- 1College of Economics and Management, Huaiyin Normal University, Huai’an, China
- 2Research Centre for Soft Energy Science, College of Economics and Management, Nanjing University of Aeronautics and Astronautics, Nanjing, China
Due to the differences in economic development, resource endowment, and historical accumulation, different types of countries have significant technical heterogeneity in carbon emissions. Identifying the driving factors of carbon emission changes, under the premise of distinguishing national heterogeneity, can provide a basis for the formulation of the “Differentiated Responsibilities” emission reduction policies. Therefore, this study introduces the idea of Meta-frontier into the traditional production-theoretical decomposition analysis, and constructs a new influencing factor analysis framework. Based on the newly built method, the empirical study of 60 representative countries draws the following three meaningful conclusion: 1) Different types of countries have obvious heterogeneity in technology, efficiency and change trend of energy use. Specifically, countries with higher energy intensity values generally have a quicker decline rate than those with lower energy intensity values. There exists “catch-up” effects for the backward to the advanced countries. 2) Decomposition results show that potential energy intensity (PEI) is the dominant factor reducing carbon emissions, especially for those large economic output with large energy consumption (Group-L) countries (0.604). Economic activity effect (ECA) is the most significant driving force for countries with small economic output and small energy consumption (Group-S), reaching 1.806. Meanwhile, the attribution results showed different characteristics in different groups of countries. The impact of various factors that reflect the heterogeneity of production process on carbon emissions mainly comes from the contribution of Group-L. 3) We suggest that, in the process of carbon reduction, large energy consumption countries should pay more attention to the gap between the development and speed of the world’s cutting-edge technologies.
Introduction
Global warming seriously restricts the sustainable development of social and economic and has become a serious challenge for the 21st century. In 2022, IPCC (2022): Mitigating Climate Change, stated that without immediate action, it is impossible to limit global warming to 1.5°C (IPCC, 2022). This will threaten human livelihood and health, such as accelerating sea level rise (Fuchs et al., 2020; IPCC, 2021). The main cause of greenhouse effect is the excessive carbon dioxide (CO2) emission produced by the combustion of traditional fossil energy (Goh et al., 2018; Udemba and Tosun, 2022a). According to the scenario analysis by Huo et al. (2022), in 2030, global carbon emissions are most likely to increase by 30% above the 2010 level. Therefore, energy saving and CO2 emission reduction have become the consensus of all countries in the world.
A growing number of countries are taking active steps to address the challenge of climate warming. For example, many responsible countries have signed legislations and treaties to reduce greenhouse gas (GHG) emissions, including the United Nations Framework Convention on Climate Change (UNFCCC), the Kyoto Protocol, the Copenhagen Accord, and the Paris Agreement. Under these emissions constraint frameworks, measures and policies to deal with global climate change have been effectively deployed (Chen, 2021).
In the process of economic development, the conflict between emission reduction and economic growth may be exacerbated by the specific development policies adopted by countries. This conflict poses a huge challenge to countries in addressing the trade-off between emissions reductions and economic growth. In addition, due to the diverse socioeconomic backgrounds, development pattern and resource endowments, there is huge production heterogeneity in different countries (Liu et al., 2022). For example, less developed Asia and Africa countries, such as the Philippines, Indonesia, Mongolia, Congo, and India, are characterized by a weaker economy and lower socioeconomic status, but with sufficient natural mineral resources and cheap labor (Huang et al., 2021). Compared with those technology-intensive and capital-intensive countries (suah as Japan, Germany, and America), these countries generally belong to a labor-intensive mode of production. According to Lin and Zhu (2021), the same Gross Domestic Production (GDP) output in different types of production modes should have different types of energy consumption and carbon emission process (i.e., the heterogeneity of energy intensity and carbon intensity). As such, in order to comprehensively formulate regional emission reduction policies, it is necessary to deeply understand the root causes of differences in carbon emission change in different types of countries.
However, regarding the global carbon emission changes, the production heterogeneity of carbon emissions in different countries have been barely discussed, especially in the heterogeneity related driving factors identification. Thus three questions are raised for the global carbon emission reduction, as follows: 1) is there any heterogeneity in carbon emissions of different types of countries, and what is the change trend of such heterogeneity? 2) What are the core driving factors influencing the change of global carbon emissions? And how do the factors related to heterogeneity affect the change of carbon emissions? 3) From the overall carbon emission control perspective, how to identify the source of emissions for a certain influencing factor, that is, how is the contribution heterogeneity of different countries?
To reply the above three questions, considering the production heterogeneity between different countries, this paper constructs a new decomposition framework to explore the drivers of the global carbon emission changes. As such, the contribution of this paper mainly lies in three aspects:
First, previous decomposition analysis methods generally cannot explore the heterogeneity related factors. To address this issue, this study constructed a new comprehensive decomposition model to investigate the driving factors of carbon emission changes. Specifically, a new pre-defined factors, i.e., the technology gap effect (TEG) has been further discussed. This is able to yield additional insights about the impact of production heterogeneity changes on carbon emissions. In addition, the proposed decomposition method can also be used to study changes of specific indicators in other different fields. Especially for those with significant heterogeneity characteristics, which needs further explore the “technology catch-up effect.”
Second, in terms of driving factors of carbon emission changes, few studies have discussed them from the “process” perspective. In fact, the whole carbon emission process contains different stages, which played different roles in the change of carbon emissions. The identification of process contribution can make the responsibility of carbon emission reduction clearer. As such, this study constructs a new decomposition framework and systematically decomposes the influencing factors of carbon emission changes from three processes, i.e., the energy input process, the production process, and the economic output process.
Third, in existing studies, there has been an increasing focus on the internal relationship between energy consumption, economic output, and carbon emissions. However, few studies have accurately identified the transmission mechanism between them. Considering that the direct and indirect causes of carbon emissions are energy consumption and economic development respectively, this study all-around measures their internal relationships. This can help policymakers to formulate more balanced emission reduction policies from multiple dimensions of energy consumption and economic development.
Literature review
There are complex connections among energy consumption, carbon emissions, and economic development (Debone et al., 2021). In recent years, there has been an increasing focus on the field of environment protection and economic development, especially on the internal relationship between them (Ajmi et al., 2015; Acheampong and Boateng, 2019). Econometrics (Zhu et al., 2014; Dong et al., 2016) and decomposition analysis (Su and Ang, 2012; Wang et al., 2018) methods are the two mainstream tools to conduct the influencing factors analysis.
By comparison, the decomposition analysis method is to decompose the drivers according to the identical deformation, which can effectively avoid the subjective selection of influencing factors in the econometric method (Liu et al., 2022). And the decomposition results are conducive to the horizontal comparison of related research topics between different studies. As such, decomposition analysis method has been widely applied in the field of influencing factor analysis of carbon emission change (Shao et al., 2016; Wang et al., 2018; Long et al., 2022). Index decomposition analysis (IDA) and structural decomposition analysis (SDA) are the two popularly used decomposition methods, which are different but related (Debone et al., 2021). The detailed description and review of the two decomposition methods can refer to the literatures of Hoekstra and Bergh (2003), Wang et al. (2017a).
However, as a type of economic accounting method, existing SDA and IDA studies cannot capture the impact of technical factors on carbon emissions (energy consumption) change. To solve this problem, Pasurka (2006) introduced the decomposition idea into the production framework for the first time, which can identify the impact of technological progress and technical efficiency changes on carbon emissions. On this basis, Zhou and Ang (2008) further introduced Shepherd directional distance function and defined it as the production-theoretical decomposition analysis (PDA) method. Compared with the SDA and IDA methods, the main advantage of PDA is that it can separate the technical effect from other driving factors and accurately depict the impact of technical factors on carbon (energy) intensity (Kim and Kim, 2012). Nevertheless, the PDA method is only applicable to the multiplicative decomposition form. Lin and Du (2014) solved the this limitation by combining IDA and PDA methods. The combination of IDA and PDA methods is an important supplement to the existing research and application (Wang et al., 2017b; Hang et al., 2019). Representative studies including Liu et al. (2018), which proposed a joint approach combined PDA and IDA and further decomposed carbon emissions change into nine drivers.
Using the above research methods (IDA, SDA, and PDA), from the perspective of carbon emission change influencing factors, the driving factors can mainly be summarized into three aspects: the structure effect (Bulut and Muratoglu, 2018), the technology effect (Sueyoshi et al., 2019), and the scale effect (Xiao et al., 2020). But for the importance of the three, studies from different research perspectives give different answers.
Specifically, the structural effect is mainly reflected in the impact of changes in energy structure and economic structure on carbon emissions. Wang et al. (2020) pointed that gradually increasing the proportion of alternative fuels and reducing the proportion of high carbon emission industries in the economy are the keys to achieving the carbon peaking and carbon neutrality in China. From the perspective of energy structure, Wang et al. (2019) indicated that reducing energy consumption is not a wise manner for sustainable society development, especially for those developing countries. Meanwhile, for the industry structure, the results of Liu et al. (2018) showed that at the same rate of economic growth, the better the industrial structure is adjusted, the larger the carbon emission “decline range” becomes. As to the technology effect, most existing studies showed that technological advances can reduce carbon emission from the source and improve carbon efficiency in the process, thus reducing carbon emissions (Hang et al., 2019; Sueyoshi et al., 2019). Representative example by Ang (2015), which combined environmental theory and modern endogenous growth model to study the influencing factors of carbon emission (intensity) from 1953 to 2006. The results show that there is a negative correlation between carbon emissions and the ability to digest and absorb advanced technologies. Wang et al. (2018) also proves that technological progress plays an important role in reducing carbon emissions; the rebound effect of carbon emissions reduces these positive effects. Meanwhile, economic development, investment level, population size, and trade level will affect the carbon emission through their scale changes. The conclusions of mainstream studies unanimously indicated that scale growth is the most important factor to promote carbon emissions (Sarkodie et al., 2020; Xiao et al., 2020).
However, the above related existing research results few deeply analyze the impact mechanism of production process on carbon emissions; in other words, they ignored the influence of regional technology heterogeneity in the analysis of factors affecting carbon emissions. Due to the differences in market conditions, legal constraints, resource endowments and openness, production technologies related to carbon emissions are often heterogeneous in different countries or regions (Calvo-Sotomayor et al., 2019; Wei and Liu, 2022). And this in turn will inevitably generate different effect on energy consumption and hence carbon emissions in different countries (Oh, 2010; Lisenkova et al., 2013; Wongboonsin and Phiromswad, 2017). For example, typically the level of carbon emission rises with the consumption of fossil fuels; it is therefore a natural conjecture to link carbon emissions to energy endowment and energy consumption structure of a region (Wei et al., 2018). Under such conditions, it is insufficient to put countries or regions with large differences under the same production technology frontier to measure carbon emission performance, which is not conducive to the realization of the principle of “differentiated responsibility” (Shuai et al., 2017; Wang et al., 2019). Hayami (1969) first proposed the concept of a Meta-frontier to solve the problems that may arise from a single production frontier1. Subsequently, the meta-frontier thought has been widely used in many fields (Wang and Feng, 2021; Xia et al., 2022). Therefore, combined with the meta-frontier and the decomposition analysis method, the heterogeneity related factors in the carbon emissions change can be effectively identifed. However, the relevant research is still relatively rare. From a policy development perspective, highlighting what drives carbon emission with taking into consideration distribution heterogeneity will be more practical significant (Udemba and Tosun, 2022b).
Based on the above research context, considering the heterogeneity of carbon emission process, a new comprehensive decomposition framework by combining production theoretical decomposition analysis (PDA) and meta-frontier data envelopment analysis is constructed to identify the influencing factors among different types of countries. Additionally, in order to provide a reference for the differentiated designing of carbon emission reduction policy for different countries, we further apply attribution analysis method to measure how different type countries contribute to each factor. Finally, empirical analysises are carried out based on the panel data of global representative 60 countries from 2007 to 2014, and the results can help policymakers to formulate targeted emission reduction plans.
Methodology
Production technology considering heterogeneity
Assuming that there are N countries as decision making units (DMUs), and each country invests capital (K), labor (L), and energy (E) to produce desirable output—gross domestic production (GDP, denoted by Y). Meanwhile, undesirable output is inevitable in the production process, such as representative CO2 emissions (C). According to Färe et al. (2007), the production technology set (T) of this production process can be expressed as Eq. 1.
Based on production theory, T is generally a closed and bounded set, assumed to have three mathematical properties, i.e., 1) all the inputs and desirable outputs are strongly disposable; 2) undesirable outputs are weakly disposable; 3) T has null-jointness2. However, T lacks an explicit form and cannot be used directly for empirical analysis. A popular way to overcome this problem is to introduce non-parametric data envelopment analysis (DEA) method to model the production technology, T (Oh, 2010). Following Färe et al. (2007), the production technology (under the constant return scale assumption) can be expressed as Eq. 2.
Where
The above production technology has been widely applied in the field of energy and environment research (Hang et al., 2019; Shironitta et al., 2019). However, constrained by the actual production process (such as differences in economy, geography, and market), it is not appropriate that all the DMUs share a common production technology (O'Donnell et al., 2008). Meta-frontier is generally used to describe the technical heterogeneity of different groups, and it has been widely used in the problem of regional production technology heterogeneity (Liu et al., 2022).
Following Liu et al. (2022), based on the differences in production technology, we first classify all the DMUs into H subgroups. The number of DMUs in the h-th group is Nh, and
Production-theoretical decomposition analysis method considering heterogeneity
Based on the extended Kaya identify (Liu et al., 2022), global carbon emission changes can be decomposed as follows:
where
According to Lin and Du (2014), changes in energy intensity are complicatedly influenced by the production process related factors, including the production heterogeneity between different countries. As such, this study introduced meta-frontier DEA technology into traditional PDA framework, the variation of carbon emissions in the i-th country in year t can be decomposed as follows:
Compared with Eq. 3, the ENI is further decomposed into four new factors in Eq. 4, i.e., the potential energy intensity (PEI), the production technology gap between group and meta-frontier (TEG), the group energy efficiency (GEF), and the group technology (GTC). As is shown in Figure 1, from the production heterogeneity perspective, the change of energy intensity not only reflects a country’s own technology and efficiency level, but also synthesizes the gap between itself and the external optimal frontier in the actual production process.
Therefore, according to the research by Wang et al. (2018), changes in carbon emissions from time t to t + 1 can be expressed as follows:
Combined with the Logarithmic Mean Divisia Index (LMDI) decomposition method, the contribution of each influencing factor in Eq. 5 can be accurately calculated. Supplementary Appendix S1A detailed expressed the entire calculation process. As such, the influencing factors of carbon emission changes during the period of t to t + 1 can be decomposed into six components and divided into three categories, summarized in Table 1.
Data description
Although the total global carbon emissions continued to grow, there were significant fluctuations between 2007 and 2014, so it is necessary to identify the key drivers of their anomalies. As such, the research period of this study spans from 2007 to 20143. And this study sample included 60 countries (listed in Supplementary Appendix S1B). Both economic volume and carbon emissions of these 60 countries all account for more than 80% of the global total, especially in 2014, accounting for 82.4% and 88.7%, respectively. Therefore, these countries can represent the overall development trend of global economic development and carbon emissions.
Following Liu et al. (2022), we assume the production process uses labor (collected from the International Labor Organization, ILO), real capital formation (collected from the World Bank, WB), and energy consumption (collected from IEA) to product GDP (collected from WB) and undesirable output CO2 emissions (collected from World Resource Institute, WRI). The variables for real capital formation and real GDP are subtracted from the 2000 consumer price index to eliminate price effects. The deflation is shown in Eqs 6, 7.
where
Following the idea of Liu et al. (2022), we choose energy intensity, a comprehensive index to measure energy and economy, to categorize the group boundaries. Specifically, 60 countries were divided into three types using K-Means cluster analysis (see Supplementary Appendix S1B)4. Group-L is composed of 14 countries, most of the countries in Group-L are large economic output countries with relatively large energy consumption (e.g., China and America); Group-M is composed of 20 countries, most of the countries in Group-M are middle scale economic countries with middle scale energy consumption (e.g., Sweden and Switzerland); Group-S is composed of 26 regions, most of the countries in Group-S are small scale economic countries with small scale energy consumption (e.g., Nepal and Uruguay).
Table 2 shows the statistical characteristics of the four key indicators in different groups. Obviously, the differences of these indicators between different groups are significant. Among them, Group-L had the highest average energy intensity value, followed by Group-S, and Group-M. This is mainly because Group-L includes developing economies with large levels of energy consumption, such as China, Russia, and Indonesia. Taking China as an example, China’s energy structure is dominated by coal, which heat conversion rate is relatively lower compared with other type energies. In addition, due to the limited technical level, the comprehensive utilization rate of energy is poorer than those developed countries. As a result, the energy intensity is relatively higher in China. This further proves the rationality and effectiveness of the grouping.
Results and analysis
Production heterogeneity
As illustrated in Figure 1, the production heterogeneity of carbon emissions in various countries is mainly reflected in the production process, involved in the energy intensity (EI). Figure 2 shows the changes in energy intensity in different types of countries. During the study period, EI has experienced a downward trend, decreasing from 0.251 kg/dollar in 2007 to 0.204 kg/dollar in 2014. From 2008 to 2009, due to the impact of the financial crisis, economic development experienced a sharp decline, but energy consumption only slightly decreased. Therefore, EI has increased. As mentioned above, the 60 sample countries can be divided into three categories. From the group perspective, Group-S shows the highest EI, followed by Group-L and Group-M. This is mainly because the fact that Group-S is basically composed of developing countries. Compared with the other two groups, the modes of energy consumption and economic development of those countries in Group-S were relatively extensive. This is consistent with the study by Zhang and Wang (2015).
Further, to reveal the EI evolution process in the different group countries, the standard
where
The
As shown in Table 3, within the three group countries, and the overall sample, the estimated coefficients of
According to the decomposition framework constructed above, differences in energy use technology and energy efficiency are the core factors that affect the production heterogeneity. Figure 3 illustrates the time series change of different type countries’ energy use efficiency and energy use technologies.
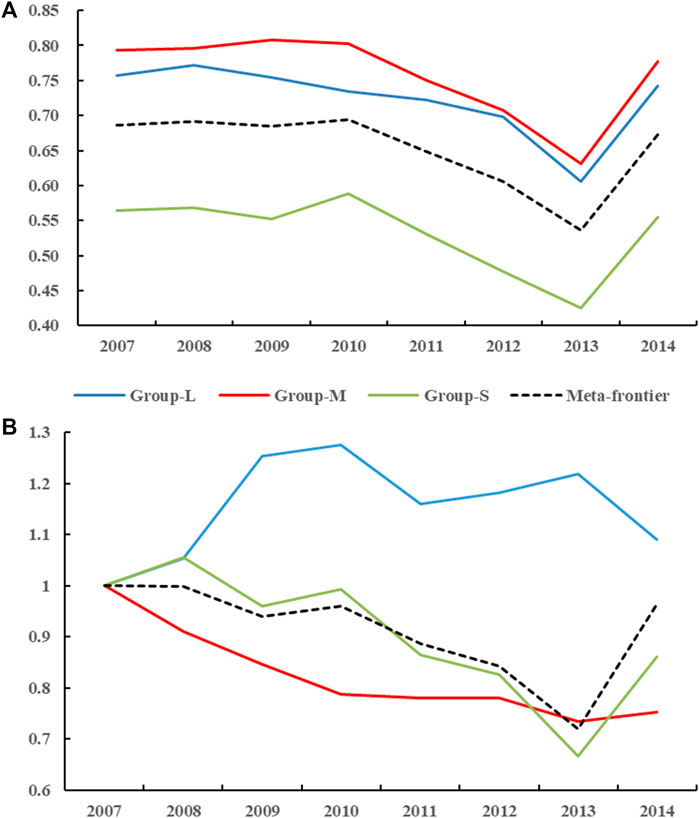
FIGURE 3. Time series changes of energy efficiency (A) and energy technology (B) of different groups in 2007–2014.
As shown in Figure 3A, the energy efficiency of the sample countries has decreased in a fluctuation way, and rebounded after reaching the lowest value in 2013. This is mainly because since 2007, countries have paid more attention to economic output scale and neglected process optimization in order to get rid of the impact of the financial crisis as soon as possible (Wei et al., 2018). Taking Indonesia as an example, in addition to the relatively lower management level, the industrial structure of high energy consumption has not been reasonably optimized, resulting in a 29% decline in energy efficiency. For different types of countries, Group-M shows the best energy efficiency, followed by Group-L and Group-S. This is mainly due to the fact that Group-M is basically composed of developed countries that consume relatively less energy, having enough advantages (talent, management, and capital investment) to improve energy efficiency.
Compared with the trend of efficiency change, the heterogeneity of technology change is relatively greater (see Figure 3B). With the exception of Group-L, the other two groups have contributed positively to the overall technology improvement. From 2007 to 2012, the cumulative technology change rate of Group-M was relatively more significant. Compared with the other two groups of countries, the countries in Group-L, despite their large energy consumption, are in a leading position in terms of energy use technology and emission reduction technology, with little room for improvement. For example, many OECD countries have shifted from relying on energy intensive manufacturing industries to using less energy intensive service based economic activities. In contrast, most countries in Group-S are developing countries, technology promotion potential and space are relatively large, and the impact is more pronounced. Although Group-L countries are world leaders in energy use technology, a good sign is that the other countries are catching-up continuously.
Decomposition results and discussion
During the study period, the carbon emissions of the sample countries showed a significant increasing trend, with an average annual growth rate of 1.78%. Using the decomposition analysis model constructed above, we can explore the driving factors of global carbon emissions changes from three aspects: energy input, production process and economic output. Table 4 presents the single-period decomposition results of the six influencing factors from 2007 to 2014. As is shown in Table 4, ECA has an enhanced effect on carbon emissions over the years, with an average annual growth rate of 1.084, which is the main obstacle for the decline of carbon emissions. Meanwhile, ENS and ENI both played positive roles in the carbon emission reduction (G-mean values are all less than 1). The findings are consistent with other studies on changes in global carbon emissions (e.g., Wang et al., 2020; Liu et al., 2022).
Energy intensity, an indicator reflecting changes in energy production technology, is the most important factor to curb the growth of carbon emissions, with an average annual contribution of 0.9396. Further decomposition of ENI showed that PEI and GEF were the key factors contributing to the reduction of carbon emissions, with the annual contribution rates of 0.933 and 0.999, respectively. However, during the period of 2008–2009, PEI not only failed to reduce carbon emissions, but also promoted the increase of carbon emissions (1.024). This phenomenon may be caused by the impact of the Asian financial crisis, which has greatly affected the investment in energy substitute (such as labor shortage and insufficient capital investment), thus consuming more energy at the same output level. This is consistent with the research conclusion by Zhang and Wang (2015). Since 2009, in order to get rid of the impact of energy shortage and economic crisis, major energy consuming countries around the world (such as the United States, China, and Russia) have taken more measures to conduct the energy saving and emission reductions. For example, China has invested more than 100 billion yuan in supporting the research, development, promotion and the application of low-carbon technologies in 2010. Thus, strengthening the role of PEI and ENI in reducing carbon emissions.
Figure 4 illustrates the multi-period decomposition results of the six influencing factors from 2007 to 2014. The average effects of GEF and GTC change on global carbon emission were 0.999 and 1.001, respectively. This reflects that neither production technology nor production efficiency can effectively curb the growth of carbon emissions. Especially for GTC, it was second only to ECA in its contribution to the growth of carbon emissions from 2007 to 2012 (see Figure 4). The good phenomenon is that, since 2012, GTC has continuously played an active role in reducing carbon emissions. This may be that global countries are gradually getting rid of the impact of the economic crisis, and energy-saving and emission reduction technologies have developed rapidly. For the GEF, except for 2007–2009 and 2012–2013, the emission reduction effect was not significant in other years. This indicates that there is still great potential in improving energy efficiency to achieve emission reduction.
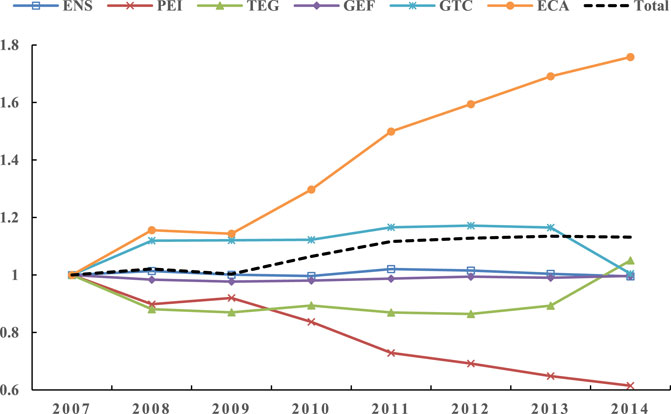
FIGURE 4. Cumulative changes of sample countries’ carbon intensity and its decomposition, 2007–2014.
As for TEG, reflecting the production heterogeneity, it has significantly promoted the carbon emission increasing. And its promoting effect is second only to ECA. From the perspective of evolutionary trend, Table 4 and Figure 4 show that the change trend of TEG is almost opposite to that of global carbon emissions. The greater the heterogeneity of production technology between different countries, the more uneven the production level, which will increase global carbon emissions. Therefore, countries should further emphasize exchange and learning from each other, thus narrowing the gap between countries with different energy use technology levels.
From the perspective of grouping, because different types of countries have obvious differences in resource endowment, production environment, technology level, management experience and other aspects, the impact degree and mechanism of carbon emission change factors on different types of countries should also be different (Wang et al., 2019). Single period decomposition results in different groups are summarized in Supplementary Appendix S1C. Figure 5 describes the cumulative decomposition results for sample countries’ carbon emission change by different factors of different groups.
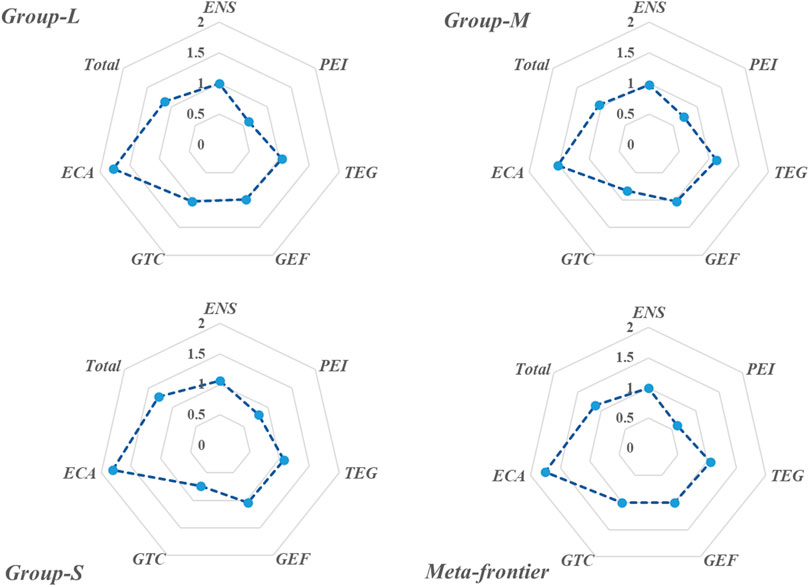
FIGURE 5. The cumulative decomposition results for sample countries’ carbon emission change by different factors of different groups.
On the whole, countries in Group-S experienced the most obvious growth rate (1.288), followed by Group-L (1.136) and Group-M (1.046). As for the specific influencing factors, ECA is the dominant factor that promotes the increase of carbon emissions for all types of countries. Meanwhile, PEI has effectively helped all types of countries reduce their carbon emissions, and PEI is the most important emission reduction factor for Group-L and Group-M. For Group-S, the contribution of GTC (0.739) to carbon emission reduction is more obvious than PEI (0.812). This is due to the fact that Group-L and Group-M are mainly developed countries, and there are significant advantages in the application of low-carbon production technology. While Group-S is mainly composed of developing countries with relatively low energy consumption, which low carbon production technology has not been widely promoted and applied. As such, countries in Group-S generally have more room for technological progress, leading the more significant carbon emission reduction effect of GTC.
As one of the main factors contributing to the increase in carbon emissions, TEG contributed 12.6% to the growth of carbon emission in Group-M, followed by Group-S (7.64%) and Group-L (4.35%). The greater the gap, the more backward the energy-saving and emission reduction technology is, the greater the contribution to carbon emissions. The TEG effect has the least impact on the change of carbon emissions in developed countries with large energy consumption. This is mainly due to the fact that Group-L type countries generally have always been in the leading position in production technology and are the “promoters” of the global technological progress. Thus, compared with other type countries, the technology gap between Group-L and global technology frontier is relatively smaller, leading to the less impact on the change of carbon emissions in Group-L countries. This further indicates that different types of countries should strengthen cooperation and exchange on production technology to narrow the technological gap, thus decreasing the carbon emissions.
Meanwhile, the effects of energy efficiency (GEF) and energy structure (ENS) on the carbon emission of different groups were relatively small. Affected by resource endowment and energy price, there is no obvious difference in energy structure among countries at present, and the optimization of energy use structure is a long-term process. In addition, the improvement of energy efficiency involves a series of long-term reserves such as management level, experience accumulation and talent training. As such, in the short term, the impact of energy efficiency and energy structure change on carbon emission change is not significant.
Attribution results and discussion
After understanding how different factors drive the change of carbon emissions, how different type countries contribute to each factor should also be clarified. This can provide a reference for the differentiated designing of carbon emission reduction policy for different countries. Attribution analysis method, proposed by Choi and Ang (2012), can effectively realize this purpose. Taking “the potential energy intensity (PEI)” as an example, the contribution of each country to PEI can be calculated by using Eq. 9.
where
During the study period, different types of countries made different contributions to the change of carbon emissions in different years. The overall carbon emissions of the sample countries increased by 13.15% from 2007 to 2014, the carbon emission of Group-S increased by 28.81%, followed by Group-L (13.60%) and Group-M (4.63%). During 2008–2009 and 2013–2014, the overall carbon emissions decreased 1.75% and 0.30%, respectively. This is mostly because, during these two periods, most of the sample countries promoted the decline of carbon, especially for the leading role that Group-L played. In order to further explore the core driving force of the contribution of different types of countries to carbon emissions, Supplementary Appendix S1D depicts the cumulative contribution of different groups to the influencing factors of carbon emission change. Specifically, sample countries with different development modes and stages have different contributions to carbon emission across the four drivers (i.e., PEI, TEG, GEF, and GTC), as shown in Figure 6.
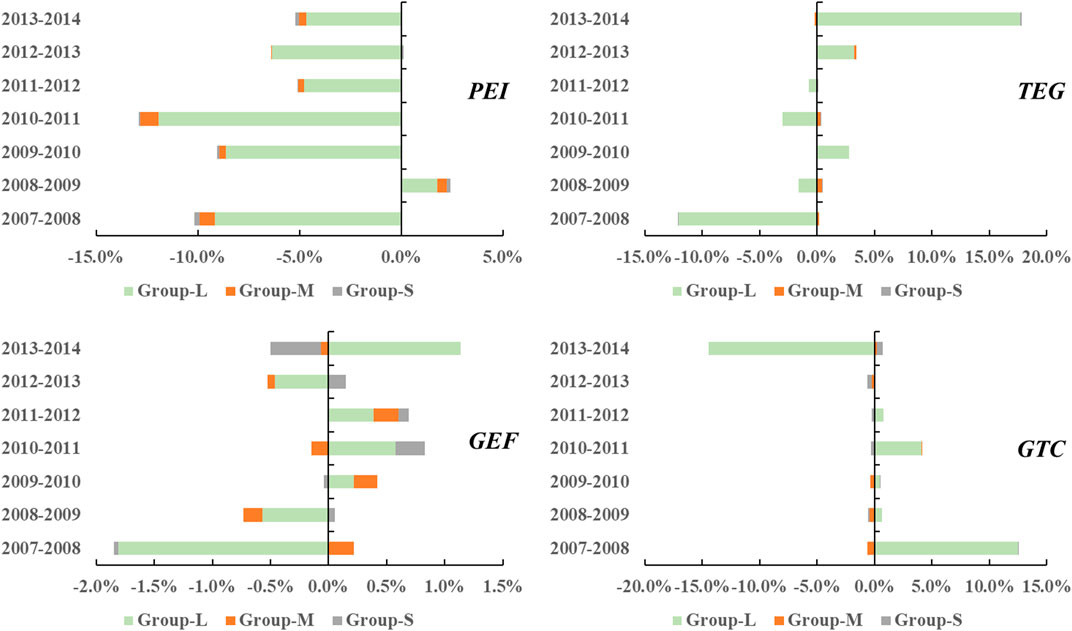
FIGURE 6. Percentage of the single-stage attribution results of the production process (PEI, TEG, GEF, and GTC) of each grouped country from 2007 to 2014 (Unit: %).
For PEI, from 2007 to 2014, except for 2008–2009, the cumulative contribution value of each country to PEI was promoting carbon emission reduction, of which Group-L was the most obvious, especially in 2010–2011 (−11.95%), 2007–2008 (−9.17%), and 2009–2010 (−8.64%). In particular, for 2012–2013 (0.12%), Group-S did not contribute to PEI reduction. This may be due to the fact that, in addition to the impact of economic environment, the energy consumption of Group-S countries is relatively small, and the decline of energy prices has a greater impact on the growth of total energy consumption. The attribution effect of each group to the GEF is almost the opposite to that of PEI. This is because the effect of PEI has a direct internal relationship with the effect of GEF. The economic connotation of PEI represents the energy intensity that peels off the ineffectiveness of energy use. When the overall energy intensity of a country changes little, the attribution effects of PEI and GEF thus have a reverse trend.
Meanwhile, for GTC, from 2007 to 2013, Group-L has always been the main body to increase the carbon emissions by GTC, with an average annual contribution rate of 3.09%. Of which, Group-L made the most significant contribution in 2007–2008 and 2010–2011. Different from Group-L, both Group-M and Group-S significantly reduce the carbon emissions of GTC. This is mainly due to the effective improvement of energy use technology in these two types of countries. Considering the fact that, with the continuous improvement of energy use technology, the technology gap will inevitably continue to narrow. As such, the attribution effect of each group to the TEG is almost the opposite to that of GTC (see Figure 6).
Conclusion
Identifying the key factors affecting the change of global carbon emissions can help targeted carbon emission reduction and curb global warming. Meanwhile, knowing the contribution degree of different countries to the influencing factors can help better share the emission reduction responsibility. Therefore, this study takes 60 representative countries as samples to decompose and attribute the changes of carbon emissions from 2007 to 2014. In particular, considering the obvious differences in resource endowment, production technology and management level among different countries, this study proposed a new decomposition analysis framework by combining PDA and Meta-frontier analysis. The newly built method can be used to further explore the impact of production technology heterogeneity on carbon emission reduction. In general, three important findings emerged from the empirical study.
First, the production process of different type countries has clear heterogeneity characteristics. The energy intensity level of different countries has a trend of
Second, the decomposition analysis shows that potential energy intensity effect (PEI) is the most important reason for carbon emission reduction. Meanwhile, group energy efficiency effect (TGE), and energy structure effect (ENS) also played positive roles in carbon emission reduction. The other factors, including group technology change effect (GTC), technology gap effect (TEG), and economic activity effect (ECA) failed to inhibit carbon emissions. In particular, ECA has an enhanced effect on carbon emissions with an average annual increase rate of 1.084, which was the main driver to the increase of carbon emissions during the study period. From group perspective, ECA had the most significant pull effect on carbon emissions in Group-S, with a cumulative effect of 1.806, followed by Group-L (1.777) and Group-M (1.531). This suggests that developing countries and those with large energy consumption countries should pay more attention to the carbon reduction in the process of economic development. For example, these two types countries can reduce the impact of economic activity on carbon emissions through industrial structure adjustment, new energy application and other ways when making economic development policies.
Third, in the production process, the structure of the contribution coefficient shows different characteristics in different groups. The cumulative contribution value of each country to PEI was a promoting to carbon emission reduction, of which Group-L was the most obvious, especially in 2010–2011 (−11.95%), 2007–2008 (−9.17%) and 2009–2010 (−8.64%). Meanwhile, the attribution effect of each group to the GEF is almost the opposite to that of PEI. For GTC, from 2007 to 2013, Group-L has always been the main body to increase the carbon emissions by GTC, with an average annual contribution of 3.09%. Different from Group-L, Group-M and Group-S both played significant role in decreasing the carbon emissions by GTC. The attribution effect of each group to the TEG is almost the opposite to that of GTC. A country must be concerned not only with its own technological progress, but also with the development of global technology frontier and the pace of technological progress.
Data availability statement
The datasets presented in this study can be found in online repositories. The names of the repository/repositories and accession number(s) can be found in the article/Supplementary Material.
Author contributions
XL contributed to the conception of the study and performed the data analyses and wrote the manuscript. YZ contributed significantly to analysis and manuscript preparation. QW helped perform the analysis with constructive discussions.
Funding
This research was financially supported by the National Natural Science Foundation of China (No. 71904059), the Major Programme of National Social Science Foundation of China (No. 21&ZD110), and the Qin Lan Project of the Jiangsu province.
Conflict of interest
The authors declare that the research was conducted in the absence of any commercial or financial relationships that could be construed as a potential conflict of interest.
Publisher’s note
All claims expressed in this article are solely those of the authors and do not necessarily represent those of their affiliated organizations, or those of the publisher, the editors and the reviewers. Any product that may be evaluated in this article, or claim that may be made by its manufacturer, is not guaranteed or endorsed by the publisher.
Supplementary material
The Supplementary Material for this article can be found online at: https://www.frontiersin.org/articles/10.3389/fenvs.2022.1062500/full#supplementary-material
Footnotes
1The basic idea of meta-frontier is that all the decision making units (DMUs) can be divided into different groups according to their inherent attributes, such as region, type, scale, etc. Each group forms a group production frontier. As such, a meta-frontier is obtained by enveloping all the group production frontier of different groups.
2Details see the research by Liu et al. (2022).
3Another reason is that, some representative studies have done the relevant studies and focused on this period (such as Liu et al., 2022; Duan et al., 2022), facilitating our comparative analysis.
4Some articles use geography to divide countries into different groups (Oh, 2010). However, Lin and Du (2013) proved that a country’s technological level has no direct relationship with its geography. As for the number of clusters, following the idea of Tibshirani et al. (2001), we adopted the Gap Statistic algorithm. And the results show that when K = 3, the value of Gap Statistic is the largest. Therefore, the 60 sample countries were divided into three categories in this study.
References
Acheampong, A. O., and Boateng, E. B. (2019). Modelling carbon emission intensity: Application of artificial neural network. J. Clean. Prod. 225, 833–856. doi:10.1016/j.jclepro.2019.03.352
Ajmi, A. N., Hammoudeh, S., Nguyen, D. K., and Sato, J. R. (2015). On the relationships between CO2 emissions, energy consumption and income: The importance of time variation. Energy Econ. 49, 629–638. doi:10.1016/j.eneco.2015.02.007
Ang, B. W. (2015). LMDI decomposition approach: A guide for implementation. Energy Policy 86, 233–238. doi:10.1016/j.enpol.2015.07.007
Barro, J., and Sala-i-Martin, X. (1992). Public finance in models of economic growth. Rev. Econ. Stud. 59, 645–661. doi:10.2307/2297991
Bulut, U., and Muratoglu, G. (2018). Renewable energy in Turkey: Great potential, low but increasing utilization, and an empirical analysis on renewable energy-growth nexus. Energy Policy 123, 240–250. doi:10.1016/j.enpol.2018.08.057
Calvo-Sotomayor, I., Laka, J. P., and Aguado, R. (2019). Workforce ageing and labour productivity in europe. Sustainability 11 (20), 5851. doi:10.3390/su11205851
Chen, J. (2021). Carbon neutrality: Toward a sustainable future. Innovation 2, 100127. doi:10.1016/j.xinn.2021.100127
Choi, K. H., and Ang, B. W. (2012). Attribution of changes in Divisia real energy intensity index-an extension to index decomposition analysis. Energy Econ. 34, 171–176. doi:10.1016/j.eneco.2011.04.011
Debone, D., Leite, V., and Miraglia, S. G. E. K. (2021). Modelling approach for carbon emissions, energy consumption and economic growth: A systematic review. Urban Clim. 37, 100849. doi:10.1016/j.uclim.2021.100849
Dong, F., Long, R., Li, Z., and Dai, Y. (2016). Analysis of carbon emission intensity, urbanization and energy mix: Evidence from China. Nat. Hazards 82, 1375–1391. doi:10.1007/s11069-016-2248-6
Duan, C., Zhu, W., Wang, S., and Chen, B. (2022). Drivers of global carbon emissions 1990–2014. J. Clean. Prod. 371, 133371. doi:10.1016/j.jclepro.2022.133371
Färe, R., Grosskopf, S., and Pasurka, C. A. (2007). Pollution abatement activities and traditional productivity. Ecol. Econ. 62, 673–682. doi:10.1016/j.ecolecon.2006.08.014
Fuchs, R., Brown, C., and Rounsevell, M. (2020). Europe’s Green Deal offshores environmental damage to other nations. Nature 586, 671–673. doi:10.1038/d41586-020-02991-1
Goh, T., Ang, B., and Xu, X. (2018). Quantifying drivers of CO2 emissions from electricity generation Current practices and future extensions. Appl. Energy 231, 1191–1204. doi:10.1016/j.apenergy.2018.09.174
Hang, Y., Wang, Q., Wang, Y., Su, B., and Zhou, D. (2019). Industrial SO2 emissions treatment in China: A temporal-spatial whole process decomposition analysis. J. Environ. Manag. 243, 419–434. doi:10.1016/j.jenvman.2019.05.025
Hayami, Y. (1969). Sources of agricultural productivity gap among selected countries. Am. J. Agric. Econ. 51 (3), 564–575. doi:10.2307/1237909
Hoekstra, R., and Bergh, J. (2003). Comparing structural decomposition analysis and index. Energy Econ. 25, 39–64. doi:10.1016/s0140-9883(02)00059-2
Huang, Q., Zheng, H., Li, J., Meng, J., Liu, Y., Wang, Z., et al. (2021). Heterogeneity of consumption-based carbon emissions and driving forces in Indian states. Adv. Appl. Energy 4, 100039. doi:10.1016/j.adapen.2021.100039
Huo, C., Hameed, J., Sharif, A., Albasher, G., Alamri, O., Alsultan, N., et al. (2022). Recent scenario and nexus of globalization to CO2 emissions: Evidence from wavelet and Quantile on Quantile Regression approach. Environ. Res. 212, 113067. doi:10.1016/j.envres.2022.113067
Intergovernmental Panel on Climate Change, (2021). “Climate change 2021: The physical science basis,”. Contribution of Working Group I to the Sixth Assessment Report of the Intergovernmental Panel on Climate Change (Geneva, NY, USA: Intergovernmental Panel on Climate Change).
Intergovernmental Panel on Climate Change, (2022). “Climate change 2022: Mitigating climate change,”. Contribution of Working Group III to the Sixth Assessment Report of the Intergovernmental Panel on Climate Change (Cambridge, UK and New York, NY, USA: Cambridge University Press). doi:10.1017/9781009157926
Kim, K., and Kim, Y. (2012). International comparison of industrial CO2 emission trends and the energy efficiency paradox utilizing production-based decomposition. Energy Econ. 34, 1724–1741. doi:10.1016/j.eneco.2012.02.009
Lin, B., and Du, K. (2014). Decomposing energy intensity change: A combination of index decomposition analysis and production-theoretical decomposition analysis. Appl. Energy 129, 158–165. doi:10.1016/j.apenergy.2014.04.101
Lin, B., and Du, K. (2013). Technology gap and China’s regional energy efficiency: A parametric metafrontier approach. Energy Econ. 40, 529–536. doi:10.1016/j.eneco.2013.08.013
Lin, B., and Zhu, J. (2021). Impact of China's new-type urbanization on energy intensity: A city-level analysis. Energy Econ. 99, 105292. doi:10.1016/j.eneco.2021.105292
Lisenkova, K., M´erette, M., and Wright, R. (2013). Population ageing and the labor market: Modelling size and age-specific effects. Econ. Model. 35, 981–989. doi:10.1016/j.econmod.2013.09.007
Liu, X., Hang, Y., Wang, Q. W., Chiu, C., and Zhou, D. (2022). The role of energy consumption in global carbon intensity change: A meta-frontier-based production-theoretical decomposition analysis. Energy Econ. 109, 105968. doi:10.1016/j.eneco.2022.105968
Liu, X., Zhou, D., Zhou, P., and Wang, Q. (2018). Factors driving energy consumption in China: A joint decomposition approach. J. Clean. Prod. 172, 724–734. doi:10.1016/j.jclepro.2017.10.238
Long, H., Xie, R., Gao, C., Sun, M., and Su, B. (2022). Analysis of China's regional differences in water consumption based on spatial structural decomposition analysis model. Sci. Total Environ. 848, 157629. doi:10.1016/j.scitotenv.2022.157629
O'Donnell, C. J., Rao, D. S. P., and Battese, G. (2008). Metafrontier frameworks for the study of firm-level efficiencies and technology ratios. Empir. Econ. 34, 231–255. doi:10.1007/s00181-007-0119-4
Oh, D. H. (2010). A metafrontier approach for measuring an environmentally sensitive productivity growth index. Energy Econ. 32, 146–157. doi:10.1016/j.eneco.2009.07.006
Pasurka, C. A. (2006). Decomposing electric power plant emissions within a joint production framework. Energy Econ. 28 (1), 26–43. doi:10.1016/j.eneco.2005.08.002
Sarkodie, S. A., Adams, S., Owusu, P. A., Leirvik, T., and Ozturk, I. (2020). Mitigating degradation and emissions in China: The role of environmental sustainability, human capital and renewable energy. Sci. Total Environ. 719, 137530. doi:10.1016/j.scitotenv.2020.137530
Shao, S., Yang, L., Gan, C., Cao, J., Geng, Y., and Guan, D. (2016). Using an extended LMDI model to explore techno-economic drivers of energy-related industrial CO2 emission changes: A case study for shanghai (China). Renew. Sustain. Energy Rev. 55, 516–536. doi:10.1016/j.rser.2015.10.081
Shironitta, K., Okamoto, S., and Kagawa, S. (2019). Cross-country analysis of relationship between material input structures and consumption-based CO2 emissions. Environ. Econ. Policy Stud. 21, 533–554. doi:10.1007/s10018-019-00241-x
Shuai, C., Shen, L., Jiao, L., Wu, Y., and Tan, Y. (2017). Identifying key impact factors on carbon emission: Evidences from panel and time-series data of 125 countries from 1990 to 2011. Appl. Energy 187, 310–325. doi:10.1016/j.apenergy.2016.11.029
Su, B., and Ang, B. W. (2012). Structural decomposition analysis applied to energy and emissions: Some methodological developments. Energy Econ. 34, 177–188. doi:10.1016/j.eneco.2011.10.009
Sueyoshi, T., Li, A., and Liu, X. (2019). Exploring sources of China's CO2 emission: Decomposition analysis under different technology changes. Eur. J. Operational Res. 279, 984–995. doi:10.1016/j.ejor.2019.06.037
Tibshirani, R., Walther, G., and Hastie, T. (2001). Estimating the number of clusters in a data set via the gap statistic. J. R. Stat. Soc. Ser. B 63 (2), 411–423. doi:10.1111/1467-9868.00293
Udemba, E. D., and Tosun, M. (2022a). Energy transition and diversification: A pathway to achieve sustainable development goals (SDGs) in Brazil. Energy 239, 122199. doi:10.1016/j.energy.2021.122199
Udemba, E. D., and Tosun, M. (2022b). Moderating effect of institutional policies on energy and technology towards a better environment quality: A two dimensional approach to China's sustainable development. Technol. Forecast. Soc. Change 183, 121964. doi:10.1016/j.techfore.2022.121964
Wang, H., Ang, B. W., and Su, B. (2017a). A multi-region structural decomposition analysis of global CO2 emission intensity. Ecol. Econ. 142, 163–176. doi:10.1016/j.ecolecon.2017.06.023
Wang, H., Ang, B. W., and Su, B. (2017b). Assessing drivers of economy-wide energy use and emissions: IDA versus SDA. Energy Policy 107, 585–599. doi:10.1016/j.enpol.2017.05.034
Wang, M., and Feng, C. (2021). The consequences of industrial restructuring, regional balanced development, and market-oriented reform for China’s carbon dioxide emissions: A multi-tier meta-frontier DEA-based decomposition analysis. Technol. Forecast. Soc. Change 164, 120507. doi:10.1016/j.techfore.2020.120507
Wang, Q., Hang, Y., Su, B., and Zhou, P. (2018). Contributions to sector-level carbon intensity change: An integrated decomposition analysis. Energy Econ. 70, 12–25. doi:10.1016/j.eneco.2017.12.014
Wang, S., Wen, Z., Chen, B., Feng, K., Zhu, X., and Song, D. (2019). Drivers of CO2 emissions from power generation in China based on modified struc-tural decomposition analysis. J. Clean. Prod. 220, 1143–1155. doi:10.1016/j.jclepro.2019.02.199
Wang, Z., Chen, H., Huo, R., Wang, B., and Zhang, B. (2020). Marginal abatement cost under the constraint of carbon emission reduction targets: An empirical analysis for different regions in China. J. Clean. Prod. 249, 119362. doi:10.1016/j.jclepro.2019.119362
Wei, L., and Liu, Z. (2022). Spatial heterogeneity of demographic structure effects on urban carbon emissions. Environ. Impact Assess. Rev. 95, 106790. doi:10.1016/j.eiar.2022.106790
Wei, T., Zhu, Q., and Glomsrød, S. (2018). How will demographic characteristics of the labor force matter for the global economy and carbon dioxide emissions? Ecol. Econ. 147, 197–207. doi:10.1016/j.ecolecon.2018.01.017
Wongboonsin, K., and Phiromswad, P. (2017). Searching for empirical linkages between demographic structure and economic growth. Econ. Model. 60, 364–379. doi:10.1016/j.econmod.2016.09.023
Xia, Y., Sun, L., and Feng, C. (2022). What causes spatial inequalities of low-carbon development in China’s transport sector? A newly proposed meta-frontier DEA-based decomposition approach. Socio-Economic Plan. Sci. 80, 101151. doi:10.1016/j.seps.2021.101151
Xiao, H., Sun, K. J., Tu, X. W., Bi, H. M., and Wen, M. (2020). Diversified carbon intensity under global value chains: A measurement and decomposition analysis. J. Environ. Manag. 272, 111076. doi:10.1016/j.jenvman.2020.111076
Zhang, N., and Wang, B. (2015). A deterministic parametric metafrontier luenberger indicator for measuring environmentally-sensitive productivity growth: A Korean fossil-fuel power case. Energy Econ. 51, 88–98. doi:10.1016/j.eneco.2015.06.003
Zhou, P., and Ang, B. W. (2008). Decomposition of aggregate CO2 emissions: A production-theoretical approach. Energy Econ. 30, 1054–1067. doi:10.1016/j.eneco.2007.10.005
Zhu, Z., Liao, H., Cao, H., Wang, L., Wei, Y. M., and Yan, J. (2014). The differences of carbon intensity reduction rate across 89 countries in recent three decades. Appl. Energy 113, 808–815. doi:10.1016/j.apenergy.2013.07.062
Nomenclature
Abbreviations
CO2 carbon dioxide
GDP gross domestic product
DEA data envelopment analysis
DMU decision making unit
ENS energy consumption structure
ENI energy intensity
ECA economic activity
PEI potential energy intensity
TEG production technology gap between group and meta-frontier
GTC group technology
PDA production-theoretical decomposition analysis
GEF group energy efficiency
Group-L large economic output countries with large energy consumption
Group-M medium economic output countries with medium energy consumption
Group-S small economic output countries with small energy consumption
LMDI logarithmic mean divisia index
SDA structural decomposition analysis
IDA index decomposition analysis
Variables
Parameters
Keywords: carbon emissions, production-theoretical decomposition analysis, heterogeneity, meta-frontier, attribution analysis
Citation: Liu X, Zhang Y and Wang Q (2022) Drivers of global carbon emission changes: A heterogeneity perspective of decomposition and attribution analysis. Front. Environ. Sci. 10:1062500. doi: 10.3389/fenvs.2022.1062500
Received: 06 October 2022; Accepted: 21 November 2022;
Published: 01 December 2022.
Edited by:
Kangyin Dong, University of International Business and Economics, ChinaReviewed by:
Edmund Udemba, Gelişim Üniversitesi, TurkeySedat Alatas, National Research University Higher School of Economics, Russia
Copyright © 2022 Liu, Zhang and Wang. This is an open-access article distributed under the terms of the Creative Commons Attribution License (CC BY). The use, distribution or reproduction in other forums is permitted, provided the original author(s) and the copyright owner(s) are credited and that the original publication in this journal is cited, in accordance with accepted academic practice. No use, distribution or reproduction is permitted which does not comply with these terms.
*Correspondence: Xiao Liu, liuxiao@hytc.edu.cn