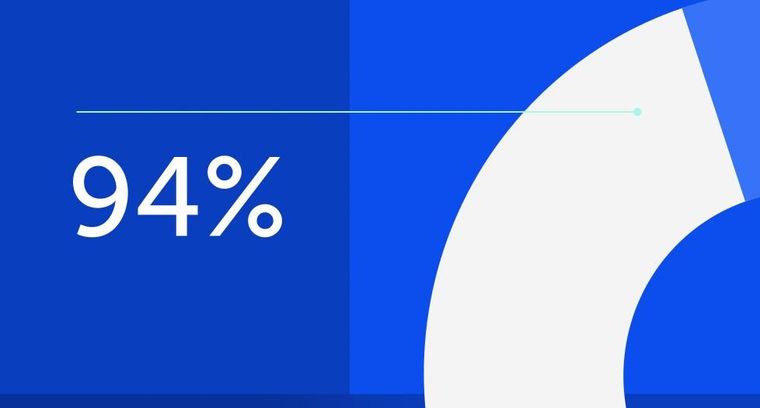
94% of researchers rate our articles as excellent or good
Learn more about the work of our research integrity team to safeguard the quality of each article we publish.
Find out more
ORIGINAL RESEARCH article
Front. Environ. Sci., 22 December 2022
Sec. Environmental Informatics and Remote Sensing
Volume 10 - 2022 | https://doi.org/10.3389/fenvs.2022.1096843
This article is part of the Research TopicRemote Sensing of Aquatic Environment and Its Implication for Environmental ManagementView all 8 articles
Dissolved oxygen (DO) is crucial for the health of aquatic ecosystems, and plays an essential role in regulating biogeochemical processes in inland lakes. Traditional measurements of DO using the probe or analysis in a laboratory are time-consuming and cannot obtain data with high frequency and broad coverage. Satellites can provide daily/hourly observations within a broad scale and have been used as an important technique for aquatic environments monitoring. However, satellite-derived DO in waters is challenging due to its non-optically active property. Here, we developed a two-step model for retrieving DO concentration in Lake Taihu from Moderate Resolution Imaging Spectroradiometer (MODIS) Aqua images. A machine learning model (eXtreme gradient boosting) was developed to estimate DO from field water temperature, water clarity, and chlorophyll-a (Chla) (root-mean-square error (RMSE) = 0.98 mg L−1, mean absolute percentage error (MAPE) = 7.9%) and subsequently was validated on MODIS-derived water temperature, water clarity, and Chla matchups with a satisfactory accuracy (RMSE = 1.28 mg L−1, MAPE = 9.9%). MODIS-derived DO in Lake Taihu from 2002 to 2021 demonstrated that DO ranged from 7.2 mg L−1 to 14.2 mg L−1, with a mean value of 9.3 mg L−1. DO in the northern region was higher than in the central and southern regions, and higher in winter than in summer. We revealed that DO in this decade (2010–2021) was considerably lower than that in the last decade (2002–2009). Meanwhile, annual mean of DO increased in 2002–2009 and decreased from 2010 to 2021. The spatial distribution of DO in Lake Taihu was related to Chla and water clarity, while seasonal and interannual variations in DO resulted from air temperature primarily. This research enhances the potential use of machine learning approaches in monitoring non-optically active constituents from satellite imagery and indicates the possibility of long-term and high-range variations in more water quality parameters in lakes.
Lakes provide human beings critical living resources, such as water, food, transportation, and recreation (Zhang et al., 2022). Under the influences of climate changes and human activities, lake environments have been altered, and some ecological effects were induced, such as lake warming (O'Reilly et al., 2015), intensified cyanobacterial scums (Huisman et al., 2018; Fang et al., 2022; Hou et al., 2022), loss of aquatic vegetation (Zhang et al., 2017), and water deoxygenation (Jane et al., 2021). Among water quality indicators, dissolved oxygen (DO) is defined as the amount of free and non-compound oxygen dissolved in water (Wetzel 2001), which is one of the most critical factors for water quality and health ecosystem. DO supports aquatic life and basic oxygen demands (e.g., decomposition of organic matter) and frequently regulates biodiversity (Schindler 2017), nutrient biogeochemistry (North et al., 2014), greenhouse gas emissions (Encinas Fernández et al., 2014), and drinking water quality (Michalak et al., 2013). However, a number of studies have reported a decline in DO and even the occurrence of hypoxia and anoxia in coastal and inland lakes (Breitburg et al., 2018; Chi et al., 2020; Jane et al., 2021). The monitoring and understanding of spatial variations and long term trends of DO in lakes is anticipated to support lake management efficiently under global change.
Traditional measurements of DO using the probe or analysis in a laboratory are time-consuming and unable to obtain high frequency and broad coverage data, considerably restricting the understanding of DO changes in lakes (Stanley et al., 2019). Satellites can provide daily/hourly observations within a broad scale, and they have been used as a crucial technique for monitoring aquatic environments (Kravitz et al., 2021). In general, the changes in optical active constituents (OACs), including chlorophyll-a (Chla), suspended particulate matter (SPM), and colored dissolved organic matter (CDOM), can be directly related to the variations in water-leaving radiance (Gordon 1983). Hence, numerous models have been developed for deriving OACs and applied to ocean color missions, such as the Moderate Resolution Imaging Spectroradiometer (MODIS) onboard Terra (1999–present) and Aqua (2002–present) (Song et al., 2014; Mouw et al., 2015; Palmer et al., 2015). Despite these successful ocean color applications, studies with respect to monitoring water quality parameters with non-optical properties (e.g., DO and nutrients) remains lacking (IOCCG 2018).
Several recent studies have tried to use empirical relations between water quality and band combinations to achieve remote sensing of non-optical parameters. For example, Shi et al. (2020) found that the reflectance of the red and near-infrared bands was useful for mapping particle phosphorus. Xiong et al. (2022) used machine learning models to estimate total phosphorus from MODIS reflectance data. Batur and Maktav (2019) employed principal component analysis to estimate several water qualities, including DO. Although these models worked satisfactorily in regional waters, empirical relationships were difficult to transfer to other areas owing to varying lake properties. In addition, the following indirect models have been proposed: 1) the use of in situ data to establish relations between OACs and non-optical water quality, and 2) the retrieval of selected OACs from satellite imagery and the estimation of non-optical substances in waters (IOCCG 2018). Thereinto, Guo et al., 2021 and Kim et al. (2020) used water temperature (WTR) and Chla to predict DO in coastal and inland lakes successfully. In essence, DO is regulated by multiple factors, including physical properties, biochemical processes, and hydrological processes in lakes (Hutchinson and Edmondson 1957; Jankowski et al., 2006; North et al., 2014), which is quite complex. Machine learning models have shown strong and robust performance in retrieving water qualities in complicated waters from remote sensing reflectance (Sagan et al., 2020; Kravitz et al., 2021; Cao et al., 2022c), providing an alternative strategy to estimate DO in lakes (Guo et al., 2021).
The goal of the current research is to monitor and understand long-term variations in DO through MODIS images. Lake Taihu, a shallow, turbid, and eutrophic lake in China, was selected as the study area. Specifically, we aim to 1) analyze the relations between DO and OACs and other properties could be retrieved by remote sensing, including Chla, Secchi-disk depth (SDD), and surface water temperature in Lake Taihu, 2) develop a machine learning model for estimating DO from MODIS images and validate its performance, and 3) generate long-term variations in the DO of Lake Taihu from 2002 to 2021 and reveal its spatiotemporal patterns and corresponding driving forces. The results are expected to support the monitoring of non-optical water quality through satellite remote sensing and provide references for evaluating the ecological health of Lake Taihu.
Lake Taihu is the third largest freshwater lake in China (Figure 1), with a water area of 2,338 km2 and an average depth of 1.9 m (Wang and Dou 1998). It is located in a subtropical area that is warm and wet in summer but cold and dry in winter. Lake Taihu is found on the lower reach of the Yangtze River, and the area around it is one of the most developed regions in China. Excessive human activities have intensified eutrophication and cyanobacterial blooms since the 1980s (Qin et al., 2007). The lake supplies water to approximately 10 million residents of surrounding cities, including Wuxi, Suzhou, and Huzhou. Thus, the water quality of Lake Taihu is essential for local human activities and needs, such as drinking, tourism, fishing, and shipping. Lake Taihu is usually divided into seven subregions: Zhushan Bay (ZSB), Meiliang Bay (MLB), Gonghu Bay (GHB), West, Center, South, and East. Some areas in the MLB, GHB, and East regions are frequently covered by macrophytes, affecting the retrieval of Chla and SDD (Shi et al., 2017), and thus, these areas are not included in the analysis.
FIGURE 1. Geographical locations of Lake Taihu and sampling stations. Lake Taihu is divided into seven subregions, such as Zhushan Bay (ZSB), Meiliang Bay (MLB), Gonghu Bay (GHB), West, South, Center and East. Note that some areas in GHB and East region with a lot of macrophytes affecting the retrievals of dissolved oxygen were not included in this study. Note that the land use data was derived from the Landsat eight OLI data in 2021.
Monthly/seasonal surveys from 2007 to 2015 were conducted by the Taihu Lake Laboratory Ecosystem Research (TLLER) Station to collect water quality parameters (Min et al., 2019) (Figure 1). A total of 847 data samples were collected here after excluding the outliers, e.g., stations covered with cyanobacterial scums and macrophytes. These data included DO, water temperature (WTR), Chla, and SDD. The WTR and DO at each station were measured using a well-calibrated YSI probe (Yellow Springs, OH 45387 United States) (Table 1). A standard 30 cm diameter Secchi disk was used to measure SDD. At each station, water samples at the surface layer (0.5 m) were collected and stored in pre-cleaned 1 L high-density polyethylene bottles. The water samples were strained through glass fiber filters (0.70 μm pore size, Whatman GF/F), and Chla concentration was spectrophotometrically determined using a Shimadzu UV2700 spectrophotometer after the extraction of pigments by using 90% ethanol (Jeffrey and Humphrey 1975).
TABLE 1. Statistics (mean ± standard deviation) of monthly water quality in Lake Taihu from 2007 to 2015. Note that SDD is the Secchi-Disk Depth m), WTR is water temperature (°C), Chla is chlorophyll-a (μg L−1), DO is dissolved oxygen (mg L−1).
In addition, daily mean temperature (°C) and wind speed (m s−1) at Dongshan meteorological station near Lake Taihu (Figure 1) from 2002 to 2021 were downloaded from the National Meteorological Information Center, China (http://data.cma.cn). These data were further aggregated into monthly and annual mean values from 2002 to 2021.
Two types of satellite data were used in this study: 1) MODIS Aqua Level 1 A data for retrieving Chla and SDD in Lake Taihu, and 2) MODIS land surface temperature (LST) (MYD11A1) products.
MODIS Aqua Level 1 A data over Lake Taihu from July 2002 to December 2021 were downloaded from the NASA Goddard Space Flight Center (https://oceancolor.gsfc.nasa.gov/). These MODIS data were calibrated and processed using the SeaWiFS Data Analysis System (SeaDAS, version 8.1) (reprocessing v2018). The full atmospheric correction in SeaDAS 8.1 failed in most pixels in Lake Taihu, possibly related to three reasons: 1) the assumption of black-pixel at the NIR bands failed in the turbid waters (Siegel et al., 2000), 2) the low signal-to-noise ratio in the shortwave infrared (SWIR) induced large uncertainty in deriving aerosol scattering in visible bands (Wang and Gordon 2018), 3) the existing aerosol models might not characterize the absorbing aerosols which was frequently in cities and towns (Wang and Jiang 2018). Alternatively, a partial atmospheric correction was employed to remove gaseous absorption (e.g., water vapor and ozone) and Rayleigh scattering to calculate Rayleigh-corrected reflectance (Rrc, dimensionless) (Hu et al., 2004). Note that the concurrent ancillary data, including air pressure and ozone, were used to generate Rrc in SeaDAS.
Rrc data at three MODIS bands (645, 555, and 469 nm) were used to generate red–green–blue (RGB) composite images at a resolution of 250 m. Note that the data of 469 nm and 555 nm with 500-m resolution were sharpened to a 250 m resolution by using the resample tool in SeaDAS. The RGB images were visually examined to exclude images which were largely contaminated by cloud and Sun glints. Among more than 7,000 granules of MODIS data over Lake Taihu, 1935 scenes were selected finally (Table 2). Furthermore, cloud-contaminated pixels were removed via a threshold set on the shortwave infrared reflectance (Aurin et al., 2013). The cloud mask strategy might recognize turbid pixels as clouds and wrongly remove them. Given that this threshold worked well for most cases, this study did not manually remove clouds scene by scene. Surface scums are often presented in Lake Taihu; hence, the pixels with Floating Algae Index more than −0.004 was recognized as algal blooms and excluded (Hu et al., 2010). To eliminate the potential impact of land adjacent effects on DO retrievals, we excluded three pixels around the land following the suggestion of Feng and Hu (2017).
TABLE 2. Temporal distribution of MODIS Aqua images used in this study. Each row represents the images number in each year while the column is the that of each month.
A concurrent dataset of MODIS/Aqua Rrc data and in situ water quality measurements was constructed to validate the performance of the model on DO retrievals. We used a time window of ±3 h between MODIS/Aqua data and in situ measurements to screen the data first. MODIS pixels with viewing zenith angles >60° and contaminated by clouds and cyanobacterial scums were also excluded (Bailey and Werdell 2006). The mean value with a coefficient of variation <10% in 3 × 3 element windows of MODIS was regarded as the matched Rrc values. Finally, we obtained 58 matching pairs in Lake Taihu.
We used an empirical algorithm proposed by Shi et al. (2017) to estimate Chla from MODIS Rrc data in Lake Taihu. Shi et al. (2017) found that a normalized spectral index that used Rrc (645) and Rrc (859) could be satisfactorily related to Chla (N = 125, root-mean-square error (RMSE) = 15.1 μg L−1, mean absolute percentage error (MAPE) = 27%) (Eq. 1).
Shi et al. (2018) demonstrated that Rrs (645) can be effectively utilized for retrieving SDD in Lake Taihu. Given the unavailability of Rrs (645) in Shi et al. (2018), Rrc (645)–Rrc (2,130) was used as the alternative of Rrs (645) (Feng et al., 2018). To eliminate the difference between reflectance, the empirical equations were recalibrated using the aforementioned matchups (Eq. (2), RMSE = 0.15 m, MAPE = 36%).
We used the LST from the MODIS Aqua (MYD11A1) products (1 km) to represent WTR, which has been proven to obtain consistent spatial and temporal thermal behavior in Lake Taihu (Liu et al., 2015; Qi et al., 2020). MYD11A1 products exclude low-quality pixels (e.g., cloud, cloud shadow, and Sun glint). To be consistent with the spatial resolution of MODIS Rrc data, MYD11A1 data were resampled to 250 m and geographically aligned to MODIS Rrc data.
Three machine learning models, namely, random forest (RF), eXtreme gradient boosting (XGB), and support vector regression (SVR), which have been used for retrieving water quality parameters (Sagan et al., 2020; Cao et al., 2022c), were utilized for retrieving DO in Lake Taihu. Given the various predicting mechanism among the three models, which one exhibited the best performance remained unknown. First, we used 864 in situ data to train and validate the models for comparing and selecting the optimal one. Then, we examined the performance of the optimal model in retrieving DO from 58 matched MODIS samples.
Compared with the use of WTR and Chla in DO estimates in previous studies (Kim et al., 2020; Guo et al., 2021), the input to the models included WTR, Chla, and SDD. The output variable was DO concentrations. WTR alters thermal properties and affects the solubility of DO (Jankowski et al., 2006), while Chla reflects primary productivity, which is closely related to photosynthesis and respiration. SDD is a crucial factor for quantifying light attenuation in a lake column, possibly regulating the lake mixing and vertical distribution of DO (Zhang et al., 2015; Liu et al., 2020). It has also been utilized to estimate carbon dioxide in lakes (Qi et al., 2020). The field data suggested that these variables exhibited considerable correlations with DO during different seasons in Lake Taihu (Figure 2). Following our experiments, all three models trained with the three inputs outperformed the models with two inputs (i.e., WTR and DO).
FIGURE 2. The relationship between water temperature (WTR), Secchi-Disk Depth (SDD), chlorophyll-a (Chla) and dissolved oxygen (DO) for different seasons in Lake Taihu.
We randomly chose approximately 70% (n = 499) of the matchups for training, and the remaining 30% of data (n = 265) were used to test model performance. All the input and output data were log-transformed and standardized using the mean and standard deviation within the 0–1 range before training the models. The hyperparameters of the RF, XGB, and SVR models were determined using a grid search method.
The well-validated machine learning model was used to retrieve DO from cloud-free MODIS Rrc and LST images. The annual and monthly mean DO values from 2002 to 2021 were further aggregated from the daily DO series. The mean DO values for each subregion were calculated from the clipped images by using specific boundaries. Pearson correlation was utilized to explain the relations between two variables, i.e., air temperature and DO. The correlation was significant at p < 0.05. We used the determination coefficient (R2), RMSE, MAPE, median symmetric accuracy (MdSA), and the symmetric signed percentage bias (SSPB) (Morley et al., 2018) to evaluate the performance of the models (Eqs. 3–5). All statistics were collected in Python 3.8 environment.
where N is the number of data pairs; the subscript i denotes individual data points; and E and M represent measured and estimated values, respectively.
The field dataset from 2007 to 2015 (Table 2) indicated that Lake Taihu had a mean DO of 9.21 ± 2.13 mg L−1 (mean ± standard deviation) ranging from 2.3 mg L−1 to 16.9 mg L−1. Seasonal variation in DO was apparent, and exhibited lower in winter than in summer, which was highest in January (11.86 ± 1.17 mg L−1) and lowest in July (7.69 ± 2.02 mg L−1). The relations of DO to the related indicators during different seasons are illustrated in Figure 2. Generally, the seasonal distribution of DO was reversed with that of WTR and similar to that of SDD and Chla. DO exhibited a significantly negative correlation with WTR (p < 0.05) except in summer, which showed a slightly positive correlation (r = 0.17, p < 0.05). The positive relation in summer might resulted from the contributions of WTR and other factors. SDD was positively correlated with DO but only significant during summer (r = 0.13, p < 0.05). In terms of Chla, we found it had a significant positive correlation with DO, except during autumn. This analysis indicated that WTR, SDD, and Chla presented significant relations to the seasonal variation of DO, and the effect of SDD on DO during summer is peculiar. The relations provided the foundation for estimating the DO concentration through the above three parameters.
The performance of the XGB, RF and SVR models on the 265 in situ samples is presented in Figure 3. Notably, the inputs of these samples (i.e., WTR, Chla, and SDD) are in situ measurements. The three machine learning models performed satisfactorily with the <1.1 mg L−1 RMSE and <10% MAPE. Moreover, these data pairs were distributed evenly along the unity for DO ranging from 2 mg L−1 to 15 mg L−1 in Lake Taihu and did not present evident deviations. Among the three models, XGB (R2 = 0.77, RMSE = 0.98 mg L−1, MAPE = 7.9%) slightly outperformed RF (R2 = 0.77, RMSE = 0.99 mg L−1, MAPE = 8.2%) and SVR (R2 = 0.77, RMSE = 1.1 mg L−1, MAPE = 9.4%).
FIGURE 3. The performance of XGBoost [XGB, (A)], Random Forest [RF, (B)], and Supporting Vector machine Regression [SVR, (C)] models based on in situ data on DO estimates for the independent testing dataset (N = 265).
The XGB model was further examined on the 58 MODIS-derived WTR, SDD, and Chla points to determine its integrity in estimating DO from satellite images (4.8–16.0 mg L−1) (Figure 4). MODIS-derived DO performed a satisfactory consistency with the measured values (RMSE = 1.28 mg L−1, MAPE = 9.9%). It should be noted that a slight underestimation for points with DO more than >15 mg L−1 was observed. The XGB model was inferred to be robust and suitable for mapping DO in Lake Taihu from MODIS images.
FIGURE 4. The validation of XGB model on retrievals of dissolved oxygen (DO) in Lake Taihu using MODIS-derived water temperature, chlorophyll-a, and Secchi-disk depth (N = 58).
The well-validated XGB model was utilized to generate the DO series in Lake Taihu from 2002 to 2021. Overall, Lake Taihu had an average DO of 9.3 ± 1.8 mg L−1 over the past 20 years (Figure 5A). DO in the northern three bays (9.8 ± 1.9 mg L−1) was higher than in the central (9.2 ± 1.7 mg L−1) and southern (9.1 ± 1.8 mg L−1) regions. Notably, DO covered with macrophytes were omitted here. We also generated the WTR, Chla, and SDD in Lake Taihu since 2002 (Figures 5B-D). The spatial pattern of DO was similar with Chla and inversely related to that of SDD. Meanwhile, it did not exhibit specific relations with WTR.
FIGURE 5. Spatial distributions of mean MODIS-derived dissolved oxygen [DO, (A)], surface water temperature [WTR, (B)], chlorophyll-a [Chla, (C)], and Secchi-Disk Depth [SDD, (D)] in Lake Taihu from 2002 to 2021, respectively.
The annual variations in DO from 2002 to 2021 are mapped in Figure 6, and the corresponding statistics are illustrated in Figure 7. The interannual DO variations of Lake Taihu were divided into two stages (Figure 7A): 1) significantly increased from 2002 to 2009, with a slope of 0.16 mg L−1 (R2 = 0.63, p < 0.05); and 2) slightly declined from 2011 to 2021, with a slope of −0.04 mg L−1 (R2 = 0.30, p < 0.05). The annual variations in DO of different subregions also presented similar trends (Figure 7B). The annual mean DO was different in various stages. For example, ZSB had the highest DO before 2010, while DO in MLB was the highest after 2010. DO in central and southern Lake Taihu was lowest all the time. We observed that annual mean air temperature decreased from 2002 to 2010 (slope = −0.07 °C, R2 = 0.20, p < 0.05) and exhibited a dramatic increase since 2010 (slope = 0.21 °C, R2 = 0.83, p < 0.05, Figure 7C). The annual air temperature was significantly negatively correlated with DO in Lake Taihu (R2 = 0.21, p < 0.05). In addition, wind speed showed a continuous decline and was not significantly correlated with DO (Figure 7D).
FIGURE 6. Climatological annual mean dissolved oxygen (DO) derived from MODIS images in Lake Taihu from 2002 to 2021. Note that MODIS images did not include data in the period of January-June in 2002.
FIGURE 7. (A) Annual variations in dissolved oxygen (DO) for entire Lake Taihu from 2002 to 2021 (not including macrophytes regions). (B) Annual variations in DO for different subregions of Lake Taihu. (C) and (D) is the annual mean air temperature and wind speed, respectively.
Figure 8 presents the monthly mean DO in Lake Taihu from 2002 to 2021. We found that DO in winter was higher than that in summer. DO was highest in January (11.9 mg L−1) and lowest in August (7.7 mg L−1) (Figure 9A). This finding was consistent with the aforementioned analysis based on the field dataset (Table 1). The monthly variations in DO were negatively correlated with air temperature (R2 = 0.80, p < 0.05), while wind speed was not significantly associated with it (p > 0.05, Figures 9C,D).
FIGURE 8. Climatological monthly mean dissolved oxygen (DO) derived from MODIS images in Lake Taihu from 2002 to 2021.
FIGURE 9. (A) Monthly variations in dissolved oxygen (DO) for entire Lake Taihu from 2002 to 2021 (not including macrophytes regions). (B) Monthly variations in DO for different subregions of Lake Taihu. (C) and (D) is the monthly mean air temperature and wind speed, respectively.
A two-step model was developed to estimate DO from MODIS images in Lake Taihu (Figures 3, 4). The model was established on the theoretical relations between thermal and optical properties and DO in lakes (Kim et al., 2020; Guo et al., 2021). Such an idea for estimating non-optically active matters have been employed in other studies (IOCCG 2018; Chen et al., 2019; Qi et al., 2020). The current work developed a machine learning model to determine the relations between DO and the aforementioned three indicators rather than the regressions (Kim et al., 2020). In general, their relations in eutrophic lakes are complicated and exhibit spatiotemporal heterogeneity (Figure 2) (Wetzel 2001; Jankowski et al., 2006; Breitburg et al., 2018). The input variables had various contributions to the DO in different seasons (Figure 2) and regions for Lake Taihu. The properties of water in norther regions were mainly influenced by algal while central and south regions were turbid. Compared with traditional regressions such as linear/non-linear regression and step-wise regression, machine learning models are particularly efficient for solving complicated non-linear regression (Sagan et al., 2020). The model was developed using 864 samples that spanned different seasons across 8 years in Lake Taihu, suggesting that the model was suitable for most cases in Lake Taihu.
Although machine learning models demonstrate the nature of a “black box,” the relative contributions of input variables to DO prediction can be useful for understanding the mechanism of a model. We calculated the decrease in the accuracy score of the models for each variable to interpret the contribution of each variable to Chla (Cao et al., 2022a). The decrease in accuracy score was defined as the difference between the baseline metric from permutating the feature column, which was implemented in the scikit-learn package of Python. Figure 10 reveals that WTR makes the highest contribution to DO estimation while Chla and SDD have low contributions. Thus, the model still estimated satisfactory DO in Lake Taihu even though the retrievals of Chla and SDD suffered from fair uncertainty (−30%) (Figure 4). The retrieval of Chla in turbid waters frequently depends on the red edge band near 700–710 nm (Gitelson 1992; Gilerson et al., 2010; Gurlin et al., 2011), which is not equipped with MODIS instrument.
Despite the satisfactory DO retrievals from MODIS images in Lake Taihu, several limitations must be improved. First, Rrc used for retrieving Chla and SDD in Lake Taihu did not remove the signals of aerosol contributions, which might limit the accuracy of DO estimations. For turbid waters, including Lake Taihu, the elevating water-leaving radiance suggested that Rrc can be utilized to retrieve water quality (Hu et al., 2004; Cao et al., 2020; Seegers et al., 2021). We also found that the XGB model underestimated DO slightly in the high range (Figure 4), possibly resulting from insufficient samples in the extremely high DO data (Stock 2022). It is anticipated to improve by adding more high DO values (Cao et al., 2020). The surface temperature products of MODIS had a resolution of 1 km, which was lower than Chla and SDD. The pixels were not changed, although we resampled it to 250 m. Since water is frequently homogenous, WTR between adjacent pixels may exhibit slight differences. In addition, DO in shallow lakes might change fast due to wind-induced reoxygenation and diurnal variations in air temperature. Thus, it would be efficient to improve the observations of DO in lakes through the Geostationary satellites (IOCCG 2012), such as Geostationary Ocean Color Imager (GOCI), GOCI-II, and Himawari-8. Our model was developed for MODIS instruments; however, the MODIS mission operation has exceeded its anticipated lifetime and is nearing its end. In the future, the model is expected to be extended to the Visible Infrared Imaging Radiometer Suite (VIIRS) onboard SNPP and NOAA-20/21 and Ocean Land Color Instrument (OLCI) onboard Sentinel-3 for continuing observations (Cao et al., 2022b).
The factors that regulate DO in lakes include the physical processes induced by light, WTR, and lake mixing, and biochemical factors, such as the photosynthesis-induced increase of DO concentration, the respiration of aquatic organisms, the bacterial oxidation of organic matter, and the consumption of DO by other reduced inorganic substances (Zhang et al., 2015). In addition, some anthropogenic modifications of the environment, such as eutrophication (Müller et al., 2012), salinization, and hydrological management (Carpenter et al., 2011) can reduce DO in lakes.
We found that the temporal (interannual and seasonal) variations in the DO of Lake Taihu were related to the variability of air temperature (Figure 7). First, air temperature determined the solute of oxygen in lakes by influencing the WTR indirectly (Jane et al., 2021). The general narrative is that climate warming induces widespread deoxidation in waters (Jankowski et al., 2006; Perron et al., 2014; Zhang et al., 2015; Jane et al., 2021). In deep waters, surface temperature warming can further intensify thermal stratification, reducing water circulation and preventing deep water DO replenishment (Jankowski et al., 2006; North et al., 2014; Kraemer et al., 2015). In Lake Taihu, a shallow lake, such an effect should mostly occur during summer (Yang et al., 2018). In addition, wind speed in Lake Taihu over the past 20 years has declined (Figure 7D), suggesting that turbulence should be weakening (Macintyre 1993; Fernández Castro et al., 2021), possibly reducing DO replenishment.
The spatial distribution of DO in Lake Taihu is consistent with that of Chla (Figure 5). Higher Chla reflects high primary productivity that releases plenty of oxygen via photosynthesis. The higher water clarity in northern areas facilitated the growth of phytoplankton in lakes (Liu et al., 2020). In addition, wind direction might regulate the spatial variations in the DO of Lake Taihu. The wind direction for Lake Taihu was usually southwest each year, suggesting that the stronger mixing process induced higher DO concentration (Zhang et al., 2014).
This research successfully tracked the long-term DO variations of Lake Taihu, allowing us to reveal its trends and elucidate its potential driving factors. In the past, the monitoring of DO in lakes was mostly based on field surveys (Jane et al., 2021). Operational surveys have been conducted on some well-studied lakes, such as Lake Taihu and Lake Erie. However, most lakes cannot be well monitored, which should largely limit our understanding of aquatic health and ecology (Plisnier et al., 2022). Our approach provides a practical idea of employing satellite images to monitor DO in lakes. This idea can be easily extended to other lakes, although the model’s coefficients should be recalibrated using the local dataset. By contrast, the methodology can be utilized to estimate other non-optical water quality parameters, such as total nitrogen, total phosphorus, and the permanganate index. With the relations between OACs and meteorological data and the non-optical parameters, it would be possible to estimate more water qualities in lakes. The MODIS-derived DO of Lake Taihu in the past 2 decades demonstrated declining trends compared with the first decade of this century due to climate warming, which is consistent with the conclusion of previous studies (Jankowski et al., 2006; North et al., 2014; Jane et al., 2021). Climate warming was also regarded as a primary regulator that affected cyanobacterial blooms in Lake Taihu (Qin et al., 2019). The warming climate will continue in the future (Woolway and Merchant 2019), and it is crucial to formulate scientific strategies to prevent the negative ecological effects of deoxidation in lakes.
This study developed a machine learning model for generating the long-term DO variation of Lake Taihu from MODIS Aqua. With the collected in situ data in Lake Taihu from 2007 to 2015, we found that DO in Lake Taihu was correlated with WTR, Chla, and SDD. Then, we established the XGB model to estimate DO from in situ temperature, Chla, and SDD by using 864 field data samples. The XGB model was applied to 58 MODIS-derived WTR, Chla, and SDD, and satisfactory DO retrievals were obtained. The MODIS-derived DO series in Lake Taihu suggested that Lake Taihu had higher DO in the northern region than in the other regions. Meanwhile, summer had lower DO than the other seasons. Annual variations in the DO of Lake Taihu revealed that DO in this decade declined relative to that in 2002–2009. We analyzed the potential driving forces of the spatial and temporal changes in the DO of Lake Taihu. We found that climate warming possibly reduced DO in Lake Taihu. Our results propose the idea of using remote sensing to obtain non-optical water qualities and provide practical references for lake management in the warming future.
The raw data supporting the conclusion of this article will be made available by the authors, without undue reservation.
ML: Conceptualization, methodology, and writing. LW and FQ: Methodology and editing. ML: Data curation. LW: Supervision and funding acquisition. ML and LW: Funding acquisition. All authors have revised the final manuscript. All authors contributed to the article and approved the submitted version.
This work was supported by the National Natural Science Foundation of China (42101056, 41301104), China Postdoctoral Science Foundation (2021M701488), Natural Science Foundation of Jiangsu Province (BR2021071), and Research Fund of Provincial Commonweal Scientific Institutes (No. GYYS2022101).
The authors would like to thank Ronghua Ma, Zhigang Cao, and Yunlin Zhang (Nanjing Institute of Geography and Limnology, Chinese Academy of Sciences) for their help in collecting and processing satellite data and suggestions to improve the manuscript. The authors thank NASA for providing MODIS Level-1 data and land surface temperature data. The authors also thank TILLER for providing some field dissolved oxygen data in Lake Taihu.
The authors declare that the research was conducted in the absence of any commercial or financial relationships that could be construed as a potential conflict of interest.
All claims expressed in this article are solely those of the authors and do not necessarily represent those of their affiliated organizations, or those of the publisher, the editors and the reviewers. Any product that may be evaluated in this article, or claim that may be made by its manufacturer, is not guaranteed or endorsed by the publisher.
Aurin, D., Mannino, A., and Franz, B. (2013). Spatially resolving ocean color and sediment dispersion in river plumes, coastal systems, and continental shelf waters. Remote Sens. Environ. 137, 212–225.
Bailey, S. W., and Werdell, P. J. (2006). A multi-sensor approach for the on-orbit validation of ocean color satellite data products. Remote Sens. Environ. 102, 12–23. doi:10.1016/j.rse.2006.01.015
Batur, E., and Maktav, D. (2019). Assessment of surface water quality by using satellite images fusion based on PCA method in the lake gala, Turkey. IEEE Trans. Geoscience Remote Sens. 57, 2983–2989. doi:10.1109/tgrs.2018.2879024
Breitburg, D., Levin, L. A., Oschlies, A., Grégoire, M., Chavez, F. P., Conley, D. J., et al. (2018). Declining oxygen in the global ocean and coastal waters. Science 359, eaam7240. doi:10.1126/science.aam7240
Cao, Z. G., Ma, R. H., Duan, H. T., Pahlevan, N., Melack, J., Shen, M., et al. (2020). A machine learning approach to estimate chlorophyll-a from Landsat-8 measurements in inland lakes. Remote Sens. Environ. 248, 111974. doi:10.1016/j.rse.2020.111974
Cao, Z. G., Ma, R. H., Liu, M., Duan, H. T., Xiao, Q., Xue, K., et al. (2022a). Harmonized chlorophyll-a retrievals in inland lakes from landsat-8/9 and Sentinel 2A/B virtual constellation through machine learning. IEEE Trans. Geoscience Remote Sens. 60, 1–16. doi:10.1109/tgrs.2022.3207345
Cao, Z. G., Ma, R. H., Pahlevan, N., Liu, M., Melack, J. M., Duan, H. T., et al. (2022b). Evaluating and optimizing VIIRS retrievals of chlorophyll-a and suspended particulate matter in turbid lakes using a machine learning approach. IEEE Trans. Geoscience Remote Sens. 60, 1–17. doi:10.1109/tgrs.2022.3220529
Cao, Z. G., Shen, M., Kutser, T., Liu, M., Qi, T. C., Ma, J. E., et al. (2022c). What water color parameters could be mapped using MODIS land reflectance products: A global evaluation over coastal and inland waters. Earth-Science Rev. 232, 104154. doi:10.1016/j.earscirev.2022.104154
Carpenter, S. R., Stanley, E. H., and Vander Zanden, M. J. (2011). State of the world's freshwater ecosystems: Physical, chemical, and biological changes. Annu. Rev. Environ. Resour. 36, 75–99. doi:10.1146/annurev-environ-021810-094524
Chen, S., Hu, C., Barnes, B. B., Wanninkhof, R., Cai, W.-J., Barbero, L., et al. (2019). A machine learning approach to estimate surface ocean pCO2 from satellite measurements. Remote Sens. Environ. 228, 203–226. doi:10.1016/j.rse.2019.04.019
Chi, L., Song, X., Yuan, Y., Wang, W., Cao, X., Wu, Z., et al. (2020). Main factors dominating the development, formation and dissipation of hypoxia off the Changjiang Estuary (CE) and its adjacent waters, China. Environ. Pollut. 265, 115066. doi:10.1016/j.envpol.2020.115066
Encinas Fernández, J., Peeters, F., and Hofmann, H. (2014). Importance of the autumn overturn and anoxic conditions in the hypolimnion for the annual methane emissions from a temperate lake. Environ. Sci. Technol. 48, 7297–7304. doi:10.1021/es4056164
Fang, C., Song, K., Paerl, H. W., Jacinthe, P. A., Wen, Z., Liu, G., et al. (2022). Global divergent trends of algal blooms detected by satellite during 1982-2018. Globe Change Biol. 28, 2327–2340. doi:10.1111/gcb.16077
Feng, L., Hou, X. J., Li, J. S., and Zheng, Y. (2018). Exploring the potential of Rayleigh-corrected reflectance in coastal and inland water applications: A simple aerosol correction method and its merits. ISPRS J. Photogrammetry Remote Sens. 146, 52–64. doi:10.1016/j.isprsjprs.2018.08.020
Feng, L., and Hu, C. (2017). Land adjacency effects on MODIS Aqua top-of-atmosphere radiance in the shortwave infrared: Statistical assessment and correction. J. Geophys. Res. Oceans 122, 4802–4818. doi:10.1002/2017jc012874
Fernández Castro, B., Bouffard, D., Troy, C., Ulloa, H. N., Piccolroaz, S., Sepúlveda Steiner, O., et al. (2021). Seasonality modulates wind-driven mixing pathways in a large lake. Commun. Earth Environ. 2, 215. doi:10.1038/s43247-021-00288-3
Gilerson, A. A., Gitelson, A. A., Zhou, J., Gurlin, D., Moses, W., Ioannou, I., et al. (2010). Algorithms for remote estimation of chlorophyll-a in coastal and inland waters using red and near infrared bands. Opt. Express 18, 24109–24125. doi:10.1364/oe.18.024109
Gitelson, A. (1992). The peak near 700 nm on radiance spectra of algae and water: Relationships of its magnitude and position with chlorophyll concentration. Int. J. Remote Sens. 13, 3367–3373. doi:10.1080/01431169208904125
Gordon (1983). Remote assessment of Ocean Color for interpretation of satellite visible imagery: A review. Washington, DC: AGU.
IOCCG (2018). “Earth observations in support of global water quality monitoring,” in Reports of the international ocean-colour coordinating group. Editors S. Greb, A. Dekker, and C. Binding (Dartmouth: Canada).
Guo, H., Huang, J. J., Zhu, X., Wang, B., Tian, S., Xu, W., et al. (2021). A generalized machine learning approach for dissolved oxygen estimation at multiple spatiotemporal scales using remote sensing. Environ. Pollut. 288, 117734. doi:10.1016/j.envpol.2021.117734
Gurlin, D., Gitelson, A. A., and Moses, W. J. (2011). Remote estimation of chl-a concentration in turbid productive waters — return to a simple two-band NIR-red model? Remote Sens. Environ. 115, 3479–3490. doi:10.1016/j.rse.2011.08.011
Hou, X. J., Feng, L., Dai, Y. H., Hu, C. A. M., Gibson, L., Tang, J., et al. (2022). Global mapping reveals increase in lacustrine algal blooms over the past decade. Nat. Geosci. 15, 130–134. doi:10.1038/s41561-021-00887-x
Hu, C., Lee, Z., Ma, R., Yu, K., Li, D., and Shang, S. (2010). Moderate resolution imaging spectroradiometer (MODIS) observations of cyanobacteria blooms in Taihu Lake, China. J. Geophys. Research-Oceans 115, C04002. doi:10.1029/2009jc005511
Hu, C. M., Chen, Z. Q., Clayton, T. D., Swarzenski, P., Brock, J. C., and Muller-Karger, F. E. (2004). Assessment of estuarine water-quality indicators using MODIS medium-resolution bands: Initial results from Tampa Bay, FL. Remote Sens. Environ. 93, 423–441. doi:10.1016/j.rse.2004.08.007
Huisman, J., Codd, G. A., Paerl, H. W., Ibelings, B. W., Verspagen, J. M. H., and Visser, P. M. (2018). Cyanobacterial blooms. Nat. Rev. Microbiol. 16, 471–483. doi:10.1038/s41579-018-0040-1
Jane, S. F., Hansen, G. J. A., Kraemer, B. M., Leavitt, P. R., Mincer, J. L., North, R. L., et al. (2021). Widespread deoxygenation of temperate lakes. Nature 594, 66–70. doi:10.1038/s41586-021-03550-y
Jankowski, T., Livingstone, D. M., Bührer, H., Forster, R., and Niederhauser, P. (2006). Consequences of the 2003 European heat wave for lake temperature profiles, thermal stability, and hypolimnetic oxygen depletion: Implications for a warmer world. Limnol. Oceanogr. 51, 815–819. doi:10.4319/lo.2006.51.2.0815
Jeffrey, S. t., and Humphrey, G. (1975). New spectrophotometric equations for determining chlorophylls a, b, c1 and c2 in higher plants, algae and natural phytoplankton. Biochem. Physiol. Pflanz. 167, 191–194. doi:10.1016/s0015-3796(17)30778-3
Kim, Y. H., Son, S., Kim, H. C., Kim, B., Park, Y. G., Nam, J., et al. (2020). Application of satellite remote sensing in monitoring dissolved oxygen variabilities: A case study for coastal waters in korea. Environ. Int. 134, 105301. doi:10.1016/j.envint.2019.105301
Kraemer, B. M., Anneville, O., Chandra, S., Dix, M., Kuusisto, E., Livingstone, D. M., et al. (2015). Morphometry and average temperature affect lake stratification responses to climate change. Geophys. Res. Lett. 42, 4981–4988. doi:10.1002/2015gl064097
Kravitz, J., Matthews, M., Lain, L., Fawcett, S., and Bernard, S. (2021). Potential for high fidelity global mapping of common inland water quality products at high spatial and temporal resolutions based on a synthetic data and machine learning approach. Front. Environ. Sci. 9, 587660. doi:10.3389/fenvs.2021.587660
Liu, G., Ou, W. X., Zhang, Y. L., Wu, T. F., Zhu, G. W., Shi, K., et al. (2015). Validating and mapping surface water temperatures in Lake Taihu: Results from MODIS land surface temperature products. IEEE J. Sel. Top. Appl. Earth Observations Remote Sens. 8, 1230–1244. doi:10.1109/jstars.2014.2386333
Liu, M., Zhang, Y. L., Shi, K., Melack, J., Zhang, Y. B., Zhou, Y. Q., et al. (2020). Spatial variations of subsurface chlorophyll maxima during thermal stratification in a large, deep subtropical reservoir. J. Geophys. Research-Biogeosciences 125, e2019JG005480. doi:10.1029/2019jg005480
Macintyre, S. (1993). Vertical mixing in a shallow, eutrophic lake - possible consequences for the light climate of phytoplankton. Limnol. Oceanogr. 38, 798–817. doi:10.4319/lo.1993.38.4.0798
Michalak, A. M., Anderson, E. J., Beletsky, D., Boland, S., Bosch, N. S., Bridgeman, T. B., et al. (2013). Record-setting algal bloom in Lake Erie caused by agricultural and meteorological trends consistent with expected future conditions. Proc. Natl. Acad. Sci. 110, 6448–6452. doi:10.1073/pnas.1216006110
Min, S., Qian, R., Zhu, G., Huang, J., Qin, B., Yang, H., et al. (2019). “A physical and chemical monitoring dataset of Taihu Lake from 2007 to 2015,” in Science Data Bank.
Morley, S. K., Brito, T. V., and Welling, D. T. (2018). Measures of model performance based on the log accuracy ratio. Space Weather-the Int. J. Res. Appl. 16, 69–88. doi:10.1002/2017sw001669
Mouw, C. B., Greb, S., Aurin, D., DiGiacomo, P. M., Lee, Z., Twardowski, M., et al. (2015). Aquatic color radiometry remote sensing of coastal and inland waters: Challenges and recommendations for future satellite missions. Remote Sens. Environ. 160, 15–30. doi:10.1016/j.rse.2015.02.001
Müller, B., Bryant, L. D., Matzinger, A., and Wüest, A. (2012). Hypolimnetic oxygen depletion in eutrophic lakes. Environ. Sci. Technol. 46, 9964–9971. doi:10.1021/es301422r
North, R. P., North, R. L., Livingstone, D. M., Köster, O., and Kipfer, R. (2014). Long-term changes in hypoxia and soluble reactive phosphorus in the hypolimnion of a large temperate lake: Consequences of a climate regime shift. Glob. Change Biol. 20, 811–823. doi:10.1111/gcb.12371
O'Reilly, C. M., Sharma, S., Gray, D. K., Hampton, S. E., Read, J. S., Rowley, R. J., et al. (2015). Rapid and highly variable warming of lake surface waters around the globe. Geophys. Res. Lett. 42, 10773–10781.
Palmer, S. C. J., Kutser, T., and Hunter, P. D. (2015). Remote sensing of inland waters: Challenges, progress and future directions. Remote Sens. Environ. 157, 1–8. doi:10.1016/j.rse.2014.09.021
Perron, T., Chételat, J., Gunn, J., Beisner, B. E., and Amyot, M. (2014). Effects of experimental thermocline and oxycline deepening on methylmercury bioaccumulation in a Canadian shield lake. Environ. Sci. Technol. 48, 2626–2634. doi:10.1021/es404839t
Plisnier, P.-D., Kayanda, R., MacIntyre, S., Obiero, K., Okello, W., Vodacek, A., et al. (2022). Need for harmonized long-term multi-lake monitoring of African Great Lakes. J. Gt. Lakes. Res. doi:10.1016/j.jglr.2022.01.016
Qi, T., Xiao, Q., Cao, Z., Shen, M., Ma, J., Liu, D., et al. (2020). Satellite estimation of dissolved carbon dioxide concentrations in China's Lake Taihu. Environ. Sci. Technol. 54, 13709–13718. doi:10.1021/acs.est.0c04044
Qin, B., Paerl, H. W., Brookes, J. D., Liu, J., Jeppesen, E., Zhu, G., et al. (2019). Why Lake Taihu continues to be plagued with cyanobacterial blooms through 10 years (2007–2017) efforts. Sci. Bull. 64, 354–356. doi:10.1016/j.scib.2019.02.008
Qin, B. Q., Xu, P. Z., Wu, Q. L., Luo, L. C., and Zhang, Y. L. (2007). Environmental issues of Lake Taihu, China. Hydrobiologia 581, 3–14. doi:10.1007/s10750-006-0521-5
Sagan, V., Peterson, K. T., Maimaitijiang, M., Sidike, P., Sloan, J., Greeling, B. A., et al. (2020). Monitoring inland water quality using remote sensing: Potential and limitations of spectral indices, bio-optical simulations, machine learning, and cloud computing. Earth-Science Rev. 205, 103187. doi:10.1016/j.earscirev.2020.103187
Schindler, D. E. (2017). Warmer climate squeezes aquatic predators out of their preferred habitat. Proc. Natl. Acad. Sci. 114, 9764–9765. doi:10.1073/pnas.1712818114
Seegers, B. N., Werdell, P. J., Vandermeulen, R. A., Salls, W., Stumpf, R. P., Schaeffer, B. A., et al. (2021). Satellites for long-term monitoring of inland U.S. lakes: The MERIS time series and application for chlorophyll-a. Remote Sens. Environ. 266, 112685. doi:10.1016/j.rse.2021.112685
Shi, K., Zhang, Y., Zhang, Y., Qin, B., and Zhu, G. (2020). Understanding the long-term trend of particulate phosphorus in a cyanobacteria-dominated lake using MODIS-Aqua observations. Sci. Total Environ. 737, 139736. doi:10.1016/j.scitotenv.2020.139736
Shi, K., Zhang, Y., Zhou, Y., Liu, X., Zhu, G., Qin, B., et al. (2017). Long-term MODIS observations of cyanobacterial dynamics in Lake Taihu: Responses to nutrient enrichment and meteorological factors. Sci. Rep. 7, 40326. doi:10.1038/srep40326
Shi, K., Zhang, Y., Zhu, G., Qin, B., and Pan, D. (2018). Deteriorating water clarity in shallow waters: Evidence from long term MODIS and in-situ observations. Int. J. Appl. Earth Observation Geoinformation 68, 287–297. doi:10.1016/j.jag.2017.12.015
Siegel, D. A., Wang, M., Maritorena, S., and Robinson, W. (2000). Atmospheric correction of satellite ocean color imagery: The black pixel assumption. Appl. Opt. 39, 3582–3591. doi:10.1364/ao.39.003582
Song, K., Li, L., Tedesco, L. P., Li, S., Hall, B. E., and Du, J. (2014). Remote quantification of phycocyanin in potable water sources through an adaptive model. ISPRS J. Photogrammetry Remote Sens. 95, 68–80. doi:10.1016/j.isprsjprs.2014.06.008
Stanley, E. H., Collins, S. M., Lottig, N. R., Oliver, S. K., Webster, K. E., Cheruvelil, K. S., et al. (2019). Biases in lake water quality sampling and implications for macroscale research. Limnol. Oceanogr. 64, 1572–1585. doi:10.1002/lno.11136
Stock, A. (2022). Spatiotemporal distribution of labeled data can bias the validation and selection of supervised learning algorithms: A marine remote sensing example. ISPRS J. Photogrammetry Remote Sens. 187, 46–60. doi:10.1016/j.isprsjprs.2022.02.023
Wang, M., and Gordon, H. R. (2018). Sensor performance requirements for atmospheric correction of satellite ocean color remote sensing. Opt. Express 26, 7390–7403. doi:10.1364/oe.26.007390
Wang, M. H., and Jiang, L. D. (2018). Atmospheric correction using the information from the short blue band. IEEE Trans. Geoscience Remote Sens. 56, 6224–6237. doi:10.1109/tgrs.2018.2833839
Wetzel, R. G. (2001). Limnology: Lake and river ecosystems. Third edition ed. San Diego: Academic Press.
Woolway, R. I., and Merchant, C. J. (2019). Worldwide alteration of lake mixing regimes in response to climate change. Nat. Geosci. 12, 271–276. doi:10.1038/s41561-019-0322-x
Xiong, J., Lin, C., Cao, Z., Hu, M., Xue, K., Chen, X., et al. (2022). Development of remote sensing algorithm for total phosphorus concentration in eutrophic lakes: Conventional or machine learning? Water Res. 215, 118213. doi:10.1016/j.watres.2022.118213
Yang, Y., Wang, Y., Zhang, Z., Wang, W., Ren, X., Gao, Y., et al. (2018). Diurnal and seasonal variations of thermal stratification and vertical mixing in a shallow fresh water lake. J. Meteorological Res. 32, 219–232. doi:10.1007/s13351-018-7099-5
Zhang, Y., Deng, J., Qin, B., Zhu, G., Zhang, Y., Jeppesen, E., et al. (2022). Importance and vulnerability of lakes and reservoirs supporting drinking water in China. Fundam. Res. doi:10.1016/j.fmre.2022.01.035
Zhang, Y. L., Jeppesen, E., Liu, X. H., Qin, B. Q., Shi, K., Zhou, Y. Q., et al. (2017). Global loss of aquatic vegetation in lakes. Earth-Science Rev. 173, 259–265. doi:10.1016/j.earscirev.2017.08.013
Zhang, Y., Shi, K., Liu, X., Zhou, Y., and Qin, B. (2014). Lake topography and wind waves determining seasonal-spatial dynamics of total suspended matter in turbid Lake Taihu, China: Assessment using long-term high-resolution MERIS data. PloS one 9, e98055. doi:10.1371/journal.pone.0098055
Keywords: satellite, eutrophication, lake, Do, water quality
Citation: Liu M, Wang L and Qiu F (2022) Using MODIS data to track the long-term variations of dissolved oxygen in Lake Taihu. Front. Environ. Sci. 10:1096843. doi: 10.3389/fenvs.2022.1096843
Received: 12 November 2022; Accepted: 09 December 2022;
Published: 22 December 2022.
Edited by:
Sijia Li, Chinese Academy of Sciences, ChinaReviewed by:
Shenglei Wang, Aerospace Information Research Institute(CAS), ChinaCopyright © 2022 Liu, Wang and Qiu. This is an open-access article distributed under the terms of the Creative Commons Attribution License (CC BY). The use, distribution or reproduction in other forums is permitted, provided the original author(s) and the copyright owner(s) are credited and that the original publication in this journal is cited, in accordance with accepted academic practice. No use, distribution or reproduction is permitted which does not comply with these terms.
*Correspondence: Li Wang, c3NoeXdhbmdsaUAxNjMuY29t
Disclaimer: All claims expressed in this article are solely those of the authors and do not necessarily represent those of their affiliated organizations, or those of the publisher, the editors and the reviewers. Any product that may be evaluated in this article or claim that may be made by its manufacturer is not guaranteed or endorsed by the publisher.
Research integrity at Frontiers
Learn more about the work of our research integrity team to safeguard the quality of each article we publish.