- 1College of Hydrology and Water Resources, Hohai University, Nanjing, China
- 2Australian AI Institute, University of Technology Sydney, Sydney, NSW, Australia
- 3Bureau of Hydrology, ChangJiang Water Resources Commission, Wuhan, China
Editorial on the Research Topic
Artificial Intelligence Methods for Water-Environment-Food-Energy Nexus
In the past few decades, the urbanization process and global population have continuously increased with the high-speed economic development. As a result, huge systems (like water, environment, food, and energy) that are strongly associated with the human society are becoming increasingly interconnected, posing a series of unprecedented challenges and opportunities. It is of great importance to better understand the intrinsic connection of four systems from the nexus perspective since the water–environment–food–energy nexus can help address global problems, like flood control, water crises, energy shortage, and ecological damage. Nevertheless, the growing climate changes and human activities have increased the identification difficulties of the complex system. By gaining potential knowledge from a mass of samples, the emerging artificial intelligence methods with the merits of high applicability and strong robustness are enjoying increasing popularity in many practical applications. Thus, artificial intelligence approaches can contribute to the research studies between water, food, environment, and energy systems under rapidly changing environments.
As shown in Figure 1, the water–environment–food–energy nexus involves a variety of interdisciplinary knowledge and research fields. This research topic aims to provide an opportunity for scholars to share their latest research findings related to advanced artificial intelligence approaches for the water–environment–food–energy nexus. It collects five papers that cover a variety of problems in the water–environment–food–energy nexus field. The specific work and related findings of each article are briefly given as follows.
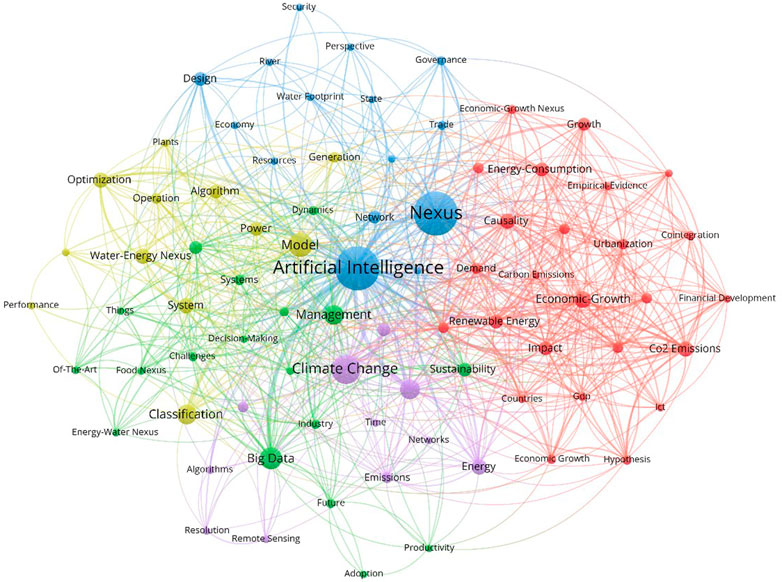
FIGURE 1. Sketch map of artificial intelligence methods for the water–environment–food–energy nexus.
Wu et al. investigated the trade-offs in the water–energy–ecosystem nexus for cascade hydropower systems in Yalong River, China. A multi-objective optimization model is developed by considering the maximization of cascade power generation and the minimization of both the reservoir water footprint and amended annual proportional flow deviation. The third edition of the non-dominated sorting genetic algorithm is used to find Pareto solutions, while the multi-objective trade-off index is developed to explore the trade-offs of different objectives. The results indicate that: 1) the incoming water has a positive correlation with power generation and ecological benefits of the hydropower system; and 2) the trade-off degrees of the water sector in the energy ecosystem and energy sector in the water ecosystem decrease as the hydrological condition changes from wet to dry, while the ecosystem sector degree in the water–energy ecosystem increases.
Xu et al. investigated the suitability evaluation of the water–energy–food (WEF) system in Sichuan Province, China. A comprehensive model considering stability, coordination, and sustainability is developed for the WEF system suitability evaluation. The results show that 1) the suitability level of the WEF system in Sichuan is above medium and shows a fluctuating trend; 2) the sustainability of the WEF system increases but the coordination decreases, posing the coordination significance of the three resources; 3) the improvement of four indicators enhances the adaptability level of the water–energy–grain system; and 4) by linking the network analysis method with Amal’s method, a combined subjective and objective weight calculation method can effectively characterize the nexus of WEF systems.
Li et al. developed a hybrid method for monthly runoff forecasting based on the ensemble empirical mode decomposition (EEMD), sparrow search algorithm (SSA), and long short-term memory (LSTM) neural networks. After dividing the original runoff series into several sub-sequences by EEMD, LSTM is used to construct the forecasting model for each sub-sequence, while the hyperparameters are optimized by SSA. The final result is equal to the total simulated results of all the optimized models. The simulated results indicate that the proposed model is better than several benchmark models, yielding satisfactory simulation results and improving the forecast accuracy.
Zamri et al. utilized an integrated interval type-2 fuzzy VIKOR with unsupervised machine learning to find the best strategies of water security for water source areas. Specially, the IT2FVIKOR method is used for ranking and selecting a set of alternatives; hierarchical clustering, SOM, and autoencoder are used for clustering, while the silhouette analysis and elbow method are used to find the most optimal cluster count; finally, the adjusted rank index is developed to determine the best comparison in two clusters. The results demonstrate the feasibility of the proposed method.
Lan et al. developed an optimized flood control method for the Three Gorges Reservoir, considering evolving flood propagation trends in the Jingjiang Reach of the Yangtze River. In order to guarantee effective flood control management, a hydraulic model is developed for simulating the potential causes and impacts. By considering various management schemes, the observed flood events in 2016 and 2017 are simulated in the developed hydraulic model, while the improved flood propagation trends along the lower reach are analyzed. Based on the simulations, the suggestions on optimizing the operation scheme of the Three Gorges Reservoir are given to reduce flood damage and protect flood safety.
All papers published in this research topic have gone through strict review processes under the support and supervision of the editorial office. The research works mentioned previously investigate various aspects of artificial intelligence methods for the water–environment–food–energy nexus, like engineering computation, mathematical modeling, and simulated analysis. Guest editors hope that this research topic can be conducive to deepen the understanding on the water–environment–food–energy nexus. Moreover, guest editors believe that with the rapid scientific and technological progress, more effective models, robust methods, and new cognition for the water–environment–food–energy nexus will appear constantly in the coming decades.
Author contributions
All authors listed have made a substantial, direct, and intellectual contribution to the work and approved it for publication.
Conflict of interest
The authors declare that the research was conducted in the absence of any commercial or financial relationships that could be construed as a potential conflict of interest.
Publisher’s note
All claims expressed in this article are solely those of the authors and do not necessarily represent those of their affiliated organizations, or those of the publisher, the editors, and the reviewers. Any product that may be evaluated in this article, or claim that may be made by its manufacturer, is not guaranteed or endorsed by the publisher.
Keywords: artificial intelligence, machine learning, water–environment–food–energy nexus, multi-objective optimization, engineering optimization
Citation: Feng Z-k, Wen S-p and Niu W-j (2023) Editorial: Artificial intelligence methods for the water- environment-food-energy nexus. Front. Environ. Sci. 10:1118406. doi: 10.3389/fenvs.2022.1118406
Received: 07 December 2022; Accepted: 12 December 2022;
Published: 04 January 2023.
Edited and reviewed by:
Alexander Kokhanovsky, German Research Centre for Geosciences, GermanyCopyright © 2023 Feng, Wen and Niu. This is an open-access article distributed under the terms of the Creative Commons Attribution License (CC BY). The use, distribution or reproduction in other forums is permitted, provided the original author(s) and the copyright owner(s) are credited and that the original publication in this journal is cited, in accordance with accepted academic practice. No use, distribution or reproduction is permitted which does not comply with these terms.
*Correspondence: Zhong-kai Feng, bXlmZWxsb3dAMTYzLmNvbQ==