- 1Indian Institute of Tropical Meteorology (IITM), Pune, India
- 2Department of Physics, Sri Venkateswara University (SVU), Tirupati, India
- 3Centre of Excellence in Ocean-Atmospheric Science and Technology (ACOAST)/Environmental Science and Health (ACESH), Amity University Haryana (AUH), Gurugram, India
In this study, we investigate the temporal variations in columnar aerosol pollutants and their possible association with the simultaneously measured black carbon (BC) aerosol mass concentration and associated biomass burning (BB) over urban (Delhi) and rural (Panchgaon) sites during the lockdown phases of the COVID-19 pandemic. We also show the impact of lockdown measures on boundary layer ozone and its primary precursors, NO2, and water vapor (H2O), potent greenhouse gases that destroy protective ozone. For this purpose, we used multiple datasets, namely, black carbon (BC) aerosol mass concentration and biomass burning (BB) aerosols using an aethalometer at Amity University Haryana (AUH), Panchgaon, India, and satellite retrievals from NASA’s MODIS and OMI at both the stations. The analysis was conducted during the pre-lockdown period (1–25 March), lockdown 1st phase (25 March–14 April), lockdown 2nd phase (15 April–3 May), lockdown 3rd phase (4–17 May), lockdown 4th phase (18–31 May), and post-lockdown (1–30 June) period in 2020. Our diagnostic analysis shows a substantial reduction in AOD (Delhi: −20% to −80%, Panchgaon: −20% to −80%) and NO2 (Delhi: −10% to −42.03%, Panchgaon −10% to −46.54%) in comparison with climatology (2010–2019) during all four phases of lockdown. The reduction in AOD is attributed to lockdown measures and less transport of dust from west Asia than climatology. Despite a reduction in NO2, there is an increase in the ozone amount (Delhi: 1% to 8% and Panchgaon: 1% to 10%) during lockdown I, II, and III phases. The observed enhancement in ozone may be resultant from the complex photochemical processes that involve the presence of NO2, CO, volatile organic compounds (VOCs), and water vapor. The reduction in AOD and NO2 and enhancement in ozone are stronger at the rural site, Panchgaon than that at the urban site, Delhi.
Introduction
The novel coronavirus first detected in late December 2019 in Wuhan, China, has eventually become a deadly virus, which is highly transmissible, along with a high mortality rate (Suresh et al., 2020). Due to its rapid spread and deadly nature, several people were affected within a month (Bull et al., 2020; He et al., 2020); it is considered as one of the major disasters, which has affected the whole world. In early March 2020, the World Health Organization (WHO) named the coronavirus as COVID-19 and declared this COVID-19 as a pandemic. After the outbreak of this COVID-19, it affected the world in a gradual manner (Raibhandari et al., 2020) mainly through cross-border travels. As the numbers of COVID-19 cases were increasing, the Prime Minister of India asked all citizens across the country to observe a 14-h public curfew on 22 March 2020. Following this, the government of India ordered a complete nationwide lockdown for 21 days, starting from midnight of 24 March 2020. To handle the worsening of the pandemic in the country, the government urged the Indian states and citizens to strictly follow the social distancing measures as a preventive strategy (https://www.mha.gov.in/).
After detecting the cases of COVID-19 in late January 2020 in India, the COVID-19 was found significantly spared in various states of India. According to the Ministry of Health and Family Welfare (MoHFW), present situation on 13 October 2020, total infected cases were 35,985,920, mortality was 450,963, and the recovery cases of 33,320,057 were reported in entire India on https://www.mygov.in/covid-19/, translating into a case fatality rate of 1.33% (MoHFW, 2020). Maharashtra, Tamil Nadu, Delhi, and Gujarat reported 50% of India’s cases, and the number of cases increased in the northeast states. In order to respond to this novel threat and to limit the spread of a virus, the Indian government has taken various precautionary measures, such as large scale COVID-19 screening tests, quarantine, social distancing, wearing of a mask, and sanitization of hands. Furthermore, considering the seriousness of the disease, the measures were taken to impose a nationwide lockdown in entire India, which was not the case for other countries (Rimesh and Urmila, 2020).
Tocontrol the transmission of COVID-19 and to measure the outcome from the precautionary measures, initially, on 22 March 2020 a 1-day Janata (people’s) curfew, followed by a 21-day nationwide lockdown (25 March 2020 to 14 April 2020: lockdown phase (LDP)-1) was announced and thereafter in succession LDP-II: 15 April to 3 May 2020, LDP-II: 4 May to 17 May 2020, and LDP-IV: 18 May to 31 May 2020 have been imposed. As a result, many industries, transport, economic, and social activity were shut down. After that, to restart the economy, two unlock phases have also been announced, respectively, on ULP-I: 1 June 2020 to 30 June 2020, and ULP-II: 1 July 2020 to 31 July 2020. These various lockdown phases reduced the mortality rate of COVID-19, improved the environment and air quality in the country. Various researchers from the world have focused on finding the effects of COVID-19 lockdown on the atmosphere. Recent studies have reported the improvement in air quality due to restrictions placed during the lockdown.
Research from other countries, namely, Germany, France, Italy, and Spain, reported a drastic decrease in concentration levels of GHGs, NO2, PM2.5, and PM10 but spikes in ozone concentration. The researchers, all over the world, have indicated a significant reduction in greenhouse gases, PM10, PM2.5, CO, NO, NO2, NH3, NOx, and SO2 concentrations dropped during the lockdown period (for example, Devara et al., 2020). Ghosh and Ghosh (2020), Srivastava (2020) reviewed various studies and concluded the improvement in the air quality due to lockdown. Gautam (2020a), Fadnavis et al. (2021) analyzed NO2 data, derived from the satellite (Sentinel–5P) and reported a significant reduction in Asian (Kanniah et al., 2020) and European countries. In another study, Gautam (2020b) analyzed the data from the National Aeronautics and Space Administration (NASA) and reported a 50% reduction in the air quality of the Indian region. Gupta et al. (2020) reported a reduction in the concentration of that influenced the a in 40 μg/m3 in particulate matter (PM2.5 and 10 ppm in CO over New Delhi, India. Mahato et al. (2020) reported a 40–50% improvement in the air quality over New Delhi. Jain and Sharma (2020) reported a ∼30–80% reduction in pollutants levels in all the major cities of India. Bera et al. (2020) found a positive correlation between PM2.5 and the lethality related to COVID-19 in Kolkata. Ranjan et al. (2020), Ranjan et al. (2021) reported from their studies of aerosol optical depth (AOD) using MODIS data, a 45% reduction in AOD during the lockdown period as compared to 19-year (2000–2019) AOD long-term mean.
The northern part of India normally experiences a poor air quality and high levels of atmospheric pollution due to various reasons, such as vehicular emissions, industrial activity, brick-kilns, and crop-residue burning (Singh et al., 2004; Prasad et al., 2006; Srivastava et al., 2021). Correlations between deviations in different pollutant mass concentrations, the spread of COVID-19, social distancing, indoor pollution, trends in morbidity, and mortality during 2020 have been derived from studies of air pollution and synchronous local and long-range meteorological parameters (Devara et al., 2020, 2021).
During this complete lockdown, there was an improvement in the air quality and a reduction in pollutant parameters in this area too (Sonbawne et al., 2021). The present study deals with such pollution scenarios, lockdown episodes in Panchgaon (Manesar) and New Delhi (Noida), situated about 50 km apart in the northern part of India.
Data and Methodology
MODIS Satellite Measurements
The moderate-resolution imaging spectroradiometer (MODIS) is a scientific instrument (radiometer) onboarded the NASA Terra and Aqua satellite platforms. Both Terra and Aqua satellite platforms were launched in 1999 and 2002, respectively. These instruments offer a look at terrestrial, atmospheric, and ocean phenomenology for a wide and various communities of users throughout the world. Terra and Aqua satellites with MODIS instruments attached fly on the sun-synchronous orbits at 705 km altitude and pass over the same spot of the Earth at about the same local time every day, that is, 10:30 a.m. in the case of Terra and 1:30 p.m. for Aqua. MODIS measures reflected solar and emitted thermal radiation in a total of 36 bands ranging at a wavelength from 0.4 to 14.4 µm and at varying spatial resolutions (2 bands at 250 m, 5 bands at 500 m, and 29 bands at 1 km).
The MODIS radiance data are inverted into nearly 40 different products having applications in various fields of considerable interest and importance in the Earth’s system to study global dynamics of the Earth’s atmosphere, land, ice, clouds, and oceans (for example, King et al., 1999; Devara, 2017; Zambrano-Monserrate et al., 2020). In this study, a deep blue product of AOD at 550 nm and water vapor observations were used.
OMI Satellite Measurements
The ozone monitoring instrument (OMI) was launched in 2004 on a 705 km sun-synchronous polar orbit on the AURA satellite with an ascending node equator crossing time of 1:45 p.m. The OMI measures the solar radiation backscattered by the Earth’s atmosphere and surface over the entire wavelength range from 270 to 500 nm with a spectral resolution of about 0.5 nm. The wavelength ranges (ultraviolet: 270–365 nm and visible: 365–500 nm) are used to retrieve total column gases such as O3 and NO2. Furthermore, OMI gives ultraviolet aerosol index (AI) parameters (Torres et al., 2007). NO2, ozone, and AI data were used for OMI version 3 data, which has daily temporal with a minimum grid of 0.25 × 0.25 spatial resolution. However, for the anomaly study, we have considered a grid of 1 × 1. The details of the OMI data and retrieval methods can be found in Krotkov et al. (2019). The data are downloaded using the NASA Giovanni portal (https://giovanni.gsfc.nasa.gov/).
Aethalometer Measurements
The aethalometer used in the present study is an advanced (next-generation) multi-beam instrument, being operated at AUH, Panchgaon, in collaboration with IITM, Pune, for the 24 × 7 measurement of black carbon (BC) aerosol mass concentration and biomass burning (Sonbawne et al., 2021). This instrument uses the latest “Dual Spot” technology that eliminates data contamination due to filter loading (Drinovec et al., 2015) A unique feature of this instrument is that it provides a simultaneous measurement of biomass burning (BB) contribution (in percentage) to the measured BC mass concentration (µgm−3). The seven operating wavelengths permit the identification of biomass emissions. More details of the instrument and its wide applications to the studies of carbonaceous (most absorbing) aerosol influence on human health and climate have been reported in the literature (for example, Bond et al., 2013; Devara et al., 2017; Dumka et al., 2019; Sonbawne et al., 2021).
The total column aerosol optical depth (AOD), from MODIS, and columnar AI (aerosol index, representative of columnar aerosol size distribution) and water vapor; total column ozone (TCO) and total column nitrogen dioxide from OMI during the period from 01 March to 30 June 2020, which encompasses the pre-lockdown (1–24 March), phase I (25 March–14 April), phase II (15 April–3 May), phase III (4–17 May), phase IV (18–31 May), and post-lockdown (1–30 June) periods have been retrieved. The simultaneous ground-based data using an aethalometer at 370 nm (brown carbon or wood-burning aerosol emissions) and at 880 nm (black carbon emissions from the burning of fossil fuel) have also been archived. These datasets have been analyzed to examine the inter-relationship between the pollutant variations and their resultant influence at two contrasting locations, one situated in the urban and the other in the rural environment, during different lockdown phases of COVID-19. It may be noted here that the norms introduced by the government during different phases affected the pollution levels in different manners (Chen et al., 2020). During phase I, nearly all the industrial and mass transportation were suspended immediately except for some of the essential services. During phase II, conditional relaxation was imposed in the regions where the spread of COVID-19 had been slowed down. During phase III, conditional relaxation continued, and the entire country has been split into three zones as red zone (higher cases), orange zone (lower cases than the red zone), and green zone (no case in the past 21 days). Normal public movement is permitted in the green zones. Red zones were further divided into the “containment” and “buffer” zones and the local bodies were given the authority for this demarcation.
We estimate anomalies (daily values in 2020 minus daily climatology) of AOD, AI, H2O, NO2, and O3. The daily climatology is obtained for the period 2010–2019 for all the species. Since biases are the same in observations in 2020 and climatology, the anomaly is benefited by the removal of systematic biases and examining the variations in relative NO2, O3, AI, H2O, and AOD in 2020. The anomalies of NO2, O3, AI, and AOD represent reduction due to anthropogenic activities during the COVID-19 lockdown period, while anomalies of H2O are mainly due to meteorology.
Trajectory Analysis
An approach to merge trajectories (driven by HYSPLIT) that are near each other and represent those groups, called clusters, by their mean trajectory (Su et al., 2015). Differences between trajectories within a cluster are minimized, while differences between clusters are maximized (Srivastava et al., 2021). Computationally, trajectories are combined until the total variance of the individual trajectories about their cluster mean starts to increase. Cluster analysis is a multivariate statistical technique increasingly used in air pollution research. This method involves splitting the data set into a number of groups that need to be as homogenous and as distinctly different from each other as possible. Generally, the result of the application of the cluster analysis technique to air trajectories is similar to a flow climatology in which trajectories are classified into groups according to certain criteria, but cluster analysis is more objective, and it accounts for variation in transport speed and direction simultaneously, yielding clusters of trajectories, which have similar length and curvature (Harris and Kahl, 1990).
In order to examine the sources that are responsible for the air masses at the study location (Panchgaon, a rural site in the present case), cluster mean backward trajectories at the optimum level and the associated TSV (total spatial variance, in percent) during each phase of lockdown were calculated for the pre-lockdown, phases I, II, III, and IV, and post-lockdown periods. When the clustering is completed, the program calculates the total spatial variance (TSV) as the trajectories are merged into one cluster. In this study, the TSV is a measure of the degree to which the chosen clusters fit the data (Harris and Kahl, 1990; Draxler et al., 2020). In the present case, the change in TSV variance for only the last 30 clusters is considered. The maximum number of clusters in each case varied from two to three, and they are marked by red, dark blue, and green, respectively, for 1st, 2nd, and 3rd clusters. A Supplementary File, covering the details of the experimental location and the results of TSV analysis, is given separately.
Results and Discussion
Variations in NO2 in Delhi and Panchgaon
The daily variation of total column nitrogen dioxide (expressed in 1015 molecules/square centimeter) during the pre-lockdown; phases I, II, III, and IV; and post-lockdown periods over New Delhi and Panchgaon is plotted in Figure 1A. The most striking feature that can be seen from Figure 1A is that the NO2 values over the urban station, New Delhi, are almost the double of the rural station, Panchgaon, throughout the study period. Figure 1B shows anomalies in NO2 at these two stations during the study period. It shows a reduction in NO2 column during phase I compared to the pre-lockdown period by −54% (5.4–2.5 × 1015 mol/cm2) in Delhi and −59% (9.2–3.2 × 1015 mol/cm2) at Panchgaon. This indicates a clear signal of reduced anthropogenic activity during the lockdown that has reduced the NO2 column at both stations. The reduction at Panchgaon is higher than that at New Delhi by 3–10%.
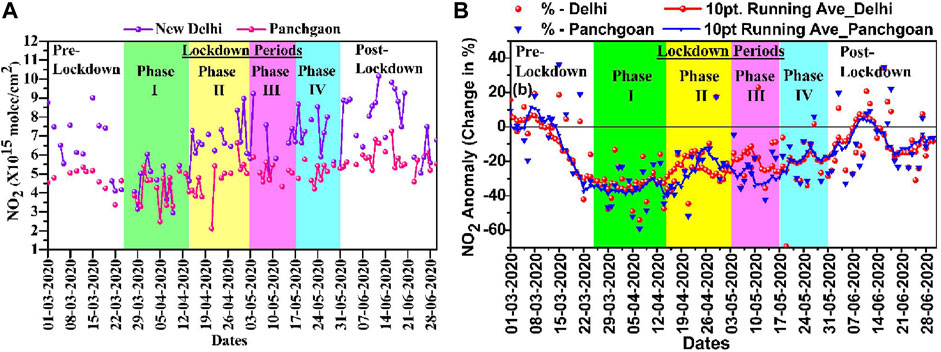
FIGURE 1. (A) Daily variation in total column nitrogen dioxide (NO2) (*1015 molecule/cm2) obtained from OMI satellite retrievals at New Delhi and Panchgaon, (B) same as (A) but for anomalies (2020 minus climatology). Climatology is obtained for the period 2010–2019.
Figure 1B shows an increasing trend in NOx during lockdown to the post-lockdown period at both stations. This could be due to relaxing the strict measures and slowly opening of the economy. However, an average reduction in NOx is by 42.03% in Delhi and by 46.54% at Panchgaon during phase I to IV lockdown periods. The positive anomaly in the pre-lockdown and post-lockdown period suggests the contribution from the anthropogenic activity that was restricted in the COVID lockdown. Previous studies have also reported a reduction in NO2 over North India (in Delhi: −52.68% during phase I–II, by Mahato et al., 2020, at Lucknow: −54% during phase I, by Karuppasamy et al., 2020). These results agree with our findings. More importantly, New Delhi, the capital of India, showed less decrease in NOx compared to Panchgaon. Other stations in India also showed a reduction in NO2 (Mumbai- 43.08%, Gandhinagar- 45.64%, Bangalore- 48.25%, and Nagpur 46.13%) (Vedrevu et al., 2020; Singh and Chauhan, 2020).
Variations in Total Column Ozone (TCO) at New Delhi and Panchgaon
The daily mean variations in total column ozone (TCO) in Dobson Unit (DU), over New Delhi and Panchgaon during the pre-lockdown; lockdown phases I, II, III, and IV; and post-lockdown periods are compared in Figure 2. It shows that most of the time the TCO values over New Delhi are larger than Panchgaon during the study periods.
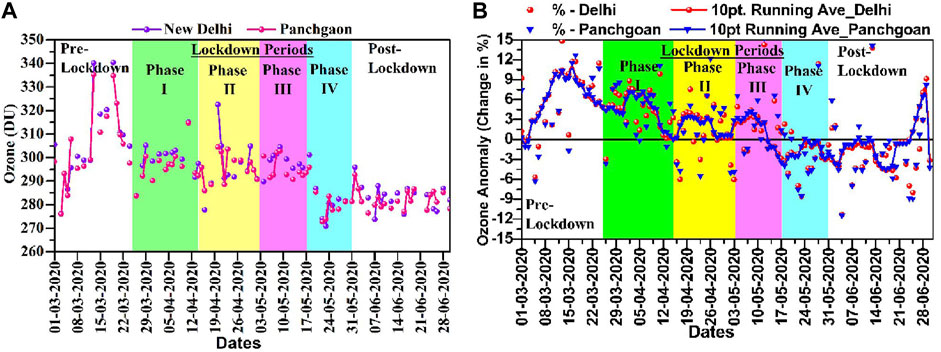
FIGURE 2. (A) Daily variation in total column ozone (O3) (DU) obtained from OMI satellite retrievals at New Delhi and Panchgaon, (B) same as (A) but for anomalies (2020 minus climatology). Climatology is obtained for the period 2010–2019.
Figure 2A shows a decrease in ozone amounts during lockdown periods in comparison to pre-lockdown phase I by 342 to 270 DU in Delhi and 334 to 274 DU at Panchgaon. The ozone concentration shows a decreasing trend over the period covering pre-lockdown to post-lockdown. Furthermore, we show ozone anomalies during the study period in Figure 2B. Its shows, positive anomalies during pre-lockdown to phase III with the range of ∼+12% to +1%, this indicates that ozone concentrations at both stations are higher than the climatology, while ozone anomalies are negative anomalies during phase IV and in the post-lockdown period. This indicates that ozone amounts are less than the climatology during phase IV and in the post-lockdown period.
The positive anomalies of ozone during the pre-lockdown period may be due to a reduction in NO2 (negative anomalies in for phase–IV and post-lockdown) during the same time at New Delhi and Panchgaon stations. A decrease in NO2 has reduced O3 destruction via the NO titration cycle that led to more ozone amounts. Ozone photochemistry is also affected by solar radiation. The reduction of aerosol pollution during the lockdown has increased the solar radiation reaching the ground (Devara et al., 2019; Fadnavis et al., 2019) that might have accelerated ozone formation. A feature of increase in ozone emphasizes the complex O3 chemistry. The variation in other ozone precursors, for example, CO, volatile organic compounds and meteorological variability and solar irradiance (cloudiness) plays an important role in ozone heterogeneous chemistry (Sillman et al., 1990) under significant aerosol reduction conditions (Shi and Brasseur, 2020). Our results are also in agreement with the reported reduction in ozone by 36% during phase-I by Girach et al. (2021) and also with the other studies (Mahato et al., 2020; Soni et al., 2021; Dhaka et al., 2020; Singh et al., 2020).
Variations in Total Column Water Vapor (TCW) at New Delhi and Panchgaon
The daily mean variations in total column water vapor (TCW, in cm), derived from MODIS over New Delhi and Panchgaon are compared in Figure 3A. It is clear from the figure that TCW shows an increasing trend at both study sites. Furthermore, TCW values over New Delhi exhibit almost close to those over Panchgaon during the study period, while it varied from 1.10 to 6.62 cm for New Delhi and from 1.13 to 5.96 cm for Panchgaon during the pre-lockdown period. Phase II, III, and IV periods show the TCW values are slightly higher than those in phase I. However, these values are higher than those of the pre-lockdown period. The increase in the total column water content could also partly be due to the increase in ground-level temperature and change in local meteorological conditions. Increasing water vapor leads to a cooling of the stratosphere and modifies stratospheric chemical processes. Thus, the cooling of the stratosphere leads to stratospheric ozone depletion processes (Tian et al., 2009).
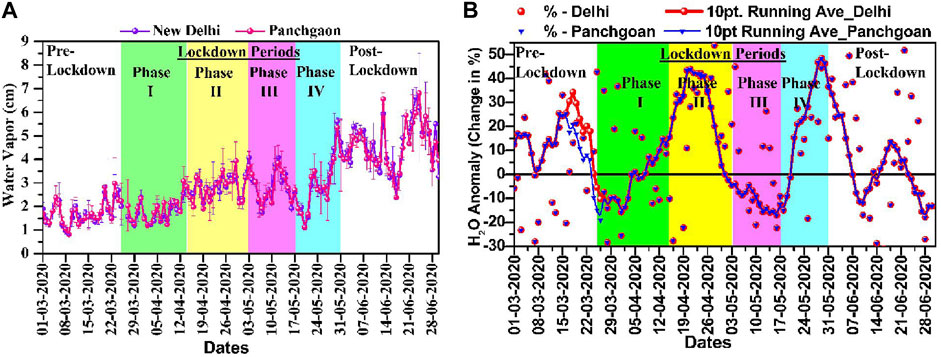
FIGURE 3. (A) Daily variation in total column water vapor (TCW) obtained from MODIS satellite retrievals at New Delhi and Panchgaon, (B) same as (A) but for anomalies (2020 minus climatology). Climatology is obtained for the period 2010–2019.
On the whole, the variations in ozone/water vapor over both locations are found to be well correlated throughout the study period; and they exhibited a monotonic decreasing/increasing trend, which coincides with the fact that the increase in the water vapor content reduces the ozone content, as mentioned before. The variations in TCW during different phases of lockdown are attributed to the relaxations imposed by the government. Thus, the variations in TCW (affected by net radiation) are found to be unique as compared to those of other pollutants at both study regions.
The anomalies computed for MODIS-derived TCW over both study regions are also plotted in Figure 3B. Although the scatter is more in the anomaly, the values are almost coincident, except at the end-stage of the transition between pre-COVID and lockdown phase I over both study regions. Moreover, the TCW anomaly values are larger and positive during the pre-COVID than those in the post-COVID period. Overall, positive values during pre-, II, IV, and post-lockdown, and negative during I and III lockdown periods were shown. These variations could partly be due to the heterogeneous lockdown policy, involving strict and relaxed structures. During the lockdown period, because of the absence of anthropogenic activity, most of the solar radiation reaches the Earth’s surface and hence there was an increase in ground level temperature which in turn causes warmer climate (Vargas et al., 2019). The warmer climate at the surface enhances the stratospheric water vapor. It may be mentioned here that, as explained before, the reduction in AOD during the phase I may be due to the combination of unusual rain and also due to the restrictions imposed by the government.
Variations in AOD in Delhi and Panchgaon
The daily mean variations in aerosol optical depth (AOD) from MODIS during pre-, I, II, III, and IV, and post-lockdown periods at New Delhi (28.35 N, 77.12 E, ∼ 215 m amsl), and Panchgaon (28.32 N, 76.92 E, 285 m amsl) stations are shown in Figure 4. In general, Figure 4A shows higher AODs at New Delhi than Panchgaon during the study period. There is a reduction in AOD during lockdown phase I in Delhi (by 0.54 to 0.15) and Panchgaon (by 0.65 to 0.42) due to the lockdown restrictions imposed by the government. The AOD increase during phase I to the post-lockdown period indicates an increasing trend. The observed increase in AOD at these stations is associated with the partial opening of the economy during phases II, III, and IV. During these phases, the transportation continued to play a significant role since industrial, construction, and economical activities were active. During the post-lockdown phase, AOD values at New Delhi shoot up to 0.50 to 1.50 and at Panchgaon 0.1 to 1.50. These nearly doubled as compared to that during the pre-lockdown period.
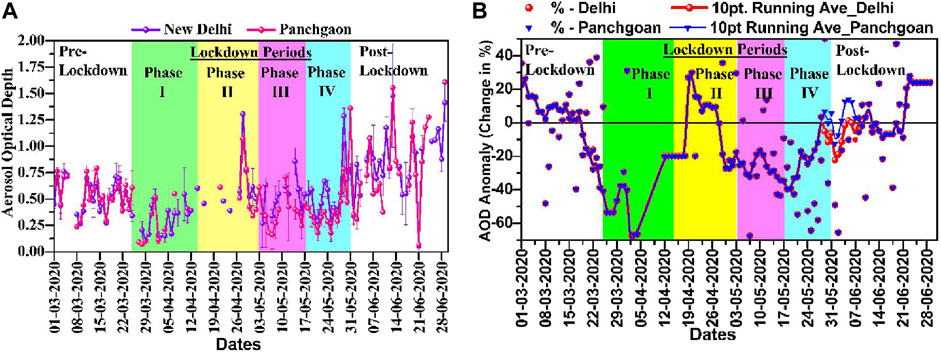
FIGURE 4. (A) Daily variation in total column aerosol optical depth (AOD) obtained from MODIS satellite retrievals at New Delhi and Panchgaon, (B) same as (A) but for anomalies (2020 minus climatology). Climatology is obtained for the period 2010–2019.
Furthermore, we show anomalies of MODIS AOD in Figure 4B, over the New Delhi and Panchgaon obtained as the difference between the daily values of March to June 2020 with respect to climatology. It shows a sharp reduction in AOD by the same amount over New Delhi and Panchgaon (−20% to −80%) during phase I. But in phase II MODIS AOD shows a positive anomaly (5% to 30%). The increase in AOD compared to climatology is due to the higher amount of fires in the vicinity of both the stations. Furthermore, in phase III, IV AOD anomaly is negative over New Delhi (−10% to −40%) and Panchgaon (−10% to −40%). Earlier studies also showed that there is a reduction in AOD during the COVID-19 period over North India (e.g., Ranjan et al., 2020; Fadnavis et al., 2021; Ranjan et al., 2021), which agree with the present work.
Variations in Aerosol Index (AI) in Delhi and Panchgaon
The OMI aerosol index (AI) is an indicator of aerosol size distribution that has been deduced from the spectral dependence of AOD observations on each day. We analyzed the OMI aerosol index (AI) over New Delhi and Panchgaon during the pre-lockdown, lockdown phase I, II, III, and IV, and post-lockdown period. Figure 5A shows day-to-day variation and Figure 5B anomaly of AI. It is clear from the figure that, overall, the AI values for Panchgaon (rural station) are larger (smaller size particles) and smaller (bigger particles) at New Delhi (Urban station). In general AI values are less than 1 at both stations indicating the presence of anthropogenic aerosols. The anomalies in AI (Figure 5B) show positive anomalies in the pre-lockdown and post-lockdown period. This is due to higher anthropogenic emissions. The negative anomalies of AI during lockdown phases are due to limited anthropogenic activity. The large grid of OMI (1 × 1) covers both Delhi and Panchgaon stations. Hence, variations in anomalies are the same at both stations. It also suggests the transport of dust is less than during the rest of the normal year (year not associated with any major ocean-atmospheric coupled phenomenon like El Nino, IOD etc.)., which is also shown by Fadnavis et al. (2021). The time-series patterns of AI at New Delhi and Panchgaon have been studied. The results show a substantial reduction in AOD (Delhi: −20% to −80%, and Panchgaon: −20% to −80%) in comparison with climatology (2010–2019) during all the four phases of lockdown. The observed reduction in AOD at both stations is attributed to lockdown measures and less transport of dust from west Asia than climatology.
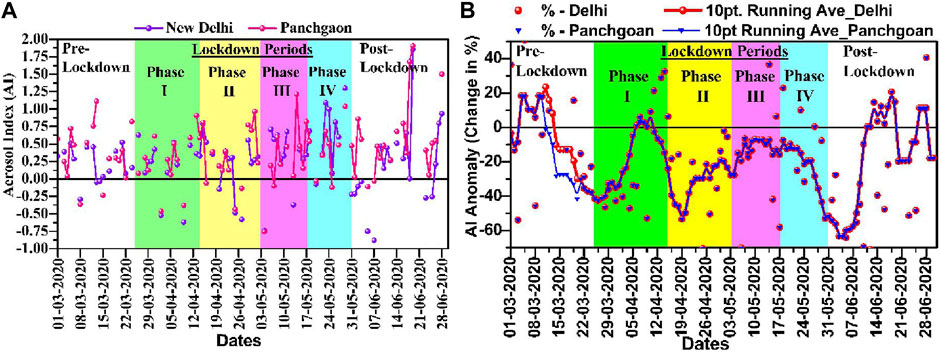
FIGURE 5. (A) Daily variation in aerosol index (AI) obtained from OMI satellite retrievals at New Delhi and Panchgaon, (B) same as (A) but for anomalies (2020 minus climatology). Climatology is obtained for the period 2010–2019.
Variations in BC and BB Over Panchgaon
Both black carbon (BC) aerosols and biomass burning (BB) have received particular attention around the world due to their impact on air quality and public health. Due to its fine size (size-mode 2.5 μm), large specific surface area, and irregular morphology, it is easily inhaled and can affect human health, causing cardiovascular, respiratory, and other diseases (Highwood and Kinnersley, 2006; Suglia et al., 2008; Cunha-Lopes et al., 2019). BC mass concentrations, measured using an aethalometer at 370 and 880 nm wavelengths during the pre-, phase I, II, III, IV, and post-lockdown periods are plotted in Figures 6A,B. The daily mean variation in black carbon (BC) mass concentration observed using a multi-spectral aethalometer at 370 and 880 nm wavelengths during the pre-, phase I, II, III, IV and post-lockdown periods is plotted in Figures 6A,B. The daily variation of the percentile of biomass burning (BB) aerosol contribution to BC is also plotted in
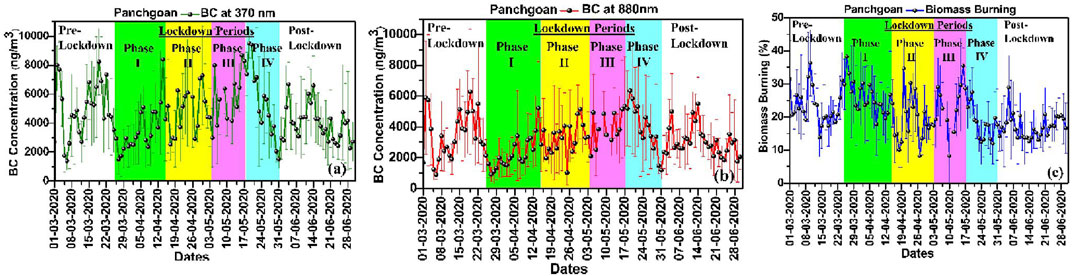
FIGURE 6. Day-to-day marching of BC mass concentration and BB % obtained using multi-spectral aethalometer measurements (A) at 370 nm, (B) 880 nm, and (C) BB%.
Figure 6B. As explained in Dumka et al. (2019), the major contribution to BC at 370 nm comes from the vehicular exhaust, while the contribution at 880 nm comes from industrial emissions. Since these estimations are mainly made by the property of absorption, the effects due to wavelength dependence are normalized in the calculations. Figure 6A shows a reduction in the BC mass concentration during phase I as compared to the pre-lockdown period (370 nm: 8,241-1,510 ng/m3; 880 nm:6,293-912 ng/m3) at Panchgaon, The observed reduction is due to the total restriction on transportation and industrial operations. BC at 370 and 880 nm (Figure 6B) increases during phase I to IV, whereas the contribution of BB % shows a decline (Figure 6C) during lockdown phases. However, these observations are not available at New Delhi.
Variations in Transport Characteristics During Lockdown
During the pre-monsoon season when the lockdown was imposed over India, variation in aerosols is influenced by long-range transport of dust from west Asia (Lau, 2006; Fadnavis et al., 2017). However, the transport pattern may be different in spring 2020 due to variation in dynamics (Fadnavis et al., 2019). Hence, we show trajectory analysis during the lockdown period. While forward trajectories describe where a particle will go, backward trajectories indicate where it came from. Air trajectories are frequently used for the interpretation of individual flow situations for several decades, but statistical methods for large sets of trajectories have been developed more recently (Stohla et al., 2002; IPCC, 2017).
To examine the sources of air mass concentrations at the study location (New Delhi), we have shown cluster mean backward trajectories and the associated total spatial variance (TSV, in percent) during the pre-lockdown; phases I, II, III, and IV; and post-lockdown periods in Figure 7. It is to note that the number of trajectories available for forming the clusters during each phase of lockdown is different for different phases.
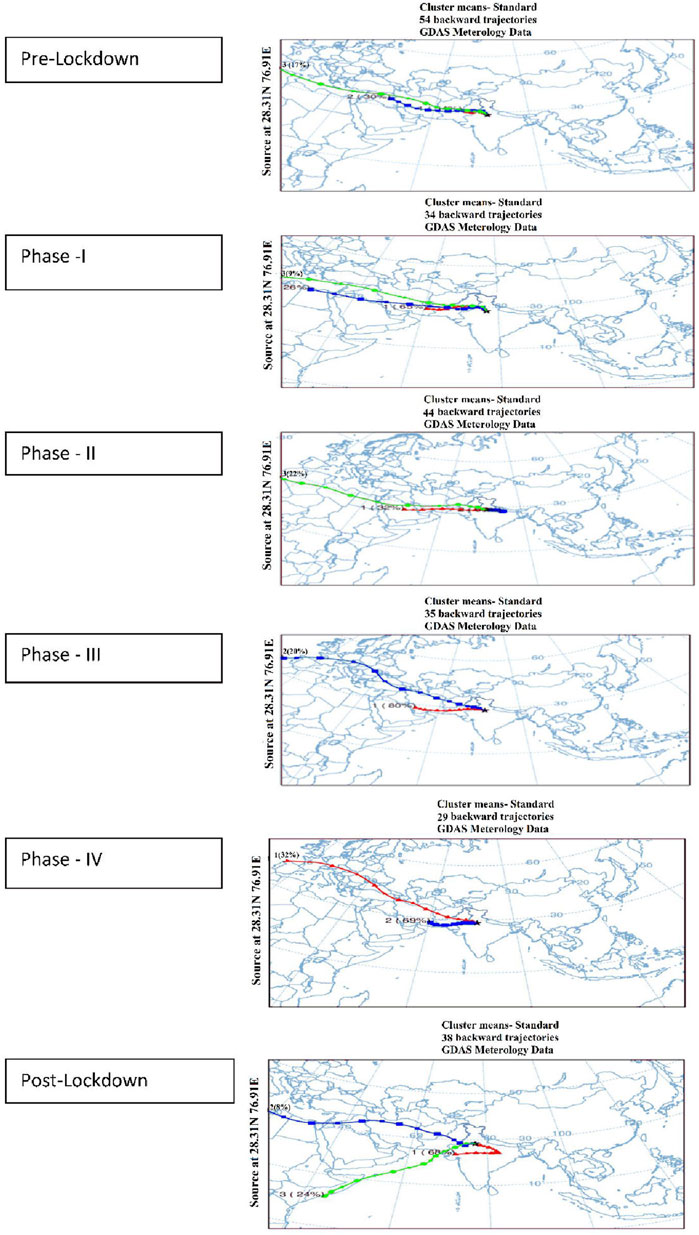
FIGURE 7. Air mass backward trajectory (Source at 28.37N, 76.91E) cluster for the BC data of pre-, I, II, III, IV, and post-lockdown phases during 1 March–30 June 2020.
In the case of pre-lockdown (1st to 25th March 2020) the 54 backward trajectories of air masses come at the study location (New Delhi) from the Mediterranean Sea and desert region. However, cluster 2 contributes 30% of the air mass, which comes from the desert region. In the case of phase I lockdown (25th March–14th April 2020), 34 backward trajectories of air masses come from the Mediterranean Sea and desert region, however, maximum contribution (65%) by 1st cluster. 44 backward trajectories were averaged in the case of the phase II lockdown and formed three clusters; the 1st cluster contributed 32% of air mass from the desert region. In the case of the phase III lockdown period, 35 backward trajectories were averaged, and two clusters were formed. The 1st cluster contributed a maximum of 80% of air mass from the desert region. Similarly, in the case of the phase IV lockdown phase, a total of 29 backward air mass trajectories participated the formation of clusters; and two clusters were formed. The 2nd cluster contributed a maximum of 69% of air mass from the desert region. On the other hand, in the case of post-lockdown, a total of 38 backward trajectories have participated in the formation of clusters; and three clusters were formed. The 1st cluster contributed 68% to the air mass from a local transport and the 2nd cluster contributed 24% of air mass coming from the Arabian Sea. This trajectory analysis indicates a transport of dust from the Mediterranean Sea and desert region (Fadnavis et al., 2021).
Summary and Conclusion
The impact of lockdown concept of COVID-19 on the synchronous near-surface carbonaceous aerosols and satellite-derived columnar aerosol optical depth, size distribution, water vapor, ozone, and nitrogen peroxide over two contrasting environmental (urban and rural) conditions during 2020 has been reported in this study. The analysis of OMI NO2 shows a substantial reduction in Delhi (−10% to −42.03%) and Panchgaon (−10% to −46.54%), while ozone amounts show enhancement in Delhi (1% to 8%) and Panchgaon (1% to 10%) than climatology during lockdown I—III phases. The observed enhancement in ozone, despite a reduction in NO2, may be a resultant of the complex photochemical processes related to the ozone formation mechanisms that involve the presence of NO2, CO, volatile organic carbon (VOC), and water vapor. The columnar density of pollutants over the urban station (New Delhi), especially in the case of columnar NO2, is found to be higher than that over the rural (Panchgaon) station.
The variation of pollutant levels showed a positive trend in tropospheric column NO2 and H2O and a negative trend in tropospheric column ozone. The behavior of pollutant concentrations during both lockdown versus no-lockdown phase, and their comparison within the lockdown periods also exhibited interesting results. It is observed that the pollutant concentration scenarios are different not only between pre- and post-lockdown periods due to local meteorology and long-range transport processes but also within the four lockdown phases implemented by the government. The significant differences observed between certain phases are considered to be due to the nature of lockdown, imposed by the government. Overall, the concentration of pollutants is found to be lower during the lockdown phases than those observed either during the pre-lockdown or post-lockdown period. This aspect clearly indicates the positive impact of the lockdown concept, and it is found more helpful not only for curbing the regional pollution but also for improving the other requirements of maintaining the social distance to minimize the health hazards leading to morbidity and mortality during the COVID-19 period.
Data Availability Statement
The raw data supporting the conclusions of this article will be made available by the authors, without undue reservation.
Author Contributions
PD: conceptualization, investigation, methodology, project administration, supervision, and original manuscript. SS, SF, KV, and PC: data curation, formal analysis, validation, and visualization of the study.
Conflict of Interest
The authors declare that the research was conducted in the absence of any commercial or financial relationships that could be construed as a potential conflict of interest.
Publisher’s Note
All claims expressed in this article are solely those of the authors and do not necessarily represent those of their affiliated organizations, or those of the publisher, the editors, and the reviewers. Any product that may be evaluated in this article, or claim that may be made by its manufacturer, is not guaranteed or endorsed by the publisher.
Acknowledgments
The research work reported in this article was carried out as a part of joint collaborative research project between Amity University Haryana (AUH), Gurugram, Indian Institute of Tropical Meteorology (IITM), Pune, and Sri Venkateswara University (SVU), Tirupati, India. The authors are thankful to Ashok K. Chauhan, founder president; Aseem Chauhan, chancellor and all other authorities of AUH for their continued support; and to the Director, IITM, and Head of Department of Physics, SVU, for their cooperation. We also acknowledge NASA for the MODIS and OMI satellite products and also NOAA-ERL for the HYSPLIT back-trajectory model analysis results for the study period. We thank the reviewers and the editor for their valuable suggestions toward the improvement of the manuscript.
Supplementary Material
The Supplementary Material for this article can be found online at: https://www.frontiersin.org/articles/10.3389/fenvs.2022.826799/full#supplementary-material
References
Bera, B., Bhattacharjee, S., Shit, P. K., Sengupta, N., and Saha, S. (2020). Significant Impacts of COVID-19 Lockdown on Urban Air Pollution in Kolkata (India) and Amelioration of Environmental Health. Environ. Dev. Sustain. 28, 1–28. doi:10.1007/s10668-020-00898-5
Bond, T. C., Doherty, S. J., Fahey, D. W., Forster, P. M., Berntsen, T., DeAngelo, B. J., et al. (2013). Bounding the Role of Black Carbon in the Climate System: a Scientific Assessment. J. Geophys. Res. Atmos. 118, 5380–5552. doi:10.1002/jgrd.50171
Bull, F. C., Al-Ansari, S. S., Biddle, S., Borodulin, K., Buman, M. P., Cardon, G., et al. (20202020). World Health Organization 2020 Guidelines on Physical Activity and Sedentary Behaviour. Br. J. Sports Med. 54, 1451–1462. doi:10.1136/bjsports-2020-102955
Chen, L.-W. A., Chien, L.-C., Li, Y., and Lin, G. (2020). Nonuniform Impacts of COVID-19 Lockdown on Air Quality over the United States. Sci. Total Environ. 745, 141105. doi:10.1016/j.scitotenv.2020.141105
Cunha-Lopes, I., Martins, V., Faria, T., Correia, C., and Almeida, S. M. (2019). Children's Exposure to Sized-Fractioned Particulate Matter and Black Carbon in an Urban Environment. Building Environ. 155, 187–194. doi:10.1016/j.buildenv.2019.03.045
Devara, P. C. S., Alam, M. P., Dumka, U. C., Tiwari, S., and Srivastava, A. K. (2017). “Anomalous Features of Black Carbon Aerosols Observed over a Rural Station during Diwali Festival of 2015,” In; Book Titled “Environmental Pollution”. Science (Berlin, Germany: Springer).
Devara, P. C. S., Kumar, A., Sharma, P. B., Banerjee, P., Khan, A. A., Sonbawne, S. M., et al. (2021). Multi-Sensor Study of the Impact of Air Pollution on COVID-19. J. Infect. Dis. Res. (Jidr) 4 (1), 157–168.2688-6537
Devara, P. C. S., Kumar, A., Sharma, P. B., Banerjee, P., Khan, A. A., Tripathi, A., et al. (2020). “Influence of Air Pollution on Coronavirus (COVID-19): Some Evidences from Studies at AUH, Gurugram, India,” in Open Research Communication in the Social Science Research Network (SSRN), USA. doi:10.2139/ssrn.3588060
Devara, P. C. S. (2017). “Remote Sensing for Environment and Climate Diagnostics. Book Chapter,” in Environmental Science and Engineering (Houston, TX: Studium Press LLC, USA), 287–312.
Dhaka, S. K., Chetna, K. V., Kumar, V., Panwar, V., Dimri, A. P., Singh, N., et al. (2020). PM2.5 Diminution and Haze Events over Delhi during the COVID-19 Lockdown Period: an Interplay between the Baseline Pollution and Meteorology. Sci. Rep. 10, 13442. doi:10.1038/s41598-020-70179-8
Draxler, R., Stunder, B., Rolph, G., Stein, A., and Taylor, A. (2020). HYSPLIT User’s Guide, Version 5. Boulder, Colorado, USA: NOAA.
Drinovec, L., Močnik, G., Zotter, P., Prévôt, A. S. H., Ruckstuhl, C., Rupakheti, M., et al. (2015). The "Dual-Spot" Aethalometer: an Improved Measurement of Aerosol Black Carbon with Real-Time Loading Compensation. Atmos. Meas. Tech. 8, 1965–1979. doi:10.5194/amt-8-1965-2015
Dumka, U. C., Kaskaoutis, D. G., Devara, P. C. S., Kumar, R., Kumar, S., Tiwari, S., et al. (2019). Year-long Variability of the Fossil Fuel and wood Burning Black Carbon Components at a Rural Site in Southern Delhi Outskirts. Atmos. Res. 216, 11–25. doi:10.1016/j.atmosres.2018.09.016
Fadnavis, S., Kalita, G., Kumar, K. R., Gasparini, B., and Li, J.-L. F. (2017). Potential Impact of Carbonaceous Aerosol on the Upper Troposphere and Lower Stratosphere (UTLS) and Precipitation during Asian Summer Monsoon in a Global Model Simulation. Atmos. Chem. Phys. 17, 11637–11654. doi:10.5194/acp-17-11637-2017
Fadnavis, S., Sabin, T. P., Rap, A., Müller, R., Kubin, A., and Heinold., B. (2021). The Impact of COVID-19 Lockdown Measures on the Indian Summer Monsoon. Environ. Res. Lett. 16 (2021), 074054. doi:10.1088/1748-9326/ac109c
Gautam, S. (2020a). COVID-19: Air Pollution Remains Low as People Stay at home. Air Qual. Atmos. Health 13 (May), 853–857. doi:10.1007/s11869-020-00842-6
Gautam, S. (2020b). The Influence of COVID-19 on Air Quality in India: A Boon or Inutile. Bull. Environ. Contam. Toxicol. 104 (6), 724–726. doi:10.1007/s00128-020-02877-y
Ghosh, S., and Ghosh, S. (2020). Air Quality during Covid-19 Lockdown: Blessing in Disguise. Indian J. Biochem. Biophys. 57, 420–430.
Girach, I. A., Narendra, O., and Sureshbabu, S. (2021). Ozone Chemistry and Dynamics at a Tropical Coastal Site Impacted by the COVID-19 Lockdown. J. Earth Syst. Sci. 130, 158. doi:10.1007/s12040-021-01666-3
Gupta, N., Tomar, A., and Kumar, V. (2020). The Effect of Covid-19 Lockdown on the Air Environment in India. Glob. J. Environ. Sci. Manage. 6, 31–40.
Harris, J. M., and Kahl, J. D. (1990). A Descriptive Atmospheric Transport Climatology for the Mauna Loa Observatory, Using Clustered Trajectories. J. Geophys. Res. 95, 13651–13667. doi:10.1029/jd095id09p13651
He, G., Pan, Y., and Tanaka, T. (2020). The Short-Term Impacts of COVID-19 Lockdown on Urban Air Pollution in China. Nat. Sustain. 3, 1005. doi:10.1038/s41893-020-0581-y
Highwood, E. J., and Kinnersley, R. P. (2006). When Smoke Gets in Our Eyes: The Multiple Impacts of Atmospheric Black Carbon on Climate, Air Quality and Health. Environ. Int. 32, 560–566. doi:10.1016/j.envint.2005.12.003
IPCC (2017). Synthesis Report. Contribution of Working Groups I, II and III to the Fourth Assessment Report of the Intergovernmental Panel on Climate Change [Core Writing Team, Pachauri, R.K and Reisinger, A. (eds.)]. Geneva, Switzerland: IPCC, 104. IPCC 4th Assessment Report.
Jain, S., and Sharma, T. (2020). Social and Travel Lockdown Impact Considering Coronavirus Disease (COVID-19) on Air Quality in Megacities of India: Present Benefits, Future Challenges and Way Forward. Aerosol Air Qual. Res. 20, 1222–1236. doi:10.4209/aaqr.2020.04.0171
Kanniah, K. D., Kamarul Zaman, N. A. F., Kaskaoutis, D. G., and Latif, M. T. (2020). COVID-19's Impact on the Atmospheric Environment in the Southeast Asia Region. Sci. Total Environ. 736, 139658. doi:10.1016/j.scitotenv.2020.139658
Karuppasamy, M. B., Seshachalam, S., Natesan, U., Ayyamperumal, R., Karuppannan, S., Gopalakrishnan, G., et al. (2020). Air Pollution Improvement and Mortality Rate during COVID-19 Pandemic in India: Global Intersectional Study. Air Qual. Atmos. Health 13, 1375–1384. doi:10.1007/s11869-020-00892-w
King, M. D., Kaufman, Y. J., Tanre, D., and Nakajima, T. (1999). Remote Sensing of Tropospheric Aerosols from Space: Past, Present and Future. Bull. Amer. Meteorol. Soc. 80 (11). doi:10.1175/1520-0477(1999)080<2229:rsotaf>2.0.co;2
Krotkov, N. A., Lamsal, L. N., Marchenko, S. V., Celarier, E. A., Bucsela, E. J., Swartz, W. H., et al. OMI Core team (2019). OMI/Aura NO2 Cloud-Screened Total and Tropospheric Column L3 Global Gridded 0.25° X 0.25 ° V3, NASA Goddard Space Flight Center, Goddard Earth Sciences Data and Information Services Center (GES DISC). Accessed:29/04/2020. doi:10.5067/Aura/OMI/DATA3007
Lau, K. M., Kim, M. K., and Kim, K. M. (2006). Asian Summer Monsoon Anomalies Induced by Aerosol Direct Forcing: the Role of the Tibetan Plateau. Clim. Dyn. 26, 855–864. doi:10.1007/s00382-006-0114-z
Mahato, S., Pal, S., and Ghosh, K. G. (2020). Effect of Lockdown amid COVID-19 Pandemic on Air Quality of the Megacity Delhi, India. Sci. Total Environ. 730, 139086. doi:10.1016/j.scitotenv.2020.139086
MoHFW (2020). Ministry of Health and Family Welfare (MoHFW), Government of India. COVID-19 India. Available at: https://www.mohfw.gov.in (Accessed June 9, 2020).
Prasad, A. K., Singh, R. P., and Kafatos, M. (2006). Influence of Coal Based thermal Power Plants on Aerosol Optical Properties in the Indo-Gangetic basin. Geophys. Res. Lett. 33 (5). doi:10.1029/2005gl023801
Rajbhandari, B., Phuyal, N., Shrestha, B., and Thapa, M. (2020). Air Medical Evacuation of Nepalese Citizen during Epidemic of COVID-19 from Wuhan to Nepal. JNMA J. Nepal Med. Assoc. 58 (222), 125–133. doi:10.31729/jnma.4857
Ranjan, A. K., Patra, A. K., and Gorai, A. K. (2021). A Review on Estimation of Particulate Matter from Satellite-Based Aerosol Optical Depth: Data, Methods, and Challenges. Asia-pacific J. Atmos. Sci. 57, 679–699. doi:10.1007/s13143-020-00215-0
Ranjan, A. K., Patra, A. K., Goraia, A. K., and Gorai, A. K. (2020). Effect of Lockdown Due to SARS COVID-19 on Aerosol Optical Depth (AOD) over Urban and Mining Regions in India. Sci. Total Environ. 745, 141024. doi:10.1016/j.scitotenv.2020.141024
Rimesh, P., and Urmila, Y. (2020). COVID-19 Pandemic in India: Present Scenario and a Steep Climb Ahead. J. Prim. Care Community Health 11, 1–4. doi:10.1177/2150132720939402
Shi, X., and Brasseur, G. P. (2020). The Response in Air Quality to the Reduction of Chinese Economic Activities during the COVID-19 Outbreak. Geophys. Res. Lett. 47, e2020GL088070. doi:10.1029/2020GL088070
Sillman, S., Logan, J. A., and Wofsy, S. C. (1990). The Sensitivity of Ozone to Nitrogen Oxides and Hydrocarbons in Regional Ozone Episodes. J. Geophys. Res. 95 (D2), 1837–1852. doi:10.1029/JD095iD02p01837
Singh, R. P., and Chauhan, A. (2020). Impact of Lockdown on Air Quality in India during COVID-19 Pandemic. Air Qual. Atmos. Health 13, 921–928. doi:10.1007/s11869-020-00863-1
Singh, R. P., Dey, S., Tripathi, S. N., Tare, V., and Holben, B. (2004). Variability of Aerosol Parameters over Kanpur, Northern India. J. Geophys. Res-atmos. 109 (D23). doi:10.1029/2004jd004966
Singh, V., Singh, S., Biswal, A., Kesarkar, A. P., Mor, S., and Ravindra, K. (2020). Diurnal and Temporal Changes in Air Pollution during COVID-19 Strict Lockdown over Different Regions of India. Environ. Pollut. 266, 115368. doi:10.1016/j.envpol.2020.115368
Sonbawne, S. M., Devara, P. C. S., and Bhoyar, P. D. (2021). Multisite Characterization of Concurrent Black Carbon and Biomass Burning Around COVID-19 Lockdown Period. Urban Clim. 39, 100929. doi:10.1016/j.uclim.2021.100929
Soni, M., Ojha, N., and Girach, I. (2021). Impact of COVID-19 Lockdown on Surface Ozone Build-Up at an Urban Site in Western India Based on Photochemical Box Modelling. Curr. Sci. 120, 376–381. doi:10.18520/cs/v120/i2/376-381
Srivastava, A. (2020). COVID-19 and Air Pollution and Meteorology-An Intricate Relationship: A Review. Chemosphere 263, 128297. doi:10.1016/j.chemosphere.2020.128297
Srivastava, A. K., Bhoyar, P. D., Kanawade, V. P., Devara, P. C. S., Thomas, A., and Soni, V. K. (2021). Improved Air Quality during COVID-19 at an Urban Megacity over the Indo-Gangetic Basin: From Stringent to Relaxed Lockdown Phases. Urban Clim. 36, 100791. doi:10.1016/j.uclim.2021.100791
Stohl, A., Eckhardt, S., Forster, C., James, P., Spichtinger, N., and Seibert, P. (2002). A Replacement for Simple Back Trajectory Calculations in the Interpretation of Atmospheric Trace Substance Measurements. Atmos. Environ. 36, 4635–4648. doi:10.1016/s1352-2310(02)00416-8
Su, L., Yuan, Z., Fung, J. C. H., and Lau, A. K. H. (2015). A Comparison of HYSPLIT Backward Trajectories Generated from Two GDAS Datasets. Sci. Total Environ. 506, 527–537. doi:10.1016/j.scitotenv.2014.11.072
Suglia, S. F., Gryparis, A., Schwartz, J., and Wright, R. J. (2008). Association between Traffic-Related Black Carbon Exposure and Lung Function Among Urban Women. Environ. Health Perspect. 16 (10), 1333–1337. doi:10.1289/ehp.11223
Suresh, A., Chauhan, D., Othmani, A., Bhadauria, N., S, A., Jose, J., et al. (2020). Diagnostic Comparison of Changes in Air Quality over China before and during the COVID-19 Pandemic. Res. Square. doi:10.21203/rs.3.rs-30482/v1
Tian, W., Chipperfield, P. M., and Lu, D. (2009). Science of the Total Environment. Adv. Atmos. Sci. 506-507 (3), 423–437. doi:10.1016/j.scitotenv.2014.11.072
Torres, O., Tanskanen, A., Veihelmann, B., Ahn, C., Braak, R., Bhartia, P. K., et al. (2007). Aerosols and Surface UV Products from Ozone Monitoring Instrument Observations: An Overview. J. Geophys. Res. 112, D24S47. doi:10.1029/2007JD008809
Vadrevu, K. P., Eaturu, A., Biswas, S., Lasko, K., Sahu, S., Garg, J. K., et al. (2020). Spatial and Temporal Variations of Air Pollution over 41 Cities of India during the COVID-19 Lockdown Period. Sci. Rep. 10, 16574. doi:10.1038/s41598-020-72271-5
Vargas, L. R. Z., Donohoe, A., and Battisti, D. S. (2019). Does Surface Temperature Respond to or Determine Downwelling Longwave Radiation? Geophys. Res. Lett. 46, 2781. doi:10.1029/2019gl082220
Keywords: aerosol pollution, MODIS, OMI, aethalometer, COVID-19 lockdown, AOD, TCO
Citation: Sonbawne SM, Fadnavis S, Vijayakumar K, Devara PCS and Chavan P (2022) Phase-Resolved Lockdown Features of Pollution Parameters Over an Urban and Adjoining Rural Region During COVID-19. Front. Environ. Sci. 10:826799. doi: 10.3389/fenvs.2022.826799
Received: 01 December 2021; Accepted: 04 March 2022;
Published: 04 April 2022.
Edited by:
Sabine Griessbach, Helmholtz Association of German Research Centres (HZ), GermanyReviewed by:
Eduardo Landulfo, Instituto de Pesquisas Energéticas e Nucleares (IPEN), BrazilChinmay Mallik, Central University of Rajasthan, India
Copyright © 2022 Sonbawne, Fadnavis, Vijayakumar, Devara and Chavan. This is an open-access article distributed under the terms of the Creative Commons Attribution License (CC BY). The use, distribution or reproduction in other forums is permitted, provided the original author(s) and the copyright owner(s) are credited and that the original publication in this journal is cited, in accordance with accepted academic practice. No use, distribution or reproduction is permitted which does not comply with these terms.
*Correspondence: Panuganti C. S. Devara, pcsdevara@ggn.amity.edu