- 1The University of Melbourne, Parkville, VIC, Australia
- 2Goulburn Broken Catchment Management Authority, Sheparton, VIC, Australia
- 3University of Canberra, Canberra, ACT, Australia
- 4Colorado State University, Fort Collins, CO, United States
The numerous environmental flows assessment methods that exist typically assume a stationary climate. Adaptive management is commonly put forward as the preferred approach for managing uncertainty and change in environmental flows. However, we contend that a simple adaptive management loop falls short of meeting the challenges posed by climate change. Rather, a fundamental rethink is required to ensure both the structure of environmental flows assessments, along with each individual technical element, actively acknowledges the multiple dimensions of change, variability and complexity in socio-ecological systems. This paper outlines how environmental flow assessments can explicitly address the uncertainty and change inherent in adaptively managing multiple values for management of environmental flows. While non-stationarity and uncertainty are well recognised in the climate literature, these have not been addressed within the structure of environmental flows methodologies. Here, we present an environmental flow assessment that is structured to explicitly consider future change and uncertainty in climate and socio-ecological values, by examining scenarios using ecological models. The environmental flow assessment methodology further supports adaptive management through the intentional integration of participatory approaches and the inclusion of diverse stakeholders. We present a case study to demonstrate the feasibility of this approach, highlighting how this methodology facilitates adaptive management. Rethinking our approach to environmental flows assessments is an important step in ensuring that environmental flows continue to work effectively as a management tool under climate change.
1 Introduction
Environmental Flows are now well recognised as a management tool to protect and restore riverine ecosystems from the impacts of extraction and river regulation (Horne A. et al., 2017; Le Quesne et al., 2010; Arthington et al., 2019). Over the last 20 years, an array of methods has been developed to assess environmental flow requirements (Poff et al., 2017; Tharme, 2003). These methods mainly stem from the physical sciences, with hydrologic, hydraulic and habitat simulation methods underpinning many of the approaches used today (Poff et al., 2017). Holistic methods aim to create a bridge to integrate the physical and social sciences, but are still largely dominated by physical considerations.
Parallel to the development of these methods has been a push for adaptive management, and the need to establish frameworks for management that deal with complexity and uncertainty of social-ecological systems by enabling flexibility in the face of unexpected events, and learning through time (Holling, 1978; Pahl-Wostl et al., 2007; Webb et al., 2018). However, to date, there has been little discussion of how environmental flow assessment methods link into and best support an adaptive management process. This is becoming even more pertinent with changes not only in our knowledge systems and values, but also with changing climate conditions.
Adaptive management focuses on learning that informs decision making through time (Allan and Watts, 2018). Learning can occur at different levels within the decision-making process (Webb et al., 2017). At one end of the spectrum, technical learning can occur supported by modelling and monitoring data; at the other, social learning can occur through monitoring the decision context and values, supported by the partnership and inclusion of diverse stakeholders in a participatory approach (Roux and Foxcroft, 2011; Fujitani et al., 2017; Kingsford et al., 2011; Allan and Watts, 2018). This social learning can help ensure that managers have the support and latitude to adjust decisions to improve progress towards desired outcomes. Importantly, however, since its introduction in the 1970s (Holling, 1978) adaptive management has been based on the philosophy of incomplete knowledge and learning through reflection (Allen et al., 2011). This incomplete knowledge, or uncertainty, has traditionally covered structural or process uncertainty, lack of observational data, and environmental variability (Williams, 2011). However recent complex natural resource management challenges also include the (very real and) present threats to environmental outcomes associated with climate change. Other dynamic influences including invasive species, changing economic and policy environments, and greater inclusion of the roles and rights of Traditional Owners in water planning processes, which in combination, all point the way to an uncertain future. This adds another layer to the current state of incomplete knowledge, or uncertainty, where structural or process uncertainty not only includes lack of observational data and environmental variability (Williams, 2011), but high uncertainty about what the future holds. Adaptive management, coupled with scenario analysis and emphasising participatory approaches that reflect diversity in stakeholders, has the ability to address both the traditional notions of uncertainty but also the new challenges of environmental non-stationarity (Allen et al., 2011). Maintaining legitimacy for environmental flows will likely require a more concerted and continual effort.
The adaptive management cycle is an iterative process divided into three key phases: planning, learning, and doing. In environmental water management, environmental flow assessments currently form a key component of planning (Mussehl et al., 2022). While environmental flows assessments have largely depended on biophysical methods, there has been increasing recognition of the role of social science and the importance of considering an interconnected socio-ecological system (Pahl-Wostl et al., 2013; Anderson et al., 2019). Methods such as SUMHA (Sustainable Management of Hydrological Alterations) explicitly represent the importance of stakeholder involvement for adaptive management. A number of environmental flow methodologies include an iterative loop to represent an adaptive approach (e.g., ELOHA, Poff et al., 2010; SUMHA, Pahl-Wostl et al., 2013). These iterative loops relate to the outer loop of adaptive management, i.e. informing broad decisions made at the longer time scale (5–10 years). However, there is also an opportunity for year-by-year incremental learning through monitoring (linking to the inner loop of adaptive management). This would require an environmental flows assessment method that allows consideration of non-stationarity through an approach of continuous learning. There are recent publications that explore how technical aspects of environmental flows and ecological modelling can adjust to better represent climate change impacts (Horne et al., 2019; Tonkin et al., 2019). However, there has been no detailed analysis of what is needed from an environmental flows assessment as a whole, or what structure it should take, to ensure adaptive management is possible at both the inner and outer loop scales.
In this paper we describe a environmental flow assessment framework that links to the adaptive management cycle and explicitly allows consideration of non-stationarity and uncertainty, including climate change. The approach described can either be adopted as is, or individual elements can be integrated with other existing environmental flow methodologies to improve the ability for adaptive management. We begin by discussing key considerations for the environmental flow assessment process (Section 2). We then introduce a possible framework for environmental flow assessments (Section 3) and demonstrate the application of this framework using the Kaiela, Victoria, Australia as a case study (Section 4). Environmental flow assessments form a foundational activity in the planning of environmental water programs. This paper demonstrates an approach to environmental flows assessment that provides a critical step forward in enabling the successful implementation of adaptive management rather than remaining an aspiration for environmental water management.
2 Elements to Enable Adaptive Management in Environmental Flow Assessments
While the role of adaptive management is embedded within the environmental flows literature, there is currently little practical guidance on how to incorporate adaptive management into the environmental flows assessment process (Mussehl et al., 2022). Here we outline a number of important elements for consideration in the structure of an environmental flows assessment, drawing on the adaptive management literature. A core theme is the need to inform trade-offs and directly consider uncertainty, two concepts that are poorly dealt with in current environmental flows assessment methods (Williams et al., 2019). These concepts are not new in themselves, but they have not been linked together within the context of an environmental flows assessment process. Note there is a body of literature more broadly on enabling factors for adaptive management (Rist et al., 2013; Gregory et al., 2006). Here we focus specifically on the approach to environmental flow assessments as a core element of an adaptive approach to environmental management. Five central concepts are details below.
2.1 Acknowledgement of Uncertainties
A core principle of adaptive management is the ability to adapt and alter decisions and adjust hypotheses over time as uncertainties narrow. This concept is well suited to environmental management where “knowledge is incomplete, and when, despite inherent uncertainty, managers and policy makers must act” (Allen and Garmestani, 2015). Despite growing implementation, our ability to predict the outcomes of environmental flows applications remains limited (Acreman M. C. et al., 2014), and they are thus well suited to being managed adaptively (Webb et al., 2017). While it is well acknowledged that uncertainties exist, they are rarely captured or articulated within environmental flows assessments. Successful implementation of adaptive management requires documentation of what we know and what we assume or predict (Williams and Brown, 2014; Allen and Garmestani, 2015). Thus, a key element of an environmental flows assessment to support adaptive management is a shift to documenting and embracing uncertainties and assumptions (Horne et al., 2018). This includes capturing and understanding the underlying assumptions and objectives that lead to specific flow recommendations, as a single flow component often supports several poorly documented aims, making adaptive management more challenging.
Importantly there are different types of uncertainties and these will be represented and addressed differently within an adaptive management framework. As noted above, much of the foundations of adaptive management consider these uncertainties in the context of a stationary environment. Therefore, climate change and other non-stationarities pose challenges to traditional adaptive management approaches (Williams, 2011). One strategy is to incorporate scenarios into the adaptive management process and develop adaptive decision making to respond to key triggers (Williams, 2011). This allows explicit consideration of a range of unknown futures and how they might impact achievement of objectives.
2.2 Stakeholder Engagement
Participatory approaches are discussed as a crucial element of planning for climate change (Burton and Mustelin, 2013; Tompkins and Adger, 2004). A number of recent publications have renewed calls for greater stakeholder participation and consideration of environmental flows within a socio-ecological system (Anderson et al., 2019; Conallin et al., 2018). Stakeholder engagement throughout planning and decision-making is critical to fostering process-based legitimacy and community acceptance. Process based legitimacy, or input legitimacy, is as important as outcome efficacy, or output legitimacy, to overall program success (Godden and Ison, 2019; O’Donnell et al., 2019). This was clearly demonstrated in the initial stages of development of the Murray-Darling Basin Plan in Australia, where a number of key stakeholder groups were not engaged in the process, leading to widespread mistrust (Colloff and Pittock, 2019). Including a wide range of stakeholders is particularly important where trade-off decisions will be required or where the science remains uncertain or contested (Pahl-Wostl et al., 2007), both of which are relevant to environmental flow management under climate change.
However, to date, much of the literature concerning stakeholder involvement in environmental flows has focussed only on objective setting (Acreman M. et al., 2014). It is well recognised that ecological objective setting involves a societal choice and a range of relevant perspectives (Pahl-Wostl et al., 2007). Including a clear and structured process to establish objectives as part of an environmental flows assessment is important in resolving conflicting objectives for a river system, and identifying fundamental objectives (those that are inherently important) and means objectives (those things important to achieve fundamental objectives) (Mussehl et al., 2022). In contrast, the literature around participation and co-design highlights the importance of involvement throughout the process including those aspects traditionally treated as a wholly technical process within environmental flows management (Mussehl et al., 2022). Rather than attach the participatory process around existing technocratic approaches to environmental flows, it may be beneficial to restructure the technical aspects of environmental flows assessment to fit within a participatory framework (Mussehl et al., 2022). This approach allows the integration of participatory and technical approaches to addressing non-stationarity (Bellard et al., 2012; Horne et al., 2019; Poff, 2018; Tonkin et al., 2019), improving decision making and resilience building (Boltz et al., 2019; Brown, 2012). This also connects to the literature around legitimacy and the importance of process for gaining stakeholder acceptance where views diverge (O’Donnell et al., 2019).
2.3 Multiple Sources of Knowledge
Most environmental flows assessments focus primarily on scientific and technical approaches to quantifying flow requirements, placing a large emphasis on ‘best available science’. This is founded in an underlying assumption that science is objective and unbiased. However, all knowledge is partial and situated within a specific perspective and context (Rosendahl et al., 2015; Haraway, 1988). Individuals who contribute knowledge to the environmental flows process will bring their own perspectives and unique values, and evidence suggests that our perceptions of risk influence decision making in water resources (Kosovac et al., 2019). Many approaches to environmental flows assessment are dominated by discipline-based knowledge articulated through expert elicitation or through data-driven modelling when enough data are available. Expert elicitation processes can be designed to minimize bias through well designed elicitation protocols, engaging with a range of disciplines, and the use of appropriate models (de Little et al., 2018; Webb et al., 2018). Such discipline-specific thinking also affects the methods and characteristics of climate change impact assessments for freshwater ecosystems (John et al., 2021b).
However, environmental flows management takes place within a complex socio-ecological system and rivers can be understood in a multitude of ways. Managing environmental flows for diverse objectives requires decision makers to consider multiple knowledge sources (Poff et al., 2003; Roux and Foxcroft, 2011). These knowledge sources can be technical, administrative, political, traditional, and local in nature, and may include supporting empirical data (Raymond et al., 2010). Given the validity of multiple types of knowledge for decision-making, environmental flows assessment methods should incorporate these different sources of knowledge. Adaptive management can be framed in a way that supports incorporating these different sources of knowledge, particularly when paired with participatory frameworks (Fujitani et al., 2017). Including participatory modelling approaches as an element of the adaptive management cycle creates spaces for knowledge coproduction, ensuring that diverse perspectives are represented within the models (Voinov and Gaddis, 2008).
2.4 Modelling That Supports Trade-off and Change
Modelling and documentation is a core component of adaptive management. There are two distinct elements of modelling to support adaptive management of environmental water (Stewardson and Rutherfurd, 2008; Kingsford et al., 2011). The first is an explicitly defined conceptual (or mental) model of how the ecological objectives link to anthropogenic processes and relevant flow management decisions (Kingsford et al., 2011). Such models are particularly important when using a participatory approach; they can assist with co-learning by multiple stakeholders by exposing different understanding of system behaviour (Kingsford et al., 2011). The second type of model is a quantitative predictive model that is used to evaluate potential management decisions. The relationships in this predictive model should be consistent with the conceptual model, but they may comprise a reduced range of responses and processes (Horne et al., 2018).
In a non-stationary environment, a major consideration is how the models will respond to conditions outside those experienced historically. Tonkin et al. (2019) highlight the challenge of commonly used regression models to predict ecological responses to flow in a non-stationary environment. These approaches assume that the current relationships between flow and management actions will extrapolate into new climate conditions. They highlight the importance of using mechanistic models that can predict outcomes under a range of future environmental regimes. These models allow for management outside of the conditions (magnitudes, frequencies and timing) that have been experienced historically. They also support a necessary shift beyond the natural flow paradigm to environmental flows management that adopts a designer flow approach (Acreman et al., 2014a; Poff, 2017).
2.5 Link to Monitoring
The key to successful adaptive management is learning. In the context of the mechanistic biophysical models mentioned above, such learning is enabled through monitoring and research in the system being managed. Environmental flows monitoring programs are often aimed at measuring progress towards environmental flows objectives (Gawne et al., 2021; Gawne et al., 2020), thus demonstrating the ‘return on investment’ on the taxpayer funds invested in environmental water. While such monitoring is an important part of the accountability of environmental flows and the social license to use water that might otherwise have been employed for consumptive purposes, it is not the best monitoring for adaptive management. This type of outcome-focused monitoring by necessity needs to have questions set at the start of the environmental flows program. Monitoring methods may be able to evolve over time (e.g., Webb et al., 2019), but the measurement endpoints are selected at the beginning of the program and cannot be readily changed.
For adaptive management, the focus of monitoring needs to be on reducing aleatory uncertainty arising from the random variability in the parameters included in existing models, but also on reducing epistemic uncertainty inherent in the model structures themselves (see Beven, 2016 for a discussion of types of uncertainty). Outcome-focused monitoring may be able to reduce aleatory uncertainty if the overall program objectives overlap with uncertain relationships in the quantitative models. However, such monitoring is very unlikely to reduce epistemic uncertainties in model structure, which are constructed to detect pre-identified outcomes (i.e., test a priori hypotheses). A need to reduce epistemic uncertainty implies the need for research, rather than monitoring as such. The two terms overlap, but we use them separately here to emphasize that research (unlike monitoring) is designed to disentangle mechanisms in the processes leading to environmental flow outcomes, or to fill specific knowledge gaps regarding important relationships. Such research also needs to be flexible and reactive to new learning as it occurs during the adaptive management cycle.
This flexibility will also be a necessary feature of research as we head into an uncertain future. We face the prospect of step changes in the systems being managed, potentially requiring modification of initial models to account for evolving mechanistic understanding of system properties under non-stationarity of environmental drivers. Being flexible and reactive inevitably leads to tension and trade-offs between the relative value of research that responds to the latest learning and identified knowledge gaps, versus long-term data sets collected using the same methods. Both types of knowledge acquisition are important, but currently environmental flows monitoring programs are biased towards long-term standardized data collection aimed primarily at demonstrating program outcomes (e.g. The Commonwealth Environmental Water Holder Office monitoring program (MER) in Australia; (Gawne et al., 2021)).
3 An Adaptive Environmental Flow Assessment Approach
Environmental flows assessment are a key aspect of environmental flow management more broadly (Horne A. et al., 2017). In the previous section we detailed five key elements that would better allow environmental flows assessments to enable adaptive management of environmental flows management. In Figure 1, we propose an approach to environmental flow assessments that addresses these five elements. The approach is based around the fundamental aspects of participatory modelling (Voinov et al., 2016) and links this with themes from adaptive management (Mussehl et al., 2022). Rather than a linear method, the approach highlights the iterative nature of participatory approaches and their multiple feedback loops. The outer loop (forming a circle) represents the longer-term iterations and feedbacks that happen through environmental flows management. The inner-loops and feedbacks represent the continuous learning that can occur iteratively on a year-to-year basis.
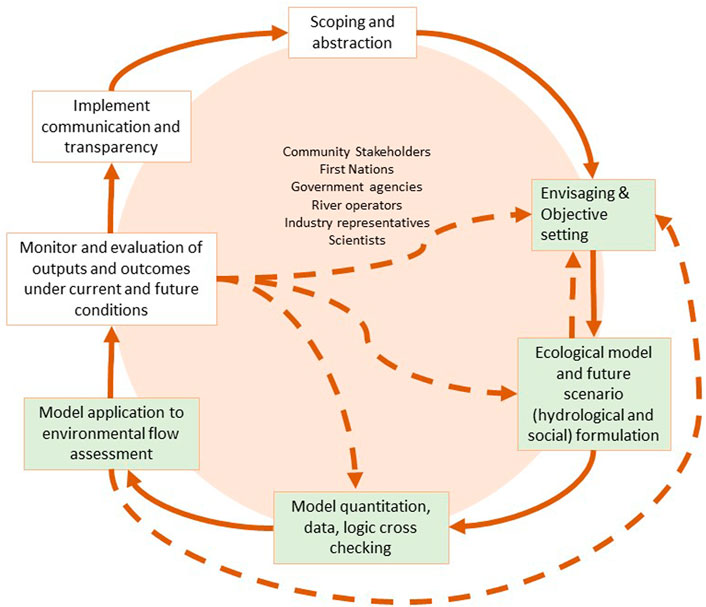
FIGURE 1. Key Elements of an Environmental Flow management that considers non-stationary environments. The overall approach is based on participatory modelling (adapted from Voinov et al., 2016). People are central to the trade-off decisions that will be required under water scarcity, and for planning for adaptation and transition. Feedback loops within the approach link to adaptive management concepts (see Mussehl et al., 2022). Outer loop of adaptive management is shown with a solid line, inner loops with dotted lines. The green activities are those that commonly form part of an Environmental Flows Assessment. In order for an environmental flows assessment to contribute to adaptive management, the link to the white blocks needs to be explicitly planned. Modelling components consider future scenarios, adopt mechanistic methods and incorporate multiple sources of knowledge. They are designed to support trade-off decisions.
Figure 1 shows the complete environmental flows management process. The stages shown in green are usually part of an environmental flows assessment. However, to support adaptive management, these stages need to be part of an iterative loop and must connect the broader stages of environmental flows management. The stakeholders are essential across all stages of environmental flows management and should actively be engaged and drive each stage. We briefly outline the steps below before demonstrating them in more detail through a case study in the following section.
1) Scoping and abstraction–This step involves understanding the context for environmental flows management, the key threats or issues of concern, policy context and decision-making process.
2) Envisaging and objective setting–A stakeholder driven approach to identifying objectives. Clearly articulating fundamental objectives and means objectives will help clarify and support management decisions, modelling and research needs at later stages.
3) Ecological modelling and future scenario formulation–Development of stakeholder informed conceptual models that link fundamental objectives, through means objectives to the decisions that can be managed (such as flow components or riparian zone management). Development of future scenarios (both climate and social) that may also impact on environmental flow outcomes. Adopting a participatory approach to build a shared understanding that incorporates multiple knowledge sources.
4) Quantifying model, data, logic, cross checking–Translation of conceptual models into quantified models, making use of expert knowledge and data. Modelling approach should be stakeholder driven and consider available resources and existing information. Modelling should be considered “living models” that can be readily updated as new research and data become available. The modelling approach used should be able to perform under non-stationarity.
5) Applying modelling to environmental flow assessment–Stakeholder driven approach informed by a combination of ecological models and flow scenarios used to sensitivity test the system and identify priority flow components. Consideration of ideal flow ranges rather than single values for each flow component to allow operational decisions to vary through time.
6) Monitoring and evaluation–a monitoring program to assess the success of the environmental flows program, informing both the short term and long term adaptive management loops, and feeding data into updated quantified models. This includes the opportunity to update ecological models based on new research through the inner loops of adaptive management.
7) Implementing broader communication and transparency–a communication strategy designed to share information about the environmental flows program beyond those stakeholders immediately involved. Note this is in addition to the participatory approach to the overall environmental flow assessment, where clearer documentation of conceptual models, data and uncertainties, should in itself improve transparency. The approach to environmental flow linking participatory processes with the bio-physical sciences will improve communication and transparency with involved stakeholders in an ongoing way throughout the environmental flows assessment.
We present the environmental flows approach here as a complete method to support adaptive management, however these concepts can readily be incorporated into existing environmental flows methods. As an example, ELOHA is a well known environmental flows assessment method (Poff et al., 2010). A key difference to our proposed approach is that ELOHA separates out the biophysical and social aspects of the assessment. In contrast, we make the case that integrating these elements is more likely to lead to legitimacy and flexibility of adaptive responses. While methods such as ELOHA include an outer-adaptive management loop, they stay silent on how individual components should be undertaken to account for non-stationarity and to facilitate adaptive approaches (Poff et al., 2010; Stein et al., 2021). Each of the key elements in ELOHA (such and the hydrological foundation and the flow-ecology modelling) could adopt the concepts outlined in this paper to better support adaptive management.
4 Case Study: The Kaiela, Victoria, Australia
4.1 Background
The Kaiela (Lower Goulburn River) in northern Victoria, Australia, is the stretch of river downstream of the Goulburn Weir to the confluence of the Murray River (Figure 2). The Kaiela (meaning “father water”) forms part of the Yorta Nation. The Kaiela has significant environmental values associated with the river and its floodplain and wetland habitats (Gawne et al., 2013).
Flows in the Kaiela have been significantly altered by the construction and operation of upstream Lake Eildon (which has a storage volume of roughly twice mean annual inflows) and Goulburn Weir. Water traded to the Murray River system (referred to as Inter-Valley Transfers) causes significant volumes of water to be transferred out of the Goulburn system over the irrigation season, leading to unseasonal and prolonged high summer flows downstream of Goulburn weir.
Environmental flows are provided in the Goulburn River through a number of different legislative mechanisms. A large proportion of environmental flows are achieved through environmental water entitlements that can be actively managed by environmental water managers (Doolan et al., 2017).
4.2 Environmental Flows Assessment
4.2.1 Scoping and Abstraction
The environmental flows assessment was initiated by the Goulburn Broken Catchment Management Authority (GBCMA). Stakeholder identification took place through consultation with GBCMA representatives, with participants broadly categorized into the following three groups: agency representatives, expert phanel scientists, and community members. Community member participants were recruited based on further conversations with the GBCMA and focused on current members of the existing Environmental Water Advisory Group (EWAG). A Yorta Nation representative was part of the advisory group for the project. There were between 20 and 35 people at each workshop. Initial phone interviews were held with all identified stakeholders to gauge key areas of interest and concern for establishing environmental flow recommendations.
4.2.2 Envisaging and Objective Setting
A workshop was used to elicit a first cut of the objectives specific to the management of environmental water and associated decisions around flow regime. In the development of an objectives hierarchy, we focused on presenting the fundamental (core driving or strategic objectives) and means objectives (the objectives that need to be met to achieve the fundamental objectives) (Gregory et al., 2012). The workshop used a series of group-based activities to elicit this information from stakeholders. Key points of contention were identified and discussed collectively in the later stages of the workshop.
Four overarching objectives for the Kaiela were identified through the workshop process:
1) Maximise native floral biodiversity
2) Maximise native faunal biodiversity
3) Maximise self-sustaining populations of icon faunal species
4) Promote community health and wellbeing through connection to river
These four overarching objectives were defined more specifically as fundamental objectives, with underpinning means objectives. The fundamental objectives were:
1) Maximise self-sustaining populations of opportunistic fish
2) Maximise self-sustaining populations of periodic fish
3) Maximise self-sustaining populations of equilibrium fish
4) Maximise self-sustaining populations of turtles
5) Maximise self-sustaining population of platypus
6) Maximise structural complexity and diversity of floodplain vegetation, including wetlands
7) Maximise structural complexity and diversity of bank vegetation
8) Ensure social and community needs of the river are met (including fishing, boating, swimming and ceremonial uses)
The workshop revealed that the overall values held across the diverse group of stakeholders for the river were quite consistent. While there were variations in the wording that different stakeholders used to describe objectives, the fundamental objectives where consistent across groups. The fundamental objectives show that the river is valued for both its intrinsic value (e.g., biodiversity), and also the social wellbeing and interactions it provides (e.g., recreation). The legislation for the region makes it clear that Environmental Water must support environmental objectives. However, the method accommodates this by allowing the stakeholders, in their diversity, rather than ecologists to set the objectives. There is one fundamental objective that is specifically about social outcomes. This can be modelled and assessed in the same way as the ecological outcomes (noting that many of the ecological objectives support the social outcome in which stakeholders are interested).
Each fundamental objective can be achieved through meeting a number of means objectives (refer to supplementary material for a complete table). For example, maximising self-sustaining populations of periodic fish requires supporting population survival (through ensuring instream habitat diversity, maintaining water quality to support refugia (minimize blackwater events), maximizing macroinvertebrate community biomass, supporting population recruitment (through provision of flow related spawning cues, and Ensuring longitudinal connectivity throughout channel and supporting population movement (through ensuring longitudinal connectivity throughout channel and to larger Murray system). These means objectives are reflection in the ecological models developed in later stages. Importantly, while supporting macroinvertebrate populations and geomorphology were not fundamental objectives identified by stakeholders, they came up repeatedly as means objectives and essential for meeting the range of fundamental objectives.
Along with specific objectives for the river, stakeholders repeatedly raised the importance of process objectives—objectives related to how decision-making should be conducted. These included the desire for community ownership, transparency and knowledge exchange (refer to supplementary material for further details). These objectives link to the overarching themes of participatory and adaptive approaches to environmental flows.
4.2.3 Ecological Models and Future Scenario Generation
Initial conceptual models were developed in a stakeholder workshop. The workshop deliberately mixed agency representatives, scientists and community members so that different knowledge systems were incorporated, and to facilitate a shared understanding. These models were then documented and refined based on discussions with technical experts which ensured consistency in terminology and approach. Many refinements were aimed at simplifying models to ensure they were appropriate to translate into quantifiable models. This was an iterative process with the technical expert in each area.
Models were developed for each of the fundamental objectives (see supplementary material), where a performance measure was chosen to represent each objective. Models were also required for a number of elements that while not fundamental objectives, are essential drivers of change for the fundamental objectives:
1) Macroinvertebrate biomass and diversity
2) Bank stability
3) Instream habitat complexity
4) Instream productivity
The ecological models developed through this process have a very specific role; they are not detailed ecological life-cycle models. They aim only to include enough detail to prioritise or support different flow release decisions by environmental managers. The models are mechanistic to support management under climate change (Tonkin et al., 2019) and incorporate aspects of reoccurrence of events through the antecedent condition node (Horne et al., 2017c). The conceptual model for periodic fish is shown as an example in Figure 3.
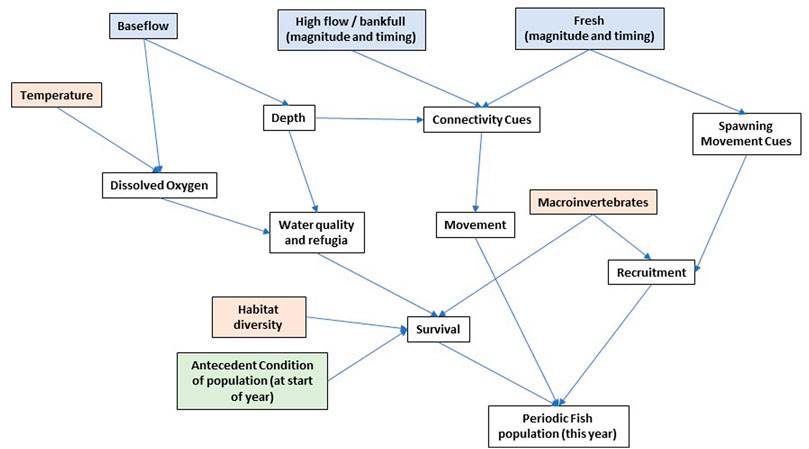
FIGURE 3. Conceptual model for periodic fish (blue indicates flow components, orange is non flow drivers and green is antecedent condition of the population). The model represents the condition of periodic fish following a year of river flows.
Future flows scenarios were based on stochastic data (Fowler et al., 2022), shifted to reflect plausible future changes in climatic conditions (Table 1). In general, these shifts are consistent with global climate models (GCMs) from CMIP5 projections for a 20-year planning horizon and a high emissions scenario (RCP8.5). Generally, the “Wet” scenario follows the upper range of wetter GCMs, the “Dry” scenario follows the lower range of hottest and driest GCMs, and the “Moderate” scenario is the multi-model mean GCM outputs. However, it is noted the various GCMs provide a range of future projections (Grose et al., 2020). The future unregulated flows provide input to a water resource model to simulate outcomes under current regulated water management rules (John et al., 2021a).
4.2.4 Model Quantification
The conceptual models were translated into conditional probability networks (Horne et al., 2018) using a formal expert elicitation process. Conditional probability networks were adopted as they allow for incorporation multiple of sources of information (data and expert views), they can be readily updated, and they show a physical structure that stakeholders could connect to the conceptual models previously developed through workshops (Horne et al., 2018). The ecological models enable the relative outcome between flow scenarios to be assessed, with an indication of the likely overall condition for each objective. (Note: A similar netica approach was taken to compare relative futures by Bestgen et al., 2020).
The expert elicitation process was based on the methods developed in de Little et al. (2018). Surveys were used to elicit expert predictions on the effects of environmental flow deliveries. Experts were asked to estimate the likely condition of a certain model element, given different combinations of its driving variables. All experts were asked to complete the survey for each ecological model, even when the model was outside their area of expertise because using a range of respondents with diverse backgrounds leads to more robust outcomes and less bias (Hanea et al., 2017). The aggregated predictions from experts became the prior probability distributions used to parameterise the models. Bayesian modelling was then used to incorporate monitoring data into the models, creating a posterior modelled output that is driven by both expert knowledge and data. Given the data available, integration of the data at this time only had minimal impact on the models. The models were created within the software package Netica. All models are provided in supplementary material. The CPNs were used to examine the best combination of flow components, and the sensitivity of overall outcome to different flow components (refer to supplementary material). The models were tested using historical flow data and the results compared with monitoring data for fish (refer to supplementary material for further details).
4.2.5 Model Application to Environmental Flow Assessment
Each model was assessed through a workshop process to check whether they were performing as expected, or that outcomes could be adequately explained. A flow tool was developed in MatLab to simulate the outcomes for each objective under different multi-year flow scenarios. This was used to test ecological model performance and compare to historical data. The flow tool was also intended to support environmental manager decisions on an ongoing basis. By incorporating antecedent conditions in the ecological models (i.e., ecological conditions at the start of the year based on either modelled output or surveyed data), the flow tool can inform the best flow strategy for the coming season and thus generate maximum performance over time. In other words, an environmental manager can assess at the start of the year what the flow priorities will be over the coming year by running the flow tool with information about current ecological conditions and predicted flows for the season.
A structured workshop was used to define the environmental flow recommendations. The ecological models and flow tool were significant inputs to this workshop. The workshop asked participants to firstly prioritise flow components for each individual objective, and then to work in small groups to prioritise flow components across objectives and understand when and why the priorities might change due to external drivers (such as climate).
While in most environmental flow assessments the flow recommendations are the key output and management tool, the key output through the Kaiela project is the flow assessment tool described above that allows different flow scenarios to be run through the ecological models. However, environmental flow recommendations were included as they are an output that managers are familiar with. The ecological models were used to determine the key flow components across the full suite of ecological objectives and their relative priority in providing outcomes. A facilitated discussion at a stakeholder workshop was used to develop a set of flow recommendations (details in supplementary material). The recommendations are given in priority order, where each year the higher priority flow components should be provided where possible before moving down the list. This reflects the variable availability of environmental water in the Goulburn River and allows planning for years with minimal water allocations. This is a very distinct approach to those environmental flows methods that might classify flow recommendations based on dry to wet years, which can be challenging for managers without knowing how the year will indeed unfold. Similarly, to allow for and indeed encourage intra annual variability, flow recommendations are made as a range, rather than the absolute numbers they have previously been provided as. For example, the year round baseflow recommendation (aimed at providing habitat diversity and sustaining the system) is given as:
1) Preferred flows are between 500–1000 ML/d (or natural) during summer and autumn
2) During summer and autumn, ensure variability in flow regime (CV > 0.2) (e.g., mean of 750 and standard deviation of 150 ML/d)
3) During winter and spring ensure flow is great than 500 ML/d.
To recognize the operational constraints of delivering particular flow components, the recommendations specify some events as “opportunistic”. For example, the overbank flow recommendation states “Opportunistic event–aim to provide as high as possible an event by piggybacking natural event with a dam release. Where overbank not possible, still provide as large an event as possible (aiming for 15,000 ML/d) for channel maintenance and forming.” Where there are trade-offs between flow components (e.g., some favor fish over vegetation), the recommendations specify that these should be considered based on antecedent ecological condition.
The flow tool was also used to test the flow recommendations and ensure their implementation leads to positive ecological outcomes through the ecological models. A timeseries of flows that achieves the full flow recommendations was developed and run through the flow tool for validation purposes (Figure 4). This approach was used to test how the models respond and confirm that the flow recommendations were leading to the best outcomes. Due to the inclusion of a node that represents antecedent conditions, it will take some time for species to reach their equilibrium behaviour if this same flow regime was provided every year. The antecedent condition for all models was set to 0 (i.e., 100% poor or equivalent) at the start of the simulation. There is a clear difference between this figure for validation purposes (where each flow component is provided every year) and the reality of how an environmental water manager would use water. In reality, the flow recommendations would not be perfectly achieved or the same hydrograph repeated year in year out. Rather, an environmental flow manger would only provide a subset of the flow components in years where adequate flows are not available, and the condition of different objectives would vary through time.
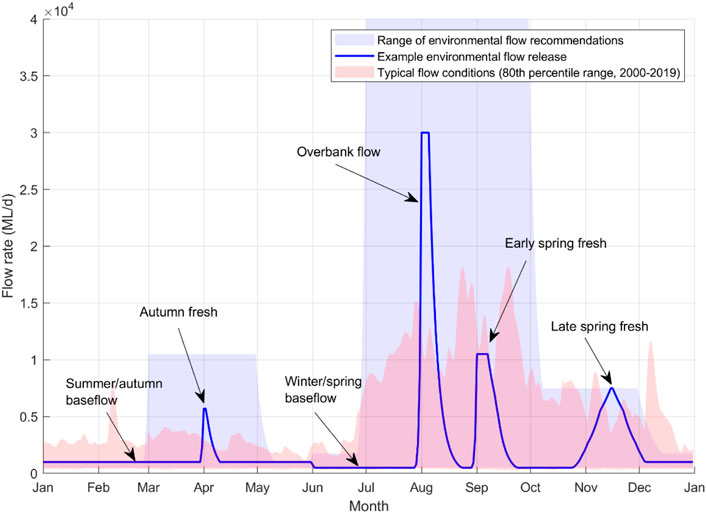
FIGURE 4. Example annual timeseries achieving the flow recommendations and average current flow (as impacted by regulation) in the Kaiela for two time periods.
The output for all models in their overall condition is shown in Figure 5. Some models respond more quickly than others, but the typical range for achieving equilibrium condition is between two and 5 years. None of the models reach a steady state condition of 100% even following repeated delivery of all environmental flow components. There are several reasons for this.
1) The results in Figure 5 are the proportion of maximum possible condition achievable through flow manipulation. The results show that it is not possible to simultaneously maximize benefit for all ecological endpoints in the river, even with unlimited environmental water.
2) The overall condition index is a composite of the different states of potential outcome (e.g., Good, Average, Poor). It is not a deterministic prediction of condition.
3) Experts stated their uncertainties in the parameterization of the ecological response models to reflect ecological outcomes under unforeseen events and poorly understood processes. Uncertainties were also incorporated into the calculation of the overall condition index.
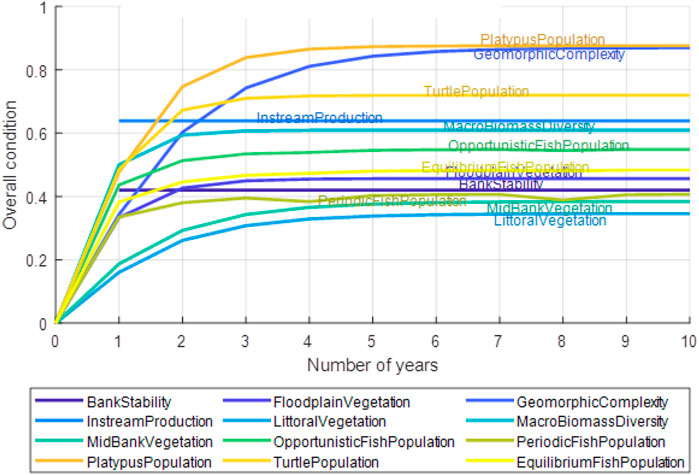
FIGURE 5. Model responses to achieving a repeating series of the flow recommendations (derived for model validation based on providing the full flow recommendations year in year out. In practice performance would vary year to year as different flow regimes were provided, acknowledging that the complete suite of flow components cannot be delivered every year).
The flow tool also allowed assessment of future climate scenarios to be considered, something that is not possible with existing environmental flow assessment approaches. This type of assessment moves away from the interannual and incremental adaptive management, to also allow longer term larger adaptive cycles to address the challenge of non-stationarity. Stochastic data sets (110–year sequences) across dry, average and wet scenarios were put through the flow tool. The performance under different scenarios was compared using the stress metric outlined in Nathan et al. (2019). Here, ecological changes were assessed by comparing the distribution of outcomes from each scenario against the distribution of outcomes from the baseline scenario. A stress index is calculated that reflects the proportion of the future distribution that does not overlap the baseline distribution. The index ranges from −1 to 1, where −1 is a distribution of outcomes wholly worse than baseline conditions, 1 is a distribution of outcomes wholly better than the baseline and 0 is future that is indistinguishable from the baseline.
Climate scenario results are shown in Figure 6. In these figures, each year of stochastic data is shown as a separate climate replicate (grey lines), with the overall flow regime inferred from the median of individual years. This allows an assessment of how natural climatic variability influences the range of hydrologic conditions. The stress scores for each ecological end point are shown in Table 2.
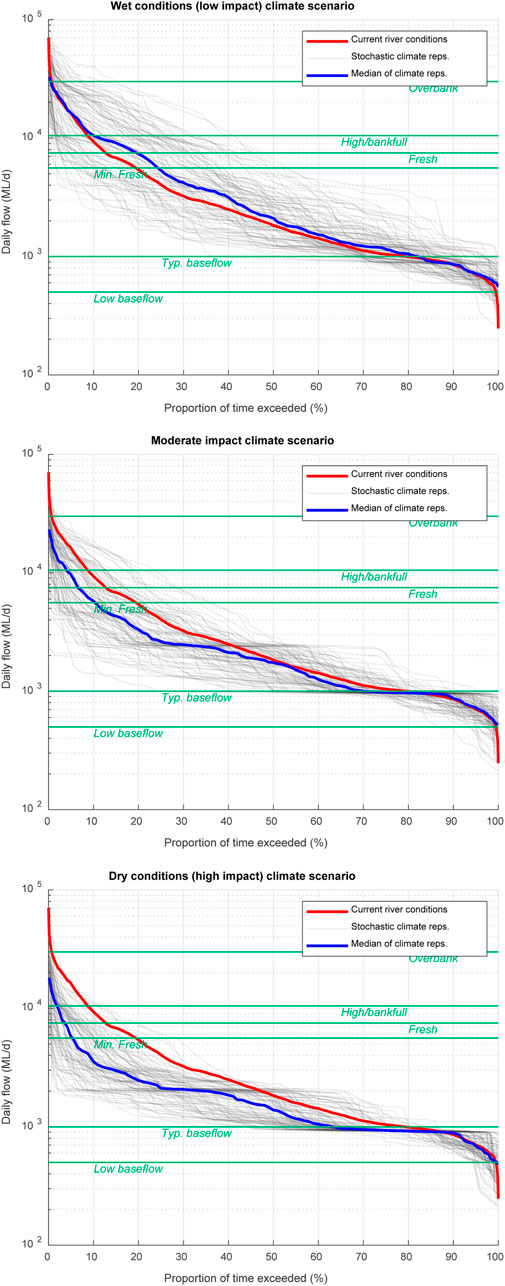
FIGURE 6. Future scenarios showing hydrologic outputs from three climate scenarios. These exceedance curves show the proportion of time (x axis) that a given flow (y axis) will be exceeded.
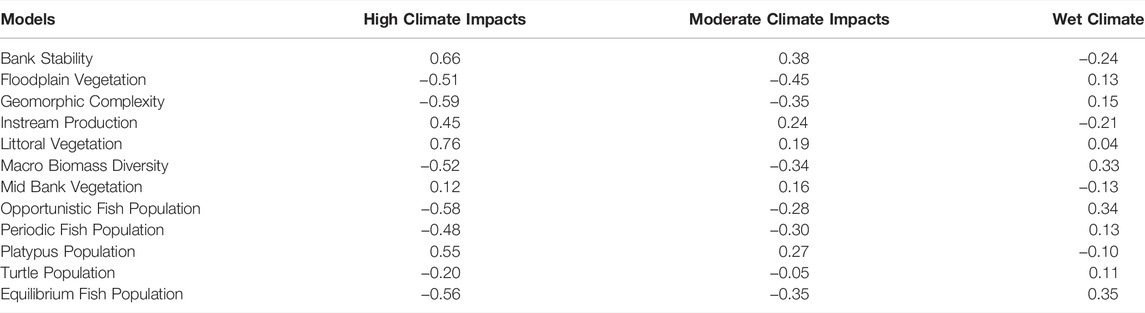
TABLE 2. Stress indices calculated for each model and scenario (where dark orange represents a high stress score and dark green represents a high benefit, stress index range from −1 to 1 where a score of 0 indicates no change in distribution of outcomes between current and climate scenario, -1 indicates a worse outcome completely outside current conditions, and 1 indicates a better outcome).
The future climate scenarios reveal some unintuitive hydrologic results. For example, while it is expected that a wetter climate future will assist in providing ecologically relevant flows, these same conditions may also lead to adverse outcomes due to increases in summertime intervalley transfers to meet trade demands in the Murray River. In a moderate climate scenario with 5% reduction in long-term annual rainfall, the ability to deliver certain high flow components can reduce by up to 10% compared to current conditions. In a high impact scenario this reduction is approximately 20%. Baseflow conditions remain less affected under climate change compared to high flows. Under both the moderate and high impact climate scenarios, high flow events are significantly reduced, which would impact the ability to deliver water to the lower Kaiela floodplain through piggy-backing storage releases of environmental flows to natural flow events. These overbank flows are important across a range of the ecological objectives. Under drying climates, high flow components are amongst the first to be affected due to lower seasonal rainfall and drier soil moisture regimes (Table 3). It is important to consider that long dry spells and wet spells typical for the Australian climate may lead to extended periods of high or low flows. For example, in the high impact climate scenario, although the average proportion of years with flows over 30,000 ML/d was 9%, within the 110 years of simulated data there was a sequence of 29 years consecutively below the threshold. Therefore, it is all the more important to be able to deliver this flow component through environmental water rights and releases from storage. Figure 7 shows the implications of not providing these large flow events (this figure can be compared with Figure 5). This initial assessment shows some major challenges for managing environmental flows under climate change based on the current policy settings and environmental flow objectives. This triggers the need for more detailed assessment of options to meet environmental flow objectives (for example, as undertaken in John et al., 2021a).
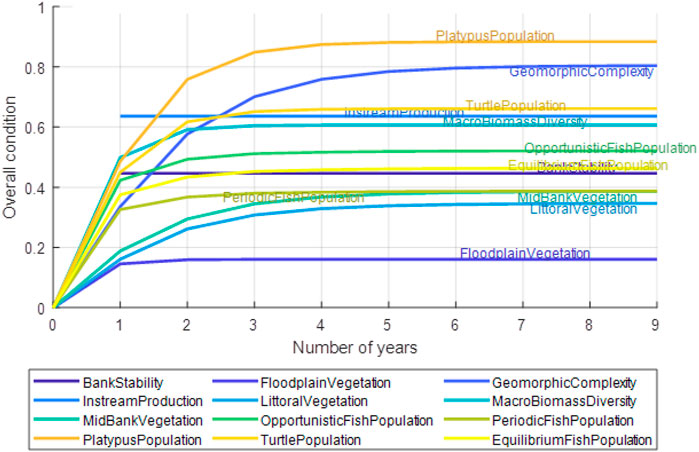
FIGURE 7. Model responses to achieving a repeating series of the flow recommendations, without the inclusion of overbank flows.
4.2.6 Monitor and Evaluation
Monitoring and evaluation for the system is not funded through the same project and funding pool as the environmental flows assessment process. This causes some disconnect within the adaptive management cycle. Monitoring on the Kaiela is funded through state and federal agency projects with the goal of creating long term, continuous ecological data sets (Webb et al., 2010; Treadwell et al., 2021). These are objectives-based programs that monitor ecological responses to flow events with the aim of evaluating the efficacy of environmental flows management. These monitoring programs were designed outside of environmental flows assessments and are an integral part of the overall monitoring scheme for the entire Murray-Darling basin. Regulatory agencies need continuity and consistency in monitoring methods and locations, reducing program flexibility. That work will continue to incrementally reduce aleatory uncertainty within the existing models, and indeed the empirical data used to update the prior relationships developed in this study were drawn from one of these programs (Gawne et al., 2020). However, the current monitoring and research design (Webb et al., 2019) pre-dates this environmental flows assessment, and was not designed specifically to reduce uncertainties in the models generated. Hence, advances in understanding are likely to be small.
4.2.7 Communication and Transparency
The Goulburn Broken Catchment Management Authority (GBCMA) is responsible for the ongoing management of environmental flows. While a communication strategy was not part of the environmental flows study, the discussion of objectives made it clear that transparency, community involvement and communication are essential for the success of the program.
The GBCMA has engaged an environmental water working group made up community members and key stakeholders to have an ongoing voice in the management of environmental flows. Every year, the GBCMA is required to publish a seasonal environmental watering plan that outlines progress in the previous year and priorities for the following year. The GBCMA also publishes regular communications in print and social media and through electronic and printed newsletters regarding the environmental flows program and individual watering events. This is being done through the current monitoring and research program (e.g. Treadwell et al., 2021), but at least partly fulfills the need for transparency identified by our flows assessment method.
5 Discussion and Conclusion
The challenges posed by non-stationarity require us to re-examine environmental flow assessments (Poff, 2018). In this paper, we have demonstrated how to explicitly consider adaptive management under an uncertain future in an environmental flows assessment approach. While many of the elements we propose including are common to existing methods, we advocate a significant shift in the mode of implementation and explicit representation of uncertainty and climate change scenarios, incorporation of multiple knowledge sources, modelling to support trade-offs and decision making, and links to monitoring. The outputs of the environmental flow assessment are tools and models that link to decision making and ideally be readily updated with new knowledge in a changing environment. This marks a significant shift from traditional environmental flows assessment methods that provide a more static approach, with flow recommendations fixed until the next iteration of a flows assessment.
The Kaiela case study was used to demonstrate a framework for environmental flow assessments that addresses the needs of managing under uncertainty and change. There are a number of key reflections from this case study.
1) Resourcing often dictates environmental flow assessment methods. The resources available for an environmental flows project, including the timeline, are normally externally dictated. It is difficult to incorporate a thorough stakeholder engagement process within these constraints. Where project funding and timeline are constrained, the proposed approach will not be possible.
2) Participatory approaches take time and flexibility and funders and stakeholders need to be on board. Environmental flow assessments often have clearly articulated legislative requirements (Acreman et al., 2017), and funders are more comfortable with a linear project management approach. The success of the Kaiela flows study was in part due to GBCMA’s willingness to explore new approaches and respond to the participatory approach, and the ability to combine the study with several students PhD topics providing additional resources that are not usually available to an environmental flows assessment. There was a large time commitment required from the various stakeholders, and having true influence over the process and project outcomes was important for sustaining their involvement. Such an involved process requires commitment over an extended period. This is challenged by not only by available resources, but also by continuity of stakeholder engagement. Even within the duration of this project, a number of participants changed roles and new members joined in their place. Participatory environmental flows assessment needs to be able to accommodate such changes.
3) Allowing adequate time to discuss objectives for the river can improve the overall project outcomes and legitimacy (Mussehl et al., 2022). The environmental flow assessment in the Kaiela included extensive discussions of objectives. This constituted a major component of the project, and one that is often glossed over in such studies. The discussions on objectives and the decision-making process filtered through the entire project. The flow objectives identified for the Kaiela are based on the values and outcomes identified by the local community and the GBCMA. This local engagement is important for building legitimacy and ensuring the environmental flow recommendations align with the objectives of those that live near and are sustained by the river (Acreman et al., 2017; Conallin et al., 2017).
4) Mechanistic models aimed at decision making provided multiple benefits to the project. The information produced in the environmental flows assessment, including the ecological models, were aimed at supporting decision making on an ongoing basis and the short-term adaptive management of environmental water (Horne et al., 2018). This focus on decision making allows scientists to shift away from building the “perfect model”, to instead construct models that represent our current understanding of how the ecosystem will respond to flow. Within these models, there are aspects that will be well understood and for which we have significant data, and other aspects that remain a hypothesis or supported by anecdotal information only. These models can be thought of as “living models” that get updated each year as river managers learn from decisions made and knowledge gained in previous years. The process of developing the models using expert elicitation and data highlighted areas of key uncertainty. It also provided a learning experience through the participatory approach, allowing stakeholders to engage in the science and inform the process with other sources of knowledge (Mussehl et al., 2022).
5) There remains a disconnect between environmental flows assessments, the models used to inform these assessments and the design of monitoring programs (Horne et al., 2017d). The documentation of clear ecological models provides the potential to link to monitoring and research, and to refine the knowledge base through time. This is a core element of adaptive management. However, in the Kaiela flows study, the scope of the project did not extend to design of a monitoring program to reduce aleatory uncertainty, or a research program to reduce epistemic uncertainty. This link between the environmental flows assessment (and the ecological models that underpin it) with monitoring and research remains a key gap that needs to be addressed before the benefits of the adaptive management cycle can be properly realised. A key aim of adaptive management is the ability to respond to changing information, values, and environments (Holling, 1978). The documentation of clear fundamental and means objectives, along with clearly detailed ecological models, provides the basis for this continual learning and updating with new knowledge (Horne et al., 2018). The nodes and links in the ecological models that are most uncertain (identified through the expert elicitation process), but which also have the most significant impact on the ecological outcome (identified through the sensitivity analysis), are those that should be the focus of future research. A key challenge for environmental flow programs going forward is how to explicitly link the models and tools used in the environmental flow assessment to the design and implementation of monitoring programs. Addressing context specific knowledge gaps present in reach-level environmental flows assessments will require responsive monitoring strategies that evolve in conjunction with environmental flows management.
6) Defining project boundaries in large river basins in challenging and has implications for decision making in environmental flows assessments. As is often the case, the environmental flows assessment was undertaken for a single river catchment. However, there is also a role for the Kaiela to contribute to downstream values and health of the larger Murray Darling Basin. This link was not explored through the flow study. However, it may be that in some environmental flows assessments of smaller rivers, it is worth explicitly bringing this type of basin-scale perspective into the discussion of objectives. This would also change the make-up of stakeholders involved in the process. Perhaps the key is the extent to which including consideration of the downstream systems has implications for decision making in the river catchment being examined.
7) Implementing a communication strategy is an essential—but often neglected or disconnected—step for environmental flow management. The use of a participatory approach builds engagement, transparency and knowledge exchange for those stakeholders involved in the process. However a structured and considered approach to broader community engagement and communication is required to ensure that there is wider support and legitimacy for environmental flows programs. There is a significant challenge within this process around communication of uncertainty and climate change risk.
This paper has presented an environmental flow assessment approach to meet the needs to managing environmental water under climate change and uncertainty. The approach is centred on adaptive management—a concept often discussed in the context of environmental flows, but rarely implemented and with little guidance for doing so (Webb et al., 2017). We raise five key considerations for environmental flow assessments under change and uncertainty 1) acknowledgement of uncertainties 2) Stakeholder engagement 3) Multiple sources of knowledge 4) Modelling that supports trade offs and change and 5) links to monitoring. While we have presented a proposed environmental flow assessment approach that addresses these five key considerations, we are aware there is a plethora of existing environmental flows methods. While many of these previous methods include the concept of adaptive management, there is little or no existing discussion of what is required to facilitate this adaptive management loop working in a non-stationary environment. The challenge of addressing non-stationarity in this context is common across NRM (Mussehl et al., 2022). Where existing methods are well embedded in practice, we suggest considering implementation of these methods in the context of the five key considerations for management under change and uncertainty.
In many river systems, the environment is the first component to be impacted due to climate change because of the way in which water is allocated (Horne et al., 2017b; Prosser et al., 2021). The use of scenarios within the adaptive management framework helps provide information for stakeholders to respond with management strategies through time. Presumptive methods that set minimum allowable deviations will still be required where resources do not allow for this level of detail (Richter et al., 2012). However, even in the case of these presumptive methods, consideration needs to be given as to how the system operation can adapt to accommodate uncertain futures.
Data Availability Statement
The original contributions presented in the study are included in the article/Supplementary Material, further inquiries can be directed to the corresponding author.
Author Contributions
AH lead the Kaiela environmental flows study and wrote the draft manuscript. DL, LR, JW, KF, AJ and MM were all involved in developing the method for the Kaiela flows study. LP, DL, LR, JW, KF, AJ and MM all provided input to the core manuscript ideas and helped with drafting the manuscript.
Funding
KF, AJ and MM were funded through the ARC Linkage Project LP170100598. AH was funded through ARC DE180100500.
Conflict of Interest
The authors declare that the research was conducted in the absence of any commercial or financial relationships that could be construed as a potential conflict of interest.
Publisher’s Note
All claims expressed in this article are solely those of the authors and do not necessarily represent those of their affiliated organizations, or those of the publisher, the editors and the reviewers. Any product that may be evaluated in this article, or claim that may be made by its manufacturer, is not guaranteed or endorsed by the publisher.
Acknowledgments
The Kaiela environmental flows assessment was funded by the Goulburn Broken Catchment Management Authority. As a participatory process, the GBCMA and stakeholders involved had a significant influence over the direction and outputs of the flows assessment. We thank them all for their time and thoughtful contributions to the process.
Supplementary Material
The Supplementary Material for this article can be found online at: https://www.frontiersin.org/articles/10.3389/fenvs.2022.808943/full#supplementary-material.
References
Acreman, M., Arthington, A. H., Colloff, M. J., Couch, C., Crossman, N. D., Dyer, F., et al. (2014a). Environmental Flows for Natural, Hybrid, and Novel Riverine Ecosystems in a Changing World. Front. Ecol. Environ. 12, 466–473. doi:10.1890/130134
Acreman, M. C., Overton, I. C., King, J., Wood, P. J., Cowx, I. G., Dunbar, M. J., et al. (2014b). The Changing Role of Ecohydrological Science in Guiding Environmental Flows. Hydrological Sci. J. 59, 433–450. doi:10.1080/02626667.2014.886019
Acreman, M., Jain, S. K., Mccartney, M. P., and Overton, I. (2017). “Chapter 2 - Drivers and Social Context,” in Water for the Environment (London, UK: Academic Press). doi:10.1016/B978-0-12-803907-6.00028-0
Allan, C., and Watts, R. J. (2018). Revealing Adaptive Management of Environmental Flows. Environ. Manage. 61, 520–533. doi:10.1007/s00267-017-0931-3
Allen, C. R., Fontaine, J. J., Pope, K. L., and Garmestani, A. S. (2011). Adaptive Management for a Turbulent Future. J. Environ. Manage. 92, 1339–1345. doi:10.1016/j.jenvman.2010.11.019
Allen, C. R., and Garmestani, A. S. (2015). “Adaptive Management,” in Adaptive Management of Social-Ecological Systems. Editors C. R. Allen, and A. S. Garmestani (Springer Science). doi:10.1007/978-94-017-9682-8_1
Anderson, E. P., Jackson, S., Tharme, R. E., Douglas, M., Flotemersch, J. E., Zwarteveen, M., et al. (2019). Understanding Rivers and Their Social Relations: A Critical Step to advance Environmental Water Management. WIREs Water 6, e1381. doi:10.1002/wat2.1381
Arthington, A. H., Bhaduri, A., Bunn, S. E., Jackson, S., Tharme, R. E., Tickner, D., et al. (2019). The Brisbane Declaration and Global Action Agenda on Environmental Flows (2018). Front. Environ. Sci. 6. doi:10.3389/fenvs.2018.00045
Bellard, C., Bertelsmeier, C., Leadley, P., Thuiller, W., and Courchamp, F. (2012). Impacts of Climate Change on the Future of Biodiversity. Ecol. Lett. 15, 365–377. doi:10.1111/j.1461-0248.2011.01736.x
Bestgen, K. R., Poff, N. L., Baker, D. W., Bledsoe, B. P., Merritt, D. M., Lorie, M., et al. (2020). Designing Flows to Enhance Ecosystem Functioning in Heavily Altered Rivers. Ecol. Appl. 30, e02005. doi:10.1002/eap.2005
Beven, K. (2016). Facets of Uncertainty: Epistemic Uncertainty, Non-stationarity, Likelihood, Hypothesis Testing, and Communication. Hydrological Sci. J. 61, 1652–1665. doi:10.1080/02626667.2015.1031761
Boltz, F., Leroy Poff, N., Folke, C., Kete, N., Brown, C. M., St. George Freeman, S., et al. (2019). Water Is a Master Variable: Solving for Resilience in the Modern Era. Water Security 8, 100048. doi:10.1016/j.wasec.2019.100048
Brown, C. (2012). Decision-scaling for Robust Planning and Policy under Climate Uncertainty. Washington DC: World Resources Report.
Burton, P., and Mustelin, J. (2013). Planning for Climate Change: Is Greater Public Participation the Key to Success? Urban Pol. Res. 31, 399–415. doi:10.1080/08111146.2013.778196
Colloff, M. J., and Pittock, J. (2019). Why We Disagree about the Murray–Darling Basin Plan: Water Reform, Environmental Knowledge and the Science-Policy Decision Context. Australas. J. Water Resour. 23, 88–98. doi:10.1080/13241583.2019.1664878
Conallin, J., Campbell, J., and Baumgartner, L. (2018). Using Strategic Adaptive Management to Facilitate Implementation of Environmental Flow Programs in Complex Social-Ecological Systems. Environ. Manage. 62, 955–967. doi:10.1007/s00267-018-1091-9
Conallin, J. C., Dickens, C., Hearne, D., and Allan, C. (2017). “Chapter 7 - Stakeholder Engagement in Environmental Water Management,” in Water for the Environment. Editors A. C Horne, J. A. Webb, M. J. Stewardson, B. Richter, and M. Acreman (London, UK: Academic Press). doi:10.1016/B978-0-12-803907-6.00028-0
C. S. Holling (Editor) (1978). Adaptive Environmental Assessment and Management (Chichester, UK: Wiley).
de Little, S. C., Casas-Mulet, R., Patulny, L., Wand, J., Miller, K. A., Fiddler, F., et al. (2018). Minimising Biases in Expert Elicitations to Inform Environmental Management: Case Studies from Environmental Flows in Australia. Environ. Model. Softw. 100, 146–158. doi:10.1016/j.envsoft.2017.11.020
Doolan, J., Ashworth, B., and Swirepik, J. (2017). “Planning the Use of Environmental Water,” in Water for the Environment. Editors A. Horne, J. A. Webb, M. Stewardson, M. Acreman, and B. D. Richter (London, UK: Academic Press). doi:10.1016/B978-0-12-803907-6.00028-0
Fowler, K., Ballis, N., Horne Avril, C., John, A., Nathan, R., and Peel, M. (2022). Integrated Framework for Rapid Climate Stress Testing on a Monthly Timestep. Environ. Model. Software 150, 105339. doi:10.1016/j.envsoft.2022.105339
Fujitani, M., Mcfall, A., Randler, C., and Arlinghaus, R. (2017). Participatory Adaptive Management Leads to Environmental Learning Outcomes Extending beyond the Sphere of Science. Sci. Adv. 3, e1602516. doi:10.1126/sciadv.1602516
Gawne, B., Brooks, S., Butcher, R., Cottingham, P., Everingham, R., Hale, J., et al. (2020). Long Term Intervention Monitoring Project Monitoring and Evaluation Requirements Goulburn River for Commonwealth Environmental Water. Final Report prepared for the Commonwealth Environmental Water Office by the Murray-Darling Freshwater Research Centre.
Gawne, B., Hale, J., Stewardson, M. J., Webb, J. A., Ryder, D. S., Brooks, S. S., et al. (2020). Monitoring of Environmental Flow Outcomes in a Large River basin: The Commonwealth Environmental Water Holder's Long-Term Intervention in the Murray–Darling Basin, Australia. River Res. Appl. 36, 630–644. doi:10.1002/rra.3504
Gawne, B., Ryan, K. A., Coleman, M., Meehan, A., Davies, P. E., Sluggett, A., et al. (2021). “Chapter 11 - Monitoring, Evaluation, and Adaptive Management in the Murray–Darling Basin,” in Murray-darling Basin, Australia. Editors B. T. Hart, N. R. Bond, N. Byron, C. A. Pollino, and M. J. Stewardson (Elsevier). doi:10.1016/B978-0-12-818152-2.00011-5
Godden, L., and Ison, R. (2019). Community Participation: Exploring Legitimacy in Socio-Ecological Systems for Environmental Water Governance. Australas. J. Water Resour. 23, 45–57. doi:10.1080/13241583.2019.1608688
Gregory, R., Ohlson, D., and Arvai, J. (2006). Deconstructing Adaptive Management: Criteria for Applications to Environmental Management. Ecol. Appl. 16, 2411–2425. doi:10.1890/1051-0761(2006)016[2411:damcfa]2.0.co;2
Gregory, R., Failing, L., Harstone, G., Long, G., McDaniels, T., and Ohlson, D. (2012). Structured Decision Making: A Practical Guide to Environmental Management Choices John Wiley & Sons, 2411.
Grose, M. R., Narsey, S., Delage, F. P., Dowdy, A. J., Bador, M., Boschat, G., et al. (2020). Insights from CMIP6 for Australia's Future Climate. Earth's Future 8, e2019EF001469. doi:10.1029/2019ef001469
Hanea, A. M., Mcbride, M. F., Burgman, M. A., Wintle, B. C., Fidler, F., Flander, L., et al. (2017). I Nvestigate D Iscuss E Stimate A Ggregate for Structured Expert Judgement. Int. J. Forecast. 33, 267–279. doi:10.1016/j.ijforecast.2016.02.008
Haraway, D. (1988). Situated Knowledges: The Science Question in Feminism and the Privilege of Partial Perspective. Feminist Stud. 14, 575–599. doi:10.2307/3178066
Horne, A. C., Nathan, R., Poff, N. L., Bond, N. R., Webb, J. A., Wang, J., et al. (2019). Modeling Flow-Ecology Responses in the Anthropocene: Challenges for Sustainable Riverine Management. BioScience 69, 789–799. doi:10.1093/biosci/biz087
Horne, A. C., O’Donnell, E. L., and Tharme, R. E. (2017b). “Chapter 17 - Mechanisms to Allocate Environmental Water,” in Water for the Environment (London, UK: Academic Press). doi:10.1016/B978-0-12-803907-6.00028-0
Horne, A. C., Szemis, J. M., Webb, J. A., Kaur, S., Stewardson, M. J., Bond, N., et al. (2017c). Informing Environmental Water Management Decisions: Using Conditional Probability Networks to Address the Information Needs of Planning and Implementation Cycles. Environ. Manage. 61 (3), 347–357. doi:10.1007/s00267-017-0874-8
Horne, A. C., Szemis, J. M., Webb, J. A., Kaur, S., Stewardson, M. J., Bond, N., et al. (2018). Informing Environmental Water Management Decisions: Using Conditional Probability Networks to Address the Information Needs of Planning and Implementation Cycles. Environ. Manage. 61, 347–357. doi:10.1007/s00267-017-0874-8
Horne, A. C., Webb, J. A., O'Donnell, E., Arthington, A. H., Mcclain, M., Bond, N., et al. (2017d). Research Priorities to Improve Future Environmental Water Outcomes. Front. Environ. Sci. 5. doi:10.3389/fenvs.2017.00089
Horne, A., O'Donnell, E., Webb, J., Stewardson, M., Acreman, M., and Richter, B. D. (2017a). “The Environmental Water Management Cycle,” in Water for the Environment: From Policy and Science to Implementation and Management. Editors A. Horne, J. Webb, M. Stewardson, M. Acreman, and B. D. Richter (Cambridge, MA, USA: Elsevier). doi:10.1016/b978-0-12-803907-6.00001-2
John, A., Horne, A., Nathan, R., Fowler, K., Webb, J. A., et al. (2021a). Robust Climate Change Adaptation for Environmental Flows in the Goulburn River, Australia. Front. Environ. Sci. 9. doi:10.3389/fenvs.2021.789206
John, A., Horne, A., Nathan, R., Stewardson, M., Webb, J. A., Wang, J., et al. (2021b). Climate Change and Freshwater Ecology: Hydrological and Ecological Methods of Comparable Complexity Are Needed to Predict Risk. WIREs Clim. Change 12, e692. doi:10.1002/wcc.692
Kingsford, R. T., Biggs, H. C., and Pollard, S. R. (2011). Strategic Adaptive Management in Freshwater Protected Areas and Their Rivers. Biol. Conservation 144, 1194–1203. doi:10.1016/j.biocon.2010.09.022
Kosovac, A., Davidson, B., and Malano, H. (2019). Are We Objective? A Study into the Effectiveness of Risk Measurement in the Water Industry. Sustainability 11, 1279. doi:10.3390/su11051279
Le Quesne, T., Kendy, E., and Weston, D. (2010). The Implementation challenge - Taking Stock of Government Policies to Protect and Restore Environmental Flows. The Nature Conservancy and WWF. https://wwfint.awsassets.panda.org/downloads/the_implementation_challenge.pdf.
Mussehl, M., Horne, A. C., Webb, J. A., and Poff, L. N. (2022). Purposeful Stakeholder Engagement for Improved Environmental Flow Outcomes. Front. Environ. Sci. 9. doi:10.3389/fenvs.2021.749864
Nathan, R. J., McMahon, T. A., Peel, M. C., and Horne, A. (2019). Assessing the Degree of Hydrologic Stress due to Climate Change. Clim. Change 156, 87–104. doi:10.1007/s10584-019-02497-4
O’Donnell, E. L., Horne, A. C., Godden, L., and Head, B. (2019). Cry Me a River: Building Trust and Maintaining Legitimacy in Environmental Flows. Australas. J. Water Resour. 23, 1–13. doi:10.1080/13241583.2019.1586058
Pahl-Wostl, C., Arthington, A., Bogardi, J., Bunn, S. E., Hoff, H., Lebel, L., et al. (2013). Environmental Flows and Water Governance: Managing Sustainable Water Uses. Curr. Opin. Environ. Sustainability 5, 341–351. doi:10.1016/j.cosust.2013.06.009
Pahl-Wostl, C., Sendzimir, J., Jeffrey, P., Aerts, J., Berkamp, G., and Cross, K. (2007). Managing Change toward Adaptive Water Management through Social Learning. Ecol. Soc. 12. doi:10.5751/es-02147-120230
Poff, L., Arthington, A., and Tharme, R. (2017). “Evolution of Environmental Flows Assessment Science, Principles and Methodologies,” in Water for the Environment: From Policy and Science to Implementation and Management. Editors A. Horne, J. A. Webb, M. Stewardson, B. Richter, and M. Acreman. 1 ed. (Cambridge, MA: Academic Press). doi:10.1016/b978-0-12-803907-6.00011-5
Poff, L. (2017). Beyond the Natural Flow Regime? Broadening the Hydro-Ecological Foundation of Environmental Flows Sciencein a Nonstationary World. Freshw. Biol. 63 (8), 1011–1021. doi:10.1111/fwb.13038
Poff, N. L., Allan, J. D., Palmer, M. A., Hart, D. D., Richter, B. D., Arthington, A. H., et al. (2003). River Flows and Water Wars: Emerging Science for Environmental Decision Making. Front. Ecol. Environ. 1, 298–306. doi:10.1890/1540-9295(2003)001[0298:rfawwe]2.0.co;2
Poff, N. L. (2018). Beyond the Natural Flow Regime? Broadening the Hydro-Ecological Foundation to Meet Environmental Flows Challenges in a Non-stationary World. Freshw. Biol. 63, 1011–1021. doi:10.1111/fwb.13038
Poff, N. L., Richter, B. D., Arthington, A. H., Bunn, S. E., Naiman, R. J., Kendy, E., et al. (2010). The Ecological Limits of Hydrologic Alteration (ELOHA): a New Framework for Developing Regional Environmental Flow Standards. Freshw. Biol. 55, 147–170. doi:10.1111/j.1365-2427.2009.02204.x
Prosser, I. P., Chiew, F. H. S., and Stafford Smith, M. (2021). Adapting Water Management to Climate Change in the Murray–Darling Basin, Australia. Water 13, 2504. doi:10.3390/w13182504
Raymond, C. M., Fazey, I., Reed, M. S., Stringer, L. C., Robinson, G. M., and Evely, A. C. (2010). Integrating Local and Scientific Knowledge for Environmental Management. J. Environ. Manage. 91, 1766–1777. doi:10.1016/j.jenvman.2010.03.023
Richter, B. D., Davis, M. M., Apse, C., and Konrad, C. (2012). A Presumptive Standard for Environmental Flow Protection. River Res. Appl. 28, 1312–1321. doi:10.1002/rra.1511
Rist, L., Felton, A., Samuelsson, L., Sandström, C., and Rosvall, O. (2013). A New Paradigm for Adaptive Management. Ecol. Soc. 18, 63–72. doi:10.5751/es-06183-180463
Rosendahl, J., Zanella, M. A., Rist, S., and Weigelt, J. (2015). Scientists’ Situated Knowledge: Strong Objectivity in Transdisciplinarity. Futures 65, 17–27. doi:10.1016/j.futures.2014.10.011
Roux, D. J., and Foxcroft, L. C. (2011). The Development and Application of Strategic Adaptive Management within South African National Parks. Koedoe : Afr. Protected Area Conservation Sci. 53, 1–5. doi:10.4102/koedoe.v53i2.1049
Stein, E. D., Zimmerman, J., Yarnell, S. M., Stanford, B., Lane, B., Taniguchi-Quan, K. T., et al. (2021). The California Environmental Flows Framework: Meeting the Challenges of Developing a Large-Scale Environmental Flows Program. Front. Environ. Sci. 9. doi:10.3389/fenvs.2021.769943
Stewardson, M., and Rutherfurd, I. (2008). “Conceptual and Mathematical Modelling in River Restoration: Do We Have Unreasonable Confidence?,” in River Restoration. West Sussex, England. doi:10.1002/9780470867082.ch5
Tharme, R. E. (2003). A Global Perspective on Environmental Flow Assessment: Emerging Trends in the Development and Application of Environmental Flow Methodologies for Rivers. River Res. Appl. 19, 397–441. doi:10.1002/rra.736
Tompkins, E. L., and Adger, W. N. (2004). Does Adaptive Management of Natural Resources Enhance Resilience to Climate Change? Ecol. Soc. 9, 10–24. doi:10.5751/es-00667-090210
Tonkin, J. D., Leroy Poff, N., Nr, B., Horne Avril, C., Merritt, D. M., Reynoldsolden, J. D., et al. (2019). Prepare River Ecosystems for an Uncertain Future. Nature 570, 301–303. doi:10.1038/d41586-019-01877-1
Treadwell, S., Webb, J. A., Hou, X., Baker, B., Casanelia, S., Grace, M. R., et al. (2021). 2019-20 Goulburn MER Annual Summary Report. Melbourne: The University of Melbourne.
Voinov, A., and Gaddis, E. J. B. (2008). Lessons for Successful Participatory Watershed Modeling: A Perspective from Modeling Practitioners. Ecol. Model. 216, 197–207. doi:10.1016/j.ecolmodel.2008.03.010
Voinov, A., Kolagani, N., Mccall, M. K., Glynn, P. D., Kragt, M. E., Ostermann, F. O., et al. (2016). Modelling with Stakeholders – Next Generation. Environ. Model. Softw. 77, 196–220. doi:10.1016/j.envsoft.2015.11.016
Webb, A., Treadwell, S., Lakhanpal, G., Baker, B., Casanelia, S., Grace, M., et al. (2019). Lower Goulburn River Selected Area Monitoring, Evaluation and Research Plan (2019-2022). Parkville: The University of Melbournee. Prepared for the Commonwealth Environmental Water Offic.
Webb, J. A., de Little, S. C., Miller, K. A., and Stewardson, M. J. (2018). Quantifying and Predicting the Benefits of Environmental Flows: Combining Large-Scale Monitoring Data and Expert Knowledge within Hierarchical Bayesian Models. Freshw. Biol. 63, 831–843. doi:10.1111/fwb.13069
Webb, J. A., Stewardson, M. J., Chee, Y. E., Schreiber, E. S. G., Sharpe, A. K., and Jensz, M. C. (2010). Negotiating the Turbulent Boundary: the Challenges of Building a Sciencemanagement Collaboration for Landscape-Scale Monitoring of Environmental Flows. Mar. Freshw. Res. 61, 798–807. doi:10.1071/mf09059
Webb, J. A., Watts, R. J., Allan, C. A., and Warner, A. (2017). “Principles for Monitoring, Evaluation and Adaptive Management of Environmental Water Regimes,” in Water for the Environment: From Policy and Science to Implementation and Management. Editors A. Horne, J. A. Webb, M. Stewardson, M. Acreman, and B. D. Richter (London, UK: Academic Press). doi:10.1016/B978-0-12-803907-6.00028-0
Williams, B. K. (2011). Adaptive Management of Natural Resources-Fframework and Issues. J. Environ. Manage. 92, 1346–1353. doi:10.1016/j.jenvman.2010.10.041
Williams, B. K., and Brown, E. D. (2014). Adaptive Management: From More Talk to Real Action. Environ. Manage. 53, 465–479. doi:10.1007/s00267-013-0205-7
Keywords: environmental flows, uncertainty, adaptive management, non-stationarity, eflows
Citation: Horne AC, Webb JA, Mussehl M, John A, Rumpff L, Fowler K, Lovell D and Poff L (2022) Not Just Another Assessment Method: Reimagining Environmental Flows Assessments in the Face of Uncertainty. Front. Environ. Sci. 10:808943. doi: 10.3389/fenvs.2022.808943
Received: 04 November 2021; Accepted: 31 March 2022;
Published: 10 May 2022.
Edited by:
David Tickner, World Wide Fund for Nature, United KingdomReviewed by:
Sarah Yarnell, University of California, Davis, United StatesSergio A. Salinas-Rodríguez, The Southern Border College (ECOSUR), Villahermosa, Mexico
Copyright © 2022 Horne, Webb, Mussehl, John, Rumpff, Fowler, Lovell and Poff. This is an open-access article distributed under the terms of the Creative Commons Attribution License (CC BY). The use, distribution or reproduction in other forums is permitted, provided the original author(s) and the copyright owner(s) are credited and that the original publication in this journal is cited, in accordance with accepted academic practice. No use, distribution or reproduction is permitted which does not comply with these terms.
*Correspondence: Avril C. Horne, YXZyaWwuaG9ybmVAdW5pbWVsYi5lZHUuYXU=