- 1Yunnan Normal University Pan-Asia Business School, Kunming, China
- 2Central South University of Forestry and Technology, Changsha, China
For environmental sustainability and resource security, the global energy system requires a revolutionary transition from traditional energy to green energy resources. Therefore, this study investigates the influence of economic policy uncertainty, technological innovation, ecological governance, and economic growth on the green energy transition in China. We employed a bootstrap auto-regressive distributive lag (BARDL) model to evaluate the long-run association between the study variables from Q1-2000 to Q4-2020. The preliminary finding confirms the long-run cointegration relationship among model variables. The results show that economic policy uncertainty and economic growth negatively derive green energy transition in the long-run. In contrast, technology innovation and environmental governance positively influence the green energy transition. These findings propose strengthening of the environmental governance mechanism and technology innovation to accelerate the green energy transition in China.
1 Introduction
In recent years, the energy sector has experienced unprecedented dynamism due to the advent of green energy resources. Although the technology implementation transforms the old energy system into a more efficient one, on a global scale, the share of green energy in the overall energy mix is still minimal (Sun et al., 2022). Therefore, the green energy transition is at the forefront of the economic discourse due to environmental policy priorities such as carbon neutrality, energy conservation, and energy efficiency (Graham et al., 2021). Green energy resources play a significant role in environmental sustainability (Mohideen et al., 2021). At the same time, they cemented its contribution to economic stability by creating a job. The remarkable resilience of green energy shifted it from the niche to the mainstream and supported the highly polluted countries to embrace their immense pledges of carbon neutrality to achieve the goal of global temperature reduction (Gielen et al., 2019). The main pillars of the green energy transaction are green or renewable energy resources, electrification, and energy efficiency (Vanegas Cantarero, 2020). Two crucial actions are required to obtain the energy transaction: first, the high implication of cost-effective technologies to promote green energy consumption and second, the adoption of electrification for the application of end-users by providing an efficient green energy supply (De La Peña et al., 2022).
China, the world’s largest coal consumer and the highest greenhouse gas emitter, faces numerous challenges in the energy transition despite its “13th Five-Year Plan” for “Renewable Energy Development” (S. Xu, 2021). According to the recent status report of China’s energy transaction, the green energy transition (GET) was progressively slow. Figure 1 presents China’s green energy transition progress in the last 20 years. It can be observed that from 2014 to 2020, the progress shows no improvement in terms of the energy transaction index, which measures the three dimensions of energy availability, sustainability, and efficiency.
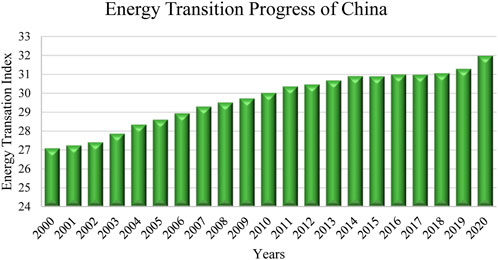
FIGURE 1. China’s progress in the energy transition. Source: United Nations Conference on Trade and Development (UNCTAD).
Based on the data from China’s energy transition status report 2021, the total mitigation of CO2 emission has reached 14.1 Gt, wherein the CO2 emission through fossil fuels and biomass has reduced by 5% and 8%, respectively. Renewable energy resources accounted for 14.3% of the total energy generation in 2021 compared to 15.9% in 2020. In contrast, energy efficiency increased and reached 13.5% in 2021. The electrification of the end-user (such as electrifying transportation, heating, and cooling system through green resources) was recorded as 10.3% in the year 2021, while green hydrogen (hydrogen produced by splitting water into hydrogen and oxygen using renewable electricity) contributed 7% to the total green energy transition (Irfan et al., 2022).
Based on the aforementioned statistics, the main challenges are the curtailment of renewable energy (reduction in the output of wind, solar, or hydropower generators) and substantial fossil fuel subsidies provided by China, considering the price gap approach. Therefore, coal remained the primary energy source for industries and electricity production in China (Hove et al., 2021). The existing literature has identified various socio-economic factors which shape the scope and pace of energy transition, such as oil price shocks (Z. Fu et al., 2022; Jiang et al., 2021) and renewable energy consumption (Murshed & Tanha, 2021), relevant legislation acts, and awareness (Pietrzak et al., 2021), economic complexity, and globalization (Sun et al., 2022). However, to achieve the full potential of GET, the role of economic policy uncertainty (EPU), technology innovation (TIN), and ecological governance (EGV) are still inconclusive, which ejected the worldwide debate among researchers and policymakers.
Economic policy uncertainty (EPU) is the “uncertainty associated with public policies, especially fiscal and monetary policies that affect the business environment of the economy” (Aastveit et al., 2017). In energy and environmental economics, EPU is measured by the widely used economic policy uncertainty index (Baker et al., 2016). The EPU impact on green energy consumption may differ due to other economic factors. When the economy heavily relies on energy and faces the high environmental consequence of energy consumption, the EPU depresses the green energy transition. For instance, Ivanovski and Marinucci (2021) and X. Zhou et al. (2022) found that EPU adversely affects the GET by creating uncertainty in investment behavior. Moreover, EPU hampered the investors’ confidence and spurred systematic risk, thereby inflating the cost of capital (Z. Xu, 2020). Similarly, the increase in EPU causes high price volatility in energy resources and subsequently enhances the demand for cheap energy resources. In addition to this, the high EPU significantly reduced the investment in innovations and R&D activities. Thus the optimal energy-related policies and investment choices are hindered by the level of EPU in the economy (Pirgaip & Dinçergök, 2020). Aastveit et al. (2017) and Amin and Dogan (2021) argued that the EPU promotes the consumption of green energy resources and enhances government attention toward implementing the green energy transition by establishing more relevant monetary policies. The uncertainties related to energy consumption, such as oil prices, exchange rates, bond markets, and stock markets, accelerate the need for a green energy transition (Lei et al., 2022). The lack of consensus in the existing literature motivated the study to investigate the impact of EPU on GET in China.
Technology plays a vital role in environmental sustainability (Hu et al., 2022), and it is an integral part of strategic development and transformation. In the era of industrialization, technological innovations have reshaped economies, improved existing product processing, enhanced energy efficiency, and promoted the consumption of green energy (Østergaard et al., 2021). Moreover, studies also found that the fast penetration of technological innovation (TIN) into the global green energy sector significantly curbs the high energy demand without compromising the world’s economic growth (Ahmed et al., 2021). TIN conserves energy using green energy resources. It is a crucial factor in the green energy supply as it enhances the relevant capabilities to produce green energy to meet the potential demand (Ramzan et al., 2022a). Thus, TIN complements the policies of GET to increase the share of green energy in the total energy consumption in China. GET also requires a reduction in the energy intensity, such as the combustion engines requiring to be replaced by more efficient electric vehicles; similarly, the hydropower generator is more energy-efficient than the natural gas generator. It infers that improvement in TIN significantly promotes green energy transition (Usman & Hammar, 2021).
On the other hand, some studies argue that an increase in TIN enhances the energy demand, as technology innovation increases the production capacity, thus requiring more energy to run the operations. Advanced heavy machinery is highly energy-intensive and negatively contributes to the green energy transition (Chen M. et al., 2021). Similarly, blockchain technology also requires huge electricity and heavily relies on traditional energy to make the process cost-effective. Likewise, the advanced machinery appliances used by households and machinery also raise the energy demand and cause resource scarcity (Hu et al., 2022). Considering the positive and negative aspects, the impact of TIN on the GET is still inconclusive.
Environmental governance also plays a paramount role to implement the green energy transition. The strict rules and strong governances reduce environmental degradation and work as the leading factor in achieving the goal of decarbonization (Asongu & Odhiambo, 2020). Environmental governance can be measured through various indicators. However, the most widely used indicator is environmental taxes which discourage the consumption of non-renewable energy resources in manufacturing and other economic activities. The environment and governance nexus is based on two theories: the “race to the bottom” theory, which indicates that the policies compromised on the environmental standards to achieve economic and financial benefits, whereas the other theory of “race to the top” suggests that policies strengthen their environmental regulations to achieve green energy transition (X. Wang & Huang, 2021). China is a pollution heaven for the world (Ramzan et al., 2022b) and requires effective environmental governance (EGV). EGV promotes energy efficiency and effective resource management, controls transaction costs, promotes green innovation, imposes carbon taxes to reduce carbon emissions, and promotes the GET (Ren et al., 2022). Therefore, the current study evaluated the impact of environmental taxes as the proxy of EGV on CGET in China.
The study has selected China as the study sample due to its attractive carbon neutralization policies for 2060, which is currently the watershed moment in the global energy transition due to its technological innovation and environmental policies, ecological civilization, and transformation of the energy production and consumption mechanisms. China has taken various steps to swift its energy transition. For example, in the last couple of years, China has made considerable investments in the green energy manufacturing capacity. Therefore, 50% of total electricity production is being contributed by green energy sources (IEA, 2021). However, the green energy market role and demand responses remain unchanged, making the carbon neutrality task difficult. Therefore, this study investigates the factors that play a significant role in achieving the full potential of green energy transformation.
The study contributes to the existing literature in many ways. For instance, it examines the combined impact of economic policy uncertainty, technological innovation, ecological governance, and economic growth on the green energy transition in China from 2000 to 2020. In addition to this, the study employed the “bootstrap auto-regressive distributive lag” model presented by McNown et al. (2018), which provides the long-run and short-run cointegration association between variables. This approach is superior, provides comprehensive outcomes, and explains more variation in the data series regardless of the power and size of the datasets. The study’s outcomes revealed that EPU and EGR (economic growth) discourage GET, whereas TIN and EGV promote GET in China. The green energy transition of China requires extra government initiatives to neutralize the EPU and EGR adverse effects; therefore, the study’s outcomes provide better direction for the policy implication.
The rest of the study is organized as follows: Section 2 of the literature review sheds light on the previous studies. Section 3 covers data, variable definitions, and the theoretical and empirical framework of the study. Section 4 illustrates the findings and discussion. Section 5 covers the conclusion and policy recommendation.
2 Literature review
2.1 Nexus between economic policy uncertainty and green energy transition
In recent years, the challenge of environmental sustainability has attracted considerable attention from researchers worldwide. Similarly, policymakers have targeted to achieve the zero-carbon goal through various steps. The main target is the energy sector’s revolution; therefore, green energy consumption as an alternate source is promoted to accelerate the energy transition process (Singh et al., 2019). The consumption of hydropower, wind, and solar energy is the mainstream of the energy sector’s transformation. The respective economic policy uncertainty is considered the significant factor in executing GET. It initially triggers the green energy sector by attracting government incentives regarding preferential tax policies, subsidies, augmented depreciation, etc. However, EPU adversely affects the green transformation mechanism in the long run by enhancing the systematic risks in the green investment portfolios (Ivanovski & Marinucci, 2021). The studies on the nexus between EPU and GET are scant (Jamil et al., 2022; Zhou et al., 2022), as the existing literature has paid more attention to green energy investment under the economic policy uncertainty (Pirgaip & Dinçergök, 2020; Lei et al., 2022) and less on the renewable energy association with EPU. Therefore, this study reviews the literature on the nexus of green or renewable energy consumption (GEC) and economic policy uncertainty to shed light on both positive and negative associations.
Shafiullah et al. (2021) applied the nonparametric (nonlinear) econometric approach to find the impact of EPU on GEC of the USA. The results revealed that EPU was negatively associated with GEC. The EPU adversely affects the energy sector due to the uncertainty in production taxes, credit policies, and shortage of investment in the green energy sector. In contrast, Shang et al. (2022) evaluated the role of EPU in demand for GEC in the USA from 2000 to 2021. The study found that EPU reduces renewable energy consumption while accelerating the long-run green energy demand due to high crude oil price fluctuations. Likewise, to investigate the impact of EPU on GEC for the panel data, Su et al. (2022) employed the “quantile on quantile” approach to the data of G-7 countries. The outcomes of this approach highly suggested that except for Italy and Japan, EPU has a significantly negative impact on the GEC, whereas for Italy and Japan, the impact of EPU on GEC was significantly positive. The technological advancement of these countries neutralized the negative role of EPU in these countries. These outcomes endorse the findings of Kang et al. (2017), who suggested a negative association between EPU and GEC and a positive association between demand-side oil price shock and EGC.
Zhang et al. (2021) accessed the association between EPU and GEC with the mediating role of financial development (FDV) for BRIC countries. The study applied the linear and nonlinear ARDL approaches to explore the nexus and revealed that FDV plays a crucial role in depressing the impact of EPU on GEC. Thus, the technological transformation and capital investment in the green energy sector support the integration of GET in high EPU. From the green energy production (GEP) perspective, Jamil et al. (2022) investigated the individual impact of EPU in terms of monetary, fiscal, and trade policy uncertainties on GEP in the USA. The ARDL method results showed that the monetary policy uncertainty dropped the GEP, while the fiscal policy uncertainty upsurged the GEP. In contrast, the trade policy uncertainty remains insignificant to promoting GEP in the long run and short run. Based on the aforementioned literature review, the study gap has been determined to investigate the EPU impact on GET in China.
2.2 Nexus between technology innovation and green energy transition
Energy transaction is the composition of accessibility, sustainability, and efficiency of the energy resources. The key focus of this study is to evaluate the impact of technological innovation on the energy efficiency of the green energy sector of China. For this, the study divided the existing literature into two strands. One represents the direct and indirect impact of TIN on energy efficiency in terms of energy intensity and its production or consumption, whereas the other deals with the negative effect on energy transition. For instance, Assi et al. (2021) evaluated the direct impact of TIN on GEC of ASEAN economies from 1998 to 2018. The outcomes of the panel ARDL test suggested that TIN positively affects the GEC in these countries. In addition to this, Ramzan, Razi, et al. (2022) discovered that the TIN conserves energy using green energy resources in the United Kingdom. TIN is a crucial factor in the green energy supply as it enhances the relevant capabilities to produce green energy to meet the potential demand. Su et al. (2022) applied the “quantile on quantile” approach to investigate the link between TIN and GEC for G-7 countries. The study found that TIN is positively and significantly linked with GEC at the medium quantile, implying that technology innovation enhances green energy efficiency and promotes GET in G-7 countries under stable economic conditions.
H. Wang & Wang (2020) employed the “generalized method of moment (GMM)” regression analysis on the data of China from 2001 to 2013 and revealed that TIN significantly and positively impacts energy efficiency at the country level. Khan et al. (2021) explored the role of TIN in energy efficiency in the small and medium enterprise (SME) supply chain networks of China and Pakistan. The study suggested that TIN improves logistics energy efficiency and reduces GEC. The impact of TIN on energy intensity has been observed by Usman and Hammar (2021) for Asia Pacific Economic Cooperation (APEC) countries. The authors used the “Stochastic Impacts by Regression on Population, Affluence, and Technology (STIRPAT) model” and suggested that the GET requires a reduction in the energy intensity, such as the combustion engines requiring to be replaced by more efficient electric vehicles; similarly, the hydropower generator is more energy efficient than the natural gas generator. It infers that improvement in TIN significantly promotes green energy transition.
Pan et al. (2019) found a positive relationship between TIN and the energy intensity in Bangladesh and suggested that TIN accelerates the energy intensity. On the other hand, some studies argued that an increase in TIN enhances the energy demand, as technology innovation increases the production capacity, thus requiring more energy to run the operations. Advanced heavy machinery is highly energy-intensive and negatively contributes to the green energy transition (Chen M. et al., 2021). Similarly, according to J. Hu et al. (2022), technological advancement promotes energy-intensive products, increasing energy demand and leading to resource scarcity. Considering the positive and negative aspects of TIN, its impact on the GET is still inconclusive, which motivates the study to provide fresh empirical evidence regarding the nexus between TIN and GET.
2.3 Nexus between environmental governance and green energy transition
The upward trend in economic growth always has a high environmental impact, as the increase in growth is facilitated through carbon-intensive production and logistics mechanisms. Therefore, strict environmental governance is critical to limit the high environmental impact of fossil fuel consumption (Khalid et al., 2022). Various countries have implemented different ways of environmental governance to seek environmental protection (Ren et al., 2022). Good governance as environmental regulation enhances the efficiency of energy (Hamid et al., 2022). This study employed the most widely used indicator of environmental taxes as the proxy for environmental governance and reviewed its link with the GET in the existing literature. The race to the top theory adopted by emerging economies suggests that policies strengthen their environmental regulations to achieve a green energy transition (X. Wang & Huang, 2021).
There are two views of researchers regarding the impact of EGV on GET. The existing literature has found that environmental taxes are paramount in implementing a green energy transition in terms of an increase in green energy consumption and energy efficiency (Tao et al., 2021). For instance, Fu et al. (2021) evaluated the EGV impact on the carbon-intensive industries’ transformation to energy-efficient industries in China. The “system generalized method of moments (GMM)” outcomes revealed that EGV significantly supported the optimization of the industrial structure to protect the environment in the highly developed regions of China from 2004 to 2016. Similarly, P. Li et al. (2021) evaluated the association of EGV with the energy intensity in the 30 provinces of China; they concluded that good governance controls the energy intensity obtained through fossil fuels. By using the “auto-regressive distributive lag (ARDL)” approach on the data of 29 OECD countries, Rafique et al. (2022) endorsed that the EGV reduced the consumption of fossil fuels and met the energy demand by supporting a green energy supply.
Likewise, with the motive of fossil fuel reduction, Andreoni (2019) examined the contribution of EGV as a revenue generator. This revenue is used as a subsidy to promote GET in the 25 European countries; the index decomposition techniques are used to analyze the data from 2004 to 2016. The study’s outcomes revealed that EGV, as revenue, promotes renewable energy resources by subsidizing the green energy sector. In contrast, few studies have highlighted the negative association of EGV with the GET process. For example, the pollution hypothesis validity has been evaluated by Qiu et al. (2021) who explored the influence of TIN on the green productivity of China. The study applied the “feasible generalized least squares (FGLS)” and “dynamic generalized method of moments (GMMs)” from 2004 to 2017 and found that weak environmental governance allows the high consumption of fossil fuels which becomes obstacles for the GET. Similarly, Nie et al. (2021) affirmed that EGV hampers the GET by impeding green energy resource consumption.
3 Methodology
3.1 Data
This study investigates the role of economic policy uncertainty, technology innovation, and ecological governance as the influencing factors in the green energy transition in China from Q1-2000 to Q-2020. We have transformed annual data into quarters using a quadratic match-sum approach following Li et al. (2022) and Razzaq et al. (2022; 2021).
3.1.1 Dependent variable
The energy transition index (ETI) has been used as the proxy to measure the dependent variable of the green energy transition, which is referred to as “measures the availability, sustainability, and efficiency of energy sources using principal component analysis” (UNCTAD, 2018). This index has been composited to evaluate the current energy system performance of the country based on its ability to support economic growth, accessibility to reliable sources of energy supply, and its support toward environmental sustainability across the whole value chain of the energy system (Singh et al., 2019). Figure 2 exhibits the energy triangle of the three categories of the energy transaction index.
3.1.2 Independent variables
On the other hand, the independent variables of the study, such as economic policy uncertainty, technology innovation, ecological governance, and economic growth, are taken as the EPU index (Baker et al., 2016), patents (Hossain et al., 2022), environmental taxes (Tao et al., 2021), and GDP (Chen J. et al., 2021), respectively. Moreover, the data have been transformed from annual to quarterly data by applying the quadratic match-sum to neutralize the cyclical and seasonal variations in the data series and to increase the number of observations to obtain reliable outcomes (Razzaq et al., 2021; Samour & Pata, 2022). In addition to this, the economic growth and technology innovation data have been transformed into the natural log to obtain data symmetries and to eliminate potential heteroskedasticity. Table 1 provides the variable’s details, proxies, measures, and sources.
3.2 Empirical model
This study investigates the role of economic policy uncertainty, technology innovation, and environmental governance in supporting the green energy transition implementation in China. Based on the discussion of the previous studies and according to the framework of Shahbaz et al. (2022), this study assumes that the green energy transition is influenced by various economic determinants such as economic digitalization, foreign direct investment, economic growth, industrialization, and urbanization. The functional form and the empirical model considering these potential drivers of GET are constructed as follows:
Here, GET, EPU, TIN, EGV, and EGR denote green energy transition, economic policy uncertainty, technology innovation, environmental governance, and economic growth, respectively, while “t” is denoted as the period from 2000 to 2020 and
3.3 Theoretical framework
China meets its energy demand through coal or traditional energy resources. The predominant reliance on these resources boosts economic growth while significantly deteriorating the environmental sustainability perspective. The green energy transition is the way to “the transformation of the global energy sector from fossil-based to zero-carbon by the second half of this century” (Murshed et al., 2021). The most prominent factor contributing to GET is technological advancement. TIN is an integral part of strategic development and transformation, which reduce energy intensity, improve energy efficiency, limit environmental impact, and enhance the green energy supply’s capacity to execute economic activities (Ramzan et al., 2022a). Therefore, the expected relationship between TIN and GET can be positive
In contrast, EPU adversely affects GET by creating uncertainty in investment behavior due to the volatility of energy prices, exchange rates, bond markets, and stock markets (X. Zhou et al., 2022). It hampered the investors’ confidence, spurred systematic risk, and increased capital costs (Z. Xu, 2020). Likewise, the high EPU significantly reduced the investment in innovations and R&D activities. Thus, optimal-energy-related policies are hindered by the level of EPU. Based on these facts, the expected association between EPU and GET is negative
Although EGR severely relies on energy-intensive activities, the high economic growth accelerates resource demand. To meet the high demand, the consumption of inexpensive and non-green energy resources upsurged, leading to environmental pollution and resource scarcity and delaying the process of the green energy transition (J. Hu et al., 2022; Sun et al., 2022). Hence, the expected association between EGR and GET is negative
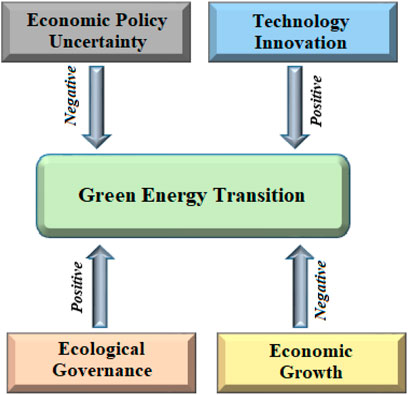
FIGURE 3. Theoretical frameworks for the influencing factors of the green energy transition. Source: authors’ creation.
3.4 Empirical framework
By considering the significant influence of economic policy uncertainty on green energy consumption (Lei et al., 2022) and the impact of technological innovation on energy efficiency (Chen M. et al., 2021), it is imperative to investigate the nexus between EPU, TIN, EGV, and GET for the long run by applying the reliable and appropriate analytical approach. This traditional “auto-regressive distributive lag” approach presented by Pesaran et al. (2001) may have econometric issues such as estimation bias for the limited observations of time series data and the power statistics, whereas the recent approach of “bootstrap auto-regressive distributive lag” presented by McNown et al. (2018) provides the long-run cointegration association between variables to direct the researchers and policymakers for the establishment of appropriate policy measures (Razzaq et al., 2021).
According to the recent studies for the long-run relationship evaluation among variables, the econometric approach required to support two conditions the coefficient values need to be significant for the error-correction model (ECM) and “the partial short-term adjustment to correct the deviation from the long-run equilibrium of series” and the lagged independent variable (Nawaz et al., 2019; Meirun et al., 2021; Razzaq et al., 2021; Samour & Pata, 2022). For the solution, Pesaran et al. (2001) have suggested the upper and lower values (critical bounds) to determine the significance level of the coefficients of the lagged independent variables in the ARDL bond testing approach. The critical bonds to determine the ECM coefficient significance level are not directed by the existing literature. Under the specific condition where the first-order integration of the variables and the significant coefficient value of ECM are obtained, the BARDL provides highly elucidative estimates, which the traditional ARDL approach cannot generate due to the weak power characteristics. Likewise, BARDL is advantageous in the following ways: it effectively handles the issue of small sample size and improves the power of T- and F-tests by integrating the additional bound test (FIND-test) to estimate the coefficients of the lagged independent variables and yield robust outcomes (Samour & Pata, 2022). Also, the BARDL approach allows the multivariant model to generate the critical values for mixed integration order. Thus, with the Monte Carlo simulations, BARDL is superior to the ARDL test due to the size and power properties (McNown et al., 2018).
With three different variables, the mathematical specification of the BARDL model is as follows:
Here, the dependent variable is presented as
The functional form of the coefficients in Eq. 4 is as follows:
Similarly, the functions of
Furthermore, to confirm the cointegration among
(1) The FJOINT-test must be related to error-correction terms, as proposed by Pesaran et al. (2001). The hypotheses for the F-test are as follows:
(2) The FIND-test must be related to all lagged independent variables. For the FIND test, following are the suggested hypotheses:
(3) The TDEP-test must be related to the lagged dependent variable. The TDEP test hypotheses are as follows:
4 Findings and discussion
The descriptive statistics of study variables such as green energy transition, economic policy uncertainty, technological innovation, environmental governance, and economic growth are illustrated in Table 2. The computed statistics show that the GET has the highest volatility, whereas the lowest dispersion among all variables is observed for EGV in China. The normality of all the time series has been proven through the outcomes of the Jarque–Bera test. The probability value of the JB test is insignificant for all variables; thus, the null hypothesis of “normal distribution” is accepted.
The long-run estimates first required confirming the data series stationarity to avoid spurious outcomes. Therefore, the “augmented Dickey–Fuller” (ADF) and “Zivot and Andrews” (ZA) unit root tests were employed in this study which provide the structural break in the data series along with their integration orders. Table 3 depicts the unit root test results, which revealed that at a level under both the tests, the data series are nonstationary at a level, while after taking the first difference, stationarity has been obtained for all series. However, the significance level has varied, i.e., EPU, TIN, and EGV are stationary at a 1% significance level, whereas the significance level of GET and EGR is at 5% and 10%, respectively. In addition to these results, the ZA test provides the structural break in Q3-2016, Q4-2016, Q1-2018, Q4-2016, and Q4-2010 for EPU, GET, TIN, EGV, and EGR, respectively. The bootstrapping ARDL approach is one of the most appropriate approaches to handle the specific integration order, dynamic stochastic trend, and structural break issue during the long-run estimations.
Subsequently, after the affirmation of stationarity in the data series, the cointegration analysis is performed to evaluate the long-run relationship between EPU, TIN, EGV, EGR, and GET. For this purpose, this study employed the “bootstrap ARDL cointegration test,” which provides the joint F-test and t-test for the lagged values of independent and dependent variables. In addition to this, the BARDL cointegration test also evaluates the estimates for the FIND-test bound test, which provides robust results of cointegration analysis by determining the cointegration equilibrium among variables. Moreover, this analysis’s crucial part is choosing the optimal lag length to reduce the risk of distorted outcomes. Therefore, the widely accepted and most reliable “Akaike information criteria” (AIC) measure has been applied to choose the optimal lag length. The outcomes of BARDL cointegration analysis and diagnostic tests are illustrated in Table 4, which also indicates the lag length of 2, 1, 2, 1, and 1 for the model of
In the next step, after the confirmation that the long-run relationship exists between the variables, the study applied the bootstrapped ARDL cointegration analysis for the long-run estimates. Table 5 demonstrates the outcomes, which revealed that a 1% increase in the EPU leads to a decrease in GET by -0.362% at a 5% level of significance; this implies that the impact of EPU on the energy transition is significant while relying on the energy price volatility and the supply–demand shocks. The price effect is highly dependent on economic activities. The high EPU raises negative expectations of economic activities and market price stability. When the price of overall energy resources fluctuates, industries and household consumers seek to consume low-cost energy resources such as coal, which adversely affects the economy’s process of the green energy transition. The relationship between EPU and GET is similar to the findings of Ivanovski and Marinucci (2021), which suggested that the high level of country risk due to the high EPU daunts to encourage green energy consumption which is inconsistent with the findings of Aastveit et al. (2017). The authors argue that the high EPU enhances the monetary policy focus on providing alternate and cost-effective energy resources, thus improving the green energy transition process by supporting the industries to invest in green energy resources to meet the supply–demand gap.
In contrast, the long-run estimates of the nexus between GET and TIN show that the 1% addition in the TIN increases the GET by 0.412% at a 1% significance level, which indicates that the technological advancement positively influences the green energy transition by aiding the productivity of the alternate energy resources such as green energy or the mixed fuels. China is a huge economic hub where the intrinsic nature of its economic activities increases energy demand. Technology innovation reduces the ineffective market by promoting energy conservation and reforming energy-efficient consumption in the electricity, logistics, and manufacturing industries. The study outcomes of Assi et al. (2021) are similar to the results of this study and suggest that technology innovation supports green energy projects to achieve economic and environmental sustainability. In contrast, the findings are inconsistent with the study of Ben Youssef (2020), who argued that technological innovation (resident patents) discourages renewable energy consumption and heavily relies on cheap non-renewable energy resources in the USA.
Likewise, the long-run BARDL cointegration estimates of the nexus between EVG and GET illustrate that an increase in the environmental taxes as the proxy of environmental governance by 1% enhances the green energy transition by 0.126% at a 1% significance level which implies that the strict regulation and environmental governances discourage the fossil fuel consumptions and boost energy efficiency. Moreover, the collected environmental taxes subsidized the green energy projects and assured China’s fastest green energy transition processes. The results are also supported by the study of Rafique et al. (2022). They explored that EVG positively contributes to transforming the economy toward less energy-intensive manufacturing and economic activities to achieve sustainable environmental goals. Nie et al. (2021) contradicted the current study findings and argued that in developing countries, EVG limits economic activities, and therefore causes hurdles in the adaptation to green economic transformation.
Table 5 shows the negative association of the EGR with GET in China for the study period. The 1% rise in EGR declines the GET by 0.518% at the 10% significance level, indicating that the EGR boosts economic activities such as production and transportation and increases energy demand. Furthermore, inexpensive and non-green energy resources are consumed to meet the high demand, leading to environmental pollution and resource scarcity and delaying the process of the green energy transition. Sun et al. (2022) and J. Hu et al. (2022) have endorsed the inverse relationship between EGR and green energy consumption.
The coefficient of the dummy variable is significantly negative, which illustrates that the dummy variable of 0.314% depressed the green energy transition in 2014 at the 1% significance level. The oil-glut year is known as the year of 2014, when the excessive supply of crude oil caused high fluctuations in oil prices, which as a result, imposed an adverse effect on the consumption of renewable energy resources (Razzaq et al., 2021). Subsequently, the outcomes of stability analysis in the BARDL cointegration (long-run) affirm the validity of the parameter and confirm the normal distribution of the model’s error term. Additionally, the serial correlation is not evident in these statistics. Also, the Ramsey’s RESET statistics have not proven auto-regressive conditional heteroskedasticity. The value of R-squared was 86.7%, showing the goodness-of-fit for the model and endorsed that for China, and the 86.7% variation in GET was explained by the model’s long-run explanatory variables. In addition to this, the estimates of the Durbin–Watson test also affirm the absence of autocorrelation in the model. In contrast, the CUSUM and CUSUMSQ tests endorse the parameter stability.
The short-run estimates of “bootstrapped ARDL cointegration analysis” are exhibited in Table 6, demonstrating that EPU and GET have a negative association. A 1% increase in the EPU declined the progress of GET by 0.294% at the significance level of 10%, while EGV presented as environmental taxes promoted the GET implication in China by 0.108% against each yuan of tax collection in the short-run. Similarly, TIN also significantly stimulates the process of GET in China. A 1% addition in the patents improves GET by 0.339% at the 1% significance level. In contrast, the EGR has an adverse impact on GET. An excessive rise in economic activities negatively impacts environmentally friendly investment and green energy consumption. The nature of association among variables in the short run follows the same long-run relationship; however, the significance level is not constant. Similar to the long run, the dummy variable negatively and significantly affects GET in the short run for 2014. Thus, the outcomes of the long-run and short-run BARDL cointegration estimators revealed that EPU and EGR are the critical drivers for depressing the GET process, while TIN and EGV accelerate the GET process in China.
Moreover, the value of the “error-correction term” (ECM) is significantly negative and indicates the convergence speed of 1.21% toward the long-run equilibrium in response to any shock. This ECM value of 1.21% also refers to the deviation adjustment from the long run. As all independent variables, such as EPU, EGV, TIN, and EGR, are highlighted by their previous year’s performance, it is essential to consider the lag effect to avoid unreliable results. The rest of the diagnostic and stability tests endorses the outcomes in the long run, for instance, “the Durbin–Watson test” affirms “no autocorrelation,” the Ramsey’s RESET test confirms “no auto-regressive conditional heteroskedasticity,” and the R-squared values (goodness-of-fit) indicate the accuracy and reliability of the estimates, while the CUSUM and CUSUMSQ confirm the stability of the parameters in short run.
In addition to the BARDL cointegration long-run and short-run analyses, this study also employed the “Granger causality test” to explore the causal association between dependent and independent variables. Table 7 shows the outcomes of the “Granger causality test” for which the null hypothesis has been established as “no causality.” The probability values of the F-statistics confirm the bidirectional association only between TIN and GET. This implies that the technology innovation supports the green energy transition by applying the technology to improve energy efficiency. At the same time, the green energy transition encourages technological innovation to achieve environmental sustainability goals in the long run.
In contrast, the association between EPU and GET, EGV and GET, and EGR and GET has a unidirectional association. These unidirectional relationships explain that the GET does not affect EPU, EGV, and EGR in China, while the EPU significantly affects GET. Similarly, EGV affects GET at a 5% significance level, whereas EGR highly and significantly affects the GET process in China; thus, any policy intervention of government and stakeholders regarding EPU, TIN, EGV, and EGR can influence GET in China.
5 Conclusion and recommendations
For environmental sustainability and resource security, the global energy system requires a revolutionary transition from traditional energy resources to green energy resources. China has become the center of attraction in the last couple of years due to its adoption and extensive investments in the green energy transition process. However, China is showing considerable measures to respond to its zero-carbon pledges under the Paris Agreement. However, due to its industrial and energy structure and other development endowments, its progress toward green energy transition has stagnated since 2014. The existing literature has identified various socio-economic factors that shape the scope and pace of energy transition; however, the role of economic policy uncertainty, technology innovation, and ecological governance in meeting the goal of the green energy transition is still unattended. Therefore, this study investigates the combined impact of EPU, TIN, and EGV as environmental taxes and EGR on the GET in China.
After confirming the stationarity and normal distribution of the data series through the preliminary finding of the unit root test and the Jarque–Bera test, the study applied the BARDL cointegration test, affirming the presence of long-run correlation between the variables. Furthermore, to evaluate the long-run and short-run association between the study variables, the study has used the “bootstrap auto-regressive distributed lag” approach by employing the quarterly data from 2000 to 2020. The outcomes of the advanced cointegration features of the dynamic process of BARDL show that economic policy uncertainty and economic growth are negatively associated with the green energy transition, which implies that EPU and EGR discourage the process of the green energy transition. In contrast, technology innovation (TIN) and environmental governance (EGV) are positively linked with the green energy transition, indicating that the TIN and EGV are critical drivers in achieving a fully green energy transition. Based on the findings, the study suggests the following policy implementation to strengthen the environmental governance mechanism and technology innovation to accelerate the green energy transition implementation.
Economic policy stability is essential to promote the green energy transition. The stable political environment reduces systematic risk and fortifies investor confidence. China’s government must focus on related policies such as renewable portfolio standards, income tax credits, production tax credits, and a clean power plan. Moreover, there is a need to establish policies to attract more foreign investment to reshape the green energy sector, which mitigates the adverse impact of EPU caused by energy prices. These foreign investments are also used to support technology innovation to obtain optimal energy efficiency. In addition, considering the positive impact of innovation on GET, it has been suggested that the government should provide funding and R&D facilities to boost energy-efficient products, such as investment in electric car plants, energy-efficient houses, and the installation and acquisition of solar panel plants. Thus, to boost the speed and spread of the green energy transition, solar energy deployment loans at a low interest rate should be provided to individuals and enterprises. In addition to these, the policymakers of China need to establish policies to foster the green energy transition by intensifying their efforts to strengthen the green energy regime.
This study is limited to China’s national-level data and cannot integrate regional heterogeneity. Thus, future studies would explore regional differences in energy transition and their influence. Similarly, cross country analysis may also help to compare the performance of the Chinese economy.
Data availability statement
Publicly available datasets were analyzed in this study. These data can be found at: Data will be made available upon reasonable request.
Author contributions
All authors listed have made a substantial, direct, and intellectual contribution to the work and approved it for publication.
Conflict of interest
The authors declare that the research was conducted in the absence of any commercial or financial relationships that could be construed as a potential conflict of interest.
Publisher’s note
All claims expressed in this article are solely those of the authors and do not necessarily represent those of their affiliated organizations, or those of the publisher, the editors, and the reviewers. Any product that may be evaluated in this article, or claim that may be made by its manufacturer, is not guaranteed or endorsed by the publisher.
References
Aastveit, K. A., Natvik, G. J., and Sola, S. (2017). Economic uncertainty and the influence of monetary policy. J. Int. Money Finance 76, 50–67. doi:10.1016/j.jimonfin.2017.05.003
Ahmed, Z., Cary, M., Shahbaz, M., and Vo, X. V. (2021). Asymmetric nexus between economic policy uncertainty, renewable energy technology budgets, and environmental sustainability: Evidence from the United States. J. Clean. Prod. 313, 127723. doi:10.1016/j.jclepro.2021.127723
Amin, A., and Dogan, E. (2021). The role of economic policy uncertainty in the energy-environment nexus for China: Evidence from the novel dynamic simulations method. J. Environ. Manag. 292, 112865. doi:10.1016/j.jenvman.2021.112865
Andreoni, V. (2019). Environmental taxes: Drivers behind the revenue collected. J. Clean. Prod. 221, 17–26. doi:10.1016/j.jclepro.2019.02.216
Asongu, S. A., and Odhiambo, N. M. (2020). Governance, CO2 emissions and inclusive human development in sub-Saharan Africa. Energy Explor. Exploitation 38 (1), 18–36. doi:10.1177/0144598719835594
Assi, A. F., Zhakanova Isiksal, A., and Tursoy, T. (2021). Renewable energy consumption, financial development, environmental pollution, and innovations in the ASEAN + 3 group: Evidence from (P-ARDL) model. Renew. Energy 165, 689–700. doi:10.1016/j.renene.2020.11.052
Baker, S. R., Bloom, N., and Davis, S. J. (2016). Measuring economic policy uncertainty. Q. J. Econ. 131 (4), 1593–1636. doi:10.1093/qje/qjw024
Ben Youssef, S. (2020). Non-resident and resident patents, renewable and fossil energy, pollution, and economic growth in the USA. Environ. Sci. Pollut. Res. 27 (32), 40795–40810. doi:10.1007/s11356-020-10047-0
Chen, J., Hu, X., Razi, U., and Rexhepi, G. (2021). The sustainable potential of efficient air-transportation industry and green innovation in realising environmental sustainability in G7 countries. Econ. Research-Ekonomska Istraz. 35, 3814–3835. doi:10.1080/1331677X.2021.2004190
Chen, M., Sinha, A., Hu, K., and Shah, M. I. (2021). Impact of technological innovation on energy efficiency in industry 4.0 era: Moderation of shadow economy in sustainable development. Technol. Forecast. Soc. Change 164, 120521. doi:10.1016/j.techfore.2020.120521
De La Peña, L., Guo, R., Cao, X., Ni, X., and Zhang, W. (2022). Accelerating the energy transition to achieve carbon neutrality. Resour. Conservation Recycl. 177, 105957. doi:10.1016/j.resconrec.2021.105957
Fu, S., Ma, Z., Ni, B., Peng, J., Zhang, L., and Fu, Q. (2021). Research on the spatial differences of pollution-intensive industry transfer under the environmental regulation in China. Ecol. Indic. 129, 107921. doi:10.1016/j.ecolind.2021.107921
Fu, Z., Chen, Z., Sharif, A., and Razi, U. (2022). The role of financial stress, oil, gold and natural gas prices on clean energy stocks: Global evidence from extreme quantile approach. Resour. Policy 78, 102860. doi:10.1016/J.RESOURPOL.2022.102860
Gielen, D., Boshell, F., Saygin, D., Bazilian, M. D., Wagner, N., and Gorini, R. (2019). The role of renewable energy in the global energy transformation. Energy Strategy Rev. 24, 38–50. doi:10.1016/j.esr.2019.01.006
Graham, J. D., Rupp, J. A., and Brungard, E. (2021). Lithium in the green energy transition: The quest for both sustainability and security. Sustain. Switz. 13 (20), 11274. doi:10.3390/su132011274
Hamid, I., Alam, M. S., Kanwal, A., Jena, P. K., Murshed, M., and Alam, R. (2022). Decarbonization pathways: The roles of foreign direct investments, governance, democracy, economic growth, and renewable energy transition. Environ. Sci. Pollut. Res. 29, 49816–49831. doi:10.1007/s11356-022-18935-3
Hossain, M. E., Islam, M. S., Bandyopadhyay, A., Awan, A., Hossain, M. R., and Rej, S. (2022). Mexico at the crossroads of natural resource dependence and COP26 pledge: Does technological innovation help? Resour. Policy 77, 102710. doi:10.1016/j.resourpol.2022.102710
Hove, A., Wenyun, Q., Kaiming, Z., Geres, P., and Yuzhao, L. (2021). China energy transition status report 2021. Available at https://transition-china.org/energyposts/china-energy-transition-status-report-2021-2/.
Hu, J., Xu, J., Tong, L., and Razi, U. (2022). The dynamic role of film and drama industry, green innovation towards the sustainable environment in China: Fresh insight from NARDL approach. Econ. Research-Ekonomska Istraz. 35, 5292–5309. doi:10.1080/1331677X.2022.2026239
IEA (2021). China has a clear pathway to build a more sustainable, secure and inclusive energy future. Available at https://www.iea.org/news/china-has-a-clear-pathway-to-build-a-more-sustainable-secure-and-inclusive-energy-future.
Irfan, M., Razzaq, A., Sharif, A., and Yang, X. (2022). Influence mechanism between green finance and green innovation: Exploring regional policy intervention effects in China. Technol. Forecast. Soc. Change 182, 121882. doi:10.1016/j.techfore.2022.121882
Ivanovski, K., and Marinucci, N. (2021). Policy uncertainty and renewable energy: Exploring the implications for global energy transitions, energy security, and environmental risk management. Energy Res. Soc. Sci. 82, 102415. doi:10.1016/j.erss.2021.102415
Jamil, M., Ahmed, F., Debnath, G. C., and Bojnec, Š. (2022). Transition to renewable energy production in the United States: The role of monetary, fiscal, and trade policy uncertainty. Energies 15 (13), 4527. doi:10.3390/en15134527
Jiang, C., Zhang, Y., Razi, U., and Kamran, H. W. (2021). The asymmetric effect of COVID-19 outbreak, commodities prices and policy uncertainty on financial development in China: Evidence from QARDL approach. Econ. Research-Ekonomska Istraz. 0 (0), 2003–2022. doi:10.1080/1331677X.2021.1930092
Kang, W., Perez de Gracia, F., and Ratti, R. A. (2017). Oil price shocks, policy uncertainty, and stock returns of oil and gas corporations. J. Int. Money Finance 70, 344–359. doi:10.1016/j.jimonfin.2016.10.003
Khalid, F., Razzaq, A., Ming, J., and Razi, U. (2022). Firm characteristics, governance mechanisms, and ESG disclosure: How caring about sustainable concerns? Environ. Sci. Pollut. Res. 29, 82064–82077. doi:10.1007/S11356-022-21489-Z
Khan, S. A. R., Godil, D. I., Jabbour, C. J. C., Shujaat, S., Razzaq, A., and Yu, Z. (2021). Green data analytics, blockchain technology for sustainable development, and sustainable supply chain practices: Evidence from small and medium enterprises. Ann. Oper. Res., 1–25. doi:10.1007/s10479-021-04275-x
Lei, W., Liu, L., Hafeez, M., and Sohail, S. (2022). Do economic policy uncertainty and financial development influence the renewable energy consumption levels in China? Environ. Sci. Pollut. Res. 29 (5), 7907–7916. doi:10.1007/s11356-021-16194-2
Li, P., Lin, Z., Du, H., Feng, T., and Zuo, J. (2021). Do environmental taxes reduce air pollution? Evidence from fossil-fuel power plants in China. J. Environ. Manag. 295, 113112. doi:10.1016/j.jenvman.2021.113112
Li, Q., Sharif, A., Razzaq, A., and Yu, Y. (2022). Do climate technology, financialization, and sustainable finance impede environmental challenges? Evidence from G10 economies. Technol. Forecast. Soc. Change 185, 122095. doi:10.1016/j.techfore.2022.122095
McNown, R., Sam, C. Y., and Goh, S. K. (2018). Bootstrapping the autoregressive distributed lag test for cointegration. Appl. Econ. 50 (13), 1509–1521. doi:10.1080/00036846.2017.1366643
Meirun, T., Mihardjo, L. W., Haseeb, M., Khan, S. A. R., and Jermsittiparsert, K. (2021). The dynamics effect of green technology innovation on economic growth and CO2 emission in Singapore: New evidence from bootstrap ARDL approach. Environ. Sci. Pollut. Res. 28 (4), 4184–4194. doi:10.1007/s11356-020-10760-w
Mohideen, M. M., Ramakrishna, S., Prabu, S., and Liu, Y. (2021). Advancing green energy solution with the impetus of COVID-19 pandemic. J. Energy Chem. 59, 688–705. doi:10.1016/j.jechem.2020.12.005
Murshed, M., Ahmed, R., Kumpamool, C., Bassim, M., and Elheddad, M. (2021). The effects of regional trade integration and renewable energy transition on environmental quality: Evidence from South Asian neighbors. Bus. Strategy Environ. 30 (8), 4154–4170. doi:10.1002/bse.2862
Murshed, M., and Tanha, M. M. (2021). Oil price shocks and renewable energy transition: Empirical evidence from net oil-importing South Asian economies. Energy Ecol. Environ. 6 (3), 183–203. doi:10.1007/s40974-020-00168-0
Nawaz, K., Lahiani, A., and Roubaud, D. (2019). Natural resources as blessings and finance-growth nexus: A bootstrap ARDL approach in an emerging economy. Resour. Policy 60, 277–287. doi:10.1016/j.resourpol.2019.01.007
Nie, X., Wu, J., Chen, Z., Zhang, A., and Wang, H. (2021). Can environmental regulation stimulate the regional porter effect? Double test from quasi-experiment and dynamic panel data models. J. Clean. Prod. 314, 128027. doi:10.1016/j.jclepro.2021.128027
Østergaard, P. A., Duic, N., Noorollahi, Y., and Kalogirou, S. A. (2021). Recent advances in renewable energy technology for the energy transition. Renew. Energy 179, 877–884. doi:10.1016/j.renene.2021.07.111
Pan, X., Uddin, M. K., Han, C., and Pan, X. (2019). Dynamics of financial development, trade openness, technological innovation and energy intensity: Evidence from Bangladesh. Energy 171, 456–464. doi:10.1016/j.energy.2018.12.200
Pesaran, M. H., Shin, Y., and Smith, R. J. (2001). Bounds testing approaches to the analysis of level relationships. J. Appl. Econ. Chichester. Engl. 16 (3), 289–326. doi:10.1002/jae.616
Pietrzak, M. B., Igliński, B., Kujawski, W., and Iwański, P. (2021). Energy transition in Poland—Assessment of the renewable energy sector. Energies 14 (8), 2046. doi:10.3390/en14082046
Pirgaip, B., and Dinçergök, B. (2020). Economic policy uncertainty, energy consumption and carbon emissions in G7 countries: Evidence from a panel granger causality analysis. Environ. Sci. Pollut. Res. 27 (24), 30050–30066. doi:10.1007/s11356-020-08642-2
Qiu, S., Wang, Z., and Geng, S. (2021). How do environmental regulation and foreign investment behavior affect green productivity growth in the industrial sector? An empirical test based on Chinese provincial panel data. J. Environ. Manag. 287, 112282. doi:10.1016/J.JENVMAN.2021.112282
Rafique, M. Z., Nadeem, A. M., Xia, W., Ikram, M., Shoaib, H. M., and Shahzad, U. (2022). Does economic complexity matter for environmental sustainability? Using ecological footprint as an indicator. Environ. Dev. Sustain. 24 (4), 4623–4640.
Ramzan, M., Raza, S. A., Usman, M., Sharma, G. D., and Iqbal, H. A. (2022a). Environmental cost of non-renewable energy and economic progress: Do ICT and financial development mitigate some burden? J. Clean. Prod. 333, 130066. doi:10.1016/j.jclepro.2021.130066
Ramzan, M., Razi, U., Quddoos, M. U., and Adebayo, T. S. (2022b). Do green innovation and financial globalization contribute to the ecological sustainability and energy transition in the United Kingdom? Policy insights from a bootstrap rolling window approach. Sustain. Dev. doi:10.1002/sd.2399
Razzaq, A., Sharif, A., Najmi, A., Tseng, M. L., and Lim, M. K. (2021). Dynamic and causality interrelationships from municipal solid waste recycling to economic growth, carbon emissions and energy efficiency using a novel bootstrapping autoregressive distributed lag. Resour. Conservation Recycl. 166, 105372. doi:10.1016/j.resconrec.2020.105372
Razzaq, A., Wang, S., Adebayo, T. S., and Al-Faryan, M. A. S. (2022). The potency of natural resources on ecological sustainability in PIIGS economies. Resour. Policy 79, 102941. doi:10.1016/j.resourpol.2022.102941
Ren, S., Hao, Y., and Wu, H. (2022). Digitalization and environment governance: Does internet development reduce environmental pollution? J. Environ. Plan. Manag., 1–30. doi:10.1080/09640568.2022.2033959
Samour, A., and Pata, U. K. (2022). The impact of the US interest rate and oil prices on renewable energy in Turkey: A bootstrap ARDL approach. Environ. Sci. Pollut. Res. 29 (33), 50352–50361. doi:10.1007/s11356-022-19481-8
Shafiullah, M., Miah, M. D., Alam, M. S., and Atif, M. (2021). Does economic policy uncertainty affect renewable energy consumption? Renew. Energy 179, 1500–1521. doi:10.1016/j.renene.2021.07.092
Shahbaz, M., Sinha, A., Raghutla, C., and Vo, X. V. (2022). Decomposing scale and technique effects of financial development and foreign direct investment on renewable energy consumption. Energy 238, 121758.
Shang, Y., Han, D., Gozgor, G., Mahalik, M. K., and Sahoo, B. K. (2022). The impact of climate policy uncertainty on renewable and non-renewable energy demand in the United States. Renew. Energy 197, 654–667. doi:10.1016/j.renene.2022.07.159
Singh, H. V., Bocca, R., Gomez, P., Dahlke, S., and Bazilian, M. (2019). The energy transitions index: An analytic framework for understanding the evolving global energy system. Energy Strategy Rev. 26, 100382.
Su, C. W., Khan, K., Umar, M., and Chang, T. (2022). Renewable energy in prism of technological innovation and economic uncertainty. Renew. Energy 189, 467–478. doi:10.1016/j.renene.2022.02.110
Sun, Y., Bao, Q., Siao-Yun, W., Islam, M. ul, and Razzaq, A. (2022). Renewable energy transition and environmental sustainability through economic complexity in BRICS countries: Fresh insights from novel Method of Moments Quantile regression. Renew. Energy 184, 1165–1176. doi:10.1016/j.renene.2021.12.003
Tao, R., Umar, M., Naseer, A., and Razi, U. (2021). The dynamic effect of eco-innovation and environmental taxes on carbon neutrality target in emerging seven (E7) economies. J. Environ. Manag. 299, 113525. doi:10.1016/j.jenvman.2021.113525
Usman, M., and Hammar, N. (2021). Dynamic relationship between technological innovations, financial development, renewable energy, and ecological footprint: Fresh insights based on the STIRPAT model for Asia pacific economic cooperation countries. Environ. Sci. Pollut. Res. 28 (12), 15519–15536. doi:10.1007/s11356-020-11640-z
Vanegas Cantarero, M. M. (2020). Of renewable energy, energy democracy, and sustainable development: A roadmap to accelerate the energy transition in developing countries. Energy Res. Soc. Sci. 70, 101716. doi:10.1016/j.erss.2020.101716
Wang, H., and Wang, M. (2020). Effects of technological innovation on energy efficiency in China: Evidence from dynamic panel of 284 cities. Sci. Total Environ. 709, 136172. doi:10.1016/j.scitotenv.2019.136172
Wang, X., and Huang, Y. (2021). The heterogeneous impact of environmental regulations on low-carbon economic transformation in China: Empirical research based on the mediation effect model. Greenh. Gas. Sci. Technol. 11 (1), 81–102. doi:10.1002/ghg.2033
Xu, S. (2021). The paradox of the energy revolution in China: A socio-technical transition perspective. Renew. Sustain. Energy Rev. 137, 110469. doi:10.1016/j.rser.2020.110469
Xu, Z. (2020). Economic policy uncertainty, cost of capital, and corporate innovation. J. Bank. Finance 111, 105698. doi:10.1016/j.jbankfin.2019.105698
Zhang, Y., Qamruzzaman, M., Karim, S., and Jahan, I. (2021). Nexus between economic policy uncertainty and renewable energy consumption in bric nations: The mediating role of foreign direct investment and financial development. Energies 14 (15), 4687. doi:10.3390/en14154687
Keywords: economic policy uncertainty, technology innovation, environmental governance, green energy transition, bootstrapping ARDL
Citation: Yu Z and Guo X (2023) Influencing factors of green energy transition: The role of economic policy uncertainty, technology innovation, and ecological governance in China. Front. Environ. Sci. 10:1058967. doi: 10.3389/fenvs.2022.1058967
Received: 30 September 2022; Accepted: 07 November 2022;
Published: 17 February 2023.
Edited by:
Asif Razzaq, Dalian University of Technology, ChinaReviewed by:
Xiaodong Yang, Xinjiang University, ChinaTayyaba Rani, Xi’an Jiaotong University, China
Copyright © 2023 Yu and Guo. This is an open-access article distributed under the terms of the Creative Commons Attribution License (CC BY). The use, distribution or reproduction in other forums is permitted, provided the original author(s) and the copyright owner(s) are credited and that the original publication in this journal is cited, in accordance with accepted academic practice. No use, distribution or reproduction is permitted which does not comply with these terms.
*Correspondence: Zhichao Yu, eXV6aGljaGFvMTIxNEBzaW5hLmNu