- 1Queen’s Management School, Queen’s University Belfast, Belfast, United Kingdom
- 2School of Business, Gachon University, Seongnam, South Korea
- 3Program of Environmental Science, Beijing Normal University-Hong Kong Baptist University United International College, Zhuhai, China
- 4School of Big Data and Statistics, Anhui University, Hefei, Anhui, China
- 5School of Public Health, Xiamen University, Xiamen, China
The construction of China’s Free Trade Zone (FTZ) is an important strategy for China’s thorough deepening of opening up and achievement of long-term high-quality development. Based on the panel data of 283 prefecture-level and above cities in China from 2008–2019, this paper adopts the methods of Spatial Differences-in-Differences (SDID), Spatial Differences-in-Differences-in-Differences (SDDD), and spatial intermediary effect test to empirically examine the impact and the spatial spillover of China’s free trade zone on the environmental pollution of the pilot areas and its influencing mechanism. According to the findings of the study, the establishment of pilot free trade zones may suppress urban PM2.5 emissions by around 2.9 percent, and FTZs can also greatly enhance the air quality of neighboring cities. Further examination of the influencing mechanism reveals that the establishment of a FTZ inhibits PM2.5 pollution and has a significant positive spillover on PM2.5 reduction in surrounding cities by the following means: attracting more foreign direct investment; improving the industrial structure through increasing the proportion of tertiary industry; prompting the local government to strengthen environmental regulation as part of the FTZ’s supporting policies; increasing the investment in science and technology innovation, developing scientific and technological level to achieve green production. The empirical results of this paper are still robust after a series of robustness tests when the explained variable is replaced by the traditional air pollution indicator industrial nitrogen oxide emissions, another sort of spatial matrix is introduced, the propensity score matching SDID (PSM-SDID) and placebo tests as well as winsorize method are carried out. Furthermore, the inhibitory effect of FTZs on air pollution is modified by changes in city size, geographic location and city type, according to heterogeneity analysis. Finally, this paper proposes feasible policy recommendations.
1 Introduction
China’s economy has grown miraculously during the decades of reform and opening up. Yet, environmental pollution caused by conventional enormous economic expansion in the early years of the opening up has become an incredibly significant issue. The most visible type of environmental contamination is air pollution. The average annual PM2.5 pollution level in Chinese cities across the country was greater than 30 μg/m3, until 2019, there were still more than 100 cities with severe pollution ( >35 μg/m3). Air pollution not only seriously impedes the high-quality economic development, but also has a substantial adverse impact on the physical and mental health of Chinese residents (Ingole et al., 2022). If air pollution is not controlled, PM2.5 pollution might cost China 2.0% of GDP, 210 billion Yuan in health spending, and one trillion Yuan in Value of Statistical Lives (VSL) by 2030. (Xie et al., 2019). When pollution levels reach a particular level, the government will refuse bribes and impose tighter environmental rules (Pommeret et al., 106048). In light of this backdrop, the Chinese government has launched a series of policy initiatives to reduce pollution and improve urban population health (Xu et al., 2022). One of them is the Free Trade Zone policy examined in this research. Although FTZs are established to attract investment to drive regional trade liberalization and economic development, China’s FTZs are not just about expanding openness to the outside world and attracting foreign investment. FTZs attract advanced industries and technologies in large numbers through preferential tariff policies to achieve industrial transformation and upgrading, and foreign high-tech enterprises bring industrial structure optimization to reduce energy use and thus pollution; and FTZs bring market liberalization, and make the mismatch of environmental resource factor allocation continue to be optimized, thereby reducing environmental pollution. So, will China’s FTZ affect China’s environmental quality in the process of promoting trade and investment liberalization to achieve high-quality economic development, and will FTZ reverse the traditional development model and improve China’s environmental quality? Based on these doubts, this paper investigates the impact of FTZ establishment on urban air pollution to provide a reference for decision making to promote China’s green economic development.
China’s Pilot Free Trade Zone refers to a multi-functional special economic zone formed outside the country’s customs, with preferential taxation and special customs supervision policies as the primary method of achieving trade liberalization and facilitation. The benefits of the pilot free trade zone include no customs involvement in the import, production, and re-export of commodities, unfettered entry and exit for foreign ships, and tax-free import of foreign goods. But at the same time, it will also bring about problems such as distortion of commodity flow and tax avoidance. Under the background of stagnant WTO multilateral negotiations, the surging trend of anti-globalization and trade protectionism, the regional trade liberalization led by free trade areas has developed rapidly (Zheng et al., 2014). In China, after the establishment of the China (Shanghai) Pilot Free Trade Zone in 2013, 21 FTZs have been established till 2021. The specific establishment time and locations are given in Table 1.
The role of trade openness policies on the environment has been controversial, Managi et al. (2009) revealed that the opening of trade leads to the increase of CO2 and SO2 emissions in less developed countries, Lin (2017) also concluded that trade openness in China was detrimental to the environment. This is because the open countries adopt loose environmental investment standards to attract more foreign companies and pollution intensive goods, and this theory is so-called the pollution haven hypothesis (Cole, 2004). However, Frankel et al. (2005) (Jeffrey and Rose, 2005); Antweiler et al. (2001) believed that trade openness was good for the environment. As the largest developing country in the world, is FTZ, the new trade openness policy in China, good or harmful for the environment? The answers to the above questions might not only help promote China to form a higher level of opening-up pattern, but also provide decision-making reference for promoting high-quality green development of China’s economy.
2 Literature review and hypothesis development
At present, there are few literature on the construction of China’s free trade zones, and most of the existing literature discuss the impact of FTZs on economic development. Eaton (1985) (Eaton, 1985) believed that the foreign trade zone has significantly promoted the development of regional trade and innovation, Chen et al., (2018) (Chen et al., 2018)also believed that FTZ is of great significance to China’s opening up and sustainable economic development. Liu et al,. (2021) used super SBM and other models to get the results of the promotion of Free Trade Zone on port efficiency. (Li et al., 2021) used the panel data of Chinese port listed companies from 2010 to 2016, and adopted DID to study the impact of free trade zone policies on the performance of port listed companies, then concluded that free trade zones can promote the continuous growth of port listed companies’ development performance. Apart from the economic and development effects of FTZs, Ana and Dana (2006) (Liberato and Fennell, 2007) also used logistic regression to empirically prove the favorable impact of free trade zones on social security. Ji et al., (2015) (Ji et al., 2015) also found the boosting effect of the China Free Trade Zone on Hong Kong’s tourism industry. Cheng et al., (2022) (CHENG et al., 2022) used provincial data from 2009 to 2017 to find that implementing free trade zone policies significantly narrowed China’s income gap. In terms of the environmental effect of the FTZs, Jiang et al., (2021) (Jiang et al., 2021) used the synthetic control method to empirically prove that the establishment of Shanghai Free Trade Zone has an increasing effect on the development of green total factor productivity. Aloise and Macke(2017) (PedroAloise and Macke, 2017) proved the beneficial effect of the establishment of Manaus Free Trade Zone in Brazil on local green and sustainable development. However, Zhuo et al., (2021) (Zhuo et al., 143856) estimated the environmental welfare utility of the Guangdong Free Trade Zone and concluded that the Guangdong Free Trade Zone did not improve the environment. Spacial spillover is the specific property of air pollution, many articles regarding adopt spacial models to conduct the empirical research. Ge et al., (2022) (Ge et al., 2022) used the spatial Durbin model to study the impact of economic growth targets on CO2 emissions. Han et al., (2022) (Han et al., 2022) used panel data from 282 prefecture level cities in China, and adopted spatial Durbin and STIRPAT models to study the impact of industrial agglomeration on nitrogen dioxide emissions. Thus, when it comes to air pollution, it is more proper using spatial models, and consider the spatial spillover effect like most scholars do (Jiang et al., 2022) instead of ordinary panel models.
First of all, the primary role of free trade zones is to attract foreign direct investment, the pilot free trade zone can significantly attract foreign-invested enterprises to enter the free trade zone (Qi et al., 2021), and the effect of foreign direct investment on air pollution reduction has been widely proven (Wang et al., 2022), foreign capital brings advanced technology and resources, promotes industrial transformation and technological upgrading, and thus reduces regional air pollution (Cheng et al., 2020). In addition the free trade area also has a significant knowledge spillover effect, which can drive the rise of regional education and science level (Li et al., 2020). Businessmen from all over the country and the world will be more willing to trade on the free trade zone (Hazari and Sgro, 1996), thus bringing business prosperity and leading the industrial structure tending to be the third industries, which inhibits the air pollution (Zhu et al., 2019). Cleaner production technologies brought about by foreign investment and preferential policies can significantly improve regional air quality (Zhu et al., 119515). At the same time, when implementing the FTZ policy, the government will introduce a series of supporting environmental regulations to better attract foreign investment, and environmental regulations can significantly curb pollution emissions (Cole et al., 2005)and also have spillover effects on air pollution in surrounding cities (Tong et al., 110998). In addition, the free trade zone policy can not only promote the regional innovation of the policy pilot, but also promote the innovation of the surrounding cities, this is because the preferential policies of the free trade zone attract a large number of enterprises to enter, which will intensify the competition among enterprises, and the competitive effect will drive the improvement of regional innovation ability, and drive the innovation improvement of surrounding areas through population flow and inter-city trade (Lin et al., 2021).
Based on the analysis above, we can propose the following assumption:
Hypothesis 1 FTZ can reduce air pollution emissions.
This paper also intends to investigate the mechanism of FTZ on air pollution. In 2015, the Chinese government declared the FTZ policy, coupled with specific measures, and later in 2019, policies addressing the revolution and supporting measures for FTZ were issued. In the government documents, the central government urges the government of the free trade zone to further strengthen environmental protection legislation and law enforcement, restrict the introduction of goods that cause environmental pollution, and strengthen environmental regulation on foreign enterprises (Central Government of the People, 2015). At the same time, the free trade zone will further promote investment liberalization and facilitation, and attract more foreign direct investment by improving the tracking service of foreign investment projects, providing investment protection mechanisms, and supporting the establishment of foreign investment financial institutions in the pilot free trade zone. In addition, for science and education, FTZ promotes the innovation driven development strategy, supports enterprises to jointly build industrial innovation platforms with financial institutions, universities and scientific research institutes, and encourages famous foreign universities to cooperate in running schools in China. FTZ is also committed to optimizing the trade structure, restricting the crude oil use qualification of enterprises in the free trade zone, promoting the use of natural gas, updating the industrial structure, and introducing more foreign enterprises in the primary and tertiary industries (Central Government of the People, 2019).
According to the background above, the following assumption is given.
Hypothesis 2 FTZ can reduce air pollution through industrial upgrading, attracting foreign investment, promoting technological development, and strengthening environmental regulations.
According to Hypothesis 2, the mechanism flow chart are presented in Figure 1.
The specific contributions of this article compared with the existing literature are as follows: 1) This paper studies the spatial spillover impact of the free trade zone on urban air pollution in China, and through spatial models and robustness check, the reduction effect with spatial spillover of FTZ policy on air pollution is found. 2) This article also investigates the influencing paths of free trade zone on air pollution, it is revealed that through industrial upgrading, attracting foreign investment, promoting technological development, and strengthening environmental regulations, FTZ policy can help the city to inhibit air pollution. 3) In addition, this paper also explores the heterogeneity of FTZs’ effects on air pollution, and the conclusion is drawn that the pilot cities of various sizes, types, and locations have different policy effects.
3 Materials and methods
3.1 Variables selection
3.1.1 Explanatory variables
The core explanatory variable of this article is the variable FTZ, which represents the implementation of free trade zone policies. To describe FTZ, we may use two dummy indicators: event dummy variable “Treat” and time dummy variable “Post”. We separated 283 cities into treatment groups and control groups according to whether the cities were pilot cities in the free trade zone. Treatment group includes 24 cities while control group contains 259 cities. The variable “Treat” for pilot city is 1, otherwise it is 0; the time dummy variable “Post” is assigned a value of 1 in the year in which the policy is implemented as well as in the subsequent years, otherwise it was 0. The interaction term Treat × Post is FTZ, which represents whether the city is affected by the FTZ pilot or not in a certain year.
Figure 2 adopts a map to illustrate the spatial distribution of the pilot and non-pilot cities of the FTZ policy.
3.1.2 Explained variables
At present, the main indicators to measure air pollution are particulate matters (PM2.5 and PM10), sulfur dioxide (SO2), carbon monoxide (CO), nitrogen oxides (NOx), ozone (Kampa and Elias, 2008), but the most commonly used for research is PM2.5 (Zeng et al., 105816). Hence, this paper uses PM2.5 concentrations as an indicator of air pollution for benchmark regression, and based on data availability, we further selects NOx with the least missing data in 283 cities as the explained variable for robustness check.
3.1.3 Control variables
The control variables used in this paper are as follows: economic scale (PGDP, Yuan/person) i.e.,GDP per capita, the use of fossil fuels in economic activities, especially in industrial production, will bring more air pollution emissions, so the economic scale is closely related to air pollution (Kukla-Gryz, 2009); government intervention (GI), i.e., the shares of urban finance expenditure in regional GDP (Yu et al., 2022a), the government can intervene in the emission of air pollutants through fiscal expenditure, policy intervention and market regulation, hence GI is also a crucial aspect in air pollution (Wang et al., 143738); population density (PD, 103person/km2) i.e.City population divided by city land area urban population agglomeration leads to industrial agglomeration, the use of public transport and urban clean energy, so population density has a significant impact on air pollution (Chen et al., 139456); Road traffic (RT) i.e., road passenger traffic each day, automobile exhaust emissions from urban traffic are an important source of air pollution, so the flow of road traffic is an vital factor affecting air pollution (Zeng et al., 2021); Internet development (INT) i.e., Number of households connected to the Internet (10 thousand households), take logarithm, digital economy brought by the use of the internet has changed the mode of production, promoted the development of green economy and reduced environmental pollution (Li and Wang, 2022); Science and education (SAE) i.e., Scientific and educational investment from government finance, the development of green technology and low-carbon education are beneficial to reducing air pollution.
3.1.4 Mediating variables
Based on the previous literature review, this paper uses the following four mediating variables to investigate the mechanism of FTZs: industrial structure (IDS): i.e., The ratio of the added value of the secondary industry to the added value of the tertiary industry; Science expenditure (SE) i.e., Government investment in science and technology; foreign direct investment (FDI) i.e., The actual amount of foreign direct investment, take logarithm (Dollars); environmental regulation (ER), i.e., Comprehensive utilization rate of industrial solid waste.
3.1.5 Spatial weight matrix
Spatial weight matrix is the most crucial element of spatial models, this paper utilizes a spatial inverse geographic distance weight matrix to conduct spatial regression. By obtaining the latitude and longitude of 283 prefecture level and above cities covered by the sample, using the software Matlab to compute the distance between cities. Then solving formula (1)、(2), we obtain matrix W, which is a 283 × 283 spatial weight matrix.
Where dij denotes the distance between cities i and j as measured by their latitudes and longitudes.
3.2 Data sources and summary statistics
After removing cities with missing data, this study employed the 2008–2019 balanced panel data of 283 prefecture-level and above cities as the empirical dataset, with missing values imputed using the interpolation approach based on trends. The data in this study mainly come from the China City Statistical Yearbook 2009–2020, PM2.5 data are from NOAA (National Oceanic and Atmospheric Administration) weather station data, we use the inverse distance weighted interpolation method to divide into grid data, and then match to city level data. The descriptive statistics of all variables in this paper are presented in Table 1.
3.3 Econometrics models
Since there are various types of spatial autocorrelation, spatial models are also divided into various types. Referring to Chenyang Yu et al. (2022b), this paper uses three of major spatial econometric models to evaluate the effect of FTZ construction on air pollution and its spillover effects, the models are as follows:
Spacial Durbin Differences-in-Differences Model (SDM-DID):
Spatial Autoregressive Differences-in-Differences Model (SAR-DID), also known as Spatial Lag Differences-in-Differences model (SLM-DID):
Spatial Error Differences-in-Differences Model (SEM-DID):
Where
Our key coefficients of interest in the three main spatial regression models discussed above are
After conducting a spatial Hausman test (Kelley Pace and LeSage, 2008), two-way fixed effects (city effects and year effects) are controlled in the models. Besides, in the benchmark regression we also report the information criterion (Akaike information criterion, AIC and Bayesian information criterion, BIC) and the log-likelihood to explore which model is better, the model is better when the results have a higher absolute value of log-likelihood and a lower value of AIC/BIC (De XiaoLi and Cheng, 2020).
4 Regression results
4.1 Spatial correlation test
Before performing spatial econometric regression, we need to test the spatial correlation of the explained variables, this paper utilizes Global Moran’s I and Geary’s C to conduct the spatial association test, the expression of the two indexes are as follows (L Ping et al., 2004):
Table 3 shows the Moran’s I index and Geary’s C index of urban PM2.5 emissions of the sample from 2008–2019. If the values of these two indices are between 0 and 1, then there is a positive spatial correlation of PM2.5 emissions spills over to surrounding cities. The Moran’s I index is a number that varies from −1 to 1, a larger value of Moran’s I index indicates a stronger positive spatial correlation of PM2.5 pollution. Geary’s C-index ranges from 0–2, the smaller the value of Geary’s C index, the greater the positive spatial correlation.
The results illustrate that the Moran’s I index and Geary’s C index values of PM2.5 are in the range of [0, 1], and the results are all significant. The results implies that there is a significant positive spatial correlation of PM2.5 in Chinese cities. Therefore, when studying the impact of FTZ policies on air pollution, we should consider the spatial characteristics of PM2.5 pollution and adopt spatial models instead of non-spatial models for estimation, otherwise, the results of estimation would be biased. In Figure 3 below, we demonstrate the scatter plots of Moran’s I Index of PM2.5 emissions in China’s cities in chosen years, and the results demonstrated that in the sample time span, most cities are located in the first and third quadrants, indicating that there is a spatial effect of high-high aggregation and low-low aggregation in the air pollution between cities, that is, the phenomenon of pollution clusters exists.
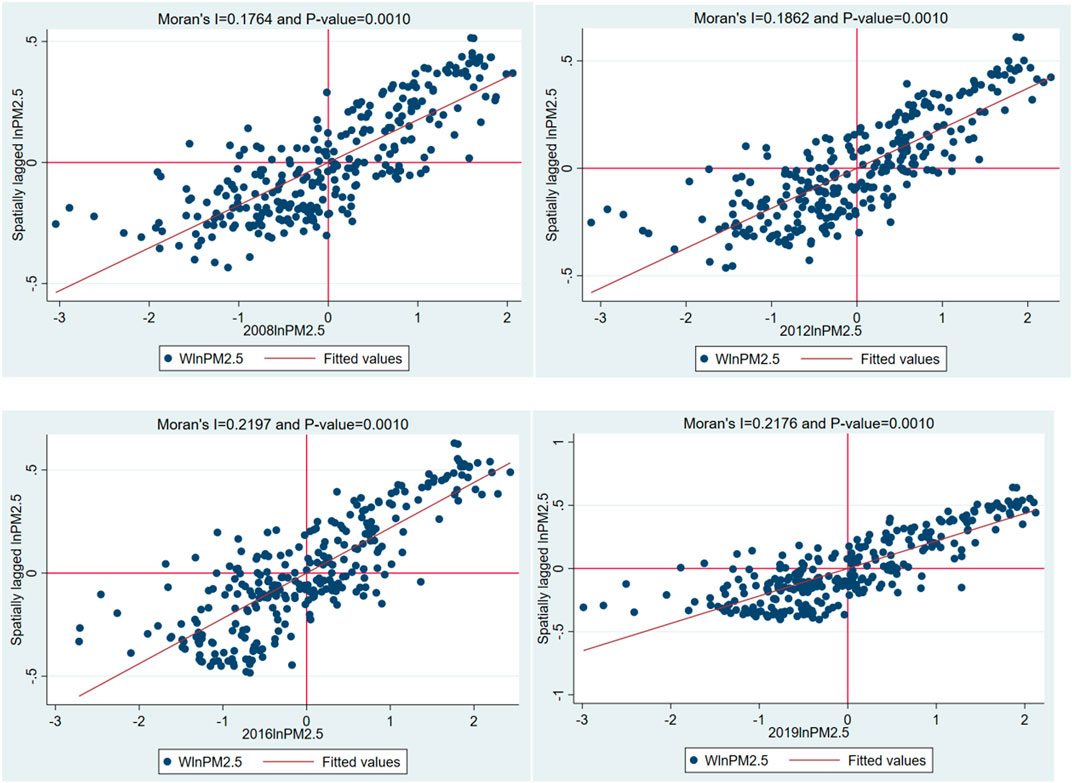
FIGURE 3. Scatter plots of Moran’s I Index of PM2.5 emissions in China’s cities with W in chosen years: 2008, 2012, 2016, 2019.
4.2 Benchmark regression
Table 4 presents the results of the baseline regression, no matter which model is used, the coefficient of FTZ is significantly negative above the 5% significant level, which preliminarily indicates that the establishment of FTZ reduces air pollution emissions. Meanwhile, the spatial autocorrelation or autoregressive coefficients of all models are significantly positive, showing that the policy effect has spatial spillovers to surrounding cities. From the perspective of AIC, BIC and log-likelihood, the optimal model should be SDM-DID, as the AIC and BIC value of SDM-DID model are the smallest and its log-likelihood is largest at the same time. From the results of SDM-DID, after introducing the control variables, the coefficient of FTZ on air pollution reached -.029, and it is significant at the 1% level, indicating that the establishment of FTZ reduced air pollution by 2.9%. The spatial spillover coefficient of WFTZ is -.929 and significant at the 1% level, indicating that the establishment of FTZ also has a significant inhibitory effect on air pollution in surrounding cities.
Since SDM-DID is the best model, Table 5 reports the detailed direct, indirect, and total effects results of SDM-DID model.
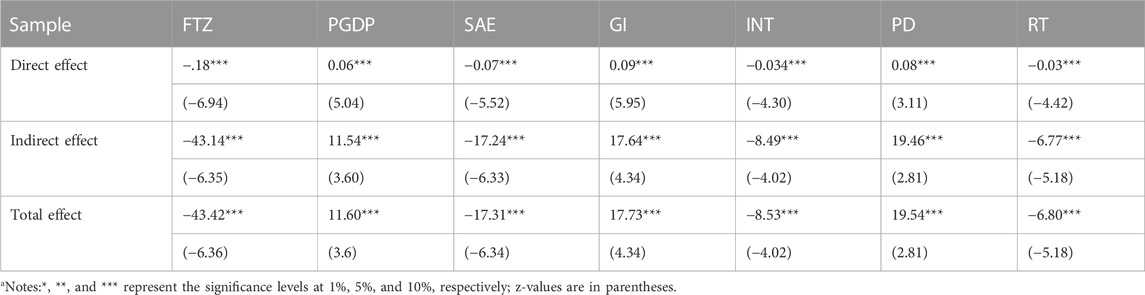
TABLE 5. Direct, indirect, and total effects of SDM-DID in column (2) in Table 4.
The direct effect of FTZ is −.18 and is statistically significant at the 1% level. This shows that setting up a free trade zone can reduce local air pollution by 18%. Therefore, Hypothesis 1 of this paper has been verified, that is, the construction of free trade zones reduces air pollution emissions. In addition, the indirect effect of FTZ reaches -43.14 and is significant at the level of 1%, indicating that the establishment of the free trade zone had a significant effect on the improvement of air pollution in surrounding areas. This conclusion might be due to the fact that air pollution has a spatial effect, air pollution can spread between cities through natural factors such as atmospheric flow and economic channels like industrial transfer, and the improvement of air pollution can also be transmitted to the surrounding areas. Factors of production enter the free trade zone, and the preferential policies of the free trade zone and advanced technologies with spillover effects (Elena and Galeotti, 2011) can drive the greening and efficiency of production, energy saving and hence emission reduction in surrounding cities. The total effect of FTZ on air pollution is -43.42, indicating that each additional free trade zone reduces the overall PM2.5 emission by about 4,342%, that is, the PM2.5 emission of the 283 cities in the sample is reduced by an average of 15.3%. It can also be seen from Table 5 that the direct and indirect effects of PGDP, PD and GI are significantly positive at the 1% level, indicating that economic development, population aggregation and government intervention will all lead to aggravation of environmental pollution, and the impact has Spatial spillover effect, which is consistent with previous studies (Chen et al., 139456; Chen and Chen, 2021). The direct and indirect effects of SAE and INT were significantly negative, indicating that investment in science and education and the popularization of the Internet can significantly reduce air pollution in local and surrounding areas.
4.3 Robustness check
A set of robustness tests are developed in this section to verify that the regression findings obtained beforehand are resilient and trustworthy.
4.3.1 Parallel trend test
The DID model has an important premise, that is, the parallel trend test. The parallel trend assumes that the trends of the treatment group and the control group are consistent before the policy is implemented. In order to prove whether this premise holds, this paper uses the following model to conduct the parallel trend test:
We delete the year before the implementation of the policy as the base period, and the results are plotted with time relative to policy implementation as the x-axis and the regression coefficient of policy dynamic effect as the y-axis. The results are given in Figure 4.
As is illustrated in Figure 4, in the all five years before policy implementation, the coefficient is positive and not significant, while from the current period to 3 years after the implementation of the policy, the coefficients are all significantly negative. Hence, the parallel trend test is passed, and the SDID methods can be applied to evaluate the policy effect.
4.3.2 Replace the explained variable
In this section, NOx, which is another frequently used index describing air pollution, is adopted as the explained variable to conduct the regression. The results are in Table 6 below.
As can be seen from Table 6, no matter which spatial model is used, FTZ policy significantly reduces urban NOx emissions at the 5% significant level.
4.3.3 PSM-SDID
The FTZ policy studied in this paper is a quasi-natural experiment, the SDID approach utilized to assess policy impacts will obviously have self-selection bias. At this time, the PSM-SDID method can be used to match each treatment group sample to a specific control group sample, making the experimental group and the control group cities as similar as possible in all aspects to eliminate selection bias, thereby increasing the credibility of the policy effect estimate (Jia et al., 105271). The balance test and matching results are in Table 7.
As is shown in Table 7, before matching, the control group is significantly different from the treated group, but after PSM, the biases of each matching variable in the control group and the treated group are all within 11%. The t-test results show that both the variables are significantly different before matching since the t values are all .000 before matching, while the t values after matching are all exceeded .1, that is, the hypothesis that the two groups of variables are the same cannot be rejected after matching. Besides, the Pseudo R2 is close to 0 and the p-value is insignificant after matching, so the PSM is successful.
Using the data after matching, we conduct PSM-SDID and the results are shown in Table 8.
As we can see from Table 8, the coefficients of FTZ are still significantly negative in all models, and the absolute value of the coefficient just slightly increase or decrease compared to the results in benchmark regression, hence the PSM-SDID robustness check is passed.
4.3.4 Placebo test
In this part, we designed a placebo test to further confirm the effectiveness of the benchmark regression results. Since FTZ is a 0–1 variable, which is likely to produce accidental effects, we conducted a placebo trial, specifically, we denote each city a random FTZ value (0 or 1) to conduct a wrong estimation, then repeating the process for 500 times and producing 500 wrong coefficients through regression. Figure 5 shows the distribution of coefficients estimated of the fake FTZ of the regression results randomly sampled 500 times by bootstrap method, the results include the placebo test of total, direct and indirect effects.
In Figure 5, the value represented by the solid line perpendicular to the coordinate axis is 0, and the sampling result obeys a normal distribution centered at 0, so the sampling results are all credible. The dotted line represents the actual influence coefficient of FTZ on PM2.5 of −49.70, −.027, and −49.67. Only a few coefficients obtained by sampling fall near the dotted line, thus, the placebo test is passed.
4.3.5 Adjacency spatial matrix
Instead of using the inverse distance matrix, in this part, we use the adjacency spatial matrix to conduct the benchmark regression as a supplement. The adjacency matrix is a 0–1 matrix representing the adjacency relationship of cities. If two cities are neighboring, the value takes 1, and if they are not adjacent, it takes 0.
Table 9 illustrates the results of regression results with the adjacency matrix, the coefficients of FTZ are all significantly negative, verifying the robustness of the benchmark regression again.
4.4 Mechanism analysis
Based on the theoretical analysis above, this paper conjectures that pilot free trade zones can reduce air pollution through mechanisms such as attracting foreign direct investment, upgrading industrial structures, and strengthening environmental regulations. All the four variables have distinct spatial spillover properties, which means that FTZ may have spatial effects on these variables, the change of ER, IDS, SE, FDI in FTZ will possibly lead to a change in the surrounding areas, because the enhancement of environmental regulation may cause the imitation of the surrounding governments or may lead to the escape of some companies in the local area (Deng et al., 2021); the upgrading of the industrial structure in FTZ may cause the secondary industry to flow to the surrounding areas, or the preferential policies of FTZ may drive the industries in the surrounding areas to transfer to the tertiary industry (Chen et al., 2022); the development of science and technology may attract more corporations from neighboring cities as the adcanced technology can save their costs (Yuan and Yuan, 20221020); FDI attracted by FTZ also can bring benefits to adjacent cities (Tanaka and Hashiguchi, 2015). Thus, in this part, this paper establishes the following two-stage mediating effect models based on the SDM-DID model to conduct empirical research on the influencing mechanism.
In the first stage, we investigate the effects of FTZ on the mediating variables with the following formula:
Where
In the second stage, we explore the effects of the mediating variables on air pollution level based on the following formula:
Where Mi represents the mediating variables,
It can be seen in Table 10 that the coefficients of all variables are significant at the 10% level and above, indicating that all the mediating variables pass the mediating effect test. Specifically, from the sign of the coefficient we can see that FTZ can significantly reduce air pollution by reducing the ratio of the secondary industry to the tertiary industry and promoting industrial upgrading. Meanwhile, since foreign direct investment, environmental regulation, and scientific research investment have a positive impact on controlling air pollution, FTZ also reduces air pollution by increasing foreign investment, scientific and technological investment, and strengthening environmental regulation.
4.5 Heterogeneity analysis
China has a vast territory, and there are great regional differences in various regions, such as differences in energy endowments, economic development, and geographical conditions. However, the previous empirical results based on the overall sample did not consider regional differences in city location, size and city type, thus we perform heterogeneity tests in this section. First of all, as the eastern region carried out reform and opening up earlier than the central and western regions, the opening degree and economic development level of the eastern region are generally better than that of the central and western regions, and the pollution control efficiency of eastern regions might be better (Zhang et al., 141478). From the perspective of city size, the expansion of cities produces agglomeration effects, but large cities have more urban diseases, such as traffic congestion and environmental pollution, thus the air pollution reduction effect should vary by different size of each city. As for city type, provincial capitals, municipalities directly under the central government and sub-provincial cities are regarded as central cities, which are different from other cities in many aspects like economic scale and political characteristics, which may result in different policy impact. Therefore, the impact of FTZs may differ depending on their location, type, and scale. Thus, this paper adopts Spatial Differences-in-Differences-in-Differences (SDDD) for further heterogeneity analysis (Cai et al., 2016).
According to the criterion of the National Bureau of Statistics in China (Feng et al., 2021), we divided the 283 cities in 30 provinces into two parts:eastern、western and central areas.1 The SDDD model is expressed as model (11):
Where H are variables describing heterogeneity including EAST (dummy variable, 1 if city is in eastern China, otherwise is 0), SIZE (city size represented by the logarithm of city population) and CAPITAL (dummy variable, 1 if it is a Provincial capital city 2, otherwise is 0), the other variables are the same as that in the benchmark regression. Table 11 shows the results of the direct and indirect effects of the heterogeneity analysis.
The results reveal that the heterogeneity in city location, city size and city type exist. From the perspective of the coefficients, the signs of the coefficients of the direct effect and the indirect effect remain the same. In column 1), the coefficients of FTZ are both significantly negative at the 1% level, while the coefficients of FTZ×EAST are significantly positive at the 5% level. It means that the air pollution reduction effect of FTZ on cities in the eastern region is weaker than that in the central and western regions. That’s because the proportion of the tertiary industry in the eastern cities is higher than that in the central and western cities. At the same time, the eastern coastal areas have abundant rainfall and pleasant climate, so the air pollution is generally low, hence the pollution reduction effect is not obvious. In column 2), the coefficient of FTZ is significantly positive at the 1% level and the coefficient of FTZ×SIZE is significantly negative at the 1% level, indicating that the effect of the FTZ policy on reducing air pollution only exists when the scale of the city reaches a certain level. The trade volume of the free trade zone in large cities is higher, attracting more foreign capital and technological innovation, and the emission reduction effect will be shown. As for column 3), the coefficients of FTZ×CAPITAL in both direct effects and indirect effects are significantly negative, which means that FTZ in central cities have more air pollution reduction effects on both provincial capital city itself and neighboring cities. Provincial capital cities are typically more developed, with a massive economy, comprehensive systems, and strong government enforcement, which leads to more efficient execution of FTZ regulations and attracting more foreign investors, causing a greater decrease in emission levels.
5 Discussion
The statistical evidence above imply that the two assumptions of this research are validated. Nowadays, governments all around the world are concerned about environmental changes; the Chinese government is not excepted, and has introduced several environmental laws, such as carbon emission trading, to limit pollution emissions. (Xu and Lin, 2022). At the same time, China’s president has announced plans for carbon peaking in 2030 and carbon neutrality in 2060, demonstrating China’s willingness to inhibit pollution emissions (Xu, 2021). The original intention of the free trade zone policy is to promote the trade and exchanges between China and other countries under the background of economic globalization, so as to achieve the purpose of promoting the high-speed growth of the bilateral economy. When promoting economic growth, if the free trade zone can reduce environmental pollution at the same time, it will be of great significance for the green development of Chinese cities (Antweiler et al., 2001). The empirical results of this paper prove that the implementation of the free trade zone policy not only significantly improves the air quality in the region, but also significantly suppresses the air pollution in the surrounding cities, which illustrates the necessity of the implementation of the FTZ policy, and shows that implementing the FTZ policy is worthwhile for the Chinese government, and FTZ should continue to be promoted in China (Li and Liu, 20201191). Moreover, the emission reduction spillover effects of FTZs derived in this paper may also indicate that the establishment of FTZs will lead to a trend of regional integration of FTZs with surrounding cities, and regional integration can improve energy use efficiency and reduce emissions (Yu et al., 104285).
In addition, this paper also explores the mechanism of the action of FTZ policy, and provides a reference for further realizing the effect of FTZ in reducing air pollution. The original intention of FTZ is to attract foreign capital to promote regional economic growth, and while economic growth, capital flow can also drive industrial structural changes and technological development (Jiang et al., 2021). As a result of integration, more business possibilities are drawn. As a result, there is no dispute that FTZs can assist to reduce air pollution. Finally, the heterogeneity test discovered that the FTZ policy’s emission reduction effect is more useful for large cities, as well as central and western cities, which may be due to the fact that eastern regions and small cities are less polluted and difficult to further reduce, or that large cities are more polluted. The function of industrial agglomeration in central and western cities, as well as the agglomeration problem. The heterogeneity analysis findings can also be utilized to inform future policy formulation.
6 Conclusions and policy recommendations
Based on the above results, this paper draws the following conclusions: 1) The establishment of free trade zones can inhibit the promotion of air pollution in cities and surrounding cities. 2) The establishment of the free trade zone improves air quality by attracting foreign investment, upgrading the industrial structure, prompting the local government to increase environmental regulation and scientific and technological investment. 3) The air pollution reduction effect of free trade zones is more effective for central and western cities and cities with large population.
According to the results, the following recommendations can be drawn: 1) The government should continue to implement the free trade zone policy and establish free trade zones in most cities across the country in the future. 2) Relax policies to attract foreign investment, strengthen local environmental regulations in free trade zones, increase the proportion of investment in science and technology, and use free trade zones to drive the overall upgrading of the industrial structure. 3) Optimize the spatial layout of the free trade zone area. Under the premise of meeting the conditions for FTZ establishment, the FTZ policy can be tilted to central and western cities and large cities with large populations when appointing the next batch of free trade zones. 4) For cities with strong radiation capability, more subzones can be set up around FTZ to play the role of network nodes and spatial spillover to drive regional green economic development in a wider scope. 5) In the process of FTZ construction, local government should develop the supporting industrial infrastructure, absorbing domestic and foreign high-tech enterprises to join and accelerate the transformation of the industrial structure in the FTZ area. 6) At the same time, based on the spillover effect of FTZ, the cities around FTZ should actively absorb the emission reduction effect generated by FTZ construction, promote industrial structure transformation as well as green innovation development, and realize the unity of economic development and environmental protection.
Despite the extensive work done in this paper, there are still some shortcomings exist in this paper: 1) PM10, SO2, CO and other major air pollutants were not included as explained variables; 2) SAC-DID model was not applied in this study; 3) This paper did not introduce the economic distance matrix as a supplement to the inverse distance matrix and adjacency matrix; 4) Apart from the spatial effects, this paper did not study the local effects of FTZ on air pollution by DID. In future research, the green effects of FTZs can be studied in the following aspects: 1) Exploring the relationship between FTZ and regional green development; 2) Studying the relationship between FTZ and carbon emissions; 3) Using Data from listed companies in the FTZ and import and export data of customs to study specific paths of reducing environmental pollution in the FTZ.
Data availability statement
The original contributions presented in the study are included in the article/supplementary material, further inquiries can be directed to the corresponding author.
Author contributions
BL conducted research, make tables and graphs and revised the paper. BX helped perform empirical research, wrote the original paper and proofreading the paper. PL helped making figures and tables, writing codes and colleted data, as well as revising the paper. MZ helped collect data, doing literature review and revise the paper. WX conceived the concepts, conducted the empirical reseach, and wrote the original manuscript. All authors reviewed and approved the final version of this paper. BL, BX, and PL share the first authorship.
Conflict of interest
The authors declare that the research was conducted in the absence of any commercial or financial relationships that could be construed as a potential conflict of interest.
Publisher’s note
All claims expressed in this article are solely those of the authors and do not necessarily represent those of their affiliated organizations, or those of the publisher, the editors and the reviewers. Any product that may be evaluated in this article, or claim that may be made by its manufacturer, is not guaranteed or endorsed by the publisher.
References
Antweiler, W., Copeland, B. R., and Scott Taylor, M. (2001). Is free trade good for the environment? Am. Econ. Rev. 91 (4), 877–908. doi:10.1257/aer.91.4.877
Cai, X., Lu, Y., Wu, M., and Yu, L. (2016). Does environmental regulation drive away inbound foreign direct investment? Evidence from a quasi-natural experiment in China. J. Dev. Econ. 123, 73–85. doi:10.1016/j.jdeveco.2016.08.003
Central government of the people's republic of China, 2015, http://www.gov.cn/zhengce/zhengceku/2015-12/17/content_10424.htm.
Central government of the people's republic of China, 2019, http://www.gov.cn/zhengce/zhengceku/2019-08/26/content_5424522.htm.
Chen, F., and Chen, Z. (2021). Cost of economic growth: Air pollution and health expenditure. Sci. Total Environ. 755, 142543. 1. doi:10.1016/j.scitotenv.2020.142543
Chen, J., Wang, B., Huang, S., and Song, M. (2020). The influence of increased population density in China on air pollution. Sci. Total Environ. 735, 139456. doi:10.1016/j.scitotenv.2020.139456
Chen, J., Zheng, W., Zhang, F., Park, N.-k., Zheng, A., and Zhao, J. (2018). Evaluation and comparison of the development performances of typical free trade port zones in China. Transp. Res. Part A Policy Pract. 118, 506–526. doi:10.1016/j.tra.2018.09.009
Chen, Q., Wei, H-M., and Zhi, Y-P. (2022). The impact of health investment on industrial structure: “Spillover” or “crowding out”?—evidence from emerging market countries. Front. Public Health 9, 833961. doi:10.3389/fpubh.2021.833961
Cheng, H. U. I. F. A. N. G., Zhang, Y. I., and Hongyi, L. I. (2022). Effects of free trade zone policy on China’s income disparity. Singap. Econ. Rev. 67 (02), 709–732. doi:10.1142/S0217590820500320
Cheng, Z., Li, L., and Liu, J. (2020). The impact of foreign direct investment on urban PM2.5 pollution in China. J. Environ. Manag. 265, 110532. doi:10.1016/j.jenvman.2020.110532
Cole, M. A. (2004). Trade, the pollution haven hypothesis and the environmental kuznets curve: Examining the linkages. Ecol. Econ. 48 (1), 71–81. doi:10.1016/j.ecolecon.2003.09.007
Cole, M. A., Elliott, R. J. R., and Shimamoto, K. (2005). Industrial characteristics, environmental regulations and air pollution: An analysis of the UK manufacturing sector. J. Environ. Econ. Manag. 50 (1), 121–143. doi:10.1016/j.jeem.2004.08.001
De, X., Li, B., and Cheng, S. (2020). The effect of subway development on air pollution: Evidence from China. J. Clean. Prod. 275, 124149. doi:10.1016/j.jclepro.2020.124149
Deng, Y., You, D., and Zhang, Y. (2021). Can the behavioural spillover effect affect the environmental regulations strategy choice of local governments? Int. J. Environ. Res. Public Health 18, 4975. doi:10.3390/ijerph18094975
Eaton, J., Market structure and foreign trade: Increasing returns, imperfect competition and the international economy: Elhanan Helpman and Paul R. Krugman, 1985) ,, J. Int. Econ., 21, 1–2 183–187. doi:10.1016/0022-1996(86)90014-0
Elena, V., and Galeotti, M. (2011). At home and abroad: An empirical analysis of innovation and diffusion in energy technologies. J. Environ. Econ. Manag. 61 (2), 119–134. doi:10.1016/j.jeem.2010.08.004
Feng, Y., Wang, X., Zhou, L., Hu, S., Xie, Y., and Wu, G. (2021). Effects of emission trading system on green total factor productivity in China: Empirical evidence from a quasi-natural experiment. J. Clean. Prod. 294, 126262. doi:10.1016/j.jclepro.2021.126262
Ge, T., Ma, L., and Wang, C. (2022). Spatial effect of economic growth targets on CO2 emissions: Evidence from prefectural-level cities in China. Front. Environ. Sci. 10, 857225. doi:10.3389/fenvs.2022.857225
Han, C-Y., Gu, Z-L., and Yang, H. (2022). Investigate the effects of industrial agglomeration on nitrogen dioxide pollution using spatial panel Durbin and panel threshold models. Front. Environ. Sci. 10, 844479. doi:10.3389/fenvs.2022.844479
Hazari, B. R., and Sgro, P. M. (1996). Free trade zones, tariffs and the real exchange rate. Open Econ. Rev. 7, 199–217. doi:10.1007/BF01886821
Ingole, V., Dimitrova, A., Sampedro, J., Sacoor, C., Acacio, S., Juvekar, S., et al. (2022). Local mortality impacts due to future air pollution under climate change scenarios. Sci. Total Environ. 823, 153832. doi:10.1016/j.scitotenv.2022.153832
Jeffrey, A., and Rose, A. K. (2005). Frankel, andrew K. Rose; is trade good or bad for the environment? Sorting out the causality. Rev. Econ. Statistics 87 (1), 85–91. doi:10.1162/0034653053327577
Ji, M., Li, M., and King, B. (2015). The Impacts of China's new free-trade zones on Hong Kong tourism. J. Destination Mark. Manag. 4 (4), 203–205. doi:10.1016/j.jdmm.2015.08.001
Jia, R., Shao, S., and Yang, L. (2021). High-speed rail and CO2 emissions in urban China: A spatial difference-in-differences approach. Energy Econ. 99, 105271. doi:10.1016/j.eneco.2021.105271
Jiang, S., Tan, X., Hu, P., Wang, Y., Shi, L., Zhong, M., et al. (2022). Air pollution and economic growth under local government competition: Evidence from China, 2007–2016. J. Clean. Prod. 334, 130231. doi:10.1016/j.jclepro.2021.130231
Jiang, Y., Wang, H., and Liu, Z. (2021). The impact of the free trade zone on green total factor productivity ——Evidence from the shanghai pilot free trade zone. Energy Policy 148, 112000. doi:10.1016/j.enpol.2020.112000
Kampa, M., and Elias, C. (2008). Human health effects of air pollution. Environ. Pollut. 151 (2), 362–367. doi:10.1016/j.envpol.2007.06.012
Kelley Pace, R., and LeSage, J. P. (2008). A spatial Hausman test. Econ. Lett. 101 (3), 282–284. doi:10.1016/j.econlet.2008.09.003
Kukla-Gryz, A. (2009). Economic growth, international trade and air pollution: A decomposition analysis. Ecol. Econ. 68 (5), 1329–1339. doi:10.1016/j.ecolecon.2008.09.005
L Ping, J., J Green, C., E Zartman, R., and F Bronson, K. (2004). Exploring spatial dependence of cotton yield using global and local autocorrelation statistics. Field Crops Res. 89 (2–3), 219–236. doi:10.1016/j.fcr.2004.02.009
Li, C., and Liu, B. (2019). Air pollution embodied in China’s trade with the BR countries: Transfer pattern and environmental implication. J. Clean. Prod. 247, 119126. doi:10.1016/j.jclepro.2019.119126
Li, H., Chen, J., Wan, Z., Zhang, H., Wang, M., and Bai, Y. (2020). Spatial evaluation of knowledge spillover benefits in China’s free trade zone provinces and cities. Growth Change 51, 1158–1181. doi:10.1111/grow.12410
Li, S., Liu, J., and Kong, Y. (2021). Pilot free trade zones and Chinese port-listed companies performance: An empirical research based on quasi-natural experiment. Transp. Policy 111, 125–137. doi:10.1016/j.tranpol.2021.07.022
Li, Z., and Wang, J. (2022). The dynamic impact of digital economy on carbon emission reduction: Evidence city-level empirical data in China. J. Clean. Prod. 351, 131570. doi:10.1016/j.jclepro.2022.131570
Liberato, A. S. Q., and Fennell, D. (2007). Gender and well-being in the Dominican republic: The impact of free trade zone employment and female headship. World Dev. 35 (3), 394–409. doi:10.1016/j.worlddev.2006.11.003
Lin, F. (2017). Trade openness and air pollution: City-level empirical evidence from China. China Econ. Rev. 45, 78–88. doi:10.1016/j.chieco.2017.07.001
Lin, X., Leng, L., and Qiu, Y. (2021). Research on the policy effect of free trade zone on regional innovation capability: Evidence from China. Appl. Econ. Lett. 2021, 1–6. doi:10.1080/13504851.2021.2011101
Liu, J., Wang, X., and Guo, J. (2021). Port efficiency and its influencing factors in the context of pilot free trade zones. Transp. Policy 105, 67–79. doi:10.1016/j.tranpol.2021.02.011
Managi, S., Hibiki, A., and Tsurumi, T. (2009). Does trade openness improve environmental quality? J. Environ. Econ. Manag. 58 (3), 346–363. doi:10.1016/j.jeem.2009.04.008
PedroAloise, G., and Macke, J. (2017). Eco-innovations in developing countries: The case of Manaus free trade zone (Brazil). J. Clean. Prod. 168, 30–38. doi:10.1016/j.jclepro.2017.08.212
Pommeret, A., Yu, X., and Zhang, L. (2022). Stringency of environmental policy in China: When pollution drives bribery. Econ. Model. 117, 106048. doi:10.1016/j.econmod.2022.106048
Qi, C., Yuan, B., Zhou, Y., Chen, H., and Hu, Y. (2021). Will bad air quality affect the siting of foreign-invested manufacturing enterprises? The evidence from China. Appl. Econ. 53 (45), 5221–5241. doi:10.1080/00036846.2021.1922588
Tanaka, K., and Hashiguchi, Y. (2015). Spatial spillovers from foreign direct investment: Evidence from the yangtze river delta in China. China and World Econ. 23, 40–60. doi:10.1111/cwe.12106
Tong, F., Du, H., Lin, Z., and Zuo, J. (2020). Spatial spillover effects of environmental regulations on air pollution: Evidence from urban agglomerations in China. J. Environ. Manag. 272, 110998. doi:10.1016/j.jenvman.2020.110998
Wang, F., He, J., and Yuan, N. (2022). Role of foreign direct investment and fiscal decentralization on urban haze pollution in China. J. Environ. Manag. 305, 114287. doi:10.1016/j.jenvman.2021.114287
Wang, K.-L., Zhao, B., Ding, L.-L., and Miao, Z. (2020). Government intervention, market development, and pollution emission efficiency: Evidence from China. Sci. Total Environ. 757, 143738. doi:10.1016/j.scitotenv.2020.143738
Xie, Y., Dai, H., Zhang, Y., Wu, Y., Hanaoka, T., and Masui, T. (2019). Comparison of health and economic impacts of PM2.5 and ozone pollution in China. Environ. Int. 130, 104881. doi:10.1016/j.envint.2019.05.075
Xu, W., and Lin, J. (2022). Fiscal decentralization, public health expenditure and public health–evidence from China. Front. Public Health 10, 773728. doi:10.3389/fpubh.2022.773728
Xu, W., Xie, B., Lou, B., Wang, W., and Wang, Y. (2022). Assessing the effect of energy poverty on the mental and physical health in China—evidence from China family panel studies. Front. Energy Res. 10, 944415. doi:10.3389/fenrg.2022.944415
Xu, W. (2021). The impact and influencing path of the pilot carbon emission trading market--evidence from China. Front. Environ. Sci. 9, 787655. doi:10.3389/fenvs.2021.787655
Yu, C., Long, H., Tu, C., Tan, Y., Zang, C., and Zhou, Y. (2022). Assessing the effectiveness of innovative city pilots in improving urban carbon emission performance: A spatial difference-in-difference approach. Front. Environ. Sci. 10, 983711. doi:10.3389/fenvs.2022.983711
Yu, C., Long, H., Zhang, X., Tu, C., Tan, Y., Zhou, Y., et al. (2022). Regional integration and city-level energy efficiency: Evidence from China. Sustain. Cities Soc. 88, 104285. doi:10.1016/j.scs.2022.104285
Yu, C., Tan, Y., Zhou, Y., Zang, C., and Tu, C. (2022). Can functional urban specialization improve industrial energy efficiency? Empirical evidence from China. Energy 261, 125167. doi:10.1016/j.energy.2022.125167
Yuan, i., and Yuan, Y. (2022). Government intervention, spillover effect and urban innovation performance: Empirical evidence from national innovative city pilot policy in China. Technol. Soc. 70, 102035. doi:10.1016/j.techsoc.2022.102035
Zeng, J., Bao, R., and Michael, M. F. (1058). Clean energy substitution: The effect of transitioning from coal to gas on air pollution. Energy Econ. 107, 105816. doi:10.1016/j.eneco.2022.105816
Zeng, J., Wen, Y., Bi, C., and Feiock, R. (2021). Effect of tourism development on urban air pollution in China: The moderating role of tourism infrastructure. J. Clean. Prod. 280, 124397. doi:10.1016/j.jclepro.2020.124397
Zhang, H., Song, Y., and Zhang, L. (2020). Pollution control in urban China: A multi-level analysis on household and industrial pollution. Sci. Total Environ. 749, 141478. doi:10.1016/j.scitotenv.2020.141478
Zheng, W., Zhang, Y., Wang, X., and Chen, J. (2014). Policy and politics behind shanghai’s free trade zone program. J. Transp. Geogr. 34, 1–6. doi:10.1016/j.jtrangeo.2013.10.015
Zhu, L., Yu, H., Lu, Z.-N., Wu, H., and Ran, Q. (2019). Do economic activities cause air pollution? Evidence from China’s major cities. Sustain. Cities Soc. 49, 101593. doi:10.1016/j.scs.2019.101593
Zhu, Y., Wang, Z., Yang, J., and Zhu, L. (1195). Does renewable energy technological innovation control China’s air pollution? A spatial analysis. J. Clean. Prod. 250, 119515. doi:10.1016/j.jclepro.2019.119515
Keywords: spatial differences-in-differences, spatial spillover, intermediary effect test, free trade zone, spatial differences-in-differences-in-differences, urban PM2.5 emission
Citation: Lou B, Xie B, Liu P, Zhu M and Xu W (2023) Evaluating the impact of free trade zone construction on urban air pollution in China—Empirical evidence from a spatial differences-in-differences approach. Front. Environ. Sci. 10:1075138. doi: 10.3389/fenvs.2022.1075138
Received: 20 October 2022; Accepted: 28 December 2022;
Published: 11 January 2023.
Edited by:
Lin Zhang, City University of Hong Kong, Hong Kong SAR, ChinaCopyright © 2023 Lou, Xie, Liu, Zhu and Xu. This is an open-access article distributed under the terms of the Creative Commons Attribution License (CC BY). The use, distribution or reproduction in other forums is permitted, provided the original author(s) and the copyright owner(s) are credited and that the original publication in this journal is cited, in accordance with accepted academic practice. No use, distribution or reproduction is permitted which does not comply with these terms.
*Correspondence: Wangzi Xu, xuwangzi1016@163.com
†These authors have contributed equally to this work and share first authorship