- 1Department of Urban Engineering, Graduate School of Engineering, The University of Tokyo, Tokyo, Japan
- 2Antimicrobial Resistance Research Center, National Institute of Infectious Diseases, Tokyo, Japan
- 3Research Center for Water Environment Technology, Graduate School of Engineering, The University of Tokyo, Tokyo, Japan
Antimicrobial resistance (AMR) is a serious public health concern. Many countries have implemented AMR surveillance programs for humans and animals, but a scheme for monitoring AMR in the environment has not yet been established. Class 1 integrons, which can acquire antimicrobial resistance genes (ARGs) to gene cassettes, were proposed as a candidate to evaluate the anthropogenic impacts on AMR. However, the association between class 1 integrons and ARGs in aquatic environments is less studied and requires further elucidation. This study used high-throughput quantitative polymerase chain reaction (HT-qPCR) to characterize the pollution profiles of ARGs and mobile gene elements (MGEs) in 24 urban rivers in Tokyo and its surrounding area. The abundance of class 1 integron-integrase gene (intI1) and the array of class 1 integron gene cassettes were also determined. In total, 9–53 target genes were detected per sample, and their abundances increased following effluent discharge from wastewater treatment plants. The river and wastewater samples were categorized based on their HT-qPCR profiles, indicating that this method was useful for characterizing the pollution status in aquatic environments. The prevalence of intI1 in the rivers was observed. Some ARGs and MGEs were positively correlated with intI1, indicating that intI1 could be used as a proxy for monitoring these ARGs and MGEs in urban rivers. Long-read sequencing of class 1 integron gene cassettes revealed that one to three ARGs were present in the gene cassettes. Regardless of the sample type, blaGES-24, aadA2, and qacH were dominant in the gene cassettes. The source and spread of class 1 integrons carrying these ARGs in aquatic environments should be further monitored.
Introduction
The health burden of antimicrobial resistance (AMR) is a crucial issue across the world (O'Neill, 2016). While the overuse or abuse of antimicrobial agents for humans and animals has led to the emergence of antimicrobial resistant bacteria (ARB) and antimicrobial resistance genes (ARGs), the recipient environments could serve as their reservoirs (Nnadozie and Odume, 2019). Therefore, One Health, which is a comprehensive and multisectoral approach to address AMR issues in humans, animals, and the environment, serves as an essential initiative to mitigate the spread of AMR in the society. Many countries have implemented surveillance programs for pathogenic ARB in humans and animals (WHO, 2021a). On the other hand, the dimension of environmental AMR remains unknown (Larsson et al., 2018; Samreen et al., 2021; Zhuang et al., 2021), and the interaction between pathogens and environmental bacteria carrying ARGs could be facilitated by horizontal gene transfer (Martínez, 2019). WHO recently launched the Tricycle project, which is aimed at One Health surveillance by focusing on extended-spectrum beta-lactamase (ESBL)-producing Escherichia coli (Pruden et al., 2021; WHO, 2021b). However, monitoring targets and goals to control AMR in the environment is still challenging owing to the lack of basic information such as ARG abundance and diversity.
Metagenomic analysis and high-throughput quantitative polymerase chain reaction (HT-qPCR) are promising tools for the comprehensive surveillance of ARGs and mobile gene elements (MGEs) in the environment. Metagenomic analysis is a non-target screening method in which no preliminary information of genes is known (Chen et al., 2019b; Hendriksen et al., 2019; Liang et al., 2020; Lira et al., 2020). HT-qPCR, which enables the simultaneous quantification of hundreds of target genes, is generally more sensitive than metagenomic analysis for ARGs and MGEs surveillance (Waseem et al., 2019). Although HT-qPCR provides semiquantitative data, it is still rapid and inexpensive for screening complex AMR profiles in the environment. Therefore, HT-qPCR has been employed to evaluate ARGs and MGEs in various aquatic environments, including rivers (Khan et al., 2019; Lai et al., 2021; Yu et al., 2021), water sources (Han et al., 2020), drinking water (Xu et al., 2016), urban sewage (Huang et al., 2019; Pärnänen et al., 2019; Quintela-Baluja et al., 2019), and aquaculture systems (Muziasari et al., 2016). HT-qPCR provides information to help determine factors that shape environmental resistome, such as bacterial community, antibiotic concentration (Han et al., 2020), and ARG sources in the environment (Khan et al., 2019).
In urban rivers, wastewater treatment plants (WWTPs) are hotspots that release ARB and ARGs as well as residual antibiotics (Michael et al., 2013; Mao et al., 2015; Guo et al., 2017; Amarasiri et al., 2019). In a previous study, HT-qPCR based on 384 primer sets was used for the comprehensive surveillance of ARGs and MGEs in WWTP influent and effluent in seven European countries, and 289 primer sets showed positive results (Pärnänen et al., 2019). HT-qPCR revealed that the total abundances of ARGs in recipient surface water bodies were higher than those at upstream sites, suggesting that WWTP effluent was a major source of ARGs in urban aquatic environments (Huang et al., 2019; Quintela-Baluja et al., 2019; Lai et al., 2021).
Representative indicators are useful for the efficient and routine monitoring of various ARGs and MGEs. Class 1 integrons have been proposed as an anthropogenic pollution marker for AMR (Amos et al., 2015; Gillings et al., 2015; Zheng et al., 2019; Li et al., 2020). Integrons are bacterial genetic elements that can incorporate multiple exogenous genes, including ARGs, into gene cassettes (GCs) by the site-specific recombination function of integrase (Gillings, 2014). Because the incorporated genes can be expressed through the integron-associated promoter (Collis and Hall, 1995), integron GCs containing ARGs can spread multidrug resistance (Gillings, 2014). Five classes of mobile integrons (class 1–5) are involved in the spread of ARGs as they are frequently associated with transposons and conjugative plasmids (Gillings, 2014). The class 1 integron-integrase gene (intI1) was found to be prevalent in wastewater and river water (Ma et al., 2017). A strong correlation was observed between intI1 and ARGs, such as aminoglycoside resistance genes and sulfonamide resistance genes, in aquatic environments (Gillings, 2014; Ma et al., 2017; Dong et al., 2019; Zheng et al., 2019; Agramont et al., 2020; Nguyen et al., 2021). Previous studies further investigated class 1 integron GCs in wastewater using clone library analysis or next-generation sequencing (Gatica et al., 2016; Ma et al., 2017; An et al., 2018). In wastewater, many ARGs conferring resistance to aminoglycoside, beta-lactam, and trimethoprim were often detected in class 1 integron GCs (Ma et al., 2017; An et al., 2018). As there is a large diversity in the types of GCs (Moura et al., 2009), they can be regarded as fingerprints of AMR in the environment.
In Japan, several studies have reported the presence of antimicrobial-resistant E. coli in rivers (Ham et al., 2012; Urase and Sato, 2016; Gomi et al., 2017; Yamashita et al., 2017; Suzuki et al., 2019; Tsutsui and Urase, 2019). Although some studies investigated specific ARGs in rivers (Nguyen et al., 2019; Liu et al., 2020), to the best of our knowledge, no comprehensive profiles of ARGs and MGEs in urban rivers have been reported in Japan. In the present study, HT-qPCR was performed to determine the prevalence of 67 ARGs and MGEs in 24 urban rivers in Tokyo and surrounding prefectures. Based on the results of HT-qPCR, the applicability of intI1 as a surrogate marker was assessed. The arrays of class 1 integron GCs were determined by using nanopore long-read sequencing to further evaluate the association between class 1 integrons and ARGs in urban rivers.
Materials and Methods
Sampling
From September 2019 to February 2020, river water samples (n = 30) from 24 rivers in Tokyo and its surrounding prefectures (Kanagawa, Chiba, Saitama, and Ibaraki prefectures) were collected. According to Ministry of Land, Infrastructure, Transport and Tourism in Japan, sewage coverage rates in Tokyo Metropolis, Kanagawa, Chiba, Saitama, and Ibaraki prefectures in 2019 were 99.6%, 96.9%, 75.5%, 81.9%, and 63.0%, respectively. The sampling sites are summarized in Figure 1 and Table 1. They were categorized into three groups based on the rough estimation of the percentages of treated effluent from WWTPs to river flow rates (annual average) at the sampling sites (Table 1). Group A included sampling sites with no WWTPs located upstream. Group B included sampling sites in which the percentages of effluent were estimated to be <10% (1–6%, average: 2%). Group C included sampling sites in which the percentages of effluent could be >10% (11–100%, average: 43%). Additional pollution sources such as the livestock industry and decentralized wastewater treatment facilities were not considered in the grouping. In Tamagawa River, Iruma River, and Arakawa River, water samples were collected from upstream (TM1, TM2, IR1, and AR1) and downstream sites (TM3, TM4, TM5, IR2, and AR2) to evaluate the impact of treated effluent discharged between two sites. Influent (Group INF) and treated effluent (Group EFF) samples (n = 8) were collected from four municipal WWTPs (WWTP A–D) in the region. All plants use a conventional activated sludge process followed by chlorine disinfection to treat domestic wastewater. The treatment capacities of WWTP A–D were 20,000 m3/day, 140,000 m3/day, 290,000 m3/day, and 450,000 m3/day, respectively.
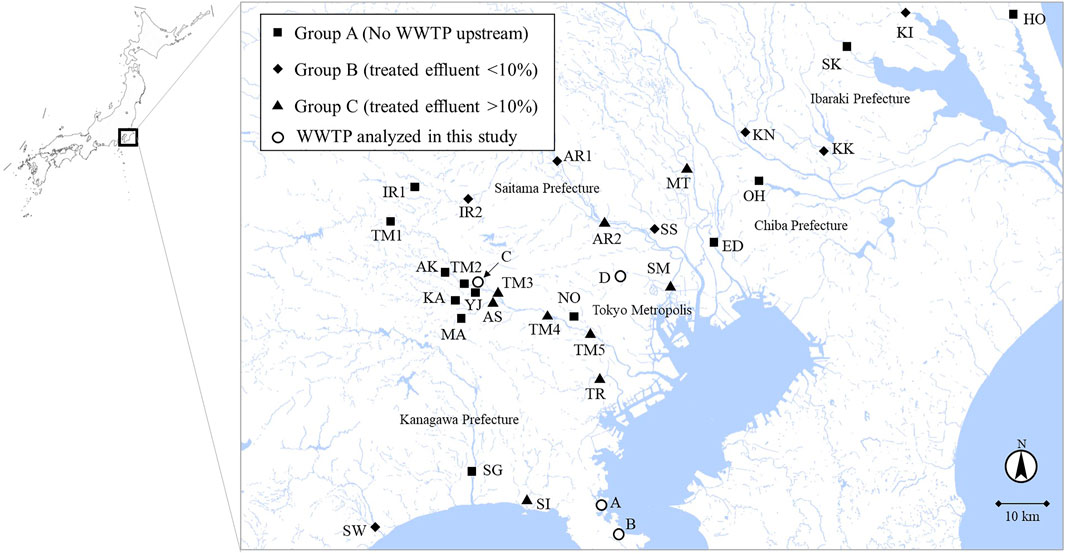
FIGURE 1. River water sampling sites. The sampling sites were categorized into Group A (no WWTP upstream), Group B (effluent percentage <10%), and Group C (effluent percentage >10%) according to the percentages of treated effluent from WWTPs to annual average river flow rates. The map was adapted from the original data of Geospatial Information Authority of Japan (https://maps.gsi.go.jp/).
Water Quality Analysis
The water temperature, pH, and conductivity were measured onsite using a portable Combo meter (Hanna Instruments, RI, United States). Total coliforms and E. coli were cultured on Chromocult coliform agar (Merck Millipore, MA, United States) at 37°C for 24 h. The water samples were filtered through 0.2-µm mixed cellulose ester membranes (DISMIC-25 AS, Advantec, Japan) and subjected to ammonium analysis. Ammonium concentrations were measured using a salicylate method with a spectrophotometer (TNT830A, DR2800-01B, Hach, CO, United States). Total cell counts (TCC) were determined using a flow cytometer (Accuri C6, BD, NJ, United States) by staining samples with SYBR Green I (Thermo Fisher Scientific, MA, United States) (Kasuga et al., 2020).
DNA Extraction
The water samples (100 ml) were filtered through 0.22-µm Isopore polycarbonate membrane filters (Merk Millipore, MA, United States) to harvest bacteria. The filters were dissolved in phenol:chloroform:isoamyl alcohol solution (25:24:1) (Nippon Gene, Japan) and treated by bead beating using a FastPrep 24 Instrument (MP Biomedicals, CA, United States). DNA extraction was performed by using the FastDNA SPIN Kit for Soil (MP Biomedicals) (Kasuga et al., 2020). DNA concentrations were measured with a Nanodrop ND-1000 spectrophotometer (Thermo Fisher Scientific).
Quantitative Polymerase Chain Reaction
Bacterial 16S rRNA genes, intI1, sul1 (sulfonamide resistance gene), and tetA (tetracycline resistance gene) were quantified using LightCycler 480 SYBR Green I Master (Roche, Switzerland) to compare the results of HT-qPCR, and human-specific cross-assembly phage (crAssphage) (Dutilh et al., 2014; Stachler et al., 2019) was quantified using LightCycler 480 Probe Master (Roche). The primers and probes used in the analysis are listed in Supplementary Table S1. PCR was performed in triplicate using a LightCycler 480 II (Roche). The PCR conditions for 16S rRNA genes, intI1, sul1, and tetA comprised of 95°C for 5 min, followed by 45 cycles of 95°C for 10 s, 55°C for 20 s, and 72°C for 20 s (detection). Melting curve analysis was performed by increasing the temperature from 65 to 95°C to check for nonspecific amplification. The PCR conditions for crAssphage comprised of 95°C for 5 min, followed by 45 cycles of 95°C for 10 s, 60°C for 50 s, and 72°C for 1 s (detection). A 10-fold dilution series (5.0 × 101 to 5.0 × 106 gene copies/µl) was prepared for standard curves using an artificially synthesized plasmid containing the target genes. The average PCR amplification efficiencies of 16S rRNA genes, intI1, sul1, tetA, and crAssphage were 99.3%, 93.7%, 98.5%, 92.5%, and 97.8%, respectively.
High-Throughput-Quantitative Polymerase Chain Reaction
The DNA extracts of 38 samples were sent to Resistomap Oy (Helsinki, Finland) for HT-qPCR analysis using a SmartChip Real-time PCR system (TaKaRa Bio, Japan) (Stedtfeld et al., 2018). The target genes, including ARGs, MGEs, and 16S rRNA genes, were analyzed using 68 primer sets validated by Primer Set 2.0 (Stedtfeld et al., 2018) (Supplementary Table S2). The PCR reaction mixture (100 nL) was prepared using 1× SmartChip TB Green Gene Expression Master Mix (TaKara Bio, Japan), nuclease-free PCR-grade water, 300 nM of each primer, and 2 ng/μL DNA template. After initial denaturation at 95°C for 10 min, PCR comprised 40 cycles of 95°C for 30 s and 60°C for 30 s, followed by melting curve analysis for each primer set (Wang et al., 2014). The threshold cycle (CT) of 27 was selected as the detection limit (Muziasari et al., 2016; Muziasari et al., 2017). Amplicons with nonspecific melting curves and multiple peaks were excluded. The mean CT of three technical replicates in each reaction was used to calculate the ΔCT values (CT of detected gene–CT of 16S rRNA gene). The relative abundances of the detected gene to 16S rRNA gene were estimated using the ΔCT method (Schmittgen and Livak, 2008).
Amplicon Sequencing of Class 1 Integron Gene Cassettes
Class 1 integron GCs were amplified from all DNA extracts of rivers and WWTPs samples using 5′CS (5′-GGCATCCAAGCAGCAAG-3′) and 3′CS (5′-AAGCAGACTTGACCTGA-3′), which are specific to the conserved segments of both ends of class 1 integron GCs (Levesque et al., 1995; Ma et al., 2017; An et al., 2018). The thermal conditions of the first PCR were as follows: 25 cycles of 94°C for 30 s, 55°C for 1 min, and 72°C for 2.5 min, with a final extension at 72°C for 10 min. TaKaRa EX Taq Hot Start version (TaKaRa Bio) was used for PCR. The first PCR product was purified using the MinElute PCR Purification Kit (Qiagen, Germany). The second PCR was performed using the same primers with nanopore sequencing adapters (underlined): 5′CS-adp (5′-TTTCTGTTGGTGCTGATATTGCGGCATCCAAGCAGCAAG-3′) and 3′CS-adp (5′-ACTTGCCTGTCGCTCTATCTTCAAGCAGACTTGACCTGA-3′). The thermal conditions of the second PCR were as follows: 15 cycles of 94°C for 30 s, 55°C for 1 min, and 72°C for 2.5 min. A final extension at 72°C for 10 min was added. The second PCR products were purified using the MinElute PCR Purification Kit and checked by electrophoresis on 1.0% agarose gel at 100 V for 20 min.
For multiplex nanopore sequencing, barcoding adapters were attached to the second PCR products using PCR Barcoding Expansion Pack 1-96 (Oxford Nanopore Technologies, United Kingdom) and LongAmp Taq 2× Master Mix (New England BioLabs, MA, United States). The barcoding PCR involved the following steps: initial denaturation at 95°C for 3 min, followed by 15 cycles of 95°C for 15 s, 62°C for 15 s, and 65°C for 5 min. A final extension step at 65°C for 5 min was added. The products were purified using AMPure XP (Beckman Coulter, CA, United States). Finally, equal amounts of the barcoded PCR products were pooled and mixed with DNA CS (Ligation Sequencing Kit 1D, Oxford Nanopore Technologies), NEBNext FFPE DNA Repair Buffer (New England BioLabs), NEBNext FFPE DNA Repair Mix (New England BioLabs), Ultra II End-prep reaction buffer (New England BioLabs), and Ultra II End-prep enzyme mix (New England BioLabs). They were incubated at 20°C for 5 min and at 65°C for 5 min. After purification, adapter ligation was performed using the Ligation Sequencing Kit 1D (SQK-LSK109) (Oxford Nanopore Technologies). The prepared library was loaded onto an FLO-MIN106D flow cell (R9.4.1) (Oxford Nanopore Technologies) and sequenced on a MinION (Oxford Nanopore Technologies). Base-calling and debarcoding were then performed using Guppy (version 5.0.16) (Oxford Nanopore Technologies) with the super-accuracy mode. Reads with shorter than 500 bp of sequence length and lower than Q10 of mean quality were excluded using Filtlong (version 0.2.0) (https://github.com/rrwick/Filtlong) from further analysis. Error correction of the filtered reads was performed using Canu (version 2.1.1) (Koren et al., 2017) with default parameters. ARGs were detected using Staramr (version 0.7.2) (https://github.com/phac-nml/staramr) with the setting of identity ≥90% and overlap ≥60%. The nucleotide sequence data are available at the DDBJ Sequence Read Archive under the accession number DRA013066.
Microbial Community Analysis
Microbial community structures were analyzed for all samples from rivers and WWTPs by amplicon sequencing of V4 regions of 16S rRNA genes. A primer set of 515F (5′-GTGCCAGCMGCCGCGGTAA-3′) and 806R (5′-GGACTACHVGGGTWTCTAAT-3′) with the adapter sequence was used (Caporaso et al., 2011). Paired-end sequencing analysis was performed on the Miseq platform (Illumina, CA, United States) using MiSeq Reagent Kit v3 kit (2 × 300 bp) at Bioengineering Lab (Japan). Quality filtering was conducted using the FASTX-Toolkit (version 0.0.14) (http://hannonlab.cshl.edu/fastx_toolkit/) to extract reads, which showed a perfect match with the primer sequences. Chimeric sequences and noise were removed in DADA2 in QIIME 2.0 pipeline (version 2021.4) (Bolyen et al., 2019). Phylogenetic analysis was performed using the q2 feature-classifier plugin of QIIME 2.0 with reference sequences in Greengene (version 13_8) (DeSantis et al., 2006). Operational taxonomic units were defined by a sequence similarity threshold of 97%. The nucleotide sequence data are available at the DDBJ Sequence Read Archive under the accession number DRA013028.
Statistical Analysis
Statistical tests were performed using BellCurve for Excel (version 3.21) (Social Survey Research Information Co., Ltd., Japan). Cluster analysis based on the Ward method and principal coordinate analysis based on the Bray–Curtis dissimilarity index was performed using R (version 4.0.5) with the vegan package. Network analysis was performed based on Spearman’s rank correlation coefficient between the relative abundances of the target genes and the genus-level abundances of the microbial community. Spearman’s rank correlation coefficient was calculated using the psych package in R. Associations with a correlation coefficient of >0.600 (p < 0.05) were visualized using Gephi (version 0.9.2) (Bastian et al., 2009).
Results
Comparison Between Quantitative Polymerase Chain Reaction and High-Throughput-Quantitative Polymerase Chain Reaction
The ratio of target genes against 16S rRNA gene such as intI1/16S rRNA gene, sul1/16S rRNA gene, and tetA/16S rRNA gene assessed using conventional qPCR was compared with the results of HT-qPCR to evaluate the quantitative performance of HT-qPCR (Supplementary Figure S1). Both data showed significantly positive correlations (Pearson’s correlation coefficient r = 0.876 for intI1, r = 0.827 for sul1, and r = 0.613 for tetA; p < 0.05). However, the conventional qPCR results were 6.2, 2.6, and 5.0 times higher than those of HT-qPCR for intI1, sul1, and tetA, respectively.
Detection Frequency of Antimicrobial Resistance Genes and Mobile Gene Elements in Rivers
Water samples were collected from 30 sites from 24 rivers. They were categorized to Group A–C based on the percentages of treated effluent from WWTPs to river flow rates. The water quality parameters are summarized in Supplementary Table S3. The abundance of E. coli in Group C (mean ± SD = 7.5 × 102 ± 7.5 × 102 CFU/100 ml, n = 9) was significantly higher than that in Group A (2.2 × 102 ± 3.1 × 102 CFU/100 ml, n = 13) and Group B (1.9 × 102 ± 3.0 × 102, n = 8) (Steel–Dwass test, p < 0.05). In addition, the TCC of Group C (1.3 × 107 ± 1.1 × 107 cells/ml, n = 9) was significantly higher than that of Group A (2.6 × 106 ± 1.7 × 106 cells/ml, n = 13) (Steel–Dwass test, p < 0.05).
Among 67 target ARGs and MGEs, 9–37, 21–37, and 23–53 genes were detected in Groups A, B, and C, respectively. There was a significant difference in the number of detected genes between Groups A and C (Steel–Dwass test, p < 0.05). Furthermore, 56–60 and 28–42 genes were detected in Groups INF and EFF, respectively. After the entry of WWTP effluent, the number of ARGs and MGEs increased from 21 (TM2) to 41 (TM3), from 14 (IR1) to 36 (IR2), and from 37 (AR1) to 53 (AR2) in Tamagawa River, Iruma River, and Arakawa River, respectively.
The genes that were frequently detected from >70% of river water samples are listed in Table 2. ARGs conferring resistance to aminoglycoside, beta-lactam, phenicol, multidrug, macrolide-lincosamide-streptogramin B (MLSB), and sulfonamide were included. For MGEs, class 1 integrons (intI1), transposons (tnpA), and insertion sequences (ISPps and IS26) were included. qacEdelta1 (multidrug resistance), aadA (aminoglycoside resistance), and sul1 were detected in >90% of the samples. These genes were also detected in most of the influent and effluent samples. The average detection frequencies of the top 20 genes were 71% (Group A), 85% (Group B), 85% (Group C), 85% (Group INF), and 80% (Group EFF).
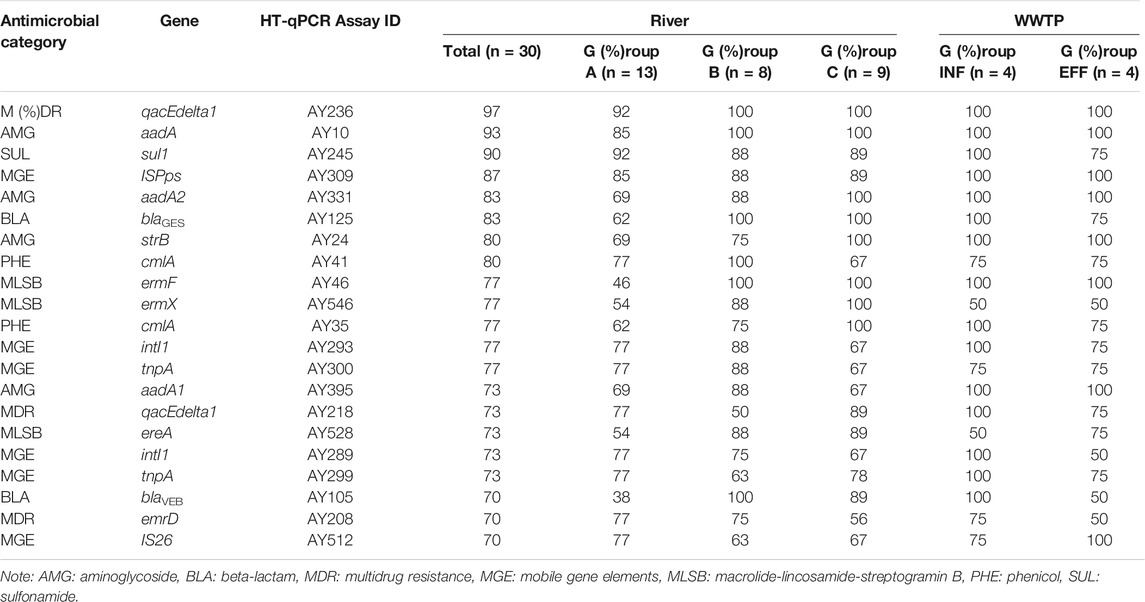
TABLE 2. Target genes detected by HT-qPCR from >70% of river water samples. The percentages of positive samples in each group are shown.
Profiles of River Water Resistome
The relative abundances of the ARGs and MGEs in the samples are shown in Figure 2. There was a positive correlation between total ARGs/16S rRNA genes and total MGEs/16S rRNA genes (Pearson’s correlation coefficient r = 0.840, p < 0.05) (Supplemetary Figure S2). The total relative abundances of the ARGs and MGEs were not significantly different among Groups A, B, and C (Steel–Dwass test, p > 0.05). ARGs conferring resistance to aminoglycoside, multidrug, and sulfonamide were dominant among the analyzed ARGs in the river water samples. No general relationship was noted between the relative gene abundances and group category. The total relative gene abundances of ARGs and MGEs at the downstream sites of Tamagawa River, Iruma River, and Arakawa River were 2.4–4.7 times higher than those at the upstream sites. In particular, the downstream site of Arakawa River (AR2), which was located immediately after the effluent discharge of a large WWTP in Arakawa River, showed relative gene abundances equivalent to those of the influent samples. The relative gene abundances of the influent samples were generally higher than those of the other groups, whereas wastewater treatment reduced the relative gene abundances by 30–62%. The abundances of MLSB and tetracycline resistance genes in the influent samples decreased following treatment. In contrast, the relative abundances of multidrug resistance genes as well as aminoglycoside and sulfonamide resistance genes increased or remained constant in the effluent samples. For MGEs, the samples with higher abundances of ARGs/16S rRNA genes also showed higher abundances of MGEs/16S rRNA genes. Extraordinary higher abundances of MGEs/16S rRNA genes were observed at the sampling site in Yaji River (YJ in Group A) than those at the sites in the other tributaries of Tamagawa River.
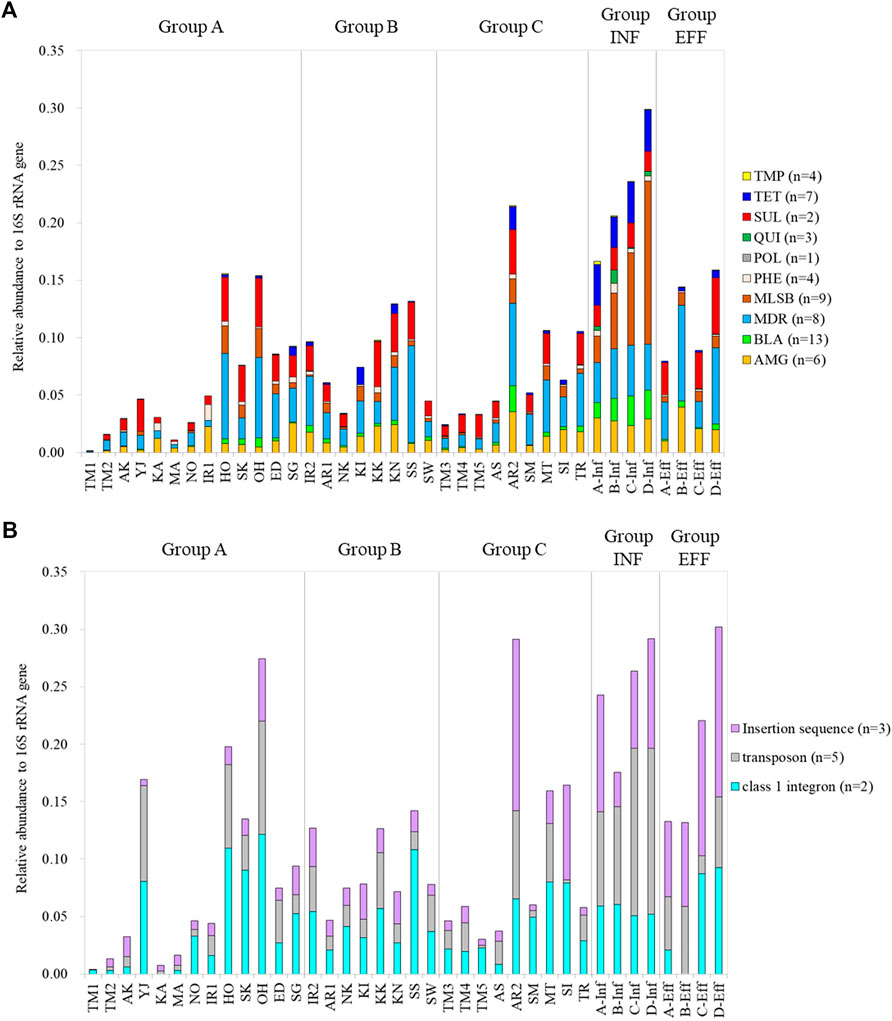
FIGURE 2. Relative gene abundances of ARGs and MGEs based on gene category: (A) ARGs and (B) MGEs. AMG: aminoglycoside, BLA: beta-lactam, MDR: multidrug resistance, MLSB: macrolide-lincosamide-streptogramin B, PHE: phenicol, POL: polymyxin, QUI: quinolone, SUL: sulfonamide, TET: tetracycline, TMP: trimethoprim.
The river and wastewater samples were grouped based on the relative abundances of ARGs and MGEs by Cluster analysis, as shown in Figure 3. They were categorized into three major clusters: Clusters 1–3. Cluster 1 included wastewater samples. Sub-clusters 1a and 1b contained effluent and influent samples, respectively. The sampling sites of Sakai River (SI) and downstream of Arakawa River (AR2) in Group C, which were considerably affected by effluent, were also included in sub-cluster 1a. The river samples in Groups A–C were mixed in Cluster 2. Cluster 3 was separated into sub-clusters 3a, 3b, and 3c. Sub-clusters 3a and 3b included the upstream sites of Tamagawa River (TM1 and TM2 in Group A) and its tributaries, and the downstream sites of Tamagawa River (TM3–TM5 in Group C) and several other rivers were included in sub-cluster 3c.
As compositional changes in ARGs and MGEs were observed between upstream and downstream sites in Tamagawa River, the impact of effluent was also observed in cluster analysis for Iruma River (IR1: Cluster 3a, IR2: Cluster 2a) and Arakawa River (AR1: Cluster 3c, AR2: Cluster 1a). Furthermore, after the entry of WWTP effluent into the river, the emergence or increase of ARGs such as ESBL genes (blaVEB, cfxA, and blaGES), MLSB genes (ermF and mphA), and tetracycline resistance genes (tetQ, tetX, and tetE), as well as MGEs (Tp614 and Tn3), were observed.
Microbial Community Structures
The microbial community structures were analyzed by amplicon sequencing of 16S rRNA genes. Comamonadaceae, Flectobacillus, and Flavobacterium were dominant in the river water samples. Arcobacter, Acinetobacter, and Bacteroides were dominant in the influent samples (Supplementary Figure S3). The community structures were compared using principal coordinate analysis (Figure 4). Axis 1 distinguished river water and wastewater samples, whereas axis 2 distinguished influent and effluent samples. The microbial community structures in Tamagawa River (upstream and downstream) and its tributaries were similar. However, the community structures in Ohori River (OH) and Hokota River (HO) in Group A were different from those in the other sites in Group A. The community structures in Sakai River (SI), Tsurumi River (TR), Sumida River (SM), and downstream of Aarakawa River (AR2) in Group C were more related to those in effluent or influent features. Although a clear transition of community structure driven by effluent discharge was observed in Iruma River (IR1 and IR2) and Arakawa River (AR1 and AR2), the difference was not clear in Tamagawa River (TM1–2 and TM3–5).
The co-occurrence of microbial taxa with ARGs and MGEs was visualized by network analysis, as shown in Figure 5. Four major modules (Modules 1–4) were identified. Module 1 was composed of taxa that were abundant in the intestinal flora, such as Enterobacteriaceae, Clostridiales, Aeromonadaceae, Streptococcus, Bifidobacterium, Prevotella, and Faecalibacterium. They were correlated with genes conferring resistance to tetracycline (tetA, tetE, tetQ, and tetX), aminoglycoside (aadA), beta-lactam (cfxA and blaVEB), MLSB (ermF), and phenicol (catB3). Transposons (Tp614 and Tn3) were also related to Module 1. Module 2 was composed of Bacteroides, Arcobacter, and Acinetobacter, which were correlated with ARGs such as aminoglycoside resistance genes (aadA1, aadA2, and strB) as well as an insertion sequence (ISCR1). In Module 3, class 1 integrons and genes frequently associated with class 1 integron GCs (sul1 and qacEdelta1) were clustered with several taxa such as Bacteroidales and Zoogloea. In Module 4, aminoglycoside resistance gene (aadA6) and multidrug resistance gene (pcoA), as well as MGEs (tnpA and IS26), were associated with several taxa such as Pseudomonas and Parabacteroides.
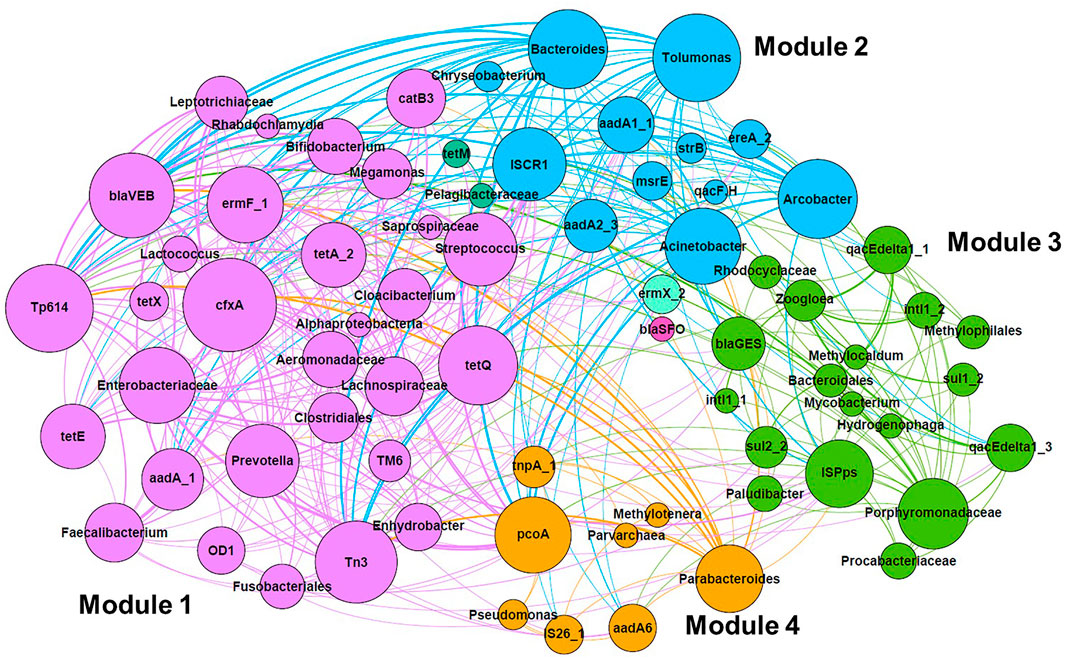
FIGURE 5. Network analysis of co-occurrence pattern among microbial taxa, ARGs, and MGE based on Spearman’s rank correlation coefficient. Associations with Spearman’s rank correlation coefficient of >0.600 (p < 0.05) were selected. Different modules are shown in different colors. The size of nodes represents the number of associations, and the width of lines is proportional to Spearman’s rank correlation coefficient.
Relationship Between Class 1 Integrons and Other Genes
Two primer sets for class 1 integrons (AY293 and AY289) were employed in HT-qPCR. These primer sets were validated for use in HT-qPCR analysis (Stedtfeld et al., 2018). AY293 was originally designed to target clinical class 1 integrons (Gillings et al., 2015), and AY289 was designed for optimizing qPCR (Muziasari et al., 2014). Both primer sets demonstrated almost consistent results (Supplementary Figure S4). Based on the HT-qPCR data, the genes, that exhibited a positive correlation coefficient with class 1 integrons (AY293 and AY289) were screened (Pearson’s correlation coefficient r > 0.400, p < 0.05) (Figure 6). The relative abundances of ARGs and MGEs such as sul1, qacF/H (multidrug resistance), dfrA27 (trimethoprim resistance), tnpA, and qacEdelta1, were highly correlated with class 1 integrons. Although most genes were correlated with intI1 determined by both AY293 and AY289, some genes, such as tetR (tetracycline resistance), dfrA1 (trimethoprim resistance), ereA (MLSB resistance), IS26, and blaGES (beta-lactamase), demonstrated significant correlation with either intI1 (AY293) or intI1 (AY289). In addition to ARGs and MGEs, qPCR analysis also showed that intI1 was correlated with crAssphage (Supplementary Figure S5, r = 0.600, p < 0.05).
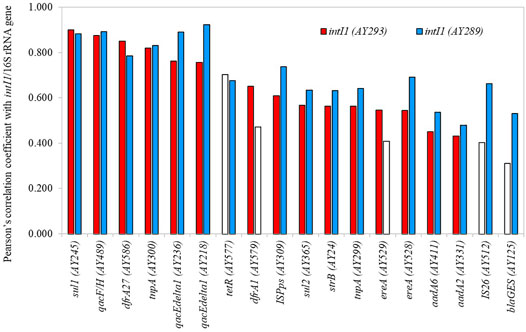
FIGURE 6. Genes with positive correlation coefficient with class 1 integron-integrase genes. Open bars indicate p > 0.05.
Characterization of Class 1 Integron GCs in River Samples
Class 1 integron GCs were analyzed by amplicon sequencing by a MinION nanopore sequencer. Amplicon sequencing depths of 12.7–29.1 Mb with average raw read lengths of 512–1,080 bp were obtained (Supplementary Table S4). The lengths of the most GCs were found to range from <500 bp to 2000 bp after quality filtering (Supplementary Figure S6). Then, 571–1,228 contigs ranging from 500 to 4,580 bp detected in each sample were analyzed (Supplementary Table S5). The median contig size ranged from 593 to 1,489 bp (Supplementary Table S5). While 35% of the contigs did not contain ARGs, 57%, 8%, and 1% of the contigs contained one, two, three ARGs, respectively. Overall, 148 GCs carrying ARGs were detected, including 65 GCs with one ARG, 76 with two ARGs, and 7 with three ARGs (Supplementary Table S6). The percentages of GCs without ARGs were >65% in TM1, TM2, AK, KA, MA (upstream of Tamagawa River and its tributaries), and IR1 (upstream of Iruma River) in Group A (Supplementary Figure S7). Conversely, >90% of the contigs in some rivers in Groups A–C and Group INF carried ARGs (Supplementary Figure S7). In the upstream and downstream sites of Tamagawa River and Iruma River, the percentages of GCs with ARGs increased from 35% (TM2) to 63% (TM3) and from 22% (IR1) to 68% (IR2), respectively (Supplementary Figure S7). Figure 7 shows the relative abundances of contigs of class 1 integron GCs containing ARGs. The information of representative class 1 integron GCs containing ARGs is summarized in Table 3. While ARGs encoding resistance to aminoglycoside, beta-lactam, and multidrug were dominant, specific features were also observed in different samples. blaGES-24 (beta-lactam resistance), aadA2, and qacH (multidrug resistance) were prevalent in the GCs in most of the samples. aac(6′)-31 and aadA1 (both aminoglycoside resistance) were more abundant in the samples other than some rivers in Group A. ere(A) (MLSB resistance) was frequently detected in the river samples. At the same time, it was not dominant in Groups INF and EFF. The GC containing two ARGs (aadA2-qacH) was found in rivers and effluent, but it was rare in the influent samples. Tandem array of aac(6′)-IIa-blaOXA-21-catB3 (aminoglycoside resistance, beta-lactam resistance, and phenicol resistance) was only detected in influent samples of WWTP A and B.
Discussion
AMR surveillance in wastewater and aquatic environments is required to fill the gap of One Health. The present study was the first to employ HT-qPCR to determine the prevalence of ARGs and MGEs in urban rivers in Japan. Among the genes detected from >70% of the river water samples, sul1 and qacEdelta1 were also reported as “core wastewater ARGs and MGEs”, which were present in all influent and effluent samples of 12 WWTPs in Europe (Pärnänen et al., 2019). Moreover, ARGs and MGEs, such as aadA, strB, ermF, intI1, tnpA, and ISPps, were also categorized as “persistent ARGs and MGEs”, which remained in >90% of the effluent samples (Pärnänen et al., 2019). As these genes were detected in most of the influent and effluent samples in the present study, the core/persistent ARGs and MGEs associated with wastewater could be prevalent in aquatic environments in Japan. Because intI1 and ISPps were even detected at upstream sampling sites of Tamagawa River (TM1) and Iruma River (IR1) with lower human activity impact, these genes could possibly serve as sensitive markers of anthropogenic pollution. Further study is necessary to identify the prevalence and sources of these genes in upstream area.
Effluent from WWTPs affect the resistome in recipient rivers (Rodriguez-Mozaz et al., 2015; Cacace et al., 2019; Khan et al., 2019; Pärnänen et al., 2019; Lai et al., 2021). The significantly positive correlation between intI1 and crAssphage in the studied rivers suggests that class 1 integrons and the associated ARGs and MGEs could be originated from human feces (Chen et al., 2019a; Karkman et al., 2019; Agramont et al., 2020; Nguyen et al., 2021). Clear shifts in the relative abundances and profiles were observed after the entry of treated effluent in Tamagawa River, Iruma River, and Arakawa River, which is consistent with the qPCR results in Tamagawa River (Liu et al., 2020). Drastic changes in the microbial community were also observed from upstream to downstream in Iruma River and Arakawa River, suggesting that the microbial community in the effluent could mostly determine the resistome in these rivers. The resistomes in downstream of Arakawa River (AR2) and Sakai River (SI), which had higher percentages of effluent in river flow (AR2: 34%, SI: 52%), were clustered with the effluent resistome. The similarity in resistome profiles between effluent and recipient rivers in urban areas was also demonstrated using HT-qPCR (Huang et al., 2019; Khan et al., 2019). Conversely, the classification of sampling sites based on the ratio of effluent to river flow rates (Groups A–C) was not always associated with the relative abundance and profiles of ARGs and MGEs. Even in Group A, exceptionally higher abundances of ARGs and MGEs were observed in Yaji River (YJ), Hokota River (HO), Sakura River (SK), and Ohori River (OH). As categorization of the sites in this study was simple, the actual contribution of effluent at the sampling occasion could be different from the estimated percentages. The performance of WWTPs in removing ARB and ARGs can fluctuate (Harnisz et al., 2020), and additional pollution sources such as livestock industry and decentralized treatment facilities in rural areas should be considered, especially in rivers in rural area. More intensive sampling considering watershed characteristics is necessary to demonstrate the specific resistome profiles in these rivers.
It is important to know the hosts of ARGs and MGEs to determine the health risks. While single-cell sorting (Chijiiwa et al., 2020; Wang et al., 2020); emulsion, paired isolation, and concatenation PCR (Hultman et al., 2018); and high-throughput chromosome conformation capture (Stalder et al., 2019) can more directly identify the hosts of ARGs and MGEs, co-occurrence of specific taxa and these genes is also informative to explore potential hosts (Quintela-Baluja et al., 2019; Han et al., 2020; Yu et al., 2021). Interestingly, popular taxa related to some ESKAPE (Enterobacteriaceae, Acinetobacter, Aeromonadaceae, and Pseudomonas) were screened by network analysis. The associations observed in Enterobacteriaceae and Aeromonadaceae (tetA, aadA, and catB3), Acinetobacter (aadA1, aadA2, and strB), and Pseudomonas (aadA6) were endorsed by the comprehensive antibiotic resistance database (Alcock et al., 2020). Although network analysis showed that class 1 integrons and the related genes (intI1, sul1, and qacEdelta1) were associated with miscellaneous taxa, the whole genome database revealed that class 1 integrons are mostly carried by three families: Enterobacteriaceae, Pseudomonadaceae, and Moraxellaceae in Gammaproteobacteria (Zhang et al., 2018). Spearman’s rank correlation coefficients between class 1 integrons and these taxa, such as Enterobacteriaceae, Pseudomonas, and Acinetobacter, were only 0.108–0.363, which suggests that other methods should be used to validate the result of network analysis.
Many studies reported that class 1 integrons are a promising indicator of anthropogenic pollution of ARGs (Gillings et al., 2015; Pärnänen et al., 2019; Zheng et al., 2019). The high prevalence and correlation with other ARGs and MGEs in the river samples suggests that intI1 is a representative target in rivers. Some ARGs and MGEs that showed stronger correlation with intI1 could be genetically associated with class 1 integron GCs. For instance, sul1 and qacEdetlta1 are typically fused genes in the 3′ conserved segment of class 1 integron GCs (Gillings, 2014). The other selected ARGs, such as dfrA27 (Wei et al., 2008), dfrA1 (Zhao et al., 2020), strB (Le-Vo et al., 2019), ereA (Malek et al., 2015), aadA6 (Mirahsani et al., 2016), aadA2 (Ahmed and Shimamoto, 2004), and blaGES (Maehana et al., 2021), were also detected from class 1 integron GCs of Gram-negative bacteria. For MGEs, class 1 integron-dfrA5-IS26 element was found in E. coli (Dawes et al., 2010), and transposition genes such as tnpA were associated with class 1 integrons (Ghaly et al., 2017). These reports were consistent with the results of HT-qPCR, demonstrating that HT-qPCR can dissect the relationship between class 1 integrons and other ARGs/MGEs in aquatic environments.
ARGs conferring resistance to aminoglycoside, beta-lactam, multidrug, MLSB, phenicol, and trimethoprim were frequently acquired in class 1 integron GCs in urban rivers and wastewater samples analyzed in the present study, which is consistent with previous reports (Ma et al., 2017; An et al., 2018; Gatica et al., 2019). The acquisition of specific ARG types by class 1 integrons was demonstrated by whole-genome database analysis (Zhang et al., 2018). Although various GC types were detected in the present study, major GCs were not found in other studies analyzing class 1 integron GCs in wastewater by amplicon sequencing using the same primer set (Ma et al., 2017; An et al., 2018). As the composition of class 1 GCs was found to be different in river water, sewage, feces, and livestock wastewater (Ma et al., 2017), the diversity of class 1 integron GCs in aquatic environments could likely depend on geographical and socioeconomic settings.
The percentage of GCs containing ARGs was lower in upstream rivers in Group A than that in the other groups. Moreover, the composition of GCs containing ARGs was not necessarily identical among different rivers. Thus, GC profiles in aquatic environments may indicate AMR fingerprints in each watershed. Quantitative monitoring of class1 integrons and qualitative features of its GCs can be integrated for efficient resistome monitoring in aquatic environments. For example, aac (6′)-31 and aadA1 in GCs were more abundant in the samples other than upstream rivers, indicating the impact of wastewater effluent. aadA2-qacH was present in rivers and effluent but not in influent, suggesting that class 1 integron GCs containing aadA2-qacH could be enriched in wastewater treatment. While ere(A) was frequently detected in rivers and wastewater samples, ere(A) acquired by class 1 integrons was more abundant in rivers than influent and effluent samples. This gap suggests that the genetic context of ere(A) could be different in rivers and wastewater.
Common GC types containing blaGES-24, aadA2, or qacH were observed in river (Groups A–C) and wastewater (Groups INF and EFF) samples. HT-qPCR also revealed a significantly stronger correlation between class 1 integrons and ARGs such as blaGES, aadA2, and qacF/H. Class 1 integron GCs containing aadA2 or qacH were previously reported in a riverine system (Amos et al., 2018). The prevalence of blaGES in class 1 integron GCs in wastewater effluent in Cyprus and Israel was demonstrated, while blaOXA associated with class 1 integron GCs was dominant in effluent samples in other European countries (Gatica et al., 2016). Although the GCs containing aadA2 or qacH were present in the database of integron GCs (INTEGRAL) (Moura et al., 2009), the GC containing blaGES-24 has not yet been registered in the database. blaGES-24 encodes a variant of GES, which is class A beta-lactamase. GES-4, -5, -6, -14, which are characterized by a substitution of Gly170Ser, show carbapenem hydrolysis activity (Bontron et al., 2016). As GES-24 has the same substitution, it has potential to hydrolyze carbapenem. blaGES-24 was carried by bacteria, such as Aeromonas hydrophila, Citrobacter freundii, Enterobacter cloacae, Klebsiella pneumoniae, and Pseudomonas aeruginosa (Alcock et al., 2020). Four tandem copies of blaGES-24 were detected from class 1 integron GCs on the plasmids of A. hydrophila, which was isolated from clinical wastewater in Japan (Maehana et al., 2021). Although Aeromonas spp. in aquatic environments could be the key host of blaGES-24, it is also possible that a wide range of bacteria can carry class 1 integron GCs containing blaGES-24. This ARG was prevalent in rivers in urban areas and wastewater as well as in upstream rivers. More consideration should be given to the dissemination and evolution of blaGES variants associated with class 1 integrons in aquatic environments.
As revealed using conventional qPCR, the relative abundances of the tested genes were 2.six to six times higher than those revealed using HT-qPCR. As the same thermal conditions were employed for all target genes in HT-qPCR, the amplification efficiency may not always be optimized for each gene (Waseem et al., 2019). Although the results of both methods were highly correlated, the quantified values should be carefully interpreted when they are compared with other studies. Moreover, a hydrolysis probe-based HT-qPCR protocol should be compared for more specific quantification (Khan et al., 2019). The technical limitations of class 1 integron GCs analysis include primer coverage of class 1 integron GCs and the accuracy of long-read sequencing. The primer set for class 1 integron GCs (5′CS and 3′CS) that were used in this study was also used in other studies on amplicon sequencing of class 1 integron GCs in aquatic environments (Ma et al., 2017; An et al., 2018). However, the coverage of this primer set was 23.6% of 2,153 integrons in the database (Zhang et al., 2018), which suggests a greater diversity of class 1 integron GCs in the environment. Novel ARGs have been discovered from class 1 integron GCs such as sul4 (sulfonamide resistance) (Razavi et al., 2017) and gar (garosamine-specific aminoglycoside resistance) (Bohm et al., 2020). Therefore, a comprehensive approach such as metagenomic analysis and the amplicon sequencing approach are necessary to reveal the whole picture of class 1 integron GCs in aquatic environments. As co-occurrence of class 2 and 3 integrons with specific ARGs have been reported (Lai et al., 2021), the different integrons could contribute to the spread of specific ARGs in aquatic environments (Gillings, 2014; Deng et al., 2015; An et al., 2018). Regarding long-read sequencing, nanopore technology can circumvent the assembly errors of short reads, while the sequencing error rates of long-read sequencing are generally higher (Weirather et al., 2017). Although error correction of raw reads was applied and ARGs with high identity and overlap values in the polished reads were explored in this study, the validation by short-read sequencing with higher accuracy could compensate for the limitations of long-read sequencing.
Data Availability Statement
The nucleotide sequence data are available at the DDBJ Sequence Read Archive under the accession numbers DRA013066 and DRA013028.
Author Contributions
IK and KN conducted sampling. IK, KN, and MS provided experimental data. IK, KN, and MS analyzed data. IK and KN wrote the main text. All authors corrected and approved the final text.
Funding
This work was supported by JSPS KAKENHI (Grant numbers 19K21984 and 21K18742).
Conflict of Interest
The authors declare that the research was conducted in the absence of any commercial or financial relationships that could be construed as a potential conflict of interest.
Publisher’s Note
All claims expressed in this article are solely those of the authors and do not necessarily represent those of their affiliated organizations, or those of the publisher, the editors, and the reviewers. Any product that may be evaluated in this article, or claim that may be made by its manufacturer, is not guaranteed or endorsed by the publisher.
Acknowledgments
The authors thank Tomoko Inoue for her support in field sampling.
Supplementary Material
The Supplementary Material for this article can be found online at: https://www.frontiersin.org/articles/10.3389/fenvs.2022.825372/full#supplementary-material
References
Agramont, J., Gutiérrez-Cortez, S., Joffré, E., Sjöling, Å., and Calderon Toledo, C. (2020). Fecal Pollution Drives Antibiotic Resistance and Class 1 Integron Abundance in Aquatic Environments of the Bolivian Andes Impacted by Mining and Wastewater. Microorganisms 8 (8), 1122. doi:10.3390/microorganisms8081122
Ahmed, A. M., and Shimamoto, T. (2004). A Plasmid-Encoded Class 1 Integron Carryingsat, a Putative Phosphoserine Phosphatase Gene andaadA2from enterotoxigenicEscherichia coliO159 Isolated in Japan. FEMS Microbiol. Lett. 235 (2), 243–248. doi:10.1111/j.1574-6968.2004.tb09595.x
Alcock, B. P., Raphenya, A. R., Lau, T. T. Y., Tsang, K. K., Bouchard, M., Edalatmand, A., et al. (2020). CARD 2020: Antibiotic Resistome Surveillance with the Comprehensive Antibiotic Resistance Database. Nucleic Acids Res. 48 (D1), D517–D525. doi:10.1093/nar/gkz935
Amarasiri, M., Sano, D., and Suzuki, S. (2019). Understanding Human Health Risks Caused by Antibiotic Resistant Bacteria (ARB) and Antibiotic Resistance Genes (ARG) in Water Environments: Current Knowledge and Questions to Be Answered. Crit. Rev. Environ. Sci. Technol. 50, 2016–2059. doi:10.1080/10643389.2019.1692611
Amos, G. C. A., Ploumakis, S., Zhang, L., Hawkey, P. M., Gaze, W. H., and Wellington, E. M. H. (2018). The Widespread Dissemination of Integrons throughout Bacterial Communities in a Riverine System. ISME J. 12 (3), 681–691. doi:10.1038/s41396-017-0030-8
Amos, G. C., Gozzard, E., Carter, C. E., Mead, A., Bowes, M. J., Hawkey, P. M., et al. (2015). Validated Predictive Modelling of the Environmental Resistome. ISME J. 9 (6), 1467–1476. doi:10.1038/ismej.2014.237
An, X.-L., Chen, Q.-L., Zhu, D., Zhu, Y.-G., Gillings, M. R., and Su, J.-Q. (2018). Impact of Wastewater Treatment on the Prevalence of Integrons and the Genetic Diversity of Integron Gene Cassettes. Appl. Environ. Microbiol. 84 (9), e02766-17. doi:10.1128/aem.02766-17
Bastian, M., Heymann, S., and Jacomy, M. (2009). Gephi: An Open Source Software for Exploring and Manipulating Networks. Proc. Int. AAAI Conf. Weblogs Soc. Media 3 (1), 361–362.
Böhm, M.-E., Razavi, M., Marathe, N. P., Flach, C.-F., and Larsson, D. G. J. (2020). Discovery of a Novel Integron-Borne Aminoglycoside Resistance Gene Present in Clinical Pathogens by Screening Environmental Bacterial Communities. Microbiome 8 (1), 41. doi:10.1186/s40168-020-00814-z
Bolyen, E., Rideout, J. R., Dillon, M. R., Bokulich, N. A., Abnet, C. C., Al-Ghalith, G. A., et al. (2019). Reproducible, Interactive, Scalable and Extensible Microbiome Data Science Using QIIME 2. Nat. Biotechnol. 37 (8), 852–857. doi:10.1038/s41587-019-0209-9
Bontron, S., Poirel, L., and Nordmann, P. (2016). Real-time PCR for Detection of Plasmid-Mediated Polymyxin Resistance (Mcr-1) from Cultured Bacteria and Stools. J. Antimicrob. Chemother. 71 (8), 2318–2320. doi:10.1093/jac/dkw139
Cacace, D., Fatta-Kassinos, D., Manaia, C. M., Cytryn, E., Kreuzinger, N., Rizzo, L., et al. (2019). Antibiotic Resistance Genes in Treated Wastewater and in the Receiving Water Bodies: A Pan-European Survey of Urban Settings. Water Res. 162, 320–330. doi:10.1016/j.watres.2019.06.039
Caporaso, J. G., Lauber, C. L., Walters, W. A., Berg-Lyons, D., Lozupone, C. A., Turnbaugh, P. J., et al. (2011). Global Patterns of 16S rRNA Diversity at a Depth of Millions of Sequences Per Sample. Proc. Natl. Acad. Sci. 108 (Suppl. 1), 4516–4522. doi:10.1073/pnas.1000080107
Chen, H., Bai, X., Li, Y., Jing, L., Chen, R., and Teng, Y. (2019a). Source Identification of Antibiotic Resistance Genes in a Peri-Urban River Using Novel crAssphage Marker Genes and Metagenomic Signatures. Water Res. 167, 115098. doi:10.1016/j.watres.2019.115098
Chen, H., Chen, R., Jing, L., Bai, X., and Teng, Y. (2019b). A Metagenomic Analysis Framework for Characterization of Antibiotic Resistomes in River Environment: Application to an Urban River in Beijing. Environ. Pollut. 245, 398–407. doi:10.1016/j.envpol.2018.11.024
Chijiiwa, R., Hosokawa, M., Kogawa, M., Nishikawa, Y., Ide, K., Sakanashi, C., et al. (2020). Single-cell Genomics of Uncultured Bacteria Reveals Dietary Fiber Responders in the Mouse Gut Microbiota. Microbiome 8 (1), 5. doi:10.1186/s40168-019-0779-2
Collis, C. M., and Hall, R. M. (1995). Expression of Antibiotic Resistance Genes in the Integrated Cassettes of Integrons. Antimicrob. Agents Chemother. 39 (1), 155–162. doi:10.1128/aac.39.1.155
Dawes, F. E., Kuzevski, A., Bettelheim, K. A., Hornitzky, M. A., Djordjevic, S. P., and Walker, M. J. (2010). Distribution of Class 1 Integrons with IS26-Mediated Deletions in Their 3′-Conserved Segments in Escherichia coli of Human and Animal Origin. PLoS One 5 (9), e12754. doi:10.1371/journal.pone.0012754
Deng, Y., Bao, X., Ji, L., Chen, L., Liu, J., Miao, J., et al. (2015). Resistance Integrons: Class 1, 2 and 3 Integrons. Ann. Clin. Microbiol. Antimicrob. 14, 45. doi:10.1186/s12941-015-0100-6
DeSantis, T. Z., Hugenholtz, P., Larsen, N., Rojas, M., Brodie, E. L., Keller, K., et al. (2006). Greengenes, a Chimera-Checked 16S rRNA Gene Database and Workbench Compatible with ARB. Appl. Environ. Microbiol. 72 (7), 5069–5072. doi:10.1128/AEM.03006-05
Dong, P., Cui, Q., Fang, T., Huang, Y., and Wang, H. (2019). Occurrence of Antibiotic Resistance Genes and Bacterial Pathogens in Water and Sediment in Urban Recreational Water. J. Environ. Sci. 77, 65–74. doi:10.1016/j.jes.2018.06.011
Dutilh, B. E., Cassman, N., McNair, K., Sanchez, S. E., Silva, G. G. Z., Boling, L., et al. (2014). A Highly Abundant Bacteriophage Discovered in the Unknown Sequences of Human Faecal Metagenomes. Nat. Commun. 5, 4498. doi:10.1038/ncomms5498
Gatica, J., Jurkevitch, E., and Cytryn, E. (2019). Comparative Metagenomics and Network Analyses Provide Novel Insights into the Scope and Distribution of β-Lactamase Homologs in the Environment. Front. Microbiol. 10, 146. doi:10.3389/fmicb.2019.00146
Gatica, J., Tripathi, V., Green, S., Manaia, C. M., Berendonk, T., Cacace, D., et al. (2016). High Throughput Analysis of Integron Gene Cassettes in Wastewater Environments. Environ. Sci. Technol. 50 (21), 11825–11836. doi:10.1021/acs.est.6b03188
Ghaly, T. M., Chow, L., Asher, A. J., Waldron, L. S., and Gillings, M. R. (2017). Evolution of Class 1 Integrons: Mobilization and Dispersal via Food-Borne Bacteria. PLoS One 12 (6), e0179169. doi:10.1371/journal.pone.0179169
Gillings, M. R., Gaze, W. H., Pruden, A., Smalla, K., Tiedje, J. M., and Zhu, Y.-G. (2015). Using the Class 1 Integron-Integrase Gene as a Proxy for Anthropogenic Pollution. ISME J. 9 (6), 1269–1279. doi:10.1038/ismej.2014.226
Gillings, M. R. (2014). Integrons: Past, Present, and Future. Microbiol. Mol. Biol. Rev. 78 (2), 257–277. doi:10.1128/MMBR.00056-13
Gomi, R., Matsuda, T., Matsumura, Y., Yamamoto, M., Tanaka, M., Ichiyama, S., et al. (2017). Whole-genome Analysis of Antimicrobial-Resistant and Extraintestinal Pathogenic Escherichia coli in River Water. Appl. Environ. Microbiol. 83 (5), e02703-16. doi:10.1128/aem.02703-16
Guo, J., Li, J., Chen, H., Bond, P. L., and Yuan, Z. (2017). Metagenomic Analysis Reveals Wastewater Treatment Plants as Hotspots of Antibiotic Resistance Genes and mobile Genetic Elements. Water Res. 123, 468–478. doi:10.1016/j.watres.2017.07.002
Ham, Y.-S., Kobori, H., Kang, J.-H., Matsuzaki, T., Iino, M., and Nomura, H. (2012). Distribution of Antibiotic Resistance in Urban Watershed in Japan. Environ. Pollut. 162, 98–103. doi:10.1016/j.envpol.2011.11.002
Han, Z., Zhang, Y., An, W., Lu, J., Hu, J., and Yang, M. (2020). Antibiotic Resistomes in Drinking Water Sources across a Large Geographical Scale: Multiple Drivers and Co-occurrence with Opportunistic Bacterial Pathogens. Water Res. 183, 116088. doi:10.1016/j.watres.2020.116088
Harnisz, M., Kiedrzyńska, E., Kiedrzyński, M., Korzeniewska, E., Czatzkowska, M., Koniuszewska, I., et al. (2020). The Impact of WWTP Size and Sampling Season on the Prevalence of Antibiotic Resistance Genes in Wastewater and the River System. Sci. Total Environ. 741, 140466. doi:10.1016/j.scitotenv.2020.140466
Hendriksen, R. S., Munk, P., Munk, P., Njage, P., van Bunnik, B., McNally, L., et al. (2019). Global Monitoring of Antimicrobial Resistance Based on Metagenomics Analyses of Urban Sewage. Nat. Commun. 10 (1), 1124. doi:10.1038/s41467-019-08853-3
Huang, H., Zeng, S., Dong, X., Li, D., Zhang, Y., He, M., et al. (2019). Diverse and Abundant Antibiotics and Antibiotic Resistance Genes in an Urban Water System. J. Environ. Manage. 231, 494–503. doi:10.1016/j.jenvman.2018.10.051
Hultman, J., Tamminen, M., Pärnänen, K., Cairns, J., Karkman, A., and Virta, M. (2018). Host Range of Antibiotic Resistance Genes in Wastewater Treatment Plant Influent and Effluent. FEMS Microbiol. Ecol. 94 (4), fiy038. doi:10.1093/femsec/fiy038
Karkman, A., Pärnänen, K., and Larsson, D. G. J. (2019). Fecal Pollution Can Explain Antibiotic Resistance Gene Abundances in Anthropogenically Impacted Environments. Nat. Commun. 10 (1), 80. doi:10.1038/s41467-018-07992-3
Kasuga, I., Suzuki, M., Kurisu, F., and Furumai, H. (2020). Molecular-level Characterization of Biodegradable Organic Matter Causing Microbial Regrowth in Drinking Water by Non-target Screening Using Orbitrap Mass Spectrometry. Water Res. 184, 116130. doi:10.1016/j.watres.2020.116130
Khan, F. A., Söderquist, B., and Jass, J. (2019). Prevalence and Diversity of Antibiotic Resistance Genes in Swedish Aquatic Environments Impacted by Household and Hospital Wastewater. Front. Microbiol. 10, 688. doi:10.3389/fmicb.2019.00688
Koren, S., Walenz, B. P., Berlin, K., Miller, J. R., Bergman, N. H., and Phillippy, A. M. (2017). Canu: Scalable and Accurate Long-Read Assembly via Adaptive K-Mer Weighting and Repeat Separation. Genome Res. 27 (5), 722–736. doi:10.1101/gr.215087.116
Lai, F. Y., Muziasari, W., Virta, M., Wiberg, K., and Ahrens, L. (2021). Profiles of Environmental Antibiotic Resistomes in the Urban Aquatic Recipients of Sweden Using High-Throughput Quantitative PCR Analysis. Environ. Pollut. 287, 117651. doi:10.1016/j.envpol.2021.117651
Larsson, D. G. J., Andremont, A., Bengtsson-Palme, J., Brandt, K. K., de Roda Husman, A. M., Fagerstedt, P., et al. (2018). Critical Knowledge Gaps and Research Needs Related to the Environmental Dimensions of Antibiotic Resistance. Environ. Int. 117, 132–138. doi:10.1016/j.envint.2018.04.041
Le-Vo, H.-N., Tran, P. T.-B., Le, L., Matsumoto, Y., Motooka, D., Nakamura, S., et al. (2019). Complex Class 1 Integron in a Clinical Escherichia coli Strain from Vietnam Carrying Both Mcr-1 and blaNDM-1. Front. Microbiol. 10, 2472. doi:10.3389/fmicb.2019.02472
Lévesque, C., Piché, L., Larose, C., and Roy, P. H. (1995). PCR Mapping of Integrons Reveals Several Novel Combinations of Resistance Genes. Antimicrob. Agents Chemother. 39 (1), 185–191. doi:10.1128/aac.39.1.185
Li, L.-G., Huang, Q., Yin, X., and Zhang, T. (2020). Source Tracking of Antibiotic Resistance Genes in the Environment - Challenges, Progress, and Prospects. Water Res. 185, 116127. doi:10.1016/j.watres.2020.116127
Liang, J., Mao, G., Yin, X., Ma, L., Liu, L., Bai, Y., et al. (2020). Identification and Quantification of Bacterial Genomes Carrying Antibiotic Resistance Genes and Virulence Factor Genes for Aquatic Microbiological Risk Assessment. Water Res. 168, 115160. doi:10.1016/j.watres.2019.115160
Lira, F., Vaz-Moreira, I., Tamames, J., Manaia, C. M., and Martínez, J. L. (2020). Metagenomic Analysis of an Urban Resistome before and after Wastewater Treatment. Sci. Rep. 10 (1), 8174. doi:10.1038/s41598-020-65031-y
Liu, M., Hata, A., Katayama, H., and Kasuga, I. (2020). Consecutive Ultrafiltration and Silica Adsorption for Recovery of Extracellular Antibiotic Resistance Genes from an Urban River. Environ. Pollut. 260, 114062. doi:10.1016/j.envpol.2020.114062
Ma, L., Li, A.-D., Yin, X.-L., and Zhang, T. (2017). The Prevalence of Integrons as the Carrier of Antibiotic Resistance Genes in Natural and Man-Made Environments. Environ. Sci. Technol. 51 (10), 5721–5728. doi:10.1021/acs.est.6b05887
Maehana, S., Eda, R., Hirabayashi, A., Niida, N., Nakamura, M., Furukawa, T., et al. (2021). Natural Factories that Manufacture Antimicrobial Resistance Genes: Quadruple blaGES-Carrying Plasmids in Aeromonas and Pseudomonas Species. Int. J. Antimicrob. Agents 57 (5), 106327. doi:10.1016/j.ijantimicag.2021.106327
Malek, M. M., Amer, F. A., Allam, A. A., El-Sokkary, R. H., Gheith, T., and Arafa, M. A. (2015). Occurrence of Classes I and II Integrons in Enterobacteriaceae Collected from Zagazig University Hospitals, Egypt. Front. Microbiol. 6, 601. doi:10.3389/fmicb.2015.00601
Mao, D., Yu, S., Rysz, M., Luo, Y., Yang, F., Li, F., et al. (2015). Prevalence and Proliferation of Antibiotic Resistance Genes in Two Municipal Wastewater Treatment Plants. Water Res. 85, 458–466. doi:10.1016/j.watres.2015.09.010
Martínez, J. L. (2019). “Ecology and Evolution of Chromosomal Gene Transfer between Environmental Microorganisms and Pathogens,” in Microbial Transmission. Editors F. E. BaqueroBouza, J. A. Gutiérrez-Fuentes, and T. M. Coque (Washington D.C.: American Society for Microbiology Press), 139–160.
Michael, I., Rizzo, L., McArdell, C. S., Manaia, C. M., Merlin, C., Schwartz, T., et al. (2013). Urban Wastewater Treatment Plants as Hotspots for the Release of Antibiotics in the Environment: a Review. Water Res. 47 (3), 957–995. doi:10.1016/j.watres.2012.11.027
Mirahsani, M., Khorshidi, A., Moniri, R., and Reza Gilasi, H. (2016). Prevalence of Class 1 Integron, Resistance Gene Cassettes and Antimicrobial Susceptibility Profiles Among Isolates of Pseudomonas aeruginosa in Iran. Ojmm 6 (2), 87–96. doi:10.4236/ojmm.2016.62012
Moura, A., Soares, M., Pereira, C., Leitao, N., Henriques, I., and Correia, A. (2009). INTEGRALL: a Database and Search Engine for Integrons, Integrases and Gene Cassettes. Bioinformatics 25 (8), 1096–1098. doi:10.1093/bioinformatics/btp105
Muziasari, W. I., Managaki, S., Pärnänen, K., Karkman, A., Lyra, C., Tamminen, M., et al. (2014). Sulphonamide and Trimethoprim Resistance Genes Persist in Sediments at Baltic Sea Aquaculture Farms but Are Not Detected in the Surrounding Environment. PLoS One 9 (3), e92702. doi:10.1371/journal.pone.0092702
Muziasari, W. I., Pärnänen, K., Johnson, T. A., Lyra, C., Karkman, A., Stedtfeld, R. D., et al. (2016). Aquaculture Changes the Profile of Antibiotic Resistance and mobile Genetic Element Associated Genes in Baltic Sea Sediments. FEMS Microbiol. Ecol. 92 (4), fiw052. doi:10.1093/femsec/fiw052
Muziasari, W. I., Pitkänen, L. K., Sørum, H., Stedtfeld, R. D., Tiedje, J. M., and Virta, M. (2017). The Resistome of Farmed Fish Feces Contributes to the Enrichment of Antibiotic Resistance Genes in Sediments below Baltic Sea Fish Farms. Front. Microbiol. 7, 2137. doi:10.3389/fmicb.2016.02137
Nguyen, N. T., Liu, M., Katayama, H., Takemura, T., and Kasuga, I. (2021). Association of the Colistin Resistance Gene Mcr‐1 with Faecal Pollution in Water Environments in Hanoi, Vietnam. Lett. Appl. Microbiol. 72 (3), 275–282. doi:10.1111/lam.13421
Nguyen, T. N., Kasuga, I., Liu, M., and Katayama, H. (2019). Occurrence of Antibiotic Resistance Genes as Emerging Contaminants in Watersheds of Tama River and Lake Kasumigaura in Japan. IOP Conf. Ser. Earth Environ. Sci. 266, 012003. doi:10.1088/1755-1315/266/1/012003
Nnadozie, C. F., and Odume, O. N. (2019). Freshwater Environments as Reservoirs of Antibiotic Resistant Bacteria and Their Role in the Dissemination of Antibiotic Resistance Genes. Environ. Pollut. 254 (Pt B), 113067. doi:10.1016/j.envpol.2019.113067
O'Neill, J. (2016). “Tackling Drug-Resistant Infections Globally: Final Report and Recommendations,” in Review on Antimicrtobial Resistance (London: Government of the United Kingdom).
Pärnänen, K. M. M., Narciso-da-Rocha, C., Kneis, D., Berendonk, T. U., Cacace, D., Do, T. T., et al. (2019). Antibiotic Resistance in European Wastewater Treatment Plants Mirrors the Pattern of Clinical Antibiotic Resistance Prevalence. Sci. Adv. 5 (3), eaau9124. doi:10.1126/sciadv.aau9124
Pruden, A., Vikesland, P. J., Davis, B. C., and de Roda Husman, A. M. (2021). Seizing the Moment: Now Is the Time for Integrated Global Surveillance of Antimicrobial Resistance in Wastewater Environments. Curr. Opin. Microbiol. 64, 91–99. doi:10.1016/j.mib.2021.09.013
Quintela-Baluja, M., Abouelnaga, M., Romalde, J., Su, J.-Q., Yu, Y., Gomez-Lopez, M., et al. (2019). Spatial Ecology of a Wastewater Network Defines the Antibiotic Resistance Genes in Downstream Receiving Waters. Water Res. 162, 347–357. doi:10.1016/j.watres.2019.06.075
Razavi, M., Marathe, N. P., Gillings, M. R., Flach, C.-F., Kristiansson, E., and Joakim Larsson, D. G. (2017). Discovery of the Fourth mobile Sulfonamide Resistance Gene. Microbiome 5 (1), 160. doi:10.1186/s40168-017-0379-y
Rodriguez-Mozaz, S., Chamorro, S., Marti, E., Huerta, B., Gros, M., Sànchez-Melsió, A., et al. (2015). Occurrence of Antibiotics and Antibiotic Resistance Genes in Hospital and Urban Wastewaters and Their Impact on the Receiving River. Water Res. 69, 234–242. doi:10.1016/j.watres.2014.11.021
Samreen, , Ahmad, I., Malak, H. A., and Abulreesh, H. H. (2021). Environmental Antimicrobial Resistance and its Drivers: a Potential Threat to Public Health. J. Glob. Antimicrob. Resist. 27, 101–111. doi:10.1016/j.jgar.2021.08.001
Schmittgen, T. D., and Livak, K. J. (2008). Analyzing Real-Time PCR Data by the Comparative CT Method. Nat. Protoc. 3 (6), 1101–1108. doi:10.1038/nprot.2008.73
Stachler, E., Crank, K., and Bibby, K. (2019). Co-occurrence of crAssphage with Antibiotic Resistance Genes in an Impacted Urban Watershed. Environ. Sci. Technol. Lett. 6 (4), 216–221. doi:10.1021/acs.estlett.9b00130
Stalder, T., Press, M. O., Sullivan, S., Liachko, I., and Top, E. M. (2019). Linking the Resistome and Plasmidome to the Microbiome. ISME J. 13 (10), 2437–2446. doi:10.1038/s41396-019-0446-4
Stedtfeld, R. D., Guo, X., Stedtfeld, T. M., Sheng, H., Williams, M. R., Hauschild, K., et al. (2018). Primer Set 2.0 for Highly Parallel qPCR Array Targeting Antibiotic Resistance Genes and mobile Genetic Elements. FEMS Microbiol. Ecol. 94 (9), fiy130. doi:10.1093/femsec/fiy130
Suzuki, Y., Hashimoto, R., Xie, H., Nishimura, E., Nishiyama, M., Nukazawa, K., et al. (2019). Growth and Antibiotic Resistance Acquisition of Escherichia coli in a River that Receives Treated Sewage Effluent. Sci. Total Environ. 690, 696–704. doi:10.1016/j.scitotenv.2019.07.050
Tsutsui, H., and Urase, T. (2019). Characterization of Extended Spectrum β-lactamase-producing Escherichia coli in the Environment Isolated with Different Concentrations of Cefotaxime. J. Wat. Envir. Tech. 17 (4), 262–272. doi:10.2965/jwet.18-091
Urase, T., and Sato, T. (2016). Quantitative Monitoring of Resistance in Escherichia coli to Clinically Important Antimicrobials in an Urban Watershed. J. Wat. Envir. Tech. 14 (5), 341–349. doi:10.2965/jwet.16-002
Wang, F.-H., Qiao, M., Su, J.-Q., Chen, Z., Zhou, X., and Zhu, Y.-G. (2014). High Throughput Profiling of Antibiotic Resistance Genes in Urban Park Soils with Reclaimed Water Irrigation. Environ. Sci. Technol. 48 (16), 9079–9085. doi:10.1021/es502615e
Wang, Y., Xu, J., Kong, L., Li, B., Li, H., Huang, W. E., et al. (2020). Raman‐activated Sorting of Antibiotic‐resistant Bacteria in Human Gut Microbiota. Environ. Microbiol. 22 (7), 2613–2624. doi:10.1111/1462-2920.14962
Waseem, H., Jameel, S., Ali, J., Saleem Ur Rehman, H., Tauseef, I., Farooq, U., et al. (2019). Contributions and Challenges of High Throughput qPCR for Determining Antimicrobial Resistance in the Environment: a Critical Review. Molecules 24, 163. doi:10.3390/molecules24010163
Wei, Q., Jiang, X., Yang, Z., Chen, N., Chen, X., Li, G., et al. (2008). dfrA27, a New Integron-Associated Trimethoprim Resistance Gene from Escherichia coli. J. Antimicrob. Chemother. 63 (2), 405–406. doi:10.1093/jac/dkn474
Weirather, J. L., de Cesare, M., Wang, Y., Piazza, P., Sebastiano, V., Wang, X.-J., et al. (2017). Comprehensive Comparison of Pacific Biosciences and Oxford Nanopore Technologies and Their Applications to Transcriptome Analysis. F1000Res 6, 100. doi:10.12688/f1000research.10571.2
WHO (2021a). Global Antimicrobial Resistance and Use Surveillance System (GLASS) Report 2021. Genova: World Health Organization.
WHO (2021b). WHO Integrated Global Surveillance on ESBL-Producing E. coli Using a “One Health” Approach: Implementation and Opportunities. Genova: World Health Organization.
Xu, L., Ouyang, W., Qian, Y., Su, C., Su, J., and Chen, H. (2016). High-throughput Profiling of Antibiotic Resistance Genes in Drinking Water Treatment Plants and Distribution Systems. Environ. Pollut. 213, 119–126. doi:10.1016/j.envpol.2016.02.013
Yamashita, N., Katakawa, Y., and Tanaka, H. (2017). Occurrence of Antimicrobial Resistance Bacteria in the Yodo River basin, Japan and Determination of Beta-Lactamases Producing Bacteria. Ecotoxicology Environ. Saf. 143, 38–45. doi:10.1016/j.ecoenv.2017.04.053
Yu, Q., Feng, T., Yang, J., Su, W., Zhou, R., Wang, Y., et al. (2022). Seasonal Distribution of Antibiotic Resistance Genes in the Yellow River Water and Tap Water, and Their Potential Transmission from Water to Human. Environ. Pollut. 292, 118304. doi:10.1016/j.envpol.2021.118304
Zhang, A. N., Li, L.-G., Ma, L., Gillings, M. R., Tiedje, J. M., and Zhang, T. (2018). Conserved Phylogenetic Distribution and Limited Antibiotic Resistance of Class 1 Integrons Revealed by Assessing the Bacterial Genome and Plasmid Collection. Microbiome 6 (1), 130. doi:10.1186/s40168-018-0516-2
Zhao, X., Hu, M., Zhang, Q., Zhao, C., Zhang, Y., Li, L., et al. (2020). Characterization of Integrons and Antimicrobial Resistance in Salmonella from Broilers in Shandong, China. Poult. Sci. 99 (12), 7046–7054. doi:10.1016/j.psj.2020.09.071
Zheng, W., Huyan, J., Tian, Z., Zhang, Y., and Wen, X. (2020). Clinical Class 1 Integron-Integrase Gene - A Promising Indicator to Monitor the Abundance and Elimination of Antibiotic Resistance Genes in an Urban Wastewater Treatment Plant. Environ. Int. 135, 105372. doi:10.1016/j.envint.2019.105372
Keywords: Antimicrobial resistance gene, class 1 integron, gene cassette, high-throughput quantitative PCR, urban river
Citation: Kasuga I, Nagasawa K, Suzuki M, Kurisu F and Furumai H (2022) High-Throughput Screening of Antimicrobial Resistance Genes and Their Association With Class 1 Integrons in Urban Rivers in Japan. Front. Environ. Sci. 10:825372. doi: 10.3389/fenvs.2022.825372
Received: 30 November 2021; Accepted: 14 January 2022;
Published: 04 February 2022.
Edited by:
Zhi Wang, Innovation Academy for Precision Measurement Science and Technology (CAS), ChinaReviewed by:
Miguel Ignacio Uyaguari-Diaz, University of Manitoba, CanadaNaresh Devarajan, University of California, United States
Copyright © 2022 Kasuga, Nagasawa, Suzuki, Kurisu and Furumai. This is an open-access article distributed under the terms of the Creative Commons Attribution License (CC BY). The use, distribution or reproduction in other forums is permitted, provided the original author(s) and the copyright owner(s) are credited and that the original publication in this journal is cited, in accordance with accepted academic practice. No use, distribution or reproduction is permitted which does not comply with these terms.
*Correspondence: Ikuro Kasuga, a2FzdWdhQGVudi50LnUtdG9reW8uYWMuanA=