- 1College of Land Science and Technology, China Agricultural University, Beijing, China
- 2Key Laboratory of Urban Land Resources Monitoring and Simulation, Ministry of Natural Resources, Shenzhen, China
The Loess Plateau exemplifies the type of ecologically fragile region that faces severe poverty challenges in China. Orchards have expanded rapidly over the past few decades and now constitute a considerable part of local economy. Not only do the characteristics of orchard expansion affect local economic development, but also exert additional pressure on the ecological environment. Therefore, it is essential for sustainable development on the Loess Plateau to investigate the characteristics and driving forces of orchard expansion. The Fuxian, Luochuan, Huangling, three typical orchard planting counties were chosen as the study area. Firstly, the orchard was extracted from the land use/cover classification from 1990–2020. It broadens the research approach to the identification of expansion cash crops by using the combination of linear spectral mixture analysis (LSMA) and decision tree. Secondly, the spatiotemporal dynamics of orchard expansion were quantitatively investigated based on spatial geometry center shift, physical geographical features, landscape pattern and orchard planting suitability. Then, we constructed an evaluation indicators system to detect the feature importance and partial dependence of different factors by random forest regression. It is more innovative to employ the machine learning method to investigate driving forces. Finally, the linkages between planting suitability and orchard expansion were further discussed, and subsequent policies were proposed. Findings demonstrated the orchard had continuously expanded over the past 30 years, with the fastest expansion rate during 1990–2005. Increased cohesion was accompanied by a shift in the orchard’s spatial distribution to the north central region and highly suitable planting regions. Slope turned out to be the primary factor affecting the orchard expansion. In the future, regions with aging orchard but high planting suitability should be the preferred choice for orchard expansion. Additionally, the transportation connectivity and governmental assistance are crucial considerations for the future planning of the orchard.
1 Introduction
The Loess plateau (LP) is a globally well-recognized ecological fragile region (Fu et al., 2017; Fu, 2022), with severe social-ecological issues, including soil erosion (Zhou et al., 2016; Lu et al., 2022), water shortage (Feng et al., 2016) and poverty (Patrick, 2016). The conflict between socioeconomic development and environmental protection is a crucial challenge that needs urgent solutions. Orchard planting is characterized by high economic benefits and now serves as the backbone of the local economy in the LP (Chen et al., 2021). In addition, with the improvement of the dietary structure (Guo et al., 2022), the LP, one of the largest apple planting regions, has gone through significant orchard expansion in recent decades (Yang et al., 2020). Thus, the spatiotemporal dynamics of agricultural land will not only affect the local socio-economy, but also on its ecological condition.
Previous researches on agricultural land in the LP has focused on its economic benefits and associated environmental effects along with the land use/cover change (Zhao et al., 2018; Liang et al., 2018; Li et al., 2021; Huo et al., 2022; Xiao et al., 2022). There are many excellent reviews promoting sustainable agricultural land management in the LP, which also provide valuable instruction for socioeconomic development. However, the existing studies paid much attention to traditional food crops (Tracy, 2020; Wang et al., 2022a; Wang et al., 2022b; Chen et al., 2022; Wu et al., 2022; Wang and Cheng, 2022), while cash crops still remained unclear. Consequently, it is necessary to explore the spatiotemporal dynamics of orchard expansion and its driving forces in order to propose optimizing strategies for orchard planting in the LP.
Much work so far in terms of orchard expansion can be divided into three aspects: 1) identification of orchard expansions (Dong et al., 2013; Jason et al., 2017; Sun et al., 2017; Kaspar and Jefferson, 2018; Lin et al., 2022). Depending on multiple spectral indices and phenological information, much attention has been paid to identifying the expansion of different orchards. Zhu et al. (2020) extracted apple orchards in Qixia county from 2000–2017 by using normalized difference vegetation index (NDVI) time series and phenological vegetation information. Xuan et al. (2018) applied the random forest method to extract citrus orchards by using NDVI, soil-adjusted vegetation index (SAVI) and normalized difference moisture index (NDMI). Based on the phenological difference. The selection of the appropriate spectral indices and the extraction of the corresponding phenological information is a major challenge for different regions and different fruit tree species; 2) analysis on the characteristics of orchard expansion, including landscape pattern (Guzmán et al., 2022), dynamics (Dibs et al., 2017; Peña-Cortés et al., 2021) and planting suitability (Wu et al., 2022). As for analyzing the characteristics of orchard expansion, current studies are based on a single perspective. Naqash and Wani. (2019) discovered the spatiotemporal dynamics of orchard expansion in Kashmir Valley and proposed policy suggestions from the perspective of pest control. Guzmán et al. (2022) analyzed the landscape pattern of olive orchards expansion in Spain. A comprehensive investigation of the spatiotemporal dynamics of orchard expansion based on multiple perspectives is still lacking; 3) driving mechanism of orchard expansion (Xiao et al., 2015; Zhang et al., 2017). Driving force analysis based on statistical regression models (e.g., logistic regression model) is a common method for analyzing the driving mechanisms of orchard expansion. Although abundant potential determinants have been selected to figure out the driving mechanism, the underlying response mechanism is not further used to suggest new policy actions. More effort is needed to determine how to provide sustainable orchard management practices based on the understanding of the driving forces.
In general, scholars have made certain achievements in the study of orchard expansion. However, the comprehensive exploration of orchard expansion dynamics in the ecological fragile region and their driving forces are still lacking. Northern Shaanxi is one of the most vital regions for orchard cultivation in the LP. Therefore, this study takes the three typical apple orchard planting counties as the study area. The spatiotemporal dynamics of orchard expansion have been analyzed from the perspectives of spatial geometry center shift, physical geographical features, landscape pattern and orchard planting suitability. It broadens the research approach to the identification of expansion cash crops in ecologically vulnerable areas. Furthermore, it is more innovative to employ the machine learning method to investigate driving forces and put forward matching policies in order to promote the apple industry in the LP.
The specific objectives of this study are: 1) to map the land use/cover classification of the study area and identify orchard expansion from 1990 to 2020; 2) to analyze the spatiotemporal dynamics of orchard expansion based on the spatial geometry center shift, physical geographical features, landscape pattern and orchard planting suitability; 3) to reveal the potential determinants and analyze its driving forces based on the parameter of feature importance and partial dependence; 4) to propose optimistic strategies for local orchard expansion based on above findings for better agricultural land management and ecological conservation.
2 Materials and methods
2.1 Overall workflow
Figure 1 shows the overall workflow of this study. The whole research is carried out in two steps. Firstly, based on the three temporal remote sensing data, the land use/cover classification of the study area from 1990 to 2020 was obtained by using a combination of the LSMA and the decision tree. The spatial distribution of orchard expansion was then available by extracting the orchards. Secondly, the spatiotemporal dynamics of orchard expansion were investigated from the perspectives of spatial geometry center shift, physical geographic features, landscape pattern and planting suitability. Combined with the constructed evaluation system of influencing factors, the feature importance and partial dependence of different factors were analyzed by using random forest regression.
2.2 Study area
The counties of Fuxian, Luochuan and Huangling in Yan’an City, Shaanxi Province were selected as the study area (Figure 2). The three counties are situated between 108°29′–109°42′N and 35°44′–36°23′E, covering an area of 8,278 km2. These counties are located in the middle part of the LP, where the ecological environment is fragile and soil erosion is serious. This area belongs to the temperate continental monsoon climate, with sufficient sunlight and pleasant weather. The terrain in the study area is complex, including river valley terrace, low hills and loess valleys. The local government attaches great importance to the investment and policy support of the apple industry, guiding the farmers and promoting the fruit industry. At present, this area has become one of the largest apple production areas in China, of which Luochuan county’s apple yields rank the first, known as the “apple capital”.
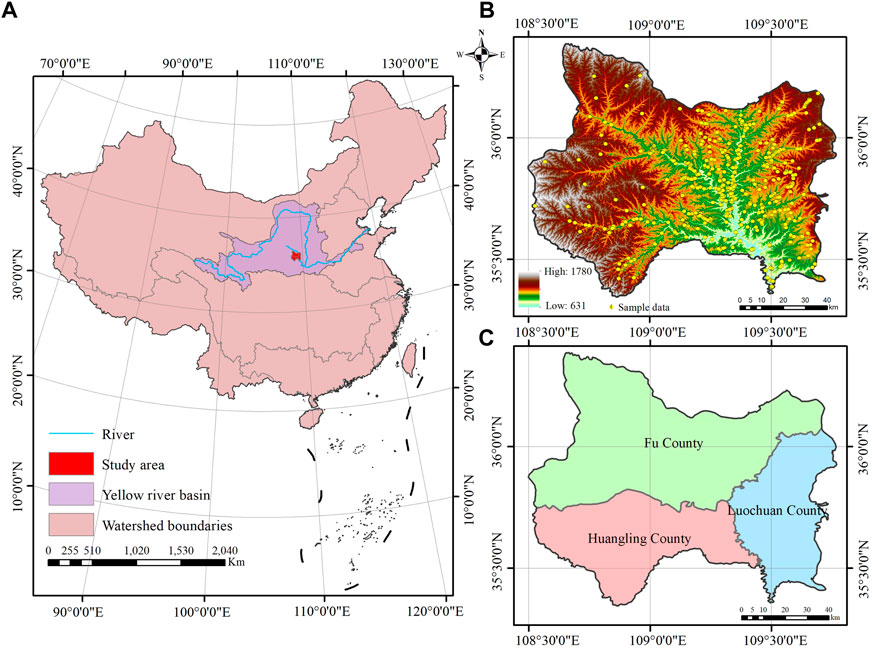
FIGURE 2. Study area and its associated information. (A) Location of the study area in the Losses Plateau; (B) Topography of the study area and samples on the field; (C) The three typical counties in the study area.
2.3 Data source
2.3.1 Satellite data
In this study, the surface reflectance data from Landsat TM/ETM +/OLI C2L2 products with radiometric calibration, geometric correction, and atmospheric correction were used. The Landsat satellite images have a 16-day return time and 30 m spatial resolution, which are available from 1972 to the present (Roy et al., 2014) and have been widely used in land use/cover classification (Azzari and Lobell, 2017; Jin et al., 2019; Souza et al., 2020). All images are freely available on the website of the United States Geological Survey (https://earthexplorer.usgs.gov). In this study, the “landsat_gapfll.sav” plug in of ENVI version 5.3 software (Exelis Visual Information Solutions, Inc., Boulder, CO, United States) was used to compensate for the loss of ETM + data. The cloud coverage of the images used in this study was less than 8%, and the cloud areas were not in the study area. Thus, no additional cloud removal processing was required.
In the study area, the periods of significant physical characteristics of the orchards include: 1) growth period (April-May): the period when fruit trees start to sprout and grow leaves; 2) peak period (August-September): the period when fruit trees have the largest green leaf area; 3) deciduous period (October-November): the period when most deciduous forests have turned yellow and deciduous, while fruit trees are still covered with green leaves without significant deciduousness. To distinguish different land types in the study area, Landsat satellite images for April, August and October of 1990, 1995, 2000, 2005, 2010, 2015, and 2020 were used in this study, among which Landsat5 TM images were used for 1990, 1995, 2005, and 2010, Landsat7 ETM+ images were used for 2000, and Landsat8 OLI images were used for 2015 and 2020. The images used in this study are shown in Table 1.
2.3.2 Field data
To obtain reference samples for decision tree construction and validation samples for land use/cover classification, we collected field data in July 2021, using a portable GPS satellite positioning navigator to collect the geographic coordinates and the land use/cover type. In addition, we selected sample sites for different land use/cover types that have not changed between 1990 and 2020 based on visual interpretation of high-resolution historical images from Google Earth. In the study area, we selected 758 sample points of various types, including 167 orchards, 162 cultivated land, 197 forest land, 47 grassland, 119 water, 18 bare land, and 48 construction land (Figure 1B).
2.4 Orchard expanding mapping
2.4.1 Linear spectral mixture analysis
The theoretical assumption of the LSMA is that the radiant brightness of the ground target pixel received by the sensor is only related to the proportion of the area occupied by each element (Li et al., 2021). Therefore, the reflectance of the ground target pixel is a linear combination of each endmember reflectance within the pixel, with the proportion of the area occupied by each endmember as a weight (Small, 2003). In the unit pixel, endmember abundance values (EMA) are calculated by the area percentage of each standard endmember at the subpixel level based on the pixel spectrum. The EMA with specific physical meaning, in contrast to other traditional vegetation indices, such as NDVI, NDMI and SAVI, can better reflect the information of landscape features (such as plants, soil, water, and so on) (Li et al., 2021). The selection of appropriate spectral indices is a major challenge for different regions when conducting land use/cover mapping. Thus, using the LSMA to convert raw spectral data from remote sensing images into physically meaningful EMA is beneficial to land use/cover classification.
Before the LSMA, there are two critical steps: principal component analysis (PCA) and endmember selection. Firstly, we used the PCA method to extract the main feature information of spectral space. Taking the PCA method results in 2020 as an example, the cumulative contribution of the top three principal components in three seasonal images exceeded 98% (Table 2), showing that the top three principal components of all images contained nearly all the spectral information. Consequently, we can determine that the intrinsic dimension of spectral information is three. According to the convex geometry theory, the number of endmembers is generally one more than the intrinsic dimension of the spectral space (Boardman, 1993). Therefore, we can select four pure spectral endmembers in the study area. Secondly, we built scatter grams based on the top three principal components to select pure endmembers. According to the spectral curve of points at the top of the scatter grams and the true color composite images, we determined four endmembers in the study area: soil (SL, rock and soil), green vegetation (GV, green photosynthetic leaves), dark (DA, shadow of mountains and vegetation) and water (WA).
There will be slight differences when extracting the endmember spectrum from the images of different satellite sensors (Han et al., 2022). To limit the influence of different sensors on the LSMA, we used the average values of six common bands (blue, green, red, near-infrared, and shortwave-infrared 1/2) from three data sources to construct a standard endmember library (Figure 3A). These endmember spectra have been proven to be effective and stable (Sun and Liu, 2015; Sun et al., 2019). Finally, we estimate the EMA through the LSMA. The EMA results in 2020 are shown in Figure 3B.
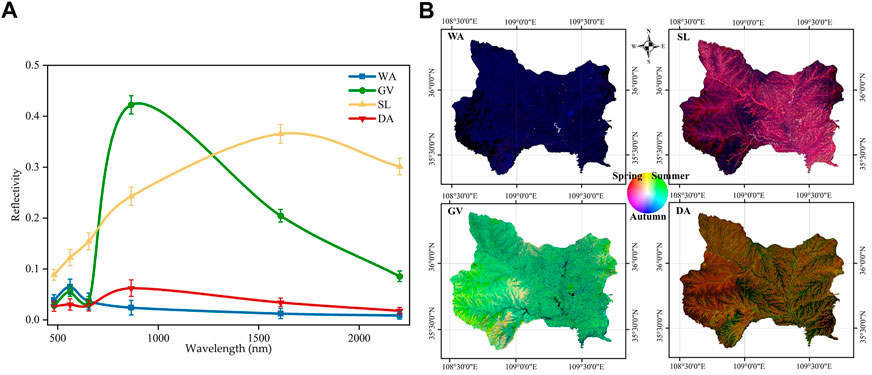
FIGURE 3. The standard spectral curves and fractional abundance of four endmembers. (A) The four endmembers include soil (SL, rock and soil), green vegetation (GV, green photosynthetic leaves), dark (DA, shadow of mountains and vegetation) and water (WA); (B) three-temporal false color composite images, where red, green, and blue represent endmember fractional abundance in spring, summer and autumn, repetitively.
2.4.2 Decision tree
In this study, the land use/cover types were selected into seven categories: Orchard, forest, grassland, water, cultivated land, construction land, and bare land. And we utilized the decision tree classification for land use/cover classification. Decision tree is a well-recognized classification method that does not need complex data processing and simply requires categorization based on the classification criteria and specific features (Mahesh and Paul, 2003; Thoreau et al., 2009). The decision tree was built by the LSMA results, ground features, and the phenological information of different crops. The segmentation threshold was determined by repeated experiments based on the histogram of the training samples’ EMA. The decision tree is shown in Figure 4. And the specific classification rules are as follows:
(1) The water area is extracted by the three seasonal EMA of WA, and the threshold values are WA4 or WA8, or WA10 ≥ 0.5.
(2) The vegetation area and non-vegetation area are divided by the three seasonal EMA of GV, and the threshold values are GV4 or GV8, or GV10 ≥ 0.1.
(3) The bare land and construction land within the non-vegetation area are divided by the summer EMA of SL, and the threshold value is SL8 ≥ 0.6.
(4) The orchard, forest, grassland, and cultivated land are divided by the autumn EMA of GV, and the threshold value is GV10 ≥ 0.6.
(5) Using slope and elevation to further extract forest, and the threshold values are DEM ≥1,300 m and slope ≥15.
(6) Using slope and autumn EMA of GV and DA to further divide the orchard and forest, threshold values are GV10 ≥ 0.125 and DA ≤ 0.5, and slope ≤ 25.
(7) The grassland and cultivated land are divided by spring and summer EMA of GV, and the threshold values are GV8 ≥ 0.45 and GV4 ≥ 0.25.
2.5 Driving force of orchard expansion
2.5.1 Evaluation indicator system
While factors like soil condition, topography and climate have a more localized impact on orchard expansion, regional development has a more external socioeconomic impact. Thus, the influencing factors were selected at the pixel and regional scale and from natural and social dimensions. The evaluation indicator system was shown in Table 3. Due to the favorable climate condition for apple planting, climate change is not considered as the chief factor for local orchard expansion. Therefore, at the pixel scale, the factors were selected from three perspectives of terrain, soil and field conditions, including elevation, slope, soil organic matter, pH, surrounding land types. In terms of social factors, we considered the transportation convenience, the distance to the urban areas and the water sources, which influence the human management processes of irrigation, harvesting and fertilization. At the regional scale, four impact factors, namely average elevation, average elevation standard deviation, average slope and average slope standard deviation, were selected to reflect the differences in natural conditions of each township. For social factors, the population and agricultural output were selected to reflect the differences in socio-economic development of each township. In summary, a total of 17 impact factors were selected to explore their driving effects on orchard expansion.
2.5.2 Random forest regression
Random forest is a machine learning algorithm proposed by Breiman. (2001), which can be used to conduct classification and regression (Tang et al., 2022). It consists of many independent decision trees, and each decision tree is constructed as a random sampling process (Song et al., 2022). The regression algorithm obtains the average of the regression results of all decision trees as the final model output. Then, the random forest regression can quantitatively assess the feature importance and partial dependence based on the existing data through modeling (Liu et al., 2021). The feature importance of each factor is an important parameter, which reflects their relative contributions to the dependent variable. Partial dependence can explain how one or two factors influence on the identified outcome of a machine learning model (Friedman, 2001). In this study, the feature importance is to investigate different influencing factors to orchard expansion. And the partial dependence is to explain the marginal effect of each influencing factor on the orchard expansion (Heilmayer and Brey, 2003). They are both especially suited for the investigation of the forces that influenced agricultural land evolution, particularly when delving into its complex, non-linear driving processes. Therefore, a random forest regression was chosen to explore the mechanisms behind the orchard expansion dynamics in the Loess Plateau. The analysis was performed using the “sklearn” package in Python.
2.6 Orchard planting suitability evaluation
Orchard location decisions and policy suggestions can benefit from a better understanding of the spatial connection between orchard planting suitability and orchard expansion. The suitability evaluation of orchard planting is divided into two parts, including land suitability and climate suitability. According to the soil environment and geographical conditions of the Loess Plateau, 13 indicators were selected considering land conditions, soil conditions, fertility status and climate conditions. Soil is the fundamental factor, providing moisture and nutrients for the growth of orchard cultivated. Topographical conditions are closed related to the growth of apple tree. The difference in elevation, slope and aspect can have a great impact on sunlight exposure, irrigation and soil erosion. Climatic conditions are also key factors, which strongly determine the phenological period of orchard planting. Due to data limitations and a lack of meteorological data at the orchard scale, we use meteorological station data for spatial interpolation, and use the natural breakpoint method for suitability classification. The specific evaluation indicators system was shown in Table 4. Based on the actual state of orchard planting in the study area, the opinions of local experts, and related literature and standards, the indicators affecting the growth of apple tree were divided into three classes: highly suitable, moderately suitable and generally suitable. The weight of the index is determined by the analytic hierarchy process in this study. According to the evaluation indicators system, all raster data were resampled and projected into the CGS_WGS 1984. Finally, we used the spatial analysis tool in ArcGIS 10.2 to predict the distribution of orchard planting suitability. The equation is as follows:
where S is the comprehensive score of the orchard planting suitability evaluation; wi is the weight of the i factor; ui is the score of the i factor; and n is the number of evaluation factors.
3 Results
3.1 Land use/cover classification and accuracy verification
Our findings indicated the LSMA model combined with the decision tree had great applicability for multi-year land use classification, especially for identifying orchard expansion. The land use/cover classification results of the study area from 1990 to 2020 were presented in Figure 6. The accuracy verification (Figure 5) was carried out based on independent random sampling validations obtained from the field survey. The overall accuracy of classification results from 1990 to 2020 ranged from 74.2% to 86.0%, with kappa coefficients ranging from 0.70 to 0.84. The producer’s accuracy and user’s accuracy of orchards, cultivated land, water bodies and forest were above 72%.
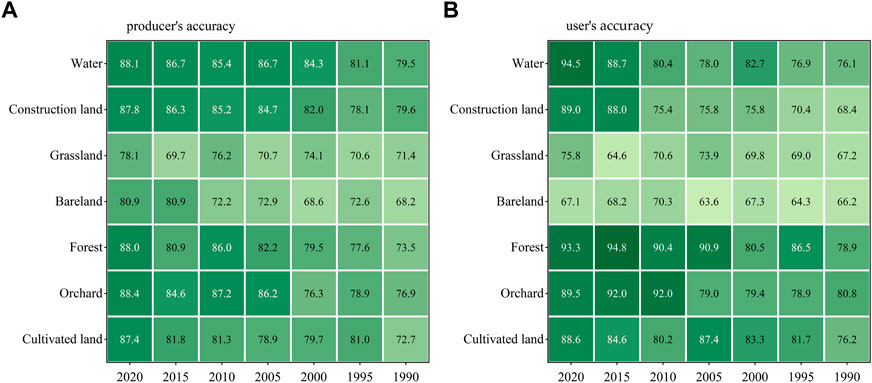
FIGURE 5. Accuracy verification of land use/cover classification from 1990 to 2020. (A) Producer’s accuracy; (B) user’s accuracy.
As shown in Figure 6, the study area has more than 80% of forest throughout all time periods. While the cultivated land dropped year over year and the size of construction land and water did not vary significantly. On the contrary, the orchard and grassland showed an overall increasing trend. In particular, the orchard experienced the greatest increase from 1990 to 2020, tripling in the total area from 1990 to 2020. Orchards now account for 7.94% of the total area, up from 2.94% in 1990. For the three counties, Luochuan, Fuxian, and Huangling, their orchard areas are currently 4.5, 2.1, and 1.8 times larger than they were in 1990, while Luochuan County experiencing the most growth. Comparatively speaking, the cultivated land shrunk by a total of 27,610 ha, representing 71% cultivated land of the study area in 2020. In Luochuan and Fuxian counties, the amount of cultivated land declined by 12,098 and 15,315 ha, with 49% and 46% decreased rate, respectively. The amount of bare land, water bodies, and construction land has increased year by year while the amount of grassland has fluctuated downward.
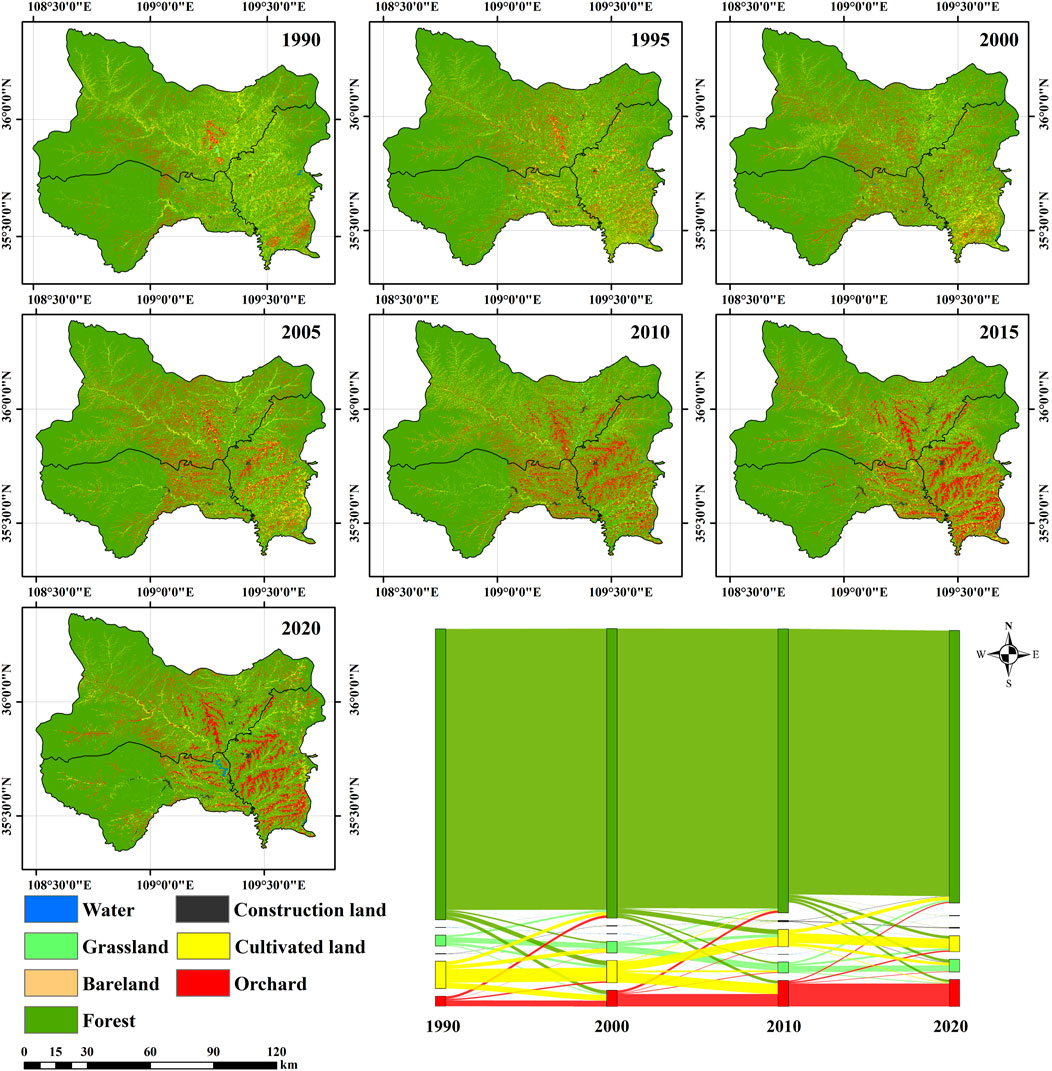
FIGURE 6. Land use/cover classification of the study area and the land use transfer matrix from 1990 to 2020.
According to the analysis of land use transfer matrix, the cultivated land, which has been converted to orchards with a total of 32,309 ha, accounts for 49.2% of total orchards. This is followed by forest, with a total of 11,833 ha converted to orchards in the study area, contributing 18% of the total orchards. With 16,254 ha of cultivated land turned into orchards, Fuxian converted the most cultivated land, contributing 67.1% to its current orchard area. Huangling and Luochuan converted 1,452 and 14,603 ha of arable land into orchards over the three decades, contributing 17.2% and 58.9%, respectively. The fastest land use change occurred between 1990 and 2000, when 81,116 ha were primarily transferred from arable land to orchards. The rate of change in land use from 2000 to 2010 is comparable to that from 1990 to 2000. From 2010 to 2020, the rate of land use change slowed down, with just 1,694 ha of arable land being converted to orchards.
3.2 Orchard expansion characteristics
3.2.1 Spatiotemporal characteristics
We found that the orchard area increased rapidly in the first fifteen years but increased slowly in the last fifteen years during the study period (Figure 7C). The whole study period can be divided into two stages: 1) rapid growth stage: from 1990 to 2005. Due to the encouragement of the local government in the process of reform and opening up, the apple planting area in the study area increased from 24,338 ha to 51,379 ha, an increase of about 111%. The orchard area of Fuxian, Huangling and Luochuan increased by 13,298, 3,151 and 10,592 ha, with growth rate up to 158%, 38% and 101%, respectively; 2) volatile growth stage: as the areas suitable for apple cultivation were gradually occupied, the expansion gradually slowed down. From 2005 to 2020, apple orchard increased by only 14,267 ha. In 2020, the total area of orchards in the study area was 67,646 ha, of which the orchard area in Fuxian, Huangling and Luochuan were 18,140, 6,735 and 29,296 ha, respectively. The orchard planting area of Luochuan has always been larger than that of Fuxian and Huangling, but its growth rate has gradually decreased. The expansion rate of orchards in Huangling has gradually exceeded that of Luochuan. The planting area of each county has increased by more than 300%.
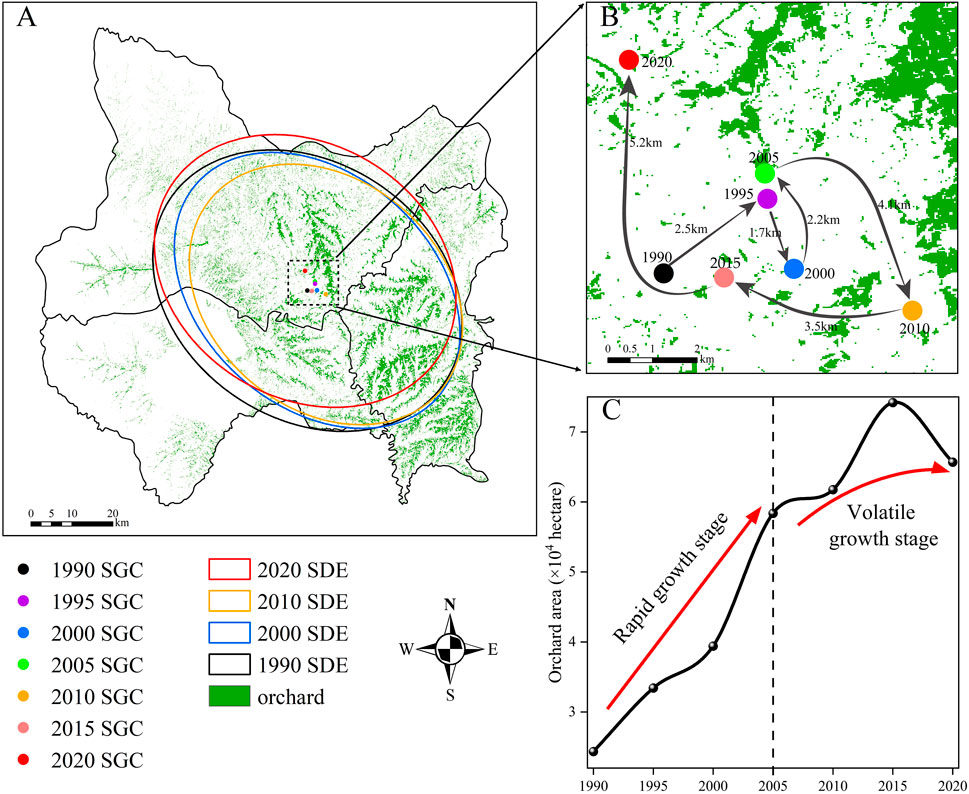
FIGURE 7. Standard deviation ellipse (SDE) analysis and total area growth of orchard. (A) The SDE and its SGC of orchard from 1990 to 2020. The ellipse represents the gathering direction. (B) Shift in SGC and its shifting distance. (C) Growth trend of orchard area in the study area and two stages were divided according to its growth rate.
Due to the local policies and climate change, orchard expanded continuously between 1990 and 2020, initially to the northeast, then to the southeast, and finally to the northwest. In the horizontal direction, the spatial geometry center (SGC) of the orchard shows the trend of eastward expansion before 2010 and then shows the trend of westward expansion. In the vertical direction, the SGC of the orchard generally shows a trend of expansion to the north (Figure 7B), with a shifting distance up to 5.2 km from 2015 to 2020.
3.2.2 Physical geographical feature
Orchard expansion has been increasingly occurring in areas at elevations of 600–900 m and above 1,200 m. We divided the elevation into three intervals of 600–900 m, 900–1,200 m and above 1,200 m based on the natural breakpoint method. The characteristics of orchard expansion at these three elevation intervals were studied in 1990, 2000, 2010 and 2020, respectively. Overall, from 1990 to 2020, orchard expansion mainly occurred in the area at 900–1,200 m, with an expansion area of 33,273 ha and growth rate up to 213% (Figure 8A). As a result of favorable soil, light and rainfall, as well as convenient proximity to transit hubs, apple orchards have primarily settled in a region between 900 and 1,200 m in altitude. Higher quality land resources at lower elevations are mostly used by residents for food production and housing, hence the orchard planting area around 600–900 m elevation was the smallest in the area. Besides, we found that the expansion of orchard was most obvious in the area at 900–1,200 m elevation between 2000 and 2010. However, the area at 900–1,200 m, which is the most suitable area for orchard planting, was gradually filled with orchards.
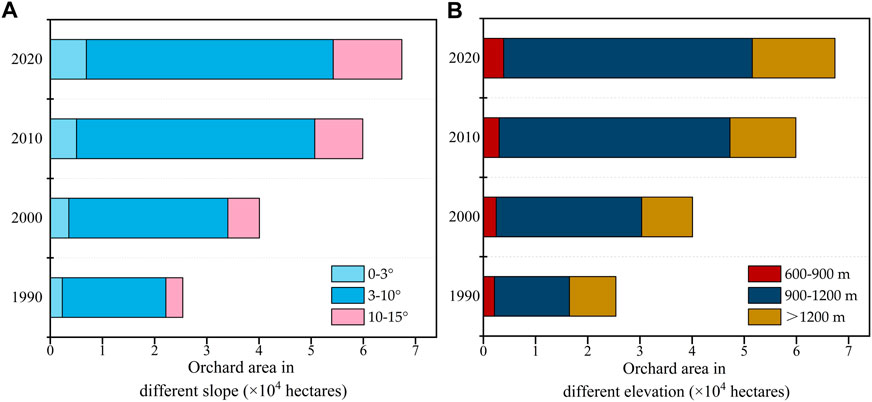
FIGURE 8. Variation in orchard expansion with elevation and slope. (A,B) Shows the orchard area under different slope and elevation, respectively.
Slope is one of the most important factors to consider in apple planting. According to the natural-geographical conditions of the study area, we divided the slope into three intervals of 0–3°, 3–10°, and 10–15°, respectively. It is clear from Figure 8B that the orchards in the study area are mainly distributed in the area with slopes of 3–10°. Analyzing the characteristics of orchard expansion in each period, we found that the orchards in the study area expanded significantly to the area with slopes of 3–10°from 1990 to 2010, and the expansion rate decreased after 2010. In contrast, the trend is reversed for slopes of 0–3° and 10–15°.
3.2.3 Landscape pattern analysis
In this study, we used landscape metrics to analyze the landscape pattern of orchard planting area. As a whole and for different land use/cover types, we selected 6 and 4 landscape metrics, respectively. The ecological significance of each landscape metrics is shown in Supplementary Table S1.
The number of patches (NP) and patch density (PD) in Fuxian, Luochuan, and Huangling counties increased between 1990 and 2020, with 17.9% and 17.2% growth rate, respectively (Supplementary Table S2). The ratio of the area of the largest patch to the total landscape area (LPI) decreased by about 5%. The landscape shape index (LSI) increased by 3%. The spread ability index (CONTAG) decreased from 62.31 to 60.28. The Shannon Diversity Index (SHDI) increased by 5%. The above results show that from 1990 to 2020, with the expansion of orchards, the land use type of the study area changed greatly. In addition, human activities have increased the landscape’s complexity and fragmentation, while also having an impact on the biological environment.
From Figure 9, it can be seen that the number of patches (NP) of orchards continued to increase by about 63.8% during 1990–2020, while the patch density (PD) continued to decrease. Meanwhile, these two indices of cropland had completely opposite trends to those of orchards. The shape indices (LSI) of all categories decreased during the study period, indicating that the landscape shapes of all categories are becoming simpler. The separateness index (SPLIT) of grassland, forest land, and arable land increased during the study period, while the separateness index of orchard decreased. Based on the above results, it can be concluded that between 1990 and 2020, the orchards were characterized by larger planting areas, more aggregated patches, less fragmentation, and simpler shapes. However, a decline in arable land and a more dispersed pattern of grassland, forest, and cropland have resulted from the expansion of orchards.
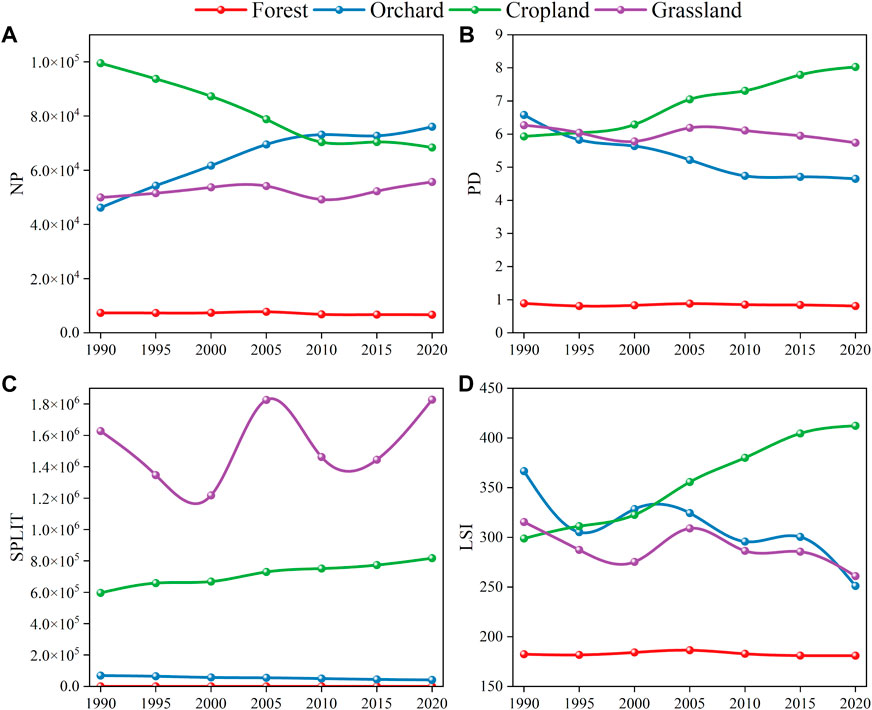
FIGURE 9. Evolution landscape metrics of different land use. Four landscape metrics were selected, including NP, PD, SPLIT and LSI. (A–D) Shows the evolution results of four land use types, including forest, orchard, cropland and grassland.
3.2.4 Orchard expansion varied with planting suitability
Orchards can be planted across the entirety study area, as shown by the planting suitability assessment, with the exception of a few spots at elevations above 1,200 m. The planting suitability evaluation of land suitability evaluation indicators were shown in Supplementary Figure S1. The highly land suitable areas for orchard planting in the study area are mainly concentrated in the central and southwestern parts. The total highly suitable area is 335,371 ha, accounting for about 39.1% of the study area, while the moderately suitable and generally suitable account for 35.1% and 25.8%, respectively. The suitability zones in the north western part of the study area are more dispersed, mainly due to the large variation in soil texture types. The overall climatic suitability of the study area is excellent, with highly suitable regions accounting for over 80%. This is due to the fact that the average annual rainfall and average temperature are in the optimum range for orchard cultivation, thus having a positive effect on apple cultivation. Orchards were primarily dispersed in regions with high suitability, with 84.5% of the total orchard area in 2020. Over the last three decades, orchards expanded mainly into highly suitable regions, covering 37,839 ha, followed by 3,347 ha in moderately suitable regions and 2,006 ha in generally suitable regions.
For the three counties, Figure 10 shows the dynamics of orchard distribution in different suitability zones during the period 1990–2020. In the high suitability zone, Luochuan increased its orchard area by 16,431 ha, surpassing Fuxian and Huangling. Although the overall plantation suitability of the study area is good, orchard expansion is nevertheless concentrated in the most suitable regions, implying their inherent strong connection. In recent years, orchard expansion has gradually slowed down and the highly suitable areas of orchards are already at capacity.
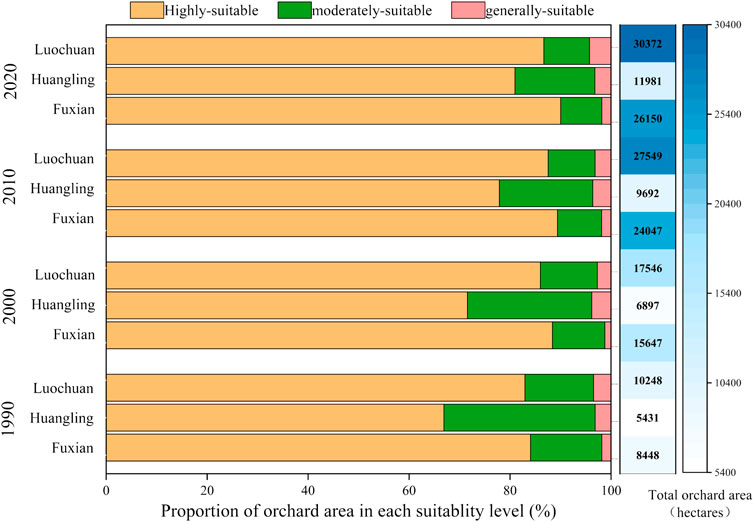
FIGURE 10. Dynamics of orchard area in Fuxian, Huangling and Luochuan under different suitability levels.
3.3 Driving forces analysis
3.3.1 Feature importance
Among all the 17 factors, the slope, proportion of adjacent farmland, agricultural output, aspect, distance from main road and standard deviation of elevation made a great contribution to orchard expansion, with the sum of their feature importance up to 70% (Figure 11A). In particular, slope, aspect and standard deviation of elevation are all topographic factors and their total feature importance is up to 50%. These findings demonstrated that topographical factors are the primary motivator for orchard expansion, of which slope is the most notable attribute due to its highest feature importance. The feature importance of soil pH, distance from urban area, soil texture type, distance from water source and the proportion of adjacent forest are less than 5%. For the remaining factors, we detected few contributions to orchard expansion.
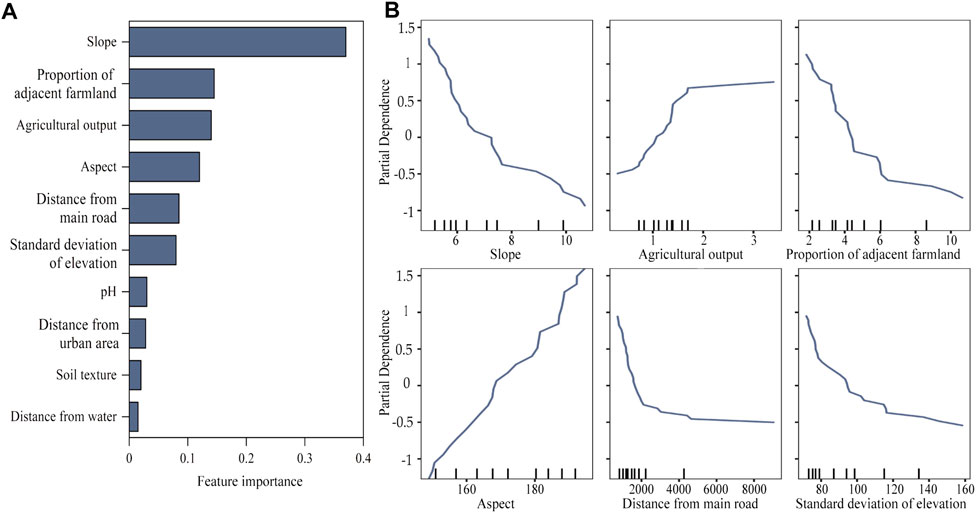
FIGURE 11. Feature importance and partial dependence of selected influencing factors on orchard expansion. (A) Shows the feature importance of primary influencing factors on orchard expansion. (B) Shows the partial dependence of the first six influencing factors.
3.3.2 Partial dependence
Figure 11B presents the quantitative relationship between the orchard expansion and the first six influencing factors. When the slope is larger than 10°, the orchard expansion significantly slowed down, implying the orchard expansion is mainly concentrated in the area with slope below 10°. Due to the policy of arable land protection, the magnitude of orchard expansion gradually decreases with the increase of the proportion of adjacent farmland. At present, the orchard expansion mainly occurs in those regions where neighboring cultivated land is below 6%. The relationship between the orchard expansion and aspect tends to be a fixed straight line, but there is no clear expansion pattern. With the increase of the distance from the main road, we observed a downward and then flat trend. In other words, the larger the distance from the road, the less likely the orchard will expand under a threshold of 2 km. When the distance exceeds 2 km, the orchard area tends to stabilize and transportation will no longer influence orchard expansion under such circumstances. On the contrary, an upward and then flat trend was observed with the increase of the local agricultural output. When the value is between 0 and 160 million-yuan, orchard area will increase rapidly due to the development of social economic development. The orchard expansion changes in two stages with the increase of the standard deviation of elevation of the township. Before the standard deviation of elevation of the township grows to 120 m, the orchard expansion decreases rapidly. After reaching 120 m, the expansion decreases at a slower rate. This is mainly due to the fact that the township with relatively large elevation standard deviation distributed many mountainous areas with large slope, which are not suitable for orchard planting.
4 Discussion
4.1 Orchard expansion and suitability evaluation
Assessing the suitability of orchard plantation can provide valuable instruction for the sustainable expansion of orchards (Pimenta et al., 2021; Arab and Ahamed, 2022). At present, with the rapid development of society, along with land use conflicts, the implementation of the scientific assessment of planting suitability not only helps to improve the efficiency of land use but also enables a rational land allocation. As people’s diets change, the demand for fruit is increasing. In order to promote economic development, the local government has adopted different policies to motivate fruit farmers.
In this study, the suitability evaluation results confirm that over 80% regions were highly suitable. It is unsurprisingly to find that the majority of orchards (84.5%) were located in highly suitable areas in 2020. Our findings demonstrate a robust positive relationship between farmers’ land-use decisions and local environment condition. Land suitability has been proved to be the crucial factor driving the expansion of local orchards, especially slope. Thus, regional policy guidelines are supposed to focus on improving land suitability. Besides, more social factors, such as infrastructure development and regional incentive strategies, need to be taken into account. Those measures are of tremendous help in places where slope is relatively higher.
In addition, areas with highly suitable orchards are facing the problem of aging orchards, which need to be renewed (Hou et al., 2021). Identifying aging orchards and improving orchard yields are other issues that need to be further addressed. Blind orchard expansion is not the only solution to enhancing economic performance, but the renewal of aging orchards in highly suitable areas deserves more attention. Not only will this raise farmers' incomes and orchard yields, but it will also help rationalize the use of land resources and strike a balance between economic development and ecological conservation.
4.2 Policy recommendations for orchard expansion
The orchard expansion in LP is hugely affected by topographical circumstances. Cultivating apples on the suitable slope will guarantee their yield and quality. Based on the analysis of suitability evaluation, the most suitable slope for apple plantation in the region is 3–10°. To boost the profitability of apple production, local authorities should encourage farmers to grow apples in optimal locations and increase access to agricultural expertise.
In addition, our findings revealed the distance of the orchard from water sources, major roads and urban areas were also key factors influencing the orchard expansion. The cultivation, harvest, and transportation of apples are profoundly influenced by the transportation accessibility. In order to reduce the apples losses during long-distance transit, the government is obligated to improve public infrastructure development in various locations. Besides transportation can be centralized, and apple purchasing markets should be established within an appropriate distance from orchard locations. At the same time, the government should organize the purchase in the fields or in households during the harvest season.
Soil conditions are another essential consideration for apple growth. With the large expansion of orchards, the biological environment of soil was greatly impacted by the excessive intake of pesticides and fertilizers. Emphasis on the application of organic fertilizers can promote the circular ecological agriculture. The government should formulate policy standard for pesticides control and apply scientific fertilization in conjunction with tree growth. This can be a potential external incentive factors driving the orchard expansion. However, such policy related impact factors were not included in this study. In the future, relevant policy projects, poverty alleviation funds and other associated policies should be considered, which can provide more valuable insights for orchard planting in the future.
5 Conclusion
In this study, the land use/cover classification of three typical orchard planting counties on the Loess Plateau from 1990 to 2020 was mapped based on the combination of the LSMA model and the decision tree method. According to the standard deviation ellipse analysis, landscape metrics, physical geographic features and planting suitability assessment, we analyzed the spatiotemporal dynamics of the orchard expansion over the past three decades. From the perspective of environment and socioeconomic, we then developed an evaluation indicator system of potential determinants on orchard expansion. The random forest regression was carried out to quantify the feature importance and partial dependence of those factors. Furthermore, we investigated the evaluation of orchard planting suitability in order to put forward favorable policy suggestions. Our study aims at providing empirical references for the sustainable and synergistic development of agricultural land and economic boom in the ecological fragile region.
The results demonstrate that the orchard has expanded rapidly over the past 30 years, especially between 1990 and 2005. The orchards continued to spread out towards the northwest, and their spatial geometry center migrated from the southeast to the north central area, with decreased fragmentation. In the last 30 years, orchards mainly expanded to highly suitable areas, which totally reached up to 37,839 ha, with a growth rate up to 197%. The natural conditions served as the chief factor to orchard expansion, among which slope contributed the most. Our results revealed the most suitable slope for orchard planting in the study area is 3–10. Therefore, for areas with more hilly and mountainous terrain, gentler slope should be preferred for orchard planting. At the same time, the transportation convenience and the policy support for agriculture also provide a “non-negligible” reference basis for future orchard planting.
Compared with previous studies, this study focused on the ecologically fragile areas of the Loess Plateau from the view of natural geographic characteristics, landscape pattern and orchard planting suitability. Our study provided novel approach for identifying cash crop expansion in ecologically fragile areas. Besides, this study explored the driving factors of orchard expansion from multiple scales by applying machine learning regression, which was innovative compared with previous studies. However, policy related impact factors were not included in this study. In the future, relevant policy projects, poverty alleviation funds and other associated policies should be considered, which can provide more valuable insights for orchard planting in the future.
Data availability statement
The original contributions presented in the study are included in the article/Supplementary Material, further inquiries can be directed to the corresponding author.
Author contributions
Conceptualization ZL; Data curation, ZL, QH, and XG; Formal analysis, ZL, QH, XG, and SW; Funding acquisition, ZL and FL; Methodology, ZL, QH, XG, SW, and QW; Software, QH and XG; Supervision, ZL and FL; Validation, YQ and WZ; Writing—original draft, ZL, QH, XG, SW, and QW; Writing—review and editing, ZL, QH, XG, SW, QW, YQ, and WZ. All authors have read and agreed to the published version of the manuscript.
Funding
This research was funded by the Open Fund of Key Laboratory of Urban Land Resources Monitoring and Simulation, Ministry of Natural Resources, grant number KF-2020-05-026 and the National Natural Science Foundation of China (No. 41801202, No. 41911530693).
Acknowledgments
Thanks to the reviewers and editors for their valuable suggestions for improving the manuscript.
Conflict of interest
The authors declare that the research was conducted in the absence of any commercial or financial relationships that could be construed as a potential conflict of interest.
Publisher’s note
All claims expressed in this article are solely those of the authors and do not necessarily represent those of their affiliated organizations, or those of the publisher, the editors and the reviewers. Any product that may be evaluated in this article, or claim that may be made by its manufacturer, is not guaranteed or endorsed by the publisher.
Supplementary material
The Supplementary Material for this article can be found online at: https://www.frontiersin.org/articles/10.3389/fenvs.2022.1097236/full#supplementary-material
References
Arab, S. T., and Ahamed, T. (2022). Land suitability analysis for potential vineyards extension in Afghanistan at regional scale using remote sensing datasets. Remote Sens. (Basel). 14, 4450. doi:10.3390/rs14184450
Azzari, G., and Lobell, D. B. (2017). Landsat-based classification in the cloud: An opportunity for a paradigm shift in land cover monitoring. Remote Sens. Environ. 202, 64–74. doi:10.1016/j.rse.2017.05.025
Boardman, J. W. (1993). Automating spectral unmixing of AVIRIS data using convex geometry concepts. AvaliableAt: https://api.semanticscholar.org/CorpusID:140591692.
Chen, G., Wu, P., Wang, J., Zhang, P., and Jia, Z. (2022). Ridge–furrow rainfall harvesting system helps to improve stability, benefits and precipitation utilization efficiency of maize production in Loess Plateau region of China. Agric. Water Manag. 261, 107360. doi:10.1016/j.agwat.2021.107360
Chen, R., Xue, X., Wang, G., and Wang, J. (2021). Determination and dietary intake risk assessment of 14 pesticide residues in apples of China. Food Chem. x. 351, 129266. doi:10.1016/j.foodchem.2021.129266
Dibs, H., Idrees, M. O., and Alsalhin, G. B. A. (2017). Hierarchical classification approach for mapping rubber tree growth using per-pixel and object-oriented classifiers with SPOT-5 imagery. Egypt. J. Remote Sens. Space Sci. 20, 21–30. doi:10.1016/j.ejrs.2017.01.004
Dong, J., Xiao, X., Chen, B., Torbick, N., Jin, C., Zhang, G., et al. (2013). Mapping deciduous rubber plantations through integration of PALSAR and multi-temporal Landsat imagery. Remote Sens. Environ. 134, 392–402. doi:10.1016/j.rse.2013.03.014
Feng, X., Fu, B., Piao, S., Wang, S., Ciais, P., Zeng, S., et al. (2016). Revegetation in China’s Loess Plateau is approaching sustainable water resource limits. Nat. Clim. Chang. 6, 1019–1022. doi:10.1038/nclimate3092
Friedman, J. H. (2001). Greedy function approximation: A gradient boosting machine. Ann. Stat. 29, 1189–1232. doi:10.1214/AOS/1013203451
Fu, B. (2022). Ecological and environmental effects of land-use changes in the Loess Plateau of China. Chin. Sci. Bull. 67, 543. doi:10.1360/TB-2022-0543
Fu, B., Wang, S., Liu, Y., Liu, J., Liang, W., and Miao, C. (2017). Hydrogeomorphic ecosystem responses to natural and anthropogenic changes in the Loess Plateau of China. Annu. Rev. Earth Planet. Sci. 45, 223–243. doi:10.1146/annurev-earth-063016-020552
Guo, Y., He, P., Searchinger, T. D., Chen, Y., Springmann, M., Zhou, M., et al. (2022). Environmental and human health trade-offs in potential Chinese dietary shifts. One Earth 5, 268–282. doi:10.1016/j.oneear.2022.02.002
Guzmán, G., Boumahdi, A., and Gómez, J. A. (2022). Expansion of olive orchards and their impact on the cultivation and landscape through a case study in the countryside of Cordoba (Spain). Land Use Policy 116, 106065. doi:10.1016/j.landusepol.2022.106065
Han, W., Liu, M., Sun, M., Zha, S., Huo, W., and Sun, D. (2022). Time-of-planting mapping method for apple orchards based on standard spectral endmembers spaces. Trans. CASE 38, 201–210. doi:10.11975/j.issn.1002-6819.2022.14.02
Heilmayer, O., and Brey, T. (2003). “Scaling of metabolic rate with body mass and temperature in scallops,” in 14th international pectinid workshop (St. Petersburg, Florida: J Anim Ecol).
Hou, L., Liu, Z., Zhao, J., Ma, P., and Xu, X. (2021). Comprehensive assessment of fertilization, spatial variability of soil chemical properties, and relationships among nutrients, apple yield and orchard age: A case study in luochuan county, China. Ecol. Indic. 122, 107285. doi:10.1016/j.ecolind.2020.107285
Huo, K., Ruan, Y., Fan, H., Guo, C., and Cai, H. (2022). Spatial-temporal variation characteristics of cultivated land and controlling factors in the Yangtze River Delta region of China. Front. Environ. Sci. 10, 871482. doi:10.3389/fenvs.2022.871482
Jason, S. B., Marcellus, M. C., Ana Claudia, S., Gabriel, G., and Vanessa, R. (2017). Indirect land use change from ethanol production: The case of sugarcane expansion at the farm level on the Brazilian cerrado. J. Land Use Sci. 12 (6), 442–456. doi:10.1080/1747423X.2017.1354937
Jin, Z., Azzari, G., You, C., Tommaso, S. D., Aston, S., Buike, M., et al. (2019). Smallholder maize area and yield mapping at national scales with Google Earth Engine. Remote Sens. Environ. 228, 115–128. doi:10.1016/j.rse.2019.04.016
Kaspar, H., and Jefferson, F. (2018). The expansion of tree-based boom crops in mainland southeast asia: 2001 to 2014. J. Land Use Sci. 13 (1-2), 198–219. doi:10.1080/1747423X.2018.1499830
Li, Y., Zhang, X., Cao, Z., Liu, Z., Lu, Z., and Liu, Y. (2021a). Towards the progress of ecological restoration and economic development in China's Loess Plateau and strategy for more sustainable development. Sci. Total Environ. 756, 143676. doi:10.1016/j.scitotenv.2020.143676
Li, Z., Han, W., Hu, Q., Gao, X., Wang, L., Xiao, F., et al. (2021b). Land use/cover classification based on combining spectral mixture analysis model and object-oriented method. Trans. CASE 37, 225–233. doi:10.11975/j.issn.1002-6819.2021.17.026
Liang, H., Xue, Y., Li, Z., Wang, S., Wu, X., Gao, G., et al. (2018). Soil moisture decline following the plantation of Robinia pseudoacacia forests: Evidence from the Loess Plateau. For. Ecol. Manage. 412, 62–69. doi:10.1016/j.foreco.2018.01.041
Lin, Z., Chen, C., Liu, Y., Liu, G., He, P., Liao, G., et al. (2022). Simulation of citrus production space based on MaxEnt. Front. Environ. Sci. 10, 993920. doi:10.3389/fenvs.2022.993920
Liu, J., Zhou, T., Luo, H., Liu, X., Yu, P., Zhang, Y., et al. (2021). Diverse roles of previous years’ water conditions in gross primary productivity in China. Remote Sens. (Basel). 13, 58. doi:10.3390/rs13010058
Lu, A., Tian, P., Mu, X., Zhao, G., Feng, Q., Guo, J., et al. (2022). Fuzzy logic modeling of land degradation in a Loess Plateau watershed, China. Remote Sens. (Basel). 14, 4779. doi:10.3390/rs14194779
Mahesh, P., and Paul, M. M. (2003). An assessment of the effectiveness of decision tree methods for land cover classification. Remote Sens. Environ. 6, 554–565. doi:10.1016/S0034-4257(03)00132-9
Naqash, F., and Wani, S. A. (2019). Assessment of farmers’ knowledge and awareness regarding pest control technologies in the apple growing belts of Kashmir Valley. Int Res J Agr. Econ Stat 10, 221–233. doi:10.15740/HAS/IRJAES/10.2/221-233
Patrick, S. W. (2016). Transient poverty, poverty dynamics, and vulnerability to poverty: An empirical analysis using A balanced panel from rural China. World Dev. 78, 541–553. doi:10.1016/j.worlddev.2015.10.022
Peña-Cortés, F., Vergara-Fernández, C., Pincheira-Ulbrich, J., Aguilera-Benavente, F., and Gallardo-Alvarez, N. (2021). Location factors and dynamics of tree farm expansion in two coastal river basins in south-central Chile: Basis for land use planning. J. Land Use Sci. 16 (2), 159–173. doi:10.1080/1747423X.2021.1882597
Pimenta, F. M., Speroto, A. T., Costa, M. H., and Dionizio, E. A. (2021). Historical changes in land use and suitability for future agriculture expansion in western bahia, Brazil. Remote Sens. (Basel). 13, 1088. doi:10.3390/rs13061088
Roy, D. P., Wulder, M. A., Loveland, T. R., Woodcock, C. E., Allen, R. G., Anderson, M. C., et al. (2014). Landsat-8: Science and product vision for terrestrial global change research. Remote Sens. Environ. 145, 154–172. doi:10.1016/j.rse.2014.02.001
Small, C. (2003). High spatial resolution spectral mixture analysis of urban reflectance. Remote Sens. Environ. 88, 170–186. doi:10.1016/j.rse.2003.04.008
Song, J., Gao, J., Zhang, Y., Li, F., Man, W., Liu, M., et al. (2022). Estimation of soil organic carbon content in coastal wetlands with measured VIS-nir spectroscopy using optimized support vector machines and random forests. Remote Sens. (Basel). 14, 4372. doi:10.3390/rs14174372
Souza, C., Shimbo, J., Rosa, M., Parente, L., Alencar, A., Rudorff, B., et al. (2020). Reconstructing three decades of land use and land cover changes in brazilian biomes with landsat archive and Earth engine. Remote Sens. (Basel). 12, 2735. doi:10.3390/rs12172735
Sun, D., and Liu, N. (2015). Coupling spectral unmixing and multi-seasonal remote sensing for temperate dryland land use/land cover mapping in Minqin County, China. Int. J. Remote Sens. 36, 3636–3658. doi:10.1080/01431161.2015.1047046
Sun, D., Zhang, P., Sun, Q., and Jiang, W. (2019). A dryland cover state mapping using catastrophe model in a spectral endmember space of OLI: A case study in minqin, China. Int. J. Remote Sens. 40, 5673–5694. doi:10.1080/01431161.2019.1580795
Sun, Z., Patrick, L., Guo, H., Huang, C., and Claudia, K. (2017). Extracting distribution and expansion of rubber plantations from Land-sat imagery using the C5.0 decision tree method. J. Appl. Remote Sens. 2, 026011. doi:10.1117/1.JRS.11.026011
Tang, J., Liu, Y., Li, L., Liu, Y., Wu, Y., Xu, H., et al. (2022). Enhancing aboveground biomass estimation for three pinus forests in yunnan, SW China, using landsat 8. Remote Sens. (Basel). 14, 4589. doi:10.3390/rs14184589
Thoreau, R. T., Nicholas, C. C., Nicholas, R. G., and James, A. V. (2009). Extracting urban vegetation characteristics using spectral mixture analysis and decision tree classifications. Remote Sens. Environ. 113, 398–407. doi:10.1016/j.rse.2008.10.005
Tracy, H. (2020). Evolving patterns of agricultural frontier expansion in Mexico’s chihuahuan desert: A political ecology approach. J. Land Use Sci. 15 (2-3), 270–289. doi:10.1080/1747423X.2019.1646332
Wang, L., Effah, Z., Setor, K. F., Li, L., Xie, J., Luo, Z., et al. (2022a). Continuous maize cultivation with high nitrogen fertilizers associated with the formation of dried soil layers in the semiarid farmland on the Loess Plateau. J. Hydrol. X. 613, 128324. doi:10.1016/j.jhydrol.2022.128324
Wang, W., Zhang, H., Mo, F., Liao, Y., and Wen, X. (2022b). Reducing greenhouse gas emissions and improving net ecosystem economic benefit through long-term conservation tillage in a wheat-maize multiple cropping system in the Loess Plateau, China. Eur. J. Agron. 141, 126619. doi:10.1016/j.eja.2022.126619
Wang, X., and Cheng, H. (2022). Dynamic changes of cultivated land use and grain production in the lower reaches of the Yellow River based on GlobeLand30. Front. Environ. Sci. 10, 974812. doi:10.3389/fenvs.2022.974812
Wu, Z., Zou, S., Yang, Y., Yang, X., Han, Q., Chen, C., et al. (2022). Spatiotemporal prediction and optimization of environmental suitability in citrus-producing areas. Front. Environ. Sci. 10, 985952. doi:10.3389/fenvs.2022.985952
Xiao, R., Su, S., Mai, G., Zhang, Z., and Yang, C. (2015). Quantifying determinants of cash crop expansion and their relative effects using logistic regression modeling and variance partitioning. Int. J. Appl. Earth Obs. Geoinf. 4, 258–263. doi:10.1016/j.jag.2014.08.015
Xiao, Y., Wang, R., Wang, F., Huang, H., and Wang, J. (2022). Investigation on spatial and temporal variation of coupling coordination between socioeconomic and ecological environment: A case study of the Loess Plateau, China. Ecol. Indic. 136, 108667. doi:10.1016/j.ecolind.2022.108667
Xuan, Z., Qi, S., Gong, P., Liu, C., and Wang, J. (2018). Long-term monitoring of citrus orchard dynamics using time-series landsat data: A case study in southern China. Int. J. Remote Sens. 39, 8271–8292. doi:10.1080/01431161.2018.1483088
Yang, M., Wang, S., Zhao, X., Gao, X., and Liu, S. (2020). Soil properties of apple orchards on China’s Loess Plateau. Sci. Total Environ. 723, 138041. doi:10.1016/j.scitotenv.2020.138041
Zhang, Q., Gao, W., Su, S., Wen, M., and Cai, Z. (2017). Biophysical and socioeconomic determinants of tea expansion: Apportioning their relative importance for sustainable land use policy. Land Use Policy 68, 438–447. doi:10.1016/j.landusepol.2017.08.008
Zhao, H., He, H., Bai, C., and Zhang, C. (2018). Spatial-temporal characteristics of land use change in the Loess Plateau and its environmental effects. China Land Sci. 32, 49–57. doi:10.11994/zgtdkx.20180622.104942
Zhou, J., Fu, B., Gao, G., Lü, Y., Liu, Y., Lü, N., et al. (2016). Effects of precipitation and restoration vegetation on soil erosion in a semi-arid environment in the Loess Plateau, China. Catena 137, 1–11. doi:10.1016/j.catena.2015.08.015
Keywords: orchard expansion, driving forces, ecological fragile region, linear spectral mixture analysis, Loess Plateau
Citation: Hu Q, Gao X, Wang S, Wang Q, Qin Y, Zhang W, Lun F and Li Z (2023) Exploring the characteristics and driving forces of orchard expansion in ecological fragile region: A case study of three typical counties in the Loess Plateau. Front. Environ. Sci. 10:1097236. doi: 10.3389/fenvs.2022.1097236
Received: 13 November 2022; Accepted: 05 December 2022;
Published: 04 January 2023.
Edited by:
Minghong Tan, Institute of Geographic Sciences and Natural Resources Research (CAS), ChinaReviewed by:
Bangbang Zhang, Northwest A&F University, ChinaYongsheng Wang, Institute of Geographic Sciences and Natural Resources Research (CAS), China
Copyright © 2023 Hu, Gao, Wang, Wang, Qin, Zhang, Lun and Li. This is an open-access article distributed under the terms of the Creative Commons Attribution License (CC BY). The use, distribution or reproduction in other forums is permitted, provided the original author(s) and the copyright owner(s) are credited and that the original publication in this journal is cited, in accordance with accepted academic practice. No use, distribution or reproduction is permitted which does not comply with these terms.
*Correspondence: Zhuo Li, zhuoli@cau.edu.cn