- 1Geographical Institute “Jovan Cvijić”, Serbian Academy of Sciences and Arts, Belgrade, Serbia
- 2Institute of Sports, Tourism and Service, South Ural State University, Chelyabinsk, Russia
This study has analyzed the traffic-related change in atmospheric pollutants levels (PM2.5, PM10, CO, NO2, SO2, and O3) caused by the COVID-19 restrictive measures, based on traffic ground-based stations data in urban areas in Serbia. The possible influence of several meteorological factors (temperature, wind, pressure, and humidity), and mobility on the pollutants’ levels were also considered. The obtained results showed a positive correlation of daily NO2 concentrations with mobility and its significant reduction during restrictive measures at all selected monitoring stations. The reduction of NO2 was higher than in other countries (71.1–111.5% for measured, and 49.3–92.6% for “deweathered” data), indicating a high traffic impact on NO2 levels in Serbia. The PM, CO, and SO2 showed a weak correlation with mobility during the period with restrictive measures, which, besides traffic, indicates the significant influence of other sources of their concentration. The O3 concentrations were increased at all measuring stations and are negatively correlated to mobility. Comparison of pollutant concentrations during restriction with the equivalent period in preceding years showed reductions in NO2 and SO2 concentrations. However, compared to previous years, the concentrations of PM2.5, PM10, and CO increased in the period with restrictive measures, indicating lower sensitivity to population mobility and higher dependence on other emission sources. The findings suggest the justification for the use of traffic reduction strategies to improve air quality.
Introduction
Air quality is one of the dominant factors that affect health and quality of life. The harmful effects of air pollution on health are well documented, especially when it comes to pollutants such as particulate matter, nitrogen, and sulfur oxides, carbon monoxide, and ozone (Setton et al., 2011; Sujaritpong et al., 2014; Likhvar et al., 2015; WHO, 2021; etc). According to WHO (2018), air pollution is responsible for 26% of deaths from respiratory diseases, 25% of cases of chronic obstructive pulmonary disease, and 17% of cases of ischemic heart disease. Besides the influence on mortality and morbidity, several studies conducted in major cities around the world have proven the harmful effects of air pollutants on the course and prognosis of acute and chronic diseases in adults and children (Elliot et al., 2016; Weber et al., 2016; Xu et al., 2016).
During the Coronavirus Disease 2019 (COVID-19) pandemic restrictive measures, many human activities in the fields of transport, economy, and education were globally limited to prevent the further spread of the virus. Restrictions on human activity have resulted in significant reductions in pollutant emissions and have given scientists a unique opportunity to assess the impact of limited anthropogenic emissions on air quality. Existing country-specific case studies have reported a reduction in air pollution during the implementation of restrictive measures, which may be the result of reduced transport. The majority of them showed that nitrogen oxide (NOx) concentrations significantly decreased, while ground-level ozone (O3) concentrations increased (Baldasano, 2020; Bekbulat et al., 2021; Briz-Redón et al., 2021; Chen et al., 2021; Munir et al., 2021; Prats et al., 2021; etc). Lockdown had a positive effect on particle concentrations (PM2.5, PM10) in many countries but with a less pronounced effect (Menut et al., 2020; Betancourt-Odio et al., 2021; Ropkins and Tate, 2021; Shi et al., 2021). Some studies also found an increase in particulate matter (PM) concentration due to unfavorable meteorological conditions, the contribution from other sources, and transport from remote areas (Munir et al., 2021; Song et al., 2021). Sulfur dioxide (SO2) and carbon monoxide (CO) also showed a decrease during the lockdown, but it has not been consistent (Grivas et al., 2020; Briz-Redón et al., 2021; Chen et al., 2021; Filonchyk et al., 2021). Lenzen et al. (2020) and Venter et al. (2020) examined the pandemic impacts on global air quality levels. Lenzen et al. (2020) found a reduction in greenhouse gases, PM2.5, and gaseous air pollutants (SO2 and NO2) by 4.6, 3.8, and 2.9% of the global annual levels, respectively, while Venter et al. (2020) reported a decrease of NO2 and PM2.5 by approximately 60 and 31%.
The first case of coronavirus in Serbia was confirmed on March 6, 2020. On March 15, 2020, the Serbian Government declared a state of emergency (from midnight on March 16). From then on, a series of partial and strict restrictive measures were successively undertaken to limit the spread of COVID-19, including the closure of schools and universities, travel restrictions, working at home, closure of catering and sales facilities, and ban on public gatherings. During the holidays and the majority of weekends, starting from Friday afternoon, a full weekend curfew was imposed on the entire territory of Serbia. After seven and a half weeks of measures, and obtaining successful outcomes, the state of emergency was lifted on May 6, 2020.
Studies on the impact caused by the COVID-19 outbreak on air pollution in Serbia are quite limited. So far, the effects of the lockdown on the level of air pollutants have been investigated in one city (Novi Sad), only for April 2020. Davidović et al. (2021) showed that PM2.5, PM10, and SO2 levels during restrictive measures, did not change noticeably, compared to the pre-restrictive measures period. Having in mind the fact that the impact of restrictive measures on air pollution has not been analyzed in other cities in Serbia, and that measures have mostly affected traffic reduction, the following study aimed to assess the changes in the air quality at traffic air pollution stations linked to COVID-19 lockdown restrictions. In particular, the study focuses on the several criteria air pollutants (PM2.5, PM10, NO2, SO2, CO, and O3), evaluating: 1) effect of meteorological factors and population mobility on air pollution levels; 2) variation in daily concentrations between February 1 and June 30, 2020 (before, during, and after restrictive measures), and 3) difference in the mean concentration in 2020 compared to average concentration during the equivalent period in previous years.
Material and Methods
Study Area
The Republic of Serbia is positioned in Southeast Europe on the Balkan Peninsula, between latitudes 41°and 47° N and longitudes 18° and 23° E. The country covers an area of 88,499 km2 (Statistical Office of the Republic of Serbia, 2020). The northern part of the country is lowland, while the altitude increases to the south. The estimated population in Serbia in 2020 was 6,899,126 (Statistical Office of the Republic of Serbia, 2021). The population is mainly concentrated at lower altitudes in northern and central areas and along the river valleys. Nearly 60% of Serbia’s population lives in urban areas (Drobnjaković and Spalević, 2017).
This study covers urban areas with traffic stations for measuring air quality, which have different sizes and population densities (Figure 1). The largest by far is the nation’s capital, Belgrade (BG), with a population of 1.7 million in the total city area (1.2 million in the urban city area). Novi Sad (NS) is the second, while Niš (NI) is the third-largest city in Serbia, with a population of 360,000 and 260,000, respectively. Subotica (SU) and Pančevo (PA) are cities with populations ranging from 100,000 to 150,000 inhabitants, while the population of other cities covered by the study (Valjevo (VA), Sremska Mitrovica (SM), Sombor (SO), and Užice (UE)) is between 70,000 and 85,000 (Statistical Office of the Republic of Serbia, 2020).
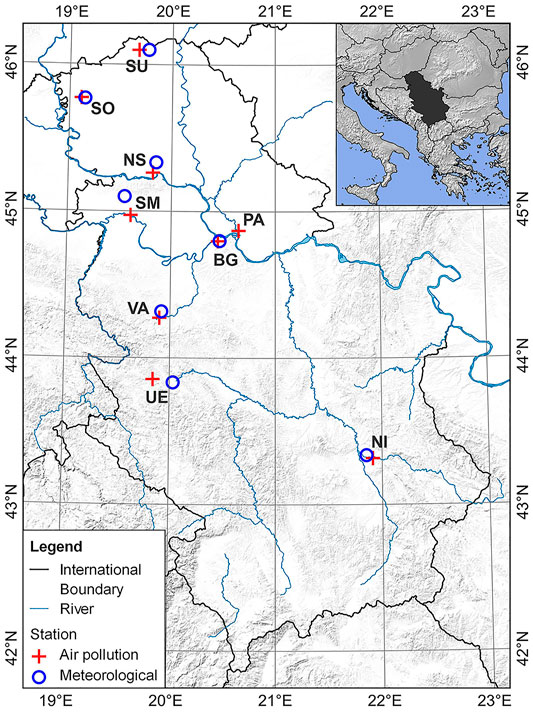
FIGURE 1. Location of the traffic air pollution monitoring and meteorological stations in Serbia used in the study.
The main sources of outdoor air pollution in Serbia comprise the energy production sector, industrial activities, and mining. In almost every urban municipality, traffic and individual domestic heating additionally pollute the air. In some cities, such as UE and VA, the unfavorable geographical position, such as valleys and gorges, further, makes air quality even worse. The major sources of air pollution in BG are electricity production, heating, and industry, while for NOx, the major source is traffic (Serbian Environmental Protection Agency, Ministry of Environmental Protection, Republic of Serbia, 2012; Serbian Environmental Protection Agency, Ministry of Environmental Protection, Republic of Serbia, 2013). The specific sources of air pollution in NS are the petrochemical industry and growing vehicle traffic (WHO, 2019), while in NI dominate boiler rooms, individual furnaces, traffic, and industry. In the UE, the metallurgical industry is responsible for the emission of SO2 and NOx. In PA, the chemical industry is a source of SO2, and NOx, while traffic and individual heating are the sources of PM. In VA, the use of wood and coal for heating households and the frequent occurrence of temperature inversion in the cold part of the year lead to a large excess of PM concentrations (Stanojević et al., 2019). In SU, SO, and SM the main sources of PM are domestic heating and traffic.
Collection and Selection of Data on Air Quality, Meteorology, and Mobility
To analyze the impact of COVID-19 lockdown on air quality, we used air pollution, meteorology, and mobility data. For that purpose, we divided all datasets into three periods: 1) before restrictive measures (BRM), from February 1 to March 14, 2020; 2) during restrictive measures (RM), from March 15 to May 5, 2020, and 3) after restrictive measures (ARM), from May 6 to June 30, 2020. In addition, we extracted and analyzed data for the curfew subperiod inside of the RM period.
Air pollutant concentrations including NO2, SO2, CO, PM2.5, PM10, and O3 for the period 2015–2020 were obtained from the national network of automatic stations for air quality monitoring managed by the Serbian Environmental Protection Agency (SEPA, 2021). All measurements were performed using precision air quality monitoring equipment with quality assurance and quality control protocols for sampling and analysis. According to Regulation on monitoring conditions and air quality requirements (2013) checking the quality of measurements, method of processing, display of results, and assessment of their reliability, as well as adjustment and calibration of measuring instruments is carried out according to the prescribed measurement methods and requirements of SRPS ISO/IEC 17025 (Institute for standardization of Serbia, 2021). The maximum measurement uncertainty of the measured data was within the limits prescribed in the Regulation on monitoring conditions and air quality requirements (2013). For a 95% confidence interval, the maximum measurement uncertainty was 15% for NO2, SO2, CO, and O3, and 25% for PM10 and PM2.5. Uncertainty evaluation was done according to the methodology described in the European Committee for Standardization (CEN, French: Comité Européen de Normalisation) report ‘‘Approach to uncertainty estimation for ambient air reference measurement methods’’ (CEN, 2002) following the European Framework Directive10 on Ambient Air Quality and the associated Daughters Directives. The national network consists of samplers/analyzers that detect and analyze the concentration of air pollutants 24 h/day throughout the year according to reference technical standards (SRPS EN 14211, SRPS EN 14212, SRPS EN 14626, SRPS EN 14907, SRPS ЕN 12,341, SRPS EN 14625) (Institute for standardization of Serbia, 2021). The air sampling takes place hourly, using TELEDYNE API Model 200A for NO2, TELEDYNE API Model 100E for SO2, TELEDYNE API Model 300A for CO, GRIMM EDM 180 for PM2.5, TCR TECORA Skypost HV for PM10, and TELEDYNE API Model 400A for O3. Measurements and data processing were identical at all stations. We downloaded data for the period 2015–2020 and restricted them to consider a specific window of days each year between February 1 and June 30 (150 or 151 days). Before processing, to remove faulty data and inaccurate measurements resulting from the response error of the sensor, each data was subject to quality control by using different control tests similar to those described by Dunn et al. (2012): the existence of gaps in data series, occurrences of physically impossible values and long consecutive, and occurrences of the same value. All data (each monitoring station and each year) were verified to determine if they will be included in the study. If any monitoring station in each year contains <70% of data in the specified window (70% × 150 days × 24 h = 2520 data) it is excluded from further analysis. Each period (before, during, and after the restrictive measures) was checked whether it contained more than 70% of the data and if not, it was excluded from further analysis. The monitoring stations with a sufficient number of years before 2020 were further processed to calculate base averages. Each station with less than 3 years of measurements in the period 2015–2019 is excluded from the analysis. After a completeness check, data from three PM2.5 (BG, NS, NI), three PM10 (BG, NS, NI), six NO2 (BG, NS, NI, VA, UE, SM), five SO2 (BG, NS, NI, UE, PA), seven CO (BG, NS, VA, UE, SU, SO, SM), and three O3 (PA, SU, SO) traffic urban air pollution monitoring stations were used.
Meteorology data including wind speed (ws), wind direction (wd), air temperature (T), air pressure (P), and relative humidity (RH) for each city were downloaded from the National Oceanic and Atmospheric Administration Integrated Surface Database (NOAA, 1901—present). Meteorological data were used to deweather air quality data and estimate the impact of meteorological conditions on air quality during the analyzed period.
Serbia’s community mobility data were downloaded from the Google Community Mobility Reports (Google, 2021) and Apple’s COVID-19 mobility trend (Apple Inc, 2020). Google datasets show daily changes in the number of visitors to specific locations (e.g., grocery stores; parks; transit stations) relative to the median baseline level before the pandemic outbreak, where the baseline level represents a normal value for that day of the week, given as median value over the five weeks from January 3 to February 6, 2020. Google reports usually contain data for the period February 15 - December 31, 2020, however, the report for Serbia lacks data for the period May 19– July 3, 2020. Apple’s mobility trend reports show how human mobility has changed relative to January 13, 2020. Google transit station data and Apple driving data were used in this analysis.
Deweathering of Air Quality Data and Statistical Analysis
Meteorology plays an important role in air pollutant concentrations. Considering trends, it is difficult to know whether a change in concentration is a consequence of emissions or meteorology. Therefore, this analysis included measured and ‘deweathered’ data. The ‘deweather’ package in the R programing language (Carslaw, 2020) was used to remove the influence of meteorology from air quality time series. This package is based on boosted regression trees statistical technique using the gbm package (Ridgeway, 2017). It takes into account the complex interactions and non-linear relationships between the variables. Without the need for prior data transformation or elimination of outliers, it is possible to select relevant variables, fit accurate functions and automatically identify and model interactions (Elith et al., 2008). The advantage of the technique is to assess how a dependent variable reacts to individual model variables, the relative importance of different variables for prediction, and the possibility to determine, rank, and visualize interactions between variables (Carslaw and Taylor, 2009). Five meteorological (T, ws, wd, P, and RH) and two temporal (hour of the day-h and day of the week-wdy) predictors were used to develop models for each air pollutant and each measuring station. Each model was built using 80% of the random data, while the rest of the data was used for validation. This resulted in the “deweathered” data showing the changes in the concentrations of air pollutants as emission products. The boosted regression tree technique is also used to examine the effect of meteorological conditions on air pollutants by exploring partial dependencies. The partial dependencies show the relationship between the pollutant and the covariates used in the model while keeping the value of the other covariates at their mean level.
Statistical data analysis was performed using R programming language and Rstudio software (R Core Team, 2013; RStudio Team, 2015). Plots of pollutant time variations and correlations between air pollutants and meteorological parameters were developed using a purpose-built for air quality analysis—openair (Carslaw and Ropkins, 2012). To determine the statistical significance of differences in 2020 air pollutant levels versus concentration during the same period in previous years, both observed and ‘deweathered’ data were compared using a t-test. Values of p < 0.05 were considered statistically significant.
Results and Discussion
Influence of Meteorological Factors and Population Mobility on Air Pollution
The interactions between the most influential covariates and air pollutants are shown in Figure 2. The air temperature and wind speed were the most important variables affecting PM2.5, PM10, and CO concentrations and show a negative correlation. The concentrations of SO2 were the most affected by low relative humidity and high temperatures, while the concentrations of NO2 are closely related to traffic flows and tend to be higher during the afternoon traffic peak. Concentrations of O3 concentrations are strongly positively correlated with air temperature, and also inversely correlated with relative humidity.
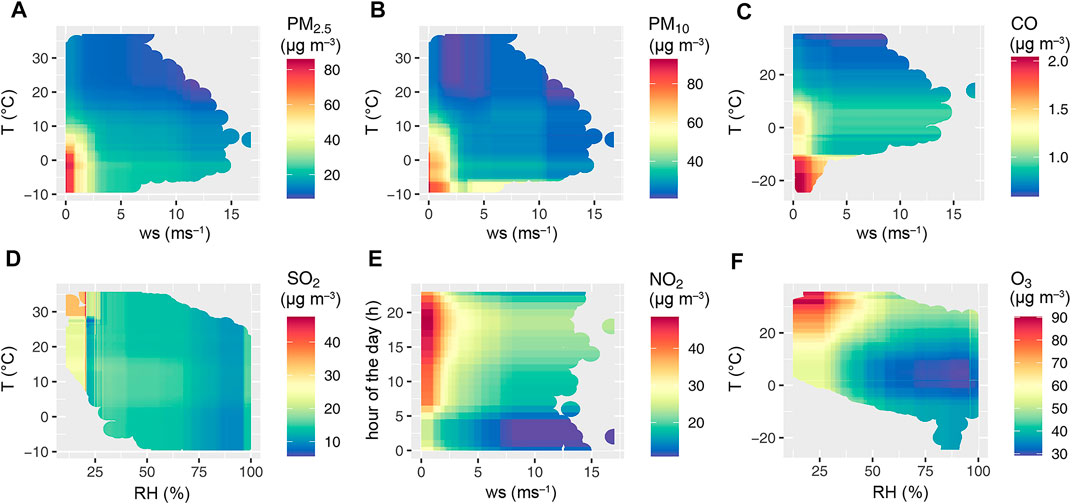
FIGURE 2. Two-way interactions between the most influential covariates: (A) PM2.5 - air temperature (T) and wind speed (ws); (B) PM10 - air temperature (T) and wind speed (ws); (C) CO- air temperature (T) and wind speed (ws); (D) SO2 - air temperature (T) and relative humidity (RH); (E) NO2 -hour of the day (h) and wind speed (ws); and (F) O3 - air temperature (T) and relative humidity (RH).
The overall effects of the weather conditions on air pollution levels before, during, and after the restrictive measures (BRM, RM, ARM) were shown in Table 1. Differences between measured and “deweathered” mean concentrations were shown as percentages (%). The negative signs show a reduction in measured air pollutant concentrations due to favorable weather conditions, while positive signs indicate an increase in the measured concentration caused by unfavorable weather conditions. During the BRM period, weather conditions were slightly unfavorable in most places, resulting in a slight deterioration in air quality. The exceptions were the stations in the north of the country (SU, SO, and PA), where the weather conditions led to the purification of the air. During the RM period, the weather conditions were the most unfavorable for PM and O3 at all stations. However, the weather had a positive impact on the levels of NO2 in all cases, and on SO2 at the majority of stations (except in NS and PA). When it comes to CO, weather conditions have worsened the air quality in the central and southwestern parts of the country, while in places in the north and northwest (SU, SO, and SM) it had a positive effect. In the ARM period, the weather had the most unfavorable effect on NO2 concentrations in all places.
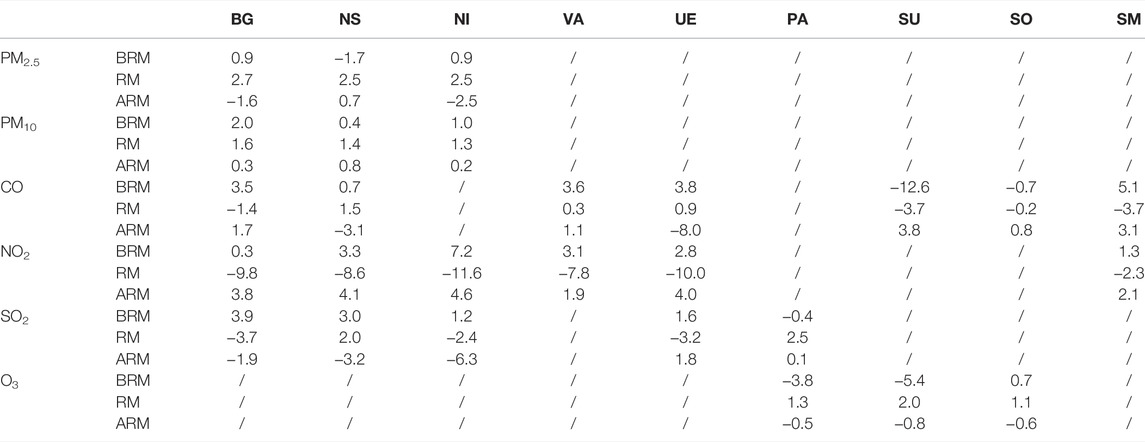
TABLE 1. Differences between measured and “deweathered” mean concentrations were shown as percentages (%) before (BRM), during (RM), and after the restrictive measures (ARM). ‘/’ means that there is no measurement of a specific air pollutant at this particular station.
A significant reduction in mobility over the RM period is expected to reduce vehicle emissions and lead to an improvement in air quality. Changes in mobility data as a percentage for the whole of Serbia for the period February 1—June 30, 2020 are shown in Figure 3. Both sets of mobility data, Google and Apple, showed a sharp decline from the weekend before the start of the implementation of the restrictive measures, which began on March 15, 2020. Later, mobility data showed a slightly growing trend from the second week of April 2020 until the end of the RM. There is a noticeable decline in mobility during curfew on weekends, preceded by a slight increase on Fridays. After the RM period, mobility was constantly growing until the second week of June, when it returned to the BRM level.
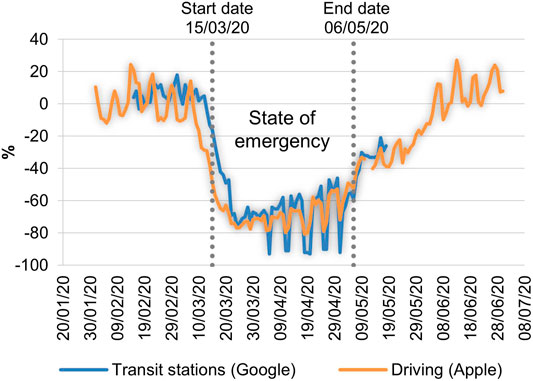
FIGURE 3. Changes in mobility data (%) in Serbia during the period February–June 2020. The vertical lines show the start and end of the restrictive measures period.
To investigate the strength of the linear relationship a correlation between the average mobility trend (Google data) and “deweathered” air pollution levels was performed (Table 2). The Pearson correlation coefficients are presented in Table 2, whereby values lower than 0.40 are considered weak, while values higher than 0.70 are considered a strong correlation.

TABLE 2. Correlation analysis between Google mobility data and air pollutant concentrations at different monitoring stations in the restrictive measures period (RM).
The highest positive correlation with mobility had NO2 concentrations, with a moderate statistically significant R-value of 0.60. This was expected as NOx is mostly emitted from traffic in urban areas. The percentage of the mobility and NO2 concentrations reduction was similar (–67% vs. –61%, respectively) indicating a close cause-and-effect relationship. The correlations between PM and SO2 on the one hand and mobility, on the other hand, were low and statistically insignificant, so they can be considered negligible indicating the higher effect of other emission sources, such as individual heating. A statistically significant positive correlation between CO and mobility was expected because CO is an important component of traffic exhaust fumes. O3 concentrations had a weak, but statistically significant, negative correlation with mobility. A negative correlation of O3 was expected because lower NOx emissions from motor vehicles cause an increase in the ratio of volatile organic compounds (VOC) to NOx, which leads to higher surface O3 concentrations.
Changes in Air Pollutant Concentrations in BRM, RM, and ARM Periods
Variations of the measured and ‘deweathered’ concentrations of air pollutants in percent (%) for the RM and ARM periods compared to the BRM period are presented in Figure 4. The hourly and weekly cycles of all measured pollutants for BRM, RM, and ARM periods are given in Figure 5.
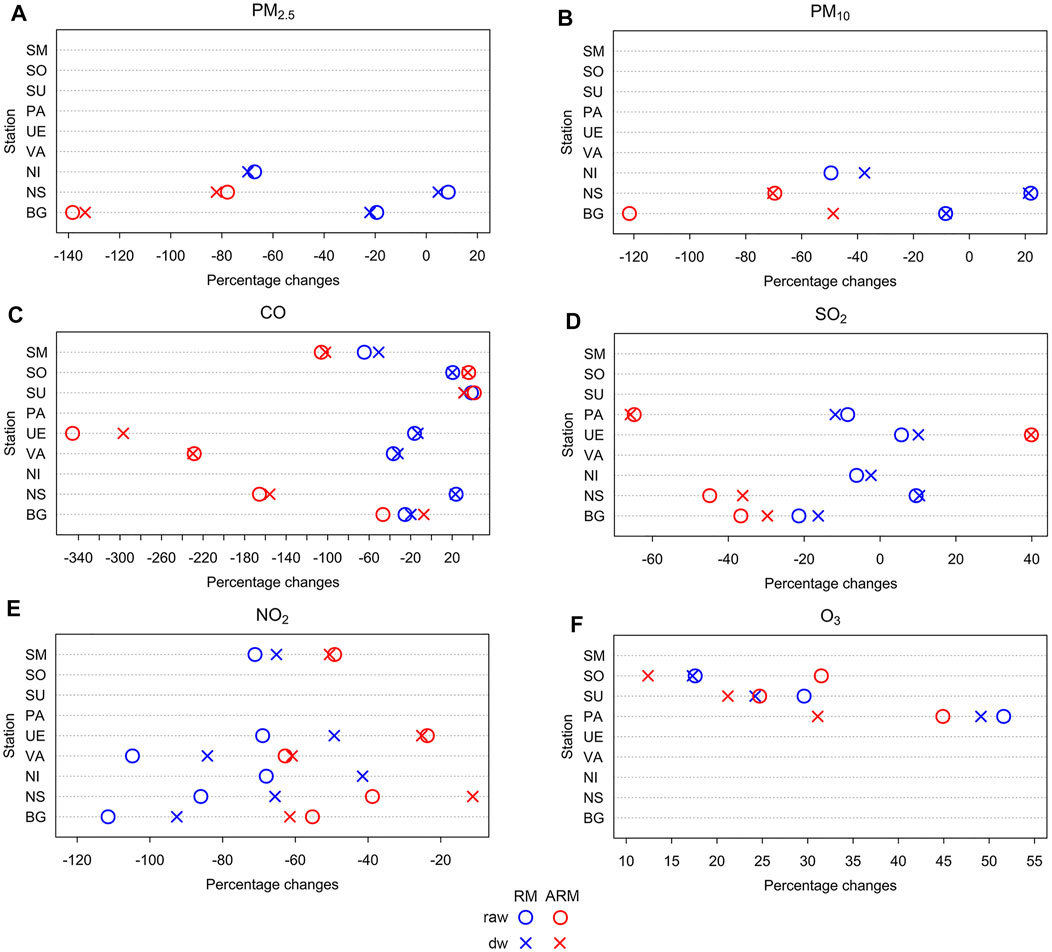
FIGURE 4. Percentage changes of city-specific pollutant concentrations in restrictive measures (RM) and after the restrictive measures (ARM) periods compared to before restrictive measures (BRM) periods for raw and “deweathered” data for (A) PM2.5, (B) PM10, (C) CO, (D) SO2, (E) NO2, and (F) O3.
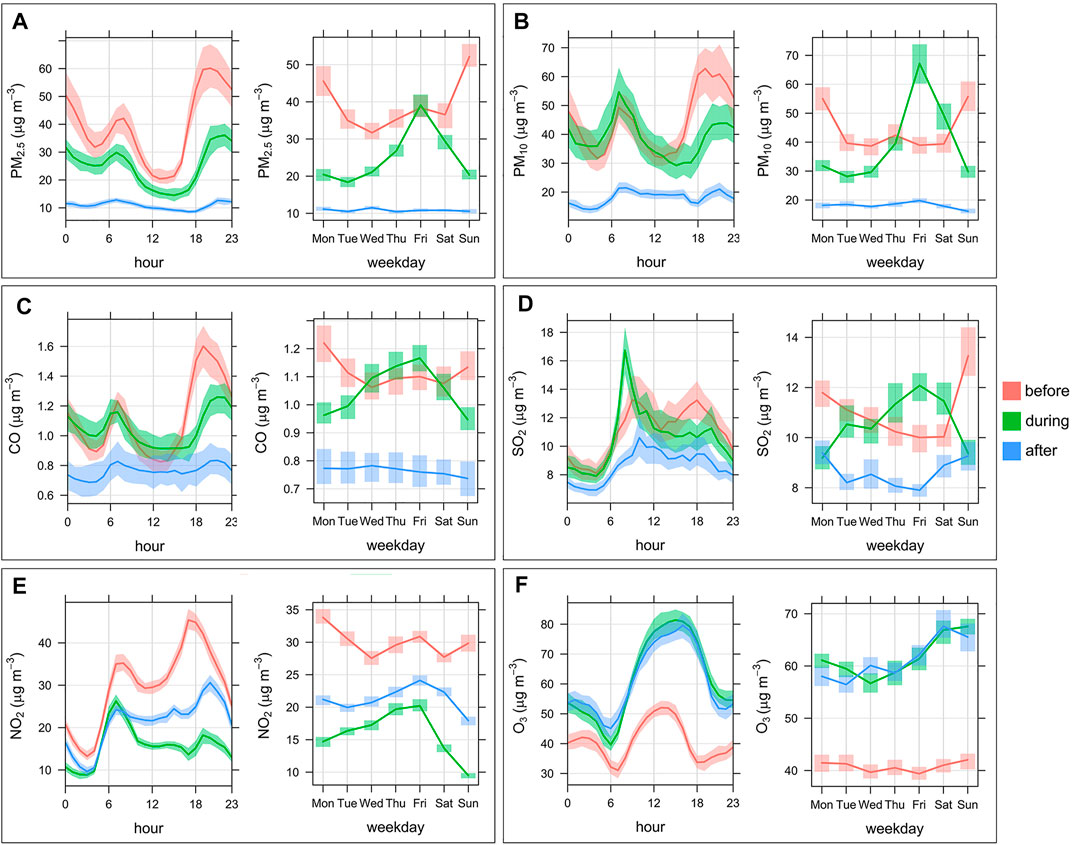
FIGURE 5. Hourly and weekly cycles of pollutants concentrations in Serbia before (BRM), during (RM), and after the restrictive measures (ARM) periods for (A) PM2.5, (B) PM10, (C) CO, (D) 2, (E) NO2, and (F) O3.
Measured and ‘deweathered’ NO2 concentrations decreased significantly at all measurement sites during the RM period (71.1–111.5%, and 49.3–92.6%, respectively). The percentage of decrease was higher than those reported in the other studies (Grivas et al., 2020; Donzelli et al., 2021; Fenech et al., 2021; Shi et al., 2021), indicating that the impact of traffic on NO2 levels in Serbia is greater than in other countries. The substantial impact of traffic on air pollution in Serbia is due to the fact that vehicles in Serbia (and in groups in Southeast Europe in general) emit significantly more air pollutants than in the rest of Europe. Although Serbia has solved the problem of importing vehicles with particularly high air pollutants by prohibiting imports of old vehicles and vehicles with low European emission standards, the vehicle stocks in Serbia consist of vehicles with an average age of more than 11 years and a low proportion of new vehicles (Velten et al., 2020). Serbia imports low-cost vehicles, which have a substantial impact on air pollution due to excessive kilometers traveled or other shortcomings of vehicles that lower their price. In the ARM period, NO2 levels increased relative to the RM period (10–30% for raw, and 9–20% for “deveathered” data), but concentrations remained below those in the BRM period (23.7–62.8% for raw, and 11.2–61.5% for “deveathered” data). This was expected because population mobility during the ARM period was still lower than during the BRM period. Changes in O3 concentrations were also uniform through all measuring stations. Similar to other studies (Chen et al., 2021; Fenech et al., 2021; Hernández-Paniagua et al., 2021; Song et al., 2021), during the RM and ARM periods, there is an increase in O3 levels (Figure 4F). Being a secondary pollutant, the production of O3 is associated with the changes in meteorological conditions and NO2 and VOCs concentrations. The decrease in NOx from motor vehicles in the RM period, while the amount drop of VOC is not as large as NOx, is linked to a reduced titration effect of O3 by NO, and leads to higher O3 concentrations. An increase in O3 in the late spring and early summer ARM period is associated with higher insolation and temperatures.
For PM, the relationship between the decrease in PM10 and PM2.5 concentration and restrictive measures was unclear and inconsistent (Figures 4A,B). A moderate reduction was observed in BG and NI in comparison to the BRM period, followed by a significant reduction during the ARM period, while NS experienced an increase in PM2.5 and PM10. An increase in PM in NS may indicate the presence of local sources emitting particles that were active in the RM period. According to Davidović et al. (2021), in the period March 26th–28th the transport of particles from the Aralkum Desert in Asia additionally contributed to the increased concentrations in NS. The raw and “deweathered” data variations (%) for PM2.5 ranged from –67.5 to +8.6% and –69.8 to +4.7%, respectively which is within the range of values reported by Gao et al. (2021). Similar to the PM, changes in SO2 and CO levels in the RM period were uneven and city-specific. At most measuring stations, the changes of SO2 during the RM period compared to the BRM period were relatively small, up to 11%, except in BG (Figure 4D). The levels of CO (both, raw and “deweathered”) showed a significant decrease in the RM period at stations in the central and southern part of the country (from –16.3% to –64.7%, and from –13.0% to –50.0%, for measured and “deweathered” data, respectively), while at stations in the north levels of CO increased (SU, SO, and NS).
Hourly and weekly profiles of pollutants in the BRM, RM, and ARM periods contribute to the understanding of the effect of traffic on air pollution (Figure 5). Hourly profiles of NO2 and SO2 showed two peaks, in the morning (7–9 h) and afternoon (16–19 h), which is expected as the levels of traffic flows are the highest during those periods. It also showed a reduction in the afternoon peaks NO2 and SO2 during the RM period indicating a link with reduced mobility. During all three periods, the hourly and weekly variations of PM2.5, PM10, and CO showed a similar trend, which is to be expected (Figures 5A–C). Measured hourly concentrations during BRM and RM periods revealed a decrease throughout the night (between 00 and 06 h), then an increase between 06 and 10 h, and a drop between 10 and 15 h. During the afternoon, concentrations increase, and the highest peak of the day was achieved between about 19 and 22 h. The late evening peak is linked to both daily changes in the thickness of the atmosphere’s boundary layer, which thins during the night, and an increase in emissions from households, such as heating (Vicente et al., 2015; Gama et al., 2018). Higher temperatures, a thicker boundary layer, and lower emissions due to heating during the warmer half of the year result in lower concentrations during the ARM. The same hourly and weekly CO to PM trends, as well as similar daily CO profiles during the BRM and RM periods, indicate that individual residential heating is the primary source of this pollutant. During the RM period, a weekly pattern of all pollutant values revealed an increase in concentrations on Fridays. It can be explained by the fact that every weekend during the RM period, a curfew was in force, therefore on Fridays, a large percentage of the population went shopping and traveled to the countryside.
Changes in Air Pollutant Concentrations During the RM Period in 2020 Vs. Average Concentration During the Same Period in Previous Years
For each city, percentage variations in pollutant levels between 2020 and average values in the corresponding period 2015–2019 were calculated to see if pollution concentrations during the RM period were different from those in previous years. Figure 6 presents variations of raw (Figure 6A) and “deweathered” data (Figure 6B).
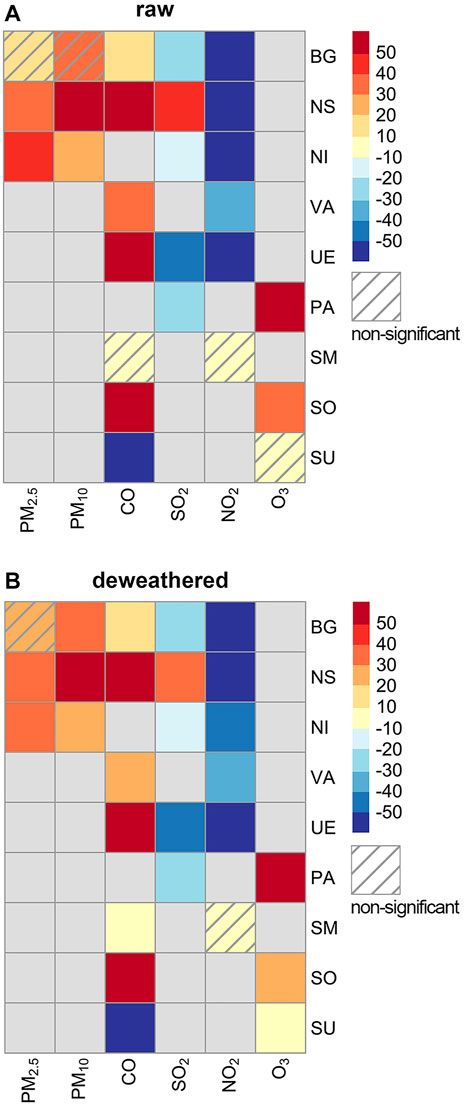
FIGURE 6. Percentage of variations in raw (A) and “deweathered” (B) pollutant levels in each city under the study between 2020 and average concentrations in the period 2015–2019.
In the majority of cities, PM, CO, and O3 levels increased, while NO2 and SO2 levels decreased in 2020 compared to previous years’ averages. Although a decrease in concentrations of PM2.5 and PM10 compared to previous years’ average can be expected, a consistent reduction cannot be seen across all cities, as is the case in Serbia. Similar to finding in Serbian cities, Briz-Redón et al. (2021) reported an increase in PM10 levels compared to the year 2019 in several Spanish cities by more than 35%, while Bar et al. (2021) reported higher PM2.5 concentrations in Springfield, Lincoln, and Bismarck in 2020 than in 2019. Higher concentrations of PM and CO in Serbian cities during the RM period in 2020 compared to the same period 2015–2020 can be attributed to an increased contribution of emissions from household heating given that the average temperature in cities during the RM period 2020 was lower between 10.5 and 16.9% than in previous years. The high levels of PM during the RM period can also be attributed to the unusually dry period in April 2020 (Republic Hydrometeorological Service of Serbia, 2021). The highest reduction relative to previous years was in NO2 concentrations, up to above 40%. Those results are similar to findings in the other studies (Baldasano, 2020; Bekbulat et al., 2021; Collivignarelli et al., 2020; Lovrić et al., 2021; etc.). For example, Munir et al. (2021) reported a decrease of 37.3–55.5% in NO2 levels in three urban areas in Northern England during the lockdown period compared to values in 2019. When compared to the same dates in 2018 and 2019, NO2 concentrations in urban areas of Poland decreased in the range of 5.3–38.7% in April and May 2020 (Filonchyk et al., 2021). These findings indicate that atmospheric NO2 is much more sensitive than PM and CO to population mobility.
Conclusion
This study focuses on the effects of the restrictive measures due to the COVID-19 pandemic on air pollutants levels at traffic measuring stations in Serbia. The influence of meteorological factors and reduced mobility on the levels of pollutants has been quantified. The analysis of pollutants concentrations was performed for the RM in comparison to BRM and ARM, as well as against the averages in preceding years.
Obtained results showed that the daily concentrations of NO2 (a primary pollutant produced directly by vehicle emissions) were significantly reduced at all the selected monitoring stations. A percentage reduction of NO2 was higher than in other countries, indicating a high impact of traffic on NO2 levels in Serbia. Unlike NO2, the relationship between particulate matters, CO and SO2 concentrations, and restrictive measures was unclear and inconsistent. The PM, CO, and SO2 showed a weak correlation with mobility during the RM period, indicating that, in addition to traffic, their concentrations are also strongly influenced by other sources. When comparing the pollution levels to previous years’ average it was discovered that PM and CO concentrations were, confirming a strong link between these pollutants and seasonal variations, and regional and cross-border transport. All together indicate that in the absence of restrictive measures the levels would have been even higher. Also, the traffic reduction during restrictive measures negatively influenced O3 levels as expected due to NO2 reduction.
This study confirmed that even a short-term reduction in traffic can significantly improve air quality, especially when it comes to NO2 concentrations. These findings are encouraging and they should encourage policymakers to limit mobility as a way to enhance air quality. Other sources such as domestic heating, industry, and transportation from other locations also contribute significantly to the increase of pollutant levels, particularly PM and CO concentrations. Therefore, further research will need to better understand the impact of individual sectors.
Data Availability Statement
Publicly available datasets were analyzed in this study. This data can be found here: (https://data.gov.rs/sr/datasets/?q = vazduh&tag = verifikovane-vrednosti (air quality data), https://covid19.apple.com/mobility, https://www.google.com/covid19/mobility/(mobility data), https://www.ncdc.noaa.gov/isd (meteorology data)).
Author Contributions
SM-M: Conceptualization, Data curation, Methodology, Software, Writing—original draft. DD: Conceptualization, Visualization, Writing—review and editing. GS: Conceptualization, Data curation, Investigation, Writing—review and editing. MM: Supervision, Writing—review and editing.
Conflict of Interest
The authors declare that the research was conducted in the absence of any commercial or financial relationships that could be construed as a potential conflict of interest.
Publisher’s Note
All claims expressed in this article are solely those of the authors and do not necessarily represent those of their affiliated organizations, or those of the publisher, the editors and the reviewers. Any product that may be evaluated in this article, or claim that may be made by its manufacturer, is not guaranteed or endorsed by the publisher.
References
Apple Inc (2020). COVID-19–Mobility Trends Reports. Available at: https://covid19.apple.com/mobility (Accessed August 15, 2021).
Baldasano, J. M. (2020). COVID-19 Lockdown Effects on Air Quality by NO2 in the Cities of Barcelona and Madrid (Spain). Sci. Total Environ. 741, 140353. doi:10.1016/j.scitotenv.2020.140353
Bar, S., Parida, B. R., Mandal, S. P., Pandey, A. C., Kumar, N., and Mishra, B. (2021). Impacts of Partial to Complete COVID-19 Lockdown on NO2 and PM2.5 Levels in Major Urban Cities of Europe and USA. Cities 117, 103308. doi:10.1016/j.cities.2021.103308
Bekbulat, B., Apte, J. S., Millet, D. B., Robinson, A. L., Wells, K. C., Presto, A. A., et al. (2021). Changes in Criteria Air Pollution Levels in the US before, during, and after Covid-19 Stay-At-Home Orders: Evidence from Regulatory Monitors. Sci. Total Environ. 769, 144693. doi:10.1016/j.scitotenv.2020.144693
Betancourt-Odio, M. A., Martínez-de-Ibarreta, C., Budría-Rodríguez, S., and Wirth, E. (2021). Local Analysis of Air Quality Changes in the Community of Madrid Before and During the COVID-19 Induced Lockdown. Atmosphere 12, 659. doi:10.3390/atmos12060659
Briz-Redón, Á., Belenguer-Sapiña, C., and Serrano-Aroca, Á. (2021). Changes in Air Pollution during COVID-19 Lockdown in Spain: A Multi-City Study. J. Environ. Sci. 101, 16–26. doi:10.1016/j.jes.2020.07.029
Carslaw, D. C. (2020). Deweather: An R Package to Remove Meteorological Variation from Air Quality Data. New York, NY, USA: University of York. Available at: https://github.com/davidcarslaw/deweather (Accessed June 1, 2021).Version 0.6
Carslaw, D. C., and Ropkins, K. (2012). Openair - an R Package for Air Quality Data Analysis. Environ. Model. Softw. 27-28, 52–61. doi:10.1016/j.envsoft.2011.09.008
Carslaw, D. C., and Taylor, P. J. (2009). Analysis of Air Pollution Data at a Mixed Source Location Using Boosted Regression Trees. Atmos. Environ. 43, 3563–3570. doi:10.1016/j.atmosenv.2009.04.001
CEN (2002). Air Quality – Approach to Uncertainty Estimation for Ambient Air Reference Measurement Methods. CR, 14377.
Chen, G., Tao, J., Wang, J., Dong, M., Li, X., Sun, X., et al. (2021). Reduction of Air Pollutants and Associated Mortality During and After the COVID-19 Lockdown in China: Impacts and Implications. Environ. Res. 200, 111457. doi:10.1016/j.envres.2021.111457
Collivignarelli, M. C., Abbà, A., Bertanza, G., Pedrazzani, R., Ricciardi, P., and Carnevale Miino, M. (2020). Lockdown for CoViD-2019 in Milan: What Are the Effects on Air Quality? Sci. Total Environ. 732, 139280. doi:10.1016/j.scitotenv.2020.139280
Davidović, M., Dmitrašinovic, S., Jovanovic, M., Radonić, J., and Jovaševic-Stojanović, M. (2021). Diurnal, Temporal and Spatial Variations of Main Air Pollutants Before and during Emergency Lockdown in the City of Novi Sad (Serbia). Appl. Sci. 11, 1212. doi:10.3390/app11031212
Donzelli, G., Cioni, L., Cancellieri, M., Llopis Morales, A., and Morales Suárez-Varela, M. M. (2021). The Effect of the Covid-19 Lockdown on Air Quality in Three Italian Medium-Sized Cities. Atmosphere 11, 1118. doi:10.3390/atmos11101118
Drobnjaković, M., and Spalević, A. (2017). “Naselja Srbije (The Settlements in Serbia),” in Geografija Srbije (Geography of Serbia). Editor M. Radovanović (Belgrade, Serbia): Geografski institut „Jovan Cvijić” SANU), 566–613.
Dunn, R. J. H., Willett, K. M., Thorne, P. W., Woolley, E. V., Durre, I., Dai, A., et al. (2012). Hadisd: A Quality-Controlled Global Synoptic Report Database for Selected Variables at Long-Term Stations From 1973–2011. Clim. Past. 8, 1649–1679. doi:10.5194/cp-8-1649-2012
Elith, J., Leathwick, J. R., and Hastie, T. (2008). A Working Guide to Boosted Regression Trees. J. Anim. Ecol. 77 (4), 802–813. doi:10.1111/j.1365-2656.2008.01390.x
Elliot, A. J., Smith, S., Dobney, A., Thornes, J., Smith, G. E., and Vardoulakis, S. (2016). Monitoring the Effect of Air Pollution Episodes on Health Care Consultations and Ambulance Call-Outs in England during March/April 2014: A Retrospective Observational Analysis. Environ. Pollut. 214, 903–911. doi:10.1016/j.envpol.2016.04.026
Fenech, S., Aquilina, N. J., and Vella, R. (2021). COVID-19-Related Changes in NO2 and O3 Concentrations and Associated Health Effects in Malta. Front. Sustain. Cities 3, 631280. doi:10.3389/frsc.2021.631280
Filonchyk, M., Hurynovich, V., and Yan, H. (2021). Impact of Covid-19 Lockdown on Air Quality in the Poland, Eastern Europe. Environ. Res. 198, 110454. doi:10.1016/j.envres.2020.110454
Gama, C., Monteiro, A., Pio, C., Miranda, A. I., Baldasano, J. M., and Tchepel, O. (2018). Temporal Patterns and Trends of Particulate Matter over Portugal: a Long-Term Analysis of Background Concentrations. Air. Qual. Atmos. Health. 11, 397–407. doi:10.1007/s11869-018-0546-8
Gao, C., Li, S., Liu, M., Zhang, F., Achal, V., Tu, Y., et al. (2021). Impact of the COVID-19 Pandemic on Air Pollution in Chinese Megacities from the Perspective of Traffic Volume and Meteorological Factors. Sci. Total Environ. 773, 145545. doi:10.1016/j.scitotenv.2021.145545
Google (2021). Google Community Mobility Reports. Available at: https://www.google.com/covid19/mobility/(Accessed August 15, 2021).
Grivas, G., Athanasopoulou, E., Kakouri, A., Bailey, J., Liakakou, E., Stavroulas, I., et al. (2020). Integrating In Situ Measurements and City Scale Modelling to Assess the COVID-19 Lockdown Effects on Emissions and Air Quality in Athens, Greece. Atmosphere 11, 1174. doi:10.3390/atmos11111174
Hernández-Paniagua, I. Y., Valdez, S. I., Almanza, V., Rivera-Cárdenas, C., Grutter, M., Stremme, W., et al. (2021). Impact of the COVID-19 Lockdown on Air Quality and Resulting Public Health Benefits in the Mexico City Metropolitan Area. Front. Public. Health 9, 642630. doi:10.3389/fpubh.2021.642630
Institute for standardization of Serbia (2021). Draft Serbian Standards on Public Enquiry. Available at: https://195.178.42.116/en/project/advanced-search (Accessed October 22th, 2021).
Lenzen, M., Li, M., Malik, A., Pomponi, F., Sun, Y.-Y., Wiedmann, T., et al. (2020). Global Socio-Economic Losses and Environmental Gains from the Coronavirus Pandemic. Plos One 15 (7), e0235654. doi:10.1371/journal.pone.0235654
Likhvar, V. N., Pascal, M., Markakis, K., Colette, A., Hauglustaine, D., Valari, M., et al. (2015). A Multi-Scale Health Impact Assessment of Air Pollution over the 21st Century. Sci. Total Environ. 514, 439–449. doi:10.1016/j.scitotenv.2015.02.002
Lovrić, M., Pavlović, K., Vuković, M., Grange, S. K., Haberl, M., and Kern, R. (2021). Understanding the True Effects of the COVID-19 Lockdown on Air Pollution by Means of Machine Learning. Environ. Pollut. 274, 115900. doi:10.1016/j.envpol.2020.115900
Menut, L., Bessagnet, B., Siour, G., Mailler, S., Pennel, R., and Cholakian, A. (2020). Impact of Lockdown Measures to Combat Covid-19 on Air Quality over Western Europe. Sci. Total Environ. 741, 140426. doi:10.1016/j.scitotenv.2020.140426
Munir, S., Coskuner, G., Jassim, M. S., Aina, Y. A., Ali, A., and Mayfield, M. (2021). Changes in Air Quality Associated with Mobility Trends and Meteorological Conditions during COVID-19 Lockdown in Northern England, UK. Atmosphere 12, 504. doi:10.3390/atmos12040504
NOAA (National Oceanic and Atmospheric Administration) (1901—present). Integrated Surface Database. Available at: https://www.ncdc.noaa.gov/isd (Accessed August 18, 2021).
Prats, R. M., van Drooge, B. L., Fernández, P., Marco, E., and Grimalt, J. O. (2021). Changes in Urban Gas-Phase Persistent Organic Pollutants During the COVID-19 Lockdown in Barcelona. Front. Environ. Sci. 9, 650539. doi:10.3389/fenvs.2021.650539
R Core Team (2013). R: A Language and Environment for Statistical Computing. Vienna, Austria: R Foundation for Statistical Computing. Available at: http://www.R-project.org/(Accessed June 1, 2021).
Regulation on monitoring conditions and air quality requirements (2013). This Regulation Enters into Force Eight Days after its Publication in the Official Gazette. Available at: http://www.pravno-informacioni-sistem.rs.
Republic Hydrometeorological Service of Serbia (2021). Annual Bulletin for Serbia the Year of 2020. Available at: http://www.hidmet.gov.rs/data/klimatologija/eng/2020.pdf (Accessed August 18, 2021).
Ridgeway, R. with contributions from others (2017). Gbm: Generalized Boosted Regression Models. R Package. Version 2.1.3. Available at: https://CRAN.R-project.org/package=gbm.
Ropkins, K., and Tate, J. E. (2021). Early Observations on the Impact of the COVID-19 Lockdown on Air Quality Trends across the UK. Sci. Total Environ. 754, 142374. doi:10.1016/j.scitotenv.2020.142374
RStudio Team (2015). RStudio. Boston, MA: Integrated Development Environment for R. Available at: http://www.rstudio.com/(Accessed June 1, 2021).
Serbian Environmental Protection Agency (SEPA) (2021). Combined Review of Automatic Air Quality Monitoring in the Republic of Serbia. Available at: http://www.amskv.sepa.gov.rs/(Accessed May 26, 2021).
Serbian Environmental Protection Agency (SEPA), Ministry of Environmental Protection, Republic of Serbia (2012). Environment Report in the Republic of Serbia for the 2011 Year (In Serbian). Available at: http://www.sepa.gov.rs/download/Izvestaj2011.pdf (Accessed August 15, 2021).
Serbian Environmental Protection Agency (SEPA), Ministry of Environmental Protection, Republic of Serbia (2013). Environment Report in the Republic of Serbia for the 2012 Year (In Serbian). Available at: http://www.sepa.gov.rs/download/Izvestaj_2012.pdf (Accessed August 15, 2021).
Setton, E., Marshall, J. D., Brauer, M., Lundquist, K. R., Hystad, P., Keller, P., et al. (2011). The Impact of Daily Mobility on Exposure to Traffic-Related Air Pollution and Health Effect Estimates. J. Expo. Sci. Environ. Epidemiol. 21, 42–48. doi:10.1038/jes.2010.14
Shi, Z., Song, C., Liu, B., Lu, G., Xu, J., Van Vu, T., et al. (2021). Abrupt but Smaller Than Expected Changes in Surface Air Quality Attributable to COVID-19 Lockdowns. Sci. Adv. 7, eabd6696. doi:10.1126/sciadv.abd6696
Song, Y., Lin, C., Li, Y., Lau, A. K. H., Fung, J. C. H., Lu, X., et al. (2021). An Improved Decomposition Method to Differentiate Meteorological and Anthropogenic Effects on Air Pollution: A National Study in China during the COVID-19 Lockdown Period. Atmos. Environ. 250, 118270. doi:10.1016/j.atmosenv.2021.118270
Stanojevic, G., Miljanovic, D., Doljak, D., Curcic, N., Radovanovic, M., Malinovic-Milicevic, S., et al. (2019). Spatio-Temporal Variability of Annual PM2.5 Concentrations and Population Exposure Assessment in Serbia for the Period 2001-2016. J. Geogr. Inst. Cvijic 69 (3), 197–211. doi:10.2298/IJGI1903197S
Statistical Office of the Republic of Serbia (2020). Municipalities and Regions of the Republic of Serbia, 2020. Available at: https://publikacije.stat.gov.rs/G2020/PdfE/G202013047.pdf (Accessed July 30, 2021).
Statistical Office of the Republic of Serbia (2021). Statistical Release. Available at: https://publikacije.stat.gov.rs/G2021/PdfE/G20211181.pdf (Accessed July 30, 2021).
Sujaritpong, S., Dear, K., Cope, M., Walsh, S., and Kjellstrom, T. (2014). Quantifying the Health Impacts of Air Pollution under a Changing Climate-A Review of Approaches and Methodology. Int. J. Biometeorol. 58, 149–160. doi:10.1007/s00484-012-0625-8
Velten, E. K., Brauer, C., and Thie, J. E. (2020). Used Vehicle Trade and Fleet Composition in EuropeFinal Report of the Project “Used Vehicle Trade and Fleet Composition in Europe” on Behalf of the EEA. Berlin, Karlsruhe: Ecologic Institute and Fraunhofer Institute for Systems and Innovation Research ISI.
Venter, Z. S., Aunan, K., Chowdhury, S., and Lelieveld, J. (2020). COVID-19 Lockdowns Cause Global Air Pollution Declines. Proc. Natl. Acad. Sci. U.S.A. 117, 18984–18990. doi:10.1073/pnas.2006853117
Vicente, E. D., Duarte, M. A., Calvo, A. I., Nunes, T. F., Tarelho, L., and Alves, C. A. (2015). Emission of Carbon Monoxide, Total Hydrocarbons and Particulate Matter during Wood Combustion in a Stove Operating under Distinct Conditions. Fuel Process. Technol. 131, 182–192. doi:10.1016/j.fuproc.2014.11.021
Weber, S. A., Insaf, T. Z., Hall, E. S., Talbot, T. O., and Huff, A. K. (2016). Assessing the Impact of Fine Particulate Matter (PM 2.5 ) on Respiratory-Cardiovascular Chronic Diseases in the New York City Metropolitan Area Using Hierarchical Bayesian Model Estimates. Environ. Res. 151, 399–409. doi:10.1016/j.envres.2016.07.012
WHO (World Health Organization) (2018). WHO Ambient (Outdoor) Air Quality Database, Summary Results, Update 2018. Available at: https://www.who.int/airpollution/data/AAP_database_summary_results_2018_final2.pdf (Accessed June 1, 2021).
WHO (World Health Organization) Regional Office for Europe (2019). Health Impact of Ambient Air Pollution in Serbia, A Call to Action. Available at: https://serbia.un.org/en/22141-health-impact-ambient-air-pollution-serbia-call-action (Accessed June 1, 2021).
WHO (World Health Organization) Regional Office for Europe (2021). Review of Evidence on Health Aspects of Air Pollution: REVIHAAP Project: Technical Report. Copenhagen: World Health Organization. Available at: https://apps.who.int/iris/handle/10665/341712 (Accessed June 1, 2021).
Keywords: air pollution, traffic, COVID-19 restrictions, meteorology, mobility, Serbia
Citation: Malinović-Milićević S, Doljak D, Stanojević G and Radovanović MM (2022) Impact of the COVID-19 Restrictive Measures on Urban Traffic-Related Air Pollution in Serbia. Front. Environ. Sci. 10:823973. doi: 10.3389/fenvs.2022.823973
Received: 28 November 2021; Accepted: 20 April 2022;
Published: 04 May 2022.
Edited by:
Suvarna Sanjeev Fadnavis, Indian Institute of Tropical Meteorology (IITM), IndiaReviewed by:
Vijay Bhaskar Bojan, Madurai Kamaraj University, IndiaAna Alebić-Juretić, University of Rijeka, Croatia
Predrag Ilić, PSRI Institute for Protection and Ecology of the Republic of Srpska, Bosnia and Herzegovina
Copyright © 2022 Malinović-Milićević, Doljak, Stanojević and Radovanović. This is an open-access article distributed under the terms of the Creative Commons Attribution License (CC BY). The use, distribution or reproduction in other forums is permitted, provided the original author(s) and the copyright owner(s) are credited and that the original publication in this journal is cited, in accordance with accepted academic practice. No use, distribution or reproduction is permitted which does not comply with these terms.
*Correspondence: Slavica Malinović-Milićević, cy5tYWxpbm92aWMtbWlsaWNldmljQGdpLnNhbnUuYWMucnM=