. doi:10.13239/j.bjsshkxy.cswt.210107
Xu, H., and Zhao, Y. (2015). Research on Economic Growth Convergence of Yangtze River Economic Belt Based on Spatial Model. Soft Sci. 29, 77–81. doi:10.13956/j.ss.1001-8409.2015.08.17
Yang, L., and Hu, X. Z. (2010). Analysis on Regional Difference and Convergence of the Efficiency Of China’s Green Economy Based on DEA. Economist 22, 46–54. doi:10.16158/j.cnki.51-1312/f.2010.02.006
Yang, Z. J., and Wen, C. X. (2017). Evaluation on China's Green Development Efficiency and Regional Disparity. Econ. Geogra. 37, 10–18. doi:10.15957/j.cnki.jjdl.2017.03.002
Ye, T. F., Zheng, H., Ge, X. Y., and Yang, K. L. (2021). Pathway of Green Development of Yangtze River Economics Belt From the Perspective of Green Technological Innovation and Environmental Regulation. Int. J. Environ. Res. Public Health 18, 10471. doi:10.3390/ijerph181910471
Young, A. (2003). Gold into Base Metals: Productivity Growth in the People's Republic of China during the Reform Period. J. Polit. Economy 111, 1220–1261. doi:10.1086/378532
Yu, J. H., and Lee, L. F. (2012). Convergence: A Spatial Dynamic Panel Data Approach. Glob. J. Econ. 1, 1250006. doi:10.1142/S2251361212500061
Zhang, G. S., Zhang, X., Xie, L., Zhang, Q., Liu, D. S., Wu, H. J., et al. (2021). Perceived Importance and Bundles of Ecosystem Services in the Yangtze River Middle Reaches Megalopolis, China. Front. Environ. Sci. 9, 739876. doi:10.3389/fenvs.2021.739876
Zhang, H., Gao, J. X., and Qiao, Y. J. (2019). The Ecological Environment Situation, Problems and Suggestions in the Yangtze River Economic Zone. Environ. Sustain. Develop. 44, 28–32. doi:10.19758/j.cnki.issn1673-288x.201905028
Zhang, Y. T., and Yang, L. (2020). National Financial Development of the “Belt and Road” Countries and Efficiency of China’s Foreign Direct Investment. J. Quant. Tech. Econ. 37, 109–124. doi:10.13653/j.cnki.jqte.2020.02.006
Zhang, Z., and Zhou, H. (2012). Spillover Effect and Industrial Differences in Transport Infrastructure - Based on a Comparison of Spatial Econometric Analysis. J. Financ. Econ. 38, 124–134. doi:10.16538/j.cnki.jfe.2012.03.006
Zheng, C. Y., Zhu, Y. H., and Cheng, F. (2018). Does Urbanization Boost the Green Total Factor Productivity? ——An Empirical Study Based on Yangtze River Economic Belt. Mod. Econ. Res. 37, 110–115. doi:10.13891/j.cnki.mer.2018.05.015
Zhou, B., Zeng, X. Y., Jiang, L., and Xue, B. (2020). High-Quality Economic Growth Under the Influence of Technological Innovation Preference in China: a Numerical Simulation from the Government Financial Perspective. Struct. Change Econ. Dyn. 54, 163–172. doi:10.1016/j.strueco.2020.04.010
Keywords: green economic efficiency, convergence test, spatial network correlation, Yangtze River Economic Belt, coordinated development
Citation: Liu S, Song L and Huang Y (2022) Measurement and Convergence Test of Green Economic Efficiency of the Yangtze River Economic Belt Under Different Spatial Network Correlation. Front. Environ. Sci. 10:828207. doi: 10.3389/fenvs.2022.828207
Received: 03 December 2021; Accepted: 28 March 2022;
Published: 19 May 2022.
Edited by:
Mengyao Han, Chinese Academy of Sciences (CAS), ChinaReviewed by:
Rafael Alvarado, National University of Loja, EcuadorDervis Kirikkaleli, European University of Lefka, Turkey
Xiaoqi Sun, Shenzhen University, China
Copyright © 2022 Liu, Song and Huang. This is an open-access article distributed under the terms of the Creative Commons Attribution License (CC BY). The use, distribution or reproduction in other forums is permitted, provided the original author(s) and the copyright owner(s) are credited and that the original publication in this journal is cited, in accordance with accepted academic practice. No use, distribution or reproduction is permitted which does not comply with these terms.
*Correspondence: Yue Huang, MTgwMTYwODcxMkBxcS5jb20=