- 1Business School, Hohai University, Nanjing, China
- 2Yangtze Institute for Conservation and Development, Hohai University, Nanjing, China
- 3College of Computer and Information, Hohai University, Nanjing, China
Eco-compensation is effective in promoting the sustainable development of a watershed. The exploration of the public’s willingness to pay for such compensation, along with the corresponding influencing factors, are important for improving the efficacy of eco-compensation programs. However, motivating the public to participate in eco-compensation remains a challenge. In this study, the influencing factors behind public willingness to participate in eco-compensation in China’s Taihu Basin are measured using a contingent valuation method and a random forest model. The main results are as follows: 1) In the Taihu Basin, the mean people are willing to pay for eco-compensation is 287.344 CNY/person/year. 2) The significant factors influencing willingness to pay are recognition of the importance of watershed protection; the impact of the watershed environment on people’s lives; annual incomes; and perceived improvements to the watershed environment over the past five years. 3) Awareness of the need for environmental protection can encourage people to pay eco-compensation. This study provides references that governments can use to formulate and implement eco-compensation policies and hence promote public participation in those policies.
Introduction
In past decades, the development of economies has led to excessive consumption of natural resources (Meng et al., 2019; Kong, et al., 2021; Deng, et al., 2022). A large number of pollutants are discharged into river basins, exceeding the self-purification capacity of the water resources and directly threatening the sustainable development of the watershed (Lu et al., 2021).
To improve watershed environments, governments and scholars have undertaken a series of explorations. Among these, payment for watershed ecosystem services (PWES) is one of the most important mechanisms ensuring the sustainable development of the ecological environment (Pan et al., 2021). Such a strategy has been piloted in many countries, including, for example, the wastewater discharge program along the United States–Mexico border, water resource protection in New York, the water–soil conservation fund in Ecuador, and compensation between the French Vittel mineral water company and farmers in water source areas (Perevochtchikova et al., 2021). Combining the flexibility of PWES with efficient government management, the Chinese government has also proposed watershed ecological compensation (EC) schemes (Ding et al., 2022). These programs have been implemented in the Dongjiang River Basin, the Xin’an River Basin, the Taihu River Basin, and at other watersheds (Shang et al., 2018; Guan et al., 2021). In piloting these EC programs, the government has propounded a “developer protects, saboteur rehabilitates, beneficiary compensates, and polluter pays” principle (Liu et al., 2022). Meanwhile, scholars have conducted considerable research into watershed eco-compensation, by focusing on its definitions, standards, and the value of the resulting ecosystem services (Alcon et al., 2019; Ding and Yao 2022).
However, EC mainly relies on government financial transfer, accompanied by many adverse effects, including insufficient funding sources, ineffective coordination, and poor outcomes in sustainability and equality (Chen et al., 2022). To resolve these problems, scholars have made a series of studies around the coordination of different stakeholders, especially governmental regulation of eco-compensation (Gao, et al., 2019; Jiang et al., 2019; Fang et al., 2022). Because the public is the most important beneficiary of the watershed ecological environment (Xiong et al., 2018), public participation can improve the level of decision-making and reduce the environmental protection cost for the government (Ren et al., 2020; Wu and Jin, 2020). Public participation is essential for resolving the aforementioned adverse effects and is also effective in balancing economic development and ecological protection (Li, et al., 2021). Furthermore, public participation can be used to supervise the environmental protection work and increase social awareness of the need for such protection. Public participation is also conducive to establishing diversified EC mechanisms (Yang et al., 2021; Ding et al., 2022). Analyzing public willingness to pay, and the factors driving that willingness, is thus important for the implementation of eco-compensation mechanisms.
In existing studies, chosen experiments and the contingent valuation method (CVM) are the most widely used methodologies (Jayalath et al., 2021; Perni et al., 2021; Sun et al., 2021; Zheng et al., 2021; Hu, et al., 2022). Loomis et al. (2000) applied the CVM method to evaluate the total value of river restoration in the United States. They also analyzed the correlation between residents’ socio-economic characteristics and their willingness to pay (WTP). Shu (2018) adopted the CVM to calculate the WTP of residents at different times. Yao et al. (2019) investigated residents’ WTP for water quality changes in a coastal aquatic ecological area. According to the existing literature, residents’ willingness to pay for ecosystem services (ES) is affected by gender, age, education, and annual income (Osiolo 2017; Li et al., 2018). A sense of ownership, satisfaction with the government’s work, and the awareness of the importance of ecological protection also influence residents’ WTP (Li et al., 2021). Generally, residents with higher levels of education and higher incomes are more motivated to pay for ES. Scholars commonly use the probit model, the logistic regression model, the Tobit model, entropy, and other methods to analyze the factors influencing residents’ WTP (Afroz et al., 2017). However, such willingness ultimately results from the interaction of multiple factors. It is typically a high-dimensional and nonlinear problem, with which traditional algorithms cannot deal well.
Machine learning is effective in resolving this type of problem and has been widely used in influence analysis (Chen et al., 2022). Elzain et al. (2022) compared three machine-learning algorithms, and found that the random forest (RF) method performed the most effectively and reliably. Through its random sampling and random feature extraction, the RF algorithm can effectively solve this kind of problem. In particular, the RF model is not sensitive to lack of data and is effective in calculating the importance of each factor. This paper thus couples the CVM with the RF model to explore the public’s willingness to pay for eco-compensation and identify the factors influencing such willingness.
The main features of this paper are as follows: Data about the willingness of the public to participate in ecological compensation schemes were collected in a questionnaire survey. The public’s willingness to pay for eco-compensation was then calculated based on the CVM. Finally, the factors influencing public participation in ecological compensation were analyzed in a data-driven way, based on the random forest model. This study ultimately provides suggestions for promoting public participation in ecological compensation and improving the efficiency of such compensation.
Materials and methods
Study area
The Taihu Basin is located on the southern edge of the Yangtze River Delta (Figure 1). It covers a water area of more than two thousand square kilometers, and a total length of nearly four hundred kilometers. The basin plays an important role in the ecosystem security of this region (Shen et al., 2021). Due to rapid development of the economy and the increasing emission of pollutants, the Taihu Basin has problems with water pollution and water ecosystem degradation (Wan et al., 2022). In 2007 the government proposed ecological compensation mechanisms to improve water quality in this watershed. In 2022, the government then proposed broadening the channels of compensation funds and mobilizing the enthusiasm and initiative of public participation.
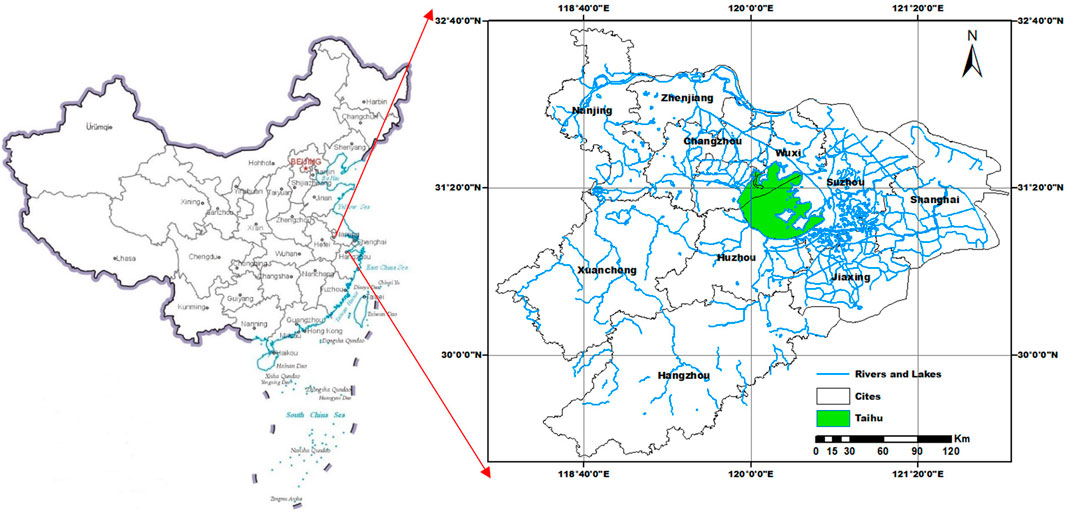
FIGURE 1. Location of Taihu Basin, China.jpg. Note: The map of China was downloaded from http://bzdt.ch.mnr.gov.cn/. Supervised by the Ministry of Natural Resources of the People’s Republic of China. Drawing review number is GS (2019)1651. Map of Taihu generated by ArcGIS 10.5. URL link: https://www.esri.com/en-us/arcgis/products/districting-for-arcgis/overview.
However, there are still some issues that need to be resolved; for example, how to analyze the willingness of the public to participate in eco-compensation and the factors influencing that willingness. There are also common issues in cross-administrative river basin ecological construction. Therefore, this paper adopts the contingent valuation method and random forest model to explore the aforementioned problems, which are of great significance for promoting the establishment and improvement of a long-term ecological compensation system in the Taihu Basin.
Survey design and data collection
The questionnaire is made up of three parts: personal characteristics of respondents, their ecological cognition, and willingness to pay. If respondents refuse to pay for eco-compensation, they are asked to explain the reasons. The personal characteristics include age, gender, education level, and annual income. Ecological cognition includes knowledge of the improvement in the watershed environment over the past five years, familiarity with payment for eco-compensation, and the impact of the watershed environment on people’s lives. Willingness to pay means people’s willingness to contribute financially to the eco-compensation. Where respondents accept paying for eco-compensation, we survey their payment methods and the amount paid. If they refuse to pay for eco-compensation, we inquire into the reasons.
People living around the Taihu Basin were randomly chosen to estimate their WTP eco-compensation. The survey was conducted face-to-face, since face-to-face interviews are convenient for explaining the purpose and procedures clearly. To avoid starting point deviation, we conducted two pre-test surveys before the formal survey and then adjusted the questions and answers to reduce confusion and misunderstanding about the questionnaire. In the first round of the pre-test survey, we collected 100 samples through the network. In the second round of the pre-test survey, we collected 50 samples through face-to-face communication with merchants, employees, students, experts, and other residents. In the formal survey, we collected a total of 635 questionnaires. Excluding questionnaires with mistakes, incomplete information, and response times that were too short or too long, we obtained 561 valid responses. The effectiveness rate was 88.35%. According to the research of Xu et al. (2020) and Li et al. (2021), the reliability testing and the validity of the questionnaire were based on the value of Cronbach’s α and factor analysis, respectively. When the value of Cronbach’s α is greater than 0.6, the reliability of the sample is feasible and acceptable (Cronbach et al., 1951; Bagozzi and Yi, 1988). When factor analysis is adopted to test the validity of samples, it is necessary to analyze the correlation between items. Generally, the Kaiser–Meyer–Olkin (KMO) and Bartlett spherical test are used to test the correlation. The KMO is used to compare the correlation and partial correlation coefficient between items, while Bartlett’s spherical test is used to test whether the correlation coefficient between items is significant or not. The principal component analysis is usually adopted to calculate the KMO value and the significance of Bartlett’s sphericity test (Azam and Rahman, 2022). When SPSS is used to calculate the value of the Bartlett spherical test, the significance (i.e., sig.<0.05) means the validity is acceptable. After removing personal characteristics, such as gender, age, and education level, the value of Cronbach’s α was 0.681, which is greater than 0.6. That is, the overall internal consistency of the questionnaire was acceptable. We then adopted PCA for factor analysis to check the validity of the questionnaire. The KMO value was 0.616, and the p-value of Bartlett’s sphericity test was 0.000. The questionnaire’s validity was therefore acceptable.
Contingent valuation method and random forest algorithm
The contingent valuation method (CVM) is the most widely adopted method in the evaluation of payment for watershed ecosystem services. The method obtains WTP based on questionnaires and the principle of utility maximization. The mean value of the WTP can be calculated as follows:
where
A respondent’s WTP involves many factors, such as education level and the degree of improvement in the ecological environment of the watershed over the past five years. It is necessary to explore which factors are more important than others in order to make more people willing to pay for eco-compensation. The random forest (RF) algorithm uses the bootstrap resampling method to extract multiple samples from the original samples and to then model the decision tree for each bootstrap sample. The results with the largest number of votes are obtained by combining the number of votes obtained from multiple decision trees. The process of the RF method is shown in Figure 2.
As shown in Figure 2, suppose there are N samples, and each sample has M features. The process of the RF is then as follows:
1) Extract the training data set with N samples from the original data set based on the bootstrap aggregation method, and then adopt the extracted N samples to construct N classification decision trees.
2) Select mtry features randomly from M features during the growth of the decision tree (mtry is invariant).
3) Repeat the aforementioned steps until the random forest is constructed.
4) Obtain the classification results based on the majority voting strategy.
Suppose the training round is N, and the classification model sequences are
where
The RF model measures the importance of each factor based on mean decrease accuracy (MDA) or mean decrease Gini (MDG). The MDA is computed by the out-of-bag (OOB) error. Generally, the greater the MDA value, the more important the factor. The MDG calculates the average reduction of Gini impurity caused by the split of the given index. Generally, the greater the MDG, the more important the factor. In this paper, the MDG is adopted to calculate the importance of various features. The formula is as follows:
where
To get better classification results, the parameters of the model are optimized based on the relationship between the generalization error and the model’s complexity. Given the generalization error has a positive quadratic relationship with the model complexity, when the complexity is insufficient or too large, it will lead to an increase of generalization error and the phenomenon of underfitting or overfitting. We therefore need to adjust the complexity of the model by adjusting the parameters to make the generalization error close to the lowest point. In this study, the parameters such as n_estimators (the number of decision trees), max_depth (the depth of the decision tree), and max_features (the random selected features) are optimized. Their best values are determined by learning curve and grid search.
Results
Descriptive statistics
Personal characteristics of respondents
The personal characteristics of the people surveyed in the questionnaire are shown in Table 1. The respondents comprised 52.41% males, which is more than the number of females (47.59%). The majority of respondents were under age 50, and the respondents under 30 years old accounted for 31.91%. Nearly half of the respondents had university education or above. There were 128 respondents (22.82%) whose annual income was less than 60,000 CNY, and 39.75% of the respondents had an annual income of more than 100 thousand yuan.
Ecological cognition of respondents
The ecological awareness of respondents is shown in Table 2. In this questionnaire survey, the majority of respondents were familiar with or had heard of paying for eco-compensation (72.73%). Meanwhile, most of the respondents believed that the ecological environment of the Taihu watershed affected their lives. Among respondents, 15.86% believed the watershed ecological environment greatly affected their lives. Concerning satisfaction with the watershed ecological environment, 19.25% of respondents chose “unsatisfied.” On the whole, the majority of respondents were neutral about, or relatively satisfied with, the ecological environment. Nearly half the respondents (49.73%) believed that the ecology of the watershed had improved over the past five years. More than 90% of respondents thought it important to protect the watershed, which means that after years of piloting eco-compensation, the public are intensely aware of environmental protection.
Willingness to pay eco-compensation
The willingness to pay eco-compensation is shown in Table 3. There were 495 respondents (88.24%) willing to pay for eco-compensation in the Taihu Basin, i.e., most respondents were willing to pay. Among respondents, 52.12% chose to pay the ecological compensation fee; 30.91% of the respondents chose to pay through water charges; and 16.97% of the respondents were willing to pay eco-compensation through donations. By contrast, 11.76% of the respondents chose not to pay eco-compensation. The main reason (accounting for 34.85%) was concern the payment would not be well used, while 22.73% of respondents considered it the government’s responsibility to protect the ecosystem.
Estimation of WTP
The estimation of the public’s WTP eco-compensation in the Taihu Basin was based on interval values. Referring to the studies conducted by Feng et al. (2018) and Chu et al. (2020), the median values of intervals were set as the value of respondents’ WTP. In the WTP survey, we provided the following compensation options for the respondents: 0–200, 200–400, 400–600, 600–800, and above 800 CNY/person/year. The proportion of respondents who chose each WTP interval is shown in Table 4.
As shown in Table 4, 11.76% of respondents expressed reluctance to pay for eco-compensation. More than half the respondents (56.15%) chose one of the two compensation options: 200–400 or 400–600 CNY/person/year. There were 11.94% of respondents who selected the option of 0–200 CNY/person/year. No more than 10% of respondents chose the options of 600–800 and more than 800 CNY/person/year. Based on the distribution data and Eq. 1, we can obtain the average expected compensation value. That is, E(WTP) = 287.344 CNY/(person/year). From the distribution data of respondents’ WTP, it is apparent that a compensation value between 200 and 400 CNY/person/year will encourage more people to participate in paying for eco-compensation.
Factors influencing the public’s WTP
In order to improve the public’s enthusiasm about paying for eco-compensation, and in order to promote the sustainable development of the Taihu Basin, we explored the factors influencing the public’s WTP based on the questionnaire data and the random forest (RF) model. First, the relationship between the factors is analyzed based on the correlation coefficient. The correlation coefficient matrix is shown in Figure 3.
As shown in Figure 3, each cell is the correlation coefficient value of the corresponding indexes. The darker the cell, the stronger the correlation between the corresponding indexes. According to the correlation coefficient matrix, the correlation coefficient between age and WTP is negative, indicating that age is negative to the WTP. Meanwhile, annual income and education level are positive for the WTP. That is, the higher the annual income and education level, the more willing people are to pay for eco-compensation. Furthermore, the correlation value between various factors is not large. Therefore, the influence of collinearity between factors on the results can be ignored. After analyzing the correlation relationship among factors, we adopt the RF model to measure the influence of each factor on the WTP (Figure 4).
Figure 4 shows the three most important factors are the importance of environmental protection of the watershed (X9), the impact of the watershed’s ecology on people’s lives (X6), annual income (X4), and the improvement in the ecology of the watershed over the past five years (X8). The greater the awareness of the ecological protection of the watershed, and the more affected people are by the environment, the greater their willingness to participate in eco-compensation. This is in accordance with the actual situation. Meanwhile, people’s annual income and improvement in the ecological environment also greatly affect their willingness to pay for eco-compensation. The government can therefore enhance its ecological protection work and improve public awareness of ecological protection. Meanwhile, when people’s incomes increase, they are more willing to pay for eco-compensation. Other important factors are education levels (X3), age (X2), and familiarity with paying for eco-compensation (X5). Generally, the more familiar people are with the mechanism of eco-compensation, the higher their education levels, and the more they are affected by the watershed ecosystem, the greater will be their willingness to pay for eco-compensation.
Discussion
Ecological compensation (EC) funds are mainly based on transfer payments from the government, resulting in insufficient funding (Li et al., 2021). A diversified EC mechanism is effective in resolving the problem (Ding et al., 2022). People who live around a river basin are the main beneficiaries and participants in the implementation of diversified watershed EC, and their responses directly influence the efficiency of EC use (Ren, et al., 2020). However, the public’s willingness to pay for eco-compensation is affected by many factors. Thus, it is important to measure the importance of each factor and identify the most important. The CVM is effective in estimating the economic value of the watershed as a natural resource and has been widely adopted to estimate people’s willingness to pay for ecosystem services (Perni et al., 2021; He et al., 2022). Meanwhile, the random forest (RF) model is effective in solving the multi-features problem and can sequence features in terms of importance. Therefore, CVM and RF are coupled together to investigate the willingness to pay for eco-compensation and to identify the influencing factors.
Taking the Taihu Basin as the case study area, and by conducting a face-to-face questionnaire survey, the results show that 75.94% of respondents are not accustomed to paying for eco-compensation or have only heard of it. The government therefore needs to take measures to improve the public’s knowledge of EC. Meanwhile, nearly half the respondents think that the ecology of the Taihu Basin has improved over the past five years. It is gratifying that more than 90% of respondents believe it is important to protect the ecology of the watershed, with 88.24% of respondents willing to pay for eco-compensation, reflecting an average WTP of 287.344 CNY/person/year. Among the respondents willing to pay for ecological compensation, 52.12% are willing to pay through an ecological compensation fee. The main reason people give for refusing to pay for eco-compensation is the concern the payment will not be well used. This means that governments need to improve the transparency of their use of ecological compensation funds. Due to the diversity of eco-compensation, in terms of both individuals and regions, the value of WTP will vary accordingly. For example, in the Xin’an River Basin, the public’s willingness to pay is 20.04 USD per year (Ren et al., 2020). However, in the survey of Taipu River, the mean WTP was 51.08 RMB per month (Li, et al., 2021), i.e., approx. 85 USD per year. The difference here stems from economic and social development, natural attributes, and the budgets of respondents (Wang and Jia, 2012).
The influence analysis results show that the most three important ecological awareness factors are the perceived importance of watershed ecosystem protection, the impact of the watershed on people’s lives, and the improvement in the watershed ecosystem over the past five years. Personal factors of age, annual income, and education also have a distinct influence on people’s WTP. These results are consistent with previous studies. For example, people who recognize the importance of environmental protection are more inclined to pay eco-compensation (Peng et al., 2022). The public’s willingness to pay for eco-compensation may be triggered by improvements in the environment (Nie et al., 2022). As education levels and annual incomes rise, people are willing to pay more for eco-compensation (Chu et al., 2020). In relation to age, younger people are more inclined to pay for eco-compensation (Ren et al., 2020). Furthermore, awareness of environmental protection and knowledge about eco-compensation can also encourage public participation (Li, et al., 2021).
Conclusion
Eco-compensation (EC) plays an important role in balancing the protection of the environment and the development of the economy. To further promote improvement in the watershed environment, it is necessary to motivate the public to participate in eco-compensation and hence to improve its efficiency. Therefore, this paper involved a survey in the Taihu Basin, analyzing people’s willingness to pay for eco-compensation, and the factors influencing that willingness. The survey results show that the majority of respondents are willing to pay for eco-compensation. Meanwhile, public awareness of environmental protection is relatively high. The majority of the public have heard of, or are familiar with eco-compensation. Most respondents believe that the watershed environment is related to their lives and consider it necessary to protect its ecology and to implement eco-compensation. Based on the survey results, we have explored the key factors influencing willingness to pay by adopting the random forest algorithm. We found that the key influencing factors are awareness of the importance of watershed ecological protection, the impact of the watershed environment on people’s lives, annual incomes, and improvements in the watershed environment over the past five years. The reason why some respondents are unwilling to pay is because they consider the ecological compensation funds are poorly supervised and not well utilized. The government should therefore increase efforts to strengthen the supervision of ecological compensation funds.
This study has expanded the research into willingness to pay watershed eco-compensation. Although the influencing factors and the WTP of the public are mainly determined by the economic and social situations of target individuals and areas, the research models proposed in this paper can be used in other regions. This study fills certain gaps in the literature, but there are nevertheless some limitations. First, this study mainly focuses on the opinions of the public. However, eco-compensation involves multiple stakeholders, such as governments, NGOs, and others. In a future study, follow-up surveys will be conducted to analyze the WTP and the corresponding influencing factors under the interaction of multi-stakeholders. Second, it would be worth extending our research to other areas and comparing the factors influencing the people of different watersheds. Third, in future studies, it will be necessary to explore the spatial-temporal trends of WTP and its influencing factors, so as to provide decision-making references for eco-compensation.
Data availability statement
The original contributions presented in the study are included in the article/Supplementary Material. Further inquiries can be directed to the corresponding author.
Ethics statement
Ethical review and approval was not required for the study on human participants in accordance with the local legislation and institutional requirements. Written informed consent from the participants was not required to participate in this study in accordance with the national legislation and the institutional requirements.
Author contributions
MD: conceptualization, methodology, formal analysis, investigation, validation, writing—original draft, and Writing—review, and editing; ZL: resources, data curation, software, and visualization. JC: conceptualization, supervision, and writing—review and editing. FT: conceptualization, supervision, and writing—original draft.
Funding
This work was funded by the National Natural Science Foundation of China (Grant No. 42001250), the Fundamental Research Funds for the Central Universities (Grant No. B200201032), and the Changzhou Introduction and Cultivation of Leading Innovative Talents Program (Grant No. CQ20210095).
Conflict of interest
The authors declare that the research was conducted in the absence of any commercial or financial relationships that could be construed as a potential conflict of interest.
Publisher’s note
All claims expressed in this article are solely those of the authors and do not necessarily represent those of their affiliated organizations, or those of the publisher, the editors, and the reviewers. Any product that may be evaluated in this article, or claim that may be made by its manufacturer, is not guaranteed or endorsed by the publisher.
Supplementary material
The Supplementary Material for this article can be found online at: https://www.frontiersin.org/articles/10.3389/fenvs.2022.1066194/full#supplementary-material
References
Afroz, R., Rahman, A., Masud, M., and Akhtar, R. (2017). The knowledge, awareness, attitude and motivational analysis of plastic waste and household perspective in Malaysia. Environ. Sci. Pollut. Res. 24, 2304–2315. doi:10.1007/s11356-016-7942-0
Alcon, F., Tapsuwan, S., Brouwer, R., Yunes, M., Mounzer, O., and de-Miguel, M. D. (2019). Modelling farmer choices for water security measures in the Litani river basin in Lebanon. Sci. Total Environ. 647, 37–46. doi:10.1016/j.scitotenv.2018.07.410
Azam, M., and Rahman, M. (2022). Assessing spatial vulnerability of Bangladesh to climate change and extremes: A geographic information system approach. Mitig. Adapt. Strateg. Glob. Chang. 27 (6), 38. doi:10.1007/s11027-022-10013-w
Bagozzl, R., and Yi, Y. (1988). On the evaluation of structural equation models. J. Acad. Mark. Sci. 16 (1), 74–94. doi:10.1007/BF02723327
Chen, J., Wang, Q., and Li, Q. (2022). A quantitative assessment on ecological compensation based on water resources value accounting: A case study of water source area of the middle route of south-to-north water transfer project in China. Front. Environ. Sci. 10, 854150. doi:10.3389/fenvs.2022.854150
Chu, X., Zhan, J., Wang, C., Hameeda, S., and Wang, X. (2020). Households’ willingness to accept improved ecosystem services and influencing factors: Application of contingent valuation method in bashang plateau, hebei province, China. J. Environ. Manage. 255, 109925. doi:10.1016/j.jenvman.2019.109925
Cronbach, L., Dou, S., and Xu, D. (1951). Coefficient alpha and the internal structure of testsThe effectiveness of eco-compensation in environmental protection: A hybrid of the government and market. J. Environ. Manage.J Environ. Manag. 16280, 297111840–297112334. doi:10.1007/BF02310555Chen10.1016/j.jenvman.2020.111840
Deng, M., Chen, J., Tao, F., Zhu, J., and Wang, M. (2022). On the coupling and coordination development between environment and economy: A case study in the Yangtze River Delta of China. Int. J. Environ. Res. Public Health 19 (1), 586. doi:10.3390/ijerph19010586
Ding, J., Chen, L., Deng, M., and Chen, J. (2022). A differential game for basin ecological compensation mechanism based on cross-regional government-enterprise cooperation. J. Clean. Prod. 362 (15), 132335. doi:10.1016/j.jclepro.2022.132335
Ding, Z., and Yao, S. (2022). Theory and valuation of cross-regional ecological compensation for cultivated land: A case study of shanxi province, China. Ecol. Indic. 136, 108609. doi:10.1016/j.ecolind.2022.108609
Elzain, H., Chung, Y., Senapathi, V., Sekar, S., Lee, S., Roy, P., et al. (2022). Comparative study of machine learning models for evaluating groundwater vulnerability to nitrate contamination. Ecotoxicol. Environ. Saf. 229, 113061. doi:10.1016/j.ecoenv.2021.113061
Fang, J., Zhou, J., and Jiang, K. (2022). Evolution of stakeholders' behavioral strategies in the ecological compensation mechanism for poverty alleviation. Resour. Conserv. Recycl. 176, 105915. doi:10.1016/j.resconrec.2021.105915
Feng, D., Liang, L., Wu, W., Li, C., Wang, L., Li, L., et al. (2018). Factors influencing willingness to accept in the paddy land-to-dry land program based on contingent value method. J. Clean. Prod. 183, 392–402. doi:10.1016/j.jclepro.2018.02.142
Gao, X., Shen, J., He, W., Sun, F., Zhang, Z., Guo, W., et al. (2019). An evolutionary game analysis of governments decision-making behaviors and factors influencing watershed ecological compensation in China. J. Environ. Manage. 251, 109592. doi:10.1016/j.jenvman.2019.109592
Guan, X. J., Liu, M., and Meng, Y. (2021). A comprehensive ecological compensation indicator based on pollution damage-protection bidirectional model for river basin. Ecol. Indic. 126 (4), 107708. doi:10.1016/j.ecolind.2021.107708
He, J., Zhou, W., Guo, S., Deng, X., Song, J., and Xu, D. (2022). Effect of land transfer on farmers’ willingness to pay for straw return in Southwest China. J. Clean. Prod. 369, 133397. doi:10.1016/j.jclepro.2022.133397
Hu, P., Zhou, Y., Zhou, J., Wang, G., and Zhu, G. (2022). Uncovering the willingness to pay for ecological red lines protection: Evidence from China. Ecol. Indic. 134, 108458. doi:10.1016/j.ecolind.2021.108458
Jayalath, T., Grala, R., Grado, S., and Evans, D. (2021). Increasing provision of ecosystem services through participation in a conservation program. Ecosyst. Serv. 50, 101303. doi:10.1016/j.ecoser.2021.101303
Jiang, K., You, D., Li, Z., and Shi, S. (2019). A differential game approach to dynamic optimal control strategies for watershed pollution across regional boundaries under eco-compensation criterion. Ecol. Indic. 105, 229–241. doi:10.1016/j.ecolind.2019.05.065
Kong, Y., He, W., Yuan, L., Zhang, Z., Gao, X., Zhao, Y., et al. (2021). Decoupling economic growth from water consumption in the Yangtze River economic belt, China. Ecol. Indic. 123, 107344. doi:10.1016/j.ecolind.2021.107344
Li, G., Sun, F., Bitian, F., Deng, L., Yang, K., Liu, Y., et al. (2021). How to promote the public participation in eco-compensation in transboundary river basins: A case from planned behavior perspective. J. Clean. Prod. 313, 127911. doi:10.1016/j.jclepro.2021.127911
Li, Q., Long, R., and Chen, H. (2018). Differences and influencing factors for Chinese urban resident willingness to pay for green housings: Evidence from five first-tier cities in China. Appl. Energy 229, 299–313. doi:10.1016/j.apenergy.2018.07.118
Liu, X., Wang, W., Wu, W., Zhang, L., and Wang, L. (2022). Using cooperative game model of air pollution governance to study the cost sharing in Yangtze River Delta region. J. Environ. Manage. 301, 113896. doi:10.1016/j.jenvman.2021.113896
Loomis, J., Kent, P., Strange, L., Fausch, K., and Covich, A. (2000). Measuring the total economic value of restoring ecosystem services in an impaired River Basin: Results from a contingent valuation survey. Ecol. Econ. 33 (1), 103–117. doi:10.1016/s0921-8009(99)00131-7
Lu, S., Lian, Z., Sun, H., Wu, X., Bai, X., and Wang, C. (2021). Simulating trans-boundary watershed water resources conflict. Resour. Policy 73, 102139. doi:10.1016/j.resourpol.2021.102139
Meng, Y., Liu, M., Guan, X., and Liu, W. (2019). Comprehensive evaluation of ecological compensation effect in the Xiaohong River Basin, China. Environ. Sci. Pollut. Res. 26 (8), 7793–7803. doi:10.1007/s11356-019-04208-z
Nie, X., Chen, Z., Yang, L., Qing, Y., and Wang, H. (2022). Does the protection of river basin ecosystems produce broken window effect? Evidence from spatial choice experiment in xijiang river basin, China. Front. Mar. Sci. 9, 842491. doi:10.3389/fmars.2022.842491
Osiolo, H. (2017). Willingness to pay for improved energy: Evidence from Kenya. Renew. Energy 112, 104–112. doi:10.1016/j.renene.2017.05.004
Pan, Y., Wu, J., Zhang, Y., Zhang, X., and Yu, C. (2021). Simultaneous enhancement of ecosystem services and poverty reduction through adjustments to subsidy policies relating to grassland use in Tibet, China. Ecosyst. Serv. 48, 101254. doi:10.1016/j.ecoser.2021.101254
Peng, Z., Pu, H., Huang, X., Zheng, R., and Xu, L. (2022). Study on public willingness and incentive mechanism of ecological compensation for inter-basin water transfer in China in the carbon neutral perspective. Ecol. Indic. 143, 109397. doi:10.1016/j.ecolind.2022.109397
Perevochtchikova, M., Castro-Díaz, R., Langle-Flores, A., and Ugalde, J. (2021). A systematic review of scientific publications on the effects of payments for ecosystem services in Latin America, 2000-2020. Ecosyst. Serv. 49 (1), 101270. doi:10.1016/j.ecoser.2021.101270
Perni, A., Barreiro-Hurle, J., and Martinez-Paz, J. (2021). Contingent valuation estimates for environmental goods: Validity and reliability. Ecol. Econ. 189, 107144. doi:10.1016/j.ecolecon.2021.107144
Ren, Y., Lu, L., Zhang, H., Chen, H., and Zhu, D. (2020). Residents' willingness to pay for ecosystem services and its influencing factors: A study of the Xin'an River basin. J. Clean. Prod. 268, 122301. doi:10.1016/j.jclepro.2020.122301
Shang, W., Gong, Y., Wang, Z., and Stewardson, M. (2018). Eco-compensation in China: Theory, practices and suggestions for the future. J. Environ. Manage. 210, 162–170. doi:10.1016/j.jenvman.2017.12.077
Shen, J., Gao, X., He, W., Sun, F., Zhang, Z., Kong, Y., et al. (2021). Prospect theory in an evolutionary game: Construction of watershed ecological compensation system in Taihu lake basin. J. Clean. Prod. 291, 125929. doi:10.1016/j.jclepro.2021.125929
Shu, L. (2018). Games between stakeholders and the payment for ecological services: Evidence from the wuxijiang river reservoir area in China. Peerj 6, e4475. doi:10.7717/peerj.4475
Sun, X., Liu, X., Zhao, S., and Zhu, Y. (2021). An evolutionary systematic framework to quantify short-term and long-term watershed ecological compensation standard and amount for promoting sustainability of livestock industry based on cost-benefit analysis, linear programming, WTA and WTP method. Environ. Sci. Pollut. Res. 28 (14), 18004–18020. doi:10.1007/s11356-020-11769-x
Wan, L., Zheng, Q., Wu, J., Wei, Z., and Wang, S. (2022). How does the ecological compensation mechanism adjust the industrial structure? Evidence from China. J. Environ. Manage. 301, 113839. doi:10.1016/j.jenvman.2021.113839
Wang, P., and Jia, J. (2012). Tourists' willingness to pay for biodiversity conservation and environment protection, dalai lake protected area: Implications for entrance fee and sustainable management. Ocean. Coast. Manag. 62, 24–33. doi:10.1016/j.ocecoaman.2012.03.001
Wu, L., and Jin, L. (2020). How eco-compensation contribute to poverty reduction: A perspective from different income group of rural households in guizhou, China. J. Clean. Prod. 275, 122962. doi:10.1016/j.jclepro.2020.122962
Xiong, K., Kong, F., Zhang, N., Lei, N., and Sun, C. (2018). Analysis of the factors influencing willingness to pay and payout level for ecological environment improvement of the Ganjiang River Basin. Sustainability 10 (7), 2149. doi:10.3390/su10072149
Xu, D., Qing, C., Deng, X., Yong, Z., Zhou, W., and Ma, Z. (2020). Disaster risk perception, sense of pace, evacuation willingness, and relocation willingness of rural households in earthquake-stricken areas: Evidence from sichuan province, China. Int. J. Environ. Res. Public Health 17 (2), 602. doi:10.3390/ijerph17020602
Yang, M., Chen, H., Long, R., Wang, Y., Hou, C., and Liu, B. (2021). Will the public pay for green products? Based on analysis of the influencing factors for Chinese’s public willingness to pay a price premium for green products. Environ. Sci. Pollut. Res. 28, 61408–61422. doi:10.1007/s11356-021-14885-4
Yao, H., Liu, H., and Ni, T. (2019). Estimation of the value of aquatic ecosystem services in the coastal area of Jiangsu Province, China. Integr. Environ. Assess. Manag. 15 (6), 1012–1020. doi:10.1002/ieam.4175
Keywords: ecological compensation, willingness to pay, contingent valuation method, random forest model, Taihu Basin
Citation: Deng M, Li Z, Chen J and Tao F (2022) Public willingness to pay watershed eco-compensation in China’s Taihu basin: A case study. Front. Environ. Sci. 10:1066194. doi: 10.3389/fenvs.2022.1066194
Received: 10 October 2022; Accepted: 01 November 2022;
Published: 17 November 2022.
Edited by:
Dingde Xu, Sichuan Agricultural University, ChinaReviewed by:
Xin Gao, Shanghai Jiao Tong University, ChinaMin An, China Three Gorges University, China
Gang Liu, Tianjin University, China
Copyright © 2022 Deng, Li, Chen and Tao. This is an open-access article distributed under the terms of the Creative Commons Attribution License (CC BY). The use, distribution or reproduction in other forums is permitted, provided the original author(s) and the copyright owner(s) are credited and that the original publication in this journal is cited, in accordance with accepted academic practice. No use, distribution or reproduction is permitted which does not comply with these terms.
*Correspondence: Menghua Deng, dengmenghua@hhu.edu.cn