- 1School of Architecture, Southwest Jiaotong University, Chengdu, China
- 2School of Design, Southwest Jiaotong University, Chengdu, China
As a critical transportation infrastructure, high-speed rail (HSR) greatly enhances accessibility and shortens the spatial-temporal distance among cities. It is well documented that HSR significantly impacts regions and cities’ economic development and spatial structure. The proportion and frequency of business passenger trips are increasing yearly, and the demand for “station as the final destination” is becoming more and more prominent. Given the pivotal role of the design and construction of HSR station areas in achieving “station as the final destination,” the study of their development characteristics and patterns has important implications for urban planning. Previous studies have focused extensively on the macro impact of the HSR operation on regional economies, urban industries, and tourism development, whereas only a few were conducted at the station level. Furthermore, the business-commercial agglomeration effects of the HSR operation on the development and construction of station areas have neither been studied nor accurately measured. To fill this gap, we first constructed a panel data set consisting of the point of interest (POI) data, China City Statistical Yearbook data, and the HSR station operation data from 2012 to 2017. Next, we developed difference-in-differences (DID) models to decipher the impact of the HSR operation on the station-level business and commercial agglomeration. The results show that the HSR operation has increased the business-commercial agglomeration index (BCAI), the commercial agglomeration index (BAI), and the business agglomeration index (CAI) by 28.3%, 29%, and 21.3%, respectively. In other words, the HSR operation has significant business-commercial agglomeration effects in the station area, and the agglomeration effect size of business is more extensive than that of commerce. Interestingly, the BCAI grew slowly in the first 3 years after the HSR operation but started to rise much faster from the fourth year, which HSR’s catalytic effects can explain. The results also reveal that the business-commercial agglomeration effects have a clear spatial threshold as the BCAI tends to decrease from 1500 m to 3000 m away from HSR stations. The plausibility of the results has been confirmed by the parallel trend, placebo, and robustness tests.
1 Introduction
Transportation infrastructure is generally considered one key factor in shortening travel time and promoting urban economic growth (Bhatta and Drennan, 2003; Hong et al., 2011; Diao, 2018; Deng et al., 2020). An efficient transportation system not only improves accessibility among cities but also plays a vital role in shaping urban spatial structure (Lakshmanan, 2011; Yu et al., 2013; Yang et al., 2019; Yang et al., 2020a; Zhou X. et al., 2022). Investments in transportation infrastructure can accelerate industrial agglomeration and meet people’s demand for goods and high-quality services. Since the beginning of the 21st century, high-speed rail (HSR) has become a significant emerging inter-city transportation service. The rapid development of the HSR has reshaped the territory’s spatial structure. In recent decades, the benefits of HSR can be characterized by high speed, high frequency, high accessibility, travel time reliability, low-carbon life, a greater chance of commuting between the two cities, and relatively low fares (compared with other transport modes, especially flights), have been widely acknowledged and emphasized (Givoni, 2006; Campos and de Rus, 2009; Zhou Y. et al., 2022). HSR weakens the absolute boundaries of natural barriers and spatial and temporal distances, creating positive spillover and agglomeration effects on cities and surrounding areas. China, Spain, Japan, Germany, France, and other countries are planning to or have already implemented large-scale HSR constructions. Among them, China’s HSR mileage ranks first across the globe. In 2003, Qinhuangdao-Shenyang HSR was operated, which can be considered the first attempt at China’s HSR. In 2008, the first real HSR in the history of China, the Beijing-Tianjin Intercity Railway, was operated. By the end of 2021, the operating mileage of China’s HSR has reached 40,000 km. China’s “four vertical and four horizontal” HSR corridors have been fully built, and the “eight vertical and eight horizontal” HSR corridors are being densely formed. As a new form of public transportation, HSR promotes urbanization and national economic growth.
As a high-quality transportation infrastructure (public social goods), HSR has various advantages. The most obvious advantage is that HSR improves the travel experiences between cities and shortens commuting time. Other advantages include, but are not limited to, enhancing train punctuality rates, providing comfortable and convenient services, increasing passenger capacity, creating a comfortable travel environment, stimulating urban economic development, promoting regional integration, and stimulating tourism development (Yang et al., 2020b; Gong and Li, 2022; Jiang et al., 2022). Thus, the government has increasingly invested in the construction of the HSR. Although the HSR has a tremendous spatial impact at the national, regional, and urban levels, HSR station areas have received the most direct spatial benefit (Meng, et al., 2018). Specifically, the development of HSR station areas brings opportunities for the construction of new cities and the renewal of old cities, offering possibilities for optimizing urban spatial structure (Dong et al., 2021; Chang et al., 2022). The design and development of HSR station areas is a long-standing topic. Scholars in planning, developers, and governments are all paying attention to the construction of HSR station areas. Corresponding research findings have significant theoretical relevance and positive practical implications.
By compressing the spatial-temporal distance and reducing travel costs, the HSR promotes the agglomeration of economic activities in station areas (Ribalaygua and Perez-Del-Cano, 2019; Cui et al., 2021; Du et al., 2021; Wang et al., 2022). The improved accessibility brings the station areas with the influx of people, logistics, capital, and information. The construction of the HSR has affected the demand for mixed land development, residence, employment, and transportation. In contrast to traditional railway stations’ single transportation function, HSR station areas accommodate travel needs and various business and commercial activities. In other words, HSR station areas are not only transportation network nodes (reflecting transportation value and node value) but also urban public places (reflecting functional value and place value) (Gui et al., 2021; Cummings and Mahmassani, 2022). The HSR helps enhance the network externalities of cities, which can complement or replace the agglomeration externalities of localization. At the same time, the spillover of passenger flow brings development opportunities to station areas. To maximize the benefits created by HSR, urban planners have tried to connect the station area with the city center (or commercial center) to reach a station-city integration situation.
HSR has promoted the regionalization of economic activities and strengthened intercity exchanges. The growth of business ridership with the travel characteristics of short distance, high frequency, and high time value has given rise to the development and agglomeration of commercial, business, and transportation facilities around stations (Wang et al., 2018). It is because that business ridership is less sensitive to the cost of transportation but more sensitive to the cost of time. In other words, business passengers want to reach their destinations quickly. In this regard, “Station as the final destination” is the inherent and essential requirement and the ultimate goal for developing HSR station areas. People have planned many newly-built HSR stations with various commercial and business spaces in station areas, trying to transform the transportation nodes into high-quality urban places. In addition to the drivers of the business ridership demand, economic forces are another critical factor contributing to business and commercial agglomeration. The change in accessibility caused by HSR directly impacts land prices in the station area, resulting in higher land lease prices in areas closer to the station. Specifically, businesses and commerce (e.g., high-end restaurants and business offices) can afford higher land lease prices and tend to congregate in core areas closer to the site. Therefore, business and commercial agglomeration under the market mechanism will be generated in HSR station areas (Wang et al., 2014; Wang et al., 2021).
Previous studies regarding the impact of HSR have primarily focused on the city or regional-level urban economic development, land use, urban vitality, spatial changes in house prices, and tourism (Masson and Petiot, 2009; Chen and Haynes, 2017; He et al., 2018; Yang et al., 2020a; Yang et al., 2020c; Jin et al., 2020; Yang et al., 2021; Wenner and Thierstein, 2022). In contrast, only a few have analyzed the development pattern and formation mechanism of HSR station areas. Furthermore, most of the station-level HSR studies are qualitative case analyses or mainly focus on the impact of the HSR operation on housing prices and economic growth (Geng et al., 2015; Zheng et al., 2019). There is still a lack of quantitative, systematic, and national-level studies on the agglomeration effect of HSR station areas. In recent years, the development of new technologies and the rise of big data, such as points of interest from online maps, cell phone signaling data, etc., have provided solid support and opportunities for studying HSR station areas at a microscopic scale. However, some debate is whether HSR station areas can produce agglomeration effects. However, what is the degree of business and commercial agglomeration generated by HSR? Is there a difference between business agglomeration and commercial agglomeration? These issues are critical to the design and development of HSR station areas.
To address the above questions, based on the data from 2012 to 2017, namely the data on China’s point of interest (POI), China City Statistical Yearbook data, and the HSR station operation data, difference-in-differences (DID) models were established to systematically examine the station-level business-commercial agglomeration effects of the HSR operation. The plausibility and validity of the model results were verified by the parallel trend test, placebo test, and robustness test. This paper contributes to the literature in the following ways: 1) enriching the current theory of the HSR station area development and adding new empirical evidence to the HSR operation; 2) estimating the business-commercial agglomeration effects of China’s HSR station areas in recent years; 3) revealing the spatial distribution patterns of business and commerce land use across different buffers; 4) proposing innovative research directions. Our investigation intended to study the BCAI by using DID models. This paper may be one of the first research efforts and fills a gap in planning and design.
The rest of this paper is structured as follows. Section 2 reviews the study of the impact of the HSR operation on the region, city, and station area. Section 3 describes the research object, the data sources for empirical analysis, and the DID model as the research methodology. Section 4 analyzes the spatial distribution of HSR stations and the business-commercial development in station areas. Section 5 presents the empirical model results and discusses the relationship between the HSR and business-commerce. Section 6 concludes and identifies future research directions.
2 Literature review
2.1 The impact of HSR at the regional and urban level
Extensive literature assesses the regional and urban impacts of the HSR (Table 1). At the regional level, many scholars have pointed out that the HSR operation brings about accessibility improvement (Zhu et al., 2016; Xu et al., 2018; Sahu and Verma, 2022), which facilitates the rapid movement of various factors across different regions. By making developed and peripheral regions closer, the HSR transforms the connectivity of peripheral regions, which provides opportunities for coordinated regional development. The HSR construction has increased the level of the transportation network and expanded the range of urban isochronous circles (Wang et al., 2020). In other words, the HSR has expanded the hinterland of the central city and triggered a spatial reshaping. Dong et al. (2020) have confirmed that the HSR can connect megacities with second-tier cities at the regional level, facilitating the flow and optimal allocation of resources. Specifically, the HSR operation can reduce the time cost of people flow and increase the spillover level of highly skilled people and knowledge.
At the urban level, the topic of the HSR has also received much attention (Garmendia et al., 2012; Long et al., 2018; Deng et al., 2019). Shao et al. (2017) showed that HSR has a positive impact on urban service agglomeration and has a significant impact on productive services. By studying the tourism industry in Spain for over 15 years, Albalate and Fageda (2016) showed that high-speed rail may positively impact tourism and be an alternative to air. Dai et al. (2018) studied 19 sub-sectors of industries in cities along the Beijing-Shanghai HSR line and found that the HSR has agglomeration and diffusion effects on tertiary industries. In general, the HSR has a positive effect on regions and cities (Garmendia et al., 2008; Shaw et al., 2014; Jia et al., 2017; Xu and Huang, 2019).
However, other studies hold a different view (Vickerman, 1997; Ortega et al., 2012; Geng et al., 2015; Mota et al., 2017; Li et al., 2019). These scholars have argued that the HSR has negatively affects regions and cities or that the impact mechanisms are complex and unclear. The HSR operation may cause traffic congestion, environmental damage, and noise and affect the development of other industries (such as the airline industry). Chen, et al. (2016) have found that the HSR operation leads to uneven regional economic development. The HSR enhances the agglomeration capacity of central nodes, while it may cause the loss of production factors in less developed areas. Chen et al. (2016) believed that the role of high-speed rail in affecting the economy was ambiguous. The economic impact of the HSR investment can be achieved by interacting with other factors, such as land use. This impact can be positive or negative. The impact may increase in the short term but may reduce economic growth in the periphery in the long term. It is worth noting that the HSR may have increased environmental costs (Givoni, 2006; Westin and Kageson, 2012).
2.2 The impact of HSR at station area level
The development of HSR station areas benefits from accessibility improvement. The HSR operation has caused changes in the land use of station areas, increasing people’s social and economic activities. Diversified industries are clustering in HSR station areas, especially commerce, business, real estate, leisure and entertainment, tourism, etc. In Europe, the development of many HSR station areas plays an important role in urban regeneration (Bertolini, 2000; Hickman et al., 2021). De Wijs et al. (2016) saw many similarities between redeveloping HSR station areas and smart cities like digital parking systems, electronic schedules for public transportation, and sustainable energy buildings. In Asia, the construction of HSR station areas is often used as one of the critical factors in the construction of new towns. The HSR station is an essential catalyst for urban transformation in the new era. The success or failure of the development of HSR station areas can directly affect whether the city or region can successfully achieve new development (Zou et al., 2016).
Commercial and business land space in HSR station areas has grown (Willigers and van Wee, 2011; Soria Caceres, 2018). Wang et al. (2014) have found that the station area development of the Beijing-Shanghai line is much greater than that of the Wuhan-Guangzhou line, and the proportion of commercial-type land use is also higher. According to the gravity model, distance affects the strength of commercial exchange. That is, the HSR shortens the time between cities, increases commercial activities, and expands the scope of the market. Beckerich et al. (2019) have argued that types of business and commerce differ between central and peripheral HSR stations. Companies in the peripheral HSR station areas want to be adjacent to the area’s industrial bases, while companies in the central HSR station areas want to find more customers. Other scholars have shown that by promoting new construction or renewal of station areas, the HSR strengthens the public space attributes of station areas and enhances commercial and business activities in station areas (Halbert, 2004; Yin et al., 2015).
A consensus has been formed on the distribution characteristics of the HSR station area. It is generally believed that the first circle is mainly for distributing transportation services and business functions; the second is mainly for business-commercial and cultural entertainment; the third is mainly for residential, R&D, and other functions. However, scholars differ significantly in defining the scope of impact of HSR stations depending on different study perspectives and purposes. Lin. (2011) has shown that the HSR station impact area is the one accessible by a 20-min walk (about 1500 m) through a study of the walking endurance time of HSR passengers. Some other literature introduced the 2 km radius’ scope around the HSR station as the core impact area (Zhao and Chen, 2015; Cao et al., 2020). To verify the model’s validity, we estimated the model according to different circle ranges. Specifically, each HSR station area was divided into 500 m units for sensitivity tests with different distance (1500 m, 2000 m, 2500 m, 3000 m) thresholds.
2.3 Thrust of this study
As shown above, many studies have investigated the impact role of HSR in regions, cities, and station areas, mainly focusing on the impact of HSR on transportation, economy, and industry. Some research results in the station area have mainly addressed traffic accessibility, land use, housing prices, and business siting. The literature lacks a quantitative assessment of the commercial and business impact of the HSR operation on the station areas. Studying the business-commercial agglomeration effects in HSR station areas can provide an innovative and practical perspective to explain the impact of the HSR on station area development. We used POI data to evaluate the agglomeration effect of the HSR operation and to explore the differences between commercial and business characteristics in different circles.
3 Data and methodology
3.1 Study area
According to the “Medium-long-Term Railway Network Plan,” the HSR is defined as a railroad system operating at a speed of over 200 km per hour. Since the HSR operation started, its ridership has progressively increased, with an average annual growth of 61.8% from 2008 to 2019 (Figure 1). The proportion of HSR ridership in railroad ridership also increased yearly to 70.7% of 2019 (http://www.china-railway.com.cn). The HSR ridership provides support for the developmental construction of station areas. Taking into account the difficulty of access to the data, we empirically investigated the business-commercial agglomeration effects of HSR station areas in China by using the panel data of 110 HSR stations from 2012 to 2017. Stations are mainly distributed along the high-speed railway lines of Chengdu-Chongqing, Nanning-Anqing, Hefei-Fuzhou, Xi’an-Chengdu, Naning-Guangzhou, Xiamen-Shenzhen, Shendan-Dandong, and some others (Figure 2).
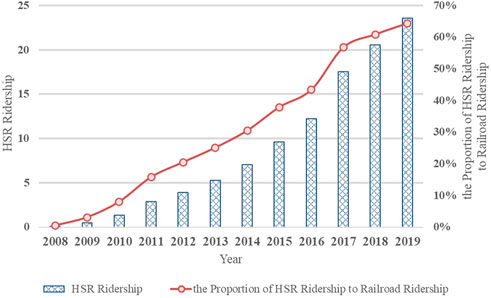
FIGURE 1. HSR ridership and the proportion of HSR ridership to railroad ridership from 2008 to 2019.
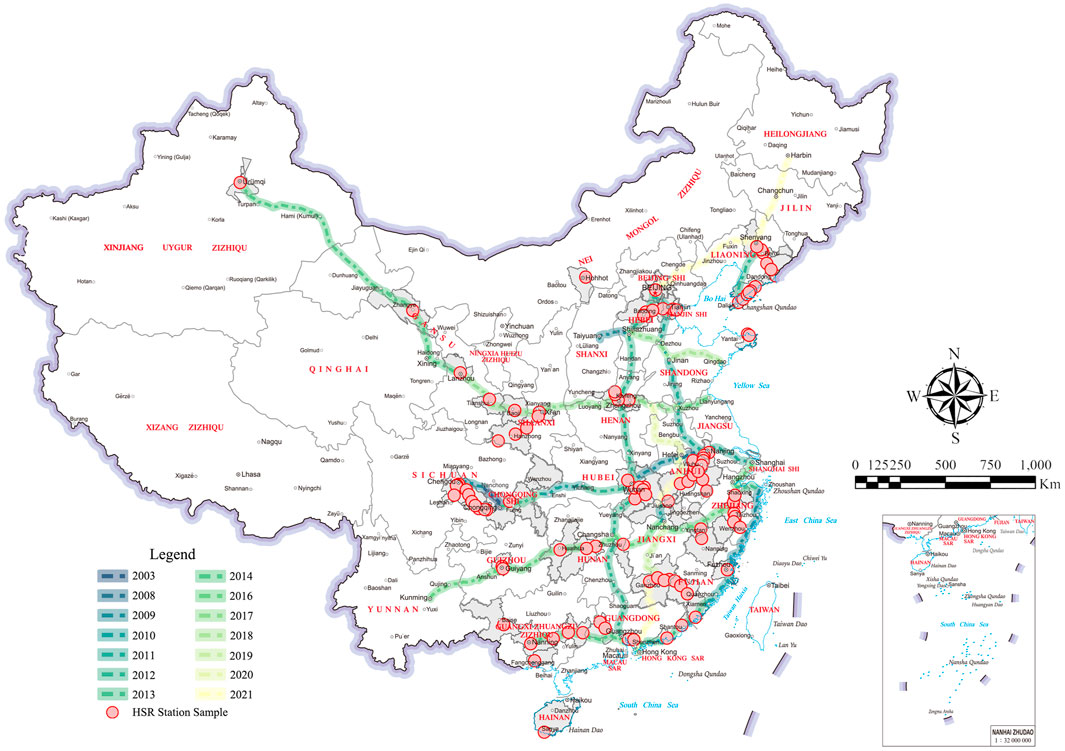
FIGURE 2. The Study on the spatial distribution of HSR stations. Note: The original map (No. GS (2019)1686) was from the China National Natural Resources Standard Map Service website (http://bzdt.ch.mnr.gov.cn/). The authors mapped the spatial distribution of HSR stations based on it.
3.2 Data and variables
The business-commercial agglomeration effects are the most basic and vital indicator of the place value in HSR station areas. Traditional data is mainly used to obtain business and commercial information by mapping site attributes through satellite maps. In short, it is more difficult to obtain data directly from the Natural Resources Bureau (due to security concerns). Mapping the land information of HSR station areas by hand drawing is enormous. Results are also subject to some inaccuracies due to the differences in the mappers. Fortunately, open access to big data in recent years has provided reliable data for research. Business-commercial agglomeration index (BCAI) is mainly reflected by the corresponding POI density of HSR station areas. POI data is typical of big geographic data, using location information to represent entity geographic attributes. POI information mainly includes latitude-longitude coordinates, names, types, address information, etc.
The data processing process is as follows. Firstly, we collected the city POI dataset from 2012 to 2017 (http://sdsp.ecnu.edu.cn/) and removed the data outside the 3 km buffer zone in HSR station areas (Shen et al., 2014; Wang and Gu, 2019; Deng et al., 2020; Wang et al., 2022). Secondly, we used ArcGIS software to extract the annual POI data of high-speed railway station areas. From a buffer of 1500 m, a dataset of HSR station areas was created for every 500 m increase. Finally, this study was to assess the changes in the BCAI that occurred before and after the HSR operation started. Therefore, we filtered the data from the HSR station area datasets for the types of catering services, shopping services, accommodation services, car sales, business residences, corporate enterprises, and financial and insurance services. These seven types of POI data measured the BCAI. The formula is as follows.
where
Where
where
In general, the business and commercial agglomeration effects of the HSR station areas may be influenced by various factors. In addition to the BAI and the CAI, we collected the economic and social development indicators of the cities having the HSR as variables. These variables included urban resident population, the share of secondary and tertiary industries in GDP, real estate investment per capita, number of industrial enterprises, number of employed persons, average wages, and urban road area per capita. The data on urban economic and social development were obtained from China City Statistical Yearbook. The HSR construction data came from the National Railway Administration of the People’s Republic of China (http://www.nra.gov.cn/), China State Railway Group Co., Ltd. (http://www.china-railway.com.cn/), and China Railway 12,306 website (https://www.12306.cn/index/). The high-speed trains in our study were those named with C, D, and G as the first letter. The latitude and longitude data of HSR stations were obtained through the API interface of Gaode Map (https://lbs.amap.com/tools/picker). Table 2 summarizes the definitions and units of the variables in this study, and Table 3 shows the descriptive statistics for all variables.
3.3 Methodology
The natural experiment evaluation method, typically such as the DID model, can effectively identify causality and address the endogeneity of omitted variables (Zhang et al., 2020b; Yang et al., 2022). The DID model effectively identifies policy or exogenous shocks (e.g., HSR operation start) under the premise of controlling for other factors. The model can remove the time effect and separate the policy treatment effect. Therefore, the DID model is now commonly used in studies on the impact of the HSR operation on urban development. Figure 3 explains the basic ideas and differences between the traditional DID model and the multi-period DID model in diagrammatic language.
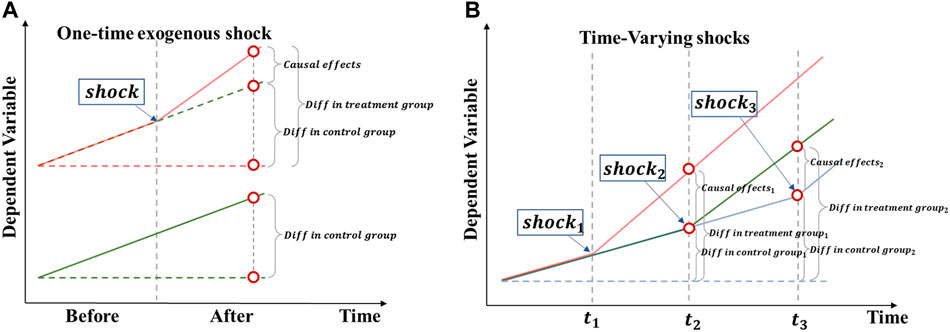
FIGURE 3. The graphical illustration of the traditional DID model and the multi-period DID model. (A) The traditional DID model. (B) The multi-period DID model.
The different opening dates of the 110 HSR stations do not comply with the traditional DID requirement of the same policy implementation time point. To accurately assess the commercial and business impact of the HSR operation, we used a multi-period DID model to measure the time-averaged effects of different policy implementations. In the DID model, samples were divided into treatment and control groups. The control group provided a counterfactual analysis. However, this paper does not have a control group utterly untouched by the policy intervention, meaning that all sample areas began the HSR operation at different times. When we calculated the treatment effects, samples without the HSR were used as a control group for those already having the HSR. The model was established for time-fixed effects and urban-fixed effects.
where
where
where
4 The characteristics of the business-commercial agglomeration in HSR station areas
We sorted out the business and commercial POI data for all HSR station areas and then compared the BCAI for different years for analysis. Figure 4 illustrates the spatial distribution of the BCAI in HSR station areas from 2012 to 2017. First, the business and commerce in HSR station areas have changed considerably. Before the HSR operation, business and commercial development was low, and even some samples presented extremely low levels. Stations with high BCAI are mainly located in the eastern coastal region, most of which were upgraded to HSR stations. Many new HSR station areas were idle before the HSR operation, with much-undeveloped land to use. After the HSR started operation, the BCAI in HSR station areas grew faster. HSR stations in provincial cities have better infrastructure for rapid business and commercial development, such as Chengdu, Zhengzhou, Wuhan, Lanzhou, and other cities.
The BCAI varies significantly from one HSR station area to another. The spatial distribution results show heterogeneity in the growth of the BCAI in station areas. The BCAI in the eastern region was growing faster than that in the western and central regions, such as Yiwu Station, Nanning East Station, and Ma’anshan East Station, whose business and commercial growth rate reached 40%–60%. In addition, BCAI growth rates are higher for HSR stations closer to the city center in large cities than that for those in suburban or distant suburban areas. For example, the average growth rate of the BCAI at Chengdu South Station after the HSR operation in 2015 was 23.2% (Figure 5). The reason for this is that the excellent location of the city’s south station provided favorable conditions for the rapid growth of the BCAI. Chengdu South Station is located 5.8 km from the traditional Chengdu city center and 2.7 km from the new city center (the Financial City). The HSR, as a catalytic point, has dramatically stimulated business and commercial development in the station areas.
Additionally, the spatial sectors of HSR station areas show considerable differences. For the development direction vertical to the HSR line, the business and commerce in the sectors with the direction close to the city center or the original built-up area of the city tends to develop better, and the business and commerce in sectors closer to the city center have a relatively balanced development. There is no significant difference in land value due to the slight difference in transport conditions between the two sides of the zone. The analysis based on the standard deviation ellipse can further illustrate the sector development pattern. For example, the center of the standard deviation ellipse of Chengdu South Station from 2012 to 2014 was located on the station’s south side. From 2015 to 2017, the southern sector improved, and the center of the standard deviation ellipse started to move southward. Before the HSR operation, the BCAI was low, mainly distributed in 1000–1500 m. With the HSR operation, the BCAI proliferates in the 500–1000 m range. The overall spatial evolution of the station area shows three stages: the slow development period, the rapid growth period, and the stable period. It indicates that during the evolution of business and commerce in HSR station areas, the HSR agglomeration effect has promoted changes in the functional distribution, spatial structure, and business composition in station areas.
In summary, the role of transportation infrastructure in the relative attractiveness to corporate businesses, catering, shopping, and accommodations is often considered critical. The HSR operation has reduced people’s travel time costs and promoted population mobility. Low costs mean that people can overcome the spatial barriers between cities, which expands urban economic activity. As areas that benefit most directly from improved accessibility, HSR station areas tend to have agglomeration effects. The HSR station areas generate more significant attraction for catering services, shopping services, accommodation services, car sales, business residences, corporate enterprises, and financial and insurance services.
5 Results
5.1 Baseline results
We established three benchmark regression models by using the BCAI, the BAI, and the CAI as dependent variables to explain the “net effect” of the HSR operation on the business and commercial agglomeration in HSR station areas. Table 4 reports the regression results of three double difference models controlling for “city fixed effects” and “time fixed effects”. First, the BCAI and the BAI are significant at 1%, the CAI is significant at 5%, and the POST_HSR coefficient estimates are all positive. The results show that the HSR operation has agglomeration effects on business and commerce in station areas. This finding is consistent with our expectations. Second, Model one shows that the BCAI improves by 28.3% after the HSR operation, which proves that HSR stations have a significant spillover effect. In other words, the HSR stations make accessibility greatly enhanced, creating favorable conditions for the development and construction of station areas. Finally, Model 2 and Model 3 indicate that the agglomeration effect of commerce is greater than that of business, with the BAI increase of 21.3% and the CAI increase of 29% after the HSR operation. It is because most customers demand commercial facilities, and business customers often favor business facilities. The high ridership creates significant commercial opportunities and development potential for the station areas.
The baseline regression model also shows that the share of the tertiary sector, the number of urban industrial enterprises, and the number of employees significantly contributes to the BCAE in general. The results of the control variables are consistent with our expectations and the previous studies on the influence on the development and construction of station areas. In models 4 and 6, the estimated coefficient of the tertiary sector share (lnGDP3) is significantly positive. In model 6, the estimated coefficient of the share of the secondary sector (lnGDP2) is significantly positive. It indicates that regions with a larger share of the tertiary sector will have a higher BCAI based on the control for other variables. One possible reason is that cities with a high share of tertiary industries can provide more labor and production factors, generating more demand for transportation. With the improved accessibility of HSR stations, the population and elements in the city move to the station areas, promoting the construction of commercial development. The estimated coefficients of the number of urban industrial enterprises (lnENTERPRISE) in models 4 and 5 are significantly positive, indicating that cities with more employment opportunities have tremendous potential for development. Enterprises often look to expand revenue by reducing costs, and the HSR operation reduces time and transportation costs. That increases the opportunities for enterprises to be located in the station area, which in turn promotes the development of station areas. The estimated coefficients of urban employment (lnEMPLOYMENT) in models 4 and 6 are significantly positive, indicating that urban employment promotes business development in station areas. During the development of HSR station areas, the labor force begins to transfer to the station areas, which can support the pooling and development of resources.
5.2 Parallel trend test and dynamic effects
With the HSR operation, business and commerce in station areas have been developed to a certain extent. To test how the impact of HSR operation on the BCAI changes over time and the equilibrium trend assumption of the baseline regression model, we included dummy variables before and after the HSR operation started, respectively. In our study, the equilibrium trend test was divided into the test before the HSR operation and the test after the HSR operation started. If all the policy impact effects before the HSR operation show no correlation, the experimental and control groups will have the same trend. If the policy impact after the HSR operation start is relevant, it shows that the HSR operation makes the experimental and the control groups will have different trends. Therefore, we chose the base period 1 year before the HSR operation and established the following regression model.
In the model,
The model regression results show that the estimated coefficients of the dummy variables are all insignificant before the HSR operation, and the estimated coefficients of the dummy variables are all positively significant after the HSR operation start. Our model satisfies the parallel trend assumption. Figure 6 depicts the estimated coefficients of our interest in Model 7, indicating that the BACI shows an increasing trend year by year after the HSR operation start. Interestingly, 4 years after the HSR operation started, the BACI has grown much faster than that in previous years. Overall, the HSR operation has a significant impact on the BACI.
5.3 Placebo test
The purpose of the placebo test is for the presence of chance in the results of the DID model. We verified that the estimates were not biased by conducting a placebo test on the main results of this paper by randomly selecting HSR stations from samples. If the estimated coefficient of the regression of the placebo treatment variable remains significantly positive, it indicates that the original model is biased. That is, changes in the explanatory variables may be influenced by other factors. In contrast, the regression coefficients for the placebo treatment variables do not deviate significantly from zero if there is no significant omitted variable bias. We have repeated the above experiment 500 times to avoid small probability events. Figure 7 portrays the kernel density distribution of the estimated coefficients of the 500 placebo treatment variables and the distribution of corresponding p-values. The placebo test results indicate that the means of the estimated coefficients are around 0, and the vast majority of p-values are greater than 0.1 (horizontal red dashed line). Also, the true estimate of the DID model (vertical dashed line) is significantly larger than the maximum value of 500 random estimates. Overall, the model estimation results are not coincidental, and there is no serious bias due to omitted variables.
5.4 Robustness test
Some may argue that the current model evaluation results are based on the 1500 m station-area impact range, and the station-area impact range threshold setting may influence the true results. Thus, we decided to develop the model robustness test further. The level of urban economic development affects the agglomeration effects of HSR station areas and may lead to differences in the influence areas of different HSR station areas. As a result, we have conducted sensitivity tests with different thresholds to test the robustness of the DID model. Specifically, we re-estimated the impact of HSR operation on the BACI by establishing a model with thresholds for the impact range of different HSR station areas. Table 5 shows the model results for the 1500 m, 2000 m, 2500 m, and 3000 m thresholds. All the estimated coefficients are significantly positive, which corroborates the robustness of the model. Interestingly, the estimates for the different thresholds are 0.283, 0.146, 0.140, and 0.137, respectively. The BACI is lower as the distance from the HSR station becomes further, which also verifies the circle effect in HSR station areas.
6 Conclusion and discussion
The HSR in China is in a rapid construction phase, and many cities are looking to construct new cities with the enhancement of the HSR stations. The proportion of business passenger travel is increasing yearly, and the demand for “station as the final destination” is becoming more and more prominent. Therefore, the HSR station area has become one of the key development objectives in urban planning. Previous studies have focused extensively on the macro impact of the HSR operation on regional economies, urban industries, and tourism development, whereas only a few were conducted at the station level. Furthermore, how to accurately understand and evaluate the effect of the HSR operation and reveal the development rules of HSR station areas is of great significance. The study presumed that the operation of HSR can bring business and commercial agglomeration effects in station areas. Given this, we collected POI data, China City Statistical Yearbook data, and the HSR station operation data from 2012-2017 and developed several DID models to verify our hypotheses. We measured business and commercial development in 110 HSR station areas in China. The empirical findings provide strong evidence and support the arguments that we have presented. The parallel trend, placebo, and robustness tests confirmed the results’ plausibility and validity. Current studies tend to focus on the impact of the HSR operation on regional economic integration, urban industrial carbon emissions, tourism development, and station area economies. We specifically analyzed the agglomeration effects of the HSR operation on business and commerce in station areas and revealed the spatial distribution patterns of business and commerce land use across different buffers, which enriches the existing literature.
The development and construction of HSR station areas is an academic and fundamental practical issue. Many countries, such as Japan, Spain, Germany, and South Korea, have already built large-scale HSRs. Although the HSR has undergone extensive construction in China, the operation cycle is relatively short. Some scholars have pointed out that a mature HSR station area generally requires 25–30 years of development. Therefore, our findings are instructive for the HSR station areas under construction and for future HSR station areas. Specifically, the HSR operation has increased the BCAI, the BAI, and the CAI by 28.3%, 29%, and 21.3%, respectively. In other words, the HSR operation has significant business-commercial agglomeration effects in the station area, and the agglomeration effect of business is significantly more than that of commerce. These findings provide recommendations for practitioners and researchers to design and plan station areas. However, the purpose of this article is not to provide a detailed interpretation of the case but to highlight an innovative perspective and research framework. Our research results have specific theoretical and practical significance for the construction of HSR station areas and can provide a reference for the formulating future urban planning-related policies and government decisions.
We must acknowledge that this study is not without limitations. First, the HSR operation is not only beneficial to economic development and land development in station areas. Some other benefits and costs warrant further exploration. Second, we have mainly used POI data and China City Statistical Yearbook data. The recent emergence of new big data, such as Baidu heat data, cell phone signaling data, Tencent location data, and Weibo check-in data, has enabled us to analyze HSR station areas more comprehensively. Future research may leverage new types of big data and use more advanced machine learning techniques to explore the complex development dynamics of the HSR station areas.
Data availability statement
The raw data supporting the conclusion of this article will be made available by the authors, without undue reservation.
Author contributions
All authors contributed to the conception and design of the study. PL, XC, and ML: Methodology and models. PL conceptualization, supervision, and writing—original draft. XC: Questing for the funding support. ML, TY, and BW: Collecting the research data. TY and BW: Validation and writing.
Funding
This study was supported by the National Natural Science Foundation of China (No. U20A20330) and the National Natural Science Foundation of China (No. 51778530).
Conflict of interest
The authors declare that the research was conducted in the absence of any commercial or financial relationships that could be construed as a potential conflict of interest.
Publisher’s note
All claims expressed in this article are solely those of the authors and do not necessarily represent those of their affiliated organizations, or those of the publisher, the editors and the reviewers. Any product that may be evaluated in this article, or claim that may be made by its manufacturer, is not guaranteed or endorsed by the publisher.
References
Albalate, D., and Fageda, X. (2016). High speed rail and tourism: Empirical evidence from Spain. Transp. Res. Part A Policy Pract. 85, 174–185. doi:10.1016/j.tra.2016.01.009
Beckerich, C., Benoit, S., and Delaplace, M. (2019). Are the reasons for companies to locate around central versus peripheral high-speed rail stations different? The cases of reims central station and champagne-ardenne station. Eur. Plan. Stud. 27 (3), 574–594. doi:10.1080/09654313.2019.1567111
Bertolini, L. (2000). Planning in the borderless city: A conceptualisation and an application to the case of station area redevelopment. Town Plan. Rev. 71 (4), 455–475. doi:10.3828/tpr.71.4.2883q121q1671185
Bhatta, S. D., and Drennan, M. P. (2003). The economic benefits of public investment in transportation. J. Plan. Educ. Res. 22 (3), 288–296. doi:10.1177/0739456x02250317
Campos, J., and de Rus, G. (2009). Some stylized facts about high-speed rail: A review of HSR experiences around the world. Transp. Policy 16 (1), 19–28. doi:10.1016/j.tranpol.2009.02.008
Cao, Y., Yu, L., and Li, S. (2020). Spatial evolution of high-speed railway station areas and planning response. City Plan. Rev. 44 (11), 88–96.
Carteni, A., Pariota, L., and Henke, I. (2017). Hedonic value of high-speed rail services: Quantitative analysis of the students' domestic tourist attractiveness of the main Italian cities. Transp. Res. Part A Policy Pract. 100, 348–365. doi:10.1016/j.tra.2017.04.018
Chang, Z., Zheng, L., Yang, T., and Long, F. (2022). High-speed rail, new town development, and the spatial mismatch of land leases in China. Land Use Policy 115, 106014. doi:10.1016/j.landusepol.2022.106014
Chen, Z., and Haynes, K. E. (2017). Impact of high-speed rail on regional economic disparity in China. J. Transp. Geogr. 65, 80–91. doi:10.1016/j.jtrangeo.2017.08.003
Chen, Z., Xue, J., Rose, A. Z., and Haynes, K. E. (2016). The impact of high-speed rail investment on economic and environmental change in China: A dynamic cge analysis. Transp. Res. Part A Policy Pract. 92, 232–245. doi:10.1016/j.tra.2016.08.006
Cui, X., Liang, P., and Fan, L. (2021). Study on the influence mechanism and planning strategy of population agglomeration effect in High-Speed railway station area based on the cases of beijing, shanghai, chongqing and chengdu. J. Hum. Settlements West China 36 (4), 19–28. doi:10.13791/j.cnki.hsfwest.20210403
Cummings, C., and Mahmassani, H. (2022). Does intercity rail station placement matter? Expansion of the node-place model to identify station location impacts on amtrak ridership. J. Transp. Geogr. 99, 103278. doi:10.1016/j.jtrangeo.2022.103278
Dai, X., Xu, M., and Wang, N. (2018). The industrial impact of the Beijing-Shanghai high-speed rail. TRAVEL Behav. Soc. 12, 23–29. doi:10.1016/j.tbs.2018.03.002
De Wijs, L., Witte, P., and Geertman, S. (2016). How smart is smart? Theoretical and empirical considerations on implementing smart city objectives – A case study of Dutch railway station areas. Innovation Eur. J. Soc. Sci. Res. 29 (4), 424–441. doi:10.1080/13511610.2016.1201758
Deng, T., Gan, C., Perl, A., and Wang, D. (2020). What caused differential impacts on high-speed railway station area development? Evidence from global nighttime light data. Cities 97, 102568. doi:10.1016/j.cities.2019.102568
Deng, T., Wang, D., Yang, Y., and Yang, H. (2019). Shrinking cities in growing China: Did high speed rail further aggravate urban shrinkage? Cities 86, 210–219. doi:10.1016/j.cities.2018.09.017
Diao, M. (2018). Does growth follow the rail? The potential impact of high-speed rail on the economic geography of China. Transp. Res. Part A Policy Pract. 113, 279–290. doi:10.1016/j.tra.2018.04.024
Dong, L., Du, R., Kahn, M., Ratti, C., and Zheng, S. (2021). Ghost cities” versus boom towns: Do China's high-speed rail new towns thrive? Regional Sci. Urban Econ. 89, 103682. doi:10.1016/j.regsciurbeco.2021.103682
Dong, X., Zheng, S., and Kahn, M. E. (2020). The role of transportation speed in facilitating high skilled teamwork across cities. J. Urban Econ. 115, 103212. doi:10.1016/j.jue.2019.103212
Du, J., Druta, O., and van Wesemael, P. (2021). Place quality in high-speed rail station areas: Concept definition. J. Transp. Land Use 14 (1), 1165–1186. doi:10.5198/jtlu.2021.1820
Garmendia, M., de Urena, J. M., Ribalaygua, C., Leal, J., and Coronado, J. M. (2008). Urban residential development in isolated small cities that are partially integrated in metropolitan areas by High Speed Train. Eur. Urban Regional Stud. 15 (3), 249–264. doi:10.1177/0969776408090415
Garmendia, M., Romero, V., Maria de Urena, J., Maria Coronado, J., and Vickerman, R. (2012). High-speed rail opportunities around metropolitan regions: Madrid and london. J. Infrastruct. Syst. 18 (4), 305–313. doi:10.1061/(asce)is.1943-555x.0000104
Geng, B., Bao, H., and Liang, Y. (2015). A study of the effect of a high-speed rail station on spatial variations in housing price based on the hedonic model. Habitat Int. 49, 333–339. doi:10.1016/j.habitatint.2015.06.005
Givoni, M. (2006). Development and impact of the modern high-speed train: A review. Transp. Rev. 26 (5), 593–611. doi:10.1080/01441640600589319
Gong, W., and Li, V. J. (2022). The territorial impact of high-speed rail on urban land development. Cities 125, 103581. doi:10.1016/j.cities.2022.103581
Gui, W., Zhang, X., and Wang, A. (2021). Research on spatial planning evaluation of Beijing Shanghai high speed railway station based on node-place model. J. Intelligent Fuzzy Syst. 40 (1), 733–743. doi:10.3233/jifs-200712
Halbert, L. (2004). The decentralization of intrametropolitan business services in the Paris region: Patterns, interpretation, consequences. Econ. Geogr. 80 (4), 381–404. doi:10.1111/j.1944-8287.2004.tb00244.x
He, Q., He, W., Song, Y., Wu, J., Yin, C., and Mou, Y. (2018). The impact of urban growth patterns on urban vitality in newly built-up areas based on an association rules analysis using geographical 'big data. Land Use Policy 78, 726–738. doi:10.1016/j.landusepol.2018.07.020
Hickman, R., Garcia, M. M., Arnd, M., and Peixoto, L. F. G. (2021). Euston station redevelopment: Regeneration or gentrification? J. Transp. Geogr. 90, 102923. doi:10.1016/j.jtrangeo.2020.102923
Hong, J., Chu, Z., and Wang, Q. (2011). Transport infrastructure and regional economic growth: Evidence from China. Transportation 38 (5), 737–752. doi:10.1007/s11116-011-9349-6
Jia, S., Zhou, C., and Qin, C. (2017). No difference in effect of high-speed rail on regional economic growth based on match effect perspective? Transp. Res. Part A Policy Pract. 106, 144–157. doi:10.1016/j.tra.2017.08.011
Jiang, C., Wang, K., Wang, Q., and Yang, H. (2022). The impact of high-speed rail competition on airline on-time performance. Transp. Res. Part B Methodol. 161, 109–127. doi:10.1016/j.trb.2022.05.004
Jin, S., Yang, J., Wang, E., and Liu, J. (2020). The influence of high-speed rail on ice-snow tourism in northeastern China. Tour. Manag. 78, 104070. doi:10.1016/j.tourman.2019.104070
Lakshmanan, T. R. (2011). The broader economic consequences of transport infrastructure investments. J. Transp. Geogr. 19 (1), 1–12. doi:10.1016/j.jtrangeo.2010.01.001
Li, Y., Yang, B., and Cui, Q. (2019). The effects of high-speed rail on air passenger transport in China. Appl. Econ. Lett. 26 (9), 745–749. doi:10.1080/13504851.2018.1494798
Lin, C. (2011). Research of impact factors of High-speed railways Hub area development in China. Urban Plan. Int. 26 (6), 72–77.
Long, F., Zheng, L., and Song, Z. (2018). High-speed rail and urban expansion: An empirical study using a time series of nighttime light satellite data in China. J. Transp. Geogr. 72, 106–118. doi:10.1016/j.jtrangeo.2018.08.011
Masson, S., and Petiot, R. (2009). Can the high speed rail reinforce tourism attractiveness? The case of the high speed rail between perpignan (France) and barcelona (Spain). Technovation 29 (9), 611–617. doi:10.1016/j.technovation.2009.05.013
Meng, X., Lin, S., and Zhu, X. (2018). The resource redistribution effect of high-speed rail stations on the economic growth of neighbouring regions: Evidence from China. Transp. Policy 68, 178–191. doi:10.1016/j.tranpol.2018.05.006
Mota, C., Lopez, M. A., and Martinez-Rodrigo, A. (2017). A mathematical study of accessibility and cohesion degree in a High-Speed rail station connected to an urban bus transport network. Open Phys. 15 (1), 160–174. doi:10.1515/phys-2017-0017
Ortega, E., Lopez, E., and Monzon, A. (2012). Territorial cohesion impacts of high-speed rail at different planning levels. J. Transp. Geogr. 24, 130–141. doi:10.1016/j.jtrangeo.2011.10.008
Ribalaygua, C., and Perez-Del-Cano, S. (2019). Assessing spatial planning strategy in high-speed rail station areas in Spain (1992-2018): Towards a sustainable model. Eur. Plan. Stud. 27 (3), 595–617. doi:10.1080/09654313.2018.1564189
Sahu, S., and Verma, A. (2022). Quantifying wider economic impacts of high-speed connectivity and accessibility: The case of the Karnataka high-speed rail. Transp. Res. Part A Policy Pract. 158, 141–155. doi:10.1016/j.tra.2022.02.011
Shao, S., Tian, Z., and Yang, L. (2017). High speed rail and urban service industry agglomeration: Evidence from China's Yangtze River Delta region. J. Transp. Geogr. 64, 174–183. doi:10.1016/j.jtrangeo.2017.08.019
Shaw, S., Fang, Z., Lu, S., and Tao, R. (2014). Impacts of high speed rail on railroad network accessibility in China. J. Transp. Geogr. 40, 112–122. doi:10.1016/j.jtrangeo.2014.03.010
Shen, Y., de Abreu e Silva, J., and Martinez, L. M. (2014). Assessing high-speed rail's impacts on land cover change in large urban areas based on spatial mixed logit methods: A case study of madrid atocha railway station from 1990 to 2006. J. Transp. Geogr. 41, 184–196. doi:10.1016/j.jtrangeo.2014.09.007
Soria Caceres, C. H. (2018). Nuevos usos espaciales en las estaciones ferroviarias de alta velocidad/New space use of high speed railway stations. GEOGRAFARES 26, 86–105. doi:10.7147/geo26.21000
Tian, M., Li, T., Ye, X., Zhao, H., and Meng, X. (2021). The impact of high-speed rail on service industry agglomeration in peripheral cities. Transp. Res. Part D Transp. Environ. 93, 102745. doi:10.1016/j.trd.2021.102745
Vickerman, R. (1997). High-speed rail in Europe: Experience and issues for future development. Ann. Regional Sci. 31 (1), 21–38. doi:10.1007/s001680050037
Wang, C., Meng, W., and Hou, X. (2020). The impact of high-speed rails on urban economy: An investigation using night lighting data of Chinese cities. Res. Transp. Econ. 80, 100819. doi:10.1016/j.retrec.2020.100819
Wang, L., and Gu, H. (2019). Studies on China's high-speed rail new town planning and development. Singapore: Springer.
Wang, L., Wang, C., Chen, C., and Gu, H. (2014). Development and planning of the surrounding area of High-Speed rail stations: Based on empirical study of Beijing-Shanghai line. Urban Plan. Forum 4, 31–37.
Wang, L., Yuan, F., and Duan, X. (2018). How high-speed rail service development influenced commercial land market dynamics: A case study of jiangsu province, China. J. Transp. Geogr. 72, 248–257. doi:10.1016/j.jtrangeo.2018.09.010
Wang, L., Zheng, W., He, S., and Wei, S. (2022). Assessing urban vitality and its determinants in high-speed rail station areas in the Yangtze River Delta, China. J. Transp. Land Use 15 (1), 333–354. doi:10.5198/jtlu.2022.2010
Wang, S., Mo, H., Lv, H., Xu, P., and Yin, H. (2021). Industrial structure of high-speed railway station areas under the influence of location: Empirical evidences from POI data. Acta Geogr. Sin. 76 (8), 2016–2031.
Wenner, F., and Thierstein, A. (2022). High speed rail as urban generator? An analysis of land use change around European stations. Eur. Plan. Stud. 30 (2), 227–250. doi:10.1080/09654313.2021.1946485
Westin, J., and Kageson, P. (2012). Can high speed rail offset its embedded emissions? Transp. Res. Part D Transp. Environ. 17 (1), 1–7. doi:10.1016/j.trd.2011.09.006
Willigers, J., and van Wee, B. (2011). High-speed rail and office location choices. A stated choice experiment for The Netherlands. J. Transp. Geogr. 19 (4), 745–754. doi:10.1016/j.jtrangeo.2010.09.002
Xu, W. A., and Huang, Y. (2019). The correlation between HSR construction and economic development - empirical study of Chinese cities. Transp. Res. Part A Policy Pract. 126, 24–36. doi:10.1016/j.tra.2019.05.017
Xu, W., Zhou, J., Yang, L., and Li, L. (2018). The implications of high-speed rail for Chinese cities: Connectivity and accessibility. Transp. Res. Part A Policy Pract. 116, 308–326. doi:10.1016/j.tra.2018.06.023
Yang, J., Yang, R., Chen, M., Su, C. J., Zhi, Y., and Xi, J. (2021). Effects of rural revitalization on rural tourism. J. Hosp. Tour. Manag. 47, 35–45. doi:10.1016/j.jhtm.2021.02.008
Yang, L., Chau, K. W., Szeto, W. Y., Cui, X., and Wang, X. (2020a). Accessibility to transit, by transit, and property prices: Spatially varying relationships. Transp. Res. Part D Transp. Environ. 85, 102387. doi:10.1016/j.trd.2020.102387
Yang, L., Chen, Y., Xu, N., Zhao, R., Chau, K. W., and Hong, S. (2020b). Place-varying impacts of urban rail transit on property prices in Shenzhen, China: Insights for value capture. Sustain. Cities Soc. 58, 102140. doi:10.1016/j.scs.2020.102140
Yang, L., Chu, X., Gou, Z., Yang, H., Lu, Y., and Huang, W. (2020c). Accessibility and proximity effects of bus rapid transit on housing prices: Heterogeneity across price quantiles and space. J. Transp. Geogr. 88, 102850. doi:10.1016/j.jtrangeo.2020.102850
Yang, L., Liang, Y., He, B., Lu, Y., and Gou, Z. (2022). COVID-19 effects on property markets: The pandemic decreases the implicit price of metro accessibility. Tunn. Undergr. Space Technol. 125, 104528. doi:10.1016/j.tust.2022.104528
Yang, L., Zhou, J., Shyr, O. F., and Huo, D. D. (2019). Does bus accessibility affect property prices? Cities 84, 56–65. doi:10.1016/j.cities.2018.07.005
Yin, M., Bertolini, L., and Duan, J. (2015). The effects of the high-speed railway on urban development: International experience and potential implications for China. Prog. Plan. 98, 1–52. doi:10.1016/j.progress.2013.11.001
Yu, N., de Jong, M., Storm, S., and Mi, J. (2013). Spatial spillover effects of transport infrastructure: Evidence from Chinese regions. J. Transp. Geogr. 28, 56–66. doi:10.1016/j.jtrangeo.2012.10.009
Zhang, X., Wu, W., Zhou, Z., and Yuan, L. (2020a). Geographic proximity, information flows and corporate innovation: Evidence from the high-speed rail construction in China. Pacific-Basin Finance J. 61, 101342. doi:10.1016/j.pacfin.2020.101342
Zhang, X., Zhang, W., and Lee, P. T. (2020b). Importance rankings of nodes in the China railway express network under the belt and road initiative. Transp. Res. Part A Policy Pract. 139, 134–147. doi:10.1016/j.tra.2020.07.003
Zhao, Q., and Chen, G. (2015). Influence of hsr station location on the development of surrounding areas: A case study of beijing-shanghai hsr and wuhan-guangzhou hsr. City Plan. Rev. 39 (7), 50–55.
Zheng, L., Long, F., Chang, Z., and Ye, J. (2019). Ghost town or city of hope? The spatial spillover effects of high-speed railway stations in China. Transp. Policy 81, 230–241. doi:10.1016/j.tranpol.2019.07.005
Zhou, X., Chen, S., and Zhang, H. (2022a). Travel on the road: Does China's high-speed rail promote local tourism? Environ. Sci. Pollut. Res. Int. 2022, 1–14. doi:10.1007/s11356-022-22114-9
Zhou, Y., Zhang, L., and Chiaradia, A. J. F. (2022b). Estimating wider economic impacts of transport infrastructure Investment: Evidence from accessibility disparity in Hong Kong. Transp. Res. Part A Policy Pract. 162, 220–235. doi:10.1016/j.tra.2022.05.014
Zhu, Y., Diao, M., and Fu, G. (2016). The evolution of accessibility surface of China in the high-speed-rail era. Environ. Plan. A 48 (11), 2108–2111. doi:10.1177/0308518x15619935
Keywords: high-speed rail, station areas, business-commercial agglomeration effects, business-commercial agglomeration index, difference-in-differences model, spatial distribution patterns
Citation: Liang P, Cui X, Lin M, Yang T and Wu B (2022) High-speed rail effects on station area-level business commercial agglomeration: Evidence from 110 stations in China. Front. Environ. Sci. 10:1045959. doi: 10.3389/fenvs.2022.1045959
Received: 16 September 2022; Accepted: 07 November 2022;
Published: 25 November 2022.
Edited by:
Jun Yang, Northeastern University, ChinaCopyright © 2022 Liang, Cui, Lin, Yang and Wu. This is an open-access article distributed under the terms of the Creative Commons Attribution License (CC BY). The use, distribution or reproduction in other forums is permitted, provided the original author(s) and the copyright owner(s) are credited and that the original publication in this journal is cited, in accordance with accepted academic practice. No use, distribution or reproduction is permitted which does not comply with these terms.
*Correspondence: Xu Cui, Y3VpeHVAc3dqdHUuZWR1LmNu