- 1Department of Industrial and Production Engineering, Bangladesh University of Engineering and Technology, Dhaka, Bangladesh
- 2Department of Mechanical and Production Engineering, Ahsanullah University of Science and Technology, Dhaka, Bangladesh
- 3Department of Mechanical and Industrial Engineering, College of Engineering, Prince Sattam Bin Abdulaziz University, Al Kharj, Saudi Arabia
The ongoing COVID-19 pandemic has manifold disastrous effect on different service and production sectors, and manufacturing industries are no exception. Emerging technologies (ETs) can play a pivotal role in reviving these ailing manufacturing industries. However, the cost of implementing and operating ETs is a prime concern. Nonetheless, the important attributes that will minimize the cost and harness the advantages of the technology are still to be explored. To address this gap, this research seeks to examine the critical attributes required for the effective and efficient deployment of ETs. At first, a detailed literature review was conducted to identify and sort the attributes influencing the effective use of ETs in manufacturing industries. After that, Fuzzy-TODIM (Portuguese abbreviation of “Interactive and Multi-Criteria Decision Making”) method was used to rate the importance of the attributes. The result reveals that, “Inventory and Resource Management” tops the attribute list responsible for exploiting the optimal usage ETs, followed by “Development of Skilled Workforce” and “Supplier and Service Management Capability,” respectively. This paper will assist industry professionals not only in using ETs but also getting the best yield from them strategically and practically.
1 Introduction
SARS CoV-2 (COVID-19/coronavirus), which was discovered in late 2019 in Wuhan, China, has become a global menace (Lai et al., 2020; Ceban et al., 2022). Unlike the 1918 Spanish Flu, the coronavirus pandemic has disrupted global markets (tourism, airlines, and manufacturing) and degraded cultural standards (Fernandes 2020). Due to the scarcity of raw materials, delayed product delivery, and disrupted logistic networks, companies and suppliers are experiencing order suspensions and decline in performance, revenue, service quality, productivity, and other key performance indicators (KPIs). Therefore, there have been significant alterations in business practices and consumer expectations (Donthu and Gustafsson 2020).
Initially considered only as a health hazard, COVID-19 has wreaked havoc on the global economy, commerce, and financial markets, with a potential economic effect of 2–4 trillion USD (Benedict 2020). For instance, 980 million pcs of orders worth about 3.17 billion US dollars have been canceled affecting around 2.27 million workers in the readymade garments (RMG) sector of Bangladesh (CNN 2020). Furthermore, other problems related to lack of commodities, failure to source products in a face-to-face exchange framework, and significant disparity between the quantity of materials accessible and their demand have risen from the crisis arisen from COVID-19 (Farrell et al., 2020). Because of the substantial drop in manufacturing feasibility, industry professionals and researchers are investigating and assessing various means to revitalize the sector’s sustainability policies. In 2021, many industries across the globe restarted the supply chain operations to mitigate the ongoing economic crisis by COVID-19 pandemic. However, recovery strategies have not yielded expected results, while industries have found it difficult to bounce back from looming extinction (Tang et al., 2021). Some researchers have suggested that post-COVID recovery may be aided by adopting emerging technologies (ETs) (Moradlou et al., 2022), as well as sustainable and resilient infrastructure (Zenghelis 2021).
The COVID-19 pandemic underlined the significance of ETs and digitalization, which can facilitate supply, production, and order via digital platforms to consumers and build institutionalized consumers. As a result, manufacturers have started to recognize the need for Industry 4.0 technologies, such as Internet of Things (IoT), block-chain technology, cyber-physical systems (CPS), cloud computing, cognitive computing, and RFID (radio frequency identification). All these technologies offer Localization-Agility-Digitization (LAD) insights in responding to industry trends or emerging consumer needs, visibility, accountability, confidentiality, real-time data, intelligent contracts, and sustainability (Bharadwaj et al., 2013; Cirera et al., 2021; Nandi et al., 2021). There has been a lot of progress made in the potential application of ETs across several industries, for example, application of IoT in facility and manufacturing process modeling as well as in reshaping and decentralizing industries, use of artificial intelligence techniques in business innovation, and optimizing the financing of ETs in industries, among others (Attaran and Gunasekaran 2019; Lee et al., 2019; Maganga and Taifa 2022). In addition, there are studies about how and where ETs can be used in industries (Zabel and Telkmann 2021; Mi et al., 2022; Zeng et al., 2022). However, the COVID-19 pandemic calls for more than just using ETs. Instead, amid the disrupted supply chain and production system during this pandemic, industrial managers must become more cost-efficient and resilient about using ETs for sustainable recovery of manufacturing industries. However, the efficient use of ETs is characterized by several key attributes. Therefore, it is crucial to recognize and evaluate the attributes. Quantitative evaluation of these attributes for manufacturing industries in the context of COVID-19 pandemic is a grand challenge. This study aims to deal with such a grand challenge and poses the following research questions.
RQ1: What are the possible attributes that characterize the use of ETs for sustainable recovery of manufacturing industries in the context of COVID-19 Pandemic?
RQ2: How can industrial managers evaluate the benefits and cost of using ETs for sustainable recovery of manufacturing industries?To answer the above research questions, this work deploys fuzzy-TODIM (an acronym in Portuguese for Interactive and Multicriteria Decision Making) to rank the attributes that are more coherent for enhancing the benefits and minimizing the cost of using ETs for the sustainable recovery of manufacturing industries. Additionally, this study examines the relationship between the problems arising from the COVID-19 pandemic, and therefore draws a comprehensive outlook to prioritize the attributes of ETs for seamless adoption of them in manufacturing industries.The rest of the paper is structured as follows: Section 2 discusses the related literature. Section 3 illustrates the proposed methods. Section 4 provides findings with theoretical and empirical implications. Section 5 concludes this paper.
2 Literature review
This section discusses emerging technology (ET) for sustainability, their attributes, and the contributions of this study.
2.1 COVID-19 and emerging technology for sustainability
Since 2019, the global business has been disrupted by a novel virus called COVID-19 (Ivanov and Dolgui 2020; Hald and Coslugeanu 2021). COVID-19 has thrown up multiple threats by simultaneously affecting the global workforce, economy, and health care. Because sustainability (“development that satisfies the demands of the present generation without compromising the capacity of future generations to satisfy their own needs”) is a process that occurs over extended periods of time, it requires more awareness and planning by industry leaders (Curren 2011). In addition to this, the COVID-19 epidemic has shown how the three aspects (economic, social, and environmental) of sustainability are intertwined (Sarkis 2021). New social sustainability standards are introduced when people’s lifestyles change, for example, work-from-home policy and less in-person encounters, due to a greater social distance. Even though social and economic sustainability problems are addressed in this article, the major discussion here will focus on emerging technology along with sustainability.
Emerging technologies (ETs) refer to scientific breakthroughs that have the potential to start a new sector or alter an existing one (Srinivasan 2008). There is a widespread view that new technology is a tool for addressing the key problems of the twenty-first century (Groen and Walsh 2013). New technological advances that have been introduced in recent years include computational sciences (Zhao et al., 2020a), nanotechnology (Patel and Pathak 2021), micro-electromechanical systems (MEMS) (Le et al., 2021), biofuels (Patel et al., 2020), mobile technologies (Leu et al., 2011), and a myriad of other advancements. ETs may encompass a number of things like artificial intelligence (AI) (Haenlein et al., 2019; Yan et al., 2021), 3D printing (Yazdi et al., 2016; Wadher et al., 2021), IoT (Zhao et al., 2020b; Pradhan and Chawla 2020) and others. Some researchers considered Big Data Analytics as an emerging technology (Kend and Nguyen 2020). The applications of ETs, which have been highlighted in the prior paragraphs, may be found everywhere in the manufacturing, healthcare, communication, and information processing sectors. There is yet to be sufficient knowledge of their consequences for business, policy, environmental, ethical, and social concerns (Groen and Walsh 2013).
One problem is that certain advances in technology may undermine the pursuit of long-term, sustainable development efforts (Cao et al., 2021). While the use of new and emerging technologies may accelerate progress toward sustainability, it can also disrupt markets and economies, aggravate social divisions, and pose moral issues (Qiu et al., 2021). Because the field of sustainability research is still not properly defined, developed, or applied, many businesses in developing economies today lack the knowledge needed to successfully adopt and adapt sustainable strategies (Luthra et al., 2017). The foregoing remarks demonstrate a strong necessity to address sustainability to create balance and provide emerging countries the possibilities for long-term growth. The issue of safety, cleanliness, and sustainability is becoming bigger concerns in the industrial sectors, where Covid 19 has significant effects in various sectors. In order to successfully face the current situation, emerging technologies may be useful (Sigov et al., 2022).
2.2 Proposed attributes
COVID-19 has manifold impacts on different sectors of the manufacturing industries. However, hygiene, safety, and sustainability are of major concern. ETs can undoubtedly help to address the current challenges effectively and efficiently. Li et al. (2022) have found in their study that product shortage, which causes customer dissatisfaction and extra cost, can be avoided by applying ET. Krammer et al. (2018) have also concluded that technology can be used to build a multi skilled workforce leading to enhanced competency to get strategic fit amid pandemic. Likewise, Bakalis et al. (2020) have observed that the online wing of many businesses experienced significant boom during the pandemic, which created ample job opportunity and swelled company profits. Ahmad and Zhang (2020) have found that using new forecasting methods, companies may better use inventory and avoid over- or under-stocking. ET has also enabled a better interface that allows a real time upgrade and flawless communication (Buhalis et al., 2019). According to Zafar et al. (2019), the use of many green technology innovations can help achieve sustainable use of natural resources. Reliable and secure management system based on the “latest tracking technology’ is a vital component to overcome complexity of systems and reduce emissions, effluents, and waste of manufacturing life cycle. Cherrafi et al. (2018) conclude that green supplier development (GSD) achieved by different implementation of technology is important in the green supply chain management activity. Furthermore, Demekas (2018) submit that interdepartmental integration can be achieved through modern technology. In healthcare, IT-based communication can be combined with safety practices to maintain employee safety and hygiene (Tamers et al., 2020).
The aforesaid conditions imply the necessity of selecting a subset of crucial attributes that are both pertinent to the COVID-19 pandemic and have a significant effect to glean the essential features needed for the successful deployment of emerging technologies. To identify the critical attributes that can maximize the benefit and reduce the cost of adopting ETs towards sustainable recovery, this study has reviewed the published literature and taken experts’ opinion. For identifying the critical attributes, a literature review was conducted utilizing many key terms: “using emerging technologies for sustainable recovery” or “emerging technologies for manufacturing industries” and “sustainability drivers assessment following COVID-19” or “possible attributes of emerging technology” or “impacts/effects of COVID-19 outbreak on sustainability and sustainable developments” or “effective deployment of ETs.” For this literature search, Google scholar and the Scopus database were used between the years 2018 and 2022. Moreover, systematic literature search (SLS) has been done to aid in the identification of the factors. The inclusion and exclusion criteria for SLS technique are presented in the Table 1.
Finally, based on the existing literature and expert opinions, twelve critical attributes of ETs have been selected. Among the twelve attributes, ten attributes are found from literature and two are found from expert opinion. The experts have been selected with diverse knowledge in advanced technology and manufacturing processes. They suggested a couple of attributes that have recently gained attention to local industrial practices. They recommend for better environmental-friendly product life management techniques since technological advances in product lifespan and disposal not only make production sustainable but also reduce production costs in the long run. Experts have also recommended enhancing the supply chain’s economic structure by expanding supply chain finance, which allows businesses to automatically optimize the whole systems to boost efficiency. Table 2 presents the selected attributes identified in the context of the COVID-19 pandemic.
2.3 Existing methods and contributions of our study
It is quite helpful to use Multi-criteria Decision-making Method (MCDM) and Multi-attribute Decision-making (MADM) techniques while formulating and choosing strategies. Considering impartial data may provide individuals with additional knowledge, allowing them to make better choices. Taking impartial information into consideration during the decision-making process clearly affects the outcome (Abdel-Basset et al., 2020). A similar issue may be observed in the problem of supplier selection: fuzzy Analytic Hierarchal Process (AHP) (Ho et al., 2021), fuzzy Technique for Order Preference by Similarity to Ideal Solution (TOPSIS) (Kannan et al., 2014), fuzzy Adaptive Resonance Theory (ART) algorithm (Sivanagaraju et al., 2021), fuzzy Vise Kriterijumsa Optimizacija I Kompromisno Resenje (VIKOR) (Bahadori et al., 2020) and fuzzy Decision Making Trial and Evaluation Laboratory (DEMATEL) (Nasrollahi et al., 2021). The important things to bear in mind with regard to creating decisions are the components of alternative sets, attribute sets, decision-making matrix, and attribute weights. In this study authors have focused on some important attributes that can improve the benefits and reduce the cost of using ETs for sustainable recovery in the manufacturing industries. This problem is a MADM due to the presence of many attributes as well as unpredictability. Thus, the authors in this study have used a unique fuzzy-TODIM hybrid method for the evaluation of the given attributes.
The contributions of this research can be summarized as follows. Firstly, some attributes for improving the benefits and minimizing the cost of using ETs for the sustainable recovery of manufacturing industries were identified through an extensive literature review. Next, authors incorporated some evaluation criteria in the process to define the weight of each attribute, which made the weight of various characteristics more reasonable. Additionally, many strategies have been developed for dealing with MCDM and MADM issues. One of the newest techniques for this purpose is the TODIM strategy, which ranks the choices by means of paired comparisons (Mahdiraji et al., 2020). In addition, the authors sought to add the capabilities to consider all potential rule interactions in the MADM process by extending the TODIM technique to include a fuzzy environment. Furthermore, the authors put forward an enhanced technique of computing to rank characteristics inside a fuzzy framework. In this research, a fuzzy-TODIM hybrid technique was used and made accessible for evaluating the provided attributes.
3 Methods
3.1 Process of fuzzy-TODIM
The ambiguity in the original TODIM was added to overcome the repercussions of bias and prejudice in the ranking of alternatives. The linguistic variables of the attribute values were represented by triangular fuzzy numbers. With these ambiguous statistics and the idea of the TODIM method, the gains and losses of each alternative were compared to each other. The overall value of each option was then calculated by comparing each alternative’s dominance rate to that of the other alternatives. Therefore, the proposed fuzzy-TODIM method is described in the following (Tosun and Akyüz 2015; Zhang et al., 2022):
Step 1. Expression of the linguistic factors
Step 2. Assessment of the criteria and options
Step 3. Defuzzification of the fuzzy criteria weights
Step 4. Determination of gains and losses
Step 5. Calculation of the relative weight of each criterion based on the reference criterion
Step 6. Construction of the dominance degree matrix for every criterion
Step 7. Determination of the total dominance degree matrix
Step 8. Determination of the total value of each alternative and mark the alternativesThe summary is depicted in Figure 1.
3.2 Samples and data
With a view to selecting the best fit attributes based on the experience of experts, a questionnaire was prepared containing relative information (extracted from the literature assessment) and sent to industry experts and researchers. The sampling of the experts was done based on relevant manufacturing industries to fulfil purposive sampling, where ET is already in application or about to start. The experts were also chosen based on different manufacturing industry category-small, medium, and heavy, consisting of both mature and ET. The experts also had hands-on experience in shielding their industry against the impact of COVID-19 by taking prompt measure for scaling up the usage of ET. The most impactful attributes (Table 2) were extracted from the responses of the 160 (47%) respondents (Table 3) out of the 340 experts contacted.
Again, another extensive literature revealed the suitable criteria against which the attributes were evaluated accordingly. A team was formed consisting of four (4) experienced people ranging from managing director to CEO to confirm the selection and weight of the criteria extracted from the literature review. The criteria included: K1- Cost, K2-Viability of Technology, K3-Time Span, K4-Alignment with Company Goal, K5-Political Aspect, K6-Geographical Position, and K7-Demographical Aspect (Davies et al., 2021; Dubey et al., 2021; Gupta et al., 2021; Qyyum et al., 2021).
After the validation of the attributes and criteria, the decision makers were asked to complete the questionnaires as part of the quantitative evaluation process. Selective alternatives were given a triangular fuzzy number representation to facilitate decision-making, which were defuzzied afterwards during result processing.
An expert team (Table 4) of seven (7) members from diverse backgrounds and with sound knowledge on emerging trends and manufacturing industry were formed with a view to giving quantitative input on the attributes. The points of view and opinions of these experts were taken into account while executing Step 2.
4 Findings
After evaluating each attribute against every criterion using the triangular fuzzy numbers, gain and loss matrices for all single criteria are calculated using Step 3 and Step 4. The optimism index (α) in this study is set to 0.5 to provide a middle ground between the extremes of optimism and pessimism. Aggregate fuzzy weights of the criteria depicted in Table 5 are defuzzied and homogenized using Step 2 and Step 3 along with the information shown in Table 6. Step 5 is used to determine the relative weights.
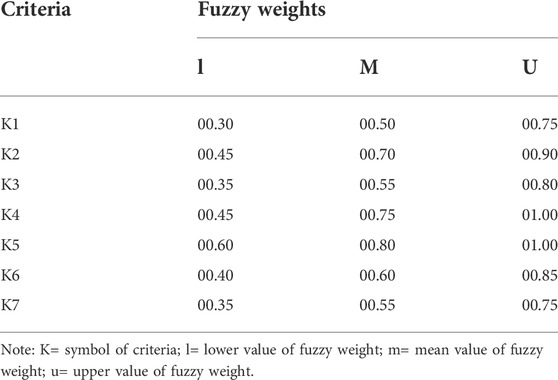
TABLE 5. Aggregated fuzzy weights of the criteria (Tosun and Akyüz 2015).
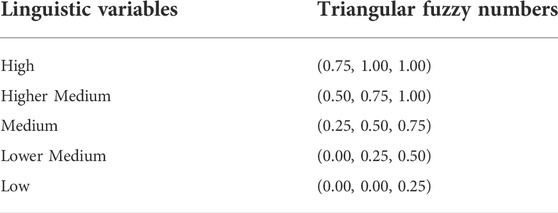
TABLE 6. Fuzzy numbers for the corresponding linguistic variables (Roghanian and Alipour 2014).
Gain and loss of the attributes, and their associated dominance matrices are constructed using Step 6, and then the overall dominance matrix is formed (Table 7) using Step 7. Furthermore, application of Step 8 and the information from Table 7 gives the overall total weightage, hence the ranking of the attributes is evaluated accordingly (Table 8).
The result shows that attribute A11 (Inventory and resource management) is the most important one followed by attribute A8 (Development of skilled workforce) and A2 (Supplier and service management capability). These attributes (A11, A8, and A2) exhibit large weights and should be addressed immediately and with outmost concern. These attributes are ranked with θ taken as 1 and their values are evaluated to account for losses included in the global value with their respective real values.
To observe the sensitivity and effect of parameter θ, the value of this parameter is changed from 1 to 5, which is an acceptable value range for this actuation factor (Tosun and Akyüz 2015). The result for changing the parameter θ is given below in Table 9.
From the Table 9, it can be observed that attribute A11 is always ranked highest. For θ =2, both A11 and A8 share the same importance. For θ =4, A3 is replaced by A12, only where the first phase of attributes with higher priority gets changed. However, for the other values of θ, the first five attributes remain unchanged though their order varies. Hence, the robustness of the model is ensured.
4.1 Discussion of the findings
After applying the fuzzy-TODIM technique based on the fuzzy input from the experts, the necessary attributes are ranked to find out the crucial attributes required to maximize the benefit and minimize the cost of ET amid this pandemic with respect to the coherent criteria. The criteria with relatively high importance are discussed as follows.
The first attribute with the highest importance is “inventory and resource management” (A11). Inventory plays a crucial role as ET is mostly used to estimate and maintain proper level of inventory-which is essential during the disruptive production situation experienced during the pandemic. An optimum level of inventory along with adequate resource helps to ensure responsiveness and reduces cost significantly. This explains the ranking of this attribute as the most important for the efficient deployment of ETs (Ferrari et al., 2021).
Attribute “development of skilled workforce” (A8) is the next most important attribute. Obviously, the ultimate success of applying technology largely depends on how zealous and technically sound the workforce is. During the covid time, many workers were laid off due to factory closure and disruption in supply chains. Since then, manufacturing industries have prioritized skill development. If the personnel are devoid of proper knowledge, deploying new technology may not yield the desired result. So, skilled workforce is a must for maximizing the yield from ET (Huy et al., 2021).
The third-ranked attribute is “supplier and service management capability” (A2). Emerging technology like AI, big data, and block chain are mainly used to connect and synchronize different branches of business. And these technologies are mostly used to link the complex network of suppliers with service management. The more flexible this link, the better the efficiency. Therefore, the capability of the service management to connect to the supplier service is essential for the success of deploying technology (Li et al., 2021).
“Sustainable usage of natural resources” (A5) is the fourth most important attribute. This pandemic makes the researcher and business-expert feel the necessity of making the efficient and just use of scarce resources. The drying up/disruption in the supply of resources due to the pandemic has created an avenue for the deployment of Big Data and other ETs for the exact utilization of natural resources in the case of urgency. It also allows new technologies to be put to more efficient and effective use (Jhariya et al., 2021).
Next in rank is the attribute “demand management and forecasting” (A3). One of the most prominent applications of ETs and data science is in demand management. Better demand management technique and greater investment in this field to forecast the unforeseen will certainly have impact on the overall efficiency of the usage of ET especially with the uncertainty associated with the COVID-19 pandemic (Hörcher et al., 2021).
“Ensuring hygiene and safety of employees” (A12) comes next in the descending order of importance of the attributes. During this pandemic, safety and hygiene of workers and technicians are a global concern. A lot of machines and interfaces need to be run by professionals for the smooth function of the applied technology. Accordingly, a safe and hygienic way of operating the technology is very essential to maximize its benefits (Laciok et al., 2021).
4.2 Implications
4.2.1 Theoretical implications
The current research has systematically and methodically identified the significant attributes for enabling the use of ET for the recovery of the manufacturing industry. The closely related research articles examined mainly discuss the implementation of ETs. However, in our work, we have explored the critical attributes required for the adoption of ETs for the recovery of the manufacturing sector. Here, have employed a fuzzy-TODIM procedure with based on wide-ranging literature review and real-life experiences of experts. Therefore, our research outcomes are efficient and compatible with implementation stage. Fuzzy-TODIM is used for the robust intake of the inputs from the experts comparing. Therefore, this research has three-faceted contributions. These include identification of the essential attributes for maximizing the benefits of ETs for rapid recovery of the manufacturing industry. In addition, we have marked out the criteria based on which the attributes will be determined by the help of the blended essence of subject expertise and field experience. The last one ranks the attributes with their corresponding importance value. Admittedly, maximizing the benefit of emerging technology is resource-heavy and encounters several challenges like financial capability, design constraints, skilled workforce, viability of implementation etc. Hence, it requires a careful consideration of all attributes from all possible aspects for the successful implementation of emerging technology, which will help to accelerate the recovery of the manufacturing industry from the challenges of the pandemic.
4.2.2 Practical implications
This research has addressed the need for a strategic analysis model to assist management in evaluating several alternatives for tailoring a policy pathway. This pathway will yield the desired beneficial output by employing ETs in terms of cost and associated features. COVID-19 has resulted in drastic shifts in the way companies and customers function. Getting past this pandemic, industries are bound to come up against the business climate not favorable as it was before the epidemic. Costing and incorporating of the recent technological norms have been thwarting the professionals since then. This research may help the management to acknowledge the difficulty in incorporating the current practice of emerging technologies in the manufacturing industry along with their behavioral aspects to confront the harm wrought by COVID-19.
Implementation of certain technology need careful consideration of circumstances; therefore, management may utilize the ranking of attributes and analyze the components to determine which attribute should to be adopted first. This research comes up with a method to aid in this process considering cost, feasibility, maturity of technology, regulations etc. Firstly, we have identified different attributes, such as “supplier and service management capability,” “inventory and resource management,” “demand management and forecasting,” etc. among many others. Our results show that “inventory and resource management” (A11) is the most important attribute that can facilitate the deployment of ET for rapid and efficient recovery of manufacturing industry. The managers, therefore, should carefully monitor the overall inventory structure, work-in-process system, finance regarding warehouse, and resource management. Controlling these factors regarding resource and inventory will reduce the cost and pave the way for better implementation of recent technology to tackle the pandemic effect.
“Development of skilled workforce” (A8) ranks as the second most important attribute it has always been a prime factor for ensuring the overall development of an industry. Managers must investigate the development and learning progress of their respective workforce along with their learning curve for the new and ongoing technological trends. It is, indeed, a crucial attribute to combat the challenges of COVID-19. Thirdly, managers should have a focus on “supplier and service management capability” (A2). A strong backend connection based on reliable supplier connection and service channel may handle any form of disruption caused by both internal and external factors. In short, this research will not only help the managers in selecting the attributes but also with the priority list-to focus on which attributes should be deployed in the first stage.
5 Conclusion
This research aimed to evaluate the critical attributes required for the effective and efficient deployment of ETs. Thus, Fuzzy-TODIM (Portuguese abbreviation of “Interactive and Multi-Criteria Decision Making”) method was effectively used to evaluate the importance of the attributes. The result reveals that “Inventory and Resource Management” is the most significant attribute for exploiting the optimal usage ETs. Industrial managers are expected to put their efforts in inventory and resource management policies for optimal use of ETs in the manufacturing industry.
This research has some limitations, which offers windows for future research. First, the analysis performed in this study was carried out based on manufacturing industries only. Other industrial sectors like food, healthcare, agricultural, mining etc. can be examined in future by using similar approach. Second, the inputs used in the research were the opinions of experts. Therefore, there might be some element of human biases in our results. To convey the uncertainty of decision information produced from subjective judgments and to increase the ability to rank alternatives, the suggested decision-making framework may be examined under the intuitionistic fuzzy environment and hybrid models like neutrosophic TODIM (Pramanik and Mallick 2019), Pythagorean fuzzy TODIM (Ren et al., 2016) approach as future work.
Data availability statement
The original contributions presented in the study are included in the article/supplementary material, further inquiries can be directed to the corresponding authors.
Ethics statement
Ethical review and approval was not required for the study on human participants in accordance with the local legislation and institutional requirements. Written informed consent from the participants was not required to participate in this study in accordance with the national legislation and the institutional requirements.
Author contributions
Credit authorship contribution statement SN: Conceptualization, Methodology, Software, Formal analysis, Investigation, Resources, Writing—original draft, Writing—review, and editing. AH: Conceptualization, Methodology, Software, Formal analysis, Investigation, Resources, Writing—original draft, Writing—review, and editing. SS: Conceptualization, Methodology, Software, Formal analysis, Investigation, Resources, Writing—original draft, Writing—review, and editing. SA: Conceptualization, Methodology, Software, Formal analysis, Investigation, Resources, Writing—original draft, Writing—review, and editing. AA: Investigation, Resources, Writing—review, and editing. HT: Conceptualization, Methodology, Software, Formal analysis, Investigation, Resources, Writing—original draft, Writing—review, and editing
Conflict of interest
The authors declare that the research was conducted in the absence of any commercial or financial relationships that could be construed as a potential conflict of interest.
Publisher’s note
All claims expressed in this article are solely those of the authors and do not necessarily represent those of their affiliated organizations, or those of the publisher, the editors and the reviewers. Any product that may be evaluated in this article, or claim that may be made by its manufacturer, is not guaranteed or endorsed by the publisher.
References
Abdel-Basset, M., Mohamed, R., Sallam, K., and Elhoseny, M. (2020). A novel decision-making model for sustainable supply chain finance under uncertainty environment. J. Clean. Prod. 269, 122324. doi:10.1016/j.jclepro.2020.122324
Ahmad, T., and Zhang, D. (2020). A critical review of comparative global historical energy consumption and future demand: The story told so far. Energy Rep. 6, 1973–1991. doi:10.1016/j.egyr.2020.07.020
Attaran, M., and Gunasekaran, A. (2019). Blockchain-enabled technology: The emerging technology set to reshape and decentralise many industries. Int. J. Appl. Decis. Sci. 12, 424–444. doi:10.1504/ijads.2019.102642
Bahadori, M., Hosseini, S. M., Teymourzadeh, E., Ravangard, R., Raadabadi, M., and Alimohammadzadeh, K. (2020). A supplier selection model for hospitals using a combination of artificial neural network and fuzzy VIKOR. Int. J. Healthc. Manag. 13, 286–294. doi:10.1080/20479700.2017.1404730
Bakalis, S., Valdramidis, V. P., Argyropoulos, D., Ahrne, L., Chen, J., Cullen, P. J., et al. (2020). Perspectives from CO+RE: How COVID-19 changed our food systems and food security paradigms. Curr. Res. food Sci. 3, 166–172. doi:10.1016/j.crfs.2020.05.003
Benedict, E. T. (2020). The implication of the outbreak of COVID-19 on the world economy. Kolkata, India: Exceller Books.
Bharadwaj, A., El Sawy, O. A., Pavlou, P. A., and Venkatraman, N. (2013). Digital Business Strategy: Toward a Next Generation of Insights. MIS Q. 37, 471–482. doi:10.25300/misq/2013/37:2.3
Buhalis, D., Harwood, T., Bogicevic, V., Viglia, G., Beldona, S., and Hofacker, C. (2019). Technological disruptions in services: Lessons from tourism and hospitality. J. Serv. Manag. 30, 484–506. doi:10.1108/JOSM-12-2018-0398
Cao, J., Law, S. H., Samad, A. R. B. A., Mohamad, W. N. B. W., Wang, J., and Yang, X. (2021). Impact of financial development and technological innovation on the volatility of green growth—Evidence from China. Environ. Sci. Pollut. Res. 28, 48053–48069. doi:10.1007/s11356-021-13828-3
Ceban, F., Ling, S., Lui, L. M. W., Lee, Y., Gill, H., Teopiz, K. M., et al. (2022). Fatigue and cognitive impairment in post-COVID-19 syndrome: A systematic review and meta-analysis. Brain Behav. Immun. 101, 93–135. doi:10.1016/j.bbi.2021.12.020
Cherrafi, A., Garza-Reyes, J. A., Kumar, V., Mishra, N., Ghobadian, A., and Elfezazi, S. (2018). Lean, green practices and process innovation: A model for green supply chain performance. Int. J. Prod. Econ. 206, 79–92. doi:10.1016/j.ijpe.2018.09.031
Cirera, X., Cruz, M., Davies, E., Grover, A., Iacovone, L., Cordova, J. E. L., et al. (2021). Policies to support businesses through the COVID-19 shock: A firm level perspective. World Bank. Res. Obs. 36, 41–66. doi:10.1093/wbro/lkab001
CNN (2020). Bangladeshi garment workers face ruin as global brands ditch clothing contracts amid coronavirus pandemic - CNN.
Curren, R. (2011). “Sustainable development BT - encyclopedia of global justice,” in, ed. D. K. Chatterjee (Dordrecht: Springer Netherlands), 1048–1050. doi:10.1007/978-1-4020-9160-5_396
Davies, B., Kenia, P., Nagakumar, P., and Gupta, A. (2021). Paediatric and adolescent asthma: A narrative review of telemedicine and emerging technologies for the post‐COVID‐19 era. Clin. Exp. Allergy 51, 393–401. doi:10.1111/cea.13836
Demekas, D. (2018). “Emerging technology-related issues in finance and the IMF—a stocktaking,” in IEO backgr. Pap. Indep. Eval. Off. Int. Monet. Fund., 2019. Retrieved 2.
Donthu, N., and Gustafsson, A. (2020). Effects of COVID-19 on business and research. J. Bus. Res. 117, 284–289. doi:10.1016/j.jbusres.2020.06.008
Dubey, R., Bryde, D. J., Foropon, C., Tiwari, M., Dwivedi, Y., and Schiffling, S. (2021). An investigation of information alignment and collaboration as complements to supply chain agility in humanitarian supply chain. Int. J. Prod. Res. 59, 1586–1605. doi:10.1080/00207543.2020.1865583
Farrell, P., Thow, A. M., Wate, J. T., Nonga, N., Vatucawaqa, P., Brewer, T., et al. (2020). COVID-19 and pacific food system resilience: Opportunities to build a robust response. Food Secur. 12, 783–791. doi:10.1007/s12571-020-01087-y
Fernandes, N. (2020). Economic Effects of Coronavirus Outbreak (COVID-19) on the World Economy. IESE Business School Working Paper No. WP-1240-E. doi:10.2139/ssrn.3557504
Ferrari, A. M., Volpi, L., Settembre-Blundo, D., and García-Muiña, F. E. (2021). Dynamic life cycle assessment (LCA) integrating life cycle inventory (LCI) and Enterprise resource planning (ERP) in an industry 4.0 environment. J. Clean. Prod. 286, 125314. doi:10.1016/j.jclepro.2020.125314
Groen, A. J., and Walsh, S. T. (2013). Introduction to the field of emerging technology management. Creativity Innovation Manag. 22, 1–5. doi:10.1111/caim.12019
Gupta, N., Gupta, S., Khosravy, M., Dey, N., Joshi, N., Crespo, R. G., et al. (2021). Retracted article: Economic IoT strategy: The future technology for health monitoring and diagnostic of agriculture vehicles. J. Intell. Manuf. 32, 1117–1128. doi:10.1007/s10845-020-01610-0
Haenlein, M., Kaplan, A., Tan, C.-W., and Zhang, P. (2019). Artificial intelligence (AI) and management analytics. J. Manag. Anal. 6, 341–343. doi:10.1080/23270012.2019.1699876
Hald, K. S., and Coslugeanu, P. (2021). The preliminary supply chain lessons of the COVID-19 disruption—what is the role of digital technologies? Oper. Manag. Res. 15, 282–297. doi:10.1007/s12063-021-00207-x
Ho, J. Y., Ooi, J., Wan, Y. K., and Andiappan, V. (2021). Synthesis of wastewater treatment process (WWTP) and supplier selection via Fuzzy Analytic Hierarchy Process (FAHP). J. Clean. Prod. 314, 128104. doi:10.1016/j.jclepro.2021.128104
Hörcher, D., Singh, R., and Graham, D. J. (2021). Social distancing in public transport: Mobilising new technologies for demand management under the covid-19 crisis. Transp. (Amst) 49, 735–764. doi:10.1007/s11116-021-10192-6
Huy, D. T. N., Van, P. N., and Ha, N. T. T. (2021). Education and computer skill enhancing for Vietnam laborers under industry 4.0 and evfta agreement. Ilk. Online 20 (4) , 1033–1038. doi:10.17051/ilkonline.2021.04.112
Ivanov, D., and Dolgui, A. (2020). Viability of intertwined supply networks: Extending the supply chain resilience angles towards survivability. A position paper motivated by COVID-19 outbreak. Int. J. Prod. Res. 58, 2904–2915. doi:10.1080/00207543.2020.1750727
Jhariya, M. K., Meena, R. S., and Banerjee, A. (2021). “Ecological intensification of natural resources towards sustainable productive system,” in Ecological intensification of natural resources for sustainable agriculture (Springer), 1–28.
Kannan, D., Beatriz, A., Sousa, L. D., José, C., and Jabbour, C. (2014). Selecting green suppliers based on GSCM practices: Using Fuzzy TOPSIS applied to a Brazilian electronics company. Eur. J. Oper. Res. 223, 432–447. doi:10.1016/j.ejor.2013.07.023
Kend, M., and Nguyen, L. A. (2020). Big data analytics and other emerging technologies: The impact on the Australian audit and assurance profession. Aust. Account. Rev. 30, 269–282. doi:10.1111/auar.12305
Krammer, S. M. S., Strange, R., and Lashitew, A. (2018). The export performance of emerging economy firms: The influence of firm capabilities and institutional environments. Int. Bus. Rev. 27, 218–230. doi:10.1016/j.ibusrev.2017.07.003
Kümmerer, K., Dionysiou, D. D., Olsson, O., and Fatta-Kassinos, D. (2019). Reducing aquatic micropollutants – increasing the focus on input prevention and integrated emission management. Sci. Total Environ. 652, 836–850. doi:10.1016/j.scitotenv.2018.10.219
Laciok, V., Sikorova, K., Fabiano, B., and Bernatik, A. (2021). Trends and opportunities of tertiary education in safety engineering moving towards safety 4.0. Sustainability 13, 524. doi:10.3390/su13020524
Lai, C. C., Shih, T. P., Ko, W. C., Tang, H. J., and Hsueh, P. R. (2020). Severe acute respiratory syndrome coronavirus 2 (SARS-CoV-2) and coronavirus disease-2019 (COVID-19): The epidemic and the challenges. Int. J. Antimicrob. Agents 55, 105924. doi:10.1016/j.ijantimicag.2020.105924
Le, H. T., Haque, R. I., Ouyang, Z., Lee, S. W., Fried, S. I., Zhao, D., et al. (2021). MEMS inductor fabrication and emerging applications in power electronics and neurotechnologies. Microsyst. Nanoeng. 7, 59. doi:10.1038/s41378-021-00275-w
Lee, J., Suh, T., Roy, D., and Baucus, M. (2019). Emerging technology and business model innovation: The case of artificial intelligence. JOItmC. 5, 44. doi:10.3390/joitmc5030044
Leu, F. Y., You, I., and Tang, F. (2011). Emerging wireless and mobile technologies. Mob. Inf. Syst. 7, 165–167. doi:10.1155/2011/690987
Li, M., Geng, P. Y., Liu, G., Gao, Z., Rui, X., and Xiao, S. (2022). Uncovering spatiotemporal evolution of titanium in China: A dynamic material flow analysis. Resour. Conserv. Recycl. 180, 106166. doi:10.1016/j.resconrec.2022.106166
Li, X., Garcia-Saavedra, A., Costa-Perez, X., Bernardos, C. J., Guimarães, C., Antevski, K., et al. (2021). 5Growth: An end-to-end service platform for automated deployment and management of vertical services over 5G networks. IEEE Commun. Mag. 59, 84–90. doi:10.1109/mcom.001.2000730
Luthra, S., Govindan, K., Kannan, D., Mangla, S. K., and Garg, C. P. (2017). An integrated framework for sustainable supplier selection and evaluation in supply chains. J. Clean. Prod. 140, 1686–1698. doi:10.1016/j.jclepro.2016.09.078
Maganga, D. P., and Taifa, I. W. R. (2022). Quality 4.0 conceptualisation: An emerging quality management concept for manufacturing industries. TQM J. doi:10.1108/tqm-11-2021-0328
Mahdiraji, H. A., Zavadskas, E. K., Skare, M., Kafshgar, F. Z. R., and Arab, A. (2020). Evaluating strategies for implementing industry 4.0: A hybrid expert oriented approach of B.W.M. And interval valued intuitionistic fuzzy T.O.D.I.M. Econ. Research-Ekonomska Istraz. 33, 1600–1620. doi:10.1080/1331677X.2020.1753090
Mi, Z., Shang, Y., and Zeng, G. (2022). The impact of knowledge base on technological innovation of emerging industries from the perspective of related variety: A case study of China’s fuel cell industry. Growth Change 53, 57–75. doi:10.1111/grow.12559
Moradlou, H., Roscoe, S., and Ghadge, A. (2022). Buyer–supplier collaboration during emerging technology development. Prod. Plan. Control 33, 159–174. doi:10.1080/09537287.2020.1810759
Nandi, S., Sarkis, J., Hervani, A. A., and Helms, M. M. (2021). Redesigning supply chains using blockchain-enabled circular economy and COVID-19 experiences. Sustain. Prod. Consum. 27, 10–22. doi:10.1016/j.spc.2020.10.019
Nasrollahi, M., Fathi, M. R., Sobhani, S. M., Khosravi, A., and Noorbakhsh, A. (2021). Modeling resilient supplier selection criteria in desalination supply chain based on fuzzy DEMATEL and ISM. Int. J. Manag. Sci. Eng. Manag. 16, 264–278. doi:10.1080/17509653.2021.1965502
Patel, A. K., Choi, Y. Y., and Sim, S. J. (2020). Emerging prospects of mixotrophic microalgae: Way forward to sustainable bioprocess for environmental remediation and cost-effective biofuels. Bioresour. Technol. 300, 122741. doi:10.1016/j.biortech.2020.122741
Patel, J. K., and Pathak, Y. V. (2021). Emerging technologies for nanoparticle manufacturing. Springer. doi:10.1007/978-3-030-50703-9
Pradhan, K., and Chawla, P. (2020). Medical Internet of things using machine learning algorithms for lung cancer detection. J. Manag. Anal. 7, 591–623. doi:10.1080/23270012.2020.1811789
Pramanik, S., and Mallick, R. (2019). TODIM strategy for multi-attribute group decision making in trapezoidal neutrosophic number environment. Complex Intell. Syst. 5, 379–389. doi:10.1007/s40747-019-0110-7
Qiu, R., Hou, S., Chen, X., and Meng, Z. (2021). Green aviation industry sustainable development towards an integrated support system. Bus. Strategy Environ. 30, 2441–2452. doi:10.1002/bse.2756
Qyyum, M. A., Dickson, R., Shah, S. F. A., Niaz, H., Khan, A., Liu, J. J., et al. (2021). Availability, versatility, and viability of feedstocks for hydrogen production: Product space perspective. Renew. Sustain. Energy Rev. 145, 110843. doi:10.1016/j.rser.2021.110843
Ren, P., Xu, Z., and Gou, X. (2016). Pythagorean fuzzy TODIM approach to multi-criteria decision making. Appl. Soft Comput. 42, 246–259. doi:10.1016/j.asoc.2015.12.020
Roghanian, E., and Alipour, M. (2014). A fuzzy model for achieving lean attributes for competitive advantages development using AHP-QFD-PROMETHEE. J. Ind. Eng. Int. 10, 68. doi:10.1007/s40092-014-0068-4
Sarkis, J. (2021). Supply chain sustainability: Learning from the COVID-19 pandemic. Int. J. Oper. Prod. Manag. 41, 63–73. doi:10.1108/IJOPM-08-2020-0568
Sigov, A., Ratkin, L., Ivanov, L. A., and Xu, L. D. (2022). Emerging enabling technologies for industry 4.0 and beyond. Inf. Syst. Front., 1–11. doi:10.1007/s10796-021-10213-w
Sivanagaraju, P. D., Hussaian, M., and Govardhan, D. (2021). A review on multi attribute decision making for evaluation and selection of supplier for materials. Mater. Today Proc. 39, 296–300. doi:10.1016/j.matpr.2020.07.201
Srinivasan, R. (2008). Sources, characteristics and effects of emerging technologies: Research opportunities in innovation. Ind. Mark. Manag. 37, 633–640. doi:10.1016/j.indmarman.2007.12.003
Tamers, S. L., Streit, J., Pana-Cryan, R., Ray, T., Syron, L., Flynn, M. A., et al. (2020). Envisioning the future of work to safeguard the safety, health, and well-being of the workforce: A perspective from the CDC’s national institute for occupational safety and health. Am. J. Ind. Med. 63, 1065–1084. doi:10.1002/ajim.23183
Tang, J., Zhang, S. X., and Lin, S. (2021). To reopen or not to reopen? How entrepreneurial alertness influences small business reopening after the COVID-19 lockdown. J. Bus. Ventur. Insights 16, e00275. doi:10.1016/j.jbvi.2021.e00275
Tosun, Ö., and Akyüz, G. (2015). A fuzzy TODIM approach for the supplier selection problem. Int. J. Comput. Intell. Syst. 8, 317–329. doi:10.1080/18756891.2015.1001954
Wadher, K., Trivedi, R., Wankhede, N., Kale, M., and Umekar, M. (2021). 3D printing in pharmaceuticals: An emerging technology full of challenges. Ann. Pharm. Francaises 79, 107–118. doi:10.1016/j.pharma.2020.08.007
Yan, R., Chen, X., Li, W., and Gao, R. X. (2021). AI-enabled monitoring, diagnosis & prognosis. Chin. J. Mech. Eng. 34, 68. doi:10.1186/s10033-021-00589-w
Yazdi, A. A., Popma, A., Wong, W., Nguyen, T., Pan, Y., and Xu, J. (2016). 3D printing: An emerging tool for novel microfluidics and lab-on-a-chip applications. Microfluid. Nanofluidics 20, 50. doi:10.1007/s10404-016-1715-4
Zabel, C., and Telkmann, V. (2021). The adoption of emerging technology-driven media innovations. A comparative study of the introduction of virtual and augmented reality in the media and manufacturing industries. J. media Bus. Stud. 18, 235–266. doi:10.1080/16522354.2020.1839172
Zafar, M. W., Zaidi, S. A. H., Khan, N. R., Mirza, F. M., Hou, F., and Kirmani, S. A. A. (2019). The impact of natural resources, human capital, and foreign direct investment on the ecological footprint: The case of the United States. Resour. Policy 63, 101428. doi:10.1016/j.resourpol.2019.101428
Zeng, G., Guo, H., and Geng, C. (2022). Mechanism analysis of influencing factors on financing efficiency of strategic emerging industries under the “dual carbon” background: evidence from China. Environ. Sci. Pollut. Res., 1–20. doi:10.1007/s11356-022-22820-4
Zenghelis, D. (2021). Why sustainable, inclusive, and resilient investment makes for efficacious post-COVID medicine. WIREs Clim. Change 12, e708. doi:10.1002/wcc.708
Zhang, Z., Guo, J., Zhang, H., Zhou, L., and Wang, M. (2022). Product selection based on sentiment analysis of online reviews: An intuitionistic fuzzy TODIM method. Complex Intell. Syst. 8, 3349–3362. doi:10.1007/s40747-022-00678-w
Zhao, S., Li, S., Qi, L., and Xu, L. D. (2020a). Computational intelligence enabled cybersecurity for the Internet of things. IEEE Trans. Emerg. Top. Comput. Intell. 4, 666–674. doi:10.1109/TETCI.2019.2941757
Keywords: emerging technology, fuzzy-TODIM, sustainable supply chain, triple bottom line, COVID-19
Citation: Nur SMSA, Salman S, Habib AKMA, Ali SM, AlArjani A and Taqi HMM (2022) Improving the benefits and cost of using emerging technologies for sustainable recovery from COVID-19 in manufacturing industries. Front. Environ. Sci. 10:1025048. doi: 10.3389/fenvs.2022.1025048
Received: 22 August 2022; Accepted: 11 October 2022;
Published: 24 October 2022.
Edited by:
Khurram Shehzad, Southeast University, ChinaReviewed by:
Surapati Pramanik, Nandalal Ghosh B. T. College, IndiaJoanna Rosak-Szyrocka, Częstochowa University of Technology, Poland
Copyright © 2022 Nur, Salman, Habib, Ali, AlArjani and Taqi. This is an open-access article distributed under the terms of the Creative Commons Attribution License (CC BY). The use, distribution or reproduction in other forums is permitted, provided the original author(s) and the copyright owner(s) are credited and that the original publication in this journal is cited, in accordance with accepted academic practice. No use, distribution or reproduction is permitted which does not comply with these terms.
*Correspondence: Syed Mithun Ali, bWl0aHVuQGlwZS5idWV0LmFjLmJk, c3llZC5taXRodW5AZ21haWwuY29t; Ali AlArjani, YS5hbGFyamFuaUBwc2F1LmVkdS5zYQ==