- 1School of Humanities and Laws, Hebei University of Technology, Tianjin, China
- 2School of Economics, Beijing Technology and Business University, Beijing, China
- 3School of Insurance, University of International Business and Economics, Beijing, China
As digital finance ushers into a new era, carbon emissions in China have been peaking, highlighting the necessity of carbon neutrality. This work uses a dynamic spatial Durbin model, combined with a mediating effect model of the data from 30 provinces from 2011 to 2019, to explore the impact, transmission paths, and spatio-temporal heterogeneity of digital finance (Df) on carbon emission intensity (Cg). Meanwhile, the validation explores the mediating role of technological innovation (Rd), industrial restructuring (Is), and entrepreneurial effects (Es) in the process of digital finance influencing green low-carbon development. The empirical results show that: first, digital finance (Df) has a promoting effect on regional CO2 reduction capacity (Cg), and this conclusion still holds under multiple robustness tests; second, digital finance (Df) can promote the regional CO2 reduction capacity (Cg) through two paths, namely, promoting technological progress (Rd) and optimizing industrial structure (Is); third, the impact of inclusive digital finance on CO2 emission intensity is heterogeneous. By analyzing regions with different economic development levels, we found that digital inclusive finance in the eastern region can enhance CO2 reduction capacity, while in the central and western regions, the impact is not significant. Given this situation, China, to achieve carbon neutrality, should boost financial development’s ability to reduce carbon emission, promote technological progress, and optimize the industrial structure, thus forming a green and low-carbon economic cycle. This paper fills the research gap on how digital finance can effectively promote green development while exerting economic effects, and at the same time, enriches the literature on factors influencing green and low-carbon development.
1 Introduction
1.1 Research background
Since the reform and opening up, many great achievements have been made in China’s economic development, but China’s economy has been running in a crude growth model. Over time, rapid economic growth has come at the expense of the environment and consumed enormous amounts of energy. China’s economy has suffered from huge environmental costs, and one of the prominent problems is the sharp increase in CO2 emissions. Therefore, energy conservation and emission reduction have become strategic needs for China’s sustainable economic development in the new era. In late 2014, China made its first commitment to peak CO2 emissions around 2030 and to work toward an early peak in the U.S.-China Joint Announcement on Climate Change (House, 2014; Ji et al., 2014). The targets serve not only as a challenge but also as an important opportunity and lever for the green transformation of China’s economy.
Green development, however, can hardly be achieved by end-of-pipe governance alone. Forming a long-term mechanism for the governance of environmental pollution through effective financial instruments remains critical. The relationship between finance and the environment has been studied by the academic community for a long time. For example, some studies pointed out that financial development reduces CO2 emissions by promoting technological innovation and reducing energy consumption per unit of GDP (Li Y. et al., 2021; Gao et al., 2022); however, other voices hold quite the opposite opinion as they found that financial development empowers firms to produce on a larger scale, ultimately increasing CO2 emissions (Zaidi et al., 2019; Acheampong et al., 2020); other studies also showed that financial development leads to more spending on home appliances, which also increase CO2 emissions (Yue et al., 2019).
The booming information technology revolution has seen the integration of traditional finance with information technology. As one of the representations of new finance, digital finance is the key driving force for high-quality economic development. Compared with traditional finance, digital finance has further broken free from the boundaries of time and space, and has wider service coverage and more frequent usage, reducing the related transaction and operation costs. Therefore, it is of great theoretical value and practical significance to further investigate the carbon emission reduction effect of digital finance and explore its inherent mechanism.
1.2 Literature review and marginal contribution
Current scholars have more abundant research on the factors affecting CO2 emissions and environmental pollution, which is mainly reflected in the following characteristics: wide coverage of content, refinement and variation of topics, and many disciplines involved (Isik et al., 2021a; Rehman et al., 2021; Jiang et al., 2022). Meanwhile, the influencing factors of CO2 emissions can be divided into four aspects: population, economy, industry, and energy in total. Existing studies point out that economic development significantly affects carbon emissions (Ma and Cai, 2019; Isik et al., 2021b). Whereas there is no consensus on whether the level of external openness promotes or reduces carbon emissions, fiscal revenue is thought to promote carbon emissions. In terms of population, urbanization is considered to lead to an increase in carbon emissions (Zhang et al., 2017). Energy structure and industrial structure, on the other hand, are very important influences on carbon emissions (Lenzen, 2016; Dong et al., 2020). In particular, with the increasing importance of innovation, the level of technological innovation is also included in the study and is considered to suppress carbon emission intensity (Erdogan, 2021).
Also on the economic side, earlier studies have explored more the impact of traditional financial development on the environment, arguing that financial development can affect environmental quality through technological progress effects, scale effects, capital allocation effects, and structural effects. The main views are the followings: 1) Inhibition theory. Traditional finance inhibits carbon dioxide emissions (Bouyghrissi et al., 2022). Traditional finance reduces CO2 emissions through channels such as changing production factor inputs and economic structure (Murshed et al., 2022), financing environmental protection projects at lower capital costs, increasing investments related to environmental protection, and promoting the development of carbon trading activities and technological innovation. 2) Facilitation theory. Conventional finance is an important factor in increasing CO2 emissions. It promotes economic growth and energy consumption through channels such as increased consumer purchases of bulky goods, corporate investments in new equipment and projects (Sheraz et al., 2021), and financial support to natural resource-intensive polluting industries and polluting industries (Isik et al., 2022), thereby rising CO2 emissions (Qin et al., 2021; Szymczyk et al., 2021). 3) Dynamic relations. Studies have shown an inverted U-shaped relationship between conventional finance and CO2 emissions (Ali et al., 2021; Al-Silefanee et al., 2022). The relationship depends on the trade-off between the two effects, as traditional finance promotes CO2 emissions by increasing the economy volume, but it also reduces CO2 emissions by improving technology (Li J. et al., 2020; Wang et al., 2022).
With the advent of the digital economy, digital finance, which combines digital technology with traditional finance, has gradually become the focus of academic circles (Cao et al., 2021; Cao et al., 2022). Digital finance refers to a new business model that combines the internet and new-generation information technology such as big data, cloud computing, blockchain and artificial intelligence with traditional finance (Risman et al., 2021; Daud et al., 2022). Its marginal innovations to financial theory include digital footprint that provides new information for credit scoring (Agarwal and Zhang, 2020; Benami and Carter, 2021), big data that serve as a new collateral security tool, blockchain that can improve the efficiency of financial contracts, and new ways of investment, financing, and risk management (Rozario and Thomas, 2019; Tian et al., 2020).
Based on the above studies, there are also scholars who believe that digital finance also has an important impact on CO2 emissions or environmental pollution (Ozturk and Ullah, 2022; Wu and Huang, 2022; Yang et al., 2022). On the one hand, it can lower the access barrier to financial services (Ji et al., 2021), provide financial support for commodity production and consumption (Liu G. et al., 2021), and promote economic growth and inclusive growth in China (Liu L. et al., 2021), thus increasing energy consumption and CO2 emissions. On the other hand, digital finance can promote technological innovation (Hasan et al., 2022) and industrial structure transformation and upgrading (Breidbach et al., 2020; Farouq et al., 2021), thus reducing the dependence of economic growth on fossil energy and ultimately reducing CO2 emissions (Faisal et al., 2020; Li Z. et al., 2021). Therefore, digital finance development has both positive and negative effects on CO2 emissions, and whether the overall effect is positive or negative becomes an important issue worth studying.
In summary, the environmental impacts of digital finance has not received sufficient attention compared to economic performance. Studies have focused on the impact of digital finance on mitigating industrial pollution (Puschmann et al., 2020; Borowski, 2021), but there is a lack of research on how digital finance can effectively promote green development while playing an economic role in the context of “double carbon target,” thus promoting the low-carbon development of the whole society. Given these issues, this paper explores the impact, transmission paths, and spatio-temporal heterogeneity of digital finance on green low-carbon development using a dynamic spatial Durbin model combined with a mediating effect model constructed of data from 30 provinces from 2011 to 2019. The internal logic between digital finance and green low-carbon development is analyzed; the mediating role of technological innovation, industrial restructuring, and entrepreneurial effect in the process of digital finance influencing green low-carbon development is explored. The findings will enrich the literature on the influencing factors of green low-carbon development.
The marginal contributions of this paper are: first, the relationship between digital finance and carbon emission reduction capacity is explored by using the China Digital Inclusive Finance Index combined with relevant provincial panel data to verify the promotion effect of digital finance on regional carbon emission reduction capacity. Second, it focuses on the question of through what mechanism digital finance affects carbon emission reduction capacity, and verifies that digital finance can promote the improvement of regional carbon emission reduction capacity through technological innovation and industrial structure transformation. Third, the spatial effect of digital finance on regional carbon emission reduction capacity is further analyzed, with a view to enriching the literature on carbon reduction from digital finance; finally, the policy basis for China’s low-carbon economic development is provided from the perspective of digital finance development.
The remainder of this paper is structured as follows: the second part is the theoretical analysis and research hypothesis; the third part is the description of the empirical model setting and indicator selection; the fourth part is the empirical analysis of the impact effect and transmission mechanism of digital financial development acting on CO2 emissions; the fifth part is the conclusion and implication.
2 Theoretical analysis and research hypothesis
2.1 The impact of digital finance on carbon emission
In recent years, the focus of environmental pollution literature has shifted to the analysis of the determinants of CO2 emissions. The relatively more important studies adopted time series analysis methods with a particular country as the subject, concluding that economic growth, urbanization, trade openness, and financial development are all important factors influencing CO2 emissions (Tamazian et al., 2009; Shahbaz et al., 2013a; Zhao et al., 2021). Tamazian et al. (2009) examined the effect of financial development on CO2 emissions using data from Brazil, Russia, India, and China from 1992 to 2004, and found that financial development led to a decrease in per capita CO2 emissions, with the development of capital markets and expanding impact of the banking sector. Shahbaz et al. (2013b) collected data from 1971 to 2011 in Malaysia and found that there is a long-run equilibrium relationship between financial development and CO2 emissions through cointegration test, and financial development is conducive to reducing CO2 emissions. Jalil and Feridun (2011) used data from 1953 to 2006 in China and examined by ARDL method the development and carbon dioxide emissions in the long-run equilibrium relationship, and their study showed that financial development is conducive to emissions reduction.
However, digital finance started with its widespread application in the payment field and it extended rapidly to other fields beyond the financial industry. Chinese scholars have also studied the development of digital finance and the low carbon economy. First, the development of digital finance promotes the availability of low-carbon funds. On the one hand, more advanced digital finance facilitates conceptual change in banks, which would strengthen green credit organization management and green credit capacity building. On the other hand, the growth of the capital market and diversified direct financing tools, such as stocks and bonds, can broaden the financing channels for green projects and boost the green and low-carbon economic transformation. Second, consumers would exhibit a greater preference for low-carbon goods with the development of digital finance, with which, green consumer credit products would be on the rise, strengthening consumers’ awareness of environmental protection and increasing the demand for green products, ultimately reducing CO2 emissions for society as a whole. The resulting virtuous circle of digital finance has injected new vitality into low-carbon living and generated significant green economic benefits.
The first hypothesis is put forward here on this basis:
Hypothesis 1. The development of digital finance is conducive to reducing carbon emissions.If digital finance can drive regional emissions reductions, what are the specific pathways through which this driving effect can be generated?
2.2 The mechanism of the effect of digital finance on carbon emission
2.2.1 Technological innovation pathway
Wang and Zhu, (2020) found that one of the essential factors curbing carbon emissions is technological advancement, and the variation in their degrees can result in differences in energy use efficiency. Yang W et al. (2019) attributed the difference in regional carbon emissions in China to varying degrees of technological development and the rationality of regional economic structure. It was also found that R&D input has the greatest impact on carbon emission efficiency among all factors (Jiao et al., 2018; Alam et al., 2019; Churchill et al., 2019), while technology spillover can play a negative role in carbon emission efficiency (Shahzad et al., 2017; Cheng et al., 2018; Zhang and Zhang 2018). They also found that endogenous innovation efforts are the key to improving regional carbon emission efficiency, and therefore China needs to act in this regard (Lin and Zhu, 2019).
At the same time, the development of science and technology innovation activities cannot be carried out without the support of the financial system (Maskus et al., 2012), but the long-term undersupply of traditional financial services has been a stumbling block (Stiglitz and Weiss, 1981). To overcome the restriction imposed by this disadvantage, big data technology and other support are needed, which will help to rectify the problems arising from “attribute mismatch” due to insufficient supply of traditional financial services. Digital finance can support the technological innovation development of science and innovation enterprises in the following ways: first, providing more diverse financing channels and methods such as supply chain finance and consumer finance (Ahmad et al., 2021a); second, ameliorating the external financing environment for enterprises, thus promoting efficient R&D investment, raising industrial total factor productivity at the enterprise level under the synergy of spatial knowledge spillover (Bakhtiari et al., 2020), enhancing energy utilization (He and Lin, 2019; Li et al., 2022), and empowering sustainable regional economic development intrinsically (Hang et al., 2019; Razzaq et al., 2021).
In view of this, this paper proposes Hypothesis 2:
Hypothesis 2. The development of digital finance may have a positive impact on carbon emissions through transmission channels such as technological innovation.
2.2.2 Industrial restructuring pathway
The evolution of industrial structure can reflect changes in the economic growth and development pattern of a country or region, shaping the ecological environment of the country (Ahmad et al., 2021b). The level of regional carbon emissions is intrinsically related to its industrial structure (Zhang et al., 2014; Dong et al., 2020; Zhang et al., 2020). Most existing studies have shown that the share of the secondary sector has a significant and positive effect on carbon emissions and energy consumption at all regional scales, and some empirical analyses have found that the expansion of almost all industries exacerbates carbon emissions, but the intensity of this effect in the tertiary sector is significantly lower than that in the secondary sector (Wang et al., 2019; Wang and Wang, 2021). At this stage, the domestic and international macro-environment facing China’s economic and social development has undergone profound changes, followed by a new round of accelerating trends of domestic industrial transfer. The regional transfer of labor-intensive industries and resource-based processing industries provides new room for strategically emerging industries and modern service industries (Rauf et al., 2018), and the emission reduction effect exerted by the digital financial market will also usher in a new height. Exploring the issue of carbon emission intensity from the perspective of industrial structure is conducive to correctly judging and grasping the industrial factors affecting it, offering insights in guiding industrial development policies to control carbon emissions (Yang et al., 2018).
The industrial upgrading effect of digital finance is reflected in both supply and demand sides: 1) On the supply side: digital finance reduces the external financing cost of enterprises by optimizing the information asymmetry problem within and across industries (Yang and Zhang, 2020). In addition, the user information and consumption-side data obtained by digital finance and mobile payment platforms are used to better analyze user preferences and market demand, identify market gaps timely, and promote the transformation and upgrading of industrial structure. Finally, it enables enterprises to invest more resources in product development and reduce ineffective supply and waste of link resources (Haberly et al., 2019). 2) On the demand side: digital finance, as a new driver to stimulate consumption and boost domestic demand, is conducive to the expansion of China’s consumption scale and the upgrading of consumption patterns. On the one hand, the consumer credit function of digital finance can alleviate the liquidity constraint on residents’ consumption and improve the convenience of converting savings or investment returns into consumption (Lai et al., 2020). On the other hand, digital finance also reduces the difficulty in accessing financial services, especially in rural areas with poor traditional financial services that have led to chronic underconsumption; it enhances the inclusive effect of financial services and fully releases the consumption potential (Li Z. et al., 2020). Ultimately, the expansion and upgrading of consumer demand can force industrial upgrading and accelerate the processes of energy saving and emission reduction in enterprises and markets.
In view of this, this paper proposes Hypothesis 3:
Hypothesis 3. The development of digital finance may affect carbon emissions through transmission channels such as industrial restructuring.
2.2.3 Entrepreneurial effect pathway
As a long-term economic growth “engine”, entrepreneurship is an important vehicle for implementing the innovation-driven development strategy, which can increase social employment rates, encourage engagement in more economically efficient and energy-efficient work, alleviate factor mismatch, and reduce pollution (Boasson and Wettestad, 2014; Rogalev et al., 2018; Liang et al., 2019; Sun et al., 2020). Finance is an important component of the entrepreneurial environment (Luc, 2018), and the extent to which a country’s financial system supports entrepreneurial ventures influences the country’s entrepreneurial activity (Del Giudice et al., 2019; Estrin et al., 2019). It has been widely argued among the academic society that financial development can ease the liquidity constraints on potential entrepreneurs by allocating resources rationally and efficiently, thus promoting entrepreneurial activity (Zaidi et al., 2019; Khan et al., 2020).
The effect on entrepreneurship exerted by digital finance is mainly manifested as follows: 1) Digital finance has shifted financial activities from offline to online, completing the “last mile” of financial services by offering services such as remittances and lending whenever the internet is available, making up for the deficiencies in many places particularly rural areas, thus promoting regional entrepreneurial activities (Philip and Williams, 2019; Jafari-Sadeghi et al., 2021). 2) The cost of risk assessment for micro and small enterprises has been lowered by big data-based digital finance, and a more complete credit rating system can be established by cloud computing technology, lowering the financing threshold for micro and small firms and supporting their development, ultimately lightening the enthusiasm of public entrepreneurship (Hommel and Bican, 2020). 3) Digital finance, as financial infrastructure, provides the foundation for innovation and space for entrepreneurship. Digital finance further facilitates the internet-oriented transformation of offline businesses, such as bike-sharing, online car-hailing, and take-away food delivery, releasing a large number of new business opportunities and providing greater entrepreneurial space. The effect on entrepreneurship exerted by digital finance, while alleviating financial resource mismatch and providing more career options, indirectly raises corporate labor costs, thus forcing companies to innovate technologically, adopt cleaner and more efficient production methods, and reduce environmental pollution (Parrish and Foxon, 2006; Omri and Afi, 2020).
In view of this, this paper proposes Hypothesis 4:
Hypothesis 4. The development of digital finance may affect carbon emissions through transmission channels such as the entrepreneurial effect.
3 Research design
3.1 Model setting
3.1.1 Spatial econometric model
3.1.1.1 Spatial econometric regression model selectivity test
According to the needs of the actual research problem, we draw on the research method of Elhorst (2014) to test the applicability of the model, and the test shows that the spatial Durbin model with fixed effects is more appropriate for this paper.
3.1.1.2 Setting of spatial durbin model
Considering the possible time-dependent path-dependent characteristics of carbon emission changes, i.e., time-lagged effects, the possible bi-directional causality between carbon emissions and economic growth, technological progress, and other factors leading to endogeneity problems (Shao et al., 2011), this paper introduces the one-period lag of carbon emission variables into the standard static spatial panel Durbin model and constructs the following dynamic spatial panel Durbin model.
where Cgit is the explanatory variable (regional carbon emission intensity); Dfit is the key explanatory variable (digital finance index); Rd (technological innovation), Is (industrial structure), and Es (mass entrepreneurship) are the mediating variables,
3.1.1.3 Selection of the spatial weight matrix
Based on the consideration of the fit and operability of the research problem, this paper will refer to Shao et al. (2011) and use the geographic distance matrix to describe the spatial panel model, which is calculated as follows:
3.1.2 Intermediary effect model
To test whether these three factors act as mediating variables, this paper uses a canonical mediating effects model and conducts further empirical investigation based on a spatial econometric model. A more widely adopted method to test for mediating effects is the stepwise method proposed by Baron and Kenny (1986). The specific mediating effect model is set as follows.
According to hypotheses H2∼H4, this paper considers carbon emission intensity (Cgit) as the explanatory variable, technological innovation, (Rdit) industrial structure (Isit), and mass entrepreneurship (Esit) as the mediating variables to be tested M, and digital finance (Dfit) as the explanatory variable. The time-lagged and spatial-lagged terms of carbon emission intensity, technological innovation (Rdit), industrial structure (Isit), mass entrepreneurship (Esit), digital finance (Dfit), and other control variables are used as control variables in the mediating variable model.
3.2 Variable specification
3.2.1 Explained variable: Carbon emission intensity
Carbon emissions intensity (Cg): carbon emissions based on energy consumption are used to characterize the carbon emissions, and its measurement method is borrowed from the carbon emissions formula for energy consumption in IPCC, and the formula for carbon emissions is shown below:
Where, Cgijt denotes the carbon emission of
3.2.2 Core explanatory variable: Digital finance development
Digital Finance Development Index (Df): this paper uses the 2011–2019 Peking University Digital Inclusive Finance Index (Phase I and Phase II) at the provincial level compiled by the Digital Finance Research Center of Peking University to represent the level of digital finance development in each region.
3.2.3 Mediating variable
In this paper, the technological innovation (Rd) is measured using the logarithm of the number of patented technology applications by region. The value added of the tertiary sector, as a share of GDP, is used to represent the industrial upgrading effect (Is). The mass entrepreneurship (Es) is measured using the ratio of the number of private and individual employees to the number of employees in urban areas.
3.2.4 Control variable
With reference to existing research on factors influencing carbon emissions, the following indicators are selected as control variables to be included in the model in this paper: 1) Level of economic development (lnGdp): measured by the natural logarithm of regional gross domestic product per capita. 2) Level of opening up to the outside world (Open): measured by the share of foreign direct investment (FDI) in GDP. The U.S. dollar is converted according to the mid-price of the annual RMB exchange rate in the processing (Zhang and Zhang, 2018). 3) Urbanization level (City): measured by the share of the urban population in the total population (Liddle, 2014). 4) Informatization level (lnIntel): The logarithm of the number of regional Internet domain names is used to measure this index. 5) Human capital level (lnH_c): measured by the number of students enrolled in higher education per 10,000 people (Marvel and Lumpkin, 2007). 6) Government intervention (lnGov): measured by the logarithm of regional government spending on energy conservation and environmental protection. 7) Energy consumption (lnE_c): Measured by per capita coal consumption, which directly determines the size of carbon emission intensity of energy consumption (i.e., carbon emission per unit of energy consumption) (Akhmat et al., 2014). Table 1 describes the main variables in this article.
3.3 Data source
Given the availability of digital financial inclusion data, this paper selects panel data collected from 30 provinces in China from 2011 to 2019. Among them, the data on carbon emission intensity are obtained from China Energy Statistical Yearbook, the data on proxy variables of digital financial development index are obtained from Peking University Digital Financial Inclusion Index (2011–2019) by Peking University Digital Finance Research Center, and the data on intermediary variables as well as control variables are obtained from China Statistical Yearbook. To eliminate the effect of price fluctuations, economic variables were deflated using 2011 as the base period. To eliminate the effect of heteroskedasticity, the variables with non-ratio values are treated as logarithmic.
4 Results and discussion
4.1 Discussion of the spatial autocorrelation test
Before the empirical analysis, Moran’s I index is first used to measure the spatial correlation between the level of digital finance as well as the intensity of carbon emissions. Its calculation formula is as follows.
where,
Table 2 shows the Moran’s I indices of digital finance level and carbon emission intensity for 30 provinces in China from 2011 to 2019. As can be seen from the Table 2, the Moran’s I indices of regional digital finance levels are all around 0.4 and all pass the 1% significance level test. The Moran’s I indices of regional carbon emission intensity are all located around 0.2, and all of them also pass the 1% significance level test. This result indicates that there is a significant positive spatial correlation of carbon emissions in 30 provinces in China from 2011 to 2019, so the carbon emission intensity based on spatiality analysis is reasonable, and also lays a solid foundation for the following empirical analysis.
4.2 Discussion of the benchmark regression results
As shown in Table 3, the estimated coefficients of digital finance are not significant in columns (1) and 2) without considering endogeneity and spatial correlation issues, which means that not considering both endogeneity and spatial correlation may lead to biased estimation results. The estimation results of columns (3) and (4), which consider both endogeneity and spatial correlation, exhibited better statistical characteristics. Further, compared with column (3), the coefficient of the time-lagged term of carbon emission intensity in column (4) is significantly positive at the 1% level, verifying that the change in carbon emission intensity has a time-dependent path characteristic, as described in the previous section. Undoubtedly, compared with the rest of the models, column (4) has the most excellent theoretical expectations and econometric technical performance as it considers both the endogeneity problem, spatial correlation, and the results of time lagged effects of the explanatory variables. Therefore, we focus on the estimation results of column (4) in the following discussion.
The results obtained from column (4) show that carbon emission intensity has both a significant time lag effect and a spatial spillover effect. On the one hand, carbon emission intensity has a strong path-dependent characteristic in the time dimension, which is also called the “snowball” effect. High emission intensity in the previous period may indicate high emission in the next one. On the other hand, the coefficient of the spatial lag of carbon emission intensity is significantly positive at the 1% level, indicating that there is an obvious strategic competition effect among provinces and regions in terms of carbon emission intensity.
Meanwhile, the coefficient corresponding to the key variable of this paper, i.e., digital finance index, is significantly negative (the higher the level of digital finance development, the lower the carbon emission intensity), indicating that digital finance development helps reduce carbon emission intensity. 1) Firstly, the convenience of mobile payment brought by digital finance has led to the reduction of cash use, and various online cell phone APPs provide financial services to meet users’ needs, reducing the frequency of visits to bank counters. The increase in financial efficiency significantly reduces the original transaction costs and possible resource consumption (Bachas et al., 2018). 2) Secondly, the development of digital finance expands the channels for public participation in environmental protection, promotes resource recycling, and improves the utilization rate to avoid the waste of resources caused by repeated production (Ting et al., 2019); meanwhile, it closely integrates the public’s daily life with the environmental protection cause, thus establishing the relevant awareness in public. 3) Finally, compared with the traditional financial system that controls carbon emissions by technological innovation driven by bank lending, digital finance builds a carbon financial market and uses inter-regional carbon emissions trading to achieve this goal (Shahbaz et al., 2013a). The development indicators of digital finance show a negative impact on the intensity of CO2 emissions in this research, enriching existing literature. However, the estimated coefficient of its spatial lag term “W∗Df” is not significant under the adjacency matrix. Although it indicates that the development of digital finance in this province has a significant contribution to carbon emission reduction in this province, it has no significant spatial spillover effect on carbon emission reduction in neighboring provinces.
In terms of control variables: 1) The coefficient corresponding to the level of external openness is negative and passes the significance test at a level of 5%, which indicates that the higher the level of openness, the lower the CO2 intensity. The possible reason for this is that the level of openness has a dampening effect on CO2 emissions through the introduction of new technologies. 2) Urbanization corresponds to a negative coefficient and passes the significance test at the 10% level, which means that accelerated urbanization may contribute to lower CO2 emissions in a society. This may be due to the fact that urbanization accelerates the modernization process, and that the economies of scale effects of urbanization and the division of labor have led to an increase in the factor agglomeration effect, which upgrades technology and production organization patterns, reducing the CO2 intensity. 3) The coefficient corresponding to government intervention is negative and passes the significance test at the 10% level. The improvement of carbon emission performance is closely related to governments’ role in setting the price of energies, natural resources, and other factors. 4) The coefficient of energy consumption is positive and significant at the 1% level, which again verifies that the current energy consumption structure with an abnormally high proportion of coal in China is an important factor hindering the reduction of carbon emission intensity.
4.3 Discussion of the heterogeneity results
The above section empirically examines the overall effect of digital finance on regional carbon emission intensity. The next section analyze China by dividing it into three regions, i.e., East, Central and West, based on the level of economic development, and then explore the impact of digital finance development on carbon emissions in different regions. The results of column (1) in Table 4 show that the regression coefficient of digital finance in the east is significantly negative at 1%, indicating that digital finance can significantly reduce carbon emissions in the eastern region. However, the regression coefficients of digital finance are not significant in both columns (2) and (3), indicating that although the development of digital finance in China as a whole has contributed to CO2 reduction in certain regions, the degree varies across regions. That is, the positive impact of digital finance on regional carbon emission reduction is stronger in the eastern region, and the reason for this variability may be its earlier development of digital finance brought relatively higher level of development and more comprehensive implementation of the innovation-driven development strategy. Therefore, it is more conducive to eliminating the mismatch of production factors and pollution emission reduction, thus allowing the dividends played by digital finance to be fully released.
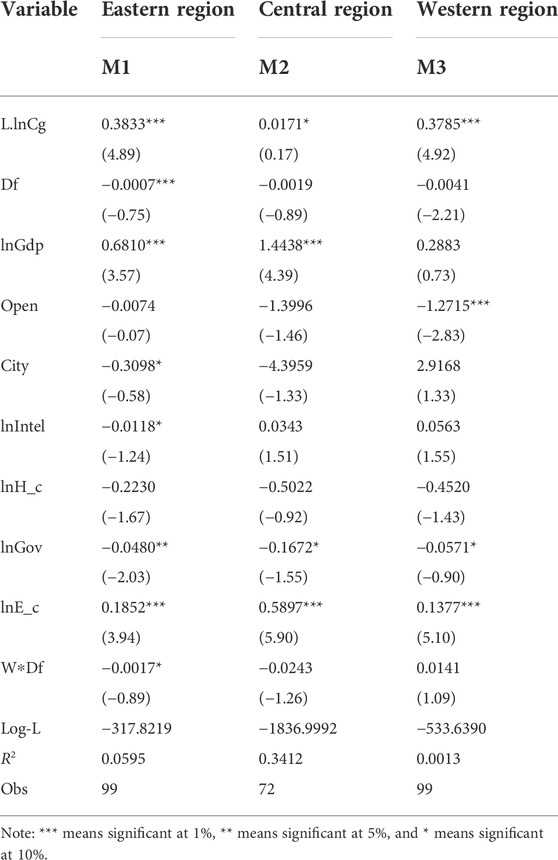
TABLE 4. Estimation results of the effect of digital finance on carbon emissions in regions with different development situations.
4.4 Discussion of the robustness results
First, the explanatory variables are replaced. In this step, the logarithm of regional per capita carbon emissions (lnCg_p) is used to remeasure the regional carbon emission intensity, with static spatial Durbin as well as dynamic spatial Durbin regressions being conducted on it. The results are shown in the first and second columns of Table 5, according to which, the data of each variable changed slightly, but most of the positive and negative signs and significance did not change much, verifying the robustness of our results. Second, the sample time years are adjusted. The original sample time range of 2011–2019 was shortened to 2014–2019, for which static spatial Durbin and dynamic spatial Durbin regressions are conducted. The results are shown in the third and fourth columns of Table 5, and like columns 1 and 2, the data changed slightly, but the signs and significance did not change much, also proving the robustness of the results of this paper.
4.5 Discussion of the intermediary effect results
The above empirical results show that digital finance helps suppress regional CO2 emissions, but this work focuses on how this impact works. Therefore, we gave column (1) in Table 6, which is the baseline model without any mediating variables, and columns (2)∼(7), which are the six spatial dynamic Durbin models constructed by testing the mediating variables. Then the mediating effects played by technological innovation, industrial structure optimization, and mass entrepreneurship are explored.
4.5.1 The mediating variable technological innovation
1) It is clear from baseline column (1) that local digital financial development has a negative and significant effect on regional carbon emission intensity. 2) Column (2) shows a significant positive effect of local digital financial development on technological innovation, indicating that the improvement of digital financial level helps promote technological progress. 3) After adding technological innovation into column (1), column (3) is obtained, and it can be found that the absolute value of the regression coefficient of digital finance in this region decreases from 0.0059 in column (1) to 0.0026 in column (3), and the coefficient remains significant. Then it is known from the criterion of mediating effect that technological innovation plays a partial mediating effect in the promotion of digital finance on regional carbon emission intensity, which is consistent with hypothesis 2. The increase in technological innovation and R&D expenditure in China has a suppressive effect on CO2 emissions. A higher level of financial development can contribute to corporate R&D financing, with which, CO2 emissions can be reduced by boosted innovation (Quan et al., 2020).
In summary, digital financial development indirectly curbs carbon dioxide emissions by promoting technological progress. The specific working mechanism is as follows: First, technological progress improves the energy consumption structure by developing new energy sources and finding alternative clean sources to replace traditional fossil energy sources. Second, technological advances help to improve energy efficiency by consuming less energy for the same level of output. In addition, digital finance provides a larger room for technological innovation. The business ecosystem in the digital economy era created by Alipay, WeChat, and other payment platforms has penetrated into every aspect of life, giving rise to a variety of new business models such as shared travel and crowdfunding, further controlling the intensity of carbon emissions.
4.5.2 The mediating variable industrial structure optimization
1) The benchmark column (1) clearly shows that local digital finance development has a negative and significant effect on regional carbon emission intensity; 2) Column (4) shows that there is a significant positive effect of local digital finance development on industrial structure, i.e., the improvement of digital finance level helps to promote the development of the tertiary industry; and 3) Column (5)adds industrial structure optimization to column (1), and it can be found that the absolute value of the regression coefficient of digital finance in the region decreases from 0.0059 in column (1) to 0.0039 in column (5), and the coefficient remains significant. Then it is clear from the criterion of mediating effect that the industrial structure plays a partially mediating effect in the promotion of regional carbon emission intensity by digital finance, indicating that the increase in the proportion of GDP added value in the service sector reduces the CO2 intensity. The possible reason is that the process of service sector development implies that technological progress and factor reallocation elevates production efficiency, which is consistent with hypothesis 3.
In short, more advanced digital finance will promote industrial restructuring, thus suppressing CO2 emissions. With the continuous improvement of the financial system, financial services can effectively alleviate or even address information asymmetry, and lower transaction costs, ultimately stimulating capital accumulation and promoting the optimization of industrial structure and economic development (Chava et al., 2013). Second, there is a long-term equilibrium relationship between financial operation efficiency and industrial structure upgrading; by lowering the access threshold of financial services and promoting the free development of the capital market, we can effectively promote the transformation of industrial structure, improve the efficiency of economic operation and growth vitality (Kahia et al., 2016), and finally achieve pollution emission reduction.
4.5.3 The mediating variable mass entrepreneurship
1) The baseline column (1) shows that local digital financial development has a negative and significant effect on regional carbon emission intensity. 2) Column (6) indicates a positive effect of local digital financial development on mass entrepreneurship, but the impact is not significant. 3) Column (7) adds mass entrepreneurship based on column (1), and according to it, the coefficient corresponding to mass entrepreneurship is negative, but still not significant. From the criterion of mediating effect, it can be seen that mass entrepreneurship has no mediating effect on the promotion of regional carbon emission intensity by digital finance; therefore, it has no positive effect on carbon emission intensity. The “green bias” held by informal workers will be beneficial to energy saving and emission reduction; however, if the production behavior is oriented to increase productivity, the impact will be detrimental as it leads to the expansion of the production scale.
5 Conclusions and policy implications
This paper first theoretically analyzes the mechanism of the role of digital inclusive finance in influencing carbon emission intensity; secondly, it uses panel data of 30 provinces across China from 2011 to 2019 to test the relationship between digital finance and green low-carbon development by constructing a spatial econometric model. The empirical analysis showed that the impact of digital finance on green low-carbon development is mediated by factors such as technological innovation and industrial restructuring. The conclusions are: first, digital finance has a promoting effect on regional CO2 reduction capacity, and this conclusion still holds under multiple robustness tests. Second, digital finance can promote regional CO2 reduction capacity through two paths: promoting technological progress and optimizing industrial structure. Third, the impact of digital inclusive finance on CO2 emission intensity is heterogeneous. Seeing from regional economic development levels, the development of digital inclusive finance in the eastern region can enhance CO2 reduction capacity. In contrast, in the central and western regions, the impact of digital inclusive finance on carbon emission reduction is not significant.
Based on the above empirical findings, this paper puts forward the following three suggestions to promote green and low-carbon development: First, strengthen the exchange and cooperation of carbon emission reduction among regions. Efforts as such are interlinked among regions, so it is necessary to establish a sound exchange and cooperation mechanism to strengthen project exchange and cooperation, and to accelerate the cooperation in environmental governance and industrial docking, so as to enhance the systemic and linkage of energy conservation and emission reduction. Second, continue to develop digital finance. First, improve the regulatory system of the digital finance industry. Regulators should set entry thresholds for financial enterprises, while establishing a reliable risk disclosure mechanism to make the operation of financial enterprises transparent. Secondly, relevant departments should pay attention to the innovative and entrepreneurial incentive functions of digital finance and work on its promotion, so as to enhance the entrepreneurship level and boost enterprise research and innovation activities. Finally, the advantages of traditional finance in supporting infrastructure should be given full play to achieve complementary advantages and improve the efficiency of financial services (Gossling et al., 2013). Third, financial policies should be tailored to local conditions. For the eastern region, relevant enterprises should innovate in technologies, products and services in digital finance to meet the diversified needs of consumers, which will drive force the development of digital finance. For the central and western regions, relevant departments should improve the financial infrastructure, promote financial knowledge in laggard regions, and improve their acceptance and recognition of digital finance.
It should also be noted that this paper’s theoretical model and empirical study have certain shortcomings. First, some of the settings in the theoretical model are overpowered to reflect the real economy and to comply with the technical conditions of the endogenous growth model. Therefore, future scholars will try to relax the assumptions to verify the universality of the conclusions. Second, due to the limitation of data availability, the research interval of this paper only covers 9 years, so the time series of data can be added in future research to enhance the effectiveness of the results. Third, the macro-level data at the provincial level do not reflect the micro-decision making behavior, and a more micro-level data analysis, such as industry or firm data, may be better.
Data availability statement
The original contributions presented in the study are included in the article/supplementary material, further inquiries can be directed to the corresponding author.
Author contributions
Conceptualization: XD; data collection and analysis: XD; methodology: XD, LG, and YN; formal analysis and investigation: XD, LG, YN and GW; writing of the original draft: XD and LG; writing, review, and editing of the final paper: XD, GW, LG, YN; funding acquisition: GW; supervision: XD and GW. All authors have read and agreed to the published version of the manuscript.
Conflict of interest
The authors declare that the research was conducted in the absence of any commercial or financial relationships that could be construed as a potential conflict of interest.
Publisher’s note
All claims expressed in this article are solely those of the authors and do not necessarily represent those of their affiliated organizations, or those of the publisher, the editors and the reviewers. Any product that may be evaluated in this article, or claim that may be made by its manufacturer, is not guaranteed or endorsed by the publisher.
References
Acheampong, A. O., Amponsah, M., and Boateng, E. (2020). Does financial development mitigate carbon emissions? Evidence from heterogeneous financial economies. Energy Econ. 88, 104768. doi:10.1016/j.eneco.2020.104768
Agarwal, S., and Zhang, J. (2020). FinTech, lending and payment innovation: A review. Asia. Pac. J. Financ. Stud. 49 (3), 353–367. doi:10.1111/ajfs.12294
Ahmad, M., Khan, Z., Rahman, Z. U., Khattak, S. I., and Khan, Z. U. (2021b). Can innovation shocks determine CO2 emissions (CO2e) in the oecd economies? A new perspective. Econ. Innovation New Technol. 30 (1), 89–109. doi:10.1080/10438599.2019.1684643
Ahmad, M., Majeed, A., Khan, M. A., Sohaib, M., and Shehzad, K. (2021a). Digital financial inclusion and economic growth: Provincial data analysis of China. China Econ. J. 14 (3), 291–310. doi:10.1080/17538963.2021.1882064
Akhmat, G., Zaman, K., Shukui, T., Irfan, D., and Khan, M. M. (2014). Does energy consumption contribute to environmental pollutants? Evidence from SAARC countries. Environ. Sci. Pollut. Res. 21 (9), 5940–5951. doi:10.1007/s11356-014-2528-1
Al-Silefanee, R. R., Mamkhezri, J., Khezri, M., Karimi, M. S., and Khan, Y. A. (2022). Effect of islamic financial development on carbon emissions: A spatial econometric analysis. Front. Environ. Sci. 272. doi:10.3389/fenvs.2022.850273
Alam, M. S., Atif, M., Chien-Chi, C., and Soytaş, U. (2019). Does corporate R&D investment affect firm environmental performance? Evidence from G-6 countries. Energy Econ. 78, 401–411. doi:10.1016/j.eneco.2018.11.031
Ali, K., Bakhsh, S., Ullah, S., Ullah, A., and Ullah, S. (2021). Industrial growth and CO2 emissions in vietnam: The key role of financial development and fossil fuel consumption. Environ. Sci. Pollut. Res. 28 (6), 7515–7527. doi:10.1007/s11356-020-10996-6
Bachas, P., Gertler, P., Higgins, S., and Seira, E. (2018). Digital financial services go a long way: Transaction costs and financial inclusion. AEA Pap. Proc. 108, 444–448. doi:10.1257/pandp.20181013
Bakhtiari, S., Breunig, R., Magnani, L., and Zhang, J. (2020). Financial constraints and small and medium enterprises: A review. SSRN J. 96 (315), 506–523. doi:10.2139/ssrn.3534484
Baron, R. M., and Kenny, D. A. (1986). The moderator–mediator variable distinction in social psychological research: Conceptual, strategic, and statistical considerations. J. personality Soc. Psychol. 51 (6), 1173–1182. doi:10.1037/0022-3514.51.6.1173
Benami, E., and Carter, M. R. (2021). Can digital technologies reshape rural microfinance? Implications for savings, credit, & insurance. Appl. Econ. Perspect. Policy 43 (4), 1196–1220. doi:10.1002/aepp.13151
Boasson, E. L., and Wettestad, J. (2014). Policy invention and entrepreneurship: Bankrolling the burying of carbon in the EU. Glob. Environ. Change 29, 404–412. doi:10.1016/j.gloenvcha.2014.09.010
Borowski, P. F. (2021). Digitization, digital twins, blockchain, and industry 4.0 as elements of management process in enterprises in the energy sector. Energies 14 (7), 1885. doi:10.3390/en14071885
Bouyghrissi, S., Murshed, M., Jindal, A., Berjaoui, A., Mahmood, H., and Khanniba, M. (2022). The importance of facilitating renewable energy transition for abating CO2 emissions in Morocco. Environ. Sci. Pollut. Res. 29 (14), 20752–20767. doi:10.1007/s11356-021-17179-x
Breidbach, C. F., Keating, B. W., and Lim, C. (2020). Fintech: Research directions to explore the digital transformation of financial service systems. SSRN J. 30 (1), 79–102. doi:10.2139/ssrn.3649758
Cao, J., Law, S. H., Wu, D., Tang, X., and Yang, X. (2022). Effect of digital financial inclusion on dredging the path of green growth—new evidence from front-end and back-end perspectives. Front. Environ. Sci. 485. doi:10.3389/fenvs.2022.865229
Cao, S., Nie, L., Sun, H., Sun, W., and Taghizadeh-Hesary, F. (2021). Digital finance, green technological innovation and energy-environmental performance: Evidence from China's regional economies. J. Clean. Prod. 327, 129458. doi:10.1016/j.jclepro.2021.129458
Chava, S., Oettl, A., Subramanian, A., and Subramanian, K. V. (2013). Banking deregulation and innovation. SSRN J. 109 (3), 759–774. doi:10.2139/ssrn.2174420
Cheng, Z., Li, L., Liu, J., and Zhang, H. (2018). Total-factor carbon emission efficiency of China's provincial industrial sector and its dynamic evolution. Renew. Sustain. Energy Rev. 94, 330–339. doi:10.1016/j.rser.2018.06.015
Churchill, S. A., Inekwe, J., Smyth, R., and Zhang, X. (2019). R&D intensity and carbon emissions in the G7: 1870–2014. Energy Econ. 80, 30–37. doi:10.1016/j.eneco.2018.12.020
Daud, I., Nurjannah, D., Mohyi, A., Ambarwati, T., Cahyono, Y., Haryoko, A. E., et al. (2022). The effect of digital marketing, digital finance and digital payment on finance performance of Indonesian SMEs. 6(1), 37–44. doi:10.5267/j.ijdns.2021.10.006
Del Giudice, M., Garcia-Perez, A., Scuotto, V., and Orlando, B. (2019). Are social enterprises technological innovative? A quantitative analysis on social entrepreneurs in emerging countries. Technol. Forecast. Soc. Change 148, 119704. doi:10.1016/j.techfore.2019.07.010
Dong, B., Ma, X., Zhang, Z., Zhang, H., Chen, R., Song, Y., et al. (2020). Carbon emissions, the industrial structure and economic growth: Evidence from heterogeneous industries in China. Environ. Pollut. 262, 114322. doi:10.1016/j.envpol.2020.114322
Erdogan, S. (2021). Dynamic nexus between technological innovation and building sector carbon emissions in the BRICS countries. J. Environ. Manag. 293, 112780. doi:10.1016/j.jenvman.2021.112780
Estrin, S., Mickiewicz, T., Stephan, U., and Wright, M. (2019). “Entrepreneurship in emerging markets,” in The Oxford handbook of management in emerging markets, 457.
Faisal, F., Tursoy, T., and Pervaiz, R. (2020). Does ICT lessen CO2 emissions for fast-emerging economies? An application of the heterogeneous panel estimations. Environ. Sci. Pollut. Res. 27 (10), 10778–10789. doi:10.1007/s11356-019-07582-w
Farouq, I. S., Sambo, N. U., Ahmad, A. U., Jakada, A. H., and Danmaraya, I. A. (2021). Does financial globalization uncertainty affect CO2 emissions? Empirical evidence from some selected SSA countries. Quant. Financ. Econ. 5, 247–263. doi:10.3934/qfe.2021011
Gao, P., Wang, Y., Zou, Y., Su, X., Che, X., and Yang, X. (2022). Green technology innovation and carbon emissions nexus in China: Does industrial structure upgrading matter? Front. Psychol. 13, 951172. doi:10.3389/fpsyg.2022.951172
Gössling, S., Scott, D., and Hall, C. M. (2013). Challenges of tourism in a low‐carbon economy. WIREs Clim. Change 4 (6), 525–538. doi:10.1002/wcc.243
Haberly, D., MacDonald-Korth, D., Urban, M., and Wójcik, D. (2019). Asset management as a digital platform industry: A global financial network perspective. Geoforum 106, 167–181. doi:10.1016/j.geoforum.2019.08.009
Hang, Y., Wang, Q., Zhou, D., and Zhang, L. (2019). Factors influencing the progress in decoupling economic growth from carbon dioxide emissions in China’s manufacturing industry. Resour. Conservation Recycl. 146, 77–88. doi:10.1016/j.resconrec.2019.03.034
Hasan, M. M., Yajuan, L., and Khan, S. (2022). Promoting China’s inclusive finance through digital financial services. Glob. Bus. Rev. 23 (4), 984–1006. doi:10.1177/0972150919895348
He, Y., and Lin, B. (2019). Investigating environmental kuznets curve from an energy intensity perspective: Empirical evidence from China. J. Clean. Prod. 234, 1013–1022. doi:10.1016/j.jclepro.2019.06.121
Hommel, K., and Bican, P. M. (2020). Digital entrepreneurship in finance: Fintechs and funding decision criteria. Sustainability 12 (19), 8035. doi:10.3390/su12198035
House, W. (2014). US-China joint announcement on climate change. White House Available at: http://www.whitehouse.gov/the-press-office/2014/11/11/us-china-joint-announcement-climatechange.
Işık, C., Ahmad, M., Ongan, S., Ozdemir, D., Irfan, M., and Alvarado, R. (2021a). Convergence analysis of the ecological footprint: Theory and empirical evidence from the USMCA countries. Environ. Sci. Pollut. Res. 28 (25), 32648–32659. doi:10.1007/s11356-021-12993-9
Işık, C., Ongan, S., Bulut, U., Karakaya, S., Irfan, M., Alvarado, R., et al. (2022). Reinvestigating the Environmental Kuznets Curve (EKC) hypothesis by a composite model constructed on the Armey curve hypothesis with government spending for the US States. Environ. Sci. Pollut. Res. 29 (11), 16472–16483. doi:10.1007/s11356-021-16720-2
Işık, C., Ongan, S., Ozdemir, D., Ahmad, M., Irfan, M., Alvarado, R., et al. (2021b). The increases and decreases of the environment Kuznets curve (EKC) for 8 OECD countries. Environ. Sci. Pollut. Res. 28 (22), 28535–28543. doi:10.1007/s11356-021-12637-y
Jafari-Sadeghi, V., Garcia-Perez, A., Candelo, E., and Couturier, J. (2021). Exploring the impact of digital transformation on technology entrepreneurship and technological market expansion: The role of technology readiness, exploration and exploitation. J. Bus. Res. 124, 100–111. doi:10.1016/j.jbusres.2020.11.020
Jalil, A., and Feridun, M. (2011). The impact of growth, energy and financial development on the environment in China: A cointegration analysis. Energy Econ. 33 (2), 284–291. doi:10.1016/j.eneco.2010.10.003
Ji, X., Wang, K., Xu, H., and Li, M. (2021). Has digital financial inclusion narrowed the urban-rural income gap: The role of entrepreneurship in China. Sustainability 13 (15), 8292. doi:10.3390/su13158292
Ji, Z., Xiaohua, Z., Sha, F., Yue, Q., Ji, C., and Hairan, G. (2014). Implications and challenges of the US-China joint announcement on climate change cooperation.
Jiang, G., Alvarado, R., Murshed, M., Tillaguango, B., Toledo, E., Méndez, P., et al. (2022). Effect of agricultural employment and export diversification index on environmental pollution: Building the agenda towards sustainability. Sustainability 14 (2), 677. doi:10.3390/su14020677
Jiao, J., Jiang, G., and Yang, R. (2018). Impact of R&D technology spillovers on carbon emissions between China’s regions. Struct. Change Econ. Dyn. 47, 35–45. doi:10.1016/j.strueco.2018.07.002
Kahia, M., Kadria, M., and Aïssa, M. S. B. (2016). “What impacts of renewable energy consumption on CO2 emissions and the economic and financial development? A panel data vector autoregressive (pvar) approach,” in 2016 7th International Renewable Energy Congress (IREC) (IEEE), 1–6. doi:10.1109/irec.2016.7478912
Khan, Z., Hussain, M., Shahbaz, M., Yang, S., and Jiao, Z. (2020). Natural resource abundance, technological innovation, and human capital nexus with financial development: A case study of China. Resour. Policy 65, 101585. doi:10.1016/j.resourpol.2020.101585
Lai, J. T., Yan, I. K., Yi, X., and Zhang, H. (2020). Digital financial inclusion and consumption smoothing in China. China & World Econ. 28 (1), 64–93. doi:10.1111/cwe.12312
Lenzen, M. (2016). Structural analyses of energy use and carbon emissions–an overview. Econ. Syst. Res. 28 (2), 119–132. doi:10.1080/09535314.2016.1170991
Li, G., Gao, D., and Li, Y. (2022). Dynamic environmental regulation threshold effect of technical progress on green total factor energy efficiency: Evidence from China. Environ. Sci. Pollut. Res. 29 (6), 8804–8815. doi:10.1007/s11356-021-16292-1
Li, J., Wu, Y., and Xiao, J. J. (2020). The impact of digital finance on household consumption: Evidence from China. Econ. Model. 86, 317–326. doi:10.1016/j.econmod.2019.09.027
Li, Y., Yang, X., Ran, Q., Wu, H., Irfan, M., and Ahmad, M. (2021). Energy structure, digital economy, and carbon emissions: Evidence from China. Environ. Sci. Pollut. Res. 28 (45), 64606–64629. doi:10.1007/s11356-021-15304-4
Li, Z., Li, N., and Wen, H. (2021). Digital economy and environmental quality: Evidence from 217 cities in China. Sustainability 13 (14), 8058. doi:10.3390/su13148058
Li, Z., Liao, G., and Albitar, K. (2020). Does corporate environmental responsibility engagement affect firm value? The mediating role of corporate innovation. Bus. Strategy Environ. 29 (3), 1045–1055. doi:10.1002/bse.2416
Liang, S., Zhao, J., He, S., Xu, Q., and Ma, X. (2019). Spatial econometric analysis of carbon emission intensity in Chinese provinces from the perspective of innovation-driven. Environ. Sci. Pollut. Res. 26 (14), 13878–13895. doi:10.1007/s11356-019-04131-3
Liddle, B. (2014). Impact of population, age structure, and urbanization on carbon emissions/energy consumption: Evidence from macro-level, cross-country analyses. Popul. Environ. 35 (3), 286–304. doi:10.1007/s11111-013-0198-4
Lin, B., and Zhu, J. (2019). Determinants of renewable energy technological innovation in China under CO2 emissions constraint. J. Environ. Manag. 247, 662–671. doi:10.1016/j.jenvman.2019.06.121
Liu, G., Huang, Y., and Huang, Z. (2021). Determinants and mechanisms of digital financial inclusion development: Based on urban-rural differences. Agronomy 11 (9), 1833. doi:10.3390/agronomy11091833
Liu, L., Luan, L., Wu, W., Zhang, Z., and Hsu, Y. (2021). Can digital financial inclusion promote China's economic growth? Int. Rev. Financial Analysis 78, 101889. doi:10.1016/j.irfa.2021.101889
Luc, P. T. (2018). The relationship between perceived access to finance and social entrepreneurship intentions among University students in Vietnam. J. Asian Finance, Econ. Bus. 5 (1), 63–72. Department of Economics, Thu Dau Mot University, Binh Duong, Vietnam. doi:10.13106/jafeb.2018.vol5.no1.63
Ma, M., and Cai, W. (2019). Do commercial building sector-derived carbon emissions decouple from the economic growth in tertiary industry? A case study of four municipalities in China. Sci. Total Environ. 650, 822–834. doi:10.1016/j.scitotenv.2018.08.078
Marvel, M. R., and Lumpkin, G. T. (2007). Technology entrepreneurs’ human capital and its effects on innovation radicalness. Entrepreneursh. Theory Pract. 31 (6), 807–828. doi:10.1111/j.1540-6520.2007.00209.x
Maskus, K. E., Neumann, R., and Seidel, T. (2012). How national and international financial development affect industrial R&D. Eur. Econ. Rev. 56 (1), 72–83. doi:10.1016/j.euroecorev.2011.06.002
Murshed, M., Apergis, N., Alam, M. S., Khan, U., and Mahmud, S. (2022). The impacts of renewable energy, financial inclusivity, globalization, economic growth, and urbanization on carbon productivity: Evidence from net moderation and mediation effects of energy efficiency gains. Renew. Energy 196, 824–838. doi:10.1016/j.renene.2022.07.012
Omri, A., and Afi, H. (2020). How can entrepreneurship and educational capital lead to environmental sustainability? Struct. Change Econ. Dyn. 54, 1–10. doi:10.1016/j.strueco.2020.03.007
Ozturk, I., and Ullah, S. (2022). Does digital financial inclusion matter for economic growth and environmental sustainability in OBRI economies? An empirical analysis. Resour. Conservation Recycl. 185, 106489. doi:10.1016/j.resconrec.2022.106489
Parrish, B. D., and Foxon, T. J. (2006). Sustainability entrepreneurship and equitable transitions to a low-carbon economy. Greener Manag. Int. 55, 47–62. doi:10.9774/gleaf.3062.2006.au.00006
Philip, L., and Williams, F. (2019). Remote rural home based businesses and digital inequalities: Understanding needs and expectations in a digitally underserved community. J. Rural Stud. 68, 306–318. doi:10.1016/j.jrurstud.2018.09.011
Puschmann, T., Hoffmann, C. H., and Khmarskyi, V. (2020). How green FinTech can alleviate the impact of climate change—The case of Switzerland. Sustainability 12 (24), 10691. doi:10.3390/su122410691
Qin, L., Raheem, S., Murshed, M., Miao, X., Khan, Z., and Kirikkaleli, D. (2021). Does financial inclusion limit carbon dioxide emissions? Analyzing the role of globalization and renewable electricity output. Sustain. Dev. 29 (6), 1138–1154. doi:10.1002/sd.2208
Quan, C., Cheng, X., Yu, S., and Ye, X. (2020). Analysis on the influencing factors of carbon emission in China's logistics industry based on LMDI method. Sci. Total Environ. 734, 138473. doi:10.1016/j.scitotenv.2020.138473
Rauf, A., Zhang, J., Li, J., and Amin, W. (2018). Structural changes, energy consumption and carbon emissions in China: Empirical evidence from ARDL bound testing model. Struct. Change Econ. Dyn. 47, 194–206. doi:10.1016/j.strueco.2018.08.010
Razzaq, A., Sharif, A., Najmi, A., Tseng, M. L., and Lim, M. K. (2021). Dynamic and causality interrelationships from municipal solid waste recycling to economic growth, carbon emissions and energy efficiency using a novel bootstrapping autoregressive distributed lag. Resour. Conservation Recycl. 166, 105372. doi:10.1016/j.resconrec.2020.105372
Rehman, A., Ma, H., Ahmad, M., Ozturk, I., and Işık, C. (2021). An asymmetrical analysis to explore the dynamic impacts of CO2 emission to renewable energy, expenditures, foreign direct investment, and trade in Pakistan. Environ. Sci. Pollut. Res. 28 (38), 53520–53532. doi:10.1007/s11356-021-14537-7
Risman, A., Mulyana, B., Silvatika, B., and Sulaeman, A. (2021). The effect of digital finance on financial stability. 11(7), 1979–1984. doi:10.5267/j.msl.2021.3.012
Rogalev, A., Komarov, I., Kindra, V., and Zlyvko, O. (2018). Entrepreneurial assessment of sustainable development technologies for power energy sector. Entrepreneursh. Sustain. Issues 6 (1), 429–445. doi:10.9770/jesi.2018.6.1(26)
Rozario, A. M., and Thomas, C. (2019). Reengineering the audit with blockchain and smart contracts. J. Emerg. Technol. Account. 16 (1), 21–35. doi:10.2308/jeta-52432
Shahbaz, M., Solarin, S. A., Mahmood, H., and Arouri, M. (2013b). Does financial development reduce CO2 emissions in Malaysian economy? A time series analysis. Econ. Model. 35, 145–152. doi:10.1016/j.econmod.2013.06.037
Shahbaz, M., Tiwari, A. K., and Nasir, M. (2013a). The effects of financial development, economic growth, coal consumption and trade openness on CO2 emissions in South Africa. Energy policy 61, 1452–1459. doi:10.1016/j.enpol.2013.07.006
Shahzad, S. J. H., Kumar, R. R., Zakaria, M., and Hurr, M. (2017). Carbon emission, energy consumption, trade openness and financial development in Pakistan: A revisit. Renew. Sustain. Energy Rev. 70, 185–192. doi:10.1016/j.rser.2016.11.042
Shao, S., Yang, L., Yu, M., and Yu, M. (2011). Estimation, characteristics, and determinants of energy-related industrial CO2 emissions in Shanghai (China), 1994–2009. Energy Policy 39 (10), 6476–6494. doi:10.1016/j.enpol.2011.07.049
Sheraz, M., Deyi, X., Ahmed, J., Ullah, S., and Ullah, A. (2021). Moderating the effect of globalization on financial development, energy consumption, human capital, and carbon emissions: Evidence from G20 countries. Environ. Sci. Pollut. Res. 28 (26), 35126–35144. doi:10.1007/s11356-021-13116-0
Stiglitz, J. E., and Weiss, A. (1981). Credit rationing in markets with rationing credit information imperfect. Am. Econ. Rev. 71 (3), 393–410.
Sun, H., Pofoura, A. K., Mensah, I. A., Li, L., and Mohsin, M. (2020). The role of environmental entrepreneurship for sustainable development: Evidence from 35 countries in sub-saharan africa. Sci. Total Environ. 741, 140132. doi:10.1016/j.scitotenv.2020.140132
Szymczyk, K., Şahin, D., Bağcı, H., and Kaygın, C. Y. (2021). The effect of energy usage, economic growth, and financial development on CO2 emission management: An analysis of OECD countries with a high environmental performance index. Energies 14 (15), 4671. doi:10.3390/en14154671
Tamazian, A., Chousa, J. P., and Vadlamannati, K. C. (2009). Does higher economic and financial development lead to environmental degradation: Evidence from BRIC countries. Energy policy 37 (1), 246–253. doi:10.1016/j.enpol.2008.08.025
Tian, Y., Lu, Z., Adriaens, P., Minchin, R. E., Caithness, A., and Woo, J. (2020). Finance infrastructure through blockchain-based tokenization. Front. Eng. Manag. 7 (4), 485–499. doi:10.1007/s42524-020-0140-2
Ting, C. T., Hsieh, C. M., Chang, H. P., and Chen, H. S. (2019). Environmental consciousness and green customer behavior: The moderating roles of incentive mechanisms. Sustainability 11 (3), 819. doi:10.3390/su11030819
Wang, K., Wu, M., Sun, Y., Shi, X., Sun, A., and Zhang, P. (2019). Resource abundance, industrial structure, and regional carbon emissions efficiency in China. Resour. Policy 60, 203–214. doi:10.1016/j.resourpol.2019.01.001
Wang, Q., and Wang, L. (2021). The nonlinear effects of population aging, industrial structure, and urbanization on carbon emissions: A panel threshold regression analysis of 137 countries. J. Clean. Prod. 287, 125381. doi:10.1016/j.jclepro.2020.125381
Wang, R., Zhao, X., and Zhang, L. (2022). Research on the impact of green finance and abundance of natural resources on China's regional eco-efficiency. Resour. Policy 76, 102579. doi:10.1016/j.resourpol.2022.102579
Wang, Z., and Zhu, Y. (2020). Do energy technology innovations contribute to CO2 emissions abatement? A spatial perspective. Sci. Total Environ. 726, 138574. doi:10.1016/j.scitotenv.2020.138574
Wu, Y., and Huang, S. (2022). The effects of digital finance and financial constraint on financial performance: Firm-level evidence from China's new energy enterprises. Energy Econ. 112, 106158. doi:10.1016/j.eneco.2022.106158
Yang, L., Yang, Y., Zhang, X., and Tang, K. (2018). Whether China's industrial sectors make efforts to reduce CO2 emissions from production?-A decomposed decoupling analysis. Energy 160, 796–809. doi:10.1016/j.energy.2018.06.186
Yang, L., and Zhang, Y. (2020). Digital financial inclusion and sustainable growth of small and micro enterprises—Evidence based on China’s new third board market listed companies. Sustainability 12 (9), 3733. doi:10.3390/su12093733
Yang, W., Wang, W., and Ouyang, S. (2019). The influencing factors and spatial spillover effects of CO2 emissions from transportation in China. Sci. Total Environ. 696, 133900. doi:10.1016/j.scitotenv.2019.133900
Yang, X., Su, X., Ran, Q., Ren, S., Chen, B., Wang, W., et al. (2022). Assessing the impact of energy internet and energy misallocation on carbon emissions: New insights from China. Environ. Sci. Pollut. Res. 29 (16), 23436–23460. doi:10.1007/s11356-021-17217-8
Yue, S., Lu, R., Shen, Y., and Chen, H. (2019). How does financial development affect energy consumption? Evidence from 21 transitional countries. Energy Policy 130, 253–262. doi:10.1016/j.enpol.2019.03.029
Zaidi, S. A. H., Zafar, M. W., Shahbaz, M., and Hou, F. (2019). Dynamic linkages between globalization, financial development and carbon emissions: Evidence from asia pacific economic cooperation countries. J. Clean. Prod. 228, 533–543. doi:10.1016/j.jclepro.2019.04.210
Zhang, F., Deng, X., Phillips, F., Fang, C., and Wang, C. (2020). Impacts of industrial structure and technical progress on carbon emission intensity: Evidence from 281 cities in China. Technol. Forecast. Soc. Change 154, 119949. doi:10.1016/j.techfore.2020.119949
Zhang, N., Yu, K., and Chen, Z. (2017). How does urbanization affect carbon dioxide emissions? A cross-country panel data analysis. Energy Policy 107, 678–687. doi:10.1016/j.enpol.2017.03.072
Zhang, Y. J., Liu, Z., Zhang, H., and Tan, T. D. (2014). The impact of economic growth, industrial structure and urbanization on carbon emission intensity in China. Nat. Hazards (Dordr). 73 (2), 579–595. doi:10.1007/s11069-014-1091-x
Zhang, Y. J., Sun, Y. F., and Huang, J. (2018). Energy efficiency, carbon emission performance, and technology gaps: Evidence from CDM project investment. Energy Policy 115, 119–130. doi:10.1016/j.enpol.2017.12.056
Zhang, Y., and Zhang, S. (2018). The impacts of GDP, trade structure, exchange rate and FDI inflows on China's carbon emissions. Energy Policy 120, 347–353. doi:10.1016/j.enpol.2018.05.056
Keywords: digital finance, carbon emissions, impact mechanism, spatial spillover, environment
Citation: Ding X, Gao L, Wang G and Nie Y (2022) Can the development of digital financial inclusion curb carbon emissions? Empirical test from spatial perspective. Front. Environ. Sci. 10:1045878. doi: 10.3389/fenvs.2022.1045878
Received: 16 September 2022; Accepted: 10 October 2022;
Published: 24 October 2022.
Edited by:
Cem Isik, Anadolu University, TurkeyCopyright © 2022 Ding, Gao, Wang and Nie. This is an open-access article distributed under the terms of the Creative Commons Attribution License (CC BY). The use, distribution or reproduction in other forums is permitted, provided the original author(s) and the copyright owner(s) are credited and that the original publication in this journal is cited, in accordance with accepted academic practice. No use, distribution or reproduction is permitted which does not comply with these terms.
*Correspondence: Lifei Gao, lifeigao@btbu.edu.cn