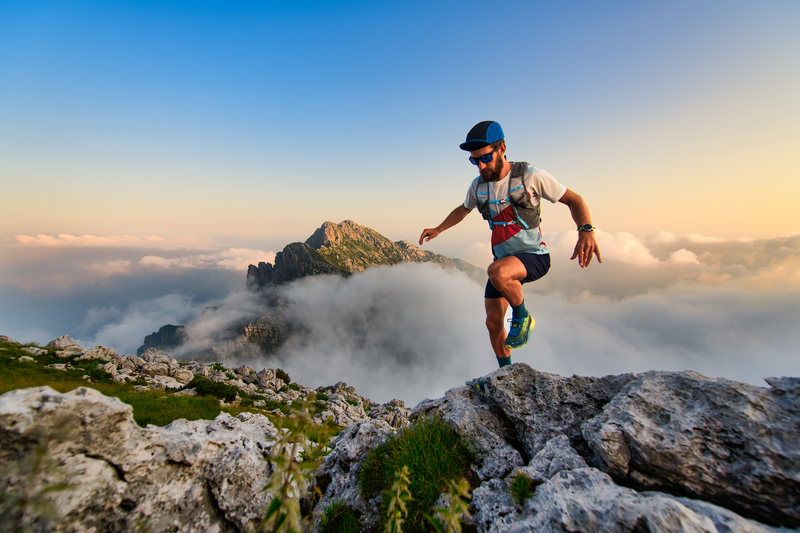
95% of researchers rate our articles as excellent or good
Learn more about the work of our research integrity team to safeguard the quality of each article we publish.
Find out more
ORIGINAL RESEARCH article
Front. Environ. Sci. , 25 November 2022
Sec. Atmosphere and Climate
Volume 10 - 2022 | https://doi.org/10.3389/fenvs.2022.1068861
This article is part of the Research Topic Physical and Chemical Processes within the Planetary Boundary Layer and Their Impacts on Air Pollution View all 12 articles
Based on the current air pollution control and CO2 emission reduction policies, this study analyzed the energy structure, number of motor vehicles and nonroad mobile machinery, energy consumption and pollutant emissions in Beijing. Furthermore, the diesel consumption characteristics and challenges for emission reduction in key fields were investigated, such as medium- and heavy-duty diesel trucks, long-distance passenger and tourist diesel vehicles, and nonroad machinery, which are areas with difficult-to-reduce diesel consumption. Control targets and measures for total diesel consumption were also proposed. The results indicated that the higher diesel consumption per unit area in Beijing is related to the larger passenger car and freight truck populations. In recent decades, the number of diesel vehicles has increased, the vehicle type structure has been optimized, the proportion of vehicles with high emission standards has increased, and the absolute pollutant emissions have decreased. Among these, nitrogen oxides (NOx), fine particulate matter (PM2.5) and volatile organic compound (VOC) emissions of different models decreased by 39.5%, 75.3% and 42.8%, respectively, while carbon dioxide (CO2) emissions from diesel combustion decreased by 32%. Moreover, medium and large passenger vehicles, medium- and heavy-duty trucks and construction machinery are the main contributors to diesel consumption. These vehicle types are also difficult to control and reduce, and their replacement by new-energy vehicles is relatively limited. The main control measures for diesel consumption are as follows. First, a green transportation mode can be adopted for goods that can be converted from roads to railways. Second, fuel consumption reduction for nonroad mobile machinery can be realized by tightening fuel consumption limits, setting appropriate maximum retirement life, establishing low- or ultralow-emission zones, and establishing demonstration plots for electric vehicle (EV) substitution for mobile machinery. To improve the air quality and take the lead in carbon neutrality in the future, Beijing must further accelerate the energy structure adjustment and the development of new-energy vehicles in the transportation sector. Carbon neutralization is an important opportunity for diesel consumption reduction, and the synergistic control of atmospheric pollution and carbon emissions from diesel combustion must be strengthened.
After years of efforts, the total emissions of motor vehicles and nonroad mobile machinery in Beijing continue to decline (Fu et al., 2012; Yao et al., 2014; Zhang et al., 2014; Lv et al., 2016). However, due to the high motor vehicle population, overall emissions remain very high, and the proportion of motor vehicles and nonroad mobile machinery emissions in local pollution sources continues to rise (Song et al., 2006; Lee et al., 2008; Ni et al., 2017; Wu et al., 2022). In 2018, source apportionment of fine particulate matter (PM2.5) showed that the contribution of mobile-source emissions in the local PM2.5 pollution reached 45%, the largest source of local PM2.5 in Beijing. Therefore, the task of emission management and reduction remains arduous (Zhang et al., 2017; Fan et al., 2018; Gao et al., 2020; Zhang et al., 2020).
Diesel vehicles in Beijing account for only 5% of all vehicles, but single-vehicle emissions are relatively high. The nitrogen oxides (NOx) emissions of diesel vehicles account for approximately 80% of the total NOx emissions from motor vehicles (Xue et al., 2022); therefore, diesel vehicles are a significant source of NOx emissions. Beijing’s 2019 Action Plan for Pollution Prevention and Control proposes promoting the control and reduction of total diesel consumption by vehicles in Beijing and accelerating the construction of an indicator system of statistics, monitoring, and total reduction for diesel consumption by vehicles in public transport, tourism, freight transport, sanitation, and postal services.
Therefore, in this study, based on Beijing registration data, the emission factor method is used to calculate the emissions of air pollutants from mobile sources, and carbon dioxide (CO2) emissions are measured according to the CO2 emission accounting guidelines. Additionally, the existing policies related to the pollution control of diesel vehicles in Beijing are evaluated. Pollution emission control measures are proposed, considering air quality improvement, emission reduction needs, diesel consumption characteristics, and existing problems in emission reduction.
In this study, through data collection, investigation and expert consultations, the current diesel consumption and carbon emission reduction policies in Beijing are reviewed; fuel reduction measures for medium- and heavy-duty diesel trucks, long-distance passenger transport and tourist vehicles, and nonroad mobile machinery are studied; control targets for total diesel consumption are proposed; and the impact on air quality is analyzed.
The data include the number of various motor vehicles, the types and number of nonroad mobile machinery units, and energy data. The number of motor vehicles is obtained from the Beijing Traffic Management Bureau, the types and number of nonroad mobile machinery units are based on the Beijing nonroad mobile machinery information code registration data, and the energy data are obtained from the Statistical Yearbook released by the Beijing Municipal Bureau of Statistics. (as can be seen in Table 1).
The air pollutant emissions of diesel vehicles in Beijing are calculated based on registration data, road length, and the emission factor method (Gu et al., 2019; Hao et al., 2022).
where E1 is the annual emission of pollutants corresponding to vehicle emission source i, in tonnes; EFi is the emission factor, which is the amount of pollutants emitted by type i diesel vehicles per kilometer, in g/km; Pi is the number of registered diesel vehicles in Beijing; and VKTi is the average annual mileage of type i diesel vehicles, in km/vehicle.
For construction machinery and agricultural machinery, emissions are calculated by using the emission factor based on the mechanical power with the following formula:
where E is the emission of various pollutants from nonroad mobile machinery, in tonnes; j is the type of nonroad mobile machinery; k is the emission standard; n is the power range; P is the number of nonroad mobile machinery units registered in Beijing, with vehicle as the unit; G is the average rated net power, in kilowatts/vehicle; LF is the load factor; hr is the total hours of use per year, in hours; EF is the pollutant emission factor, in g/kWh, and the mobile source survey method and emission coefficient in the Second National Pollution Source Census in China are applied.
CO2 emissions include emissions from terminal coal consumption, terminal fuel consumption, terminal gas consumption, and regional electricity consumption.
where i refers to the various energy types, such as coal, fossil fuel and gas. Ei represents CO2 emissions from type i. EFi represents the emission factor of type i, as shown in Table 2, and the EFs of coal, fossil fuel and gas were 2.66, 1.73, and 1.56 tonnes of CO2 per ton of standard coal, respectively (NDRC Energy Research Institute, 2011). The local power grid emission factor is 0.6168 CO2 emissions (kgCO2/kWh).
The ADMS-Urban model is used to quantify the impact of air pollutant emissions from vehicles on the surrounding atmospheric environment; it is a steady-state atmospheric dispersion modeling system developed by Cambridge Environmental Research Consultants (CERC). Based on atmospheric boundary layer parameters, the ADMS-Urban model can better handle the complex topography and wind conditions of megacities (Righi et al., 2009; Audrius and Auksė, 2015). First, the traffic flow coefficients are assigned to roads of different levels, and a 1 km × 1 km grid is used for Beijing. Second, the data mentioned above are used to calculate the emissions of air pollutants in each grid, and the dispersion concentration of air pollutants in Beijing throughout the year is then simulated to obtain the dispersion concentration map of various pollutants. At present, ADMS-Urban is widely used and can accurately simulate the diffusion of traffic pollutants. The uncertainty of its simulation results is related mainly to meteorological conditions. Generally, when wind speed or direction changes sharply, the simulation value of ADMS-Urban is lower than the actual monitoring value, while when weather conditions are relatively stable, the simulation results are more accurate.
The total amount of various pollutants, meteorological data, typical road vehicle flow data, and model parameters are imported into the model. In the simulation of pollutant dispersion in the Beijing area, the total pollutant data for Beijing are used, and the data are gridded for the dispersion simulation. The data source is based on the amount of pollutant emissions from vehicles in Beijing. The meteorological data required for the model simulations are from the China Meteorological Data Service Centre (http://data.cma.cn), and the model defaults are used for other data, such as road slopes and loads.
Figure 1 shows the changes in diesel vehicles and diesel consumption in Beijing from 2013 to 2020. The overall diesel consumption from 2013 to 2020 decreased, with interannual fluctuations. In 2020, the diesel consumption of diesel vehicles in Beijing was 0.90 million tonnes, which is significantly lower than that in 2019 and is related mainly to the decline in passenger and freight traffic due to the coronavirus 2019 (COVID-19) pandemic. In the long term, diesel consumption also declined by 41% from 1.51 million tonnes in 2013 as a result of the adjustment of the diesel vehicle structure, i.e., new diesel vehicles replacing old ones, since the fuel consumption of new diesel vehicles is significantly lower. Fuel consumption limits for heavy-duty commercial vehicles were implemented in 2014 (GB 30510-2014) and 2018 (GB 30510-2018), clearly and effectively reducing the fuel consumption of new vehicles. In the second year after implementation, i.e., 2015 and 2019, diesel consumption in Beijing was greatly reduced.
As shown in Figure 2, the changes and proportions of air pollutant emissions from diesel vehicles in Beijing show that from 2013 to 2020, the adjustment and optimization of vehicle structure caused declines of 39.5%, 75.3%, and 42.8% in the NOx, PM2.5, and volatile organic compound (VOC) emissions, respectively, of different vehicle types, with PM2.5 decreasing most significantly. Large diesel passenger cars and trucks contributed significantly to NOx and PM2.5. There were also significant differences in the contributions of vehicles with different emission standards. The ownership of medium-sized diesel trucks meeting China III emission levels accounts for 37%, contributing 46% of NOx and 45% of PM2.5 emissions; the ownership of heavy-duty diesel trucks meeting China III emission standards accounts for 11%, contributing 15% of NOx emissions and 13% of PM2.5 emissions. The emissions of pollutants from vehicles at or below China III emission standards were also relatively high, and their percentage of pollutant emissions exceeded the proportion of vehicles. It is necessary to further promote the adoption of measures to ensure that the emission standards are met and to accelerate the elimination and updating of these vehicles.
According to the corresponding assessment criteria of the Work Plan for the Evaluation of the Responsibility of Energy Saving and Carbon Reduction Targets in Beijing during the 13th Five-Year Plan Period, the annual CO2 emissions during 2013–2020 in Beijing are calculated as shown in Figure 3. CO2 emissions peaked at 151 million tonnes in 2019, followed by a plateau period. Thanks to the large-scale coal-to-clean energy policy, CO2 emissions declined from 2015 to 2017, followed by a slight rebound from 2018 to 2019. The proportion of CO2 emissions from coal continuously declined, the proportion of emissions from electricity significantly increased, and the proportion of emissions from fossil fuel remained stable.
CO2 emissions from diesel combustion decreased from 3.85 million tonnes in 2013 to 2.24 million tonnes in 2020, a reduction of 41%. The contribution of diesel combustion to total CO2 emissions decreased from 3% in 2013 to 2% in 2020 (as can be seen in Figure 4). At present, some road transport enterprises are included in the list of key carbon emission units. For the future prevention and control of air pollution from road transport vehicles, more road transport enterprises, especially those with numerous medium- and heavy-duty trucks and medium and large passenger vehicles, should be highlighted based on the current carbon emission management mechanism.
FIGURE 4. Changes in CO2 emissions from diesel combustion and the proportion of CO2 emissions from diesel combustion in Beijing.
Based on the actual situation in Beijing, the total amount of diesel should be set, and the diesel consumption in key industries should be controlled, including road transportation (passenger and freight transport), the construction industry, and the building materials industry, which are best combined with key carbon emission units (Jiang et al., 2008; Kim et al., 2010). The annual diesel consumption reduction targets of each industry should be reasonably allocated, and diesel consumption statistics should be collected and monitored. Based on publicity and policy implementation, key diesel vehicle users should be guided to increase their awareness of energy conservation and emissions reduction, to actively update and eliminate low-standard diesel vehicles and machinery with high energy consumption and emissions levels, and to gradually upgrade to new standards or new-energy vehicles and machinery (Axsen and Kurani, 2010; Bessa and Matos, 2012; Yang et al., 2018).
The control target of total diesel consumption is closely related to the targets of air quality improvement and carbon neutrality in Beijing. In 2020, the concentration of PM2.5 in Beijing decreased to 38 μg/m3 for the first time, which is related to the decrease in the activity level of pollution sources caused by COVID-19 (Cao et al., 2021). This decrease is also an environmental benefit of air pollution control measures. To achieve air quality standards and meet the World Health Organization (WHO)’s recommended health limits for PM2.5 in the future, there is still a need to strengthen air pollution control, especially concerning the continuous reduction of emissions from mobile pollution sources. Therefore, based on future air quality improvement targets in Beijing (low, medium, and high PM2.5 concentration scenarios will be 25, 20, and 15 μg/m3, respectively, in 2035), reductions of at least 40%, 45%, and 50% in the low, medium, and high gasoline and diesel consumption scenarios, respectively, in Beijing should be met by 2035 (Figure 5A).
To meet the target of carbon neutrality by 2050 and the carbon reduction target of halving greenhouse gas emissions by 2035, reductions of at least 45%, 50%, and 60% in the low, medium, and high diesel consumption scenarios or gasoline and diesel consumption scenarios, respectively, in Beijing should be met by 2035 (Figure 5B).
For major diesel vehicle types, there should be no diesel consumption by small passenger vehicles by 2035, by which time all buses should also be electric vehicles (EVs). The development of new-energy tourist buses and medium and large buses can be accelerated by the establishment of a zero-emission zone in the city center to increase the proportion of these vehicles. Light trucks are being phased out naturally, and the economic stimulus for accelerating the phaseout and renewal of older vehicles and policies facilitating access to new-energy vehicles could increase the proportions of the latter. Due to the implementation of the new energy development strategy for medium- and heavy-duty trucks, technological progress and lower production costs, and the anticipation of a ban on the sale of traditional-fuel vehicles, the adoption of new-energy medium- and heavy-duty trucks could be accelerated. Based on the bottom-up measures for key diesel vehicles, it is estimated that diesel consumption in the low, medium, and high scenarios in 2035 could be reduced, respectively, by 54%, 62%, and 69% (Figure 6) compared to 2020, which would essentially meet Beijing’s carbon emission reduction targets.
The ADMS-Urban model is used to simulate the effects of pollution emissions from diesel combustion on primary PM2.5, VOC, and NOx concentrations in 2020 and the decreases in major atmospheric pollutant concentrations due to reduced diesel consumption under different control scenarios in 2035.
The impact of diesel vehicles on the surrounding air quality in Beijing in 2020 is obtained through simulation, as shown in Figure 7. Five traffic stations are selected as sensitive receptors, and the annual average concentrations of NOx, PM2.5, and VOCs are 17.6, 0.4 and 0.8 μg/m3, respectively.
FIGURE 7. Impacts of diesel vehicles in Beijing in 2020 (A) and high- (B), medium- (C), and low-control scenarios (D) in 2035 on air quality.
Under the low diesel reduction scenario, the annual average concentrations of the three major atmospheric pollutants due to diesel vehicles are reduced by approximately 53%, and the annual average concentrations of NOx, PM2.5, and VOCs at the five traffic stations with sensitive receptors are 10.51 μg/m3, 0.14 μg/m3 and 0.48 μg/m3, respectively. Under the medium diesel reduction scenario, the annual average concentrations of the three major atmospheric pollutants due to diesel vehicles are reduced by approximately 65%, and the annual average concentrations of NOx, PM2.5, and VOCs at the five traffic stations with sensitive receptors are 6.69 μg/m3, 0.1 μg/m3, and 0.32 μg/m3, respectively.
Under the high diesel reduction scenario, the annual average concentrations of the three major atmospheric pollutants due to diesel vehicles are reduced by approximately 74%, and the annual average concentrations of NOx, PM2.5, and VOCs at the five traffic stations with sensitive receptors are 2.26 μg/m3, 0.07 μg/m3, and 0.24 μg/m3, respectively.
To reduce the pollution emissions from diesel vehicles, over the past decade, Beijing has actively adopted legislation (Beijing Regulations on the Prevention and Control of Emissions from Motor Vehicles and Nonroad Mobile Machinery), upgraded diesel vehicle and oil product standards, implemented a subsidy policy for the elimination of old diesel vehicles, and strengthened emission supervision and law enforcement (online supervision, remote sensing monitoring, etc.). These measures and policies have effectively promoted the adjustment and optimization of the diesel vehicle structure in Beijing, and the proportion of vehicles with high emission scores has continuously increased. Some key models of new energy have been well developed, especially in the light truck and bus industries. However, several problems remain: (1) It is necessary to further optimize the traffic structure and increase the proportion of railway transport. (2) The efficiency of transport should be further improved. The current transport intensity is still high. (3) The low level of new-energy development of medium-heavy goods vehicles and buses is insufficient to support the realization of higher air quality improvement and carbon neutrality goals.
In addition to ensuring major livelihood, emergency, and special scenarios, policies should be developed starting from the licensing process to ensure that new and updated light trucks are essentially new-energy vehicles. A series of incentive plans for new-energy trucks in Beijing should be formulated to expand the incentive range from light trucks under 4.5 tonnes to medium- and heavy-duty trucks. A series of priority passage policies for new-energy logistics distribution vehicles should be formulated and implemented, and all vehicles with truck passes should be purely EVs or hydrogen fuel cell vehicles, thus promoting EVs through policies that begin with licensing and use processes.
The current policies and measures should be continued, including the subsidy for the elimination of gasoline vehicles meeting the China III emission standard, the ban on gasoline vehicles meeting China I and II emission standards within the Fifth Ring Road during weekdays, and the city-wide ban on diesel trucks meeting the China III emission standard. Additionally, phaseout subsidies and restrictions on vehicles with higher emission standards and more vehicle models should be studied. In 2022, light gasoline vehicles meeting the China III emission standard should be banned within the Fifth Ring Road during weekdays; in 2023, phaseout subsidies for gasoline vehicles meeting the China IV emission standard and large and medium-sized passenger vehicles meeting the China III emission standard should be implemented; in 2024, diesel trucks meeting the China IV emission standard should be banned in Beijing; and in 2025, light gasoline vehicles meeting the China IV emission standard and large and medium-sized passenger vehicles meeting the China III emission standard should be banned within the Fifth Ring Road.
Adjustment of the freight transportation structure must be continuously promoted. The special implementation plans for medium- and long-term transportation structural adjustment should be studied and formulated, infrastructure facilities should be improved, and the use of rail transportation for bulk goods, such as sand and gravel aggregates, commercial vehicles, steel, and productive coal, should be promoted. The construction of green sand and gravel bases should be promoted to further increase the proportion of sand and gravel transportation by rail and new-energy vehicles.
This study analyzes the energy structure, number of motor vehicles and nonroad mobile machinery units, and their energy consumption and emissions in Beijing. It combines the current air pollutant and CO2 emissions in Beijing and proposes synergistic control measures and recommendations for the existing policies and problems related to diesel vehicle pollution control in Beijing. The main conclusions are as follows:
From 2013 to 2020, the adjustment and optimization of vehicle structure caused decreases of 39.5%, 75.3%, and 42.8% in the NOx, PM2.5, and VOC emissions of different vehicle types, respectively, with PM2.5 reduction being the most significant. Diesel trucks contribute significantly to NOx and PM2.5 emissions. CO2 emissions from diesel combustion decreased from 3.85 million tonnes in 2013 to 2.24 million tonnes in 2020, a reduction of 41%. The contribution of diesel combustion to total CO2 emissions decreased from 3% in 2013 to 2% in 2020.
To improve air quality and take the lead in carbon neutrality in the future, Beijing must further accelerate energy structure adjustment and the development of new-energy vehicles in the transportation sector. Existing policies are insufficient to promote the updating of in-use vehicles into new-energy vehicles, and the purchase cost, technology, and supporting facilities have not reached the ideal standard. In the future, full-scale electrification depends on social consensus and transformation, the establishment and guarantee of relevant systems, the safe supply of electricity, and breakthroughs in EV control and fast charging technologies. The need for large-scale production and reduced purchase cost must also be addressed.
Carbon neutralization is an important opportunity for diesel reduction to strengthen the synergistic control of atmospheric pollution and carbon emissions from diesel combustion. Energy consumption reduction is a systematic project, but it is not appropriate to control the consumption of diesel and gasoline separately, and coordination should be enhanced. Diesel consumption and emissions can be reduced through dual control of the number of industries and the total amount of emissions. Combined with the management of carbon transportation and carbon emission units, the prevention and reduction of energy and emissions in key vehicle-using industries should be promoted.
The original contributions presented in the study are included in the article/Supplementary Material; further inquiries can be directed to the corresponding author.
Conceptualization, YA; methodology, YC and YG; formal analysis, XW, TW, and XL; data curation, YS, ML, YW, and HY; writing—original draft preparation, YA; writing—review and editing, XY.
The authors declare that the research was conducted in the absence of any commercial or financial relationships that could be construed as a potential conflict of interest.
The reviewer TJ declared a past co-authorship with the author YA to the handling editor.
All claims expressed in this article are solely those of the authors and do not necessarily represent those of their affiliated organizations, or those of the publisher, the editors and the reviewers. Any product that may be evaluated in this article, or claim that may be made by its manufacturer, is not guaranteed or endorsed by the publisher.
Audrius, D., and Auksė, M. (2015). The statistical evaluation and comparison of ADMS-Urban model for the prediction of nitrogen dioxide with air quality monitoring network. Environ. Monit. Assess. 187 (578), 578–612. doi:10.1007/s10661-015-4810-1
Axsen, J., and Kurani, K. S. (2010). Anticipating plug-in hybrid vehicle energy impacts in California: Constructing consumer-informed recharge profiles. Transp. Res. Part D Transp. Environ. 15 (4), 212–219. doi:10.1016/j.trd.2010.02.004
Bessa, R. J., and Matos, M. A. (2012). Economic and technical management of an aggregation agent for electric vehicles: A literature survey. Euro. Trans. Electr. Power 22 (3), 334–350. doi:10.1002/etep.565
Cao, X. Z., Tian, Y., Shen, Y., Wu, T. R., Li, R. F., Liu, X. Y., et al. (2021). Emission variations of primary air pollutants from highway vehicles and implications during the COVID-19 pandemic in beijing, China. Int. J. Environ. Res. Public Health 118 (8), 4019. doi:10.3390/ijerph18084019
Fan, C. Y., Song, C. L., Lv, G., Wang, G. Y., Zhou, H., and Jing, X. J. (2018). Evaluation of carbonyl compound emissions from a non-road machinery diesel engine fueled with a methanol/diesel blend. Appl. Therm. Eng. 129, 1382–1391. doi:10.1016/j.applthermaleng.2017.10.086
Fu, M. L., Ge, Y. S., Tan, J. W., Zeng, T., and Liang, B. (2012). Characteristics of typical non-road machinery emissions in China by using portable emission measurement system. Sci. Total Environ. 437, 255–261. doi:10.1016/j.scitotenv.2012.07.095
Gao, C. K., Gao, C. B., Song, K. H., Xing, Y. H., and Chen, W. W. (2020). Vehicle emissions inventory in high spatial–temporal resolution and emission reduction strategy in Harbin-Changchun Megalopolis. Process Saf. Environ. Prot. 138, 236–245. doi:10.1016/j.psep.2020.03.027
Gu, X. K., Yin, S. S., Lu, X., Zhang, H., Wang, L. L., Bai, L., et al. (2019). Recent development of a refined multiple air pollutant emission inventory of vehicles in the Central Plains of China. J. Environ. Sci. 84 (10), 80–96. doi:10.1016/j.jes.2019.04.010
Hao, L. J., Zhao, Z. H., Yin, H., Wang, J. F., Li, L. J., Lu, W. H., et al. (2022). Study of durability of diesel vehicle emissions performance based on real driving emission measurement. Chemosphere 297, 134171. doi:10.1016/j.chemosphere.2022.134171
Jiang, J., Gu, F., Gennish, R., Moore, D. J., Harris, G., and Ball, A. D. (2008). Monitoring of diesel engine combustions based on the acoustic source characterisation of the exhaust system. Mech. Syst. Signal Process. 22, 1465–1480. doi:10.1016/j.ymssp.2007.12.003
Kim, C. H., Qi, G. S., Dahlberg, K., and Li, W. (2010). Strontium-doped perovskites rival platinum catalysts for treating NOx in simulated diesel exhaust. Science 327, 1624–1627. doi:10.1126/science.1184087
Lee, S. J., Jeong, S. J., Kim, W. S., and Lee, C. B. (2008). Computational study on the effects of volume ratio of DOC/DPF and catalyst loading on the PM and NOx emission control for heavy-duty diesel engines. Int. J. Automot. Technol. 9 (6), 659–670. doi:10.1007/s12239-008-0078-6
Lv, B., Zhang, B., and Bai, Y. (2016). A systematic analysis of PM2.5 in beijing and its sources from 2000 to 2012. Atmos. Environ. X. 124, 98–108. doi:10.1016/j.atmosenv.2015.09.031
NDRC Energy Research Institute (2011). Data from: Guidelines for the preparation of provincial GHG inventories. http://www.cbcsd.org.cn/sjk/nengyuan/standard/home/20140113/download/shengjiwenshiqiti.pdf.
Ni, X. Y., Huang, H., and Du, W. P. (2017). Relevance analysis and short-term prediction of PM2.5 concentrations in beijing based on multi-source data. Atmos. Environ. X. 150, 146–161. doi:10.1016/j.atmosenv.2016.11.054
Righi, S., Lucialli, P., and Pollini, E. (2009). Statistical and diagnostic evaluation of the ADMS-Urban model compared with an urban air quality monitoring network. Atmos. Environ. X. 43 (25), 3850–3857. doi:10.1016/j.atmosenv.2009.05.016
Song, Y., Xie, S., Zhang, Y., Zeng, L., Salmon, L. G., and Zheng, M. (2006). Source apportionment of PM2.5 in beijing using principal component analysis/absolute principal component scores and unmix. Sci. Total Environ. 372 (1), 278–286. doi:10.1016/j.scitotenv.2006.08.041
Wu, T. R., Cui, Y. Y., Lian, A. P., Tian, Y., Li, R. F., Liu, X. Y., et al. (2022). Vehicle emissions of primary air pollutants from 2009 to 2019 and projection for the 14th Five-Year Plan period in Beijing, China. J. Environ. Sci. 124, 513–521. doi:10.1016/j.jes.2021.11.038
Xue, Y. F., Liu, X. Y., Cui, Y. Y., Shen, Y., Wu, T. R., Wu, B. B., et al. (2022). Characterization of air pollutant emissions from construction machinery in Beijing and evaluation of the effectiveness of control measures based on information code registration data. Chemosphere 303, 135064. doi:10.1016/j.chemosphere.2022.135064
Yang, F., Xie, Y., Deng, Y., and Yuan, C. (2018). Predictive modeling of battery degradation and greenhouse gas emissions from U.S. state-level electric vehicle operation. Nat. Commun. 9 (1), 2429–29. doi:10.1038/s41467-018-04826-0
Yao, Z., Wei, H., Perugu, H., Liu, H., and Li, Z. X. (2014). Sensitivity analysis of project level MOVES running emission rates for light and heavy duty vehicles. J. Traffic Transp. Eng. 1 (2), 81–96. doi:10.1016/S2095-7564(15)30092-1
Zhang, Q. J., Yang, L., Ma, C., Zhang, Y. J., Wu, L., and Mao, H. J. (2020). Emission characteristics and chemical composition of particulate matter emitted by typical non-road construction machinery. Atmos. Pollut. Res. 11 (4), 679–685. doi:10.1016/j.apr.2019.12.018
Zhang, S. J., Wu, Y., Wu, X. M., Li, M. L., Ge, Y. S., Liang, B., et al. (2014). Historic and future trends of vehicle emissions in beijing, 1998–2020: A policy assessment for the most stringent vehicle emission control program in China. Atmos. Environ. X. 89, 216–229. doi:10.1016/j.atmosenv.2013.12.002
Keywords: mobile source, diesel consumption, total pollutants control, control measures, atmospheric pollution, carbon dioxide
Citation: Ai Y, Cui Y, Ge Y, Wu X, Wu T, Liu X, Shen Y, Liu M, Wan Y, Yi H and Xue Y (2022) Study on the control targets and measures for total diesel consumption from mobile sources in Beijing, China. Front. Environ. Sci. 10:1068861. doi: 10.3389/fenvs.2022.1068861
Received: 13 October 2022; Accepted: 16 November 2022;
Published: 25 November 2022.
Edited by:
Chaoyang Xue, UMR7328 Laboratoire de physique et chimie de l’environnement et de l'Espace (LPC2E), FranceReviewed by:
Taosheng Jin, Nankai University, ChinaCopyright © 2022 Ai, Cui, Ge, Wu, Wu, Liu, Shen, Liu, Wan, Yi and Xue. This is an open-access article distributed under the terms of the Creative Commons Attribution License (CC BY). The use, distribution or reproduction in other forums is permitted, provided the original author(s) and the copyright owner(s) are credited and that the original publication in this journal is cited, in accordance with accepted academic practice. No use, distribution or reproduction is permitted which does not comply with these terms.
*Correspondence: Yifeng Xue, eHVleWlmZW5nQGNlZS5jbg==
Disclaimer: All claims expressed in this article are solely those of the authors and do not necessarily represent those of their affiliated organizations, or those of the publisher, the editors and the reviewers. Any product that may be evaluated in this article or claim that may be made by its manufacturer is not guaranteed or endorsed by the publisher.
Research integrity at Frontiers
Learn more about the work of our research integrity team to safeguard the quality of each article we publish.