- 1Business School, The University of Queensland, Brisbane, QLD, Australia
- 2College of Geography and Environmental Science, Henan University, Kaifeng, China
- 3Guangdong Provincial Key Laboratory of Water Quality Improvement and Ecological Restoration for Watersheds, School of Ecology, Environment and Resources, Guangdong University of Technology, Guangzhou, China
Promoting animal husbandry industry is important to help strengthen the agricultural sector. This study starts from both socio-economic and natural factors perspectives. Based on an exploratory spatial analysis, spatial econometric model, and geographically weighted regression (GWR) model, selecting the 13 Provinces of North China as the study area, this study analyzes the spatio-temporal differences and the driving factors. This study found: 1) Between 2006 and 2017, the spatial and temporal differences of GDP in the 13 provinces were statistically significant, with increased total production values and a slowed growth of the industry. In addition, the study area showed an east-west dichotomy in husbandry industry. 2) GDP had shown obvious spatial agglomeration, of which areas with the high production value were in east of Beijing, and areas with low production value were in western Inner Mongolia, Xinjiang and Gansu. 3) Population, animal husbandry output values, numbers of large livestock, and cultivated areas had greatly influenced the GDP of 13 provinces in north China. 4) The three influencing factors on GDP in the 13 provinces were found to be population, animal husbandry output values, and the sizes of cultivated areas. We should synthesize these influencing factors, supplemented with public policy concessions and compensatory measures to develop policies for improving the level of local economic development. Based on this, this study aims to provide a reference for decision making to promote the coordinated development of livestock husbandry and economic growth in China, and even provide a scientific reference for the management of similar pastoral areas in the world.
1 Introduction
Animal husbandry, an important component of agriculture, is also a pillar industry that supports the survival and development of farmers and herdsmen (Weindl et al., 2017; Aleshina et al., 2020; Maryunani, 2021; Dai et al., 2022), especially in north China. The animal husbandry industry accounts for about half of the global agricultural economy and makes important contributions to global food security (Foley et al., 2011; Wang G. et al., 2016; Maryunani, 2021). However, global food demands are expected to continue to increase in the coming decades (Tilman et al., 2002; Tilman et al., 2011; Mueller et al., 2012). This is particularly the case in developing countries, where the consumption of meat, eggs, and milk is expected to increase significantly due to increased population and rising income levels (Godfray et al., 2010; Thornton, 2010; Kastner et al., 2012; Gerber et al., 2013; Ethier et al., 2017; Valenzuela-Lamas and Albarella, 2017; Parlato et al., 2022).
China has 393 million hectares of grasslands, accounting for 42% of the country’s total land area. With the continuing economic development in China and the living standards of herresidents continue to rise, along with the trend that the animal husbandry industry is shifting from traditional household production (self-consumption and local market allocation) to intensive industrial production (Wang X. et al., 2016; Tan et al., 2017; Cheng et al., 2022), demands for livestock output in China are projected to continue to increase in the near future. This growing demand for animal products, such as meat, eggs, and milk (Shimokawa, 2015; Makkar, 2016; Zhao X et al., 2017), has stimulated further development of the animal husbandry economy. As a result, the share of animal husbandry promoted from 14.98% during the early implementation of the Reform and Opening Up Policy in early 1980s to 28.28% in 2016 (Fu et al., 2012; Qu et al., 2021). However, compared to developed countries, this proportion is still relatively low when considering conditions in other developed countries. For example, the outputs of animal products in the United States, United Kingdom, and France account for 48%, 70%, and 80% of total agricultural output, respectively. Since animal husbandry in China relies mainly on natural and cultivated forage resources (Thornton and Gerber, 2010; Godber and Wall, 2014) and 90% of grasslands has suffered different some degrees of degradation due to overgrazing (Li et al., 2021), it is essential to explore the driving factors that influence the development of animal husbandry and their effects on the animal husbandry economy in order to adapt to and mitigate the impact of global changes in economic development.
Developing the animal husbandry economy to drive up GDP growth without sacrificing the ecological environment has become a focus of international research. Jayaraman and Nyachoti (2016) approached the subject from the perspective of livestock breeding and proposed that the effectiveness in breeding could be the key to a healthy livestock development. Liu et al. (2022a) and Yu et al. (2021) considered that the development of animal husbandry was, to some extent, affected by demographic factors. Influenced by global warming, climate in many regions had changed greatly, which could directly affect vegetation dynamics and biomass. Furthermore, climate changes would also influence the structure and a region’s carrying capacity of livestock (Bernués et al., 2011; Middleton et al., 2015; Yao et al., 2017). Morgan-Davies et al. (2012) studied animal husbandry in the hilly areas of northwestern Europe and found that climate changes and production barriers were the primary factors that hindered the production of animal husbandry and economic development in their study areas. Xie et al. (2022) assessed the vulnerability of animal husbandry toward global climate changes from a macro perspective. Similarly, the Qinghai-Tibet Plateau, an important pastoral area in China, was considered by Wei et al. (2017) and Liu et al. (2014) that global warming and the shortage of forage are the main reasons for the high vulnerability of the local animal husbandry. At the same time, the rapid growth in animal husbandry has led to further increases in the concentration of global greenhouse gases (GHG) in many regions (Hyland et al., 2016; Pardo et al., 2016; Sakadevan and Nguyen, 2017). To that end, Wang et al. (2021) and Zhuang and Li (2017) suggested that GHG emissions were influenced by animal husbandry industry in China. Scholars have also investigated the effects of grazing on the concentration of soil organic carbon (Hewins et al., 2018; Windirsch et al., 2022), phosphorus in grasslands (Sattari et al., 2016), and carbon emissions (Fischer et al., 2022).
In the aforementioned studies, although scholars have made certain achievements in research on the animal husbandry economy, the main regions of focus in those studies have been the United States and European countries, both are developed economies. But studies about China, on the other hand, have tended to adopt the perspective of environmental protection. Since China joined the World Trade Organization (WTO) in 2001, the proportion of animal products in exported agricultural products in its total economy has increased. Animal husbandry has gradually become a primary industry of the nation’s economy. There is insufficient research on the spatial spillover effects of factors that could affect the animal husbandry economy and their comprehensive impacts from both anthropogenic and natural environmental factors. In addition, the majority of the studies have approached the subject from a provincial and municipal level. However, studies that targeted larger, more generalized regions are few and limited. Moreover, existing research mostly utilized time-series analyses rather than a geospatial perspective to examine the spatial dependency of animal husbandry development at a regional level. Such approaches tend to assume one model fits all regions in a study area, i.e., spatial stationarity, which of course, is usually not the case in reality. Therefore, in light of the gaps in existing research and limitations of animal husbandry development in China, it is necessary to conduct a comprehensive study to uncover the driving factors of the development of animal husbandry and its contributions to China’s GDP. This study selected 13 provinces, including provincial municipalities and autonomous regions in north China, as the focus of research and 2006 to 2017 as the research period in order to comprehensively investigate the impact of regional animal husbandry on GDP. A spatio-temporal evolutionary perspective was adopted and a spatial analytical method was employed to explore influencing factors; an exploratory analysis method was introduced to measure the spatial differentiation of GDP of the 13 investigated provinces; an econometric model was constructed to verify the existence of a spatial spillover effect; and finally, GWR model was used to quantitatively analyze the different levels of influence by factors that affect the spatial distribution of GDP.
The purpose of the study is to provide a theoretical basis as a reference for decision-making and policies by concerned stakeholders, to facilitate the transformation of traditional animal husbandry to modern animal husbandry, to assist in the alleviation of the imbalance in the forage–animal relationship, and to explore new development models for animal husbandry that are in accordance with the characteristics of pasture areas. And in order to maintain a healthy development in animal husbandry and to create a solid foundation for a sustainable development of the agricultural economy in China.
2 Methods and data sources
2.1 Study area
The 13 provinces of north China (31°-54°N, 73°-136°E) in this study, consisting of 124 prefecture-level cities that span over three “steps” in China’s topographic “staircase” and four climate zones (wet/semi-wet and arid/semi-arid), accounts for about 57% of the total area of China (see Figure 1). The area has four zones four areas crossing wet, semi -humid, semi -drought and drought. The regional differences and transitionability of the climate are obvious, and the types of vegetation on the surface are diverse and the ecological environment is relatively fragile. The main grasslands, the agro-pastoral zone, and most of China’s desert areas are all located in the study area. With abundant water and forage resources, the region’s animal husbandry was the most widespread in the country. The 13 provinces are a representative area for research on animal husbandry development in China as well as serving as a model region for other developing countries with a similar scale of animal husbandry (Zhen et al., 2020; Yang et al., 2022).
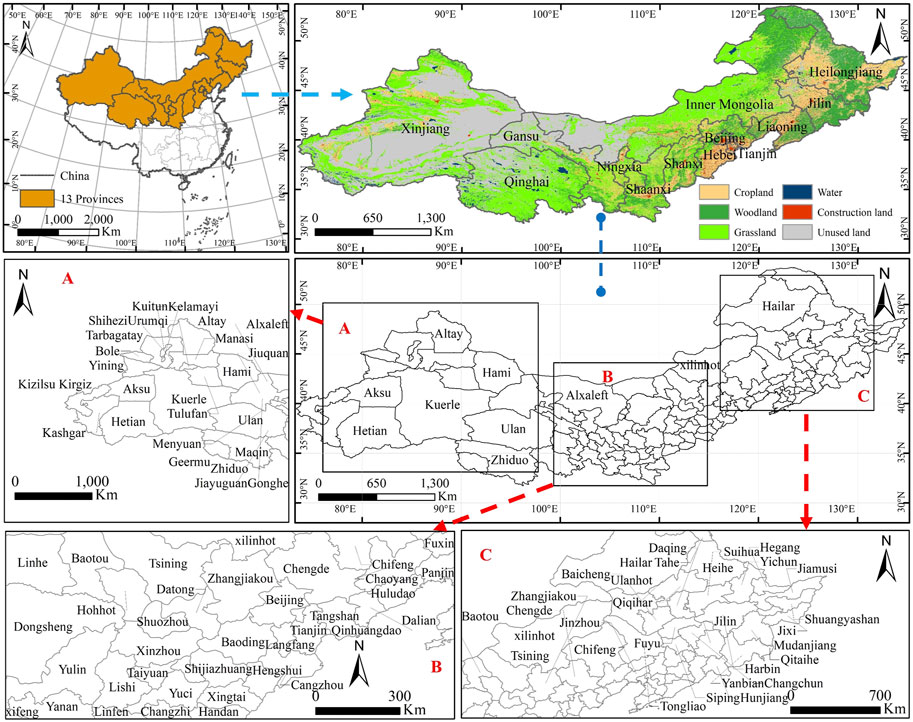
FIGURE 1. Map of study area. Note: A represents the western part of the study area, B represents the central part of the study area, C represents the eastern part of the study area.
2.2 Data sources
The data employed in this study were mainly included the following three types, which are shown in Table 1:
(1) Vector data of administrative regions: The vector data were created with maps at a scale of 1:250,000.
(2) Socio-economic data: The socioeconomic data used in this study include GDP, population, output values of animal husbandry, numbers of large livestock, numbers of cattle, numbers of sheep, and area sizes of arable land of all geographic units in the study area.
(3) Raster data: The raster data used in the study include classified images of grassland areas, surface temperature, and precipitation. The spatial resolution of the data was 1 km, which was sufficient to meet the research needs, considering the extent of the study area.
2.3 Methods
2.3.1 ESDA model
Exploratory spatial data analysis (ESDA) examines the spatial dependency (spatial autocorrelation) of attribute values of the 124 cities through data aggregation and spatial interaction to reveal the regional structure of spatial variables, including levels of global and local spatial autocorrelation (Rong et al., 2022; Shi et al., 2022). This study used the Global Moran’s I to examine if there existed statistically significant spatial autocorrelation among data values of the spatial units.
Global spatial autocorrelation describes only the level of spatial dependency among attribute values of the spatial units being examines. It does not consider any local spatial heterogeneity that may lead to the identification of the locations of the clusters and types of spatial associations. Therefore, this study used local spatial autocorrelation coefficients to describe the levels of spatial dependence and their different levels among the attribute values of the local area units. A local indicators of spatial association (LISA) map was constructed to identify the patterns of their local spatial differentiation.
2.3.2 Spatial econometric model
Spatial autocorrelation analysis helps to reveal the patterns and the levels of spatial clustering of GDP of the studied provinces from a spatial perspective; however, the method is unable to reveal the factors that influence the corresponding spatial effects. To explore the potential effect of a set of carefully selected indicators on the levels and patterns of spatial agglomeration of GDP under the spatial spillover effect, this study used the Lagrange multiplier (LM) test, which is the most commonly used test in ordinary least square (OLS) models, to construct a spatial lag model (SLM) and a spatial error model (SEM) (Zhao Y et al., 2017).
2.3.2.1 Variable selection
Economic development is the product of human activities and is influenced by factors from various dimensions. Most studies have approached the subject from the perspectives of physical geography, transportation advantages, human activities, and economic policies (Amarasinghe et al., 2005; Liu et al., 2022b). There exists only limited research on the impact of animal husbandry on economic development in China if compared to what had been done in other countries. In recent years, human activities, climate changes, and grassland management policies and management levels have been considered as the main drivers of grasslands in north China (Cheng et al., 2022). Therefore, based on past research and real-life factors in the investigated area, and considering the scientific nature, completeness, and availability of data, this study analyzed the influencing factors of GDP of the 13 provinces using both socio-economic and physical attributes of the cities in 13 provinces (Table 2). With GDP as the dependent variable, husbandry output, population, number of livestock, arable land, grassland, temperature and precipitation level were selected as the independent variables to explain the variation in GDP among the cities of the 13 provinces.
2.3.2.2 Econometric model
The SLM model mainly examines whether the spatial dependency between variables leads to spatial autocorrelation and whether adjacent regions have a diffusion (spillover) effect in the study area. The SEM model is mainly used to measure the influence of error in the dependent variable of adjacent regions on local observed values (Zhang et al., 2020a). The models can be presented by the following formula:
where, y is the dependent variable; ρ is the regression coefficients; W is the weight matrix; β is the correlation coefficients of the independent variables X; ε is the random error term; λ is the spatial error coefficient; and μ is the random error vector.
2.3.3 Geographically weighted regression (GWR)
The traditional OLS model is a non-spatial model that assumes spatial stationarity of the association between dependent and independent variables. It does not consider the spatial heterogeneity of model parameters and cannot reflect the differences in the impact of animal husbandry development on GDP when the geographic environment is not constant (i.e., spatial non-stationarity). This problem can be addressed by using the GWR model. Extended from the OLS model, GWR model embeds spatial factors and integrates the spatial dependency and spatial heterogeneity in the model (Zhang et al., 2020b; Chen et al., 2022). For that reason, this study introduced the GWR model as part of the analysis.
The GWR model can be used to quantitatively evaluate spatial instability, or what is often referred to as spatial non-stationarity, of data values. It has been applied widely in different fields (Cohen et al., 2015; Huang et al., 2015). The model can be represented by the following formula:
where, (ui, vi) signifies the spatial position of region i; β0 (ui, vi) is a constant term; k indicates the number of independent variables; βk (ui, vi) is the value of the continuous function β (u, v) at point (ui, vi); xik is the value of the kth independent variable of i; and εi is the error term.
3 Results
3.1 Characteristics of the spatio-temporal evolution of animal husbandry
GDP is perhaps the best indicator that can reflect the economic level for a country/region comprehensively. The contribution of animal husbandry to GDP is of particular importance for the analysis in this study. Given that the development of animal husbandry is mainly manifested by its output value, this study used the output values of animal husbandry to support the analysis of the contribution of animal husbandry development on GDP.
Figure 2 shows that between 2006 and 2017, the GDP of the investigated provinces continued to grow with decreasing fluctuations in the growth rates over time. Specifically, GDP increased from 3.073 trillion yuan (RMB) in 2006 to 16.907 trillion yuan in 2017. Animal husbandry has long been the main driving force for economic development in the study area. Owing to the implementation of key region-wide projects, such as animal protection, improvement in the breeding system of livestock and poultry, and restoring grazing lands to grasslands, the increases of GDP reached a peak value in 2004. Thereafter, affected by the global financial crisis and livestock and poultry diseases in 2012 and 2013, the growth began to slow down. Thus, this study selected 2006 (the first year of the study period), 2009, 2012 (both years with noticeable changes in the growth of GDP), and 2017 (the last year of the study period) to further explore the spatial differences in GDP growth and output values of animal husbandry in the study area (Figure 3). The results showed that GDP were higher in the eastern regions but lower in the western regions of the study area. This agglomerated trend became more apparent as time went on. Moreover, the outputs of animal husbandry were positively correlated with changes in GDP. These trends could be related to the characteristics of different climate zones and land types of the region. Specifically, western areas of the study areas mostly suffered from desertification and grassland desertification. Owing to environmental factors (e.g., land desertification and reduced biodiversity), GDP and output of animal husbandry in the western areas of the study areas were lower than that of the eastern areas in the study areas.
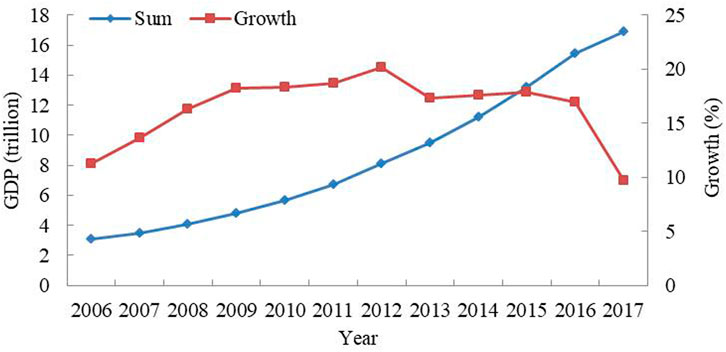
FIGURE 2. Evolutional Trends of GDP and Growth in the 13 Provinces of North China (2006–2017). Note: Sum (Total GDP); Growth (Growth of GDP).
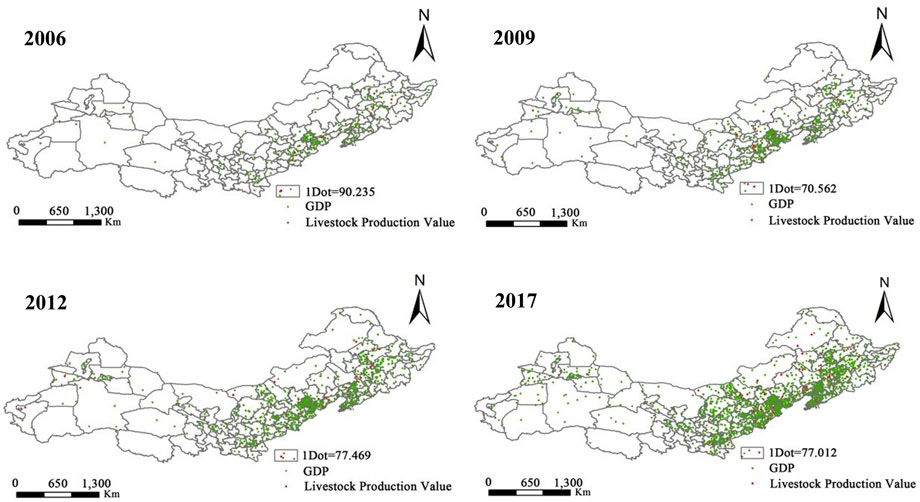
FIGURE 3. Spatial Patterns of GDP and Livestock Production Values in the 13 Provinces of North China (2006, 2009, 2012, and 2017).
3.2 Verification of spatial dependency
3.2.1 Verification of global spatial autocorrelation of GDP
To explore the spatial relationship of the adjacent areas, this study calculated the Moran’s I values of GDP in the 13 Provinces of North China between 2006 and 2017. Table 3 shows, as for GDP, the values of Global Moran’s I were calculated (Table 3) based on Eq. 1. The values of Moran’s I were all positive and were statistically significant. They indicate a spatially agglomerated distribution of the variable. The Z-scores of the calculated index values were greater than 2.58 (p < 0.01), indicating that the GDP of the provinces had significant and positive spatial autocorrelation, which means provinces with high GDP tended to cluster together as did among those with and low GDP. In addition, as shown in Table 2, GDP in 2009 and 2012 showed a trend of recovery following a declining trend before 2004. This trend is consistent with the overall growth trend. These results further verify that 2006, 2009, and 2012 are critical years during the study period.
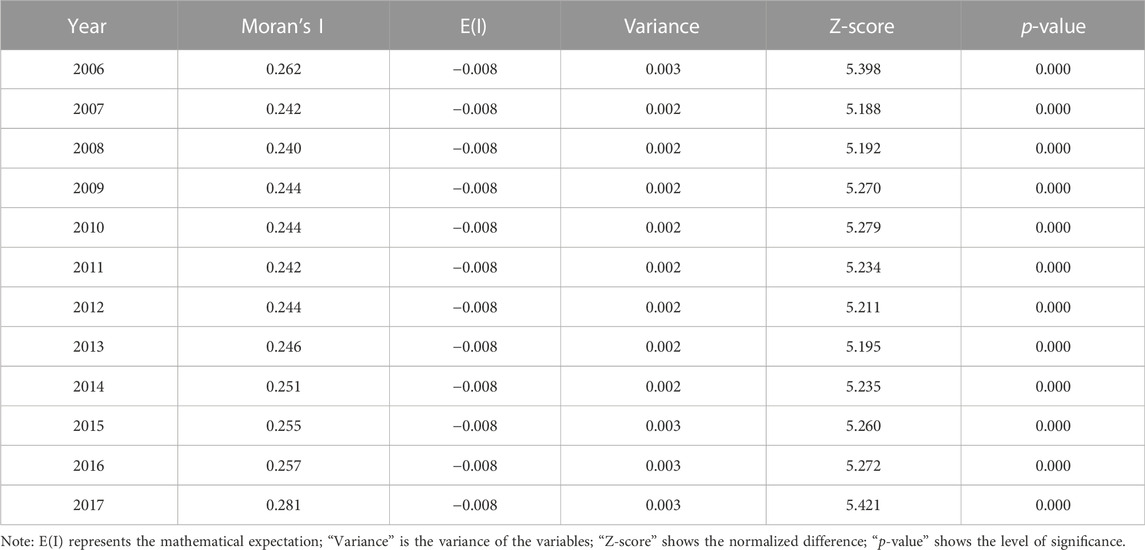
TABLE 3. Spatial autocorrelation index of GDP in the 13 provinces of North China between 2006 and 2017.
3.2.2 LISA map of animal husbandry development
The global spatial autocorrelation analysis shows the overall spatial agglomeration pattern of GDP. Results assist the selection of explanatory variables when constructing the research model; however, they do not indicate the trend of aggregation of high and low values between regions in the study area. Thus, a series of LISA maps were created to visualize the spatial patterns of GDP over time to explore the spatial agglomeration of the GDP between neighboring cities in the 13 provinces of north China (Figure 4).
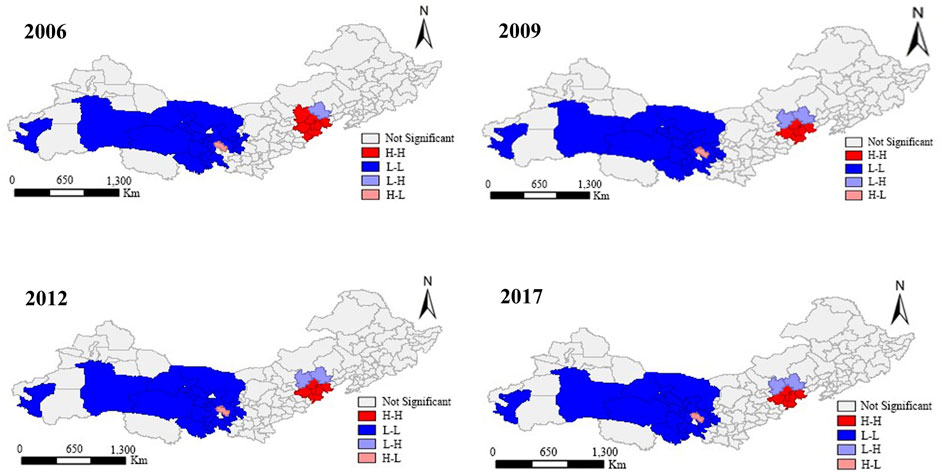
FIGURE 4. LISA Aggregation Map of GDP in the 13 Provinces of North China (2006–2017). Note: H–H, L–L, L–H, and H–L represent high–high, low–low, low–high, and high–low aggregation, respectively.
Figure 4 shows, cities with high GDP, such as Beijing, Baoding, Langfang, Tianjin, Zhangzhou, and Tangshan in the eastern area, were found to be aggregated spatially (high–high agglomeration, H–H). Zhangjiakou and Chengde, two cities to the north of the H–H zone, appeared to be agglomerated towards the H–H zone. Cities with low GDP, such as Inner Mongolia, Xinjiang, and Gansu in the western part of the study areas, were also found to be spatially agglomerated (low–low agglomeration). The local spatial agglomerations among other provinces and cities were not significant. Moreover, during the study period, no apparent changes were observed in the statistically spatial agglomeration pattern of GDP among the investigated provinces. The results showed fewer H–H agglomerations in the eastern parts of the study area. A possible explanation is that, although the climate changes and land use types in the eastern areas are more suitable for animal husbandry development, the economic development and livestock and poultry farming techniques of the investigated cities were different. As a result, the overall GDP of cities in the eastern areas were higher than that in the west; however, local agglomeration was not prominent.
3.3 Verification with spatial econometric model
Moran’s I and LISA maps above revealed that the pattern of spatial autocorrelation of the GDP among 13 provinces were statistically significant. To further uncover the quantitative relationship between animal husbandry development and GDP, this study carried out a spatial econometric analysis, using the factors that had significant associations with animal husbandry.
3.3.1 Influencing factors of animal husbandry development
Referring to the determining criteria proposed by Anselin (1995), when the results of the LM tests for the missing spatially lagged dependent variable (LM-lag) and error dependence (LM-err) are both insignificant, the estimation results of the OLS model are considered better to explain the spatial autocorrelation among cities; however, if only one of the test results is significant, the OLS estimation results can be seen as biased and require further analysis with a more appropriate spatial econometric model (Lin and Long, 2014; Zhu et al., 2017). In other words, the basic principle is that, when the results of the LM-lag test are statistically more significant than those of the LM-err test, and the robust LM-lag is significant while the robust LM-err is insignificant, an SLM should be adopted; otherwise, the SEM ought to be adopted (Anselin, 1988; Geng et al., 2022). These results can be seen in Table 4.
Table 3 shows that the LM-err test of GDP in 2006, 2009, 2012, and 2017 were more prominent than those of the LM-lag test, indicating that the SEM is more suitable for analyzing GDP of the investigated years, as the spatial effect of GDP in the region depended on the influence of adjacent cities, rather than solely on itself. For that reason, it was necessary to further verify and analyze the SEM of GDP in the corresponding years.
3.3.2 Testing the spatial econometric model
According to the Table 4, the R2 values of the SEM of GDP in the years of 2006, 2009, 2012, and 2017 were 0.829, 0.813, 0.796, and 0.792, respectively. These levels were higher than those of the fit of the OLS models. Hence, the SEM was more suitable than OLS estimation. Moreover, population was found to be the most influential factor in promoting GDP growth throughout the 4-year period (p < 0.01), followed by the output values of animal husbandry (p < 0.01). However, the area of arable land and the number of large livestock units appeared to inhibit GDP growth.
3.4 Results of the GWR model
GWR model was constructed to address the issue of spatial non-stationarity that may exist among attribute data of the cities in the study area. GDP, Y, for each city in 2006, 2009, 2012, and 2017 were introduced as the dependent variable of the model, and X1, X2, X3, X4, X5, X6, X7, X8, and X9 in Table 1 were the independent variables. The OLS test was performed several times and the variables with multi-collinearity problem were eliminated according to significance levels and variance inflation factor (VIF). In order to eliminate the dimensional difference between metrics, the logarithm of each indicator was used for the analysis (Table 5).Since the estimated parameters vary with the independent variables, to further explore the spatial differences in the effects of the variables on GDP, the estimated coefficients of the cities were spatially visualized in Figures 5–8.
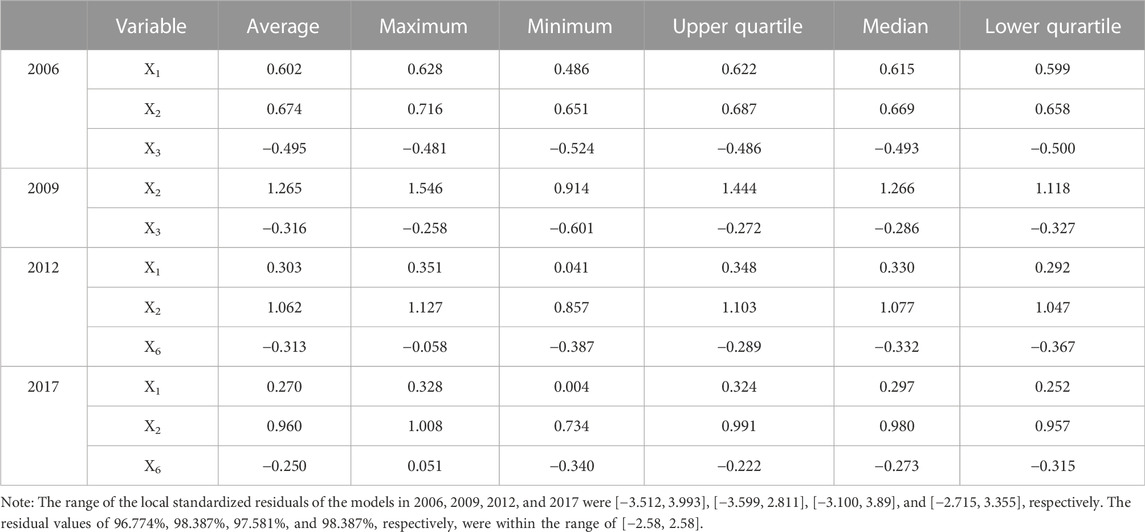
TABLE 5. Descriptive statistics of the regression coefficients in the GWR model (2006, 2009, 2012, and 2017).
Table 6 shows that, the impacts of X1, X2, and X3 on GDP were spatially different in 2006. Specifically, X1 and X2 had a positive effect on GDP, indicating that the output values of animal husbandry remained the primary income of the region. In addition, population, as the main influencing factor of economic development, played an irreplaceable role. The effect of X3 on GDP was negative, which is consistent with the results obtained from the spatial econometric models, confirming that the models’ goodness of fit was satisfactory.
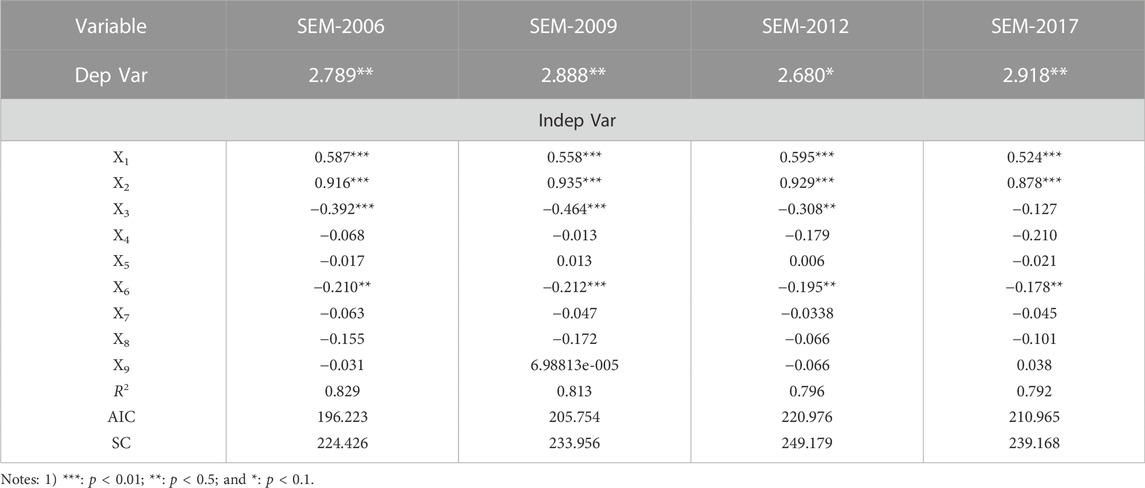
TABLE 6. Spatial regression results of GDP of the 13 province’s in North China (2006, 2009, 2012, and 2017).
The distribution of the regression coefficients (Figure 5) reveals that, apart from the coefficients of X2 of 11 cities (e.g., Kashgar Prefecture, Kizilsu Kirghiz Autonomous Prefecture, and Aksu City region), the coefficients of X1, X2, and X3 of the eastern regions were higher than those of the western regions. The reason that the coefficient distribution of the 11 cities did not follow the overall distribution pattern could be that the 11 cities are locations of military bases; hence, the coefficients of population were higher than those of other regions. In 2009, affected by animal diseases, only the influences of X2 (positive effect) and X3 (negative effect) on GDP were found to be spatially different. Apart from a few cities that showed spatial fluctuations of the regression coefficients, the pattern of changes was statistically significant (Figures 5, 6, respectively). In 2012 and 2017, the influences of X1, X2, and X6 showed spatial differences. In addition, no apparent changes were observed in the spatial distribution of the regression coefficients of the three variables (Figures 5, 7, 8, respectively). The coefficients of X1 and X2 were higher in the east than those in the west, while that of X6 was higher in the west than that in the east. This phenomenon is mainly associated with the physical conditions in the region (distribution of land type and topography), in addition to the influence of human activities. Specifically, forest resources in northeast China accounted for one-third of the country’s resources; while the level of influence on husbandry by arable land resources in Xinjiang was the highest in the country. Therefore, the regression coefficients of arable land in the western regions were higher than those in the eastern regions.
In summary, during 2006–2017, the factors that have a significant impact on the GDP of the 13 provinces in north China were the output values of animal husbandry, population, numbers of large livestock units, and areas of arable land. Apart from the areas of arable land, the regression coefficients of the influencing factors were higher in the east and lower in the west. These findings suggest that, in order to promote the economic development in the study area, it is necessary to stimulate the growth of animal husbandry and increase investment in animal husbandry based on the local conditions. In addition, the imbalance in the forage-animal relationship should be addressed and new methods that suit the pasture area should be designed both to promote animal husbandry development and to ensure the protection of local ecology.
4 Discussion
China has a large animal husbandry industry. Targeting the main region of animal husbandry production, this study investigated the spatial characteristics of GDP of the provinces and applied GWR model to reveal the spatial heterogeneity of the corresponding drivers.
4.1 Spatiotemporal heterogeneity analysis
According to the spatial differences in GDP growth and output values of animal husbandry, the results showed that GDP were higher in the eastern regions but lower in the west of the study area. This agglomerated trend became more apparent as time went on. Moreover, the outputs of animal husbandry were positively correlated with changes in GDP. These trends could be related to the characteristics of different climate zones and land types of the region. Wei et al. (2022) study found that the accelerated expansion of cities in the middle of the study area, and the habitat quality has also declined. Yu et al. (2021) found that regional heterogeneity of water resources severely limits the development of animal husbandry. Specifically, western areas of the study areas mostly suffered from desertification and grassland desertification. Owing to environmental factors (e.g., land desertification and reduced biodiversity), GDP and output of animal husbandry in the western areas of the study areas were lower than that of the eastern areas.
4.2 Exploring localized development
The spatial error model results (Table 4) shows that promotion of agricultural and animal husbandry development should suit local conditions, such as climate and land use type. Rapid urbanization causes large urban conversions of natural and agricultural land to non-agricultural use (Zhou et al., 2021). This result will inevitably affect the animal husbandry industry. Moreover, the results show that GDP growth was not necessarily linked to the number of livestock and poultry units, but rather, it may be associated with the production, processing, and marketing methods for animal products. Thus, the results suggest that concerned parties should control the total number of livestock in each city based on the local conditions of the cities, accelerate the transition from traditional to modern animal husbandry, increase the industrialization of animal husbandry, and improve the protection of livestock against diseases. These practices would help to promote an animal husbandry industry geared towards standardization, scalability, and industrialization. From 2006 to 2017, the factors that have a significant impact on the GDP of the 13 northern provinces of China are the output value of animal husbandry, population, the number of livestock, and the area of arable land. Except for the area of arable land, the regression coefficients of other factors are all east high in spatial distribution. The low west situation indicates that to improve the regional economic development level, it is necessary to promote the growth of animal husbandry output according to local conditions, increase investment in animal husbandry, alleviate the imbalance of grass-livestock relationship, and explore the new model of ecological protection and animal husbandry development suitable for the characteristics of pastoral areas.
4.3 Limitations and future directions
This study still has uncertainties. This study explores the spatio-temporal differences and the spatial heterogeneity of factors influencing the varying animal husbandry development of the 13 provinces in the northern China. Animal husbandry is a major component of agriculture. The development of animal husbandry could help alleviate the poverty of farmers and herders significantly and promote effective social supply (Wang and Tan, 2022). The modernization of agriculture through the modernization of animal husbandry would help promote the goal of constructing a strong agricultural sector (Ministry of Agriculture and rural affairs of the people’s republic of China, 2018). The influence of human and factors of physical environment on the development of animal husbandry has become increasingly complex. Therefore, concerned parties may want to consider additional influencing factors, make use of relevant favorable policies and government subsidies, and explore new developmental models to facilitate the development of the local economy.
It is noteworthy that, since this study focused on the effect of animal husbandry on GDP, the direct influences of some indicators on GDP were not explored in detail. To further investigate the impact of animal husbandry development on the national economy, we suggest that future studies use the size of the agricultural sector as an independent variable.
5 Conclusion
Exploring the development of animal husbandry is of great significance to stabilizing the people’s living standards. This study found, the spatio-temporal differences of GDP were prominent, presenting an increasing trend from west to east. Owing to regional differences in economic development and techniques of livestock and poultry farming, cities with higher GDP tended to concentrate in the east. The impact of the factors (excluding numbers of large livestock units) on GDP, from the largest to the smallest were population, output values of animal husbandry, and areas of arable land. At the early stage of the study period, controlling population (human activities), stimulating the output of animal husbandry, and controlling the numbers of large livestock units (improving techniques for livestock and poultry farming) had a great impact on GDP. At the latter stage of the study period, besides population and animal husbandry output, controlling the areas of arable land gradually became an important driving factor of GDP growth. These results can serve as a scientific reference for accelerating the transformation of animal husbandry in the 13 provinces of north China and for exploring new ways suitable for promoting local animal husbandry development.
Data availability statement
The original contributions presented in the study are included in the article/Supplementary Material, further inquiries can be directed to the corresponding author.
Author contributions
Conceptualization, CW and WZ; methodology, CW and JZ; software, CW and JZ; formal analysis, CW and WZ; investigation, CW and JZ; resources, CW; data curation, CW and WZ; writing—original draft preparation, CW; writing—review and editing, CW and WZ; project administration, WZ; funding acquisition, WZ. All authors have read and agreed to the published version of the manuscript.
Funding
The GDAS’ Project of Science and Technology Development (Grant No. 2022GDASZH-2022010202), and the Science and Technology Program of Guangdong (Grant No. 2021B1212100006).
Conflict of interest
The authors declare that the research was conducted in the absence of any commercial or financial relationships that could be construed as a potential conflict of interest.
Publisher’s note
All claims expressed in this article are solely those of the authors and do not necessarily represent those of their affiliated organizations, or those of the publisher, the editors and the reviewers. Any product that may be evaluated in this article, or claim that may be made by its manufacturer, is not guaranteed or endorsed by the publisher.
References
Aleshina, E. A., Anisimova, E. I., and Serdobintsev, D. V. (2020). Agroindustrial clustering as a driver of the activization of breeding work in animal husbandry. IOP Conf. Ser. Earth Environ. Sci. 459 (6), 062018. doi:10.1088/1755-1315/459/6/062018
Amarasinghe, U., Samad, M., and Anputhas, M. (2005). Spatial clustering of rural poverty and food insecurity in Sri Lanka. Food Policy 30 (5-6), 493–509. doi:10.1016/j.foodpol.2005.09.006
Anselin, L. (1995). Local indicators of spatial association—Lisa. Geogr. Anal. 27 (2), 93–115. doi:10.1111/j.1538-4632.1995.tb00338.x
Anselin, L. (1988). Spatial econometrics: Methods and models. Econ. Geogr. 65 (2), 160. doi:10.1007/978-94-015-7799-1
Bernués, A., Ruiz, R., Olaizola, A., Villalba, D., and Casasús, I. (2011). Sustainability of pasture-based livestock farming systems in the European Mediterranean context: Synergies and trade-offs. Livest. Sci. 139 (1-2), 44–57. doi:10.1016/j.livsci.2011.03.018
Chen, Z., Zhang, S., Geng, W., Ding, Y., and Jiang, X. (2022). Use of geographically weighted regression (GWR) to reveal spatially varying relationships between Cd accumulation and soil properties at field scale. Land 11 (5), 635. doi:10.3390/LAND11050635
Cheng, W., Shen, B., Xin, X., Gu, Q., and Guo, T. (2022). Spatiotemporal variations of grassland ecosystem service value and its influencing factors in inner Mongolia, China. Agronomy 12 (9), 2090. doi:10.3390/AGRONOMY12092090
Cohen, J., Cromley, R., and Banach, K. (2015). Are homes near water bodies and wetlands worth more or less? An analysis of housing prices in one C onnecticut town. Growth Change 46 (1), 114–132. doi:10.1111/grow.12073
Dai, Y., Guo, J., Li, Y., Dong, Z., and Li, H. (2022). Soil physical and chemical properties affected by long-term grazing on the desert steppe of Inner Mongolia, China. Catena 211, 105996. doi:10.1016/J.CATENA.2021.105996
Ethier, J., Bánffy, E., Vuković, J., Leshtakov, K., Bacvarov, K., Roffet-Salque, M., et al. (2017). Earliest expansion of animal husbandry beyond the Mediterranean zone in the sixth millennium BC. Sci. Rep. 7 (1), 7146. doi:10.1038/s41598-017-07427-x
Fischer, W., Thomas, C., Zimov, N., and Göckede, M. (2022). Grazing enhances carbon cycling but reduces methane emission during peak growing season in the Siberian Pleistocene Park tundra site. Biogeosciences 19 (6), 1611–1633. doi:10.5194/BG-19-1611-2022
Foley, J. A., Ramankutty, N., Brauman, K. A., Cassidy, E. S., Gerber, J. S., Johnston, M., et al. (2011). Solutions for a cultivated planet. Nature 478 (7369), 337–342. doi:10.1038/nature10452
Fu, Q., Zhu, Y., Kong, Y., and Sun, J. (2012). Spatial analysis and districting of the livestock and poultry breeding in China. J. Geogr. Sci. 22 (6), 1079–1100. doi:10.1007/s11442-012-0984-4
Geng, W., Li, Y., Zhang, P., Yang, D., Jing, W., and Rong, T. (2022). Analyzing spatio-temporal changes and trade-offs/synergies among ecosystem services in the Yellow River Basin, China. Ecol. Indic. 138, 108825. doi:10.1016/J.ECOLIND.2022.108825
Gerber, P. J., Hristov, A. N., Henderson, B., Makkar, H., Oh, J., Lee, C., et al. (2013). Technical options for the mitigation of direct methane and nitrous oxide emissions from livestock: A review. Animal 7, 220–234. doi:10.1017/S1751731113000876
Godber, O. F., and Wall, R. (2014). Livestock and food security: Vulnerability to population growth and climate change. Glob. Chang. Biol. 20 (10), 3092–3102. doi:10.1111/gcb.12589
Godfray, H., Beddington, J. R., Crute, I., Haddad, L., Lawrence, D., Muir, J., et al. (2010). Food security: The challenge of feeding 9 billion people. Science 327 (5967), 812–818. doi:10.1126/science.1185383
Hewins, D., Lyseng, M., Schoderbek, D., Alexander, M., Willms, W., Carlyle, C., et al. (2018). Grazing and climate effects on soil organic carbon concentration and particle-size association in northern grasslands. Sci. Rep. 8 (1), 1336–1339. doi:10.1038/s41598-018-19785-1
Huang, J., Huang, Y., Pontius, R., and Zhang, Z. (2015). Geographically weighted regression to measure spatial variations in correlations between water pollution versus land use in a coastal watershed. Ocean. Coast. Manag. 103, 14–24. doi:10.1016/j.ocecoaman.2014.10.007
Hyland, J., Styles, D., Jones, D., and Williams, A. (2016). Improving livestock production efficiencies presents a major opportunity to reduce sectoral greenhouse gas emissions. Agric. Syst. 147, 123–131. doi:10.1016/j.agsy.2016.06.006
Jayaraman, B., and Nyachoti, C. M. (2016). Husbandry practices and gut health outcomes in weaned piglets: A review. Anim. Nutr. 3 (3), 205–211. doi:10.1016/j.aninu.2017.06.002
Kastner, T., Rivas, M. J., Koch, W., and Nonhebel, S. (2012). Global changes in diets and the consequences for land requirements for food. Proc. Natl. Acad. Sci. U. S. A. 109 (18), 6868–6872. doi:10.1073/pnas.1117054109
Li, M., Liu, S., Sun, Y., and Liu, Y. (2021). Agriculture and animal husbandry increased carbon footprint on the Qinghai-Tibet Plateau during past three decades. J. Clean. Prod. 278, 123963. doi:10.1016/j.jclepro.2020.123963
Lin, G., and Long, Z. (2014). Spatial economic measurement: Theory and demonstration. Beijing: Science Press.
Liu, F., Mao, X., Zhang, Y., Chen, Q., Liu, P., and Zhao, Z. (2014). Risk analysis of snow disaster in the pastoral areas of the Qinghai-Tibet Plateau. J. Geogr. Sci. 24 (3), 411–426. doi:10.1007/s11442-014-1097-z
Liu, Z., Ahmad, M., Li, G., Yang, Y., Liu, Y., Gao, M., et al. (2022a). Decoupling of greenhouse gas emissions from livestock industrial development: Evidence from China agricultural green development modern zone. Front. Environ. Sci. 10 (1), 979129. doi:10.3389/FENVS.2022.979129
Liu, Z., Yang, D., Zhang, P., Jiang, L., Li, Y., Rong, T., et al. (2022b). Spatial–temporal characteristics and scenario simulation of carbon emissions from energy consumption based on multiscale in the affected areas of the lower Yellow River. Int. J. Low-Carbon Tec. 17, 818–830. doi:10.1093/IJLCT/CTAC027
Makkar, H. P. (2016). Animal nutrition in a 360-degree view and a framework for future R&D work: Towards sustainable livestock production. Anim. Prod. Sci. 56 (10), 1561–1568. doi:10.1071/AN15265
Maryunani, M. (2021). Establishment of regional-owned food business entities as a means to eradicate poverty and increase economic growth. SOCA J. Sosial Ekonomi Pertanian. 15 (1), 42–54. doi:10.24843/SOCA.2021.v15.i01.p04
Middleton, N., Rueff, H., Sternberg, T., Batbuyan, B., and Thomas, D. (2015). Explaining spatial variations in climate hazard impacts in Western Mongolia. Landsc. Ecol. 30 (1), 91–107. doi:10.1007/s10980-014-0091-2
Ministry of Agriculture and rural affairs of the people’s republic of China (2018). Essentials of animal husbandry. Available at http://www.moa.gov.cn/ztzl/ncgzhy2017/zxdt/201802/t20180203_6136401.htm.
Morgan-Davies, C., Waterhouse, T., and Wilson, R. (2012). Characterisation of farmers’ responses to policy reforms in Scottish hill farming areas. Small Rumin. Res. 102 (2-3), 96–107. doi:10.1016/j.smallrumres.2011.07.013
Mueller, N. D., Gerber, J. S., Johnston, M., Ray, D. K., Ramankutty, N., and Foley, J. A. (2012). Closing yield gaps through nutrient and water management. Nature 490 (7419), 254–257. doi:10.1038/nature11420
Pardo, G., Martin-Garcia, I., Arco, A., Yañez-Ruiz, D., Moral, R., and Del Prado, A. (2016). Greenhouse-gas mitigation potential of agro-industrial by-products in the diet of dairy goats in Spain: A life-cycle perspective. Anim. Prod. Sci. 56 (3), 646–654. doi:10.1071/AN15620
Parlato, M., Valenti, F., Midolo, G., and Porto, S. (2022). livestock wastes sustainable use and management: Assessment of raw sheep wool reuse and valorization. Energies 15 (9), 3008. doi:10.3390/EN15093008
Qu, Y., Zhao, Y., Ding, G., Chi, W., and Gao, G. (2021). Spatiotemporal patterns of the forage-livestock balance in the xilin gol steppe, China: Implications for sustainably utilizing grassland-ecosystem services. J. Arid. Land 13 (2), 135–151. doi:10.1007/S40333-021-0053-X
Rong, T., Zhang, P., Zhu, H., Jiang, L., Li, Y., and Liu, Z. (2022). Spatial correlation evolution and prediction scenario of land use carbon emissions in China. Ecol. Inf. 71, 101802. doi:10.1016/J.ECOINF.2022.101802
Sakadevan, K., and Nguyen, M. (2017). Livestock production and its impact on nutrient pollution and greenhouse gas emissions. Adv. Agron. 141, 147–184. doi:10.1016/bs.agron.2016.10.002
Sattari, S., Bouwman, A., Martinez Rodríguez, R., Beusen, A., and Van Ittersum, M. (2016). Negative global phosphorus budgets challenge sustainable intensification of grasslands. Nat. Commun. 7 (1), 10696. doi:10.1038/ncomms10696
Shi, Y., Han, R., and Guo, L. (2022). Temporal–spatial distribution of ecosystem health and its response to human interference based on different terrain gradients: A case study in gannan, China. Sustainability 12 (5), 1773. doi:10.3390/su12051773
Shimokawa, S. (2015). Sustainable meat consumption in China. J. Integr. Agric. 14 (6), 1023–1032. doi:10.1016/S2095-3119(14)60986-2
Tan, S. H., Liu, B., Zhang, Q. Y., Zhu, Y., Yang, J. H., and Fang, X. J. (2017). Understanding grassland rental markets and their determinants in eastern inner Mongolia, PR China. Land Use Policy 67, 733–741. doi:10.1016/j.landusepol.2017.07.006
Thornton, P. K., and Gerber, P. J. (2010). Climate change and the growth of the livestock sector in developing countries. Mitig. Adapt. Strateg. Glob. Chang. 15 (2), 169–184. doi:10.1007/s11027-009-9210-9
Thornton, P. K. (2010). Livestock production: Recent trends, future prospects. Phil. Trans. R. Soc. B 365 (1554), 2853–2867. doi:10.1098/rstb.2010.0134
Tilman, D., Balzer, C., Hill, J., and Befort, B. L. (2011). Global food demand and the sustainable intensification of agriculture. Proc. Natl. Acad. Sci. U. S. A. 108 (50), 20260–20264. doi:10.1073/pnas.1116437108
Tilman, D., Cassman, K. G., Matson, P. A., Naylor, R., and Polasky, S. (2002). Agricultural sustainability and intensive production practices. Nature 418 (6898), 671–677. doi:10.1038/nature01014
Valenzuela-Lamas, S., and Albarella, U. (2017). Animal husbandry across the western roman empire: Changes and continuities. Eur. J. Archaeol. 20 (3), 402–415. doi:10.1017/eaa.2017.22
Wang, F., Chen, Y., Li, T., Wang, C., Wang, D., Fu, B., et al. (2021). Grazing reduces the soil-atmosphere exchange of greenhouse gases during freeze-thaw cycles in meadow steppes in inner Mongolia. Front. Ecol. Evol. 9, 795203. doi:10.3389/FEVO.2021.795203
Wang, G., Wang, M., Wang, J., Yang, C., and Liu, Y. (2016). Characteristics and influencing factors of grass-feeding livestock breeding in China: An economic geographical perspective. J. Geogr. Sci. 26 (4), 501–512. doi:10.1007/s11442-016-1282-3
Wang, L., and Tan, H. (2022). Economic analysis of animal husbandry based on system dynamics. Comput. Intell. Neurosci. 2022, 1–13. doi:10.1155/2022/5641384
Wang X.,, , Wu, X., Yan, P., Gao, W., Chen, Y., and Sui, P. (2016). Integrated analysis on economic and environmental consequences of livestock husbandry on different scale in China. J. Clean. Prod. 119, 1–12. doi:10.1016/j.jclepro.2016.01.084
Wei, L., Zhou, L., Sun, D., Yuan, B., and Hu, F. (2022). Evaluating the impact of urban expansion on the habitat quality and constructing ecological security patterns: A case study of jiziwan in the yellow river basin, China. Ecol. Indic. 145, 109544. doi:10.1016/j.ecolind.2022.109544
Wei, Y., Wang, S., Fang, Y., and Nawaz, Z. (2017). Integrated assessment on the vulnerability of animal husbandry to snow disasters under climate change in the Qinghai-Tibetan Plateau. Glob. Planet. Change 157, 139–152. doi:10.1016/j.gloplacha.2017.08.017
Weindl, I., Bodirsky, B. L., Rolinski, S., Biewald, A., Lotze-Campen, H., Müller, C., et al. (2017). Livestock production and the water challenge of future food supply: Implications of agricultural management and dietary choices. Glob. Environ. Change 47, 121–132. doi:10.1016/j.gloenvcha.2017.09.010
Windirsch, T., Grosse, G., Ulrich, M., Forbes, B., Göckede, M., Wolter, J., et al. (2022). Large herbivores on permafrost— A pilot study of grazing impacts on permafrost soil carbon storage in northeastern siberia. Front. Environ. Sci. 1, 893478. doi:10.3389/FENVS.2022.893478
Xie, S., Ding, W., Ye, W., and Deng, Z. (2022). Agro-pastoralists’ perception of climate change and adaptation in the Qilian Mountains of northwest China. Sci. Rep. 12 (1), 12689. doi:10.1038/S41598-022-17040-2
Yang, D., Zhang, P., Jiang, L., Jiang, L., Zhang, Y., Liu, Z., et al. (2022). Spatial change and scale dependence of built-up land expansion and landscape pattern evolution—case study of affected area of the lower yellow river. Ecol. Indic. 141, 109123. doi:10.1016/J.ECOLIND.2022.109123
Yao, Z., Zhang, L., Tang, S., Li, X., and Hao, T. (2017). The basic characteristics and spatial patterns of global cultivated land change since the 1980s. J. Geogr. Sci. 27 (7), 771–785. doi:10.1007/s11442-017-1405-5
Yu, L., Xiong, X., Samim, S., and Hu, Z. (2021). Analysis of water resources and water environmental carrying capacity of animal husbandry in China—based on water footprint theory. Water 13 (23), 3386. doi:10.3390/W13233386
Zhang, P., Yang, D., Qin, M., and Jing, W. (2020a). Spatial heterogeneity analysis and driving forces exploring of built-up land development intensity in Chinese prefecture-level cities and implications for future urban land intensive use. Land Use Policy 99, 104958. doi:10.1016/j.landusepol.2020.104958
Zhang, Y., Geng, W., Zhang, P., Li, E., Rong, T., Liu, Y., et al. (2020b). Dynamic changes, spatiotemporal differences and factors influencing the urban eco-efficiency in the lower reaches of the Yellow River. Int. J. Environ. Res. Public Health 17 (20), 7510. doi:10.3390/ijerph17207510
Zhao, X., Deng, C., Huang, X., and Kwan, M. (2017). Driving forces and the spatial patterns of industrial sulfur dioxide discharge in China. Sci. Total Environ. 577, 279–288. doi:10.1016/j.scitotenv.2016.10.183
Zhao, Y., Xie, K. Y., Wan, J. C., and Zhang, Y. J. (2017). Development and prospects of ‘grain-forage supply’ in modern animal husbandry. Pratacultural Sci. 34 (3), 653–660. doi:10.11829/j.issn.1001-0629.2016-0361
Zhen, H., Cheng, H., and Chun, Y. (2020). Spatial econometric analysis of environmental total factor productivity of ranimal husbandry and its influencing factors in China during 2001–2017. Sci. Total Environ. 723, 137726. doi:10.1016/j.scitotenv.2020.137726
Zhou, L., Dang, X., Mu, H., Wang, B., and Wang, S. (2021). Cities are going uphill: Slope gradient analysis of urban expansion and its driving factors in China. Sci. Total Environ. 775, 145836. doi:10.1016/j.scitotenv.2021.145836
Zhu, L., Gan, Q., Liu, Y., and Yan, Z. (2017). The impact of foreign direct investment on SO2 emissions in the beijing-tianjin-hebei region: A spatial econometric analysis. J. Clean. Prod. 166, 189–196. doi:10.1016/j.jclepro.2017.08.032
Keywords: animal husbandry, GDP growth, spatio-temporal differentiation, spatial econometric model, North China
Citation: Wang C, Zhang J and Zhou W (2023) The effect of animal husbandry on economic growth: Evidence from 13 provinces of North China. Front. Environ. Sci. 10:1085219. doi: 10.3389/fenvs.2022.1085219
Received: 31 October 2022; Accepted: 01 December 2022;
Published: 20 January 2023.
Edited by:
Wenlong Jing, Guangzhou Institute of Geography, ChinaReviewed by:
Xin Lyu, Beijing Normal University, ChinaLaing Zhou, Lanzhou Jiaotong University, China
Copyright © 2023 Wang, Zhang and Zhou. This is an open-access article distributed under the terms of the Creative Commons Attribution License (CC BY). The use, distribution or reproduction in other forums is permitted, provided the original author(s) and the copyright owner(s) are credited and that the original publication in this journal is cited, in accordance with accepted academic practice. No use, distribution or reproduction is permitted which does not comply with these terms.
*Correspondence: Wenneng Zhou, emhvdXduQGdkdXQuZWR1LmNu