- 1Key Laboratory of Digital Earth Science, Aerospace Information Research Institute, Chinese Academy of Sciences, Beijing, China
- 2International Research Center of Big Data for Sustainable Development Goals, Beijing, China
- 3School of Land Science and Technology, China University of Geoscience, Beijing, China
- 4Research Center for Eco-Environmental Sciences, Chinese Academy of Sciences, Beijing, China
- 5Airborne Remote Sensing Center, Aerospace Information Research Institute, Chinese Academy of Sciences, Beijing, China
Cities in tropical regions are experiencing high heat risks by overlaying the urban heat island (UHI) effect. Urban green space (UGS) can provide local cooling effect and reduce UHI. However, there still lack a comprehensive exploration of the characteristics of UHI and cooling effect of UGS due to high cloud coverage and limited number of available remote sensing observations. In this study, the enhanced spatial and temporal adaptive reflectance data fusion method was employed to develop an enhanced land surface temperature data in winter seasons in three tropical megacities, Dhaka, Kolkata, and Bangkok. The spatiotemporal variations of surface urban heat island (SUHI) were explored from 2000 to 2020 with a 5-years interval. The optimal size of UGS associated with its cooling effects was assessed by using the threshold value of efficiency (TVoE). The relationship between the intensity and range of urban cooling island (UCI) and four landscape metrics of green space patches, total area (P_Area), shape index (P_SI), normalized difference vegetation index (P_NDVI), and land surface temperature (P_LST), were analyzed. The results show that the average SUHI intensity increased by 0.98°C, 1.42°C, and 0.73°C in Dhaka, Kolkata, and Bangkok, respectively, from 2000 to 2020. The maximum intensity of UCI ranges from 4.83°C in Bangkok to 8.07°C in Kolkata, and the maximum range of UCI varies from 300 m in Bangkok to 420 m in Kolkata. The optimal size of green space is 0.37 ha, 0.77 ha, and 0.42 ha in Dhaka, Kolkata, and Bangkok, respectively. The P_NDVI and P_Area had significant positive effects on UCI intensity and range, while the background temperature had significant negative effects. With higher background temperature, the optimal patch size of UGS is larger. This study provides useful information for developing effective heat mitigation and adaptation strategies to enhance climate resilience in tropical cities.
Introduction
The world has been experiencing an inevitable trend of urbanization (Girardet, 2020). According to the World Urbanization Prospects report (United Nations, 2019), the urban population of the world will continue to rise and 90% of the increase concentrates in Asia and Africa. As cities continue to expand, roads and buildings replace some natural surfaces such as trees, water bodies and soil. The transformation of land use changes biophysical properties of the land surface, which could generate the local warming effect or urban heat island (UHI) effect (Oke, 1973; Arnfield, 2003). The UHI effects and global scale climate change are projected to cause a large number of extreme heat events, thereby contributing to heat-related mortality (Stone et al., 2014). It was estimated that with a 2.0°C increase in temperature, the annual heat-related mortality increased from 32.1 per million people in 1986 to 81.3 per million people in 2005 in China (Wang et al., 2019). Megacities in tropical regions of Asia have dense population density, and were predicted to experience more frequent and intense heatwaves for the next decades (Coleman, 2022). He 11th goal of United Nation’s sustainable development goals (SDGs) advocates making cities inclusive, safe, resilient, and sustainable (United Nations, 2015). Exploring the UHI effect and the effectiveness of mitigation measures are crucial for reducing heat risk and enhancing climate resilience in these cities.
Since spaceborne remote sensing can provide timely and objective observations over vast areas, spatiotemporal changes in surface urban heat island (SUHI) at local, regional and global scales were examined by using remotely-sensed land surface temperature (LST) data (Liu et al., 2020b; Weng 2009; Lu et al., 2019). Due to high cloud coverage, the amount of cloud-free and high quality remote sensing data in tropical regions is limited. In order to acquire remote sensing data with both high spatial and temporal resolution, spatiotemporal data fusion methods have been developed (Gao et al., 2006; Zhu et al., 2010). Gao et al. (2006) developed a Spatial and Temporal Adaptive Reflectance Fusion Model (STARFM) to product daily surface reflectance data at a spatial resolution of 30 m. To address landscape heterogeneity, Zhu et al. (2010) further developed the enhanced spatial and temporal adaptive reflectance fusion model data fusion method (ESTARFM) to improve the accuracy of predicted images. The ESTARFM model achieved a satisfied accuracy in generating high spatio-temporal land surface temperature data in previous studies (Liu et al., 2020b).
Urban green space (UGS) including parks, street vegetation, green roofs, and woodlands is a commonly adopted measure to mitigate the UHI effect (Aram et al., 2019). UGS provides cooling effects to their surroundings through several processes such as shading and evapotranspiration. However, the cooling effect of UGS is spatially limited and generally decreases with distance from UGS. Previous studies reported that beyond a certain distance, the cooling effect of UGS patches disappears (Feyisa et al., 2014). To quantify the cooling effect of UGS, Yu et al. (2017) defined the intensity, range and efficiency of urban cooling island (UCI), as well as the threshold value of efficiency (TVoE). The TVoE has been used to acquire the most suitable patch size of urban green space in different study areas (Fan et al., 2019; Yang et al., 2020; Tan et al., 2021). Numerous evidences from field observations and remote sensing data proved that the cooling intensity and range of parks have a positive relationship with their areas (Lin et al., 2015; Algretawee 2022; Vaz Monteiro, 2016). Besides the size of green space, factors such as the composition, configuration, background temperature, neighboring vegetation cover of green space patches also impact their cooling effects (Akbari and Kolokotsa, 2016; Gillner et al., 2015; Yu et al., 2018b; Zhou et al., 2022).
Although the megacities in tropical Asia are vulnerable to extreme heat events, the unavailability of satellite data with both high spatial and temporal resolution hindered the in-depth investigation of urban heat islands and cooling effects in these cities (Giridharan, 2018). Uddin et al. (2022) used the day and night time temperature data provided by MODIS to quantify UHI in Dhaka. They found that the UHI effect was significantly related to population change, urban expansion, and meteorological factors such as cloud cover. Parvin and Abudu (2017) used Landsat data to assess UHI in Dhaka from 2002 to 2014 and reported that the temperature of all types of land cover was rising. Das et al. (2020) used MODIS data to study UHI seasonal changes in Kolkata and the relationship between UHI and vegetation. Halder et al. (2021) investigated the relationship between LST and vegetation and built-up areas using Landsat data and predicted the urban expansion in Kolkata in the next 30 years. Khamchiangta and Dhakal (2019) used Landsat data to study the temperature difference of different land cover types and the relationship between heat island and urban physical structure and non-physical factors in Bangkok. Although the heat island phenomenon has been investigated in previous studies, the analysis based on MODIS data failed to provide sufficient spatial details, while the observation of Landsat was relatively sparse in time. In addition, the regulation capacity of urban green space on thermal environment has barely been explored in these cities.
The megacities located in the tropical and subtropical regions have similar climate background conditions. By adopting standardized analysis methodologies, a comparative analysis of these cities could provide comprehensive information for the design and planning of green space in cities in tropical climate zones. Taking three megacities, Dhaka, Kolkata, and Bangkok, as the study area, the objectives of our study include 1) to generate land surface temperature data with high spatial and temporal resolution in winter season from 2000 to 2020 using data fusion model, 2) to quantify spatiotemporal changes in SUHI from 2000 to 2020 and explore their relationship with land cover using the enhanced LST data, and 3) to calculate the threshold value of efficiency (TVoE) of green space and analyze the relationship between their cooling effect and landscape metrics.
Study area and data sets
Study area
The study areas consist of three megacities in Asia: Dhaka, Kolkata, and Bangkok (Figure 1). The three megacities are located in tropical or subtropical regions, and the seasonality of temperature and precipitation in these cities are similar. They are all categorized as tropical savannah (Aw) climate zone in the Köppen-Geiger classification scheme (Beck et al., 2018). Dhaka is the largest city in Bangladesh and one of the major cities in South Asia with a population of over 15 million. Kolkata is the capital of West Bengal and the third largest city in India after Mumbai and New Delhi. In addition, it is the largest trading and commercial center in eastern India which is located 150 km north of the Bay of Bengal above the plains of the Ganges Delta (Nath et al., 2015). As the capital and largest city of Thailand, Bangkok is the second largest city in Southeast Asia. It is the center of politics, economy, trade, transportation, culture, science and technology, education, religion and all aspects of Thailand. Since the study focused on SUHI analysis, urban functional areas of each city were delineated as main study areas.
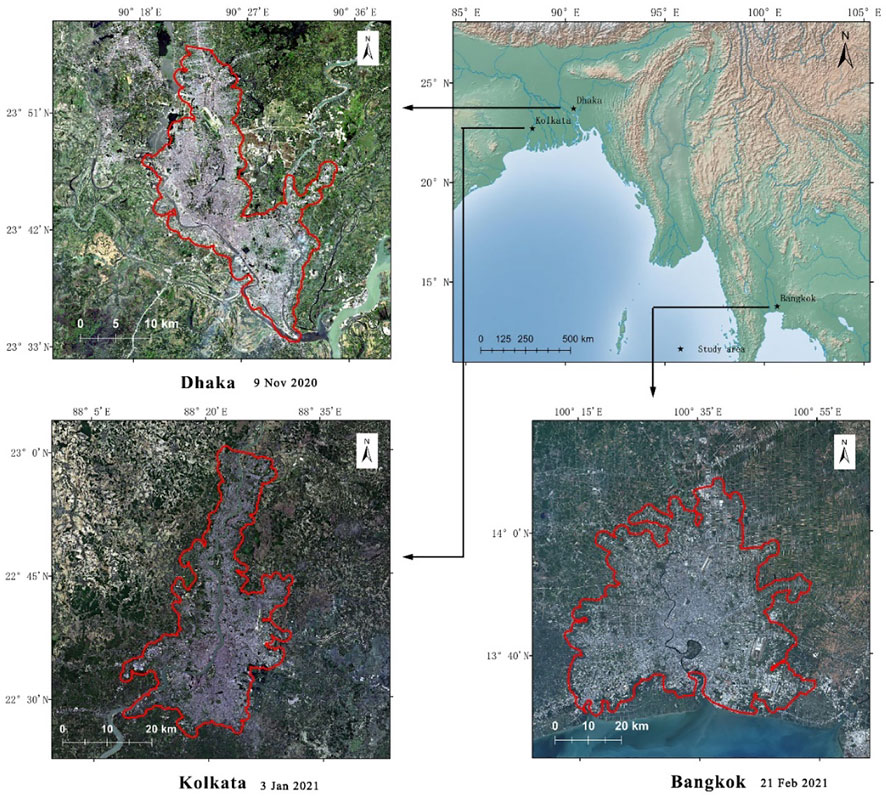
FIGURE 1. The geographic location of the study area. The red polygons represent the urban functional boundary. The underlying satellite images are from Landsat-8 OLI sensor.
Datasets and preprocessing
Landsat data of the study area covering five time periods: 2000, 2005, 2010, 2015, and 2020 were acquired in this study. It was obtained from the United States Geological Survey (USGS) (https://earthexplorer.usgs.gov/). One Landsat image with good data quality in each time period was selected for land cover classification and two Landsat images were both used for data fusion. The details about Landsat images for land cover classification are listed in Table 1 and the details for data used for fusion are listed in Table 2. Radiometric calibration and atmospheric correction were performed for the preprocessing of the Landsat images. The red, near-infrared, and thermal infrared bands were extracted for data fusion.
MODIS data is provided by the United States National Aeronautics and Space Administration (NASA) (https://ladsweb.modaps.eosdis.nasa.gov/). The MODIS data we used includes surface reflectance data from MOD09GA and MOD09A1 products and daytime land surface temperature data from MOD11A1 and MOD11A2 8-day composite products. Due to the frequent cloud coverage in the study areas, cloud-free remote sensing images in winter months (December to February) were selected to predict the Landsat-like land surface temperature data (Table 2).
The original MODIS data is stored in HDF format. The bands selected from MOD09GA/MOD09A1 are B01 and B02, while Emis_31 and LST_Day_1 km bands were selected from MOD11A1/MOD11A2. Batch data processing codes were used to convert the original MODIS data to geotiff format using MODIS Reprojection Tool. The extracted data bands were converted to surface reflectance by applying the scale factor and offset using the following equation:
where
The blackbody radiance can be calculated according to Eq. 2:
where
The radiant brightness in thermal infrared band received by MODIS satellite sensor is calculated using the radiative transfer equation:
where
Methodology
The data processing workflow mainly includes four steps (Figure 2): land cover classification, land surface temperature (LST) retrieval, surface urban heat island (SUHI) analysis and cooling effects analysis for urban green space (UGS).
Land cover classification
Land cover types including impervious surface, bare land, green space and water were classified using Support Vector Machine (SVM) classifier in the study areas for 2000, 2005, 2010, 2015, and 2020. Training and validation samples were randomly collected on Google Earth. The definition criteria issued by the United Nations Human Settlements Programme was used to determine the urban functional boundary (Lu et al., 2022). The urban functional boundary could reflect the urbanization situation in the study area more accurately than the administrative boundary. According to the land cover classification, the pixels with above 50% built-up density was defined as the urban pixel, 25%–50% was defined as the suburban pixel, and less than 25% was defined as the rural pixel. The area within 100 m around the urban or suburban pixels was defined as the urban open space, and the urban, suburban and urban open space pixels were merged. Then, the area of each polygon was expanded by 25% to establish buffer zones. The buffer zone with the largest area is the urban functional area, and its boundary is the functional boundary of the city. The urban functional boundaries of the three study areas were created using the land cover classification results in 2020 (Figure 1).
Land surface temperature retrieval
We used ESTARFM to fuse Landsat and MODIS data in this study (Zhu et al., 2010). Landsat and MODIS images at the time of
Among the commonly used land surface retrieval algorithms, the algorithm based on radiative transfer equation has a solid physical basis and high inversion accuracy (Lu et al., 2020; Duan et al., 2021). Radiative transfer equation-based method and the fused Landsat-like data were used to retrieve the LST in our study. The red band and near-infrared band of the fused image were used to calculate the normalized difference vegetation index (NDVI) (Lu et al., 2015; Yuan et al., 2018):
where NIR is the near-infrared band and RED is the red band of the image.
Then, the vegetation coverage
where
The land surface emissivity was then calculated as:
by using typical constant values for the emissivity of vegetation and soil, m and n were set as 0.004 and 0.986, respectively (Sobrino et al., 2008).
The blackbody radiance was calculated using the radiative transfer equation:
The land surface temperature was calculated as:
The temperature,
Surface urban heat island analysis
The surface urban heat island intensity (SUHII) can be defined as the difference between the surface temperature of built-up area and green space (Estoque and Murayama, 2017; Bechtel et al., 2019). At each built-up area pixel, the SUHII was calculated using the following equation:
where
The SUHII of the study area was further divided into five levels as: very low level (
where
Quantifying the urban cooling effect
UGS patches were manually selected using Google Earth for each study area. Due to the complex composition of green space, only green space patches with dense trees were selected in order to control the influencing variables and dense trees are recognised as having strong cooling effects. The selection of green space patches follows several rules: select green patches that are primarily covered by trees, green patches that have no internal water spots and are more than 300 m away from other green spaces or water bodies to avoid interaction between samples, and green patches smaller than 900 m2 (30 m*30 m) were removed. Based on these rules, 73, 90, and 107 green space patches were delineated in Dhaka, Kolkata and Bangkok, respectively.
The intensity and range of urban cooling island (UCI) were used to quantify the cooling effect of green space patches in our study. The intensity of UCI was calculated as the temperature difference between the temperature of the green patch and the peak temperature at the first turning point when the temperature decreased (Yu et al., 2018b). The cooling range is the distance from the edge of the patch to the first turning point on the temperature curve (Yu et al., 2017). In order to study UGS cooling effect, we used Arcpy scripts to create 18 buffers for each green patch. Since the image resolution is 30 m, the buffer distance is set to 30 m, and then the average LST of each buffer was calculated. According to previous studies (Fan et al., 2019), 540 m (18*30 m) could cover far more of the cooling range that a green space patch can produce. Then, the UCI intensity and range of each green space patch were calculated. The patches which LST dropped at the beginning were treated as abnormal patches and removed in our analysis.
Yu et al. (2017) defined the threshold value of efficiency (TVoE) to find the optimal patch size of urban green space in different study areas. UCI intensity increases rapidly in the first half of the period with the increase of patch area, while the change of UCI intensity is no longer obvious after exceeding a certain value (Yu et al., 2018a; Yu et al., 2018b; Fan et al., 2019). The log function is used to fit the change in UCI intensity according to Eq. 11:
where a is the coefficient of the log function; b is the constant of the log function. The critical point where the slope of the log function is equal to one is defined as TVoE. The UCI intensity increases significantly with the increase in patch size before the TVoE, and increase insignificantly after exceeding the TVoE. The logarithmic regression was used to extract the TVoE or optimal green space patch size in each city.
Statistical analysis
Following previous studies (Sun and Chen, 2017; Fan et al., 2019; Yu et al., 2020; Shah et al., 2021; Tan et al., 2021), four dominant factors, namely normalized difference vegetation index (P_NDVI), land surface temperature (P_LST), shape index (P_SI) and area (P_Area) of UGS patches were selected to explore their influence on the cooling intensity and range of UGS. The shape index represents the complexity of patch shape. The larger the value of the shape index, the more complex the shape is. The four metrics, their definition and description are listed in Table 3.
Pearson correlation was used to examine the relationship between the UCI effect and relevant landscape metrics. In specific, The UCI effect includes cooling intensity and cooling range and landscape metrics include P_NDVI, P_LST, P_SI, and P_Area. Pearson correlation coefficients were calculated to represent the magnitude of the correlation between UCI effect and relevant landscape metrics. Landscape metrics can affect UCI effect independently as well as interactively. Due to the possible correlation between landscape metrics, partial correlation analysis was performed to exclude the interaction between the landscape metrics.
Landscape metrics were used as independent variables, and the intensity and range of UCI effect were used as dependent variables. For landscape metrics with significant correlation relationship with UCI, regression analysis was conducted to analyze their effects on cooling effects. Because the relationship between the two is not necessarily a simple linear relationship, multiple functions were used to find the optimal regression. The R2 represents the degree to which the dependent variable can be explained by the model. The coefficient reflects the expected change in the dependent variable for every unit change in the associated independent variable. The ordinary least squares (OLS) regression was performed using SPSS25.0 software.
Results
Land cover changes
Table 4 shows the validation results of land cover maps for these three Asian megacities in five time periods. The overall accuracies range from 85.75% to 91.78%, and the Kappa coefficients range from 0.82 to 0.88. For Dhaka and Kolkata, low overall accuracies were obtained in 2000. It might be caused by the relatively low image quality.
The three cities have experienced significant urban development from 2000 to 2020. The urban areas have continuously expanded and green space has shrunk (Figure 3). The land cover changes in the three cities experienced similar changing trajectories. From 2000 to 2020, the green space of the three cities decreased by 1138.69 ha, 13894.52 ha, and 14416.24 ha respectively. Bare land decreased by 15257.98 ha, 20315.92 ha, and 109164.43 ha, respectively. The area of water bodies changed slightly in Dhaka and Kolkata. The impervious surface of each city expanded rapidly and increased by 16062.34 ha, 34707.11 ha, and 112698.38 ha respectively. The built-up areas in the three cities are rapidly expanding outward. The green space and bare land areas were mainly converted into impervious surface areas.
Spatiotemporal variation of surface urban heat island
According to previous studies (Weng et al., 2014; Liu et al., 2020b), the observed Landsat data can be used to evaluate the accuracy of prediction results. The LST data obtained from the Landsat image on 13 December 2003 was used to estimate the accuracy of the LST from the predicted image. At the significance level of 0.01, MAD and RMSE between the two datasets were 0.26°C and 2.02°C, respectively, and Pearson’s value was 0.99. These results indicated that there was a strong correlation between predicted and observed LST (Figure 4).
Figure 5 shows the spatial pattern changes of SUHII in the three cities from 2000 to 2020. The High Level and Very High Level areas in all three cities continue to extend outward. The high level areas are concentrated in the city center in 2000, while they spread to the whole functional area of the city in 2020. The SHUII in the three cities show different spatial patterns. Multiple high intensity areas were observed in Dhaka in 2020. Some marginal areas even have higher heat island intensity than the central areas, and most high-intensity areas are concentrated in the central and northwest of the city. The SUHI in Kolkata sprawls in all directions from the city center. In Bangkok, the SUHI gradually spreads to the east and west from 2000 to 2020. Compared with the land cover map, it is obvious that the spatial evolution of SUHI corresponds well with the spatial pattern of urban expansion.
The temporal variations of SUHII and LST differences in the three cities were analyzed from 2000 to 2020 (Figure 6). The intensity of SUHI showed a monotonic increasing trends, and experienced a fluctuation in 2010 only in Bangkok. The changing slope of SUHII indicated that all three cities has been experiencing intensified SUHI effect. The continuous rise of SUHII is related with the increase of the difference between urban temperature and background temperature. The increase of urban surface temperature is significantly faster than that of background temperature. From 2000 to 2020, the urban surface temperature in three cities increased by 4.03°C, 8.39°C, and 4.47°C respectively, and the background temperature increased by 3.05°C, 6.97°C, and 3.74°C respectively.
Urban cooling effects
Figure 7 shows the logarithmic regression analysis results in each city. Based on the fitted logarithmic regression models, the TVoE values were estimated. The p-value of each city is less than 0.01, indicating that the relationship is significant. The TVoE is 0.37 ha (R2 = 0.08), 0.77 ha (R2 = 0.31) and 0.42 ha (R2 = 0.12) in Dhaka, Kolkata and Bangkok, respectively.
Table 5 shows the statistics of landscape metrics and UCI intensity in the study areas. The mean patch size of greens space is larger in Kolkata and Bangkok than Dhaka. The shape index of green patches is largest in Bangkok. The average NDVI and LST of UGS are higher in Kolkata than the other two cities. The maximum UCI intensity ranges from 4.83°C in Bangkok to 8.07°C in Kolkata. The mean UCI intensity is highest in Kolkata and lowest in Dhaka. The maximum UCI range varies from 300 m in Bangkok to 420 m in Kolkata. The mean UCI range varies from 67 m in Bangkok to 81 m in Dhaka.
The correlation between landscape metrics and cooling effects in the megacities was also analyzed (Table 6). Among the four metrics, P_NDVI has a significant positive correlation with UCI intensity in Kolkata and Bangkok, but only has a significant positive correlation with UCI range in Bangkok. P_LST has a strong influence on UCI intensity and UCI range in all the cities, and the absolute values of Pearson correlation coefficients are greater than 0.50 on UCI intensity. For P_SI index, although correlation is negative on UCI intensity, the relationship is no significant. P_Area has a strong influence on UCI intensity and UCI range in the study areas.
For the significant correlations, the effects of landscape metrics were further analyzed using OLS regression (Table 7). The increase in NDVI leads to increase of UCI intensity in Kolkata (coefficient = 8.37) and Bangkok (coefficients = 4.83) and increase of UCI range in Bangkok (coefficient = 201.99). An increase in average LST is significantly associated with the decrease of UCI intensity and range in all the megacities. The effect of LST on UCI intensity in strongest in Kolkata, and its effect on UCI range is most significant in Dhaka. In addition, the increase of patch area is significantly associated with the increase of UCI intensity and range in all the megacities.
Discussion
Spatiotemporal variation of surface urban heat island
The study areas have a tropical monsoon climate, which is cloudy and rainy almost all year round. These lead to the difficulty in obtaining continuous gap-free remote sensing images. The blending of MODIS and Landsat data produced LST data with high spatio-temporal resolution. Since MODIS data have a spatial resolution of 1 km, narrow rivers and small-sized land features cannot be identified from the LST images. Compared with Hassan et al. (2021) which used MODIS data to explore SUHI effect, our data captured more detailed information (Figure 5). The overall land cover change results are similar to previous studies (Estoque et al., 2017; Si et al., 2022). The comparison between the land cover and SUHI map indicates that the expansion of urban impervious surface areas affects the variations of heat island. Due to the different physical properties of visible band and thermal infrared band, the visible bands and thermal infrared bands were fused separately, which effectively improved the accuracy of data fusion. The data fusion methods and processing framework have been developed to improve the efficiency and accuracy of data fusion. Since data gaps often exist in MODIS data, Liu et al. (2020b) used a mean filter or linear regression to fill the missing pixels, which enhanced data availability and provided richer information for fusion. Since the moving window of ESTARFM model has a fixed size, Liu et al. (2020a) used surface heterogeneity information to develop a new spatiotemporal data fusion algorithm which could automatically adjust the size of the moving window. These methods can be tested and applied to improve the accuracy of fused LST data in future studies. Based on the multi-temporal land cover and SUHII maps, the variations of land cover and urban thermal environment from 2000 to 2020 was captured clearly in the study areas.
Urban cooling effects and policy implications
The maximum intensity of UCI was found to range from 4.83°C to 8.07°C, and the maximum range of UCI varies from 300 m to 420 m in the three megacities. Our result show that the largest park in Kolkata (39 ha) provided a maximum cooling island effect with an intensity of 8.07°C and range of 420 m. Surveys in different seasons, times of day, and measuring methods indicated that the maximum cooling of large-sized parks could reach 5°C–8°C (Aram et al., 2019; Yin et al., 2022). The average night-time cooling range of Kensington Gardens (111 ha) in London was found to vary between 20 and 440 m (Doick et al., 2014). The Heiwa Park (147 ha), Nagoya had a cooling distance of 200–300 m during night and 300–500 m during daytime (Aram e al., 2019). The mean cooling range and intensity of blue-green space are 150 m and 2.47°C in summer in Copenhagen (Yang et al., 2020). Different TVoE values to provide the optimal green space patch area were obtained for each megacity. Our study shows that the TVoE is 0.37 ha, 0.77 ha, and 0.42 ha in Dhaka, Kolkata and Bangkok, respectively. Geng et al. (2022) applied the TVoE method to 207 urban parks in 27 cities in East China with four different local background climates, including warm temperate sub-humid monsoon, northern subtropical sub-humid monsoon, northern subtropical humid monsoon, and middle subtropical humid monsoon climate, and found that the TVoE was significantly affected by the background climate. Fan et al. (2019) found that the TVoE was about 0.6–0.62 ha in Hong Kong, Jakarta, Mumbai, and Singapore, and 0.92–0.96 ha in Kaohsiung, Kuala Lumpur, and Taiwan. Tan et al. (2021) found that the TVoE of three-based green spaces was about 0.31 ha in Nanning, China. The TVoE was found to be 4.55 ha in Fuzhou (Yu, et al., 2017). According to Fan et al. (2019), the TVoE was influenced by the background temperature and NDVI of green patches in the seven hot humid Asian cities. With higher background temperature, the optimal size of green space will be larger. This agrees well with our study.
Our results indicate that UCI intensity is correlated negatively with P_LST and positively with P_NDVI and P_Area, while UCI range is correlated negatively with P_LST and positively with P_Area. Since dense vegetation can increase the NDVI value and UCI intensity, the planting of woody vegetation is suggested to be increased in urban green space. The results also show that patch shape index has insignificant relationship with UCI intensity and range. Thus, city planners should choose the most convenient and economic way to plan green patches in terms of green space shapes. The effective method to enhance UCI intensity and range include increasing the area and biomass of green space, and lowering its background temperature. However, different results have been reported in previous studies. Some studies found that the correlation between P_Area, P_NDVI, P_SI, and UCI was insignificant (Shashua-Bar and Hoffman, 2000; Derkzen et al., 2017). The cooling effect of UGS is a huge and complex system (Murakawa et al., 1991). It is not only related to these landscape metrics, but also to other factors such as local climate background (Oliveira et al., 2011), geographical location (Hathway and Sharples, 2012), detailed landscape components (Zhao et al., 2014), humidity and evaporation (Vaz Monteiro et al., 2016; Zhou et al., 2017). In addition, ordinary linear regression (OLS) was used in our study. The OLS assumes that a linear relationship exists between the dependent and the independent variables. It also assumes error terms are independent and normally distributed. Spatial regression models such as spatial lag model, spatial error model and geographically weighted regression can be applied in order to account for spatial dependencies in the relationship between characteristic of UGS and intensity and range of UCI (Bartesaghi-Koc., 2022; Baqa et al., 2022).
Urban ecology is a huge system, and any environmental factors are likely to affect urban cool effects (Yu et al., 2017). The proposed methods can be adopted in other tropical cities to obtain more generalizable findings about the effects of urban greening characteristics on cooling effects in tropical climates (Bartesaghi-Koc et al., 2018). The physical characteristics of trees, the patterns of planting, and arrangement can also influence the air temperature and cooling effects of green infrastructures (Hami et al., 2019). The effects of these factors can be investigated by using more precise and comprehensive approaches in future studies. Moreover, the cooling effect of city parks varies in different seasons (Yang et al., 2020). The investigation of cooling effect of urban green spaces in different seasons can provide more information for the design of UGS patches. Urban morphology/geometry parameters such as sky view factor, aspect ratio, building and tree height, vegetation structure or stratification, orientation, altitude may also influence the thermal environment in cities (Irger, 2014; Morakinyo et al., 2020). They affect air circulation, heat dissipation, and thermal energy absorption in open spaces and urban canyons (Morakinyo et al., 2017; Ahmadi Venhari et al., 2019; Bartesaghi-Koc et al., 2022). A comprehensive investigation of urban and green space characteristics on urban thermal environment will be helpful to the planning of urban spaces to mitigate heat and improve thermal environment of cities in future studies.
Conclusion
In this study, ESTARFM data fusion method was applied to produce enhanced LST data for SUHI analysis the tropical megacities. Impervious surface has increased by 30%–40% and bare land and green space have decreased by 20%–40% in the megacities from 2000 to 2020. The average SUHI intensity increased by 0.98°C, 1.42°C, and 0.73°C in Dhaka, Kolkata, and Bangkok, respectively in last 2 decades. The urban cooling effects analysis results indicate that the maximum intensity of urban cooling island ranges from 4.83°C in Bangkok to 8.07°C in Kolkata, and the maximum range of urban cooling island varies from 300 m in Bangkok to 420 m in Kolkata. The optimal patch size of green space is 0.37 ha, 0.77 ha, and 0.42 ha in Dhaka, Kolkata and Bangkok, respectively. UCI intensity is negatively correlated with P_LST and positively correlated with P_NDVI and P_Area, while UCI range was correlated negatively with P_LST and positively correlated with P_Area. The optimal size of green space patches is larger in cities with higher background temperature. These results provide valuable information for the scientific planning of urban green space to produce effective cooling effects. Comprehensive investigation of urban characteristics on thermal environment can be performed for heat mitigation and adaptation in future studies.
Data availability statement
The raw data supporting the conclusion of this article will be made available by the authors, without undue reservation.
Author contributions
All authors listed have made a substantial, direct, and intellectual contribution to the work and approved it for publication.
Funding
This research was funded by the Director Fund of the International Research Center of Big Data for Sustainable Development Goals (grant number CBAS2022DF016); and the National Natural Science Foundation of China (grant number 42071321).
Acknowledgments
The authors appreciate the comments and suggestions from the editors and reviewers.
Conflict of interest
The authors declare that the research was conducted in the absence of any commercial or financial relationships that could be construed as a potential conflict of interest.
The reviewer XS declared a shared affiliation with the authors except for ZF to the handling editor at the time of review.
Publisher’s note
All claims expressed in this article are solely those of the authors and do not necessarily represent those of their affiliated organizations, or those of the publisher, the editors and the reviewers. Any product that may be evaluated in this article, or claim that may be made by its manufacturer, is not guaranteed or endorsed by the publisher.
References
Ahmadi Venhari, A., Tenpierik, M., and Taleghani, M. (2019). The role of sky view factor and urban street greenery in human thermal comfort and heat stress in a desert climate. J. Arid Environ. 166, 68–76. doi:10.1016/j.jaridenv.2019.04.009
Akbari, H., and Kolokotsa, D. (2016). Three decades of urban heat islands and mitigation technologies research. Energy Build. 133, 834–842. doi:10.1016/j.enbuild.2016.09.067
Algretawee, H. (2022). The effect of graduated urban park size on park cooling island and distance relative to land surface temperature (LST). Urban Clim. 45, 101255. doi:10.1016/j.uclim.2022.101255
Aram, F., García, E. H., Solgi, E., and Mansournia, S. (2019). Urban green space cooling effect in cities. Heliyon 5 (4), e01339. No. doi:10.1016/j.heliyon.2019.e01339
Arnfield, A. J. (2003). Two decades of urban climate research: A review of turbulence, exchanges of energy and water, and the urban heat island. Int. J. Climatol. 23 (1), 1–26. doi:10.1002/joc.859
Baqa, M. F., Lu, L., Chen, F., Nawaz-ul-Huda, S., Pan, L., Tariq, A., et al. (2022). Characterizing spatiotemporal variations in the urban thermal environment related to land cover changes in karachi, Pakistan, from 2000 to 2020. Remote Sens. 14 (9), 2164. doi:10.3390/rs14092164
Bartesaghi-Koc, C., Osmond, P., and Peters, A. (2018). Evaluating the cooling effects of green infrastructure: A systematic review of methods, indicators and data sources. Sol. Energy 166, 486–508. doi:10.1016/j.solener.2018.03.008
Bartesaghi-Koc, C., Osmond, P., and Peters, A. (2022). Innovative use of spatial regression models to predict the effects of green infrastructure on land surface temperatures. Energy Build. 254, 111564. doi:10.1016/j.enbuild.2021.111564
Bechtel, B., Demuzere, M., Mills, G., Zhan, W., Sismanidis, P., Small, C., et al. (2019). SUHI analysis using local climate zones—a comparison of 50 cities. Urban Clim. 28, 100451. doi:10.1016/j.uclim.2019.01.005
Beck, H. E., Zimmermann, N. E., McVicar, T. R., Vergopolan, N., Berg, A., and Wood, E. F. (2018). Present and future Köppen-Geiger climate classification maps at 1-km resolution. Sci. Data 5 (1), 180214. doi:10.1038/sdata.2018.214
Coleman, J. (2022). Climate change made South Asian heatwave 30 times more likely. Nature 606. doi:10.1038/d41586-022-01444-1
Das, P., Vamsi, K. S., and Zhenke, Z. (2020). Decadal variation of the land surface temperatures (LST) and urban heat island (UHI) over Kolkata city projected using MODIS and ERA-interim DataSets. Aerosol Sci. Eng. 4 (3), 200–209. doi:10.1007/s41810-020-00067-1
Derkzen, M. L., van Teeffelen, A. J. A., and Verburg, P. H. (2017). Green infrastructure for urban climate adaptation: How do residents’ views on climate impacts and green infrastructure shape adaptation preferences? Landsc. Urban Plan. 157, 106–130. doi:10.1016/j.landurbplan.2016.05.027
Doick, K. J., Peace, A., and Hutchings, T. R. (2014). The role of one large greenspace in mitigating London's nocturnal urban heat island. Sci. Total Environ. 493, 662–671. doi:10.1016/j.scitotenv.2014.06.048
Duan, S., Ru, C., Li, Z., Wang, M., Xu, Q., Li, H., et al. (2021). Reviews of methods for land surface temperature retrieval from Landsat thermal infrared data. J. Remote Sens. 25 (8), 1591–1617. doi:10.11834/jrs.20211296
Estoque, R. C., and Murayama, Y. (2017). Monitoring surface urban heat island formation in a tropical mountain city using Landsat data (1987–2015). ISPRS J. Photogrammetry Remote Sens. 133, 18–29. doi:10.1016/j.isprsjprs.2017.09.008
Estoque, R. C., Murayama, Y., and Myint, S. W. (2017). Effects of landscape composition and pattern on land surface temperature: An urban heat island study in the megacities of Southeast Asia. Sci. Total Environ. 577, 349–359. doi:10.1016/j.scitotenv.2016.10.195
Fan, H., Yu, Z., Yang, G., Liu, T. Y., Liu, T. Y., Hung, C. H., et al. (2019). How to cool hot-humid (asian) cities with urban trees? An optimal landscape size perspective. Agric. For. Meteorology 265, 338–348. doi:10.1016/j.agrformet.2018.11.027
Feyisa, G. L., Dons, K., and Meilby, H. (2014). Efficiency of parks in mitigating urban heat island effect: An example from Addis Ababa. Landsc. Urban Plan. 123, 87–95. doi:10.1016/j.landurbplan.2013.12.008
Gao, F., Masek, J., Schwaller, M., and Hall, F. (2006). On the blending of the Landsat and MODIS surface reflectance: Predicting daily Landsat surface reflectance. IEEE Trans. Geosci. Remote Sens. 44 (8), 2207–2218. doi:10.1109/TGRS.2006.872081
Geng, X., Yu, Z., Zhang, D., Li, C., Yuan, Y., and Wang, X. (2022). The influence of local background climate on the dominant factors and threshold-size of the cooling effect of urban parks. Sci. Total Environ. 823, 153806. doi:10.1016/j.scitotenv.2022.153806
Gillner, S., Vogt, J., Tharang, A., Dettmann, S., and Roloff, A. (2015). Role of street trees in mitigating effects of heat and drought at highly sealed urban sites. Landsc. Urban Plan. 143, 33–42. doi:10.1016/j.landurbplan.2015.06.005
Girardet, H. (2020). People and nature in an urban world. One Earth 2 (2), 135–137. doi:10.1016/j.oneear.2020.02.005
Giridharan, R., and Emmanuel, R. (2018). The impact of urban compactness, comfort strategies and energy consumption on tropical urban heat island intensity: A review. Sustain. Cities Soc. 40, 677–687. doi:10.1016/j.scs.2018.01.024
Halder, B., Bandyopadhyay, J., and Banik, P. (2021). Monitoring the effect of urban development on urban heat island based on remote sensing and geo-spatial approach in Kolkata and adjacent areas, India. Sustain. Cities Soc. 74, 103186. doi:10.1016/j.scs.2021.103186
Hami, A., Abdi, B., Zarehaghi, D., and Maulan, S. B. (2019). Assessing the thermal comfort effects of green spaces: A systematic review of methods, parameters, and plants’ attributes. Sustain. Cities Soc. 49, 101634. doi:10.1016/j.scs.2019.101634
Hassan, T., Zhang, J., Prodhan, F. A., Pangali Sharma, T. P., and Bashir, B. (2021). Surface urban heat islands dynamics in response to LULC and vegetation across South Asia (2000–2019). Remote Sens. 13 (16), 3177. doi:10.3390/rs13163177
Hathway, E. A., and Sharples, S. (2012). The interaction of rivers and urban form in mitigating the urban heat island effect: A UK case study. Build. Environ. 58, 14–22. doi:10.1016/j.buildenv.2012.06.013
Irger, M. (2014). “The effect of urban form on urban microclimate,” (Sydney: UNSW) (Doctoral dissertation.
Khamchiangta, D., and Dhakal, S. (2019). Physical and non-physical factors driving urban heat island: Case of Bangkok Metropolitan Administration, Thailand. J. Environ. Manag. 248, 109285. doi:10.1016/j.jenvman.2019.109285
Lin, W., Yu, T., Chang, X., Wu, W., and Zhang, Y. (2015). Calculating cooling extents of green parks using remote sensing: Method and test. Landsc. Urban Plan. 134, 66–75. doi:10.1016/j.landurbplan.2014.10.012
Liu, M., Liu, X., Dong, X., Zhao, B., Zou, X., Wu, L., et al. (2020a). An improved spatiotemporal data fusion method using surface heterogeneity information based on ESTARFM. Remote Sens. 12 (21), 3673. doi:10.3390/rs12213673
Liu, X., Zhou, Y., Yue, W., Li, X., Liu, Y., and Lu, D. (2020b). Spatiotemporal patterns of summer urban heat island in Beijing, China using an improved land surface temperature. J. Clean. Prod. 257, 120529. doi:10.1016/j.jclepro.2020.120529
Lu, L., Kuenzer, C., Wang, C., Guo, H., and Li, Q. (2015). Evaluation of three MODIS-derived vegetation index time series for dryland vegetation dynamics monitoring. Remote Sens. 7 (6), 7597–7614. doi:10.3390/rs70607597
Lu, L., Qureshi, S., Li, Q., Chen, F., and Shu, L. (2022). Monitoring and projecting sustainable transitions in urban land use using remote sensing and scenario-based modelling in a coastal megacity. Ocean Coast. Manag. 224, 106201. doi:10.1016/j.ocecoaman.2022.106201
Lu, L., Weng, Q., Guo, H., Feng, S., and Li, Q. (2019). Assessment of urban environmental change using multi-source remote sensing time series (2000–2016): A comparative analysis in selected megacities in eurasia. Sci. Total Environ. 684, 567–577. doi:10.1016/j.scitotenv.2019.05.344
Lu, L., Weng, Q., Xiao, D., Guo, H., Li, Q., and Hui, W. (2020). Spatiotemporal variation of surface urban heat islands in relation to land cover composition and configuration: A multi-scale case study of xi’an, China. Remote Sens. 12 (17), 2713. doi:10.3390/rs12172713
Morakinyo, T. E., Kong, L., Lau, K. K.-L., Yuan, C., and Ng, E. (2017). A study on the impact of shadow-cast and tree species on in-canyon and neighborhood's thermal comfort. Build. Environ. 115, 1–17. doi:10.1016/j.buildenv.2017.01.005
Morakinyo, T. E., Ouyang, W., Lau, K. K.-L., Ren, C., and Ng, E. (2020). Right tree, right place (urban canyon): Tree species selection approach for optimum urban heat mitigation - development and evaluation. Sci. Total Environ. 719, 137461. doi:10.1016/j.scitotenv.2020.137461
Murakawa, S., Sekine, T., Narita, K.-i., and Nishina, D. (1991). Study of the effects of a river on the thermal environment in an urban area. Energy Build. 16 (3), 993–1001. doi:10.1016/0378-7788(91)90094-J
Nath, S. K., Adhikari, M. D., Devaraj, N., and Maiti, S. K. (2015). Seismic vulnerability and risk assessment of Kolkata City, India. Nat. Hazards Earth Syst. Sci. 15 (6), 1103–1121. doi:10.5194/nhess-15-1103-2015
Oke, T. R. (1973). City size and the urban heat island. Atmos. Environ. 7 (8), 769–779. doi:10.1016/0004-6981(73)90140-6
Oliveira, S., Andrade, H., and Vaz, T. (2011). The cooling effect of green spaces as a contribution to the mitigation of urban heat: A case study in lisbon. Build. Environ. 46 (11), 2186–2194. doi:10.1016/j.buildenv.2011.04.034
Parvin, N. S., and Abudu, D. (2017). Estimating urban heat island intensity using remote sensing techniques in Dhaka city. Int. J. Sci. Eng. Res. 8, 289–298. doi:10.14299/ijser.2017.04.009
Shah, A., Garg, A., and Mishra, V. (2021). Quantifying the local cooling effects of urban green spaces: Evidence from Bengaluru, India. Landsc. Urban Plan. 209, 104043. doi:10.1016/j.landurbplan.2021.104043
Shashua-Bar, L., and Hoffman, M. E. (2000). Vegetation as a climatic component in the design of an urban street: An empirical model for predicting the cooling effect of urban green areas with trees. Energy Build. 31 (3), 221–235. doi:10.1016/S0378-7788(99)00018-3
Si, M., Li, Z., Nerry, F., Tang, B., Leng, P., Wu, H., et al. (2022). Spatiotemporal pattern and long-term trend of global surface urban heat islands characterized by dynamic urban-extent method and MODIS data. ISPRS J. Photogrammetry Remote Sens. 183, 321–335. doi:10.1016/j.isprsjprs.2021.11.017
Sobrino, J. A., Jimenez-Munoz, J. C., Soria, G., Romaguera, M., Guanter, L., Moreno, J., et al. (2008). Land surface emissivity retrieval from different VNIR and TIR sensors. IEEE Trans. Geosci. Remote Sens. 46 (2), 316–327. doi:10.1109/TGRS.2007.904834
Stone, B., Vargo, J., Liu, P., Habeeb, D., DeLucia, A., Trail, M., et al. (2014). Avoided heat-related mortality through climate adaptation strategies in three US cities. PLoS One 9 (6), e100852. doi:10.1371/journal.pone.0100852
Sun, R., and Chen, L. (2017). Effects of green space dynamics on urban heat islands: Mitigation and diversification. Ecosyst. Serv. 23, 38–46. doi:10.1016/j.ecoser.2016.11.011
Tan, X., Sun, X., Huang, C., Yuan, Y., and Hou, D. (2021). Comparison of cooling effect between green space and water body. Sustain. Cities Soc. 67, 102711. doi:10.1016/j.scs.2021.102711
Uddin, A. S. M. S., Khan, N., Islam, A. R. M. T., Kamruzzaman, M., and Shahid, S. (2022). Changes in urbanization and urban heat island effect in Dhaka city. Theor. Appl. Climatol. 147 (3), 891–907. doi:10.1007/s00704-021-03872-x
United Nations Department of Economic and Social Affairs, Population Division (2019). World urbanization prospects:the 2018 revision. New York, NY, USA: United Nations.
United Nations (2015). Transforming our world:the 2030 agenda for sustainable development. New York, NY, USA: United Nations.
Vaz Monteiro, M., Doick, K. J., Handley, P., and Peace, A. (2016). The impact of greenspace size on the extent of local nocturnal air temperature cooling in London. Urban For. Urban Green. 16, 160–169. doi:10.1016/j.ufug.2016.02.008
Wang, Y., Wang, A., Zhai, J., Tao, H., Jiang, T., Su, B., et al. (2019). Tens of thousands additional deaths annually in cities of China between 1.5 °C and 2.0 °C warming. Nat. Commun. 10 (1), 3376. doi:10.1038/s41467-019-11283-w
Weng, Q., Fu, P., and Gao, F. (2014). Generating daily land surface temperature at Landsat resolution by fusing Landsat and MODIS data. Remote Sens. Environ. 145, 55–67. doi:10.1016/j.rse.2014.02.003
Weng, Q. (2009). Thermal infrared remote sensing for urban climate and environmental studies: Methods, applications, and trends. ISPRS J. Photogrammetry Remote Sens. 64 (4), 335–344. doi:10.1016/j.isprsjprs.2009.03.007
Yang, G., Yu, Z., Jørgensen, G., and Vejre, H. (2020). How can urban blue-green space be planned for climate adaption in high-latitude cities? A seasonal perspective. Sustain. Cities Soc. 53, 101932. doi:10.1016/j.scs.2019.101932
Yin, S., Peng, L. L. H., Feng, N., Wen, H., Ling, Z., Yang, X., et al. (2022). Spatial-temporal pattern in the cooling effect of a large urban forest and the factors driving it. Build. Environ. 209, 108676. doi:10.1016/j.buildenv.2021.108676
Yu, Z., Guo, X., Jørgensen, G., and Vejre, H. (2017). How can urban green spaces be planned for climate adaptation in subtropical cities? Ecol. Indic. 82, 152–162. doi:10.1016/j.ecolind.2017.07.002
Yu, Z., Guo, X., Zeng, Y., Koga, M., and Vejre, H. (2018a). Variations in land surface temperature and cooling efficiency of green space in rapid urbanization: The case of Fuzhou city, China. Urban For. Urban Green. 29, 113–121. doi:10.1016/j.ufug.2017.11.008
Yu, Z., Xu, S., Zhang, Y., Jørgensen, G., and Vejre, H. (2018b). Strong contributions of local background climate to the cooling effect of urban green vegetation. Sci. Rep. 8 (1), 6798. doi:10.1038/s41598-018-25296-w
Yu, Z., Yang, G., Zuo, S., Jørgensen, G., Koga, M., and Vejre, H. (2020). Critical review on the cooling effect of urban blue-green space: A threshold-size perspective. Urban For. Urban Green. 49, 126630. doi:10.1016/j.ufug.2020.126630
Yuan, H., Wu, C., Lu, L., and Wang, X. (2018). A new algorithm predicting the end of growth at five evergreen conifer forests based on nighttime temperature and the enhanced vegetation index. ISPRS J. Photogrammetry Remote Sens. 144, 390–399. doi:10.1016/j.isprsjprs.2018.08.013
Zhao, L., Lee, X., Smith, R. B., and Oleson, K. (2014). Strong contributions of local background climate to urban heat islands. Nature 511 (7508), 216–219. doi:10.1038/nature13462
Zhou, W., Wang, J., and Cadenasso, M. L. (2017). Effects of the spatial configuration of trees on urban heat mitigation: A comparative study. Remote Sens. Environ. 195, 1–12. doi:10.1016/j.rse.2017.03.043
Zhou, W., Yu, W., and Wu, T. (2022). An alternative method of developing landscape strategies for urban cooling: A threshold-based perspective. Landsc. Urban Plan. 225, 104449. doi:10.1016/j.landurbplan.2022.104449
Keywords: urban heat island, green space, threshold value of efficiency, cooling intensity, megacity, sustainable development goals
Citation: Li C, Lu L, Fu Z, Sun R, Pan L, Han L, Guo H and Li Q (2022) Diverse cooling effects of green space on urban heat island in tropical megacities. Front. Environ. Sci. 10:1073914. doi: 10.3389/fenvs.2022.1073914
Received: 19 October 2022; Accepted: 14 November 2022;
Published: 24 November 2022.
Edited by:
Zhaowu Yu, Fudan University, ChinaReviewed by:
Chunlei Meng, Chinese Academy of Forestry, ChinaChaoyang Wu, Chinese Academy of Forestry, China
Xiaoning Song, University of Chinese Academy of Sciences, China
Copyright © 2022 Li, Lu, Fu, Sun, Pan, Han, Guo and Li. This is an open-access article distributed under the terms of the Creative Commons Attribution License (CC BY). The use, distribution or reproduction in other forums is permitted, provided the original author(s) and the copyright owner(s) are credited and that the original publication in this journal is cited, in accordance with accepted academic practice. No use, distribution or reproduction is permitted which does not comply with these terms.
*Correspondence: Linlin Lu, bHVsbEByYWRpLmFjLmNu