- 1Science and Decisions Center, U.S. Geological Survey, Reston, VA, United States
- 2Arizona State University, Consortium for Science, Policy and Outcomes, Washington DC, United States
- 3Engineering Laboratory, Applied Economics Office, National Institute of Standards and Technology, Gaithersburg, MD, United States
- 4National Land Imaging Program and Social and Economic Analysis Branch, Fort Collins Science Center, U.S. Geological Survey, Fort Collins, CO, United States
Information used in decision making arises from the structuring of observations and data. The collection, dissemination, and use of information has monetary and non-monetary costs (e.g., competition for attention) and necessitates trade-offs. Understanding the benefits of having information (i.e., the value of information, VOI), including resulting societal outcomes, is useful to information producers/funders and decision makers. Using theory, use cases, and hypotheticals, we describe how information (e.g., geospatial information) is valued and incorporated in decisions and actions related to managing natural resources, environments, and the impacts of natural and anthropogenic hazards. We discuss the nature of information and how it relates to models (conceptual, mental, scientific), beliefs, knowledge, and economic analyses. VOI approaches and behavioral factors that potentially affect information use and value are summarized. Framing of information and VOI through data to decision pathways (DDPs) at first simplifies understanding, then illustrates the benefits of information, and the human and societal challenges encountered in valuing and using it. We present approaches to overcome these challenges. Our transdisciplinary analysis concludes with a summary of critical issues affecting DDPs and VOI, and suggestions for improving both economic analyses and the actionability and use of information.
1 Introduction
Much of humanity now exists in a knowledge economy (Powell and Snellman, 2004) in which there is an ever-increasing demand for information, and for its applicability to perceived needs. This demand is propelled by society’s growing reliance on digital information made rapidly available to a widening range of people. The growing volume and accessibility to information indisputably offers benefits to society. It can also be critical to policymakers and managers who rely on accurate information to make decisions and engage in actions that influence many people. The collection, management, and use of information has associated costs, as does making it available to a broad consumer base. These costs often fall on government agencies or are distributed across a wide range of funding sources (Kitchin et al., 2015; Kitchin and Lauriault, 2015; World Bank Group, 2016). Given limited resources, understanding the potential or realized benefits resulting from the use of certain information can illuminate its utility, including in the digital economy (Wilson, 2015; Goldfarb and Tucker, 2019; Laybats and Tredinnick, 2020). Economists refer to such benefits as the value of information (VOI), which can be quantitative or qualitative in nature (Keisler et al., 2014; Molder et al., 2022). Although VOI studies (Howard, 1966; Raiffa, 1970) can be extremely useful for elucidating how information changed or could change decisions and resulting actions, they may fail to incorporate a full understanding of the behavioral and societal factors affecting the value and use of particular information (Glynn et al., 2022, this issue).
What matters to people and society is subjective, differs among people, and may differ from what people and society should perhaps more objectively, or more explicitly and consciously, consider (Bacon, 1620; Kahneman, 2011). Such realities influence the “value” and use of information, and should be examined to the extent possible in VOI assessments and in any type of economic or other scientific studies that evaluate or point to the value of information. Additionally, although what matters should be reflected in the stated purpose(s) of each study, these aims may be more aligned with what scientists perceive or assume matters, than what matters to people and society more broadly – either what people may perceive to matter, or what may more truly matter. Individual and group biases (including cognitive and perception biases), beliefs, heuristics (i.e., shortcuts and ways of thinking), and values (including cultural and moral norms) (BBHV) all affect human judgments about what matters (cf. discussion and references in Glynn et al., 2022, this issue). BBHV and other behavioral and social factors mentioned in this paper affect everyone: information generators, consumers, funders, evaluators, decision makers, and even the most objective economists and scientists (Glynn, 2014; Glynn, 2017; Glynn et al., 2017; Glynn et al., 2018). Improving incorporation of these factors in VOI studies could provide a more holistic assessment of how and to what degree information influences decisions and resulting actions.
Information’s role in decision making fits into a progressive process that we term a data to decision pathway (DDP). At its most basic level, a DDP involves progressing from data and observations to decision making and action (Figure 1). Distinguishing among data, information, and knowledge are essential (Glynn et al., 2017) to understanding this progression. Data are formal or informal observations (perceived through human or machine sensing) that may have been transformed, structured, and documented to allow understanding. Data can have uncertainties and assumptions associated with their characterization or representation; and data that have been transformed or structured may be associated with additional uncertainties, assumptions, simplifications, or models of all types (conceptual, mental, scientific). Hereafter, the term models, unless otherwise specified, refers to models (i.e., representations) of all types, whether innately held, explicitly articulated, or developed through scientific practice. Information is produced when data, possibly of many different types, are picked, organized, structured, appropriately documented, and something is produced that we sense or realize can be useful. Knowledge builds on information and comes in two basic forms. Tacit knowledge is a form of knowledge usually acquired by doing – without continuing conscious reflection or explicit deciphering of observations and causalities, and therefore without conscious explicit reference to information (Polanyi, 1962). A more reflective, explicit, form of knowledge includes scientific knowledge. In this specific form – which we believe should be the focus of most economic analyses and scientific models – having knowledge means “knowing” something is true and having a justified belief in its truth (Plato 369 BCE; Ichikawa and Steup, 2014). Obtaining knowledge of this type means that (Glynn et al., 2017)
“…information becomes “internalized,” that an alignment occurs between preexisting beliefs and information. Old beliefs become reinforced or, more rarely, new beliefs emerge. Knowledge development is also often linked to simplifications and abstractions of available data and information. More importantly, having knowledge at the level of an individual or at the level of a community means that decisions may now be made.”
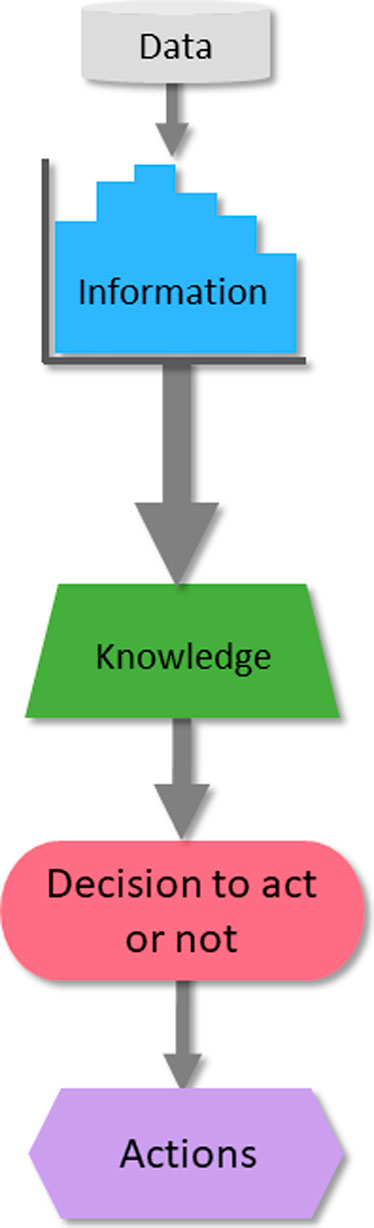
FIGURE 1. Basic data to decisions pathway (DDP), showing the transitions from data to information to knowledge and to decisions, and possibly to actions. The changing width of arrows correlates with a likelihood for each transition (qualitatively perceived by the authors). In reality, the transitions illustrated may not occur, for explicitly stated reasons (such as a decision not to act), or for other reasons.
We use the word “decisions” generally to imply that an actor (an individual, institution, or collectivity) or a group of actors decides to act (or not act) based on acquired and/or newly generated knowledge. We use “action” only when the nature or consequence of a specific action is relevant.
Efforts to understand the value certain information adds to a DDP can take two forms (Graham-Tomasi, 1988; Gardner et al., 1993; Hashemi et al., 2019). One form, an ex ante VOI study, seeks to estimate the potential benefits or VOI prior to any decisions made with the information. Ex ante studies, more generally, may consider and seek to predict 1) which information should be produced through data-gathering, study, or experimentation, 2) where to devote extra resources for dissemination of the data or information, and 3) the potential value of specific types of information to improve decision-making. Ex ante studies can also evaluate hypothetical outcomes from the use of information to determine to what degree it will change decisions and actions. The second form, an ex post VOI study, examines the benefits and costs of decisions or policies after they have been implemented. For example, researchers may investigate what information and associated knowledge proved to be most useful within the initial set of decisions, or as part of a continuing effort to interactively assess and manage a given issue. Ex post analyses conducted outside the field of economics are sometimes called post-audits and are rarely undertaken (Konikow and Bredehoeft, 1992; Bredehoeft and Konikow, 2012; Nordstrom, 2012) because 1) clear points of comparison or reference are often not available and 2) incentives to conduct such studies are limited, especially if they might highlight failures, rather than positive benefits (Tomlinson and Atkinson, 1987; Dipper et al., 1998).
Regardless of whether they are ex ante or ex post analyses, assessing VOI is critical for improving decisions and actions across different scales of community, from individuals to institutions and collectivities, and for improving and prioritizing societal investments in the production and use of information. The types of economic studies that we focus on in this paper are those related to the management of natural resources, environments, and anthropogenic and natural hazards. The use of geospatial information is a particular area of interest. All studies we focus on are shaped by at least three critical components (that may encompass additional characterizations):
1) A driving purpose for the given analysis or model. Ideally, some quantitative or qualitative measures of progress toward achieving the purpose are also provided.
2) Data, information, or knowledge used to conduct the analysis or to construct and use the model. This includes providing a definition and analysis of baseline or reference conditions where data, information, or knowledge are unavailable.
3) Some determination or assumptions about what matters to people and to society, and what they value. In this regard, it may be useful to assess a) discounting and differences caused through the timing of benefits, costs, and impacts; and b) differential distributions of costs and benefits across different groups of payers and beneficiaries. Understanding who pays the costs, who receives the benefits, and externalities that might occur, is crucial.
A driving purpose for conducting a VOI study is usually present before any analysis, model construction, synthesis, or evaluation (or use) of information occurs. This may take the form of an express intention to improve an identified issue within society or the environment. Stating that purpose is important, as is identifying what follow-up studies will be supported through the effort, and what societal decisions and actions will be served. Nonetheless, it is important to recognize that multiple purposes are commonly served through use of the same information or knowledge, and that some useful purposes may become apparent only later.
1.1 Aims and Structure of This Article
This paper explores how information – and especially geospatial information – is valued and brought to bear in decisions and actions related to managing natural resources, environments, and the impacts of natural and anthropogenic hazards. The decisions and actions may be those of managers or policy makers, or those of individuals, or of other actors. We first discuss the nature and merging of information and beliefs, in relation to models and economic analyses that are potentially brought into societal decisions and actions. In doing so, we refer to some behavioral and social factors that affect the perception, processing, communication, and valuing of information, i.e., what people and society perceive to matter. [Glynn et al. (2022, this issue) provide a more detailed discussion of these behavioral and social factors]. We then provide examples of different valuations and uses of information, where we frame information and VOI in the context of DDPs. Our examples illustrate some of the benefits of information, some of the human and societal challenges encountered in valuing and using it, and possible ways to overcome these challenges. Lastly, we provide a summary of some critical issues affecting DDPs and VOI, while providing recommendations to improve economic analyses and the actionability and use of information in societal decisions and actions.
2 Information, Beliefs, and Models for Decisions
Models intersect with data and information in many ways. As mentioned previously (cf. also Glynn et al., 2022, this issue), the seeking and construction of information from data and facts implies human agency and some conscious or unconscious purpose(s) for possible use of the information. Models – as the term is used in this article – encompass the mental models, pre-existing beliefs, and conceptual models that are used, consciously or unconsciously, in this seeking and construction of information. In a more explicit form, models can also include conceptual or numerical models constructed in the pursuit of science. Beyond their use in the seeking and construction of information, models (conscious and unconscious) are also used to process information, transforming it first into knowledge, then applying it to decisions.
As we navigate through and manage our world(s), as individuals and as part of different collectivities, we create, learn, or appropriate mental, cultural, and conceptual models of all kinds. Those models are used, innately or consciously, to make evaluative judgments, to make decisions, and to take actions. As we learn or acquire these models, we have the opportunity to use observations and information to modify or test them. However, once we strongly anchor or habitually use these models, we generally do not question or critically examine them further: they become individual and/or collective beliefs (Harari, 2015). These beliefs help simplify our complex world(s), so that we can more efficiently manage ourselves and our communities. It is difficult to change deeply ingrained beliefs, even when they conflict with new information. It is human nature to ignore conflicting information and internal inconsistencies in our beliefs, and to select information that happens to support our beliefs (Anderson et al., 1980; Anderson, 1983; Fagin and Halpern, 1987; Ariely, 2010).
So how do these models and beliefs relate to information, its value, and to the mathematical and conceptual models created by scientists? The models that we create as researchers are highly influenced by our mental models and beliefs (Polanyi, 1962; Damasio, 1994; Glynn, 2017), but scientific models have the great advantage of being explicit (rather than innate or subconscious) representations. Scientific models can be tested, modified, and improved, not only by the model developers but also by others. Scientific models can be updated (or determined invalid) as new information is obtained. Because of their extrinsic nature, scientific models may also not be held quite as tightly or reflexively defended as human beliefs and more innate mental constructs.
The rigorous scientific approach that we hold as a standard is not a process hermetically shielded from human assumptions and biases. The studies that we conduct and that we report on as scientists and engineers (or in other professions) generally incorporate a combination of clear and explicit representations (e.g., documented scientific models), as well as a less-explicitly stated set of innately held assumptions, beliefs, and mental models. Similarly, the people with whom we interact (or whose values, actions, or decisions that we attempt to describe) during our studies also hold innate assumptions, beliefs, and mental models. Figuring out how information may (or may not) transform into decisions and actions can be complex and difficult to determine. The distinction that we raise between more innate and more explicit models parallels the distinction between what psychologists (e.g., Evans and Stanovich, 2013a; Evans and Stanovich, 2013b) and behavioral economists (e.g., Kahneman, 2011) have called System 1 thinking (fast and innate) and System 2 thinking (slow and more reflective, requiring more effort). Such dual process theories are in themselves a simplification that does not always recognize that System 1 processes often control, or are intertwined with, System 2 thinking (Damasio, 1994; Stanovich, 2010). Because it is more innate, System 1 thinking, and what has been termed fast and frugal heuristics (Gigerenzer, 2007), often offers well-adapted, fast and accurate responses to situations frequently or acutely experienced (Glynn et al., 2017).
Francis Bacon (Bacon, 1620) understood many of these issues when he discussed the “Idols of the Mind” (i.e., of the Tribe, of the Cave, of the Marketplace, and of the Theater). His description of the Idols of the Tribe relates particularly well to human perceptions and valuations of “what matters”:
The Idols of Tribe have their foundation in human nature itself, and in the tribe or race of men. For it is a false assertion that the sense of man is the measure of things. On the contrary, all perceptions as well of the sense as of the mind are according to the measure of the individual and not according to the measure of the universe. And the human understanding is like a false mirror, which, receiving rays irregularly, distorts and discolors the nature of things by mingling its own nature with it.
So, what can we say about the value of information (VOI)? Answering that question raises additional questions. Value for whom? Value to what purpose? Potential value or realized value? Perceived value or actual value? By actual value we mean an unperceived or less perceived value that is found only through critical thinking and reasoning about what truly matters. Can the two types of value be distinguished? Human beings will likely never be able to fully determine values reflective of what truly matters because the human grasp of reality and truths will always be subjective. Nonetheless, making the effort can improve understanding.
There are some clear differences between “perceived values” and “actual values” that can be explored, in addition to different types of information, values, and valuation methods. Indeed, human and societal behavioral factors affect the valuation of information, how information is consumed, how it is provided, and the methods used to assess VOI. Fifteen “Value Explorations” discuss these factors and methodologies in detail while providing illustrative examples (cf., Glynn et al., 2022, this issue). The Value Explorations (VEs) provide a foundation for the VOI and “Data to Decision Pathway” (DDP) discussions in this paper. A summary is provided here for quick reference (Table 1).
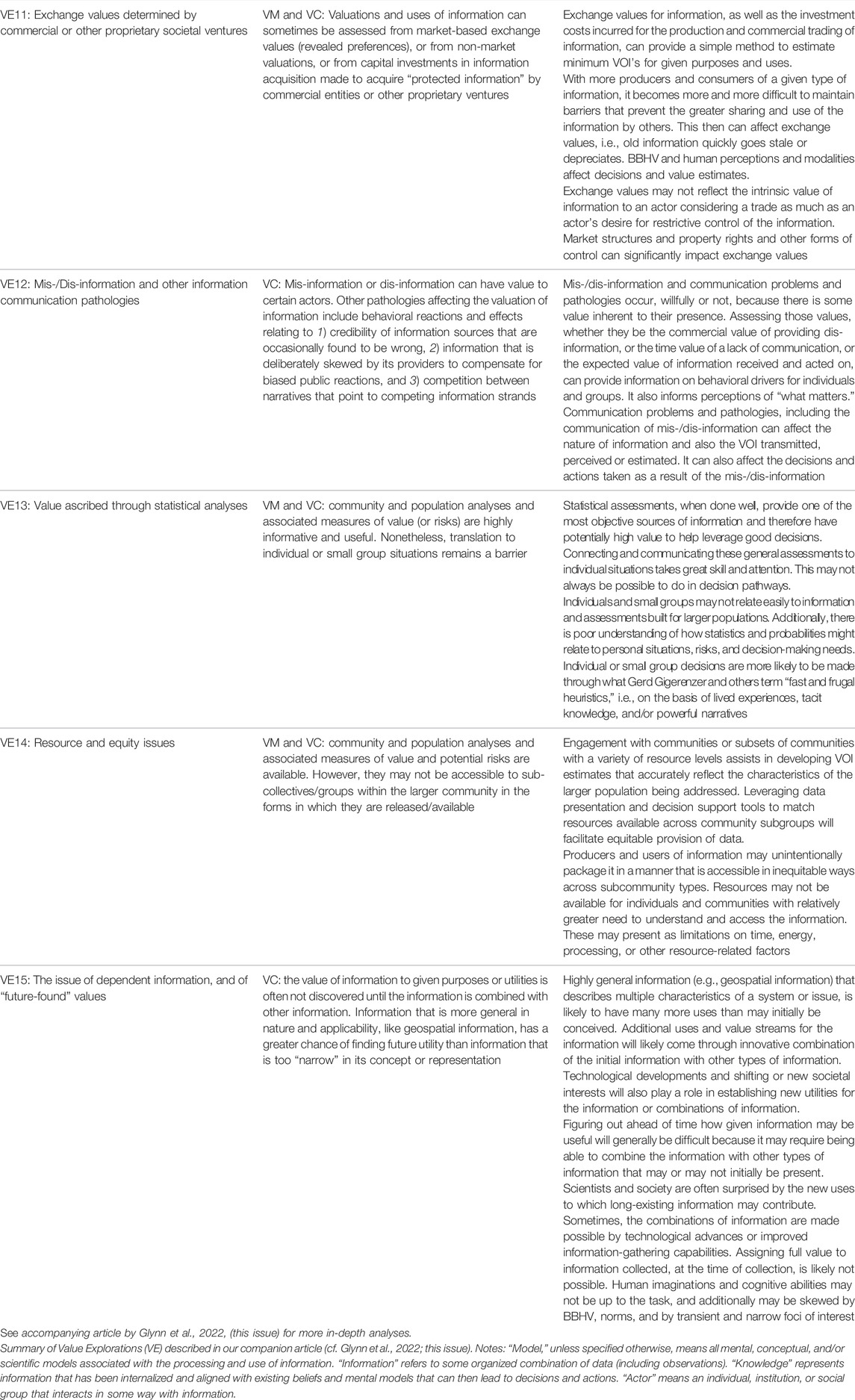
TABLE 1. Summary of 15 “Value Explorations” of VOI methodologies and of behavioral and social factors influencing information and VOI.
Our modern world offers access to a tremendous and ever-increasing amount of information. Availability and access to geospatial information is also increasing and is increasingly provided as a public good. In economics (Cowen and Henderson, 2022), a public good is a good that has both non-rivalrous and non-excludable consumption characteristics. Non-rivalrous consumption means that many users can benefit from the same good (e.g., information in this case) simultaneously, without one use or user diminishing use by another. Non-excludable means that users cannot be excluded from using the good even if they don’t pay for it.
Having access to an increasing amount of information, much of it available as a public good (Kaul et al., 1999), does not necessarily mean that the information is effectively and efficiently used by decision makers to address the complex issues faced by society. Indeed, the richness of available information (and mis- or dis-information) constantly competes for our attention. As Herbert Simon (Simon, 1971) said:
[I]n an information-rich world, the wealth of information means a dearth of something else: a scarcity of whatever it is that information consumes. What information consumes is rather obvious: it consumes the attention of its recipients. Hence a wealth of information creates a poverty of attention and a need to allocate that attention efficiently among the overabundance of information sources that might consume it.
The following sections provide a partial analysis of some factors that affect individual and societal attention and the provision and use of information (including geospatial) in addressing complex issues. We focus on issues relating to natural resources, environments, and anthropogenic and natural hazards, but suggest that the findings and discussions of this article and of its companion (Glynn et al., 2022, this issue) have broad relevance to the use of information in decision making.
3 The Value of Information in Data to Decision Pathways: Examples
We now provide some DDP examples that help illustrate some of the challenges and opportunities identified in our “Value Explorations” (Table 1, and Glynn et al., 2022, this issue). Many of the examples (DDPs 2–5) are constructs based on the experiences and knowledge of the co-authors, with respect to the biophysical sciences and VOI and economic studies (e.g., Watson et al., 1984; Bernknopf and Shapiro, 2015; Chiavacci et al., 2020; Molder et al., 2022).
Our first example (DDP 1) differs from later ones: it reports on a study that sought to estimate the value of improving publicly accessible geospatial information. The study, and our discussion, does not elaborate on the many uses of geospatial information identified in the study to enable different types of decisions. Geospatial information can be used and has value for many different types of applications. From the diversity of applications mentioned – and from our discussions of other DDP examples – it becomes clear that the value of geospatial information is not just a sum of values determined through an assessment of uses at a given moment in time; or even through improvements in resolution, or in production and use technologies (Watson et al., 2022). Instead, the value of geospatial information keeps increasing as new ways, and associated processing and decision models, are found to use it: often through innovative combinations with other types of information (e.g., Chiavacci et al., 2020).
3.1 Public Goods for Multiple Data to Decision Pathways: Geospatial Information (DDP 1)
The U.S. Geological Survey (USGS) worked closely with the Office of Management and Budget (OMB) in developing the Primary Mapping Economic Analysis (PMEA) in the late 1980s (Amos et al., 1989). The objective was to identify the optimum revision cycle for topographic (topo) 7.5-min quadrangle maps. The USGS had recently completed once-over coverage of the 57,000 topo sheets covering the contiguous 48 states, and there were questions about the level of funding needed for the USGS to keep the data in the maps current. OMB requested the study to examine societal benefits from alternative revision cycles to provide information about future funding needs (cf. VE10). Statements below reflect first-hand knowledge by our co-author, Carl Shapiro, a lead participant in the study.
Initially USGS suggested that a contract be developed with academic experts in the study of the value of scientific information. OMB rejected that option because they wanted the USGS to develop a better understanding of the use of topo maps and the benefits they provided to society. OMB stated that they wanted USGS to gain expertise and understanding in how its map data were being used.
As the study was initiated in 1987, USGS discussed the possibility of positive net benefits being identified and documented through the PMEA effort. OMB explained that they had no doubt that positive net benefits from USGS revising its topo maps would be identified through either a USGS study or a USGS-funded study with academic experts. OMB’s key interest was in assessing the process for identification of the uses of revised map data and of the benefits that resulted – and whether that process was appropriate (VE12). Through frequent consultations, OMB’s questions and concerns were integrated into the study aims and methodology.
The basis for the study was that the 57,000 USGS topographic 7.5-min quadrangle maps are a multipurpose product designed for multiple applications ranging from transportation, agriculture, and urban planning, to recreational uses such as hiking and fishing. The information contained in USGS topographic maps, as in many other geospatial products currently available, has public good (i.e., non-rival, non-exclusive) characteristics. Consistent with the Paperwork Reduction Act of 1995 (Paperwork Reduction Act, 2015), topographic map information is in the public domain without restrictive barriers to use (such as a copyright or license).
The benefits identified in the PMEA from topographic map information were based on this non-rival non-exclusive premise. As applications were identified, the estimated benefits from the applications were summed for given topo maps covering specific geographic areas. The results from the study (Amos et al., 1989) suggested that the optimum revision cycle for topo maps in the late 1980s was between three and 5 years, depending on the characteristics of the area within the map quadrangle. Fast changing urban areas were typically determined to need more frequent revision than remote areas without as much change. The study results were based on costs in the late 1980s.
Many technological advances have occurred in the 35 years since the PMEA, and the costs of topographic mapping and distribution have dramatically reduced as digital technology has replaced analog techniques. As a result, today’s revision needs are very different from what they were in the late 1980s. The findings from the PMEA concerning optimum revision cycles are no longer meaningful. Nonetheless, the value provided by topographic maps (and other geospatial information) and the societal benefits that can be derived have likely only increased, due to reductions in costs of production, identification of new applications, and near instantaneous uses made feasible through advances in information and communications technology. Geospatial information today can be and is used to plan and control the location of pig farms, drinking water wells, and other human infrastructure in Denmark (Refsgaard et al., 2010; Jørgensen et al., 2013), to enforce regulations or policies based on remote sensing of land use (Forney et al., 2012; Bernknopf and Shapiro, 2015) or of drought conditions (Bernknopf et al., 2018), to assess vulnerabilities or to provide damage assessments following catastrophic events (Liu et al., 2014; Bernknopf et al., 2020), to create visual representations of statistical assessments or models (VE13), to create crowd-sourced maps for tribal or cultural purposes (Herlihy and Knapp, 2003; Turnbull, 2007; Elwood, 2012; McCall et al., 2015) or for various socio-economic assessments (VE14), and for a wide range of commercial uses (VE11). As additional information becomes accessible and usable to improve or complement geospatial information, new-found uses will continue to be discovered (VE15).
3.2 Data to Decision Pathways and Well Perceived Hazards (DDP 2)
3.2.1 Evacuation of a Town Given Information About an Impending Critical Hazard (DDP 2 Example 1)
A town manager faces a critical decision to evacuate, or not, a town in the face of a quickly impending clearly perceived hazard, such as a flood or a wildfire. Accurate, dynamic geospatial information, such as that provided by remote sensing (e.g., temperature hotspots detected by a satellite) and/or by ground measurements (e.g., one or more stream gages upstream of the town), may be available for effective decision-making. It is generally assumed that when the needed information (and associated processing and use model) enable a go/no-go evacuation decision, there is sufficient time for an evacuation to proceed.
Estimating VOI for the dynamic geospatial information (and its associated model) is typically done by comparison with a counterfactual (cf. VE2) situation, where a go/no-go decision is made in the absence of the geospatial information. The estimation contrasts the number of lives saved and property damages avoided following an evacuation (go) decision made with the benefit of the geospatial information, with a situation where the information was not available. Similarly, a decision (no-go) to not evacuate, and avoid evacuation costs, made with the benefit of the geospatial information, is compared with a situation where the geospatial information was not available. A generalized VOI estimate can then be made contrasting 1) a combination of values for the informed go and no-go decisions with 2) values for the less-informed alternative no-go and go combination. The VOI estimate may be done ex ante, or as informed by prior experiences.
As mentioned in Table 1, and in our companion article (Glynn et al., 2022, this issue), there are key questions to consider in the use of counterfactuals to estimate a VOI for the dynamic geospatial information. Specifically, how does the model(s) used to process information and make decisions when the dynamic geospatial information is available compare with the model(s) used when the geospatial information is absent? [There are always some information, beliefs, and knowledge that are used in go/no-go decisions: regardless of the availability of the dynamic geospatial information whose value is being assessed (cf. Figure 1)]. Are the processing and use model(s) in the presence and absence of the geospatial information sufficiently similar, or are they completely different? In the latter case, the counterfactual comparison method does not provide an estimate of VOI specifically for the value of the dynamic geospatial information. Instead, it provides an estimate of the value of 1) a new set of information with a new associated model – relative to the value of 2) some previously available information associated with a different model for processing and use. In turn, this means that there might be a need to compare the actors, purposes, scopes, conditions, and other characteristics associated with the two different situations of information use (i.e., the factual and the counterfactual).
Let’s assume for the moment that the model(s) for information use and decisions are sufficiently similar and applicable to both the factual and counterfactual situations. Adding the net benefits (compared to the counterfactual case) then allows a VOI estimate for the dynamic geospatial information. The value of lives saved can potentially be monetized through an estimate of the applicable “value of a statistical life” (VSL) for the community in question (Viscusi and Masterman, 2017; Kniesner and Viscusi, 2019). Usually though, VSLs are defined nationally (US EPA, 2006; US EPA, 2014) and may not necessarily be reflective of the VSL for the given town. They may not reflect the individual economic characteristics of the lives saved (Viscusi, 2010; Greenstone, 2021) or not saved (cf. VE13). For example, the applicable VSL for the case of a town or of a community with relatively low economic prospects would likely be lower (other demographic characteristics being held constant) than the VSL that might apply at a national level (Viscusi, 2010; Viscusi and Masterman, 2017; Broughel, 2020). Applying a locally applicable VSL instead of a national one brings up questions of social justice and equity (cf. VE14).
Perhaps the main purpose of monetizing the estimated “lives saved” is that once done, this particular benefit can be compared and/or added to the estimated monetary values of the property damages avoided, and/or of the evacuation costs incurred or avoided. How useful is it to be able to do this monetary summation and to have a single dollar number for VOI? Consider the case where a town (or region or nation) wants to assess the benefits and costs of investing (or not) in the procurement and operation of various technologies providing dynamic geospatial information. With proper monetization and VOI estimates, different alternatives (including the choice of not investing) could potentially be compared, ex ante, to each other, thereby helping the town (or the funding entities) make decisions within the constraints of available financial resources. Assessing the extent of societal and funding commitments, estimating the expected useful life of the technology procured, and establishing a discounting model for its value and the VOI, are also likely to be important.
Consider the structure of a DDP (Figure 2) that incorporates consideration of the possible use of both ex ante and ex post valuation of information studies. (“Models,” in this article, means all mental, conceptual, and/or scientific models associated with the processing and use of information). The “models” and the curved arrows – i.e., all transformations from data to decisions – are influenced by human BBHV and other behavioral and social factors (cf. Glynn et al., 2022, this issue). Decisions to conduct an ex ante study, or to include new sources of expertise, information, models, or to involve new actors, can be made anywhere on the pathway. Ideally, ex post or post-audit studies are conducted once outcomes of the decision pathway become clear.
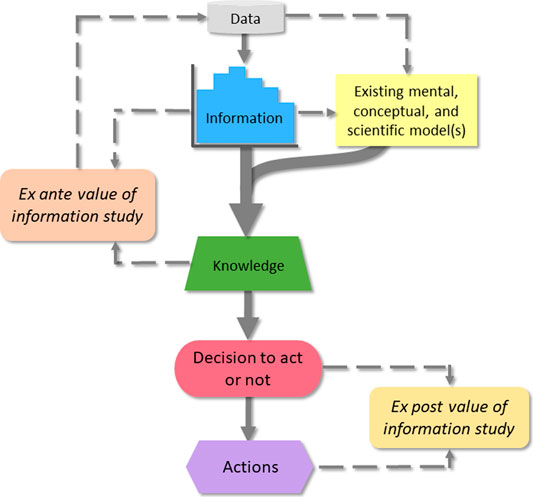
FIGURE 2. Structure of a data to decisions pathway (DDP), now showing the role of models and some feedbacks and interactions in a progression to decisions (and possible actions). Additional feedbacks and interactions may occur beyond those represented. Behavioral and social factors affect all aspects of the DDP. Value of information studies are not restricted to classical economic VOI studies.
Figure 2 shows models (e.g., mental, conceptual) and BBHV and behavioral/social influences affecting, 1) from the start, the seeking of information (by DDP actors), 2) the internalizations of information into knowledge, 3) the seeking of new data or information or new valuations, 4) the use of information to update models (of all types), and 5) the final transition of knowledge (tacit or scientific) into decisions.
Adding further complexity, a diversity of actors can be included in all these transformations, and in the decisions to prioritize, add, update, and evaluate information and models. Filters and barriers can arise in the transition from decision to action. For example, a legislature might pass a law telling an administration to do something but does not designate or provide the funding needed. Figure 3 illustrates these challenges and alludes to differences between decisions made by different types of actors (e.g., a policymaker and a citizen).
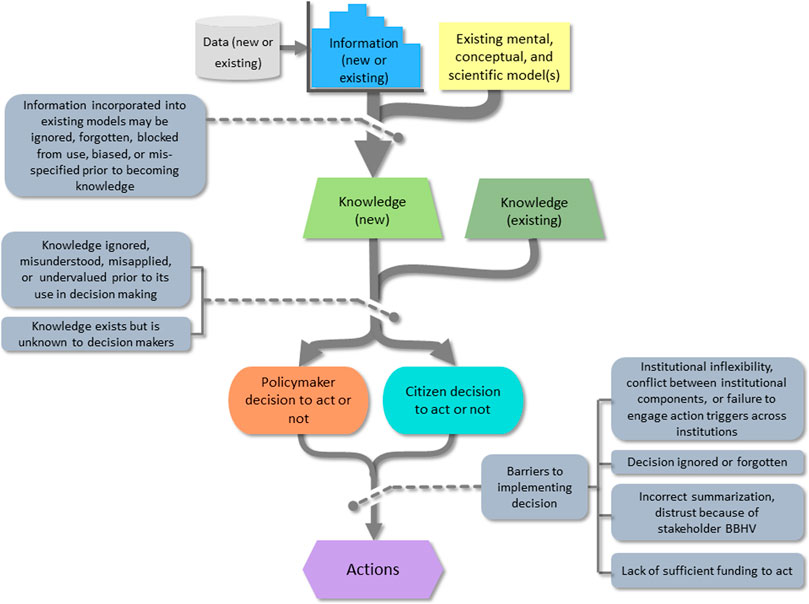
FIGURE 3. Additional complexities and some potential barriers and challenges affecting DDP progressions. Knowledge sources and actors involved also affect progressions, including resulting decisions and actions.
Value of information and pathways to decisions are actor dependent. Lastly, Figure 4 describes the differences in values and choices that could occur between a Town Manager (TM) and a Local Resident (LR) confronting a decision to evacuate or not in the face of an impending hazard, such as a hurricane. The TM trusts the new geospatial information that the town has acquired, and the information is properly interpreted and applied. The availability and use of the new geospatial information results in more necessary, and fewer unnecessary, evacuation orders than would be issued without the information. From the TM’s perspective, the net
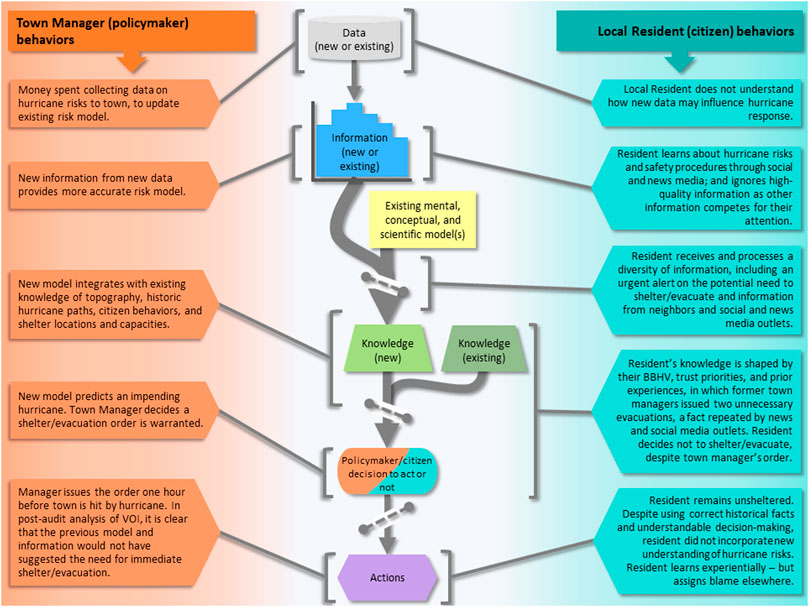
FIGURE 4. Differences in DDP perceptions and decision making for two different actors – a town manager and a local resident – confronted with different knowledge sources, and different roles and decisions, relating to a well perceived hazard, a hurricane in this example.
Other costs and benefits may also need to be considered in estimating the VOITM, such as those associated with property damages, public insurance, etc.
A local resident might perceive and value available information differently, however. They may not trust local information or the TM. Residents may see potentially conflicting information on national news or on social media. They may place a value on their life considerably different from the VSL used in the TM’s calculation. The local resident may not feel the same ownership and emotional investment in the new dynamic geospatial technology and information that the town manager feels. Many other factors may affect a resident’s likely innate evaluation of
Ideally, the TM used best science in making an evacuation call. Residents may not have. If the TM had invested in the new geospatial technology and also in a public outreach campaign, could that have changed the decisions of residents and raised the
3.2.2 Investment Decision for Future Mitigation, 15 Years After an Extreme Event (DDP 2 Example 2)
Making decisions to invest in the procurement of information, or in infrastructure that might help mitigate a future catastrophe with information acquired through the lived experience of a previous event, is difficult (Wachinger and Renn, 2010; Wachinger et al., 2013). Decisions compete with other investment decisions. Behavioral and social factors affect that competition (Hoffmann and Muttarak, 2017; Castañeda et al., 2020). As just one example, the time elapsed since a prior experienced catastrophe will affect community memory and cognition of such events. With the passing of time, community memory and cognition of the event will decrease (VE8). The town of Nürburgring (Germany) faced a choice in 1925 of investing in either 1) building a reservoir to help contain flood waters above the town (i.e., following a devastating 1910 flood) or 2) building a racetrack to improve the town’s economic situation. The town chose to build the racetrack, which brought the benefit of 2,500 local jobs for 2 years, and the attraction of car-racing and the automobile to one of Germany’s poorest regions (Bennhold, 2021). In July 2021, a flood devastated the town. Did the town make the best investment decisions in 1925? Did it have all the information needed to make a good decision? Did the town properly perceive and estimate the possible impacts of flooding in the absence of constructing a new reservoir (cf. VE4 and VE5)? Could the experiences of other towns and regions have been useful to consider (cf. VE6 and VE7)? Did the town make appropriate prioritizations and discounting with the information that it did have (cf. VE8)? Did the town leaders and decision-makers re-consider the need to build a reservoir once the town’s economic situation had improved? What dynamic geospatial information did it have, or did it acquire later on, that might affect an assessment of the town’s decision making, in 1925 but also in more recent years? What should it consider going forward? In answering all these questions, it could be that decisions to not take further action are entirely appropriate, given information available, the need to consider competing priorities for resources, and rational discounting of the uncertain future.
We suggest that post-audits of the town’s decision making that tried to answer some of these questions and others might be useful. Ex post economic analyses assessing the value and use of available information would be part of this post-audit which could also help elucidate and highlight behavioral and societal challenges to more rationalistic decision making. We also realize that there is generally little incentive to look back at situations like that of Nürburgring: crises of the moment tend to take precedence over post-audits. Society rarely extracts the full value of information provided by past crises (Barry, 1997; Diamond, 2004; Meyer and Kunreuther, 2005; Ponting, 2007).
3.3 Data to Decision Pathways: Poorly-Perceived Broadly-Occurring Hazards (DDP 3)
As human beings, we have difficulty perceiving and appreciating the value of information that pertains to health risks that we cannot easily detect through our senses. This difficulty is further compounded when 1) the most perceivable health impacts occur only after years of exposure to risk factors (i.e., contaminants, pathogens, risk behaviors), and/or 2) multiple factors combine (VE5a). We may have information describing all these risk factors, but the information has no value to us if we do not pay attention to it, cannot understand it, do not know how to process it and use it to make decisions, or if we do not act on it – assuming action was a warranted outcome of available information, which is not always the case. Does that really mean the VOI is zero? Only if the information is never used, by any actor. Should policymakers, and others just give up on the possible use of such information because they assume that the only thing that matters is what people perceive to matter? Or should they instead try to find ways to make the information matter, and/or find ways for it to be used to improve decision-making?
3.3.1 Manipulations and Community Realization Problems (DDP 3 Example 1)
There are many examples that document the failures of society, people, and institutions (including scientists) to properly address available information about health risks. These failures have usually been unintentional, but there are also examples of intentional manipulations of information and abuses for the sake of profits (Oreskes and Conway, 2010), or for the retention of political power (Finocchiaro, 2010; Strassheim and Kettunen, 2014; Goldberg, 2017). For example, the COVID-19 pandemic has shown that in many cases (Gilmore et al., 2020; Hyland-Wood et al., 2021; Kachanoff et al., 2021; Rosenfeld et al., 2021), communities and their leaders do not share and use information effectively (VE6, VE7), or devise policy based on available statistical information and probabilistic assessments of the risks of infection and transmission (VE13). Moreover, some media outlets have a commercial interest (VE11) in providing mis-information and catering to mis-perceptions rather than in properly communicating factual information and educating their constituencies (VE12) (Greifeneder et al., 2020). Aside from the benefits of mis-information or dis-information to certain actors (but not to society on net), there are many different pathologies that affect the communication and receipt of information by communities (VE12). They go well beyond the distractions and negative value of irrelevant information (cf. VE 5b).
3.3.2 Arsenic in Groundwater (DDP 3 Example 2)
Let us consider a case where information about a health risk has been difficult for communities to perceive, value, and effectively act on. In a World Health Organization report, Smith et al. (2000) called the contamination of groundwater by arsenic in Bangladesh “the largest poisoning of a population in history, with millions of people exposed.” Drinking water use of groundwater from tube wells accelerated in Bangladesh in the 1980s, prompted by the well-intentioned aim to reduce infant and child mortality caused by microbial contaminants and pathogens in surface water supplies. The shift in water supplies was encouraged through efforts of the United Nations Children’s Fund, the Bangladesh Department of Public Health Engineering, and studies by the British Geological Survey. However, the problem of high arsenic concentrations in groundwater and potential health impacts was not considered.
It is estimated that, as a result, at least 31 million people in the Bengal Delta were exposed to arsenic drinking water concentrations above 50 μg/L and at least 50 million people were exposed to concentrations above 10 μg/L (Chakraborti, 2002). Smith et al. (1992) estimated that a US population exposed to drinking 1 L/day of water with an arsenic concentration of 50 μg/L could have a resulting lifetime cancer mortality risk from liver, lung, kidney, or bladder cancer of up to 13 in 1000, which translates to about 400,000 cancer deaths if a population of 31 million were exposed, as in the case of the Bengal Delta. The health risks posed by arsenic in groundwater are global. A 2018 WHO Fact Sheet (WHO Arsenic Key Facts, 2018) mentions that at least 140 million people in 50 countries around the world (including in the United States) are exposed to arsenic concentrations above the recommended WHO limit of 10 μg/L. The consequences of exposure extend beyond the increased risks of cancer in the affected populations. Indeed, a study that included the use of molecular genetic information to account for family links and arsenic retention in Bangladesh, (Pitt et al., 2021) found that 1) high levels of arsenic retention were correlated with lower performance on cognition tests and with lower levels of schooling attainment; and suggested that 2) halving the amount of retained arsenic would boost the proportion of young men in skilled jobs by 24% and the number of entrepreneurs by 26% (cf. Yale Economic Growth Center, 2020). The study followed a cohort of families and individuals over a period of 26 years.
Despite the well-documented health risks and economic impacts of arsenic in drinking water, the issue often remains unaddressed by public health agencies and individuals in the Bengal Delta and in other countries, including the United States (e.g., New England, New Mexico), because drinking waters from private wells are often not tested or appropriately treated. A June 24 2021 article in The Guardian (Padmanaban, 2021) documented the continuing problem with arsenic in India, including the fact that many people remain unaware of the causal link with mortality from cancer. In such a situation, what are the chances that they might be aware of more subtle types of economic impacts? Global society has a wealth of information that relates to the probable occurrence of arsenic in groundwater. Geologic maps provide documentation of the regional areas, types of geologic formations, and aquifer depths where arsenic is likely to be a problem (VE15). This information has been available and accessible for decades. But not everyone potentially affected by the issue knows how to retrieve and understand the information; or has the resources, prioritization capacity, or the will to action to get their drinking water tested or treated or changed as a result. This is true for most individuals, but especially so for those in socio-economically disadvantaged communities (VE14).
Actors and decision-makers that understand and appreciate the full value of information about arsenic in drinking water may have the power to usefully act on the information, for themselves and their communities. They can also share knowledge of the information and its uses across communities (VE6 and VE7) and encourage additional production of information (VE10) where gaps exist. In conjunction with this approach, efforts can be made to greatly sensitize communities to understand, fully value, and act on available information. This may be needed to obtain sufficient political support for persons in a position to fully enable a DDP for the available or potentially available information. Other benefits may accrue from community learning about the issue.
3.4 Data to Decision Pathways: Gradual Change and VOI Temporal Dynamics (DDP 4)
Here we provide a hypothetical example of how VOI could change through time. Imagine a lake surrounded by houses and used for fishing and recreation. Water quality monitoring is done on a regular basis and provides information on temperature, turbidity, nutrients, and chlorophyll-a (VE10). Over time the lake’s water quality slowly worsens. Lake temperatures are also rising, possibly preventing seasonal overturning and mixing of the lake waters. Nutrients from the septic systems of homes around the lake and in the watershed enter the lake. Cattle and horse ranching are also present. Nutrient levels are slowly increasing. Based on available information, experts suggest (VE4) that nutrient controls and other measures need to be implemented to prevent further degradation of water quality. They are concerned that algal blooms might develop, including possibly harmful algal blooms that make the lake toxic for recreational activities and/or drinking water use. The experts cannot predict with any certainty if or when algal blooms might develop in the lake. They aim to reduce their uncertainty by obtaining information from similar lakes that also saw declines in water quality and ultimately, algal blooms (VE6).
However, expert knowledge confronts political realities (Xu et al., 2013; Van Dolah et al., 2016). Some homeowners may not understand or value the environmental benefits of getting rid of septic tanks relative to the costs of sewer connection charges (VE5). Some ranchers and farmers may not believe that their operations meaningfully impact nutrient loadings and/or may be uninterested in following recommended Best Management Practices (VE6). Various constituencies may not feel a need to take responsibility for the deteriorating situation (VE7). The behavior of these diverse groups suggests that water quality information and expert knowledge have little to no value at that moment in time (Berardo et al., 2019).
Continuing our hypothetical example, harmful algal blooms develop. The blooms are visible, smell bad, and dead fish are found (VE4) (Paerl et al., 2001). Recreation and fishing in the lake is stopped. New sources of drinking water need to be found (Stroming et al., 2020). The price of homes near the lake crashes (VE11) (Wolf and Klaiber; Kuwayama et al. 2022). Tourism and the area’s economy are affected (Heil and Muni-Morgan, 2021). Lakeside homes are among the most expensive in the area, and such homeowners exert strong political influence (VE14) (Hall and Yoder, 2022). The visual and olfactory information provided by the algal blooms is supplemented by posted health advisories. All this attention suddenly increases the perceived value of previously obtained or available water quality information (VE15). Experts are hired (VE10) and actions get taken. However, because phosphorus and nutrients have built up for years in the lake sediments, improving the lake’s water quality is more difficult than if the situation had been addressed earlier (Carpenter, 2005). The area’s reputation for recreation and tourism is affected and takes years to recover. House prices also take time to recover. These factors create negative feedback loops in the local economy, further worsening the situation (Brock and Carpenter, 2007).
3.5 Data to Decision Pathways: VOI and Complex Pathways (DDP 5)
The hypothetical examples presented above reveal challenges that could affect people’s perceptions of the value of information. Such challenges include: 1) limits on human sensory cognition and information processing capabilities (DDP 4), 2) the dilutive effects of an aggregation of multiple causes and/or actors (DDP 4), and 3) dislocations and problems caused when information relating to a previously experienced event is too far in the past (DDP 2, example 2), or when information about the cause and potential impact of a risk is diluted over time and not well perceived, because the impact takes time to develop (DDP 3).
DDP 4 described a case where both the causes, and the consequences, of an environmental issue occurred in the same geographic area. Actionable DDPs become much more challenging when the benefits of action are provided to a community or region that is spatially and/or socially disconnected from the community or region bearing the costs of the action, especially if the costs are immediate and easily perceived. The distant communities and actors will place very different values and prioritizations on the use of any information that is available (Van Dolah et al., 2016). For example, there are many areas around the world where farming occurs in the upper parts of a basin, but the pollution impacts of fertilizer use (e.g., hypoxia) occur far downstream (Hufnagl-Eichiner et al., 2011), in estuaries and coastal systems (e.g., Chesapeake estuary, Gulf of Mexico), and/or much later in time (Van Meter et al., 2017). Why would a farmer accept individual responsibility for past or potential contributions to summer hypoxic zones occurring 1000s of miles away, especially when actions of a single farm may not have appreciable influence?
So, what effective DDPs might be found to address such spatial and social disconnection problems? One possibility is to find or create local benefits, in the area that we will call “the causal domain,” to compensate for the local costs of addressing the problem. For example, will actions to reduce nutrients in the upper part of a basin result in a smaller occurrence of harmful algal blooms in lakes and reservoirs in that area? Alternatively, broadening frames of reference or considering co-benefits might be helpful: placing attention on other contaminants associated with farm operations (e.g., pesticides), considering new economic opportunities made possible by reducing fertilizer use (e.g., establishment of trout fishing), and/or convincing or requiring a community to act in the national interest rather than for its local interest. Broadening frames of reference affects VOI and DDPs because it likely requires bringing in new information and possibly new actors. In many countries, states, communities, and individuals want the freedom to make decisions and act locally, independently of national or global needs (Schultz et al., 2014); polycentric governance (Jacobs et al., 2007) and an evolution of social norms (Kinzig et al., 2013) may be needed. Considering wider spatial or social framing, however, may raise governance and trust issues and complex social dynamics that may be difficult to resolve, especially if timeliness is important. Legal actions remain possible, and various mechanisms for collective redress of environmental damages have been considered internationally (Wagner, 2011; Litwin and Feder, 2014; Rhee and Harsagi, 2014; Tóth, 2017).
4 Data to Decision Pathways: Societal Challenges Affecting Use and Actionability of Information
There is a natural order to DDPs, their use across communities, and possibly across issues and needs. The following DDP steps are only one way to describe how actors who generate, communicate, and use information might interact with a DDP.
S1. Recognize an individual or community need, usually in response to prompting event(s).
S2. Realize the consequences of inaction in face of the need.
S3. Determine, given some initial knowledge, a need to obtain additional information. This step presumes an ex-ante valuation of information – and of associated processing and use model(s) – by actors who will use and communicate the information for others to act on. Individual perceptions, BBHV, norms, and social constructions of reality, as opposed to some set of absolute truths and facts, may strongly influence this valuation step.
S4. Possibly adjust the information processing and use model(s), and perceptions of the need for action.
S5. Decide to act (or not).
S6. Conduct ex post studies of VOI and associated audits of the valuation and actual use of information (and associated models). Determine improvements in the valuation and use of information. Enhance the planning and governance of DDPs (including actors involved) to maximize the effective use and actionability of information for future decisions.
S7. Share knowledge gained with other communities (e.g., Anderson et al., 2019).
S8. Recognize the application of information (and associated models) to other issues.
The DDP sequence above builds on the “recognize, internalize, evaluate, advance” (RIEA) sequence for the processing of information presented in our companion paper on the behavioral and social factors affecting VOI (Glynn et al., 2022, this issue).
4.1 Social Cognition and Communication Challenges
Community cognition events (CCEs) will likely play an important role at various points in this series of potential steps for DDPs. In our definition, a CCE is an event that raises, at least temporarily, a community’s broad social understanding and perception of the potential risks and consequences of similar events. CCEs bring additional knowledge to communities. Often their importance lies not first in the additional information being brought, but rather in the fact that the events have the power to change community perceptions, mental models, and possibly prevailing beliefs and attitudes. CCEs can be provided by natural disasters, or by events that make the previously invisible (unperceived) event visible (perceived), such as the occurrence of algal blooms in a lake.
The level of conscious cognition and perception provided in a CCE depends on the magnitude of the event, and the number of people directly impacted. Cognition wanes rapidly with time for at least two reasons. First, the memory of the event, like that of many painful events, dissipates quickly in a community’s consciousness. Second, the community itself changes and evolves with time: children become adults, people move into and out of the community. The result is that the experiential information acquired by a community can quickly dissipate. The 1976 Big Thompson Canyon flood in Colorado (Pohl, 2016) provides an example. The flood killed 144 people and resulted in $35 million in damages to 418 homes. The flood however did not prevent people from eventually rebuilding homes along the banks of the Big Thompson Canyon. People in many of those homes had to be rescued by helicopter from their rooftops when the September 2013 floods occurred (McKee, 2021). Similarly, communities affected by hurricanes and floods in Louisiana, Florida, and along the Gulf Coast seem to forget in just a few years many lessons provided by those events (Colten and Sumpter, 2009; Colten and Giancarlo, 2011; Grossman, 2018). In other words, the VOI provided by a CCE gets discounted quickly by a community, probably exponentially with time; knowledge gets lost and often remains unshared. Because of this, we consider that efforts to create international repositories of knowledge, such as the proposed Global Harmful Algal Bloom Observing System (Anderson et al., 2019), are important. Additional insights on CCEs and community memory and cognition are provided by transdisciplinary papers investigating “the extended mind” (Clark and Chalmers, 1998), “socially extended cognition” (Gallagher, 2013; Huebner, 2013; Lyre, 2018), and “the collective mind” and the social construction of knowledge (Brown, 2015).
Communication of information, and communication of VOI between different actors is also a factor to consider in the analysis of DDPs. As mentioned earlier (VE12), various problems, pathologies, issues of credibility, and other human and social factors can affect the transmission and reception of information, how information is valued and used, and in some cases can even change the very nature of the information that is communicated (e.g., weather forecaster’s wet bias). In some DDPs, perhaps especially those that involve multiple steps and multiple actors, effective communication between actors may be essential to implementation. For example, in the study and DDP described by Chiavacci et al. (2020), healthcare professionals were important for communicating with homeowners about the relevance of radon potential maps to their health risks. This communication was crucial and increased the likelihood that homeowners would at least test their houses for high radon levels. The timeliness of information and VOI communication between actors is also often critical, and must be considered in relation to the temporal aspects controlling VOI (e.g., discounting) and more generally to the useability and actionability of information. For example, it might take time for an information provider to establish credibility with an information consumer, especially if the provider is socially distant (Akerlof, 1997; e.g., DDP 5). Social group effects (cf. VE14), including those related to social identity and identity economics (Akerlof and Kranton, 2010; Kranton, 2016; Huettel and Kranton, 2012), and to group (e.g., DDP4) and actor motivations (Akerlof and Shiller, 2015) are also likely to affect the nature, perception, transmission, acceptance, and valuation of information and beliefs (cf. VE12, DDP 3, DDP 5).
4.2 Monetization and Prioritizations of Information Investments
Value of information measures are based on basic economic approaches. There is an expected outcome that occurs with the use of information and a comparison is made to expected outcomes if the information did not exist or was not used (Macauley and Laxminarayan, 2010; Keisler et al., 2014). In many cases, this value is expressed in monetary terms. In some cases, however, it may be appropriate to express value using different types of metrics. For example, Molder et al. (2022) describe use of a qualitative approach to provide a better understanding of the value and value chains for Landsat information (Wu et al., 2019). The approach mapped the Landsat data ecosystem to include the diversity of actors and uses of the information, and thereby provided a useful system perspective on Landsat VOI. In other cases, it may be difficult or impossible to develop meaningful monetary measures. The value of cultural assets can be expressed in many cases using metrics that are not monetary. Due to the complexity of behavioral and other factors affecting VOI and DDPs, we suggest that caution is warranted in the production, interpretation, and use of monetary valuations, including in regulatory impact analyses (OMB, 2003) and in budget justifications to support information production investments.
There are many questions regarding the meaning and objectives of monetary valuations, especially as part of DDP analyses, policy assessments, and trade-off comparisons. Monetary metrics used in VOI and in other types of economic studies should have clearly stated purpose(s). Monetary metrics have the advantage of allowing comparisons and appearing to have additive or multiplicative properties. But any such operations assume a similarity of methods and (behavioral and social) conditions across different monetization estimates. Caution is further warranted if a monetary valuation is provided by one actor to another with the expectation that the monetary metric will inform the receiver in making a given decision, or taking a given action. For example, how well do both actors understand the meaning of the quantitative monetary metric? What and who does the metric relate to? What reference states or baselines are used to scale the metric? How was the metric developed, for what sort of conditions and context, and importantly, what were the assumptions used?
Understanding and critically assessing how monetary valuation(s) are used in decision making is essential. This means determining if the valuation(s) will be used for binary type decisions (e.g., go/no-go), to rank-order a set of possible choices, or to add greater resolution and continuity for some other type of operation (Watson et al., 1984). The likelihood of error, or inappropriate use of valuations, increases with the expectation that finer resolutions support choice-enabling differentiations (Spash, 2007; Dallimer et al., 2014).
To date, many VOI studies have focused on producing single estimates of VOI. This can give the impression that information had a single economic value (albeit perhaps a minimum value). Instead, the value is likely conditioned by various factors, such as the actors involved, the characteristics of the information and the associated processing and decision models involved, discounting effects, and the general DDP context. Economists commonly provide a range of values with confidence intervals and estimate values under different scenarios. In the personal experience of the authors however, policymakers, the media, and others often want a marquee take-away number. Context and subtleties get simplified away. The advantages of such simplification also carry disadvantages.
4.3 Knowledge Repositories: One Possible Next Step
Greater understanding of the complex societal and behavioral factors that affect VOI and DDPs, and the use and actionability of information, may come from knowledge repositories that document case studies and their findings. For example in 2019, under the auspices of the GEOValue community of practice (GEOValue, 2022), the European Commission, USGS, National Oceanic and Atmospheric Administration, and others co-organized a meeting hosted by the European Space Agency’s European Space Institute (ESA-ESRIN). Meeting participants identified gaps within the valuation of the benefits (ESA-ESRIN, 2019) from Earth Observations (EO). The US Group on Earth Observations (USGEO) subcommittee identified the same gaps in their 2019 report: National Plan for Civil Earth Observations. Both USGEO and GEOValue advised the following actions:
1.Collect economic studies and synthesize the state of knowledge on the economic value of EO, including return-on-investment, ability to accelerate innovation, and contributions to economic growth.
2.Develop a catalog of ways to quantify the social and economic value of EO.
3.Collect, catalog, and publish qualitative narratives and quantitative examples on the benefits of EO.
As a result, a GEOValue working group designed a societal benefits repository for EO and geospatial case studies. Document ingest is expected in 2022, with synthesis reports starting in 2023.
We expect that the repository will help develop understanding of VOI and DDP issues that impact the use of Earth observations and geospatial information. DDP examples and discussions provided above, and the exploration of information valuation methodologies and issues that are the subject of our companion article (Glynn et al., 2022, this issue), allow an initial consideration and summary (Table 2). The GEOValue societal benefits repository includes a “functional classification” to help characterize and group case studies of the benefits of geospatial information. Table 3 uses our VOI explorations and DDP examples to indicate possible social and behavioral factors that may also impact valuation of information and DDPs for the different functional classifications in the GEOValue case studies.
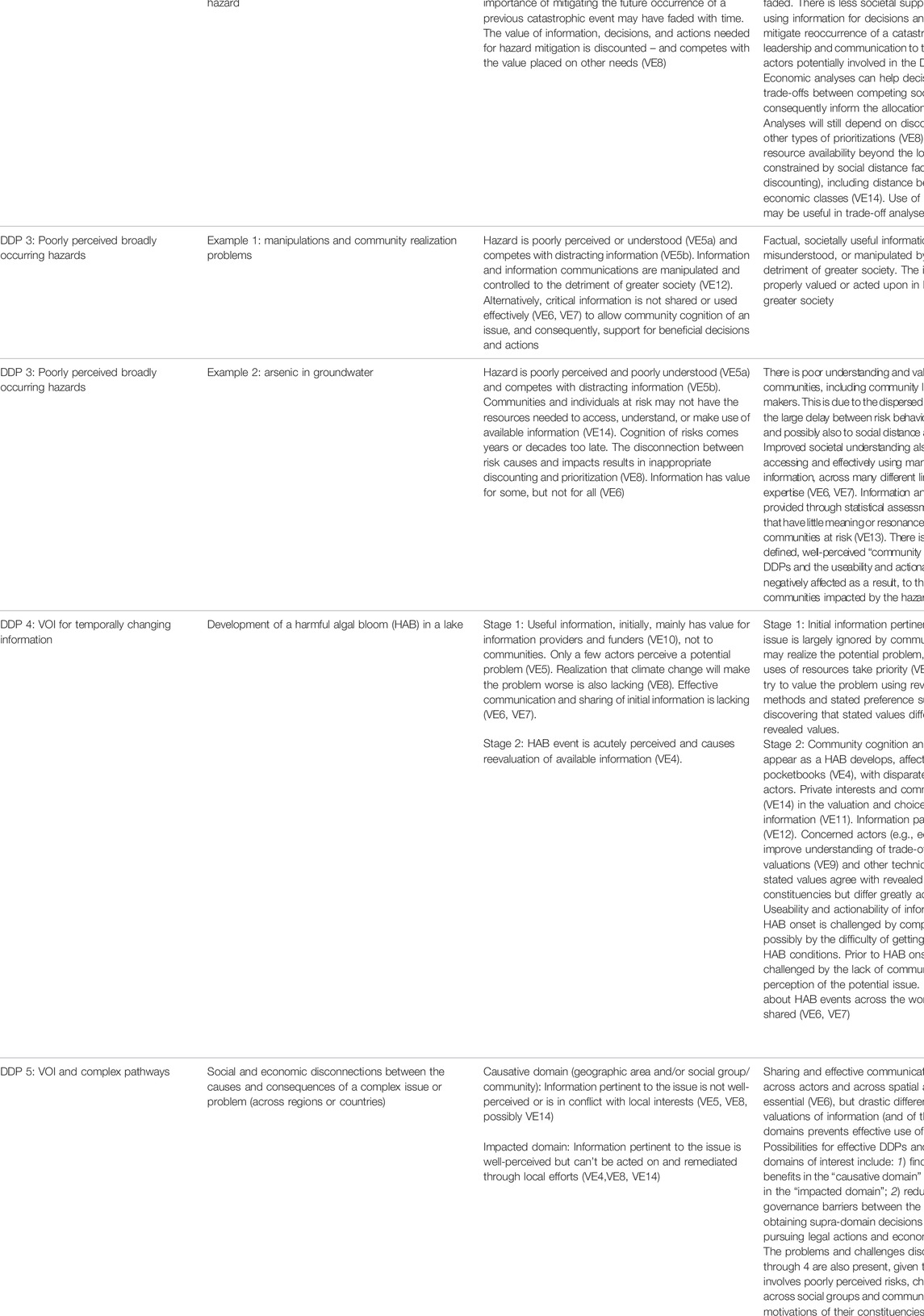
TABLE 2. Summary analysis of some critical factors and issues affecting different types of data to decision pathways, and the valuation, useability, and actionability of different types of geospatial information.
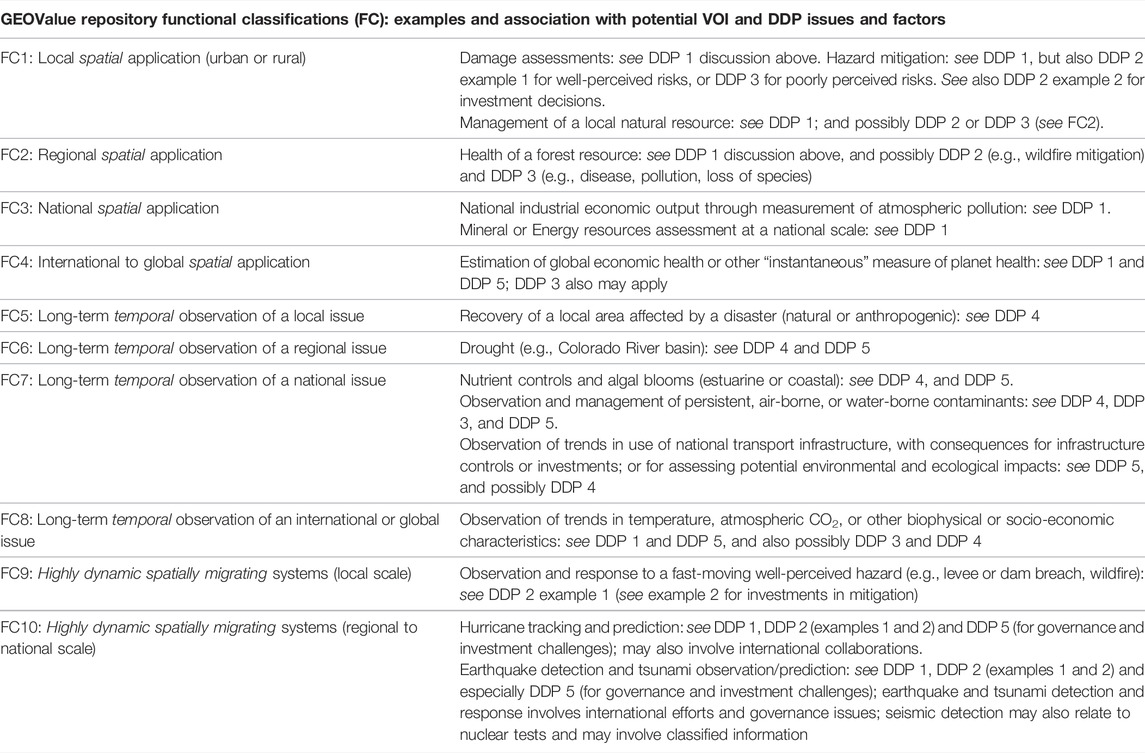
TABLE 3. Potential VOI and DDP issues related to 10 functional classifications of geospatial information created for the GEOValue Societal Benefits Repository.
4.4 Limitations and Opportunities
Our study has many limitations. It explores human and community behaviors affecting VOI and the useability and actionability of information, but places VOI in the context of a relatively linear, well-ordered, set of stages establishing a DDP. This assumes actor interest and focus on a single DDP, consideration of which could provide great societal benefit. A more complete study might have considered interactions and feedbacks that could take place between and within different DDPs, including competition between different information strands and actors of various types. Also, learning is iterative (as in Bayesian updating), so over time, the VOI and DDPs may change, including under adaptive governance and management conditions (Glynn et al., 2017). Our study could also have included consideration of narratives that are essential to human meaning-making and sense-making, to the maintenance and evolution of identities, to the creation and communication of visions for individual and community decisions for action, and to societal change (e.g., Bruner, 1991; Morgan, 2017; Chabay et al., 2019). Lastly, there is a vast literature examining Knowledge to Action frameworks and other behavioral frameworks (Kollmuss and Agyeman, 2002; Heimlich and Ardoin, 2008; Kwon and Silva, 2020; Ward et al., 2020) pertinent to making societal progress on environmental and natural hazard issues that also has much to contribute but could not be explored here.
Natural resource, environmental, and natural/anthropogenic hazard issues faced by society are highly complex and require transdisciplinary science and policy approaches. VOI studies provide one essential way of analyzing information, and assessing its value, useability, and actionability. In doing so, VOI studies enable an analysis of the role of information in DDPs; and can point out behavioral and societal factors that can prevent the effective use of information in policy and management. VOI and DDP studies can then point to possible improvements. While we provide a few examples where some of these factors may be less important and may possibly be ignored, we do not believe that this is generally the case. Our paper documents a wide range of VOI and DDP problems and challenges and suggests ways of identifying and addressing those challenges – at a minimum by recognizing when simplifications of complex realities are not appropriate. A sense of such complexities is provided by Figures 2–4 that describe how barriers, needed feedbacks, and/or actor characteristics and interactions may impede meaningful progressions to decisions and actions.
5 A Discussion Through the Framing of an Art Piece
A re-integration of the “two cultures,” the sciences and the humanities, is needed (Snow, 1959) if modern society is to successfully address the wicked problems (Rittel and Webber, 1973) and complex issues that it faces. Fostering this re-integration, scientists and other communities are increasingly recognizing the role of the arts in 1) helping communicate, elicit, and reconcile different forms of knowledge (Scheffer et al., 2015, 2017; Rathwell and Armitage, 2016; Colavito et al., 2020); 2) in helping human society reconnect with nature (Muhr, 2020); and 3) in advancing transdisciplinary and sustainability science (Steelman et al., 2019; Trott et al., 2020; Heras et al., 2021). We recognize the potential of the arts to elicit critical thinking and greater recognition of human BBHV and societal norms (Glynn, 2014; Glynn, 2017; Glynn et al., 2017; Glynn et al., 2018).
We use a painting, entitled “Living on the side of the world” (Figure 5), to frame the main points of this paper. We point out, in smaller font brackets, elements present in the painting [e.g., feature x], or present in our view in the painting’s “negative space” [NS], an artistic concept that is also finding use in science, including in artificial intelligence (Rahman et al., 2014).
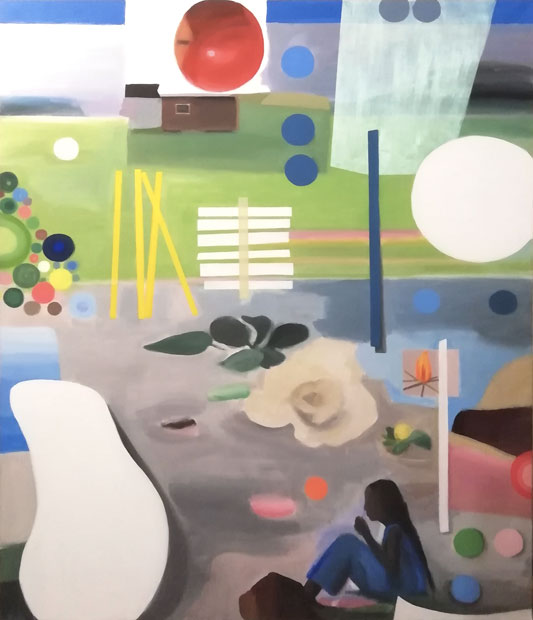
FIGURE 5. “Vivre au bord du monde”: 2020 painting by Anne Emery (http://www.anneemery.fr/). Reproduced here with the permission of the artist.
The economist [meditating lady] uses her System 2 analytical thinking abilities to reflect on the partial realities of a complex issue perceived [entire painting] or intuited to potentially exist [NS]. In pursuit of greater knowledge [apple of knowledge, from which she is distanced], she analyses her most immediate reactions, intuitions, and emotions [gradational or less well defined spaces] to determine whether they are part of her System 1 thinking and reactions, and whether they represent appropriate well-adapted and tested efficiencies (i.e., BBHV, social norms) that allow her to quickly and innately prioritize, judge, and value information and react appropriately. The economist knows that her innate judgments and responses provide an essential ordering of her perspectives [top/bottom ordering]. She understands that System 1 and System 2 thinking represent extremes that cannot be dis-associated [cross-barred vertical fence], and that Rene Descartes’ mind-body dualism was an inherently wrong, but occasionally useful, conceptual construct. She admires Francis Bacon’s contributions [faded crepe-colored rose] to the philosophy of science, including his exploration of the Idols of the Mind. She seeks to understand the effect of these Idols [big white sole on the left] on the thinking and behaviors of individuals and communities, and therefore society’s wicked and complex issues. The economist is pleased [light-throwing fire] that the field of behavioral economics is a now at the vanguard of science, and in the understanding of people. She appreciates that individuals and communities often exhibit cognitive dissonance in their constructed and selective juxtaposition of different beliefs and attitudes towards information, and in their justifications for actions and decisions [the fire sits in the water near the center]. The economist understands that any human assessment of VOI, or use of information, is necessarily affected by these behavioral factors and influences, including human cognitive and processing limitations. There is often (but not always) a difference between what matters and what people perceive to matter.
Furthermore, the economist understands that successfully addressing the complex and novel issues of today (e.g., as part of a DDP), and improving understanding of the potential effects of behavioral influences and human limitations, requires judgments, decisions, and actions, not just by individual(s) but by a well-connected community of stakeholders. Communication between actors is essential. The economist appreciates that her ideas, beliefs, and perspectives and the norms that she follows are influenced by those of the community [large white circle] that she most strongly associates with. She seeks to enhance effective communications relating to the sharing of information, of conceptual models and beliefs, of lines of knowledge [yellow lines] – and of different critically considered judgments of VOI and DDPs – with other actors that she interacts with (or who should be brought in), but that are more “socially distant” [smaller circles; and/or NS]. The economist critically considers what metrics need to be used to 1) effectively prioritize the production, transmission, and use of information; 2) appropriately combine or transform different economic indicators; 3) usefully assess VOI and other measures of value; and 4) improve understanding and implementation of DDPs. Are monetary metrics most appropriate, or would non-monetary metrics be more representative and/or more useful?
The economist understands that there are many barriers to communications, and to assembling useful collectivities to improve DDPs. She can appreciate that some barriers need to be overcome [longer white vertical line blocking the fire], but that others [thick blue line] may need to remain at least temporarily in place. Indeed, the economist knows that the urgency of addressing an issue or quickly changing situation [blowing semi-transparent curtain], and the timeliness requirements of useful VOI and DDP efforts, need to be judged in relation to the time and resources required, but also to the great benefits of including more diverse and larger collectivities. Furthermore, in her assessment (preferably shared and co-created with other actors), the economist realizes that other factors also likely come into play. For example, in the case of diffusely or poorly perceived potential hazards, or of hazards or issues that require understanding of a multiplicity of lines of knowledge or of experiences, will other communities and actors appreciate the urgency for decisions or action? Especially if the issues or hazards involve natural resources and processes [water, air, leaves, yellow flower (with pharmaceutical properties)] that they are not used to thinking about – except, in the moment, when their own homes and livelihoods [house, brown and pink horizontal paths] are endangered by the winds of change [blowing curtain], such as by flooding waters or drought conditions [water, green wetlands].
Lastly, the economist realizes that it would be beneficial, in effectively implementing DDPs, to consider the footstep(s) of history [brown footprint] as well as possible future scenarios [NS] and that doing so may require bringing in other dimensions and frames of reference. Per the title, she sits in a two-dimensional frame, on the side of a fuller reality.
The value of information, like the value of a painting, resides not only in the nature of the facts and artefacts that are represented or communicated, but also in the beliefs and models that are used to interpret, process, and potentially act (or not) on the information. Furthermore, the value(s) estimated or found depend on the actors interacting with or using the information, and their purposes. Information that is broadly communicated and made available as a public good, such as is often the case for geospatial data and information, is likely to have much more value – for the present and future needs of global society – than information that is accessible to only a limited number of actors.
6 Conclusions and Future Developments
Human attention to information, whether individual or collective, is affected by capacity and perception limits but also by behavioral and social factors. Indeed, the seeking and use of information is always accompanied by mental or conceptual models (innate or explicit) that point to the possible purpose of the information; and that also affect the valuation, useability, and actionability of information. These models, even when made explicit, are affected by various human and societal modalities that influence the use of information, and therefore the value of information (VOI). The modalities include the biases, beliefs, heuristics, and values (including norms) that affect the collection of information and accompany its transformation into knowledge – a step needed for (but that may not lead to) translation into decisions and actions. Human modalities that we consider also include lived experiences, as well as the motivations and interactions between diverse actors (individuals and collectivities). Our paper has explored some of these modalities within the context of their effects on VOI studies and data to decision pathways (DDPs). While a synopsis (Table 1) is provided here, a companion paper (Glynn et al., 2022) provides a deeper examination of VOI methods and valuation issues.
Societal support and the involvement of many different actors holding different types of knowledge and expertise is often essential to improving the sharing and valuation of information, and its effective application to complex societal issues, including those related to environmental, natural resource, and natural or anthropogenic hazard issues. In this regard, we have discussed the importance of communications between actors involved in DDPs and information assessments, including certain pathologies and motivations that negatively impact the societal use of information. We suggest that transdisciplinary integration of the sciences and of the humanities, including the use of art to improve communication and collective creative thinking, would be useful.
Effective and societally useful communication of information (and of its value) implies using metrics and indicators that are most meaningful to society. We suggest that monetary metrics are not always the best measures. Non-monetary approaches can add to the understanding of VOI. Monetary metrics can be difficult or impossible to estimate, and they may often be misused or misunderstood by decision makers. We have also discussed the importance of community cognition, and of community cognition events, in generating support for information value and use. In our view, creating knowledge repositories is essential. They allow for the transmission of experiential learning, and for the sharing of information and of the results of economic valuations with communities everywhere.
Looking to the future of knowledge repositories, we are pleased that under the purview of the international Group on Earth Observations (GEO) and its U.S. affiliate USGEO, and with the support of the organizations in the GEOValue community of practice (GEOValue, 2022), an international repository for the societal benefits of geospatial information is under development. While ingestion of case studies into the repository is only starting, we have provided an initial summary of social/behavioral issues and factors that may impact societal realization of the benefits of geospatial (and other) information.
In summary, our paper considers several behavioral and societal factors that affect information valuation, useability, actionability, and data to decision pathways. We believe that understanding these factors and pathways can improve the societal usefulness of VOI studies, and of geospatial and other information.
Author Contributions
All authors contributed to the discussions, examples, tables, figures, and writing of this paper.
Funding
The authors were supported by funds and resources from their affiliated institutions.
Author Disclaimer
Any use of trade, firm, or product names is for descriptive purposes only and does not imply endorsement by the U.S. Government.
Conflict of Interest
The authors declare that the research was conducted in the absence of any commercial or financial relationships that could be construed as a potential conflict of interest.
Publisher’s Note
All claims expressed in this article are solely those of the authors and do not necessarily represent those of their affiliated organizations, or those of the publisher, the editors and the reviewers. Any product that may be evaluated in this article, or claim that may be made by its manufacturer, is not guaranteed or endorsed by the publisher.
Acknowledgments
We owe a debt of gratitude in preparing this manuscript to the insightful comments provided by the three journal reviewers, and also by our colleagues, Patricia McKay (MSU), Meredith Nevers (USGS), and Paul White (GNS Science).
References
Akerlof, G. A., and Kranton, R. E. (2010). Identity Economics How Our Identities Shape Our Work, Wages, and Well-Being. Princeton, NJ: Princeton University Press.
Akerlof, G. A., and Shiller, R. J. (2015). Phishing for Phools: The Economics of Manipulation and Deception. Princeton, NJ: Princeton University Press.
Akerlof, G. A. (1997). Social Distance and Social Decisions. Econometrica 65, 1005. doi:10.2307/2171877
Amos, L. L., Shapiro, C. D., and Zoller, D. H. (1989). Primary Mapping Economic Analysis. United States Geological Survey Yearbook Fiscal Year 1988. Washington, DC: United States Government Printing Office.
Anderson, C. A. (1983). Abstract and concrete Data in the Perseverance of Social Theories: When Weak Data lead to Unshakeable Beliefs. J. Exp. Soc. Psychol. 19, 93–108. doi:10.1016/0022-1031(83)90031-8
Anderson, C. A., Lepper, M. R., and Ross, L. (1980). Perseverance of Social Theories: The Role of Explanation in the Persistence of Discredited Information. J. Personal. Soc. Psychol. 39, 1037–1049. doi:10.1037/h0077720
Anderson, C. R., Berdalet, E., Kudela, R. M., Cusack, C. K., Silke, J., O’Rourke, E., et al. (2019). Scaling up from Regional Case Studies to a Global Harmful Algal Bloom Observing System. Front. Mar. Sci. 6, 250. doi:10.3389/fmars.2019.00250
Ariely, D. (2010). Predictably Irrational: The Hidden Forces that Shape Our Decisions. New York, New York, USA: Harper Perennial.
Bacon, F. (1620). The New Organon (Or True Directions Concerning the Interpretation of Nature).ZUJvb2tzQEFkZWxhaWRlLmVkdS5jb20=(from Taggard and Thompson 1863 edition) (South Australia: The University of Adelaide). Available at: http://ebooks.adelaide.edu.au/b/bacon/francis/organon/complete.html.
Barry, J. M. (1997). Rising Tide: The Great Mississippi Flood of 1927 and How it Changed America. New York: Simon & Schuster.
Bennhold, K. (2021). After Deadly Floods, a German Village Rethinks Its Relationship to Nature New York City, NY: The New York Times. Available at: https://www.nytimes.com/2021/08/06/world/europe/germany-floods-recovery-climate-change-global-warming.html. (Accessed April 13, 2022).
Berardo, R., Turner, V. K., and Rice, S. (2019). Systemic Coordination and the Problem of Seasonal Harmful Algal Blooms in Lake Erie. Ecol. Soc. 24, 24. doi:10.5751/ES-11046-240324
Bernknopf, R., Brookshire, D., Kuwayama, Y., Macauley, M., Rodell, M., Thompson, A., et al. (2018). The Value of Remotely Sensed Information: The Case of a GRACE-Enhanced Drought Severity Index. Weather, Clim. Soc. 10, 187–203. doi:10.1175/WCAS-D-16-0044.1
Bernknopf, R., Kuwayama, Y., Gibson, R., Blakely, J., Mabee, B., Clifford, T. J., et al. (2021). Monetising the Savings of Remotely Sensed Data and Information in Burn Area Emergency Response (BAER) Wildfire Assessment. Int. J. Wildland Fire. 30, 18–29. doi:10.1071/WF19209
Bernknopf, R., and Shapiro, C. (2015). Economic Assessment of the Use Value of Geospatial Information. ISPRS Inter. J. Geo-Inform. 4, 1142–1165. doi:10.3390/ijgi4031142
Bredehoeft, J. D., and Konikow, L. F. (2012). Ground-Water Models: Validate or Invalidate. Ground Water 50, 493–495. doi:10.1111/j.1745-6584.2012.00951.x
Brock, W. A., and Carpenter, S. R. (2007). Panaceas and Diversification of Environmental Policy. Proc. Natl. Acad. Sci. U.S.A. 104, 15206–15211. doi:10.1073/pnas.0702096104
Broughel, J. (2020). Rethinking the Value of Life: A Critical Appraisal of the Value of a Statistical Life. Logan, UT: Center for Growth and Opportunity at Utah State University. Available at: https://www.thecgo.org/research/rethinking-the-value-of-life/ (Accessed February 22, 2022).
Brown, V. A. (2015). Utopian Thinking and the Collective Mind: Beyond Transdisciplinarity. Futures 65, 209–216. doi:10.1016/j.futures.2014.11.004
Carpenter, S. R. (2005). Eutrophication of Aquatic Ecosystems: Bistability and Soil Phosphorus. Proc. Natl. Acad. Sci. U.S.A. 102, 10002–10005. doi:10.1073/pnas.0503959102
Castañeda, J. V., Bronfman, N. C., Cisternas, P. C., and Repetto, P. B. (2020). Understanding the Culture of Natural Disaster Preparedness: Exploring the Effect of Experience and Sociodemographic Predictors. Nat. Hazards 103, 1881–1904. doi:10.1007/s11069-020-04060-2
Chabay, I., Koch, L., Martinez, G., and Scholz, G. (2019). Influence of Narratives of Vision and Identity on Collective Behavior Change. Sustainability 11, 5680–5715. doi:10.3390/su11205680
Chakraborti, D. (2002). Arsenic Calamity in the Indian Subcontinent what Lessons Have Been Learned? Talanta 58, 3–22. doi:10.1016/S0039-9140(02)00270-9
Chiavacci, S. J., Shapiro, C. D., Pindilli, E. J., Casey, C. F., Rayens, M. K., Wiggins, A. T., et al. (2020). Economic Valuation of Health Benefits from Using Geologic Data to Communicate Radon Risk Potential. Environ. Health 19, 1–9. doi:10.1186/s12940-020-00589-8
Colavito, M., Satink Wolfson, B., Thode, A. E., Haffey, C., and Kimball, C. (2020). Integrating Art and Science to Communicate the Social and Ecological Complexities of Wildfire and Climate Change in Arizona, USA, USA. Fire Ecol. 16, 19. doi:10.1186/s42408-020-00078-w
Colten, C. E., and Giancarlo, A. (2011). Losing Resilience on the Gulf Coast: Hurricanes and Social Memory. Environ. Sci. Pol. Sustainable Development 53, 6–19. doi:10.1080/00139157.2011.588548
Colten, C. E., and Sumpter, A. R. (2009). Social Memory and Resilience in New Orleans. Nat. Hazards 48, 355–364. doi:10.1007/s11069-008-9267-x
Cowen, T., and Henderson, D. R. (2022). Public Goods. Concise Encyclopedia Econ. Available at: https://www.econlib.org/library/Enc/PublicGoods.html (Accessed February 21, 2022).
Dallimer, M., Tinch, D., Hanley, N., Irvine, K. N., Rouquette, J. R., Warren, P. H., et al. (2014). Quantifying Preferences for the Natural World Using Monetary and Nonmonetary Assessments of Value. Conservation Biol. 28, 404–413. doi:10.1111/cobi.12215
Damasio, A. R. (1994). Descartes’ Error: Emotion, Reason, and the Human Brain. New York City, NY: Putnam.
Diamond, J. (2004). Collapse: How Societies Choose to Fail or Succeed. New York, New York, USA: Viking Adult.
Dipper, B., Jones, C., and Wood, C. (1998). Monitoring and Post-auditing in Environmental Impact Assessment: A Review. J. Environ. Plann. Management 41, 731–747. doi:10.1080/09640569811399
Elwood, S., Goodchild, M. F., and Sui, D. Z. (2012). Researching Volunteered Geographic Information: Spatial Data, Geographic Research, and New Social Practice. Ann. Assoc. Am. Geogr. 102, 571–590. doi:10.1080/00045608.2011.595657
ESA-ESRIN (2019). There Is Value in Earth Observations: Can You Measure it? European Space Agency. Available at: http://earsc.org/Sebs/workshop-2019.
Evans, J. S. B. T., and Stanovich, K. E. (2013a). Dual-Process Theories of Higher Cognition. Perspect. Psychol. Sci. 8, 223–241. doi:10.1177/1745691612460685
Evans, J. S. B. T., and Stanovich, K. E. (2013b). Theory and Metatheory in the Study of Dual Processing. Perspect. Psychol. Sci. 8, 263–271. doi:10.1177/1745691613483774
Fagin, R., and Halpern, J. Y. (1987). Belief, Awareness, and Limited Reasoning. Artif. Intelligence 34, 39–76. doi:10.1016/0004-3702(87)90003-8
Finocchiaro, M. A. (2010). Defending Copernicus and Galileo: Critical Reasoning in the Two Affairs. Dordrecht: Springer.
Forney, W. M., Raunikar, R. P., Bernknopf, R. L., and Mishra, S. K. (2012). An Economic Value of Remote-Sensing Information — Application to Agricultural Production and Maintaining Groundwater Quality. Reston, VA: USGS Professional Paper 1796.
Gallagher, S. (2013). The Socially Extended Mind. Cogn. Syst. Res. 25-26 (26), 4–12. doi:10.1016/j.cogsys.2013.03.008
Gardner, C. L., Marsden, J. R., and Pingry, D. E. (1993). The Design and Use of Laboratory Experiments for DSS Evaluation. Decis. Support Syst. 9, 369–379. doi:10.1016/0167-9236(93)90047-7
GEOValue (2022). GEOValue community of practice. Available at: https://geovalue.org/ (Accessed April 3, 2022).
Gigerenzer, G. (2007). Gut Feelings: The Intelligence of the Unconscious. New York City, NY: Viking.
Gilmore, B., Ndejjo, R., Tchetchia, A., de Claro, V., Mago, E., Diallo, A. A., et al. (2020). Community Engagement for COVID-19 Prevention and Control: a Rapid Evidence Synthesis. BMJ Glob. Health 5, e003188. doi:10.1136/bmjgh-2020-003188
Glynn, P. D. (2014). “W(h)ither the Oracle ? Cognitive Biases and Other Human Challenges of Integrated Environmental Modeling,” in 7th Intl. Congress on Env. Modelling and Software. Editors D. P. Ames, N. W. T. Quinn, and A. E. Rizzoli (San Diego: International Environmental Modelling and Software Society). doi:10.13140/2.1.3919.8089
Glynn, P. D. (2017). “Integrated Environmental Modelling: Human Decisions, Human Challenges,” in Integrated Environmental Modelling to Solve Real World Problems: Methods, Vision and Challenges. Editors A. T. Riddick, H. Kessler, and J. R. A. Giles (Geological Society of London), 408, 161–182. doi:10.1144/SP408.9
Glynn, P. D., Voinov, A. A., Shapiro, C. D., and White, P. A. (2017). From Data to Decisions: Processing Information, Biases, and Beliefs for Improved Management of Natural Resources and Environments. Earth's Future 5, 356–378. doi:10.1002/2016EF000487
Glynn, P. D., Voinov, A. A., Shapiro, C. D., and White, P. A. (2018). Response to Comment by Walker et al. on "From Data to Decisions: Processing Information, Biases, and Beliefs for Improved Management of Natural Resources and Environments". Earth's Future 6, 762–769. doi:10.1002/2018EF000819
Glynn, P. D., Chiavacci, S. J., Rhodes, C. R., Helgeson, J. F., Shapiro, C. D., and Straub, C. L. (2022). Value of Information: Exploring Behavioral and Social Factors. Front. Environ. Sci. 10. doi:10.3389/fenvs.2022.805245
Goldberg, J. (2017). The Politicization of Scientific Issues. Center For Inquiry Available at: https://web.archive.org/web/20180816182350/https://www.csicop.org/si/show/politicization_of_scientific_issues (Accessed February 24, 2022).
Goldfarb, A., and Tucker, C. (2019). Digital Economics. Cambridge, MA: National Bureau of Economic Research.
Graham-Tomasi, T. (1988). A Theoretical and Empirical Approach to the Value of Information in Risky Markets: A Comment. Rev. Econ. Stat. 70, 543. doi:10.2307/1926799
Greenstone, M. (2021). The Heterogeneous Value of a Statistical Life: Evidence from U.S. Army Reenlistment Decisions. Chicago, IL: University of Chicago Becker Friedman Institute. Available at: bfi.uchicago.edu/working-paper/202175 (Accessed February 23, 2022).
Greifeneder, R., Jaffé, M. E., Newman, E. J., and Schwarz, N. (2020). The Psychology of Fake News: Accepting, Sharing, and Correcting Misinformation. 1st ed. Abingdon, UK: Routledge.
Grossman, J. R. (2018). Dreaming of Disaster: Displacements of Public Memory and hurricane Katrina. Available at: https://getd.libs.uga.edu/pdfs/grossman_jeremy_r_201805_phd.pdf.
Hall, A. B., and Yoder, J. (2022). Does Homeownership Influence Political Behavior? Evidence from Administrative Data. J. Polit. 84, 351–366. doi:10.1086/714932
Hashemi, M. S., Bagheri, A., and Rizzoli, A. E. (2019). The Role of Ex-Post and Ex-Ante Integrated Assessment Frameworks in Conceptualization of the Modeling Process in the Context of Integrated Water Resources Management. Water Resour. 46, 296–307. doi:10.1134/S0097807819020106
Heil, C. A., and Muni-Morgan, A. L. (2021). Florida's Harmful Algal Bloom (HAB) Problem: Escalating Risks to Human, Environmental and Economic Health with Climate Change. Front. Ecol. Evol. 9, 646080. doi:10.3389/fevo.2021.646080
Heimlich, J. E., and Ardoin, N. M. (2008). Understanding Behavior to Understand Behavior Change: a Literature Review. Environ. Education Res. 14, 215–237. doi:10.1080/13504620802148881
Heras, M., Galafassi, D., Oteros-Rozas, E., Ravera, F., Berraquero-Díaz, L., and Ruiz-Mallén, I. (2021). Realising Potentials for Arts-Based Sustainability Science. Sustain. Sci. 16, 1875–1889. doi:10.1007/s11625-021-01002-0
Herlihy, P. H., and Knapp, G. (2003). Maps of, by, and for the Peoples of Latin America. Hum. Organ. 62, 303–314. doi:10.17730/humo.62.4.8763apjq8u053p03
Hoffmann, R., and Muttarak, R. (2017). Learn from the Past, Prepare for the Future: Impacts of Education and Experience on Disaster Preparedness in the Philippines and Thailand. World Development 96, 32–51. doi:10.1016/j.worlddev.2017.02.016
Howard, R. (1966). Information Value Theory. IEEE Trans. Syst. Sci. Cyber. 2, 22–26. doi:10.1109/TSSC.1966.300074
Huebner, B. (2013). Socially Embedded Cognition. Cogn. Syst. Res. 25-26 (26), 13–18. doi:10.1016/j.cogsys.2013.03.006
Huettel, S. A., and Kranton, R. E. (2012). Identity Economics and the Brain: Uncovering the Mechanisms of Social Conflict. Phil. Trans. R. Soc. B 367, 680–691. doi:10.1098/rstb.2011.0264
Hufnagl-Eichiner, S., Wolf, S. A., and Drinkwater, L. E. (2011). Assessing Social–Ecological Coupling: Agriculture and Hypoxia in the Gulf of Mexico. Glob. Environ. Change 21, 530–539. doi:10.1016/j.gloenvcha.2010.11.007
Hyland-Wood, B., Gardner, J., Leask, J., and Ecker, U. K. H. (2021). Toward Effective Government Communication Strategies in the Era of COVID-19. Humanit Soc. Sci. Commun. 8, 30. doi:10.1057/s41599-020-00701-w
Ichikawa, J. J., and Steup, M. (2014). “The Analysis of Knowledge,” in The Stanford Encyclopedia of Philosophy (Spring 2014 Edition. Editor E. N. Zalta. Available at: http://plato.stanford.edu/archives/spr2014/entries/knowledge-analysis/ (Accessed June 2, 2016).
Jacobs, D. E., Kelly, T., and Sobolewski, J. (2007). Linking Public Health, Housing, and Indoor Environmental Policy: Successes and Challenges at Local and Federal Agencies in the United States. Environ. Health Perspect. 115, 976–982. doi:10.1289/ehp.8990
Jørgensen, F., Thomsen, R., Sanderson, P. B. E., and Vangkilde-Pedersen, T. (2013). “Geological Survey of Denmark and Greenland: Early Sketches for a Detailed Nationwide 3D Geological Model Based on Geophysical Data and Boreholes,” in Three-Dimensional Geological Mapping. Editors L. H. Thorleifson, D. Berg, and H. Russell. Minnesota Geological Survey Open File Report OFR-13-2).
Kachanoff, F. J., Bigman, Y. E., Kapsaskis, K., and Gray, K. (2021). Measuring Realistic and Symbolic Threats of COVID-19 and Their Unique Impacts on Well-Being and Adherence to Public Health Behaviors. Soc. Psychol. Personal. Sci. 12, 603–616. doi:10.1177/1948550620931634
Kaul, I., Grunberg, I., and Stern, M. A. (1999). Global Public Goods: International Cooperation in the 21st century. New York: Oxford University Press.
Keisler, J. M., Collier, Z. A., Chu, E., Sinatra, N., and Linkov, I. (2014). Value of Information Analysis: the State of Application. Environ. Syst. Decis. 34, 3–23. doi:10.1007/s10669-013-9439-4
Kinzig, A. P., Ehrlich, P. R., Alston, L. J., Arrow, K., Barrett, S., Buchman, T. G., et al. (2013). Social Norms and Global Environmental Challenges: The Complex Interaction of Behaviors, Values, and Policy. BioScience 63, 164–175. doi:10.1525/bio.2013.63.3.5
Kitchin, R., Collins, S., and Frost, D. (2015). Funding Models for Open Access Digital Data Repositories. Online Inf. Rev. 39, 664–681. doi:10.1108/OIR-01-2015-0031
Kitchin, R., and Lauriault, T. P. (2015). Small Data in the Era of Big Data. GeoJournal 80, 463–475. doi:10.1007/s10708-014-9601-7
Kniesner, T. J., and Viscusi, W. K. (2019). “The Value of a Statistical Life,” in Oxford Research Encyclopedia of Economics and Finance (Oxford University Press). doi:10.1093/acrefore/9780190625979.013.138
Kollmuss, A., and Agyeman, J. (2002). Mind the Gap: Why Do People Act Environmentally and what Are the Barriers to Pro-environmental Behavior? Environ. Education Res. 8, 239–260. doi:10.1080/13504620220145401
Konikow, L. F., and Bredehoeft, J. D. (1992). Ground-water Models Cannot Be Validated. Adv. Water Resour. 15, 75–83. doi:10.1016/0309-1708(92)90033-X
Kranton, R. (2016). Identity Economics 2016: Where Do Social Distinctions and Norms Come From?. Am. Econ. Rev. 106, 405–409. doi:10.1257/aer.p20161038
Kuwayama, Y., Olmstead, S., and Zheng, J. (2022). A More Comprehensive Estimate of the Value of Water Quality. J. Public Econ. 207, 104600. doi:10.1016/j.jpubeco.2022.104600
Kwon, H. R., and Silva, E. A. (2020). Mapping the Landscape of Behavioral Theories: Systematic Literature Review. J. Plann. Lit. 35, 161–179. doi:10.1177/0885412219881135
Laybats, C., and Tredinnick, L. (2020). Knowledge Sharing in a Virtual World. Business Inf. Rev. 37, 140–141. doi:10.1177/0266382120979936
Litwin, E. E., and Feder, M. J. (2014). “European Collective Redress: Lessons Learned from the U.S. Experience,” in Research in Law and Economics. Editor J. Langenfeld (Emerald Group Publishing Limited), 209–248. doi:10.1108/S0193-589520140000026007
Liu, S. B., Poore, B. S., Snell, R. J., Goodman, A., Plant, N. G., Stockdon, H. F., et al. (2014). “USGS iCoast -- Did the Coast Change?,” in Proceedings of the companion publication of the 17th ACM conference on Computer supported cooperative work & social computing (CSCW Companion ’14), 17–20. doi:10.1145/2556420.2556790
Lyre, H. (2018). Socially Extended Cognition and Shared Intentionality. Front. Psychol. 9, 831. doi:10.3389/fpsyg.2018.00831
Macauley, M., and Laxminarayan, R. (2010). The Value of Information: 'Methodological Frontiers and New Applications for Realizing Social Benefit' Workshop. Space Policy 26, 249–251. doi:10.1016/j.spacepol.2010.08.007
McCall, M. K., Martinez, J., and Verplanke, J. (2015). Shifting Boundaries of Volunteered Geographic Information Systems and Modalities : Learning from PGIS. ACME: Int. E-Journal Crit. Geographies. 14, 791–826.
McKee, S. (2021). Remembering the Big Thompson Canyon Flood, Colorado’s Deadliest Natural Disaster. OutThere Colo. Available at: https://www.outtherecolorado.com/features/remembering-the-big-thompson-canyon-flood-colorado-s-deadliest-natural-disaster/article_5f5fd6a9-c97c-5083-815e-53ed9106f3ec.html (Accessed October 24, 2021).
Meyer, R., and Kunreuther, H. (2005). An Experimental Analysis of Learning from Experience about Natural-Hazards. Available at: https://repository.upenn.edu/marketing_papers/330.
Molder, E. B., Schenkein, S. F., McConnell, A. E., Benedict, K. K., and Straub, C. L. (2022). Landsat Data Ecosystem Case Study: Actor Perceptions of the Use and Value of Landsat. Front. Environ. Sci. 9, 805174. doi:10.3389/fenvs.2021.805174
Morgan, M. S. (2017). Narrative Ordering and Explanation. Stud. Hist. Philos. Sci. A 62, 86–97. doi:10.1016/j.shpsa.2017.03.006
Muhr, M. M. (2020). Beyond Words - the Potential of Arts-Based Research on Human-Nature Connectedness. Ecosyst. People 16, 249–257. doi:10.1080/26395916.2020.1811379
Nordstrom, D. K. (2012). Models, Validation, and Applied Geochemistry: Issues in Science, Communication, and Philosophy. Appl. Geochem. 27, 1899–1919. doi:10.1016/j.apgeochem.2012.07.007
OMB (2003). OMB Circular A-4, Regulatory Analysis. Available at: https://www.whitehouse.gov/omb/information-for-agencies/circulars/.
Oreskes, N., and Conway, E. M. (2010). Merchants of Doubt: How a Handful of Scientists Obscured the Truth on Issues from Tobacco Smoke to Global Warming. Bloomsbury.
Padmanaban, D. (2021). Water of Death: How Arsenic Is Poisoning Rural Communities in India. The Guardian. Available at: https://www.theguardian.com/global-development/2021/jun/24/water-of-death-how-arsenic-is-poisoning-rural-communities-in-india (Accessed October 24, 2021).
Paerl, H. W., Fulton, R. S., Moisander, P. H., and Dyble, J. (2001). Harmful Freshwater Algal Blooms, with an Emphasis on Cyanobacteria. The Scientific World JOURNAL 1, 76–113. doi:10.1100/tsw.2001.16
Paperwork Reduction Act (2015). U.S. Public Law No. 96-511, 94 Stat. 2812, codified at 44 U.S.C.§§ 3501–3521. Digital.gov. Available at: https://digital.gov/resources/paperwork-reduction-act-44-u-s-c-3501-et-seq/(Accessed April 2, 2022).
Pitt, M. M., Rosenzweig, M. R., and Hassan, M. N. (2021). Identifying the Costs of a Public Health Success: Arsenic Well Water Contamination and Productivity in Bangladesh. Rev. Econ. Stud. 88, 2479–2526. doi:10.1093/restud/rdaa087
Plato (369 BCE). Theaetetus, in The Dialogues of Plato, pp. 1–124, Project Gutenberg [B. Jowett 1892 transl.]. Available at: http://www.gutenberg.org/ebooks/1656.
Pohl, J. (2016). 40 Years Later: Scores Killed in Big Thompson Flood. The Coloradoan. Available at: https://www.coloradoan.com/story/news/2016/07/29/big-thompson-flood-killed-scores/87524858/ (Accessed October 24, 2021).
Polanyi, M. (1962). Personal Knowledge: Towards a post-critical philosophyReprint. London: Routledge & Kegan Paul.
Ponting, C. (2007). A New green History of the World: The Environment and the Collapse of Great Civilizations. Rev. New York: Penguin Books.
Powell, W. W., and Snellman, K. (2004). The Knowledge Economy. Annu. Rev. Sociol. 30, 199–220. doi:10.1146/annurev.soc.29.010202.100037
Rahman, S. A., Song, I., Leung, M. K. H., Lee, I., and Lee, K. (2014). Fast Action Recognition Using Negative Space Features. Expert Syst. Appl. 41, 574–587. doi:10.1016/j.eswa.2013.07.082
Raiffa, H. (1970). Decision Analysis: Introductory Lectures on Choices under Uncertainty. 2. Print. Reading, Mass: Addison-Wesley.
Rathwell, K. J., and Armitage, D. (2016). Art and Artistic Processes Bridge Knowledge Systems about Social-Ecological Change: An Empirical Examination with Inuit Artists from Nunavut, Canada. Ecol. Soc. 21, 21. doi:10.5751/es-08369-210221
Refsgaard, J. C., Højberg, A. L., Møller, I., Hansen, M., and Søndergaard, V. (2010). Groundwater Modeling in Integrated Water Resources Management-Visions for 2020. Ground Water 48, 633–648. doi:10.1111/j.1745-6584.2009.00634.x
Rhee, C. H., and Harsagi, V. (2014). “Collective Redress in the European Union: Comparative Perspectives,” in Multi-Party Redress Mechanisms InEurope: Squeaking Mice? Ius Commune Europaeum (Cambridge: Intersentia).
Rittel, H. W. J., and Webber, M. M. (1973). Dilemmas in a General Theory of Planning. Policy Sci 4, 155–169. doi:10.1007/BF01405730
Rosenfeld, D. L., Balcetis, E., Bastian, B., Berkman, E. T., Bosson, J. K., Brannon, T. N., et al. (2021). Psychological Science in the Wake of COVID-19: Social, Methodological, and Metascientific Considerations. Perspect. Psychol. Sci. 17, 311–333. doi:10.1177/1745691621999374
Scheffer, M., Baas, M., and Bjordam, T. K. (2017). Teaching Originality? Common Habits behind Creative Production in Science and Arts. Ecol. Soc. 22, 29. doi:10.5751/ES-09258-220229
Scheffer, M., Bascompte, J., Bjordam, T. K., Carpenter, S. R., Clarke, L. B., Folke, C., et al. (2015). Dual Thinking for Scientists. Ecol. Soc. 20, 3. doi:10.5751/es-07434-200203
Schultz, P. W., Milfont, T. L., Chance, R. C., Tronu, G., Luís, S., Ando, K., et al. (2014). Cross-Cultural Evidence for Spatial Bias in Beliefs about the Severity of Environmental Problems. Environ. Behav. 46, 267–302. doi:10.1177/0013916512458579
Simon, H. A. (1971). “Designing Organizations for an Information-Rich World,” in Computers, Communications, and the Public Interest (John Hopkins Press).
Smith, A. H., Hopenhayn-Rich, C., Bates, M. N., Goeden, H. M., Hertz-Picciotto, I., Duggan, H. M., et al. (1992). Cancer Risks from Arsenic in Drinking Water. Environ. Health Perspect. 97, 259–267. doi:10.1289/ehp.9297259
Smith, A. H., Lingas, E. O., and Rahman, M. (2000). Contamination of Drinking-Water by Arsenic in Bangladesh: A Public Health Emergency. Geneva, Switzerland: Bulletin of the World Health Organization 78, 1093–1103.
Spash, C. L. (2007). Deliberative Monetary Valuation (DMV): Issues in Combining Economic and Political Processes to Value Environmental Change. Ecol. Econ. 63, 690–699. doi:10.1016/j.ecolecon.2007.02.014
Steelman, T. A., Andrews, E., Baines, S., Bharadwaj, L., Bjornson, E. R., Bradford, L., et al. (2019). Identifying Transformational Space for Transdisciplinarity: Using Art to Access the Hidden Third. Sustain. Sci. 14, 771–790. doi:10.1007/s11625-018-0644-4
Strassheim, H., and Kettunen, P. (2014). When Does Evidence-Based Policy Turn into Policy-Based Evidence? Configurations, Contexts and Mechanisms. Evid. Policy 10, 259–277. doi:10.1332/174426514X13990433991320
Stroming, S., Robertson, M., Mabee, B., Kuwayama, Y., and Schaeffer, B. (2020). Quantifying the Human Health Benefits of Using Satellite Information to Detect Cyanobacterial Harmful Algal Blooms and Manage Recreational Advisories in U.S. Lakes. GeoHealth 4, 17. doi:10.1029/2020GH000254
Tomlinson, P., and Atkinson, S. F. (1987). Environmental Audits: A Literature Review. Environ. Monit. Assess. 8, 239–261. doi:10.1007/BF00404267
Tóth, B. (2017). Collective Redress as New Institution of Civil Procedure Code and its Applicability to Protect Environment. JAEL 12, 182–207. doi:10.21029/JAEL.2017.23.182
Trott, C. D., Even, T. L., and Frame, S. M. (2020). Merging the Arts and Sciences for Collaborative Sustainability Action: a Methodological Framework. Sustain. Sci. 15, 1067–1085. doi:10.1007/s11625-020-00798-7
Turnbull, D. (2007). Maps Narratives and Trails: Performativity, Hodology and Distributed Knowledges in Complex Adaptive Systems ? an Approach to Emergent Mapping. Geographical Res. 45, 140–149. doi:10.1111/j.1745-5871.2007.00447.x
US EPA (2014). Mortality Risk Valuation. EPA’s Mortality Risk Valuation And Estimates of the “Value Of a Statistical Life. Washington, DC: United States Environmental Protection Agency. Available at: https://www.epa.gov/environmental-economics/mortality-risk-valuation (Accessed October 28, 2021).
US EPA (2006). Report of the EPA Workgroup on VSL Meta-Analysis. Washington, DC: United States Environmental Protection Agency.
Van Dolah, E. R., Paolisso, M., Sellner, K., and Place, A. (2016). Employing a Socio-Ecological Systems Approach to Engage Harmful Algal Bloom Stakeholders. Aquat. Ecol. 50, 577–594. doi:10.1007/s10452-015-9562-z
Van Meter, K. J., Basu, N. B., and Van Cappellen, P. (2017). Two Centuries of Nitrogen Dynamics: Legacy Sources and Sinks in the Mississippi and Susquehanna River Basins. Glob. Biogeochem. Cycles 31, 2–23. doi:10.1002/2016GB005498
Viscusi, W. K., and Masterman, C. J. (2017). Income Elasticities and Global Values of a Statistical Life. J. Benefit Cost Anal. 8, 226–250. doi:10.1017/bca.2017.12
Viscusi, W. K. (2010). The Heterogeneity of the Value of Statistical Life: Introduction and Overview. J. Risk Uncertain 40, 1–13. doi:10.1007/s11166-009-9083-z
Wachinger, G., Renn, O., Begg, C., and Kuhlicke, C. (2013). The Risk Perception Paradox-Implications for Governance and Communication of Natural Hazards. Risk Anal. 33, 1049–1065. doi:10.1111/j.1539-6924.2012.01942.x
Wachinger, G., and Renn, O. (2010). Risk Perception and Natural Hazards. Stuttgart: DIALOGIK Non-Profit Institute. for Communication and Cooperative Research.
Wagner, P. G. (2011). Collective Redress – Categories of Loss and Legislative Options. L. Q. Rev. 127, 55-82.
Ward, P. J., Blauhut, V., Bloemendaal, N., Daniell, J. E., de Ruiter, M. C., Duncan, M. J., et al. (2020). Review Article: Natural hazard Risk Assessments at the Global Scale. Nat. Hazards Earth Syst. Sci. 20, 1069–1096. doi:10.5194/nhess-20-1069-2020
Watson, W., Shapiro, C. D., and Bernknopf, R. F. (1984). Costs of Geologic Information in the Exploration for Minerals: A Case Study of Porphyry Copper. J. Resour. Manag. Technol. 13(2), 97–110.
WHO Arsenic Key Facts (2018). WHO Arsenic Fact Sheet. Available at: https://www.who.int/news-room/fact-sheets/detail/arsenic (Accessed October 24, 2021).
Wilson, M. W. (2015). Paying Attention, Digital media, and Community-Based Critical GIS. cultural geographies 22, 177–191. doi:10.1177/1474474014539249
Wolf, D., and Klaiber, H. A. (2017). Bloom and Bust: Toxic Algae's Impact on Nearby Property Values. Ecol. Econ. 135, 209–221. doi:10.1016/j.ecolecon.2016.12.007
World Bank Group (2016). World Development Report 2016: Digital Dividends. Washington, DC: World Bank.
Wu, Z., Snyder, G., Vadnais, C., Arora, R., Babcock, M., Stensaas, G., et al. (2019). User Needs for Future Landsat Missions. Remote Sensing Environ. 231, 111214. doi:10.1016/j.rse.2019.111214
Xu, L., Shen, J., Marinova, D., Guo, X., Sun, F., and Zhu, F. (2013). Changes of Public Environmental Awareness in Response to the Taihu Blue-green Algae Bloom Incident in China. Environ. Dev. Sustain. 15, 1281–1302. doi:10.1007/s10668-013-9440-6
Yale Economic Growth Center (2020). New Findings on the Effects of Widespread Arsenic Poisoning in Bangladesh. Yale Economic Growth Center. Available at: https://egc.yale.edu/research/Rosenzweig_et_al_2020 (Accessed October 24, 2021).
Keywords: value of information, biases, decision science, geospatial information, natural resources, environmental issues, hazards, health risks
Citation: Glynn PD, Rhodes CR, Chiavacci SJ, Helgeson JF, Shapiro CD and Straub CL (2022) Value of Information and Decision Pathways: Concepts and Case Studies. Front. Environ. Sci. 10:805214. doi: 10.3389/fenvs.2022.805214
Received: 30 October 2021; Accepted: 06 April 2022;
Published: 20 May 2022.
Edited by:
Nnaemeka Vincent Emodi, University of Queensland, AustraliaReviewed by:
Uta Wehn, University of Gothenburg, SwedenJoseph Conran, National Oceanic and Atmospheric Administration (NOAA), United States
James Jarvis, Canadian Space Agency, Canada
Copyright © 2022 Glynn, Rhodes, Chiavacci, Helgeson, Shapiro and Straub. This is an open-access article distributed under the terms of the Creative Commons Attribution License (CC BY). The use, distribution or reproduction in other forums is permitted, provided the original author(s) and the copyright owner(s) are credited and that the original publication in this journal is cited, in accordance with accepted academic practice. No use, distribution or reproduction is permitted which does not comply with these terms.
*Correspondence: Pierre D. Glynn, cGdseW5uQHVzZ3MuZ292, cGdseW5uMkBhc3UuZWR1