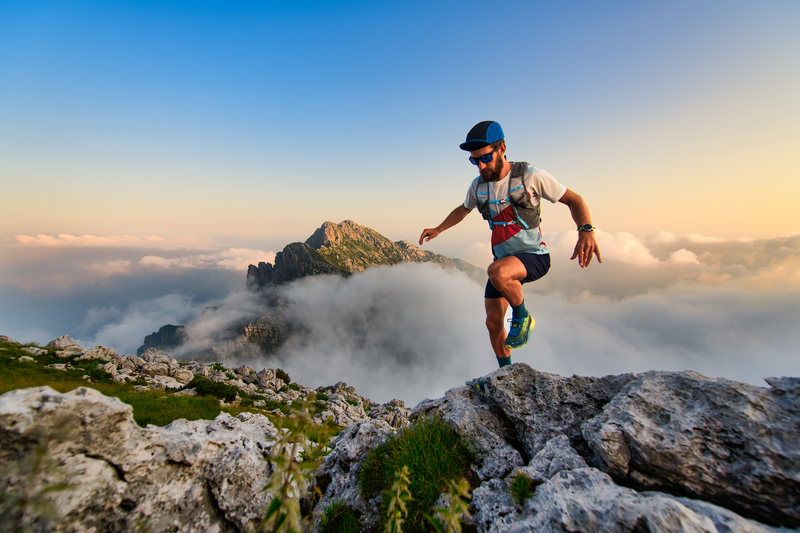
94% of researchers rate our articles as excellent or good
Learn more about the work of our research integrity team to safeguard the quality of each article we publish.
Find out more
ORIGINAL RESEARCH article
Front. Environ. Sci. , 28 October 2022
Sec. Land Use Dynamics
Volume 10 - 2022 | https://doi.org/10.3389/fenvs.2022.1028750
The dual carbon target has been the focus of domestic attention since it was proposed. At the same time, the digital economy based on new technologies such as the Internet and big data has become an important engine to boost China’s high-quality economic development. While the digital economy is promoting the development of the urban economy, can it also promote the low carbon development of cities in China? Based on panel data and multiple econometric models of 26 cities in the Yangtze River Delta urban agglomeration from 2006 to 2020, this paper empirically examines the impact of digital economy development on urban carbon emissions. The results show that the development of the digital economy can not only reduce the carbon emission intensity of this region but also effectively reduce the carbon emission intensity of surrounding areas. Therefore, it is necessary to further promote the construction of digital economy in the urban agglomeration, improve the inclusiveness and flexibility of the implementation of digital economy development strategy, and improve the construction of information infrastructure to give full play to the role of the digital economy in improving carbon emissions in urban agglomeration.
Global warming is a climate problem that all countries in the world need to face together, and it is one of the most difficult challenges that mankind needs to face in history (Lehtonen et al., 2019; Elahi et al., 2022). The increase in greenhouse gas emissions will bring irreversible changes to the climate, which is not conducive to the production, life, and sustainable development of our society (Shan et al., 2018)。 As a responsible country, China has always been committed to exploring effective ways to reduce carbon emissions and has carried out a series of carbon emission reduction measures such as carbon emission trading pilot and promotion. In September 2020, China put forward the double carbon strategy of carbon peak by 2030 and carbon neutralization by 2060 at the United Nations General Assembly, and set targets for China’s carbon emission reduction actions.
The proposal of the double carbon strategy has once again set off a research upsurge on the development of the low-carbon economy. The “Fourteenth Five Year Plan” Comprehensive Work Plan for Energy Conservation and Emission Reduction pointed out that China’s ecological civilization construction needs to focus on carbon reduction as a strategic direction to achieve a fundamental improvement in the quality of the ecological environment. Traditional factors affecting carbon emissions mainly include population size, industrial structure, energy structure, and so on. At present, a large number of studies focus on population size (Dietz and Rosa, 1997; Rahman et al., 2020), industrial structure (Di et al., 2020; Pan et al., 2021; Zhao et al., 2022)、Environmental regulation (Ouyang et al., 2020; Wang and Zhang, 2022; Xu and Xu, 2022)、technological innovation (Cheng et al., 2021; Obobisa et al., 2022; Rahman et al., 2022) on carbon emissions. Cities’ emissions account for about 75%–80% of the global carbon emissions, while China’s urban carbon emissions account for more than 80% of the total carbon emissions (Shan et al., 2017; Kai et al., 2020). An urban agglomeration is the advanced stage of urban development and the highest spatial organization of urban development in the mature stage (Tang et al., 2020). Highly integrated urban agglomerations have typical space-time characteristics of carbon emission, and their carbon emission reduction tasks are more severe than those of Ordinary Cities (Cui et al., 2020; Shen et al., 2021; Cheng et al., 2022). The diversity of influencing factors of carbon emissions leads to the economic, financial, and industrial transformation and other risks that China will face in the process of achieving the double carbon goals. Therefore, it is a necessary guarantee for China to achieve the double carbon goal to promote the governance of climate issues in multiple ways (Cai et al., 2022).
The digital economy, as a new economic form (Quah, 2003; Kajtazi, 2010), combined with the real economy, has injected new momentum into China’s economic development. According to the report released by the China Information and Communication Research Institute, the scale of the digital economy has reached 39.2 trillion yuan in 2020, accounting for 38.6% of GDP. The digital economy has become an important driver of economic development and social transformation in China. According to the existing studies, the economic benefit of the digital economy is the main research direction. For example, the role of the digital economy in promoting high-quality development (Ma and Zhu, 2022; Yang et al., 2022), the influence of the digital economy on technological innovation (Cao et al., 2021; Lin and Ma, 2022), etc. Only a few articles discuss the impact of digital economy development on carbon emissions (Chen et al., 2019; Zhang et al., 2022). The research of Xiong Wang (Wang et al., 2022) shows that the development of digital Inclusive Finance is conducive to regional carbon emission reduction. HongliWang (Wang and Guo, 2022) and other researchers found that digital inclusive finance can help reduce urban carbon dioxide emissions, and there is a significant positive spatial spillover effect in China’s urban carbon dioxide emissions.
However, most of these studies focus on the national level, and there are few studies on urban agglomeration. Therefore, studying the impact of the digital economy development on carbon emissions of urban agglomeration can effectively open up a new perspective. Presenting enormous value for creating a digital urban economy and investigating new avenues for reducing urban carbon emissions. This paper uses the panel data of 26 prefecture-level cities in the Yangtze River Delta urban agglomeration from 2006 to 2020 to calculate the carbon emissions of each city in the Yangtze River Delta and constructs a digital economy indicator system. Then, using a variety of spatial econometric models, the paper empirically tested the relationship between the development of the digital economy and carbon emissions at the level of urban agglomeration. There are possible innovations in this paper: First, it provides a new perspective, that is, from the perspective of urban agglomeration, to study the impact of digital economy development on carbon emissions. The second is to discuss the spatial spillover effect of the digital economy on carbon emission reduction based on the geographical characteristics of cities in the Yangtze River Delta, to help grasp the impact mechanism of the digital economy on urban carbon emissions.
To explore the impact of digital economic development on carbon emissions in the Yangtze River Delta urban agglomeration, the basic static panel model is constructed as follows:
Where, i represents the city,t represents the year,
Previous studies have shown that the economic and cultural links between the Yangtze River Delta urban agglomeration are close, which is beneficial to the flow of factors and products. Therefore, the carbon emissions of cities in the Yangtze River Delta urban agglomeration will not only affect themselves but also affect the ecological environment of nearby areas. Based on the spatial correlation of urban agglomeration carbon emissions, this paper uses a variety of spatial models to study the impact of digital economic development on carbon emissions.
Where,
When
When
When
This paper selects the panel data of 26 cities in the Yangtze River Delta urban agglomeration from 2006 to 2020. The data mainly comes from the statistical yearbook of Chinese cities and the statistical yearbook of cities at all levels. Some missing values are supplemented by linear interpolation.
The carbon emission sources of urban agglomerations mainly include gas, liquefied petroleum gas, and electric power. Considering the availability of data and the consistency of data sources, this paper calculates the carbon emissions generated by cities in the Yangtze River Delta Based on the energy statistics and power coefficient of cities in the Yangtze River Delta urban agglomerations and takes the logarithm.
Based on the availability of urban agglomeration data, this paper measures the level of digital economy from the perspective of digital industrialization (Table 1). Including the total amount of telecommunication services, the number of personnel in the information transmission computer services and software industry, the number of Internet users, and the number of mobile phone users at the end of the year. Since the index evaluation measured by the entropy weight method is relatively objective, this paper uses the entropy weight method to calculate the digital economic development index.
Where,
There are many influencing factors on carbon emissions. This paper refers to the existing literature and based on the availability of data at the city level, selects the following control variables:
The population is an important influencing factor of urban carbon emissions, expressed by the logarithm of the resident population. Economic growth is measured by the per capita GDP of the city. The improvement of economic development level is often accompanied by an increase in carbon emissions, which is expressed by the logarithm of per capita GDP. Government intervention: The financial input of local governments plays a key role in carbon emission reduction, which is expressed by the proportion of the expenditure in the general budget of local finance to the GDP. Level of foreign trade: foreign capital can bring advanced technology levels, to reduce urban carbon emissions, which is expressed by the proportion of the amount of foreign capital used in that year in the regional GDP. The rise in environmental pollution levels is often accompanied by the increase in urban carbon emissions, which is expressed by the logarithm of the total industrial sulfur dioxide emissions. The impact of Scientific and Technological Development on urban carbon emissions is expressed by the proportion of scientific expenditure in the general budget of local finance. The regional financial development status is expressed by the proportion of the balance of various loans of financial institutions in the regional GDP at the end of the year. The improvement of the urbanization rate is an important column in the urbanization process, which is expressed by the proportion of the urban population to the resident population.
First, the panel model is used to estimate the impact of digital economy development on carbon emissions. This paper conducts the Hausman test on the estimation results of fixed effect and random effect models, and the results show that the p value is less than 0.0001, which means that the fixed effect model should be used.
In the fixed effect model, the estimation coefficient of the core explanatory variable digital economy development level (dig) is negative and significant, which means the urban carbon emissions show a downward trend with the development of the digital economy. Indicating that the development of the digital economy can promote the reduction of urban carbon emissions.
In addition, in the estimation results of fixed effect control variables, population (pop), economic growth (gdp), government intervention (gov), Scientific and Technological Development (sci), regional financial development status (fin), urbanization rate (urb) of each city have a positive impact on the growth of urban carbon emissions (refer Table 2). The level of foreign trade (for) is negative but not significant, which indicates that the increase in a city’s foreign trade volume has not significantly reduced the city’s carbon emissions. It may be because with the increase of foreign trade, the city’s economy will have a “lock-in effect”, which makes the economy stagnate at the stage of processing with imported materials and exporting labor-intensive products, reducing the upgrading speed of the city’s industrial structure and failing to reduce the city’s carbon emissions. Environmental pollution level (env) coefficient is close to zero and is not significant (see Table 3). Urban pollution will not have an obvious impact on urban carbon emissions. This may be because the enterprises with heavy environmental pollution in the city have been included in the stricter environmental supervision system and the emission of pollutants has been strictly restricted, resulting in an unclear relationship between urban pollution and urban carbon emissions.
Carbon emission has a strong diffusivity. As a closely linked economic and geographical urban structure, urban agglomerations can easily lead to the cross-border impact of carbon emission, and then trigger a “tragedy of the commons,” leading to the reverse phenomenon of carbon emission control in some cities in the Urban Agglomerations. Therefore, the carbon emission reduction policy of urban agglomerations should not only take a single city as the optimization target, but also take the urban collaborative governance as the target to effectively reduce urban carbon emissions. In this paper, the global Moran index is selected to test the spatial autocorrelation of the carbon emissions of the Yangtze River Delta Urban Agglomeration (Table 4). The result demonstrate that the Moran index of carbon emissions of most urban agglomerations in the Yangtze River Delta from 2006 to 2020 is significantly positive, indicating that the carbon emissions of this region are positively affected by the carbon emissions of surrounding regions.
The spatial error model (SEM), spatial dobbin model (SDM), and spatial autoregressive model (SAR) are used for estimation (Table 5), and the estimation results all pass the robustness test. In all models, the digital economy development level coefficient is negative, indicating that with the development of the digital economy, the carbon emission intensity of cities in the Yangtze River Delta will continue to decrease. Compared with traditional industries, the development of a digital economy can effectively improve the utilization efficiency of urban resources, promote the recycling of resources, drive the low-carbon development of urban industries, and reduce carbon emission intensity.
On the other side, the results show that the coefficient of population size is positive, indicating that there is a positive relationship between urban population and urban carbon emission intensity. This is because the increase of population density may further consume urban energy, resulting in the reduction of resource utilization efficiency, generating more unexpected output and aggravating urban carbon emissions. The growth of economic development level will lead to the increase of urban carbon emissions, because the increase of urban economic development level will lead to the increase of energy demand, which will lead to the increase of fossil energy consumption and ultimately promote the increase of carbon emissions.
Spatial dobbin model regression cannot fully reflect the marginal impact of the digital economy and various control variables on carbon emissions, nor can it judge how much spatial spillover effect the digital economy has on neighboring regions. Therefore, to ensure the comprehensiveness of the analysis, the influence of explanatory variables on the explained variables in the spatial econometric model is divided into direct effects and indirect effects for analysis.
Table 6 shows the direct, indirect, and total effects of digital economic development and urban carbon emissions. The direct effect refers to the influence of local explanatory variables on local explanatory variables; the indirect effect refers to the influence of explanatory variables in this region on explanatory variables in neighboring regions. The total effect is the average influence degree of the explanatory variable of a certain place on the explained variable of the whole region.
TABLE 6. Direct, indirect, and total effects of digital economy development on urban carbon emissions.
The estimated coefficient of direct effect and indirect effect of digital economic development on urban carbon emissions are −1.102 and −0.558, which are both significant. This shows that the development of the digital economy can not only reduce the intensity of carbon emissions in the local area but also reduce the intensity of carbon emissions in surrounding areas. Carbon emissions are highly spatially related, therefore, conventional carbon emission control policies cannot efficiently solve the problem. However, the development of the digital economy can reduce the inter-regional transfer of carbon emissions to a certain extent. So the Yangtze River Delta urban agglomeration needs to vigorously develop the digital economy. Improve the economic synergy of the urban agglomeration, and realize the joint prevention and control of urban carbon emissions.
From the perspective of control variables, the direct and indirect effects of population size are significantly positive, indicating that the growth of population size will drive the increase of carbon emissions in surrounding areas. For the level of economic development, the direct and indirect effects of the level of economic development on carbon emissions are significantly positive. This indicate that in the neighboring areas, the increase in the level of economic development will lead to an increase in carbon emissions in the local and surrounding areas. This is aligned with the theoretical analysis of the impact of economic development on carbon emissions. The direct effect and indirect effect of the foreign trade development level are negative but not significant, indicating that the level of foreign direct investment in cities has no significant impact on urban carbon emissions. The direct and indirect effects of scientific and technological development on carbon emissions are significantly positive, which indicates that in the neighboring areas, the increase in scientific and technological expenditure will lead to an increase in carbon emissions in the region and the surrounding areas. This may be because the Yangtze River Delta urban agglomeration is still in the stage of industrial transformation. When the urban transformation is not completed, the scientific and technological innovation investment of green industries in scientific and technological expenditure is still small compared with that of traditional industries. As for regional financial development, the direct and indirect effects of regional financial development on carbon emissions are significantly positive. The increase in regional financial development will lead to an increase in carbon emissions in the region and the surrounding areas. This may be because the loan enterprises in the urban agglomeration have not invested their loans in the emerging green industries. Compared with the traditional industries, the investment return of the emerging green industries is more unstable. The direct and indirect effects of the urbanization rate on carbon emissions are significantly positive, that is, the increase in urbanization rate will lead to an increase in carbon emissions in the region and surrounding areas. With the increase in urbanization rate, energy consumption will gradually concentrate on urban agglomeration, which will lead to an increase in urban carbon emissions, which is consistent with the theoretical analysis of the impact of urbanization on carbon emissions.
The growth of most control variables will not only lead to the increase of local urban carbon emissions but also increase the carbon emissions of surrounding areas, which further illustrates the necessity of multi City collaborative governance of carbon emissions and the importance of developing a digital economy.
This paper adopts the following two robustness test methods:
(1) Replace the spatial weight matrix. Different weight matrices may lead to different regression results of spatial econometric models. To ensure the robustness of the research results, this paper replaces the geographic distance matrix with a 0–1 matrix to test the impact of digital economic development on carbon emissions.
(2) The second is to replace the explained variables, replacing the logarithm of urban carbon dioxide emissions with urban per capita carbon dioxide emissions. The above robustness test results are shown in Table 7. The sign of the digital economic development index coefficient is consistent with the previous text, and the significance is stronger. The results of this paper are robust.
This paper selects the panel data of 26 cities in the Yangtze River Delta from 2006 to 2020, and comprehensively analyzes the impact of the development degree of the digital economy on carbon emission intensity in the Yangtze River Delta by using benchmark regression and SEM, SDM, and SAR spatial econometric models.
After passing the robustness test, the conclusions of this paper are as follows:
First, in the SEM and SAR models, the development of a digital economy can effectively reduce urban carbon emissions. Second, through the analysis of direct and indirect effects, the development of the digital economy can not only reduce the carbon emission intensity of the local area but also effectively reduce the carbon emission intensity of surrounding areas.
The Yangtze River Delta urban agglomeration is currently in an important transition period, and the sustainability of urban economic development is a major challenge to be faced during the transition period. The development of the digital economy and carbon emissions are the most representative topics. Therefore, this paper systematically discusses the internal relationship between the digital economy and urban carbon emission intensity. Through empirical research, it is found that the development of the digital economy has significantly reduced the intensity of urban carbon emissions, and this conclusion is still valid after considering endogenous issues and a series of other robustness tests.
At the same time, further analysis show that most urban development indicators have a positive impact on urban carbon emissions in the process of urban agglomeration transformation, such as the degree of urban government intervention, urban science, and technology investment, etc. Therefore, the development of the digital economy should play a leading role in the process of carbon emission reduction of urban agglomeration and offset the increased carbon emissions of other development indicators of urban agglomeration through the energy conservation and emission reduction effects caused by the development of the digital economy.
Based on the above research results, the enlightenment is as follows:
First, it is necessary to accelerate the development of the digital economy and promote the development of the digital economy by cooperating in many fields such as big data, cloud computing, the Internet of things, blockchain, artificial intelligence, and 5g communication. The expansion of population scale, the improvement of economic development level, and the improvement of urbanization rate in the Yangtze River Delta urban agglomeration are all contributing to the increase of urban carbon emissions. At the same time, the digital economy should play a major role in the urban development process as soon as possible because it can promote carbon emission reduction in cities and surrounding areas. Cities in the Yangtze River Delta should work together to build a digital economy industrial system of the Yangtze River Delta urban agglomeration, jointly research and develop information technology and build a digital economy industrial cluster, promote the deep integration of the digital economy and the real economy, popularize the digital economy development dividend, improve the awareness of energy conservation and emission reduction of urban residents, promote the connotative growth of the digital economy, and ensure the effective collaborative governance of urban carbon emissions.
Second, reduce the government’s intervention in the development of the digital economy and reduce the government’s investment in science and technology. The development of the digital economy of the Yangtze River Delta urban agglomeration is still in the primary stage. The invisible hand can better stimulate the development potential of the digital economy. The digital economy without government intervention can also improve the resource output capacity of green industries and reduce the intensity of urban carbon emissions.
First, there is a lack of an authoritative framework system for digital economic development to measure the degree of digital economic development at the regional level. We can calculate the digital economy index from the aspects of digital economy infrastructure, economic scale, innovation ability, digital development environment, and build a more accurate digital economy development index system.
Secondly, the calculation of urban carbon emissions in this paper only involves the consumption of natural gas, liquefied petroleum gas, and other resources, but there are still many other ways to generate greenhouse gases in daily life. The follow-up research is based on the data availability of the research sample, and the corresponding energy is added when calculating the urban carbon emissions.
Finally, China has a vast territory and great differences in national conditions. This paper does not analyze the relationship between the development of the digital economy and carbon emissions of other urban agglomerations in China, and cannot obtain a more comprehensive system and detailed research results. The follow-up study can verify whether the development of the digital economy is universal to the carbon emissions of urban agglomerations.
The raw data supporting the conclusions of this article will be made available by the authors, without undue reservation.
All authors have an equal level of contributions.
The authors declare that the research was conducted in the absence of any commercial or financial relationships that could be construed as a potential conflict of interest.
All claims expressed in this article are solely those of the authors and do not necessarily represent those of their affiliated organizations, or those of the publisher, the editors and the reviewers. Any product that may be evaluated in this article, or claim that may be made by its manufacturer, is not guaranteed or endorsed by the publisher.
Cai, B., Zhang, L., Lei, Y., et al. (2022). A deeper understanding of the CO2 emission pathway under China’s carbon emission peak and carbon neutrality goals [J]. Engineering.
Cao, S., Nie, L., Sun, H., Sun, W., and Taghizadeh-Hesary, F. (2021). Digital finance, green technological innovation and energy-environmental performance: Evidence from China's regional economies. J. Clean. Prod. 327, 129458. doi:10.1016/j.jclepro.2021.129458
Chen, X., Gong, X., Li, D., and Zhang, J. (2019). Can information and communication technology reduce CO2 emission? Environ. Sci. Pollut. Res. 26 (2-3), 32977–32992. doi:10.1007/s11356-019-06380-8
Cheng, C., Ren, X., Dong, K., and Wang, Z. (2021). How does technological innovation mitigate CO2 emissions in OECD countries? Heterogeneous analysis using panel quantile regression [J]. J. Environ. Manag., 111818. doi:10.1016/j.jenvman.2020.111818
Cheng, J., Xu, L., Wang, H., Geng, Z., and Wang, Y. (2022). How does the marginal abatement cost of CO2 emissions evolve in Chinese cities? An analysis from the perspective of urban agglomerations [J]. Sustain. Prod. Consum. 32, 147–159. doi:10.1016/j.spc.2022.04.013
Cui, C., Cai, B., Bin, G., and Wang, Z. (2020). Decennary spatial pattern changes and scaling effects of CO2 emissions of urban agglomerations in China. Cities 105, 102818. doi:10.1016/j.cities.2020.102818
Di, Z., Xiaoru, Z., and Xueqin, W. (2020). Research on coupling degree and coupling path between China's carbon emission efficiency and industrial structure upgrading [J]. Environ. Sci. Pollut. Res. Int. 27 (20).
Dietz, T., and Rosa, E. A. (1997). Effects of population and affluence on CO 2 emissions. Proc. Natl. Acad. Sci. U. S. A. 94 (1), 175–179. doi:10.1073/pnas.94.1.175
Elahi, E., Khalid, Z., Tauni, M. Z., Zhang, H., and Lirong, X. (2022). Extreme weather events risk to crop-production and the adaptation of innovative management strategies to mitigate the risk: A retrospective survey of rural Punjab, Pakistan. Technovation 117, 102255. doi:10.1016/j.technovation.2021.102255
Kai, S. A., Dzw, A., and Wxla, B. (2020). Influencing factors and spatial patterns of energy-related carbon emissions at the city-scale in Fujian province, Southeastern China [J]. J. Clean. Prod., 244.
Kajtazi, M. (2010). “Information asymmetry in the digital economy,” in Proceedings of the International Conference on Information Society.
Lehtonen, A., Salonen, A. O., and Cantell, H. (2019). Climate change education: A new approach for a world of wicked problems [J]. Sustain. Hum. Well-Being, Future Educ.
Lin, B., and Ma, R. (2022). How does digital finance influence green technology innovation in China? Evidence from the financing constraints perspective [J]. J. Environ. Manag. 320, 115833. doi:10.1016/j.jenvman.2022.115833
Ma, D., and Zhu, Q. (2022). Innovation in emerging economies: Research on the digital economy driving high-quality green development. J. Bus. Res. 145, 801–813. doi:10.1016/j.jbusres.2022.03.041
Obobisa, E. S., Chen, H., and Mensah, I. A. (2022). The impact of green technological innovation and institutional quality on CO2 emissions in African countries. Technol. Forecast. Soc. Change 180, 121670. doi:10.1016/j.techfore.2022.121670
Ouyang, X., Fang, X., Cao, Y., and Sun, C. (2020). Factors behind CO2 emission reduction in Chinese heavy industries: Do environmental regulations matter? Energy Policy 145, 111765. doi:10.1016/j.enpol.2020.111765
Pan, X., Xu, H., Song, M., Lu, Y., and Zong, T. (2021). Forecasting of industrial structure evolution and CO2 emissions in Liaoning Province. J. Clean. Prod. 285, 124870. doi:10.1016/j.jclepro.2020.124870
Quah, D. (2003). Digital goods and the new economy [J]. LSE Res. Online Documents Econ. 167 (3), 401.
Rahman, M. M., Alam, K., and Velayutham, E. (2022). Reduction of CO2 emissions: The role of renewable energy, technological innovation and export quality. Energy Rep. 8, 2793–2805. doi:10.1016/j.egyr.2022.01.200
Rahman, M. M., Saidi, K., and Mbarek, M. B. (2020). Economic growth in south asia: The role of CO2 emissions, population density and trade openness. Heliyon 6 (5). e03903. doi:10.1016/j.heliyon.2020.e03903
Shan, Y., Guan, D., Zheng, H., Ou, J., Li, Y., Meng, J., et al. (2018). China CO2 emission accounts 1997–2015. Sci. Data 5, 170201. doi:10.1038/sdata.2017.201
Shan, Y. S., Guan, D., Liu, J., Mi, Z., Liu, Z., Liu, J., et al. (2017). Methodology and applications of city level CO2 emission accounts in China. J. Clean. Prod. 161, 1215–1225. doi:10.1016/j.jclepro.2017.06.075
Shen, W., Liang, H., Dong, L., Ren, J., and Wang, G. (2021). Synergistic CO2 reduction effects in Chinese urban agglomerations: Perspectives from social network analysis. Sci. Total Environ. 2021, 149352. doi:10.1016/j.scitotenv.2021.149352
Tang, D., Zhang, Y., and Bethel, B. J. (2020). A comprehensive evaluation of carbon emission reduction capability in the Yangtze River economic belt. Int. J. Environ. Res. Public Health 17 (2), 545. doi:10.3390/ijerph17020545
Wang, H., and Guo, J. (2022). Impacts of digital inclusive finance on CO2 emissions from a spatial perspective: Evidence from 272 cities in China. J. Clean. Prod. 355, 131618. doi:10.1016/j.jclepro.2022.131618
Wang, H., and Zhang, R. (2022). Effects of environmental regulation on CO2 emissions: An empirical analysis of 282 cities in China. Sustain. Prod. Consum. 29, 259–272. doi:10.1016/j.spc.2021.10.016
Wang, X., Wang, X., Ren, X., and Wen, F. (2022). Can digital financial inclusion affect CO2 emissions of China at the prefecture level? Evidence from a spatial econometric approach [J. Energy Econ. 109, 105966. doi:10.1016/j.eneco.2022.105966
Xu, B., and Xu, R. (2022). Assessing the role of environmental regulations in improving energy efficiency and reducing CO2 emissions: Evidence from the logistics industry. Environ. Impact Assess. Rev. 96, 106831. doi:10.1016/j.eiar.2022.106831
Yang, Q., Ma, H., Wang, Y., and Lin, L. (2022). Research on the influence mechanism of the digital economy on regional sustainable development. Procedia Comput. Sci. 202, 178–183. doi:10.1016/j.procs.2022.04.025
Zhang, J., Lyu, Y., Li, Y., and Geng, Y. (2022). Digital economy: An innovation driving factor for low-carbon development. Environ. Impact Assess. Rev. 96, 106821. doi:10.1016/j.eiar.2022.106821
Keywords: digital economy, carbon emissions, urban agglomeration, space measurement, agglomeration
Citation: Zha Q, Huang C and Kumari S (2022) The impact of digital economy development on carbon emissions -- based on the Yangtze River Delta urban agglomeration. Front. Environ. Sci. 10:1028750. doi: 10.3389/fenvs.2022.1028750
Received: 26 August 2022; Accepted: 03 October 2022;
Published: 28 October 2022.
Edited by:
Salvador García-Ayllón Veintimilla, Technical University of Cartagena, SpainReviewed by:
Ehsan Elahi, Shandong University of Technology, ChinaCopyright © 2022 Zha, Huang and Kumari. This is an open-access article distributed under the terms of the Creative Commons Attribution License (CC BY). The use, distribution or reproduction in other forums is permitted, provided the original author(s) and the copyright owner(s) are credited and that the original publication in this journal is cited, in accordance with accepted academic practice. No use, distribution or reproduction is permitted which does not comply with these terms.
*Correspondence: Sonia Kumari, U29uaWEua3VtYXJpQGliYS1zdWsuZWR1LnBr
Disclaimer: All claims expressed in this article are solely those of the authors and do not necessarily represent those of their affiliated organizations, or those of the publisher, the editors and the reviewers. Any product that may be evaluated in this article or claim that may be made by its manufacturer is not guaranteed or endorsed by the publisher.
Research integrity at Frontiers
Learn more about the work of our research integrity team to safeguard the quality of each article we publish.