- 1Department of Extension Education, Bihar Agricultural University, Bhagalpur, India
- 2Department of Agricultural Extension, Bidhan Chandra Krishi Viswavidyalaya, Nadia, India
- 3Department of Computer Science and Engineering, National Institute of Technology, Patna, India
- 4Department of Extension and Social Sciences, College of Fisheries, Central Agricultural University (Imphal), Agartala, India
- 5Director Extension Education, Bihar Agricultural University, Bhagalpur, India
The highly heterogeneous and complex farm holdings operated by the smallholders in developing countries are often deprived of optimum production and profitability. The farming systems in the state of Jharkhand, India, are heterogeneous due to biophysical (e.g., climatic conditions, fertilizer status, elevation, etc.) and socio-economic (investment potential, production goals, income preferences) factors. The extension interventions to reach the smallholders often face the one-size-fits-all approach making farming less attractive with diminished potential. There is a need to understand the diversity of the farms to classify them into different homogenous groups after studying the nature and characteristics of the farm and operators on the farms. In the current study, twenty-one different variables related to socio-economic,biophysical and geospatial features of the farms from 394 farm households were used for the analysis using Principal Component Analysis to identify six principal components explaining 73.07% of the total variability in the dataset. The first six factors were further analyzed using Euclidean Distance as distance measure and Ward’s technique as agglomerative clustering to form four clusters that were found to represent the farm households in the three villages. The four farm types identified were, Type 1. Large farm household with a diversification of crops and intensification of labour (22%), Type 2. Small farm households with major income from livestock (9%), Type 3. Small farm households with diversified cropping system and income from other sources (17%), and Type 4. Small farm households with monocropping dominated by senior farmers with an additional source of income (51%). The validation of the clusters was undertaken through qualitative methods such as focused group discussions and participatory workshops. The findings back up previous research that showed a positive association between farmer categorization and mathematical classification. The study offers a verifiable scientific methodology that could help scale agricultural technologies by forming a specific cluster of farmers based on their characteristics. The technologies applied to various farm types would be helpful to the extension system to target the interventions among the precise members of the identified farm types. Thus, the study suggests the farming system typology based on socio-economic, biophysical and geospatial factors for targeted farming systems interventions among smallholders.
1 Introduction
A geographically and demographically diverse country like India, dominated by smallholder farms (Priyadarshini and Abhilash, 2020), requires continued research towards understanding farmers’ needs, capabilities, available resources, and risk appetite (Robert et al., 2017; Antony et al., 2020). Targeting extension interventions for such heterogeneous farming systems is challenging in low-and medium-income countries due to inadequate data and classification mechanisms (Daskalopoulou and Petrou, 2002; Chatterjee et al., 2015). The development of farm typologies could be an essential step in capturing the diversity associated with the farms (Priegnitz et al., 2019; Bisht et al., 2020), along with providing due consideration to the adoption of new technologies by the farming community (Bozeman, 2000; Amadu et al., 2020). Such typologies can also be helpful in the development of robust policy initiatives for the developing countries and provide better advisory support for the farmers (Ruben and Pender, 2004).
The rapid adoption of newly developed agricultural technologies would result in better returns on investment resulting in sustainable livelihoods for the rural populace. However, many farm technologies are rejected mainly by heterogeneous smallholder farmers in developing countries since there is a need for specific technological solutions suiting their context (Anugwa et al., 2021; Sinha et al., 2021). For example, the smallholder farmers in Jharkhand, India (the study locale of the current research) are primarily dependent on double cropping of rice and wheat or intercropping of vegetables with mango orchards (Gupta, 2020; Sharma et al., 2020), which has led to the increase in risk associated with farming and threatening the farms’ sustainability over the years (Hammond et al., 2020; Kilwinger et al., 2021). The extreme dependence on income from crops and little thrust on value proposition is also responsible for the youth to look for opportunities other than agriculture (Brooks, 2018; Som et al., 2018). The efficiency of agricultural research and extension system necessitates the adoption of new technologies by the farming community (Bozeman, 2000) without considering factors such as agroecology, resource endowment, and source of income (Ehsan Elahi et al., 2021; Elahi et al., 2021). The identification and characterization of farming systems simplify the diverse farm types, helping target extension intervention and informed advisory and policy support (Köbrich et al., 2003; Sarker et al., 2021).
Previous studies on farm typologies have taken factors such as cash availability and labour requirement (Tittonell et al., 2007), gender-based decisions (Tavenner et al., 2019), ecological diversity (Kansiime et al., 2021), and management practices (Zhang et al., 2021) into consideration for capturing the diversity associated with the farming systems. The farm typologies have been extensively used to study rice-based cropping systems (Siddique et al., 2022), greenhouse gas emissions (Musafiri et al., 2020), climate-smart agricultural practices (Mujeyi et al., 2021) and cattle production systems (Zoma-Traoré et al., 2020). These typologies are sometimes representative of the crop understudy like rubber-based farming (Sankalpa et al., 2021), rice-wheat cropping system (Toorop et al., 2020), and addressing gaps in maize productivity (Villarreal et al., 2020). However, a majority of the researches on farm typologies related to smallholders have focused on socio-economic and agroecological factors (Kmoch et al., 2018), land-elevation (Rahman, 2018), physical and biological environment (Escobar et al., 2019) for the classification of farms. The gap in the existing researches was bridged by the current study in making use of both biophysical (e.g., climatic conditions, fertilizer status, elevation, etc.) and socio-economic (investment potential, production goals, and income preferences) factors for identifying the farming typology of tribal smallholders in the Jharkhand state of India. Also, the spatial analysis using geographic information systems (GIS) to assess the impact of increased construction and farm buildings was explored over a period of 5 years to identify relevant variables for the study. Finally, different variables from the above factors that outline farm typologies were decided based on the research objectives. The advantage of the integration of GIS into the typology research is to take advantage of the strengths of all partners in variable identification, such as quantitative identification and characterization methods based on expert knowledge (Righi et al., 2011) and geospatial and temporal data based on GIS data of the identified land parcel.
The research addressed two central research questions. First, does the development of farm typologies help in the apt classification of farming systems in developing countries? Second, can participatory techniques account for the successful validation of the farm typologies created through statistical analysis? The two objectives of the study were to identify the farm typologies using the current GIS-oriented methodology to determine the farm typologies of the tribal farm households. The second objective is to study the integration of participatory approaches to validate farm typologies for the efficient targeting of extension interventions. This study attempts to capture the diversity and complexity associated with the smallholder tribal farmers mainly operating on subsistence farming. To do this, we use different robust variables obtained through biophysical, socioeconomic, and GIS domains (Anyimah et al., 2021) that could help capture the diversity associated with the farms in a more precise manner. As a result, we may design and build a “recommendation domain” for each set of reasonably homogenous agricultural systems obtained from our study.
Further, the unique constraints faced by each of the identified farm types are matched with the inventory of prospective technologies for sustainable intensification of smallholder farming systems using participatory methods. This would result in sound policy interventions and advisory mechanisms for enhancing the adoption of agricultural technologies suited to the region. In this way, the typologies could also be used to formulate policy initiatives for similar less-privileged areas. The study is novel in terms of developing farm typologies for the tribal regions operating on subsistence agriculture and evaluation of the developed typologies by the group of farmers themselves. Thus, this study adds to the body of knowledge on operationalization of farm household typologies for targeting extension interventions among smallholder farmers.
The paper is divided into three major sections. The first provides information on the locale of the study, the variables selected for the research and the use of different multivariate statistics. The second section examines the construction of different typologies for the classification and validation tools. The third section discusses the concludes the study along with providing limitations of the study and recommendations for future research.
2 Materials and Methods
2.1 Study Area
The agro-climatic zone of Jharkhand comprises three zones (Zone-IV, V, and VI). Three different villages from three different districts in Zone IV (Central and northeastern plateau) were purposively selected for the study based on total enumerative sampling. All the farming households from the selected villages were chosen for the study. The selected villages were Borma (25.2098° N, 87.3538° E) in Godda district, Asanbani (24.3215° N, 87.1809° E) in Dumka district, and Raksi (24.7720° N, 87.6717° E) in Sahibganj district of Jharkhand (Figure 1).
The villages under consideration were primarily rural, with an average distance from the nearest town/city of roughly 40 km. Almost 94 percent of the village’s households relied on agriculture and related occupations for survival. During the pre-monsoon, the groundwater table in the villages ranges from 5 to 7.5 m, while during the monsoon, it ranges from 12 to 18 m. In all of the settlements, the tube well is the major source of irrigation.
One village from each of the districts was randomly selected as a representative farming system of the locale. The farming practice prevalent in the villages were a mix of subsistence farming and family farming. All the farming households were taken under consideration from each village, resulting in a sample of 394 farm households. In order to offer extensive statistical coverage over geography and time, a thorough enumeration-based survey with the established interview schedule was undertaken in the three villages. The data collection was completed from January to June 2019 and included all of the agricultural households in the communities that were identified. Based on the questionnaire, 26 surveyed bio-physical and socioeconomic variables from the three villages were subjected to careful examination to determine the missing data and identify possible outliers. Further, boxplots were used to detect outliers that were eliminated later to improve the multivariate analysis. The data refinement so performed was consistent with (Kuivanen et al., 2016a; Hammond et al., 2020).
Finally, 21 surveyed bio-physical and socioeconomic variables were selected from the three villages that were found suitable for further analysis, development of typologies, and distinct clusters as mentioned in Table 1. The survey was performed following their relevant guidelines and regulations approved by the advisory committee for doctoral study at Bidhan Chandra Krishi Viswavidyalaya, India. Strict adherence was made to the Code of Ethics of the International Sociological Association (ISA) (Head, 2009) to formulate and execute the schedule.
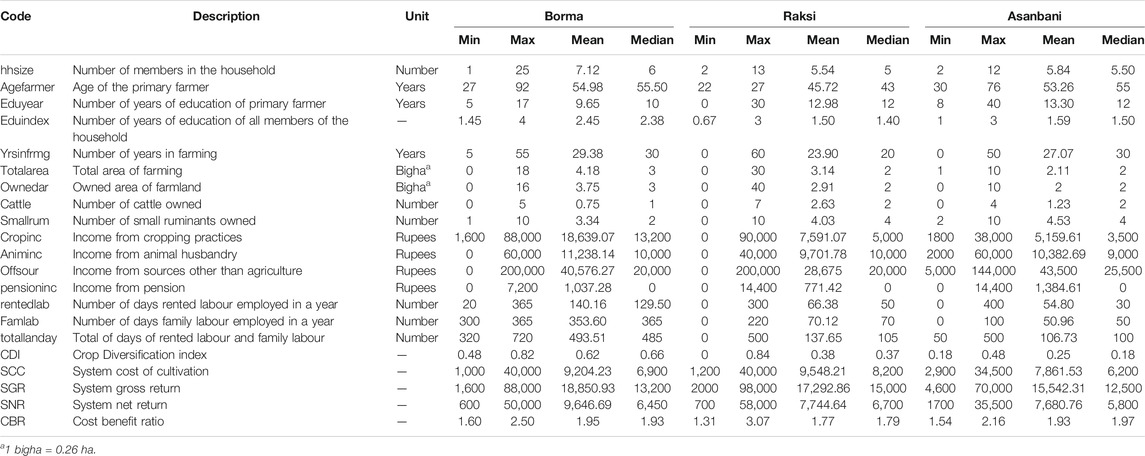
TABLE 1. Summary statistics of surveyed variables from the three villages for characterization of farm household.
2.2 Typology Construction
2.2.1 Selection of Variables
The socio-personal, economic, and biophysical characteristics pertaining to the farming household as well as the operational agricultural land were meticulously recorded. Further, the multi-temporal land use/land cover (LULC) mapping and change detection analysis was carried out for the study villages to categorize five distinct classes which are fallow land, agriculture, forest, buildup area and waterbody for a period of 5 years from the year 2010 to the year 2015 with the help of GIS data obtained from Bhuvan Geoportal of ISRO (2019) and Landsat platforms Geological Survey (U.S.) and EROS Data Center (2019)). Previously, the use of remote sensing data acquired at high spatial and temporal resolution for the classification of rice cropping systems and develop a farm typology to classify the main crop practices at the territory level was performed with high levels of accuracy (Courault et al., 2020).
To overcome the effects of multicollinearity, the 26 variables were subjected to correlation analysis. Normally, we use VIF (Variance inflation factor) to handle multicollinearity in any dataset. Multicollinearity occurs when two or more independent variables are highly correlated with one another. Hence, we use VIF to find those variables and remove them, which is also a part of data-preprocessing. Therefore, in data preprocessing, we checked for multicollinearity between independent 26 variables using VIF and manually removed the correlated variables one by one. A VIF value of 1 means no correlation between the independent variable and other variables. The VIF value exceeding 5 or 10 indicates high multicollinearity between this independent variable and the others. In the current study, we kept a VIF threshold of 5, and excluded all variables which have VIF value above 5. The significantly correlated variables were identified, and those explaining greater diversity were selected. The data was further scrutinized for the identification of missing values and outliers. Calculated boxplots were used as a part of data pre-processing as it helps to visualize the distribution of quantitative values in a field and detect outliers. Finally, 21 variables were retained for further analysis based on such analysis (Table 2).
2.2.2 Multivariate Analysis
The principal component analysis (PCA) and cluster analysis are two multivariate statistical approaches used to identify typologies (CA) in our research. The analysis was performed in SPSS Ver. 23.0 (Corp, 2016) and the visualization was executed in R, version 3.1.0, ade4 package (Dray and Dufour, 2007).
2.2.3 Principal Component Analysis
PCA is a dimensionality reduction technique that helps in increasing the interpretability of the variables and minimizes information loss. It condenses the information from the interdependent variables and converts it into a small set of factors (Jolliffe and Cadima, 2016). For the PCA analysis, a total of 21 distinct variables relating to the farms’ socioeconomic and biophysical characteristics were considered. In addition to family labour, the study looked at revenue from other sources (off-source income). The increasing cost of labour and less availability during the peak season of the crop is found to be a matter of concern in the study villages. As such, the farmers are utilizing more family labour and getting engaged in family farming practices. Also, the diversification of income to sources other than agriculture was also found to be an essential factor for classification (Kessler et al., 1998; Fernandez-Cornejo et al., 2005; Paul and Nehring, 2005).
2.2.4 Cluster Analysis
Using Euclidean Distance as a distance measure and Ward’s approach as agglomerative clustering, the PCA result in the form of a smaller dataset was employed for further analysis (Gebauer, 1987; Hair et al., 1987; Gorton et al., 2008; Sutherland et al., 2011). The schedule developed from this analysis is called agglomeration schedule, which illustrates the analysis sequence and the coefficients. The schedule is instrumental to the identification of an appropriate number of clusters that fits the dataset suitably. A closer observation of the agglomeration schedule and the Scree diagram suggests the appropriate number of clusters.
2.2.5 Validation of Farm Types
The validation of the clusters developed from the typology-based study and fitting of the agricultural technologies with the clusters was undertaken through qualitative methods. The assessment was necessary to find the best fit for the extension and farmers’ technology developed from our mathematical model based on the farmers’ socioeconomic and biophysical parameters, with the identified typologies by the practicing farmers themselves. The detailed methodology is summarized in a flowchart (Figure 2).
3 Results and Discussion
3.1 Characterization of Farming System
Principal Component Analysis: The first six main components with an eigenvalue larger than one account for 73.07 per cent of the dataset’s overall variability. The six components were used for further analysis. Further, the scree plot (plot of the eigenvalues of factors or principal components in an analysis) is used to determine the number of factors to retain in exploratory factor analysis. A scree plot (Figure 2A) displays the eigenvalues in a downward curve, ordering the eigen values from largest to smallest. The “elbow” of the graph where the eigenvalues seem to level off, which is reached after the sixth factor and the factors or components to the left of this point is retained as significant.
Further, we define each component by observing the strongly associated variables in each of the components. The first component, which is connected with crop revenue, crop diversification index, system cost of cultivation, gross system returns, and system net return, explains 30.21 percent of the variation in the dataset. As a result, the component indicates crop income and high-resource farmers who generate higher money. The second component accounts for 15.92 percent of the variation and is linked to the educational index, total hired and family labour on the farm, livestock revenue, and crop diversification. This component explains the revenue from cattle, the intensification of farm labour, and educational achievement. The third primary component accounts for 9.74 percent of the variation and is linked to the farmer’s age, the number of years committed to farming, and government pension income. The fourth component accounts for 6.29 percent of the variation and is linked to the farmer’s overall land holdings and other sources of income (off-source income). The fifth factor accounts for 5.55 percent of the variation and is linked to the size of the household and the number of years the farmer has been educated. The last factor accounts for 5.33 percent of the variation and is linked to revenue from animals and small ruminants.
“Income from crop and net income from the system” (PC1), “crop diversification and intensification of labour” (PC2), “experience in farming and income from pension” (PC3), “off-source income and total operational farm area” (PC4), “education and household size” (PC5), and “income from livestock” (PC6) could be the names for the six main components (PC6). It is also worth noting that the first two components explain 46.13% variance in the dataset, which is higher than the variance (26.91%) explained by the other four components combined. The scree plot and the correlation plot are illustrated in Figures 3A,B.
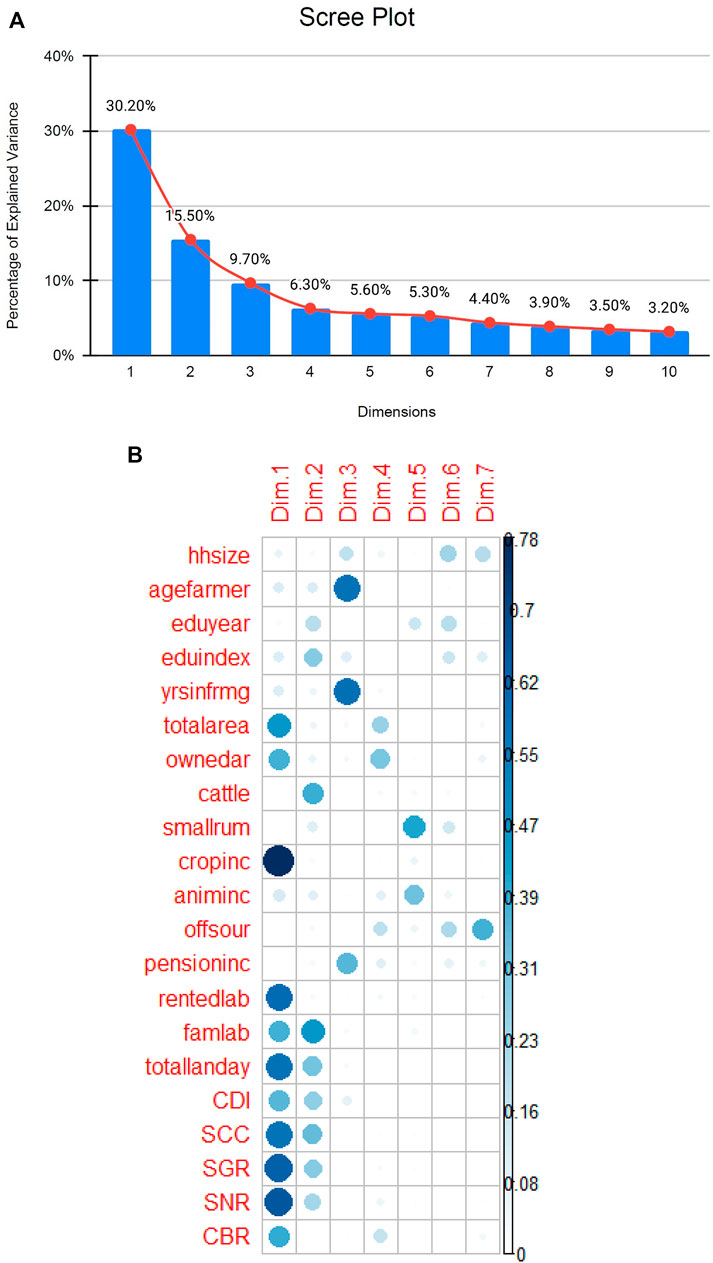
FIGURE 3. Output of PCA result. (A) Scree plot showing the cumulative variance explained by each principal component in the study. (B) The correlation plot of the variables and components
3.2 Cluster Analysis
The schedule developed from this analysis is called the agglomeration schedule, which illustrates the study sequence and the coefficients. The schedule is instrumental to the identification of an appropriate number of clusters that fits the dataset suitably. A closer observation of the agglomeration schedule and the Scree diagram suggests that the appropriate clusters should be four in number. It was further matched with the variables collected and classification of the three villages to provide an apt classification. The farm households in the three villages were found to be represented by the four clusters that had been constructed.
The four clusters were also used to create final clusters using the k-means clustering approach. The four clusters could also be identified from the dendrogram in Figure 4A and cluster silhouette plot in Figure 4B, which shows the separation distance between the resulting clusters, providing an effective visualization of the resulting results clusters. The distribution of farm households in different clusters is further visualized in Figure 4C.
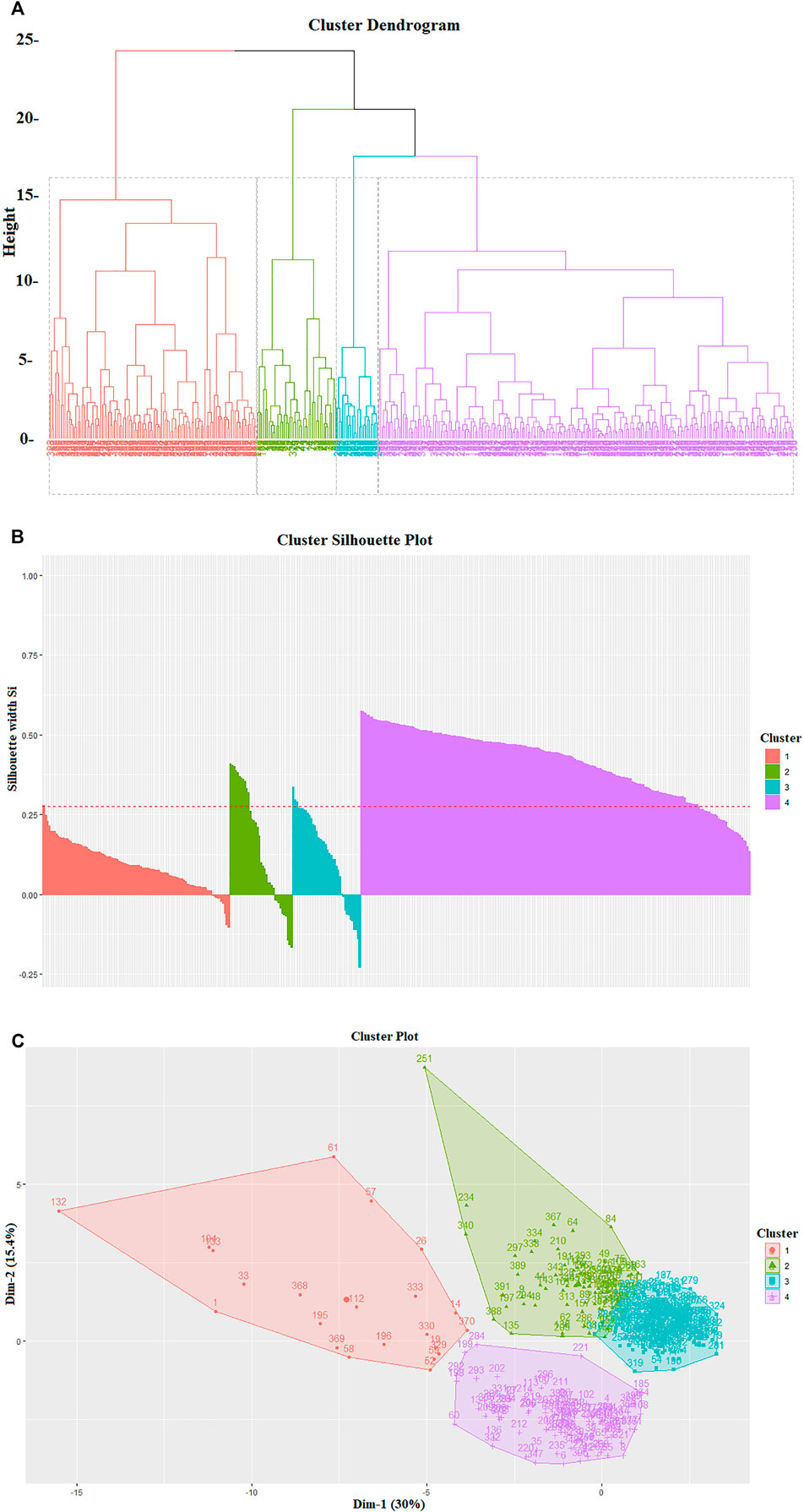
FIGURE 4. (A) Cluster Dendrogram showing the different clusters obtained through k-means clustering. (B) Cluster silhouette plot showing the separation distance between the resulting four clusters. (C) Cluster plot denoting the identified clusters and farm households.
A one-way analysis of variance for each of the major components was used to test the cluster analysis’ veracity (equality of group mean scores). The results of the one-way analysis of variance performed with the four clusters are presented in Table 3. The lower p-value denotes the distinctness of the principal component under observation. The p-value represents that all the factors, namely PC1 (Income from crop and net income from the system), PC2 (Crop diversification and intensification of labour), PC3 (Experience in farming and income from the pension), PC4 (Off source income and total operational farm area), PC5 (Education and household size), and PC6 (Income from livestock) have a p-value nearing to 0.00 (p = 0.000 for all the factors). Thus, it can be concluded that the components are significant in differentiating various clusters. The results are in congruence with the study on typology (Goswami et al., 2014). The last stage involved aggregating all data collected at field level to the farm level using open source QGIS geospatial analysis software (Flenniken et al., 2020). The most important variables obtained from remote sensing data were taken into account.
3.3 Characterization of Identified Farm Types in Different Clusters
The characteristics of the four clusters concerning the socioeconomic variables and biophysical features of the farming systems is presented in Table 4.
Type 1. Large farm household with a diversification of crops and intensification of labour (22% of sampled farms).
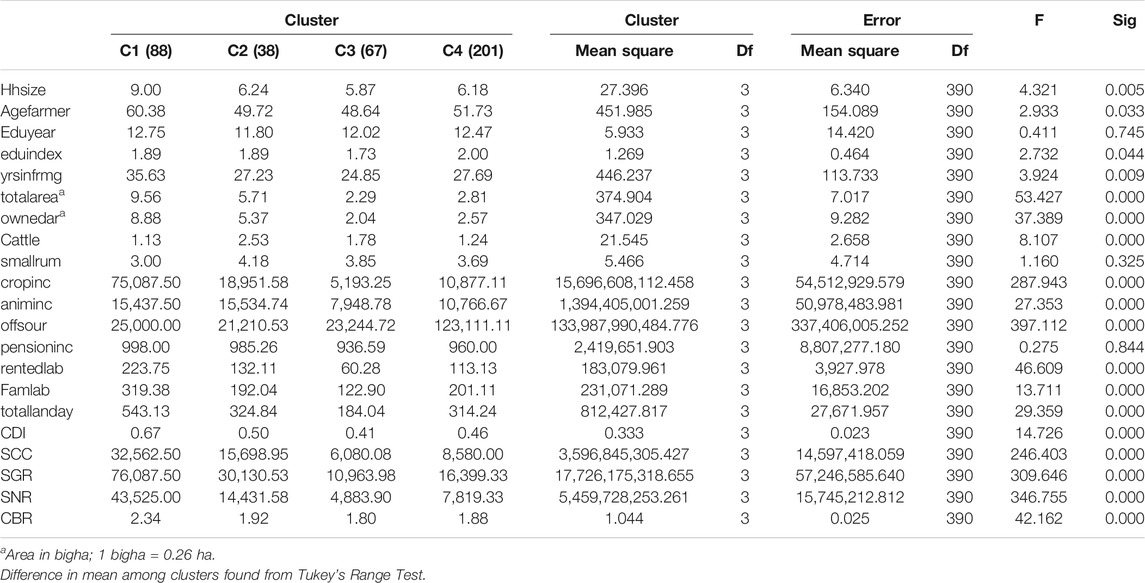
TABLE 4. Characteristics of identified clusters of farm households and p-value of one-way analysis of variance (equality of group mean) for variables used in the characterization of farm types.
The first type is represented by large households with greater age of the main farmer of the household. The income obtained from cropping practices and other sources is highest for this cluster. The use of rented labour and family labour in this cluster is found to be the highest which may be because of the presence of bigger landholding. The economic indicators indicate that this group has the largest net returns from the farming system and the maximum cost-benefit ratio.
The skills of these farmers could further be diversified in relation to poultry management through training and education. They could be provided with bank credit, taking into account their economic wellbeing and the social conditions in which they live (Guèye, 2002). With further development, poultry enterprises could deploy the family labour better with enhanced income generating opportunities. These farmers could also partner with contract farming agencies to produce wheat and maize in bulk since both commodities are widely grown in the villages but fail to fetch the minimum support price in the local market (Le Ngoc, 2018).
Type 2. Small farm households with major income from livestock (9% of sampled farms).
In the second type, the members had relatively smaller land, smaller family size, and less income generation capacity from the cropping systems (Column 2). This cluster invests the maximum among the other groups in terms of the rearing of livestock, providing additional income. These households are found to achieve lesser net returns from the cropping system and less cost–benefit ratio. Also, they are relatively young farmers who could be engaged in more remunerative aspects of farming to further increase the cost—benefit ratio. These farmers could be provided support to grow cash crops on a larger scale for meeting the demand from the market. They could further be provided farm credit, market information, and various crop management technologies to increase the yield further from small parcels of land (Mariyono, 2018). The availability of extension support in the villages is quite essential to streamline the functioning of this sector. Commercial dairy enterprises could also support them for collecting and marketing dairy products since the farm families have already integrated livestock in the farming systems.
Type 3. Small farm households with diversified cropping systems and income from other sources (17% of sampled farms).
The third type (Column 3) is featured by small landholding size, with less experienced farmers with relatively younger age among all four clusters. The rented labour and family labour use is minimum on these farms, and the net returns from the cropping practices was found minimum on these farms. The cost-benefit ratio of these farms was also the minimum among the other types. These farms mainly practice mono-cropping with little scope for diversification. The integration of the farming practices with livestock was also found to be lacking in these farm types. These farms were found to shift to other avenues for generating income than the farming practices, which is evident by the increased income source. These farms were mainly maintained for subsistence with little scope to sell the product further because of little production capacity. These farms additionally depend on social security schemes of the government to meet their food requirements. These farms could be sustained better with the integration of extension services for carrying on farming practices such as mushroom farming in their households. Mushroom farming requires little or no requirement of space. It could be successfully practiced in the house itself. The marketing of the produce could be further enhanced through groups. Such type of farming would also result in meeting the nutritional requirement of the farm families. The limited landholding size of this cluster and underemployed populations with little potential to invest could lead to the viability of this farming to boost the rural economy and provide opportunities for smallholders to diversify further to increase their profits (Easin et al., 2017).
Type 4. Small farm households with monocropping dominated by senior farmers with an additional source of income (51% of sampled farms).
The last type comprises of 51.01% of the total farm households (Column IV). This is the group with most farm households. The cluster members had smaller landholding size (owned and total land), medium family size, and moderate farm diversification. The landholding of these farmers is highly fragmented, with little scope for collective farming on a large scale. These farmers greatly depend on other sources of income generation and are observed to have higher off-source income. The cost-benefit ratio of these majority farm households is comparable to the lowest among the other clusters. These farmers may be supported with intensive technologies on small farm holdings like integrated farming systems and fisheries. Such systems would need the support of extension machinery to sustain the interest and other requirements of the smallholders. The use of an integrated approach has assisted in the uptake of new technologies in several smallholder development projects around the world (McDonald et al., 2019). Also, these farmers need enhanced marketing support to reach the market effectively with little wastages along the chain. The greater size of the family size could also be harnessed for agro-based enterprises on a small scale suitable to the interest of the villages like flour mills, vegetable processing facilities, pulp and pickle making etc.
The findings compare well with other studies on typologies conducted in Indo-Gangetic plains of India and coastal plains of Kerala in India. Kaur et al., 2021 and Innazent et al., 2022 used multidimensional analysis to describe farmer typologies in and around smallholder farmers in India. The current study even after using a different approach, compare to those of the study of the former. For example, “the small farm household with dependency on livestock” is similar to typology 2, “the resource endowed households oriented around fruits and vegetables” to typology 3, while “the resource endowed household with high levels of mechanization” can be equated with typology 4. Moreover, Goswami et al., 2014, also found similar results, which identified four predominant farm types oriented around differential income sources and resource-base. The extension agencies may be able to use the methodological approach used in the study as a decision-support tool for providing support to the farmer groups.
3.4 Validation of Farm Types
Qualitative methodologies were used to validate the four clusters produced from our typology-based study and to match agricultural technology to the clusters. The assessment was necessary to find the best fit for the extension and farmers’ technology developed from our mathematical model based on the farmers’ socioeconomic and biophysical parameters, with the identified typologies by the practicing farmers themselves. The results would also be helpful for the extension departments to generate and disseminate technologies based on the identified typologies from the villages. Incorporating a participatory research framework with typology identification might aid direct engagement with smallholders, perhaps increasing their acceptance of Decision Support Tools (DSTs). The information on different types of farmers may be used to establish a framework for discussing support systems that are more tailored to their requirements and emerging needs (Thar et al., 2021). Farmers in specific locations use their expertise to characterise and categorise their agricultural systems based on their experiences and social-cultural values in participatory approaches to farm classification (Nyambo et al., 2019). Differences in cultural attitudes on how women are tied to household chores, such as agriculture, might, for example, differ between research instances. However, statistical characterizations cannot capture these occurrences, and their explanations are often inadequate (Kuivanen et al., 2016b). As a result, it is widely accepted that scientific knowledge derived from statistical methods may be understood using local knowledge of farmers of the region (Kapalanga, 2008).
The qualitative methods aim to provide an in-depth and interpreted understanding of the current situation of the people concerning social and material circumstances along with due consideration to their experiences and varied perspectives (Ritchie et al., 2013). This approach was found suitable for this study to understand the farmers’ assessment of the typologies in their context, which would be beneficial to promote the necessary farming technologies suited to their situations based on the socioeconomic factors applicable at the household and farm level. This would help identify the critical constraints in our mathematical approach towards the development of farm typologies and provide a way to the technology adoption and dissemination process. The qualitative data would help to understand whether the farmers’ rationale in identifying farmers and technology recommendations is the same as that claimed in the study, and if that is not the case, what is the reason behind it. The qualitative data is equally helpful to identify the social and regional factors affecting different cultural situations of the farm households, which is challenging to identify by the researcher since they are an outsider to the complex farming situations underplay in the rural environments. The qualitative interviews and focus group conversations with persons on the receiving end of communications may offer in-depth understandings of how people interpret populist and understand populist messages that might provide valuable insight into the shared perspectives and opinions (Hameleers, 2018).
This is useful to the researcher and acts as valuable feedback to customize the technology basket better for the farmers. Focused group discussions (FGD) and participatory workshops were employed as qualitative tools in this study. The steps followed in the study is depicted in Figure 5.
3.5 Focused Group Discussions
The focus groups were conducted with eight key informants from each of the three villages, all of whom had at least 20 years of farming experience. It was ascertained that there is at least one key informant from each of the clusters obtained in our study. The FGDs also allowed open discussions among the participating farmers. The study also employed a local youth from the village who acted as a facilitator to clarify the questions and identify the situations in the local dialect. The group size for the FGDs was established with due consideration to the empirical data regarding adequate size (Krueger and Casey, 2014).
The key informants from each of the villages were presented with a chart from our study comprising of the clusters and their differentiating characteristics, along with separate cards with names of the farmers from the same village (Kadiyala et al., 2018). The charts were prepared in Hindi, and the basis of creation of the cluster was explained to the eight key informants from each of the villages by the local youth and the researcher. In the first step, the key informants were asked to cross-check whether the cluster created in the study and farmers of the village represented their characteristics or not? If not, they were asked to reshuffle the cards of the individual farmers to other clusters and create a distinct cluster if required.
The results of the categorization of the farmers in the cluster are represented in Table 5. It could be observed that for the village Borma, around 97% of the individuals fell into cluster 1 by the characterization performed by the key-informants, 80% of the farmers in cluster 2 were the same, approximately 91% of the farmers in cluster 3 while 100% of the farmers in cluster 4 were the same as identified by the study and the key-informants of the village. Concerning the second village Raksi, the first cluster was populated by two farmers by the key informants who did not contain any individual earlier based on our study. The second cluster has seen and addition of two farmers. The third cluster comprised around 85% same farmers, while the fourth cluster consisted of 92% same farmers as identified in our study. The third village, namely, Asanbani, consisted of the same number of farmers in the first cluster followed by 89% same farmers in cluster two. The third cluster saw two farmers’ addition while one person was shifted from cluster four to cluster three by the key informants. It is worth noting that the clusters identified in our study was found to be exhaustive by the key informants in all three villages under study. The results confirm earlier findings that reported the existence of a positive relationship between classification of farmers with that of the mathematical classification (Amare et al., 2019; Kuria et al., 2019). Furthermore, support is provided for studies reporting positive relationships between the adoption of agricultural practices and other agricultural innovations through participatory methods (Gyau et al., 2015).

TABLE 5. Number of individual farmers in each of the clusters identified through the study and cross-checked by the key-informants.
The slight mismatch in the data observed in the table is mainly due to any of the three reasons. The first major reason was attributed to the decrease in income due to the prolonged illness of any member of the family (Mwai and Muriithi, 2016)The second reason was that the main farmer was engaged in some other income source other than farming (Anderzén et al., 2020). The off-source income sources in which the farmers were found engaged were working as insurance agents, owning a local mobile/grocery shop, and engaging in land brokerage. The last reason for such deviation in categorization was because the concerned farming household was prone to alcohol addiction which reduced their capacity to invest or show interest in agriculture (Adu-Baffour et al., 2019). The findings indicate that the participation of the farmers in the focused group discussion was unaffected towards the other groups of the smallholder farms.
3.6 Participatory Workshops
Following that, after a 1-week break, participatory workshops were held with the same key informants from each village to learn how technologies were fitted to each of the identified clusters by the key informants themselves (Murgue et al., 2015). The participatory workshops aim to explore integrating local and scientific knowledge systems (D’aquino et al., 2003). The researcher visited the three villages and the local youth to collect the information that would lead to the fitting of the basket of technologies with each identified cluster.
The step followed in the participatory workshop exercise was that the key informants were shown cards of 30 different agricultural technology which were identified by the Krishi Vigyan Kendra and state extension department. The cards contained the name and brief description of the agricultural technology along with a photograph of the technology. The key informants were asked to distribute the technologies in the identified clusters for the effective targeting of the agricultural technology with the identified farmers of the clusters. The results of the exercise are depicted in Table 6.
The fitting of technology in different clusters by the key-informants is based on the characteristics of farmers in the cluster (Scoones et al., 2009; Murgue et al., 2015). The technologies deployed in the three villages demonstrate the technology’s applicability for the cluster’s farmers and might aid in targeting the intervention to a specific set of farmers. The fitted agricultural technologies were very much in alliance with the farmer’s resource and knowledge base, the options for the marketability of the produce, the skillset and cultural practices prevalent in the villages. The findings are consistent with Shukla et al., 2019 and Lopez-Ridaura et al., 2018, which proposed a typology-based targeted intervention strategy based on a systems approach for livelihood security for regions with scarcity of cultivable land. The farm household income would grow as a result of the initiatives planned for individual villages and districts, according on their resource allocation and market demands.
The findings demonstrate the importance of typology-based study in targeting extension intervention to a specific set of farmers. The study is unique in applying GIS to undertake geospatial analysis of the three villages in demarcating distinct classes that are fallow land, agriculture, forest, buildup area and waterbody for a period of 5 years from the year 2010–2015. The use of geospatial variables along with socioeconomic and biophysical variables led to the capture of heterogeneity in a far more accurate way, which was previously neglected in such typology-based studies (Ioki et al., 2019; Courault et al., 2020). In addition, the focused group discussions and participatory workshops for the validation of farm types developed from our statistical model provided a basis for the relevance of the farm typologies in the context of smallholder farmers under study.
4 Conclusion
To date, the studies on farm typologies have tended to ignore the role of subsistence smallholder farmers who are affected by several factors in addition to land and crops under cultivation. In this study, we have provided a statistical methodology for the characterization of farms in developing countries and necessary validation using variables obtained from different domains concerning agricultural practices and GIS to get environmental/geospatial data not available from other datasets. The farm typology classification offers enhanced dominance over the traditional classifications based on the size of the landholding or the characterization based on the agro-ecological characteristics of the farm. The farm types obtained by the typology classification are feasible in the current scenario and are represented by the socioeconomic parameters, bio-physical situation, resource endowment capability and management practices of the farms under study. Also, the identified clusters and underlying typology is based on robust statistical analysis in place of objective classification based on the size and nature of the farm. As such, it is much more acceptable and interpretable to the policymakers and the extension functionaries.
The results’ interpretation shows various possibilities that farmers from different clusters could adopt in their farming systems to enhance the income available from the land and increase the sustainability of the farming practices. The considerable impact on the future production systems and food availability would highly depend on the pre-existing livelihood strategies and levels of farm diversification intended by the farm households. The impact of the livestock intensification, as indicated by the positive effect on the income-generating capacity of the farmer, appeared to have a positive effect on all the identified farm types obtained in the study. Based on the different aspects of the four farm typologies observed through our dataset, we conclude that the farmers can diversify their income generation base to other alternative sources of income. For a few to several years, the typologies are prone to change. Hence such studies must be undertaken on a frequent basis to ensure that extension activities are targeted appropriately. The study shows that farm typology may aid agricultural development organisations in simplifying scaling methods and promoting targeted extension approaches to enable the proper solutions for farmers in relevant clusters. The scaling operations organisations might further classify and validate the typologies to make educated judgments on extension decision prioritising and create focused research tools and intervention support systems. The main contribution of this study is that it establishes need for geospatial analysis of the farmland along with socio-economic profiling to explain the typology of farm households based on matching of agricultural technology. In addition, extension intervention could be implemented on the district level by identifying different components affecting the farming systems including cropping, livestock and allied production interventions. The targeting of extension intervention through this typology study offers the policymakers a mix of options to reach smallholders based on their potential and interests rather than prescribing a “one-size-fits-all” strategy.
The major limitation of the study was the time and fund constraint, which allowed the researchers to zoom in on limited geographies of three villages in three different districts of Jharkhand, India. Future research in the domain should also consider intra-household interactions and dynamics to formulate innovation pathways appropriate for each level of endowment and livelihood strategies captured in the typology. The researchers should also identify farm typologies of smallholder farmers in developing countries with small landholding sizes and streamline the extension interventions based on the identified systems in active collaboration with the extension agencies and allied institutions. We conclude by reflecting on how these typologies and the heterogeneity explained by the farming systems may be effectively used by the extension agencies in designing appropriate policy interventions to support smallholders imbibing technological innovations in agriculture.
Data Availability Statement
The original contributions presented in the study are included in the article/Supplementary Material, further inquiries can be directed to the corresponding author.
Ethics Statement
The studies involving human participants were reviewed and approved by the Advisory committee for doctoral study at Bidhan Chandra Krishi Viswavidyalaya, India. The patients/participants provided their written informed consent to participate in this study. Written informed consent was obtained from the individual(s) for the publication of any potentially identifiable images or data included in this article.
Author Contributions
Conceptualization: DB and AS. Formal analysis: PP and AS. Methodology: AS, DB, and AG. Resources: DB, RKS.
Conflict of Interest
The authors declare that the research was conducted in the absence of any commercial or financial relationships that could be construed as a potential conflict of interest.
Publisher’s Note
All claims expressed in this article are solely those of the authors and do not necessarily represent those of their affiliated organizations, or those of the publisher, the editors and the reviewers. Any product that may be evaluated in this article, or claim that may be made by its manufacturer, is not guaranteed or endorsed by the publisher.
Acknowledgments
We thank Bidhan Chandra Krishi Viswavidyalaya, India for providing computer and logistic support. We would also like to thank the respondents from three tribal villages in Jharkhand, India for their overwhelming support.
References
Adelhart Toorop, R., Lopez-Ridaura, S., Bijarniya, D., Kalawantawanit, E., Jat, R. K., Prusty, A. K., et al. (2020). Farm-level Exploration of Economic and Environmental Impacts of Sustainable Intensification of rice-wheat Cropping Systems in the Eastern Indo-Gangetic plains. Eur. J. Agron. 121, 126157. doi:10.1016/j.eja.2020.126157
Adu-Baffour, F., Daum, T., and Birner, R. (2019). Can Small Farms Benefit from Big Companies' Initiatives to Promote Mechanization in Africa? A Case Study from Zambia. Food policy 84, 133–145. doi:10.1016/j.foodpol.2019.03.007
Amadu, F. O., McNamara, P. E., and Miller, D. C. (2020). Understanding the Adoption of Climate-Smart Agriculture: A Farm-Level Typology with Empirical Evidence from Southern Malawi. World Dev. 126, 104692. doi:10.1016/j.worlddev.2019.104692
Amare, D., Wondie, M., Mekuria, W., and Darr, D. (2019). Agroforestry of Smallholder Farmers in Ethiopia: Practices and Benefits. Small-scale For. 18, 39–56. doi:10.1007/s11842-018-9405-6
Anderzén, J., Guzmán Luna, A., Luna-González, D. V., Merrill, S. C., Caswell, M., Méndez, V. E., et al. (2020). Effects of On-Farm Diversification Strategies on Smallholder Coffee Farmer Food Security and Income Sufficiency in Chiapas, Mexico. J. Rural Stud. 77, 33–46. doi:10.1016/j.jrurstud.2020.04.001
Antony, A. P., Leith, K., Jolley, C., Lu, J., and Sweeney, D. J. (2020). A Review of Practice and Implementation of the Internet of Things (IoT) for Smallholder Agriculture. Sustainability 12, 3750. doi:10.3390/su12093750
Anugwa, I. Q., Agbo, P. C., Agwu, A. E., Abonyi, C. C., and Nduka, E. K. (2021). Gender Differences in Perceived Vulnerability and Adaptation Strategies to Climate Change Effects on Arable Crop Production in Enugu State, Nigeria. Clim. Pol., 1–20. doi:10.1007/978-3-030-22759-3_315-1
Anyimah, F. O., Osei Jnr, E. M., and Nyamekye, C. (2021). Detection of Stress Areas in cocoa Farms Using GIS and Remote Sensing: A Case Study of Offinso Municipal & Offinso North District, Ghana. Environ. Challenges 4, 100087. doi:10.1016/j.envc.2021.100087
Bhuvan Geoportal of ISRO (2019). Indian Space Research Organization Bhuvan Online Geoportal. Hyderabad, India: National Remote Sensing Centre (NRSC). Available at: https://bhuvan.nrsc.gov.in.
Bisht, I. S., Rana, J. C., and Pal Ahlawat, S. (2020). The Future of Smallholder Farming in India: Some Sustainability Considerations. Sustainability 12, 3751. doi:10.3390/su12093751
Bozeman, B. (2000). Technology Transfer and Public Policy: a Review of Research and Theory. Res. Pol. 29, 627–655. doi:10.1016/s0048-7333(99)00093-1
Brooks, K. (2018). Rising Absolutely, Declining Relatively: Agriculture, Climate Change, and Job Creation in Africa. Agrekon 57, 181–197. doi:10.1080/03031853.2018.1538002
Chatterjee, S., Goswami, R., and Bandopadhyay, P. (2015). Methodology of Identification and Characterization of Farming Systems in Irrigated Agriculture: Case Study in West Bengal State of India. J. Agric. Sci. Techn. 17, 1127
Courault, D., Hossard, L., Flamain, F., Baghdadi, N., and Irfan, K. (2020). Assessment of Agricultural Practices from Sentinel 1 and 2 Images Applied on rice fields to Develop a Farm Typology in the Camargue Region. IEEE J. Sel. Top. Appl. Earth Observations Remote Sensing 13, 5027–5035. doi:10.1109/JSTARS.2020.3018881
D’aquino, P., Le Page, C., Bousquet, F., and Bah, A. (2003). Using Self-Designed Role-Playing Games and a Multi-Agent System to Empower a Local Decision-Making Process for Land Use Management: The SelfCormas experiment in Senegal. J. Artif. societies Soc. simulation 6, 1–14.
Daskalopoulou, I., and Petrou, A. (2002). Utilising a Farm Typology to Identify Potential Adopters of Alternative Farming Activities in Greek Agriculture. J. Rural Stud. 18, 95–103. doi:10.1016/S0743-0167(01)00027-4
Dray, S., and Dufour, A.-B. (2007). The Ade4 Package: Implementing the Duality Diagram for Ecologists. J. Stat. Softw. 22, 1–20. doi:10.18637/jss.v022.i04
Elahi, E., Khalid, Z., Tauni, M. Z., Zhang, H., and Lirong, X. (2021b). Extreme Weather Events Risk to Crop-Production and the Adaptation of Innovative Management Strategies to Mitigate the Risk: A Retrospective Survey of Rural Punjab, Pakistan. Technovation, 102255. doi:10.1016/j.technovation.2021.102255
Elahi, E., Zhang, H., Lirong, X., Khalid, Z., and Xu, H. (2021a). Understanding Cognitive and Socio-Psychological Factors Determining Farmers' Intentions to Use Improved Grassland: Implications of Land Use Policy for Sustainable Pasture Production. Land use policy 102, 105250. doi:10.1016/j.landusepol.2020.105250
Escobar, N., Romero, N. J., and Jaramillo, C. I. (2019). Typology of Small Producers in Transition to Agroecological Production.
Geological Survey (U.S.) and EROS Data Center (2019). EarthExplorer. Reston, VA: U.S. Dept. of the Interior, U.S. Geological Survey. Available at: https://earthexplorer.usgs.gov/.
Fernandez-Cornejo, J., Hendricks, C., and Mishra, A. (2005). Technology Adoption and Off-Farm Household Income: the Case of Herbicide-Tolerant Soybeans. J. Agric. Appl. Econ. 37, 549–563. doi:10.1017/s1074070800027073
Flenniken, J. M., Stuglik, S., and Iannone, B. V. (2020). Quantum GIS (QGIS): An Introduction to a Free Alternative to More Costly GIS Platforms: FOR359/FR428, 2/2020. EDIS 7–7.
Gebauer, R. H. (1987). Socio-economic Classification of Farm Households -conceptual, Methodical and Empirical Considerations. Eur. Rev. Agric. Econ. 14, 261–283. doi:10.1093/erae/14.3.261
Gorton, M., Douarin, E., Davidova, S., and Latruffe, L. (2008). Attitudes to Agricultural Policy and Farming Futures in the Context of the 2003 CAP Reform: A Comparison of Farmers in Selected Established and New Member States. J. Rural Stud. 24, 322–336. doi:10.1016/j.jrurstud.2007.10.001
Goswami, R., Chatterjee, S., and Prasad, B. (2014). Farm Types and Their Economic Characterization in Complex Agro-Ecosystems for Informed Extension Intervention: Study from Coastal West Bengal, India. Agric. Food Econ. 2, 1–24. doi:10.1186/s40100-014-0005-2
Guèye, E. F. (2002). Employment and Income Generation through Family Poultry in Low-Income Food-Deficit Countries. World’s Poult. Sci. J. 58, 541–557.
Gupta, R. (2020). Small Scale Industries and Tribal Women of Jharkhand: A Sociological Analysis. Easjehl 3, 548–554. doi:10.36349/easjehl.2020.v03i12.001
Gyau, A., Smoot, K., Diby, L., and Kouame, C. (2015). Drivers of Tree Presence and Densities: the Case of cocoa Agroforestry Systems in the Soubre Region of Republic of Côte d'Ivoire. Agroforest Syst. 89, 149–161. doi:10.1007/s10457-014-9750-1
Hair, J. F., Anderson, R., Tatham, R., and Black, W. (1987). Multivariate Data Analysis with Readings. New Work: Macmillan.
Hameleers, M. (2018). A Typology of Populism: Toward a Revised Theoretical Framework on the Sender Side and Receiver Side of Communication. Int. J. Commun. 12, 2171
Hammond, J., Rosenblum, N., Breseman, D., Gorman, L., Manners, R., van Wijk, M. T., et al. (2020). Towards Actionable Farm Typologies: Scaling Adoption of Agricultural Inputs in Rwanda. Agric. Syst. 183, 102857. doi:10.1016/j.agsy.2020.102857
Head, E. (2009). The Ethics and Implications of Paying Participants in Qualitative Research. Int. J. Soc. Res. Methodol. 12, 335–344. doi:10.1080/13645570802246724
Innazent, A., Jacob, D., Bindhu, J. S., Joseph, B., Anith, K. N., Ravisankar, N., et al. (2022). Farm Typology of Smallholders Integrated Farming Systems in Southern Coastal Plains of Kerala, India. Sci. Rep. 12 (1), 1–14. doi:10.1038/s41598-021-04148-0
Ioki, K., Din, N. M., Ludwig, R., James, D., Hue, S. W., Johari, S. A., et al. (2019). Supporting forest Conservation through Community-Based Land Use Planning and Participatory GIS - Lessons from Crocker Range Park, Malaysian Borneo. J. Nat. Conservation 52, 125740. doi:10.1016/j.jnc.2019.125740
Jolliffe, I. T., and Cadima, J. (2016). Principal Component Analysis: a Review and Recent Developments. Phil. Trans. R. Soc. A. 374, 20150202. doi:10.1098/rsta.2015.0202
Kadiyala, S., Prost, A., Harris-Fry, H., O’Hearn, M., Pradhan, R., Pradhan, S., et al. (2018). Upscaling Participatory Action and Videos for Agriculture and Nutrition (UPAVAN) Trial Comparing Three Variants of a Nutrition-Sensitive Agricultural Extension Intervention to Improve Maternal and Child Nutritional Outcomes in Rural Odisha, India: Study Protocol for a Cluster Randomised Controlled Trial. Trials 19, 1–16. doi:10.1186/s13063-018-2521-y
Kansiime, M. K., Girling, R. D., Mugambi, I., Mulema, J., Oduor, G., Chacha, D., et al. (2021). Rural Livelihood Diversity and its Influence on the Ecological Intensification Potential of Smallholder Farms in Kenya. Food Energy Secur 10, e254. doi:10.1002/fes3.254
Kapalanga, T. S. (2008). A Review of Land Degradation Assessment Methods. Land Restoration Training Programme 2011. Available at: https://citeseerx.ist.psu.edu/viewdoc/download?doi=10.1.1.470.6256&rep=rep1&type=pdf.
Kaur, J., Prusty, A. K., Ravisankar, N., Panwar, A. S., Shamim, M., Walia, S. S., et al. (2021). Farm Typology for Planning Targeted Farming Systems Interventions for Smallholders in Indo-Gangetic Plains of India. Sci. Rep. 11 (1), 1–16. doi:10.1038/s41598-021-00372-w
Kessler, J. J., Slingerland, M. A., and Savadogo, M. (1998). Regeneration of Sylvopastoral Lands in the Sahel Zone under Village Management Conditions. Land Degrad. Dev. 9, 95–106. doi:10.1002/(sici)1099-145x(199803/04)9:2<95::aid-ldr289>3.0.co;2-y
Kilwinger, F., Mugambi, S., Manners, R., Schut, M., Tumwegamire, S., Nduwumuremyi, A., et al. (2021). Characterizing Cassava Farmer Typologies and Their Seed Sourcing Practices to Explore Opportunities for Economically Sustainable Seed Business Models in Rwanda. Outlook Agric. 50, 441–454. doi:10.1177/00307270211045408
Kmoch, L., Pagella, T., Palm, M., and Sinclair, F. (2018). Using Local Agroecological Knowledge in Climate Change Adaptation: a Study of Tree-Based Options in Northern Morocco. Sustainability 10, 3719. doi:10.3390/su10103719
Köbrich, C., Rehman, T., and Khan, M. (2003). Typification of Farming Systems for Constructing Representative Farm Models: Two Illustrations of the Application of Multi-Variate Analyses in Chile and Pakistan. Agric. Syst. 76, 141
Krueger, R. A., and Casey, M. A. (2014). Focus Groups: A Practical Guide for Applied Research. Fifth Edition. Thousand Oaks, CA: SAGE Publications, Inc.
Kuivanen, K. S., Alvarez, S., Michalscheck, M., Adjei-Nsiah, S., Descheemaeker, K., Mellon-Bedi, S., et al. (2016a). Characterising the Diversity of Smallholder Farming Systems and Their Constraints and Opportunities for Innovation: A Case Study from the Northern Region, Ghana. NJAS: Wageningen J. Life Sci. 78, 153–166. doi:10.1016/j.njas.2016.04.003
Kuivanen, K. S., Michalscheck, M., Descheemaeker, K., Adjei-Nsiah, S., Mellon-Bedi, S., Groot, J. C. J., et al. (2016b). A Comparison of Statistical and Participatory Clustering of Smallholder Farming Systems - A Case Study in Northern Ghana. J. Rural Stud. 45, 184–198. doi:10.1016/j.jrurstud.2016.03.015
Kuria, A. W., Barrios, E., Pagella, T., Muthuri, C. W., Mukuralinda, A., and Sinclair, F. L. (2019). Farmers' Knowledge of Soil Quality Indicators along a Land Degradation Gradient in Rwanda. Geoderma Reg. 16, e00199. doi:10.1016/j.geodrs.2018.e00199
Le Ngoc, H. (2018). “Contract Farming Effects on Technical Efficiency of the export-oriented rice Production Sector in Vietnam,” in 30th International Conference of Agricultural Eonomics (Vancouver: British Columbia). doi:10.22004/ag.econ.277409
Lopez-Ridaura, S., Frelat, R., van Wijk, M. T., Valbuena, D., Krupnik, T. J., and Jat, M. L. (2018). Climate Smart Agriculture, Farm Household Typologies and Food Security. Agric. Syst. 159, 57–68. doi:10.1016/j.agsy.2017.09.007
Mariyono, J. (2018). Profitability and Determinants of Smallholder Commercial Vegetable Production. Int. J. Vegetable Sci. 24, 274–288. doi:10.1080/19315260.2017.1413698
McDonald, C. K., MacLeod, N. D., Lisson, S., and Corfield, J. P. (2019). The Integrated Analysis Tool (IAT) - A Model for the Evaluation of Crop-Livestock and Socio-Economic Interventions in Smallholder Farming Systems. Agric. Syst. 176, 102659. doi:10.1016/j.agsy.2019.102659
Mujeyi, A., Mudhara, M., and Mutenje, M. J. (2021). Adoption Patterns of Climate-Smart Agriculture in Integrated Crop-Livestock Smallholder Farming Systems of Zimbabwe. Clim. Dev., 1–10. doi:10.1080/17565529.2021.1930507
Murgue, C., Therond, O., and Leenhardt, D. (2015). Toward Integrated Water and Agricultural Land Management: Participatory Design of Agricultural Landscapes. Land use policy 45, 52–63. doi:10.1016/j.landusepol.2015.01.011
Musafiri, C. M., Macharia, J. M., Ng'etich, O. K., Kiboi, M. N., Okeyo, J., Shisanya, C. A., et al. (2020). Farming Systems' Typologies Analysis to Inform Agricultural Greenhouse Gas Emissions Potential from Smallholder Rain-Fed Farms in Kenya. Scientific Afr. 8, e00458. doi:10.1016/j.sciaf.2020.e00458
Mwai, D., and Muriithi, M. (2016). Economic Effects of Non-communicable Diseases on Household Income in Kenya: a Comparative Analysis Perspective. Public Health Res. 6, 83–90. doi:10.5923/j.phr.20160603.02
Nyambo, D. G., Luhanga, E. T., and Yonah, Z. Q. (20192019). A Review of Characterization Approaches for Smallholder Farmers: Towards Predictive Farm Typologies. Scientific World J. 2019, 1–9. doi:10.1155/2019/6121467
Paul, C. J. M., and Nehring, R. (2005). Product Diversification, Production Systems, and Economic Performance in U.S. Agricultural Production. J. Econom. 126, 525–548. doi:10.1016/j.jeconom.2004.05.012
Priegnitz, U., Lommen, W. J. M., Onakuse, S., and Struik, P. C. (2019). A Farm Typology for Adoption of Innovations in Potato Production in Southwestern Uganda. Front. Sustain. Food Syst. 3, 68. doi:10.3389/fsufs.2019.00068
Priyadarshini, P., and Abhilash, P. C. (2020). Policy Recommendations for Enabling Transition towards Sustainable Agriculture in India. Land Use Policy 96, 104718. doi:10.1016/j.landusepol.2020.104718
Rahman, S. (2018). Agroecological, Land-Elevation and Socioeconomic Determinants of Raising Livestock in Bangladesh. Agriculture 8, 12. doi:10.3390/agriculture8010012
Righi, E., Dogliotti, S., Stefanini, F. M., and Pacini, G. C. (2011). Capturing Farm Diversity at Regional Level to Up-Scale Farm Level Impact Assessment of Sustainable Development Options. Agric. Ecosyst. Environ. 142, 63–74. doi:10.1016/j.agee.2010.07.011
Ritchie, J., Lewis, J., Nicholls, C. M., and Ormston, R. (2013). Qualitative Research Practice: A Guide for Social Science Students and Researchers. London, United Kingdom: sage.
Robert, M., Thomas, A., Sekhar, M., Badiger, S., Ruiz, L., Willaume, M., et al. (2017). Farm Typology in the Berambadi Watershed (India): Farming Systems Are Determined by Farm Size and Access to Groundwater. Water 9, 51. doi:10.3390/w9010051
Ruben, R., and Pender, J. (2004). Rural Diversity and Heterogeneity in Less-Favoured Areas: the Quest for Policy Targeting. Food Policy 29, 303–320. doi:10.1016/j.foodpol.2004.07.004
Sankalpa, J. K. S., Wijesuriya, W., Wimalaratana, W., and Ishani, P. G. N. (2021). Utilizing a Farm Typology for the Development of Rubber-Based Farming: a Case from Moneragala District, Sri Lanka. J. Rubber Res., 1–16. doi:10.1007/s42464-021-00127-2
Sarker, M. R., Galdos, M. V., Challinor, A. J., and Hossain, A. (2021). A Farming System Typology for the Adoption of New Technology in Bangladesh. Food Energy Secur 10. doi:10.1002/fes3.287
Scoones, I., Thompson, J., and Chambers, R. (2009). Farmer First Revisited. Rugby, United Kingdom: Practical Action Publishing Rugby.
Sharma, N. K., Kullu, N. J., and Purty, C. (2020). Crop Acreage Estimation in Jharkhand Using Multi-Temporal Satellite Data. J. Remote Sensing GIS 11, 1
Shukla, R., Agarwal, A., Gornott, C., Sachdeva, K., and Joshi, P. K. (2019). Farmer Typology to Understand Differentiated Climate Change Adaptation in Himalaya. Sci. Rep. 9, 20375–20412. doi:10.1038/s41598-019-56931-9
Siddique, M. N. E. A., Lobry de Bruyn, L. A., Osanai, Y., and Guppy, C. N. (2022). Typology of rice-based Cropping Systems for Improved Soil Carbon Management: Capturing Smallholder Farming Opportunities and Constraints in Dinajpur, Bangladesh. Geoderma Reg. 28, e00460. doi:10.1016/j.geodrs.2021.e00460
Sinha, A., Basu, D., Priyadarshi, P., and Sharma, M. (2021). Application of Geographic Information System and Multivariate Techniques for the Delineation of Farm Typologies. Natl. Acad. Sci. Lett. 45, 1–4. doi:10.1007/s40009-021-01071-w
Som, S., Burman, R. R., Sharma, J. P., Padaria, R. N., Paul, S., and Singh, A. K. (2018). Attracting and Retaining Youth in Agriculture: Challenges and Prospects. J. Community Mobilization Sustain. Dev. 13, 385
Sutherland, L.-A., Barnes, A., McCrum, G., Blackstock, K., and Toma, L. (2011). Towards a Cross-Sectoral Analysis of Land Use Decision-Making in Scotland. Landscape Urban Plann. 100, 1–10. doi:10.1016/j.landurbplan.2010.10.005
Tavenner, K., van Wijk, M., Fraval, S., Hammond, J., Baltenweck, I., Teufel, N., et al. (2019). Intensifying Inequality? Gendered Trends in Commercializing and Diversifying Smallholder Farming Systems in East Africa. Front. Sustain. Food Syst. 3, 10. doi:10.3389/fsufs.2019.00010
Thar, S. P., Ramilan, T., Farquharson, R. J., and Chen, D. (2021). Identifying Potential for Decision Support Tools through Farm Systems Typology Analysis Coupled with WParticipatory Research: A Case for Smallholder Farmers in Myanmar. Agriculture 11, 516. doi:10.3390/agriculture11060516
Tittonell, P., Vanlauwe, B., de Ridder, N., and Giller, K. E. (2007). Heterogeneity of Crop Productivity and Resource Use Efficiency within Smallholder Kenyan Farms: Soil Fertility Gradients or Management Intensity Gradients? Agric. Syst. 94, 376–390. doi:10.1016/j.agsy.2006.10.012
Villarreal, E. A. Z., Villa, T. C. C., Escoto, L. B., and Ridaura, S. L. (2020). Maize Productivity Gaps: An Explanation Based on the Heterogeneity of Mexico Central and South Farm Households. Trop. Subtropical Agroecosystems 23, 1
Zhang, J., Zhang, L., Wang, M., Brostaux, Y., Yin, C., and Dogot, T. (2021). Identifying Key Pathways in Manure and Sewage Management of Dairy Farming Based on a Quantitative Typology: A Case Study in China. Sci. Total Environ. 760, 143326. doi:10.1016/j.scitotenv.2020.143326
Keywords: farm typology, extension, tribal, smallholders, classification
Citation: Sinha A, Basu D, Priyadarshi P, Ghosh A and Sohane RK (2022) Farm Typology for Targeting Extension Interventions Among Smallholders in Tribal Villages in Jharkhand State of India. Front. Environ. Sci. 10:823338. doi: 10.3389/fenvs.2022.823338
Received: 27 November 2021; Accepted: 09 February 2022;
Published: 18 March 2022.
Edited by:
Yu-Pin Lin, National Taiwan University, TaiwanReviewed by:
Mohammad Karimi, K.N.Toosi University of Technology, IranEhsan Elahi, Shandong University of Technology, China
Copyright © 2022 Sinha, Basu, Priyadarshi, Ghosh and Sohane. This is an open-access article distributed under the terms of the Creative Commons Attribution License (CC BY). The use, distribution or reproduction in other forums is permitted, provided the original author(s) and the copyright owner(s) are credited and that the original publication in this journal is cited, in accordance with accepted academic practice. No use, distribution or reproduction is permitted which does not comply with these terms.
*Correspondence: Aditya Sinha, aW5jLmFkaXR5YUBnbWFpbC5jb20=