- 1School of Public Finance and Taxation, Central University of Finance and Economics, Beijing, China
- 2Institute of Agricultural Resources and Regional Planning, Chinese Academy of Agricultural Sciences, Beijing, China
Straw returning technology has the potential to not only enhance the crop’s nitrogen yield but also protect the ecological environment and enhance crop yield. This paper explores the impact of uncertainty on rural households’ adoption of straw returning technology using an experimental method based on 703 wheat planting households in the Loess Plateau, China. The results show that 1) most farmers are inclined to risk aversion, and farmers generally have the characteristics of ambiguity aversion. 2) Risk preference and ambiguity preference obviously and negatively impact the possibility of adopting straw returning technology, and when the farmer’s risk preference and ambiguity preference increase by 0.1 units, the probability of adopting straw returning technology will decrease by 19.4% and 17.1%, respectively. 3) When we take the risk preference and ambiguity preference together into account, risk preference has sufficiently large effects on farmers’ decision on adopting straw returning technology relative to ambiguity preference. Overall, this research provides a micro-foundation and policy recommendations for farmers’ straw returning technology promotion in rural China and sheds light upon how the government can formulate relevant policies to promote green environmental development.
1 Introduction
Crop straw has served as a basic but important energy resource for living in rural areas worldwide for a long time, especially in developing countries (Gupta, 2014; Zeng et al., 2019; Wang et al., 2022). With the improvement of rural infrastructure and living environment, as well as the widespread use of natural gas in the recent decade, the importance of crop straw as the main fuel in rural areas gradually declined (Liu et al., 2019; Lopes et al., 2020). Instead, open burning in harvest seasons is the most common disposal practice for crop straw in rural China, which not only results in the waste of resources but also causes serious environmental pollution (Wang et al., 2021; Elsayed et al., 2022). In order to effectively alleviate the direct burning of crop straw, the Chinese government has proposed a series of encouraging policies and countermeasures, the most prominent of which is the returning of straw to the fields (He et al., 2018). As a friendly nitrogen fertilizer, an increasing number of researchers pointed out that straw returning could be beneficial to enhance the crop’s nitrogen yield as well as help protect the ecological environment, which improves soil fertility and enhances crop yield, hence deserving to be promoted in rural areas (Qiu et al., 2020).
The new agricultural technology adoption is essential to enhance agricultural productivity and alleviate rural poverty (Barham et al., 2014; Hunecke et al., 2017) but is hindered by low adoption rates for yield-enhancing technologies (Evenson and Gollin, 2003; Wu et al., 2021). As the final users of technology adoption, farmers’ attitude toward crop straw returning is one of the key factors driving technology extension. Extensive literature has attempted to answer the question pertaining to the kinds of determinants of and constraints to agricultural technology adoption, as well as the effectiveness of policies to facilitate new technology. It has been established that education (Foster and Rosenzweig, 2010; Wu et al., 2021), credit constraints (Foster and Rosenzweig, 2010; Mao et al., 2021), and learning spillover (Conley and Udry, 2010; Genius et al., 2014; BenYishay and Mobarak, 2019; Takahashi et al., 2019) are among the main factors of technology adoption. Although such studies consider human and social capital, they address the individual level only, ignoring that new technology adoption always complies with risk, and farmers’ risk preference significantly impacts technology adoption (Liu, 2013; Barham et al., 2014; Ali et al., 2021).
However, most of the previous theoretical and empirical studies were carried out under deterministic conditions (Gollier, 2001; Foster and Rosenzweig, 2010). As small-scale farmers in developing countries frequently make decisions in a situation of uncertainty affected by factors such as increased extreme weather, crop failure, and cost and benefit, farmers’ decisions often need to be made under uncertain conditions (Barham et al., 2014; Bryan, 2019). It is obvious that individual uncertainty preference plays a crucial role in the cognition and diffusion of agricultural technologies (Barham et al., 2014; Qiu et al., 2020), which makes the relation between uncertainty and technology adoption an unsettled question (Ali, et al., 2021). Klibanoff et al. (2005) stated that uncertainty may stem not only from risk but also from ambiguity; from then, researchers have begun to admit that individuals’ attitudes toward uncertain events can be divided into risk attitudes and ambiguity attitudes according to whether the benefits and probabilities of uncertain events are clear (Ross et al., 2012; Ali et al., 2021).
Risk preference is recognized as the preference that the probability distribution of a set of outcomes has been known, and ambiguity preference is another preference which is unsure about the probabilities of outcomes (Klibanoff et al., 2005; Warnick et al., 2011). In addition to risk preference (Pratt, 1964), Ali et al. (2021) also found that ambiguity preference appears to be a common feature of economic behavior. For instance, Barham et al. (2014) pointed out that concerning the adoption of genetically modified soy, the individual’s ambiguity aversion shows a greater impact than the individual’s risk aversion, which suggests the necessity of distinguishing risk and ambiguity when examining the influences of uncertainty on the adoption of agricultural technology. Jin et al. (2019) and Qiu et al. (2020) also found that farmers have a characteristic of “ambiguity aversion,” and risk and ambiguity have a different impact on farmers’ technology learning and adoption. More analyses assume that new technology involves more uncertainty than traditional technologies (Feder et al., 1985); thus, we can believe that ambiguity preference plays a valuable and prominent role in farmers’ adoption decisions as well (Bryan, 2019).
Therefore, this paper aims to explore the impact of uncertainty on rural households’ adoption of straw returning technology based on 703 households in Shaanxi and Shanxi provinces, China. In order to access farmers’ uncertainty preference, we introduce a field experiment to measure it. Compared with existing studies, this paper makes three-fold contributions. First, concerning the adoption of straw returning technology in rural areas, most of literature paid attention to its investing cost and revenue, natural environmental feasibility, and traditional driving forces, ignoring the technological adopters and their risk preference and ambiguity preference, which may significantly impact technological extension. The objective of this paper is to improve our understanding of how farmers’ behavioral factors, such as uncertainty, contribute to rural households’ decisions on the adoption of straw returning technology.
Second, given that the extension of the new technology will be risky and that the benefits of new technology are uncertain, this results in the adoption of the new technology, which is the typical risky decision under the condition of uncertainty, known to contain both prior probability of risky decision-making and a mixture of unknown ambiguity probabilistic decisions. Ambiguity aversion tends to reduce the probability of technology adoption, while farmers tend to maintain the status quo. Therefore, this paper further divides uncertainty into risk preference and ambiguity preference, examining the impact of risk preference and ambiguity preference on rural households’ adoption of straw returning technology.
Moreover, when measuring farmers’ risk preference and ambiguity preference, the subjectivity of the questionnaire survey is overcome, and an experimental method is adopted to obtain data. In this method, farmers in real life are taken as the subjects, and the risk, ambiguity measurement, and experimental information are all obtained from real situations, which overcomes the problem of lack of external validity of the questionnaire survey, and the experimental results can more accurately reflect farmers’ uncertainty preference.
The rest of the paper is organized as follows. The theoretical analysis framework is explained in Section 2. Section 3 introduces the experimental design, including risk preference and ambiguity preference. Section 4 provides the data and methodology, and then the empirical results are given in Section 5. Section 6 discusses the conclusions and policy implications of this paper.
2 Theoretical framework
Next, we propose a model that divides the farmer’s uncertainty preference into two parts: risk preference and ambiguity preference, and as discussed in Section 1, if the decision-maker knows the probability distribution of random payoff, which implies risk occurs, and ambiguity happens when the decision-maker is uncertain about the probability distribution. The following model provides valuable insights into how risk preference and ambiguity preference impact farmers’ straw returning technology adoption.
The uncertainty of a farmer is expressed by a random vector
On the other hand, if the true probability distribution of payoff
where
Equation 2 illustrates that concerning a given
To identify ambiguity from
Equation 3 illustrates that the farmer’s willingness to pay for eliminating ambiguity is expressed by
Comparing Eqs 1, 2, the final and optional choice is to determine the choice of
Qiu et al. (2020) illustrated that straw returning technology indeed belongs to one of the conservation tillage technologies, which is helpful in reducing the exposure of risk and ambiguity for farmers. Thus, we propose the following hypotheses:
H1: Risk preference of farmers will be reluctant to adopt straw returning technology.
H2: Ambiguity preference of farmers will be reluctant to adopt straw returning technology.
Moreover, the aforementioned analysis of uncertainty indicates how both risk preference and ambiguity preference can impact farmers’ technology adoption decision. Whether risk preference or ambiguity preference is more important, there is currently no general conclusion. We explore this matter in the context of straw returning technology adoption. We consider the case where the adoption decision
Equation 4 shows that the adoption of straw returning technology is better if its expected payoff of technology adoption
Furthermore, applying these discussions to household adoption of straw returning technology is quite practical. Indeed, technology adoption’s uncertainties substantially exist in the whole process due to unanticipated weather shocks and unpredictable damages caused by various factors. While some previous research directly treated uncertainty impacting farmers’ technology adoption as risk, part of this could actually be ambiguity. Given that straw returning technology is likely to expose farmers to different levels of risk and ambiguity, therefore, in this paper, Eq. 4 provides useful insights for us. It illustrates that the cost of risk reduces straw returning technology adoption incentives if
According to the research studies of Qiu et al. (2020) and Ali et al. (2021), we also agree that farmers’ ambiguity preference is dependent on risk preference, and risk preference plays a more important role in influencing the adoption of straw returning technology. Thus, our third hypothesis is proposed as follows.
H3: Risk preference plays a more dominant role in impacting the farmer’s straw returning technology adoption than ambiguity preference.
3 Experimental design of uncertainty
3.1 The experiment of risk preference and ambiguity preference
Risk preference and ambiguity preference play important roles in individual behaviors and decisions, such as production decisions, household investments, and new technology adoption. Many methods have been developed to measure individual risk and ambiguity preferences (Cardenas and Carpenter, 2008; Charness et al., 2013). However, various measures rely on simple survey questions about willingness to take risks in general or specific areas, or on hypothetical lotteries, gambling, and investing, to elicit subjects’ preference for uncertainty (Liu and Huang, 2013). Other measures based on complex experimental designs with real monetary incentives are tested in the laboratory with educated students (Holt and Laury, 2002; Deck et al., 2008; Crosetto and Filippin, 2013). Due to lack of scientific uncertainty measurement methods and real experimental scenarios, the results of uncertainty measurement are not effective and extendable.
In order to obtain more real micro-data of farmers’ risk preference, this paper measures farmers’ risk preference through experimental economics. In this paper, Holt and Laury’s (2002) experimental scheme is appropriately simplified to ensure that respondents can understand it and effectively participate in the experiment. All respondents of the risk experiment of this study will receive real money, which can encourage respondents to complete the risk preference experiment truthfully so as to reduce the measurement error of risk preference. The whole experiment is carried out in four stages.
Stage 1: Test procedure. The tester introduces the rules of the test game to the farmers and lets them understand the reward results and risk options. The focus of this stage is to let the respondents understand that the draw is random and the amount of reward depends on the respondents’ choice. In order to test whether the respondents are familiar with the rules of the game, the test game is designed as shown in Table 1, and the respondents are required to choose reward plan B before they can continue the game. Otherwise, the tester needs to explain the rules of the game to the respondents again. This setting helps ensure that the respondents conduct risk measurement experiments on the basis of understanding the rules of the game. This improves the accuracy of the measurement and provides a basis for screening invalid samples in the process of empirical analysis.
Stage 2: Formal test. After the farmers are familiar with the experimental rules, the tester provides 10 sets of test games, and each test includes two reward schemes of low risk and high risk. The respondents make risk choices for all 10 sets. Respondents choose either plan A (low risk) or plan B (high risk) from each of the 10 tests. The focus of the second stage is to let the respondents understand that the risk options they choose are directly related to the final premium. This ensures that the risk preference information displayed is authentic and credible.
We first measure the farmers’ risk preference. In this condition, the farmers are explicitly told that there are three red cards and three black cards, and 10 sets of formal tests are set up (see Table 2).
We then measure the farmers’ ambiguity preference. Here, participants are told there are six red and black cards in total, but they only know that there are more cards of one color than of the other. In this case, they repeat the 10 sets of tests as shown in Table 2.
Stage 3: Draw lots for rewards. One set is randomly selected from 20 sets, and the game is implemented and rewarded according to the farmers’ choices. Among them, reward plan A is the “stable reward plan,” that is, farmers will obtain a stable reward of 20 yuan if they choose reward plan A in each set of games.
Stage 4: Validate test. In order to reconfirm that the farmers completed the aforementioned tests with a correct understanding of the rules of the game, a confirmation test is set up (see Table 3). If the farmers choose reward plan A, it proves that the respondent has correctly understood the rules and the aforementioned test is valid.
3.2 The measurement risk preference and ambiguity preference
According to the farmer’s actual selection, the risk preference is calculated as follows: the risk preference is defined as the number of reward plan B selected in Table 2 under the exact probability divided by 10, and the ambiguity preference is defined as the number of reward plan B selected in Table 2 under ambiguity probability divided by 10. Therefore, the risk preference and ambiguity preference range from 0 to 1. When the risk preference and ambiguity preference equal 0, it indicates that the farmer is extremely risk-averse and ambiguity-averse; if the risk preference and ambiguity preference equal 1, it implies that the farmer is extremely risk and ambiguity loving.
3.3 Description of risk preference and ambiguity preference
Regardless of the risk and ambiguity, most frequencies of farmers’ risk and ambiguity are concentrated at 0, indicating that these farmers are extremely risk-averse. In order to facilitate the farmers’ understanding of the experiment, the experimental scheme is appropriately simplified in this paper. Basically, farmers can steadily obtain 20 yuan, and this setting has incentive compatibility for each farmer. In other words, farmers participating in the experiment have a 100% possibility of obtaining different levels of rewards, which further stimulates them to choose the stable reward program. This is similar to the findings of Liu (2013) and Tanaka et al. (2010), that is, a high proportion of farmers continue to choose schemes that can obtain stable rewards. As shown in Figure 1, the number of farmers with a risk preference lower than 0.5 is significantly higher than that with a risk preference index higher than 0.5, indicating that most farmers have a low degree of risk preference either in the case of ambiguity preference, that is, most farmers are risk-averse. The average risk preference for farmers is 0.35 and 0.27 for ambiguity preference. This shows that in the face of high uncertainty, farmers show stronger risk aversion. This is consistent with the “ambiguity aversion” proposed by Ellsberg (1961) and Qu and Cui. (2018). That is, in the case of uncertain probability, people tend to be averse to ambiguous things.
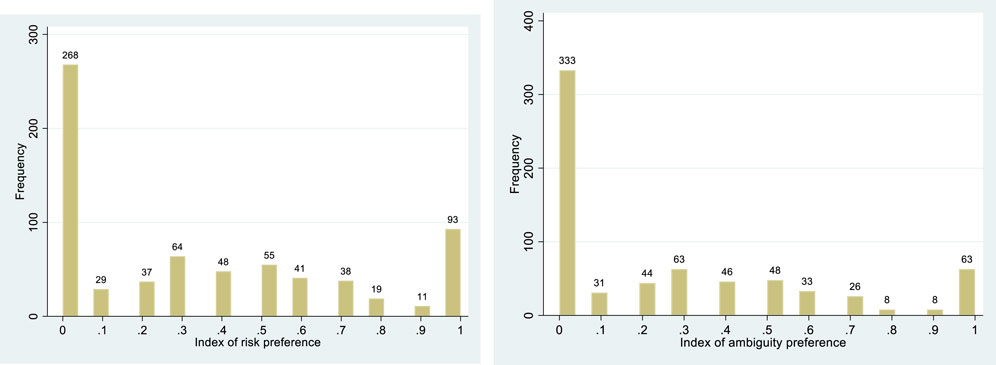
FIGURE 1. Frequencies of farmers’ risk preference and ambiguity preference. Data source: collation of survey data.
4 Data and methodology
4.1 Data specification
Climate conditions and agricultural production conditions vary significantly in different regions of the Loess Plateau. The cropping systems for grain crops here mainly include one cropping of spring corn a year, one cropping of winter wheat a year, two croppings of winter wheat–summer corn a year, and three croppings of winter wheat–summer corn (coarse grain)–spring corn every 2 years. The data used in this paper are obtained from the questionnaire survey conducted by the research group members in Yongshou and Heyang counties of Shaanxi Province and Yaodu and Pinglu counties of Shanxi Province in July 2021. Yongshou County is the main producer of spring wheat, with one cropping a year in Shaanxi Province; Heyang County and Yaodu District focus on promoting the main planting areas of winter wheat, with one cropping a year, and winter wheat–summer corn, with two croppings a year, and Pinglu County is an important wheat production base with three croppings in 2 years in Shanxi Province.
On the other hand, the Loess Plateau region is an important dryland agricultural production area in China. Since 2002, China has focused on promoting conservation tillage technology in the dryland area, among which the straw returning technology is the most important content. In the selected research area, Yaodu is the experimental and demonstration area for the introduction of conservation tillage technology under the Sino–Australian cooperation project of the Ministry of Agriculture in 1992. At present, the coverage rate of wheat straw returning has reached more than 90%. Yongshou, Heyang, and Pinglu counties have successively promoted the wheat straw returning technology since 2006. In 2010, Heyang County was listed as the demonstration county of straw returning in Shaanxi Province. Therefore, the aforementioned areas represent the popularization of straw returning technology well.
For the selection of sample farmers, this paper first selects five townships (towns) in each county (district) on the basis of the representativeness of the grain crop planting system and the advantages of grain crop production. Second, five villages are randomly selected in each township (town). Finally, 7–8 farmers’ households are randomly selected in each village. The research group collects a total of 744 questionnaires, of which 703 are valid, with an efficacy rate of 94.49%. The contents of the questionnaire mainly include the basic characteristics of farmers’ households, cultivated land characteristics, the cultivated land planting status, financial assets and liabilities, risks, and technology adoption experiments. The survey site selection and specific distribution of sample farmers are given in Figure 2 and Table 4, respectively.
4.2 Variable selection
4.2.1 The dependent variable
The dependent variable is the adoption of straw returning technology. If farmers chose to return the straw to the field after harvesting wheat, then the value of the technology adoption is 1, otherwise, the value will be 0, indicating that farmers did not adopt straw returning technology last year. As shown in Table 5, we can see that in 703 sampling farmers, approximately 85.6% samples adopted straw returning last year, indicating most of farmers.
4.2.2 Control variables
According to existing relevant literature (Gao and Niu, 2019; Adams et al., 2021), this paper selects the other important factors that affect farmers’ adoption of new technology as control variables, including individual characteristics (the household head’s age, gender, level of education, and village leaders or not), production and business operation characteristics (annual household income, household size, degree of land fragmentation, and the ratio of wheat planting area accounting for household lands), technology cognition (enhancing wheat production and environment protection), government support (punishment due to burning straw and subsidy), and village locations (distance to the nearest county center and province).
In particular, it should be noted that government support is a strong determinant of farmers’ technology adoption and one of the main channels for farmers to obtain agricultural technology information. The government attaches significant importance to the promotion of straw returning technology in the Loess Plateau region, among which the most important promotion approaches are providing subsidies to farmers who adopt straw returning technology and punishing those who burn the straw in the field, which may become an important factor influencing whether farmers adopt straw returning technology or not.
In terms of village location, it includes the distance to the nearest county center and province. The closer the distance to the county center, the more opportunities for rural households to work in the city, and the lower the proportion of agricultural production in household income. We suppose that the closer the distance to the city, the lower the possibility of adopting straw returning technology. Meanwhile, there are differences between provinces in agricultural technology extension and farmers’ own characteristics, so this study takes provincial variables into consideration.
4.2.3 Definition and descriptive statistics of variables
Table 5 shows the definition and descriptive statistical results of each variable. Among the 703 households, about 85.6% households adopted straw returning technology last year in their wheat lands, which indicates that this kind of technology has been recognized by farmers to some extent.
About 67.9% of the respondents were male, aged about 58 years, with an average of 7.30 years of education. Only 13.2% of the respondents’ family members had village cadres. The annual household income of the interviewees is about 46,800 yuan, and the family size is about five people. Most sampling households recognize that straw returning technology is beneficial to environmental protection and household earnings. Judging from the technological support provided by the government, more than half of the sampling households ever punished or heard that someone was been punished due to burning wheat straw, and 69.4% sampling households ever received government subsidy. The distance from the village to the nearest county center is about 16.12 KMs, and the samples are evenly distributed in both Shaanxi and Shanxi provinces.
4.3 Model specification
In order to investigate the influence of uncertainty on the adoption of straw returning technology, we set Y as the adoption behavior of the technology by households. If the household adopted straw returning technology last year, then we assigned the value of 1; otherwise, we assigned the value of 0. Therefore, the general form of this model can be expressed as
In order to further test which preference has a more significant impact on farmers’ straw returning technology adoption when risk preference and ambiguity preference have simultaneous effects, we construct the following moderating effect equation:
where
5 Results
5.1 Baseline results
The effect of uncertainty preference on household straw returning technology adoption is analyzed by probit regression, and the results are given in Table 6. The results show that from model 1 and model 3, the probit regressions of risk preference and ambiguity preference on household straw returning technology adoption, we can see that in the 1% significant level, the coefficients of risk preference and ambiguity preference are −1.196 and −0.992, respectively, indicating that risk preference and ambiguity preference significantly and negatively impact household straw returning technology adoption. Then, from models 2 and 4, the marginal effect regression of risk preference and ambiguity preference, we conclude that when the farmer’s risk preference increases by 0.1 units, the probability of adopting straw returning technology will decrease by 19.4%; when the households’ ambiguity preference increases by 0.1 units, the probability of adopting straw returning technology will decrease by 17.1%. It can be seen that the higher the degree of uncertainty aversion of farmers, the more inclined they will be to adopt straw returning technology. These conclusions are consistent with those obtained by Barham et al. (2014). Therefore, the hypotheses 1 and 2 are proven here.
Straw returning technology is an environmentally friendly technology, which requires less technological operation specifications for farmers, and has a significant effect of reducing fertilizer application and improving soil fertility so that farmers with higher risk aversion are more likely to accept this technology. Moreover, this technology has been popularized in rural areas for a relatively long time in China, so farmers have some understanding and information about this technology, which results in households’ possession of optimistic expectations about the prospects of benefits brought by this technology.
In addition to uncertainty preference, some factors concerning individual and household characteristics also obviously impact household straw returning technology. With the increase in the household head’s education level, the probability of straw returning technology adoption will be enhanced. The reason may be that more educated household heads possess a certain knowledge reserve, so it is easier to understand the mechanism of straw returning technology and to solve the problems arising from the adoption of this technology, so the probability of adopting this technology is higher, and this conclusion coincides with the research studies of Bollinger (2015) and Gai et al. (2020). The adoption of new technology is often accompanied by large input; thus, household size is an important factor restricting the adoption of technology. In this paper, we can see that household income positively impacts the probability of adopting straw returning technology, and this result is consistent with those of Zilberman (2002) and Wu et al. (2021).
Government promotion is a strong determinant of households’ technology adoption (Goyal and Netessine, 2007), and it is also one of the main channels for households to obtain technology information. Governments usually take measures such as subsidies for technology adoption (Chaves and Riley, 2001), training (Wang et al., 2009), setting up demonstration areas or households (Goyal and Netessine, 2007), and punishment (Wang et al., 2022) to intervene in the adoption of new technologies by households. Government subsidies significantly increased the probability of straw returning technology adoption in this study. The reason may be that government subsidy is a kind of transfer payment, which can not only reduce the cost of adopting straw returning technology adoption but also produce the spiritual incentive effect, which helps promote the adoption of technology, and this conclusion is in line with the research studies of Chaves and Riley (2001), Goyal and Netessine (2007), and Cai et al. (2019).
As rational economic people, when deciding whether to adopt the straw returning technology, farmers are bound to measure the perceived environmental value and yield enhancement of the technology. In the absence of external interference, households’ technology selection behavior must be in line with the judgment criteria of their perceived value. It can be seen that when households recognize the value of ecological environment protection and production enhancement, the adoption of this technology will be positively promoted. This conclusion is in line with that obtained by Boyer et al. (2002), Petrick (2002), and Gai et al. (2020).
5.2 Robustness check
Referring to the research studies of Gao and Niu (2019) and Qiu et al. (2020), according to the means of risk preference and ambiguity preference, this paper first defines the farmers with risk preference and ambiguity preference coefficients in the interval (0, 0.5) and (0, 0.4) as the risk-averse and ambiguity-averse farmers, respectively, and assign the value of 0. On the other hand, the risk preference and ambiguity preference coefficients in the interval [0.5, 1] and [0.4, 1] farmers are defined as the risk-loving and ambiguity-loving ones, respectively, and assign the value of 1. On this basis, we then check the robustness of the impact of farmers’ risk preference and ambiguity preference on straw returning technology adoption. As shown in columns 1) and 3) of Table 7, we can see that the coefficients of risk preference and ambiguity preference are −0.6850 and −0.5950, respectively, which imply that farmers with risk preference and ambiguity preference are reluctant to adopt the straw returning technology, and the baseline regression results are robust.
Furthermore, we change the regression model to the logit model from the probit model, and the results are presented in columns 2) and 4) of Table 7. It is obvious that the coefficients of risk preference and ambiguity preference are −2.2040 and −1.7680, respectively, indicating that farmers with higher risk and ambiguity aversion are more inclined to adopt straw returning technology. The robustness of the baseline regression results was further confirmed again. Meanwhile, we can see that the significance and robustness of other control variables have almost no difference from the baseline regression.
5.3 The interaction of risk preference and ambiguity preference
The results of the estimates of risk and ambiguity preferences on straw returning technology decisions are shown in Table 8. Column 1 illustrates the probit regression of the interaction regression results. We can see that the coefficient of risk preference is −0.6730, implying that farmers with risk aversion are more inclined to take the straw returning technology. Also, the marginal effect presents that when farmers’ risk preference increases by 0.1 units, the probability of the adoption of straw returning technology will decrease by 10.90%. However, when we consider the interaction of risk preference and ambiguity preference, the sign of ambiguity preference changes to positive but is not statistically significant, suggesting there is no effect of ambiguity preference on straw returning technology in the significance of 10%.
Meanwhile, the coefficient of interaction of risk preference and ambiguity preference is −1.3390 and significant in the 5% statistical level, and this may indicate that risk preference has sufficiently large effects on farmers’ decision on adopting straw returning technology relative to ambiguity preference. Thus, hypothesis 3 is proven. This conclusion coincides with that obtained by Ali et al. (2021) and contrary to that obtained by Barham et al. (2014), who found risk preference to have lower effects than ambiguity preference on the adoption of new technology.
6 Conclusion and policy implications
This paper aims to improve our understanding of how behavioral factors like uncertainty influence decisions on household straw returning technology. Given the imprecision of the questionnaire survey, we conducted field experiments in the wheat region of Loess Plateau, China, to investigate households’ uncertainty preferences, which is divided into risk preference and ambiguity preference. We then employed discrete probit models to analyze how individual, household, geographic, and technology cognition tend to impact the adoption of straw returning technology.
The conclusions are as follows. First, from the results of the field experiments, we found that subjects are highly risk-averse and ambiguity-averse. Second, the empirical results imply that risk preference and ambiguity preference significantly and negatively impact household straw returning technology, demonstrating that the higher the risk preference and ambiguity preference, the less reluctant farmers will be to adopt straw returning technology. Meanwhile, when we consider the interaction of risk preference and ambiguity preference together, risk preference has sufficiently large effects on farmers’ decision on adopting straw returning technology relative to ambiguity preference.
Accordingly, this paper puts forward the following policy implications. Considering that many farmers are risk-averse and ambiguity-averse, it is beneficial to facilitate the straw returning technology based on famers’ risk aversion and ambiguity aversion. First, the government could take advantage of farmers’ risk aversion to effectively guide farmers to use straw returning technology, given that straw returning technology is an important measurement to promote agricultural sustainable development. As the main users of straw returning technology, relevant departments should emphasize the function of straw returning technology to reduce the risk of natural disasters in the process of straw returning technology promotion so as to improve the technology adoption rate of farmers.
Second, the ambiguity of straw returning technology should be reduced in advance. Based on the characteristics of the “ambiguity aversion” of farmers, policymakers are required to reduce farmers’ ambiguity of straw returning technology through prior measures, enhance farmers’ understanding and trust of straw returning technology, and promote farmers’ adoption of straw returning technology. Specifically, the problem can be solved through technological training, technological demonstration, technological assistance, and other services.
Third, the government could strengthen the publicity of the risk of natural disasters in agricultural production so that farmers can have a clear and comprehensive understanding concerning the risk of natural disasters in the process of agricultural operation. Therefore, farmers could enhance their awareness to use straw returning technology to reduce the risk so as to increase the demand for straw returning technology adoption by farmers.
Moreover, we should pay attention to the impact of differences on the education level and technological recognition on farmers’ adoption of straw returning technology. Thus, we will strengthen the publicity on the risks of natural disasters in agricultural production and environmental protection and raise farmers’ awareness of risk resistance, ecological protection, and earning enhancement values of straw returning technology.
Finally, the study still has some limitations concerning data collection. The design chosen for the measurement of households’ uncertainty preference imposes some limitations that are worth noting. For instance, we did not give enough consideration of experimental design in money variation, due to constraints of research funding, which proved to be important to change household uncertainty preference (Barham et al., 2014).
Data availability statement
The original contributions presented in the study are included in the article/Supplementary Material; further inquiries can be directed to the corresponding authors.
Author contributions
YG: conceptualization, methodology, and writing—original draft preparation. HW: data curation, software, and writing—reviewing and editing.
Funding
This research was funded by National Natural Science Foundation of China Youth Fund Project “Effects of Incentive Heterogeneity on Agricultural Technology Extension: A Randomized Controlled Experiment in Northern Wheat of China”, grant number “72003215”; National Natural Science Foundation of China General Program “Economic Incentive, Peer Effects and Green Fertilization Behavior of Wheat Growers: A Randomized Controlled Trail”, grant number “71973087”; The 72nd general program of China Postdoctoral Science Foundation “Digital Agricultural Technology Extension and Farmers’ Green Fertilization Technology Adoption: Mechanism of Action, Welfare Effect and Policy Optimization”, grant number “2022M720170”; Soft Science Project of Science and Technology Department of Shaanxi Province “The impact of Incentive Heterogeneity on the Extension of Green Agricultural Technology: A case study of Wheat Water and Fertilizer Integration Technology in Weihe Plain”, grant number “2022KRM131”; The Special Fund project of Basic Scientific Research Operation funds of Central Universities “The Influence of Peer Effect on Farmers’ Green Fertilization Behavior: Based on the Analysis of the Randomized Controlled Experiment of Winter Wheat in Fen-Wei Plain”, grant number “20SZYB21”.
Conflict of interest
The authors declare that the research was conducted in the absence of any commercial or financial relationships that could be construed as a potential conflict of interest.
Publisher’s note
All claims expressed in this article are solely those of the authors and do not necessarily represent those of their affiliated organizations, or those of the publisher, the editors, and the reviewers. Any product that may be evaluated in this article, or claim that may be made by its manufacturer, is not guaranteed or endorsed by the publisher.
References
Adams, A., Jumpah, E. T., and Caesar, L. D. (2021). The nexuses between technology adoption and socioeconomic changes among farmers in Ghana. Technol. Forecast. Soc. Change 173, 121133. doi:10.1016/j.techfore.2021.121133
Ali, W., Abdulai, A., Goetz, R., and Owusu, V. (2021). Risk, ambiguity and willingness to participate in crop insurance programs: Evidence from a field experiment. Aust. J. Agric. Resour. Econ. 65 (3), 679–703. doi:10.1111/1467-8489.12434
Barham, B. L., Chavas, J. P., Fitz, D., Salas, V. R., and Schechter, L. (2014). The roles of risk and ambiguity in technology adoption. J. Econ. Behav. Organ. 97 (1), 204–218. doi:10.1016/j.jebo.2013.06.014
BenYishay, A., and Mobarak, A. M. (2019). Social learning and incentives for experimentation and communication. Rev. Econ. Stud. 86 (3), 976–1009. doi:10.1093/restud/rdy039
Bollinger, B. (2015). Green technology adoption: An empirical study of the southern California garment cleaning industry. Qme-Quantitative Mark. Econ. 13 (4), 319–358. doi:10.1007/s11129-015-9163-0
Boyer, K. K., Hallowell, R., and Roth, A. V. (2002). E-Services: Operating strategy—a case study and a method for analyzing operational benefits. J. Operations Manag. 20 (2), 175–188. doi:10.1016/s0272-6963(01)00093-6
Bryan, G. (2019). Ambiguity aversion decreases the impact of partial insurance: Evidence from african farmers. J. Eur. Econ. Assoc. 17, 1428–1469. doi:10.1093/jeea/jvy056
Cai, R., Wang, Z. Y., and Qian, L. (2019). Does joining a cooperative encourage family farms to choose environmentally friendly methods of production?: Taking reduced application of fertilizers and pesticides as an example. China Rural. Surv. 2019 (1), 51–65.
Cardenas, J. C., and Carpenter, J. (2008). Behavioural development economics: Lessons from field labs in the developing world. J. Dev. Stud. 44, 311–338. doi:10.1080/00220380701848327
Charness, G., Gneezy, U., and Imas, A. (2013). Experimental methods: Eliciting risk preferences. J. Econ. Behav. Organ. 87, 43–51. doi:10.1016/j.jebo.2012.12.023
Chaves, B., and Riley, J. (2001). Determination of factors influencing integrated pest management adoption in coffee berry borer in Colombian farms. Agric. Ecosyst. Environ. 87 (2), 159–177. doi:10.1016/s0167-8809(01)00276-6
Conley, T. G., and Udry, C. R. (2010). Learning about a new technology: Pineapple in Ghana. Am. Econ. Rev. 100 (1), 35–69. doi:10.1257/aer.100.1.35
Crosetto, P., and Filippin, A. (2013). The ‘bomb’ risk elicitation task. J. Risk Uncertain. 47, 31–65. doi:10.1007/s11166-013-9170-z
Deck, C., Lee, J., and Reyes, J. (2008). Risk attitudes in large stake gambles: Evidence from a game show. Appl. Econ. 40, 41–52. doi:10.1080/00036840701235704
Ellsberg, D. (1961). Risk, ambiguity and the savage axioms. Q. J. Econ. 75 (4), 643–669. doi:10.2307/1884324
Elsayed, M., Li, W., Abdalla, N. S., Ai, P., Zhang, Y., and Abomohra, A. E. (2022). Innovative approach for rapeseed straw recycling using black solider fly larvae: Towards enhanced energy recovery. Renew. Energy 188, 211–222. doi:10.1016/j.renene.2022.02.029
Evenson, R. E., and Gollin, D. (2003). Assessing the impact of the green revolution, 1960 to 2000. Science 300 (5620), 758–762. doi:10.1126/science.1078710
Feder, G., Just, R. E., and Zilberman, D. (1985). Adoption of agricultural innovations in developing countries: A survey. Econ. Dev. Cult. Change 33, 255–298. doi:10.1086/451461
Foster, A. D., and Rosenzweig, M. R. (2010). Microeconomics of technology adoption. Annu. Rev. Econ. 2 (1), 395–424. doi:10.1146/annurev.economics.102308.124433
Gai, H., Beath, J., Fang, J., and Lou, H. H. (2020). Alternative emission monitoring technologies and industrial internet of things–based process monitoring technologies for achieving operational excellence. Curr. Opin. Green Sustain. Chem. 23, 31–37. doi:10.1016/j.cogsc.2020.04.009
Gao, Y., and Niu, Z. (2019). Risk aversion, information acquisition ability and farmers’ adoption behavior of green control techniques. Chin. Rural. Econ. 8, 109–127.
Genius, M., Koundouri, P., Nauges, C., and Tzouvelekas, V. (2014). Information transmission in irrigation technology adoption and diffusion: Social learning, extension services, and spatial effects. Am. J. Agric. Econ. 96 (1), 328–344. doi:10.1093/ajae/aat054
Gollier, C. (2001). Wealth inequality and asset pricing. Rev. Econ. Stud. 68 (1), 181–203. doi:10.1111/1467-937X.00165
Goyal, M., and Netessine, S. (2007). Strategic technology choice and capacity investment under demand uncertainty. Manag. Sci. 53 (2), 192–207. doi:10.1287/mnsc.1060.0611
Gupta, R. (2014). Low-hanging fruit in black carbon mitigation: Crop residue burning in south asia. Clim. Change Econ. 5 (4), 1450012–1145001222. doi:10.1142/s2010007814500122
He, K., Zhang, J., and Zeng, Y. (2018). Rural households' willingness to accept compensation for energy utilization of crop straw in China. Energy 165, 562–571. doi:10.1016/j.energy.2018.09.023
Holt, C. A., and Laury, S. K. (2002). Risk aversion and incentive effects. Am. Econ. Rev. 92, 1644–1655. doi:10.1257/000282802762024700
Hunecke, C., Engler, A., Jara-Rojas, R., and Poortvliet, P. M. (2017). Understanding the role of social capital in adoption decisions: An application to irrigation technology. Agric. Syst. 153, 221–231. doi:10.1016/j.agsy.2017.02.002
Jin, Y., Xu, B., and Zhang, W. (2019). A review of risk, ambiguity and decision-making behavior and its application in technology diffusion of agriculture. J. Agrotechnical Econ. (07), 15–27.
Klibanoff, P., Marinacci, M., and Mukerji, S. (2005). A smooth model of decision making under ambiguity. Econometrica 73 (6), 1849–1892. doi:10.1111/j.1468-0262.2005.00640.x
Liu, P., He, J., Li, H., Wang, Q., Lu, C., Zheng, K., et al. (2019). Effect of straw retention on crop yield, soil properties, water use efficiency and greenhouse gas emission in China: A meta-analysis. Int. J. Plant Prod. 13 (4), 347–367. doi:10.1007/s42106-019-00060-w
Liu, E. M., and Huang, J. (2013). Risk preferences and pesticide use by cotton farmers in China. J. Dev. Econ. 103, 202–215. doi:10.1016/j.jdeveco.2012.12.005
Liu, E. M. (2013). Time to change what to sow: Risk preferences and technology adoption decisions of cotton farmers in China. Rev. Econ. Statistics 95 (4), 1386–1403. doi:10.1162/rest_a_00295
Lopes, A. A., Viriyavipart, A., and Tasneem, D. (2020). The role of social influence in crop residue management: Evidence from northern India. Ecol. Econ. 169, 106563. doi:10.1016/j.ecolecon.2019.106563
Mao, H., Zhou, L., Ying, R. Y., and Pan, D. (2021). Time preferences and green agricultural technology adoption: Field evidence from rice farmers in China. Land Use Policy 109, 105627. doi:10.1016/j.landusepol.2021.105627
Neilson, W. S. (2010). A simplified axiomatic approach to ambiguity aversion. J. Risk Uncertain. 41 (2), 113–124. doi:10.1007/s11166-010-9099-4
Petrick, J. F. (2002). Development of a multi-dimensional scale for measuring the perceived value of a service. J. Leis. Res. 34 (2), 119–134. doi:10.1080/00222216.2002.11949965
Pratt, J. W. (1964). Obustness of Some Procedures for the Two-Sample Location Problem. J. Am. Stat. Assoc. 59 (307), 665–680. doi:10.1080/01621459.1964.10480721
Qiu, H., Su, L., Zhang, Y., and Tang, J. (2020). Risk preference, risk perception and farmers’ adoption of conservation tillage. Chin. Rural. Econ. (07), 59–79.
Qu, W., and Cui, J. (2018). The new progress of research on macro uncertainty. Econ. Perspect. (03), 126–138.
Ross, M., Urbina, A., Simmermacher, T., and Paez, T. (2012). “The verification and uncertainty quantification of surrogate models used for structural analysis,” in 53rd AIAA/ASME/ASCE/AHS/ASC Structures, Structural Dynamics and Materials Conference 20th AIAA/ASME/AHS Adaptive Structures Conference 14th AIAA, 1442
Takahashi, K., Mano, Y., and Otsuka, K. (2019). Learning from experts and peer farmers about rice production: Experimental evidence from Cote d’Ivoire. World Dev. 122, 157–169. doi:10.1016/j.worlddev.2019.05.004
Tanaka, T., Camerer, C. F., and Nguyen, Q. (2010). Risk and time preferences: Linking experimental and household survey data from vietnam. Am. Econ. Rev. 100 (1), 557–571. doi:10.1257/aer.100.1.557
Wang, J., Zhang, L., Huang, J., and Scott, R. (2009). The adoption of conservation agricultural technology in the yellow river basin: Empirical research on the influential factors. Resour. Sci. 31 (04), 641–647.
Wang, S., Huang, X., Zhang, Y., Yin, C., and Richel, A. (2021). The effect of corn straw return on corn production in northeast China: An integrated regional evaluation with meta-analysis and system dynamics. Resour. Conservation Recycl. 167, 105402. doi:10.1016/j.resconrec.2021.105402
Wang, Y., Wang, N., and Huang, G. Q. (2022). How do rural households accept straw returning in Northeast China? Resour. Conservation Recycl. 182, 106287. doi:10.1016/j.resconrec.2022.106287
Warnick, J. E., Escobal, J., and Laszlo, S. C. (2011). Ambiguity aversion and portfolio choice in small-scale Peruvian farming. BE J. Econ. Analysis Policy 11 (1). doi:10.2202/1935-1682.2331
Wu, H., Hao, H., Lei, H., Shi, H., Ge, Y., and Song, Y. (2021). Farm size, risk aversion and overuse of fertilizer: The heterogeneity of large-scale and small-scale wheat farmers in northern China. Land 10 (2), 111. doi:10.3390/land10020111
Wu, H., Li, J., and Ge, Y. (2022). Ambiguity preference, social learning and adoption of soil testing and formula fertilization technology. Technol. Forecast. Soc. change 184 (11), 122037. doi:10.1016/j.techfore.2022.122037
Zeng, Y., Zhang, J., and He, K. (2019). Effects of conformity tendencies on households’ willingness to adopt energy utilization of crop straw: Evidence from biogas in rural China. Renew. Energy 138, 573–584. doi:10.1016/j.renene.2019.02.003
Keywords: uncertainty, time preference, straw returning technology, field experiment, risk preference
Citation: Ge Y and Wu H (2023) The impact of uncertainty on farmers’ adoption of straw returning technology in Northwest China. Front. Environ. Sci. 10:1078585. doi: 10.3389/fenvs.2022.1078585
Received: 28 October 2022; Accepted: 12 December 2022;
Published: 10 January 2023.
Edited by:
Dingde Xu, Sichuan Agricultural University, ChinaReviewed by:
Shili Guo, Southwestern University of Finance and Economics, ChinaPeng Jiquan, Jiangxi University of Finance and Economics, China
Copyright © 2023 Ge and Wu. This is an open-access article distributed under the terms of the Creative Commons Attribution License (CC BY). The use, distribution or reproduction in other forums is permitted, provided the original author(s) and the copyright owner(s) are credited and that the original publication in this journal is cited, in accordance with accepted academic practice. No use, distribution or reproduction is permitted which does not comply with these terms.
*Correspondence: Haixia Wu, aHhpYTAwN0AxMjYuY29t