- School of Business, Linyi University, Linyi, Shandong, China
The water environment carrying status (WECS) is the embodiment of the ability of an aquatic ecosystem to maintain healthy and stable development; as such, it is an essential factor in the sustainable social and economic development of a region. To evaluate the water environment carrying status of a region, a new Pressure-State-Response-Support (PSRS) framework is proposed firstly. Secondly, a water carrying status index system containing 21 indicators is constructed based on this new model, and a water environment carrying status index (WECSI) is introduced to describe the water environment carrying status. Then, projection pursuit and entropy methods are used to calculate the water environment carrying status index of eight cities along the Yangtze River in Jiangsu province as examples. The results reveal that although the government has made substantial effort, the pressure subsystem is still dominated the changes of water environment carrying status, the eight cities still face significant pressure. The analysis indicated that government departments should commit to alleviating pressure and monitoring and supervising surface water quality while continuing to maintain a supporting force. The proposed framework and methodology is sound and effective for measuring the carrying status of regional water environment, and the methods can provide guidance for the Ecological Environment Department in ensuring the carrying capabilities of water environment and achieving sustainable development mission at regional level.
1 Introduction
1.1 Background
Water resource is one of the most important nature resources to support human survival, socioeconomic development and the sustainability of the ecosystem. Water ecological environment system is a social-ecological systems where humans and other organisms interact with each other. With recent increases in population, urbanization, and the size of the economy, water resources have become over exploited, and the destruction of aquatic environments is causing serious water pollution crises in many regions and countries. A healthy aquatic ecological environment is a key restriction factor in sustainable social and economic development. Measuring water environment status accurately can help environmental management departments make effective decisions for environmental protection and management.
In order to measure water environment status of the water environment capacity, researchers have proposed a number of concepts such as water carrying capacity (WCC) (Magri and Berezowska-Azzag, 2019), water environmental carrying capacity (WECC) (Wang et al., 2018; Wei et al., 2019; Zhang et al., 2019), water resource carrying capacity (WRCC) (Naimi Ait-Aoudia and Berezowska-Azzag, 2016; Ren et al., 2016; Wang et al., 2021), water resource environmental carrying capacity (WRECC) (Zhou et al., 2019), water ecological carrying capacity (WECCC) (Wang et al., 2014; Bu et al., 2020), and water eco-environmental carrying capacity (WEECC) (Zhu et al., 2010). However, these concepts are given based on different research perspective, to ensure the accuracy of study, we need to define the research focus of these concepts firstly. Water environment is a status determined by a number of influencing factors, different with other concepts, water environment carrying capacity is the largest number of people and the largest economic scale that can be accommodated under the premise of meeting the requirement of water quality and quantity without destroying the ecological environment. It emphasizes more on the function of water environment system (Hu and Xie, 2022).
According to the literature, the aforementioned concepts have been used to measure the support capacity for the development of water resources and environments, defined as the maximum population size and economic scale that a water resource and an aquatic environment can sustain in a region without destroying the water ecological balance (Zhu et al., 2010; Wang and Xu, 2015; Zhou et al., 2017; Wei et al., 2019). However, evaluations using WECC, WRCC, WRECC or WECC in most research have not been consistent with these concepts. For example, Wang (Wang and Xu, 2015) classified WECC in China into different categories based on the values of WEPCC, WESCC and WERCC contributions, but assessment results were not associated with maximum population and economic scales. Such situations abound; for example Wei (Wei et al., 2019) calculated Z values to measure WECC of Wuhan in China and Bu (Bu et al., 2020) used the mean of Z value to describe WECCC of Changzhou in China.
To describe the research issues more accurately, we propose the concept water environmental carrying status (WECS) as a key index of whether socioeconomic development is coordinated with the water environment system, and whether the economics and society are sustainable for the watershed. In contrast to previous concepts, the WECS is affected by socioeconomic factors, water resource attributions, water environment natural conditions, and human activities. In order to maintain the water ecological environment balance, it would be more suitable to use such a framework to present the carrying capacity status. Most research concepts in the literature can be classified within the WECS.
1.2 Literature review
Numerous methods have been proposed to assess resource and environmental carrying capacities. These methods can be summarized as the following types: Comprehensive index evaluation (Wang et al., 2018; Bu et al., 2020; Wei et al., 2020; Wu and Hu, 2020; Peng et al., 2021), system dynamics (Feng et al., 2008; Wang et al., 2014; Yang et al., 2015; Zhou et al., 2017), multiple objective programming method (Zhu et al., 2010), principal component analysis method (Zhang et al., 2019), projection pursuit model (Zhang and Dong, 2009; Guo et al., 2018; Wei et al., 2019), variable fuzzy pattern recognition (Wang and Xu, 2015), hybrid model (Wang et al., 2021), grey clustering method (Hu et al., 2019). These evaluation methods can be classified into two types. One type is used to calculate the actual water carrying capacity, maximum population size, and the relevant economic scale; these include system dynamics (Hu et al., 2021) and multiple objective programming methods (Zhu et al., 2010). The other type is used to calculate an index that expresses the carrying status; for example, comprehensive index evaluation (Wu et al., 2021a; Peng et al., 2021), analytic hierarchy process (Wang and Xu, 2015; Wang et al., 2018), fuzzy comprehensive evaluation methods (Wu et al., 2021b), the projection pursuit model (Guo et al., 2018; Wei et al., 2019), and entropy weight methods (Ding et al., 2015). Among them, the second type—focusing on calculating an index of WECS—is more suitable for the research objective of this paper, it can integrate all of indicators into one comprehensive index, which reflect the natural resources, social, economic, technical, environmental, meteorological and other indicators’ influences to the water environment carrying status.
The most popular assessment method in water environment carrying status is comprehensive index evaluation (Liu and Borthwick, 2011; Yang et al., 2015) in which an appropriate indicator system is used as a basis for accurate assessment (Wei et al., 2019). In order to construct the indicator system, some frameworks have been used: PSR (Pressure-State-Response) (Huang et al., 2011; Zhou et al., 2013; Lai et al., 2022), DPSIR (Driving force-Pressure-State-Impact-Response) (Pirrone et al., 2005; Pandey and Shrestha S, 2016; Mosaffaie et al., 2021), DPSIRM (Driving force-Pressure-State-Impact-Response-Management) (Guo et al., 2018), eDPSIR (enhanced-Driving force-Pressure-State-Impact-Response) (Niemeijer and de Groot, 2008a), and VPOSRM (Vigor-Pressure-Organization-State-Resilience-Management) (Wei et al., 2019), which are widely used in research on environmental impacts, sustainable development, and environment management strategies. All these frameworks emphasize causality among relevant factors in environmental systems and reveal causal chains or networks that affect the environmental status (Niemeijer and de Groot, 2008b). Among these frameworks, PSR and DPSIR are the most widely used for integrated environmental reporting and assessment (Carr et al., 2007; Niemeijer and de Groot, 2008a; Gari et al., 2015), which are evolved from Stress-Response (S-R) framework. The PSR framework focus on anthropocentric pressures and responses in its evaluation of environmental problems, it tended to push natural variability aside (Carr et al., 2007). The DPSIR was created based on PSR model, which separated the driving forces from pressure subsystem, the impact subsystem form the state system. In the DPSIR framework, the causal links start with driving forces, pass through pressure to state and impact on society, eventually leading to human’s response, with the main purpose to identify the key indicators affecting the state of environment and the impact results. While these two popular frameworks contributed to water environment carrying issues, both of them have different focus. In this study, our focus is the carrying status of water environment, which is different with the frameworks aforementioned, these make the previous framework unsuitable for our study.
Socioeconomic development increases water environment pressure and degrade water aquatic environmental state. In turn, the degradation of the water environment status restricts socioeconomic development. To maintain socioeconomic development, human implementation of response measures is needed. All of these factors form a circular chain of causal relationships. In addition, technological progress and improvement of social services enhances the carrying capacity of water environment systems (Wu et al., 2021a); this can be thought of as a support system for the water environment. There are some reports in the literature that have discussed the above views; for example, Yang (Yang et al., 2015) used pressure-support ratio to measure WECC. Shen (Shen et al., 2020) introduced the ratio of urban loads to urban carrying capacity to evaluate the urban resources environment carrying capacity; they divided the forces affecting the ecosystem into “supporting” and “pressure” types. Wu (Wu and Hu, 2020) established a comprehensive system of evaluation to assess ecological carrying capacity. Different with the research object of PSR and DPSIR, the focus of this study is the evaluation of water environment carrying status, which is determined by pressure, response and support. To make the characterization of the causal chain more complete, systematic, and reasonable, a novel framework, “pressure-state-response-support” (PSRS), is proposed herein.
Among methods using the comprehensive evaluation index to evaluate carrying capacity, the entropy weight (EW) method calculates a comprehensive index based on the amount of information provide by each index, which is widely used. This method can guarantee the objectivity of the evaluation, and is an ideal scale in multi-objective decision-making and evaluation (Wu et al., 2021a). However, the comprehensive index value calculated by this method is more easy influence by the discreteness of sample data, which brings about the instability of the indicator weight. The projection pursuit (PP) method is widely used in the evaluation of resources, environments and sustainable development problems; this method projects the high-dimensional data into low-dimensional space by searching for the best projection vector to reflect the information contained in the high-dimensional data (Wei et al., 2019). This method is more suitable for multi-index evaluation problems, but it has a difficulty in finding the global optimal value. To make the evaluation result more reliable and stable, we firstly use the two methods separately to evaluate the WECS. If there is no significant difference between the results, we integrated the results of the two methods as the final evaluation results.
1.3 Research purpose and innovations
To standardize the research perspective of WECC, the WECS concept was used to deconstruct WECC from the perspective of carrying capacity status; this was done in order to consider the anthropogenic and natural influences on water environment status more appropriately. By coupling human activities with water resource and environment in the analysis of the WECS, a novel conceptual framework containing four subsystems of Pressure, State, Response, and Support was proposed on the basis of the PSR model. This framework, called PSRS, was used to accurately describe causal relationships of subsystems and major influencing factors of environmental status. Based on the PSRS framework, we established the WECS evaluation index system. The proposed evaluation index system based on novel PSRS model was used to evaluate eight cities’ WECS along the Yangtze River in Jiangsu Province of China. This paper adds to existing research in the following aspects:
• To deconstruct the WECC from the perspective of carrying status, the concept of “water environment carrying status” was proposed to normalize the research on water environment carrying capacity. This is determined by the pressure, response, support force, and their interactions with the social economy subsystem, natural water resource and environment condition, and habitat needs pressure.
• A novel PSRS concept framework model was proposed to describe the causality chain between different subsystems, and it is more suitable than other frameworks proposed by previous researchers.
• Based on the novel framework, an evaluation index system reflecting the causal relationship between human social development and water environment was constructed, which is more in line with the evaluation of water environment carrying status.
• Taking eight cities along the Yangtze River in Jiangsu province as example, the proposed PSRS framework, evaluation index system, and evaluation methods are used to assess the WECS to verify the effectiveness of the proposed framework, index system and methods. We can expand the research scope to other regional WECS evaluation.
The remainder of this paper is organized as follows: a novel PSRS framework and WECS evaluation index system are presented in Section 2; the evaluation methods of WECS are presented in Section 3; the application of proposed framework and methods is given in Section 4; and in Section 5 conclusions and future work are discussed.
2 The PSRS framework and WECS evaluation index system
The water environment carrying status is determined according to two aspects. The first consists of the nature attribute determined by the self-organization and self-regulation of the water environment; the second consists of social attributes defined as factors that are disturbed by economic and social activities. In this paper, we mainly consider the interference of economic and social actives on the water environment.
2.1 A novel pressure-state-response-support (PSRS) model framework for assessing WECS
Along with the development of society and economy, humans inevitably consume water resources and discharge pollutants into water; this exerts pressure on the water environment. At the same time, habitat requirements also bring pressures to the water environment. On the one hand, pressure from human social and economic activities on the water environment alters the state of the water environment; on the other hand, responses are made to control and address water environmental issues by humans to alleviate the pressures and change the status of the water environment. In addition, there is another force, called support, that affects the regional water environment status. The ideal state of WECS is to maintain a dynamic equilibrium of pressure-response-support forces; in other words, to maintain the coordination between human activities and water resources environment. This logic is consistent with the PSR framework. However, another force, namely support, affects the state of the water environment but has often been neglected. The WECS is not only a reflection of the natural resources’ environment characteristics but is also influenced by human society development, economic and technology development, and the protection status of the aquatic ecological environment. Coordinated development of the four parts is a precondition for the sustainable development of the society, economy, and the water ecological environment system. Based on the causal logic, a novel PSRS framework that consists of Pressure-State-Response-Support and includes causal relationships and the major influencing factors of environmental status is proposed and shown in Figure 1.
In contrast to other assessment frameworks, PSRS has four compartments: a pressure subsystem (P), a state subsystem (S), a response subsystem (R), and a support subsystem (S). Water environment status is determined by human and habitat pressures, supporting elements from natural resources and human social-economic conditions, and human response activities to maintain the balances between human socioeconomic growth and environment protection; the key point is to maintain the ecosystem equilibrium. Detailed descriptions of the subsystems and their casual relationships within the novel conceptual framework are discussed below.
In the PSRS framework, the pressure subsystem includes the pressures of human socioeconomic activities and habitat needs; in the state subsystem, the indicators represent the states of water environment system; the response subsystem includes countermeasures taken by human beings to improve the status of the water environment, and the support subsystem represents the support force from urban construction, environmental protection, and natural attributes of the water resources environment. As for the pressure subsystem, the pressures are imposed by human activities and habitat requirements. On the one hand, humans consume water resources to maintain their essential life activities and socioeconomic development; on the other hand, the human activities result in water pollution and deteriorate the water environment. Under the PSRS framework, indicators in the pressure subsystem are all negative; indicators in the response subsystem are mostly positive, and indicators in the support subsystem are all positive too.
2.2 WECS evaluation index system based on PSRS
Developing a proper evaluation index system is a critical step in WECS evaluation. In principle, the evaluation index system should be systematic, science-based, typical, measurable, policy-relevant, and available (Zhou et al., 2013; Bu et al., 2020). Indicators should neither be too numerous, to avoid cluttering the overview, nor too few to provide sufficient information (Mosaffaie et al., 2021). The WECS is influenced by the interactions between human activities and natural processes of aquatic environments. When constructing the evaluation index system of the WESC, we considered factors including human activities, the natural environment, the influences of natural states on human society, and the management policy. Referring to previous research and consulting experts’ opinion, based on classical and high-frequency characteristics, we chose evaluation indicators that could be obtained and were relevant to the water environment carrying capacity under each dimension to construct the evaluation index system. Due to the lack of available related data, the evaluation index system of WECS based on the PSRS framework concentrated on the 21 available but key indicators in Table 1.
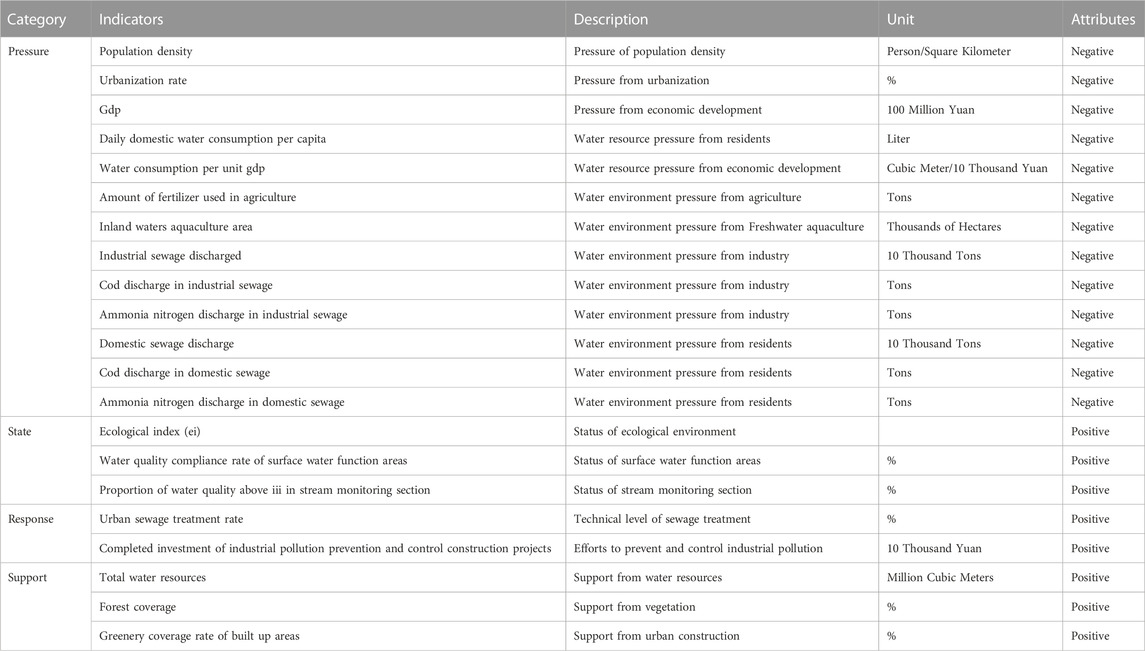
TABLE 1. Evaluation index system of Water environment carrying status based on pressure-state-response-support framework.
The pressure from humans can be classified into population, social, and economic pressures caused by human activities that affect the water resources and environment. The pressure that is imposed by the habitat requirements is difficult to measure, as there are no available data. Therefore, we only consider the human pressure in the pressure subsystem. Among the pressures on water environment from humans, pressures caused by industry, agriculture, and residents’ life activities is far greater than from other causes. To express direct and indirect impacts of human activities, this study chose 13 pressure indicators to represent the pressure from different perspectives on the water environment; these included population, urbanization, economic scale, water demand, water resource utilization efficiency, industry, agriculture, and residents’ life activities.
The state of water environmental system also affects the WECS. The Ecological index reflects the ecological environment of research region; the higher the value, the better the basic ecological environment of the region and the stronger the supporting force to the water environment. In addition, the quality of surface water function areas and stream monitoring section are two critical state indicators of the WECS.
To maintain the balance between human activity and the natural environment, human beings must take measures to reduce the damage to the water environment; for example, by improving sewage treatment rate and intensifying efforts to curb industrial pollution and control the extent of construction.
WECS is affected by Water resources and water environment endowment, both of which are support forces. In addition to these, urban infrastructure construction is another support force in the water environmental system. In the support subsystem, total water resources, forest coverage, and greenery coverage rate of build-up areas are used to represent the support force.
3 Evaluation methods of water environment carrying status
3.1 Assessment of WECS based on projection pursuit
Projection Pursuit is a statistical method. The steps of the Projection Pursuit comprehensive evaluation method are as follows.
Step 1: Standardizing evaluation indexes.
Suppose there are n evaluation objects, and each object has m indexes. To make the indexes directly comparable, we use the mini-max normalization method to normalize sample index values.
We use Eq. 1 to normalize positive indicators:
To normalize negative indicators, we use Eq. 2:
where,
Step 2: Calculating projection eigenvalues.
The projection pursuit model synthesizes m-dimensional data {
where,
Step 3: Constructing projection objective function.
The projection pursuit method requires that the local projection points of projection value zi should be as dense as possible; as a whole, the projection points should be scattered as far as possible. The projection objective function consists of three parts: the maximum of the inter class distance, the minimum of the inner class density, and the maximum entropy value in the projection direction.
The inter class distance. To extract effective information from samples to the maximum extent, projection points should be scattered as much as possible. This is represented by the maximum standard deviation of the projection eigenvalues, S(a), which is called inter class distance.
where,
The inner class density. Locally, the projection points should be clustered as much as possible. Generally, the inner class density is also called the local density and is denoted by D(a).
where, rij is the distance between samples i and j,
The projection objective function is constructed as follows:
The key of the projection pursuit method is to find the best projection direction that is most likely to represent the characteristic structure of the high-dimensional data. When evaluation objections are given, the projection objective function
This is a complicated non-linear optimization problem taking {a(j)|j = 1, 2, …, m} as optimization variables. The particle swarm optimization algorithm is used to solve the high dimensional global optimization problem in this study.
Step 4: Solving the optimal projection objective function via Particle Swarm Optimization Algorithm.
Let the population size of particles be N; the position, velocity, and fitness value of the lth
where, w is inertia weight; c1 and c2 are learning factors of the particle and population, respectively; and
The individual extremum of each particle and the global extremum of all particles are updated as follows.
where, fl(t+1) is the fitness value of particle l at time t+1; fl(pbestl(t)) is the best fitness value that particle l has experienced; and Max (pbestl(t+1)) is the particle position corresponding to the maximum f(pbestl(t)) of all particles at time t+1.
When the difference between the optimal particle fitness value at times t+1 and t is less than a set threshold value or reaches the predetermined iteration number, the algorithm is finished. The projection direction is the optimal projection direction a*.
Step 5: Calculating evaluation values.
Substituting the optimal projection direction a* obtained in step 4 into Eq. 12, the value of Ei can be obtained, representing the WECS evaluation value of the sample. We name this value be water environment carrying status index (WECSI). The greater the WECSI, the better the water environment carrying status of the region. As a relative index, WECSI can only represent the relative level and cannot be used to represent the absolute value of the carrying status of water environment.
3.2 Calculation process of the BP method based on the particle swarm optimization (PSO) algorithm
The calculation process for the BP-PSO method is shown in Figure 2. The calculation was implemented based on the MATLAB programming platform.
3.3 Assessment of WECS based on the entropy weight method
The entropy weight method is a mathematical method used to calculate the weight of an indicator that only depends on the discreteness of the sample data. The greater the dispersion of the index, the greater weight of this index in the evaluation of the sample. The steps of the entropy weight method for assessing the WECS are as follows.
Step 1: Standardizing evaluation indexes.
The same as in the projection pursuit method, we use Eqs 1, 2 to normalize positive and negative indicators, respectively.
Step 2: Determining the entropy of an index.
The entropy is calculated as:
where, ej(j = 1, 2, …, m) is the entropy of the jth index; and
Step 3: Calculating the entropy weight of index
Step 4: Calculating evaluation values.
Substituting the entropy weight wj obtained in step 3 into Eq. 15, the value Ei denoting the WECSI is obtained.
4 Case study: Eight cities along the Yangtze River in Jiangsu Province
4.1 Studied background
The Yangtze Basin stretches across eastern, central and western China, covering nine provinces and two municipalities. With its unique advantages, the regional development of the Yangtze River Basin plays an extremely important role in the construction of a new development pattern in China. Jiangsu province (30°45′–35°08′N, 116°21′–121°56′E), located in the eastern part of the Yangtze River Basin and containing numerous lakes, has 13 prefecture-level administrative regions. By the end of 2019, Jiangsu had 107200 square kilometers of total area and 80.7 million permanent residents, making it the most densely populated province in China. Its per capita GDP, regional development, and livelihood index all rank first in China, and it has reached the level of a “middle and upper class” developed country. It is the only province in China where all its prefecture-level administrative regions are among the top of all cities. In the process of promoting Yangtze River Belt development, the status of the water environment and water ecology of the Yangtze River Basin has become increasingly prominent. In this context, we considered eight cities along the Yangtze River as typical research objects of the Yangtze River Basin to evaluate their WECS, and we expect the analysis to provide reference experience for watershed water environmental management and sustainable development. The location of the eight surveyed cities are displayed in Figure 3.
4.2 Data sources
In 2012, to promote balanced economic, political, cultural, social and ecological progress, the Chinese government unveiled strategic plans named the “Five-sphere Integrated Plan” for the new era at the 18th National Congress of the Communist Party of China, in which the strategic position of ecological civilization construction has been defined. In this context, this study used data from eight cities in Jiangsu Province along the Yangtze River from 2012 to 2019. We collected the statistics for eight cities concerning the WECS indexes from the Jiangsu Province Statistical Yearbook (2012–2019), the City Statistical Yearbook (2012–2019) of each city, the Jiangsu Province Water Resources Bulletin (2012–2019), the Water Resources bulletin (2012–2019) of each city, the Jiangsu Province Environmental Statistics Yearbook (2012–2019), and the Environmental Statistics Yearbook (2012–2019) of each city.
4.3 Evaluation results analysis of WECS by the PP and EW method separately
4.3.1 WECS evaluation of eight cities along the Yangtze River in Jiangsu Province
The WECS of eight cities along the Yangtze River in Jiangsu province was evaluated from two perspectives. One was an evaluation of the WECS of each city from 2012 to 2019 from a single city view; the other was an evaluation of the WECS of eight cities in each year from a horizontal year view. The WECSI was calculated by applying the methods of projection pursuit and entropy weight as detailed in Section 3.
From the single city perspective, the original data
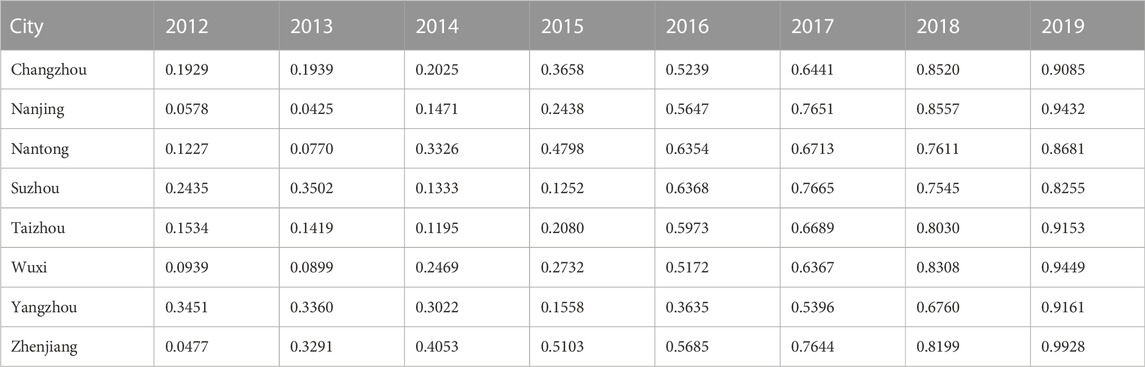
TABLE 2. Water environment carrying status index of eight cities along the Yangtze River in Jiangsu province from the city view by the projection pursuit method.
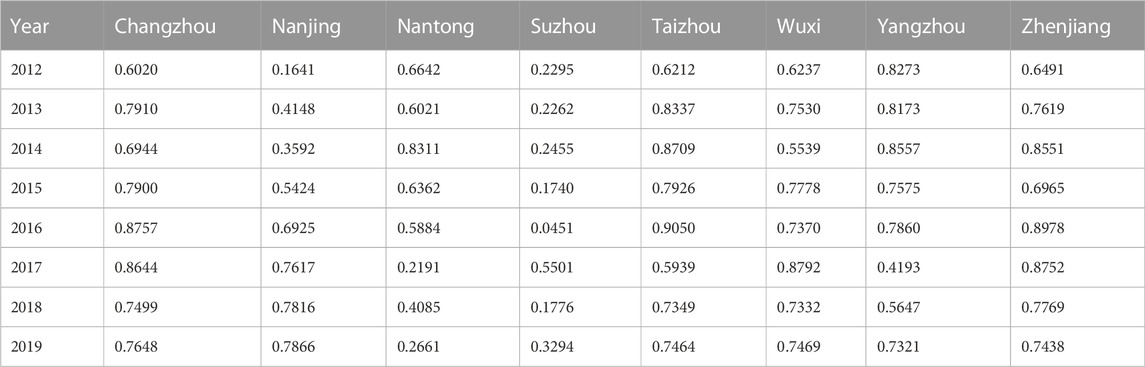
TABLE 3. Water environment carrying status index of eight cities along the Yangtze River in Jiangsu province from the horizontal year view by the projection pursuit method.
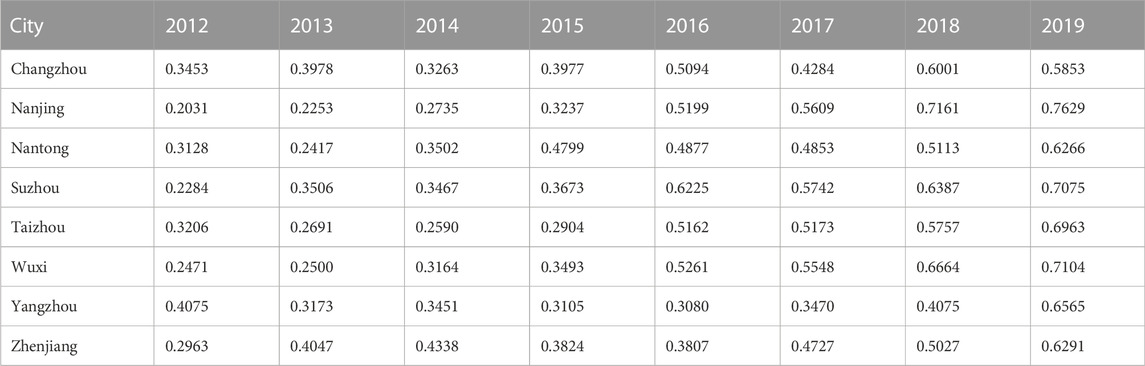
TABLE 4. Water environment carrying status index of eight cities along the Yangtze River in Jiangsu province from the city view by the EW method.
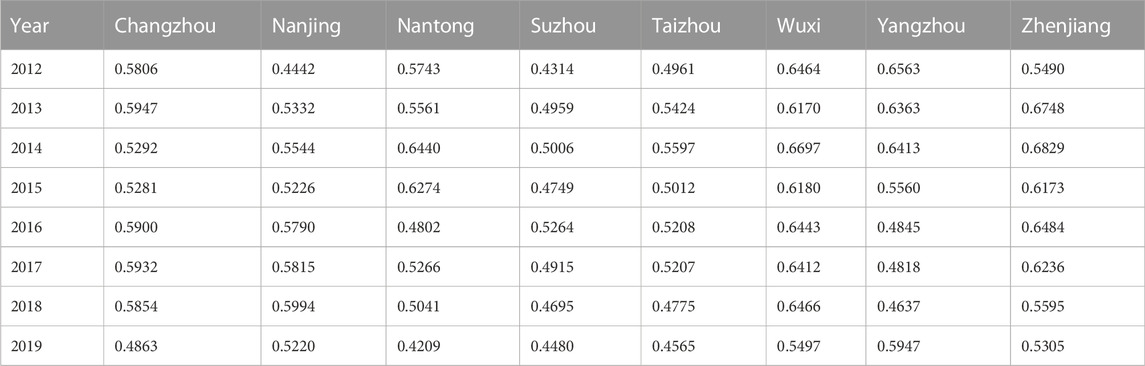
TABLE 5. Water environment carrying status index of eight cities along the Yangtze River in Jiangsu province from the horizontal year view by the EW method.
4.3.2 Trend analysis of WECS from city view
WECSI represents the level of water environment carrying status of a region. In order to compare the evaluation results of WECS more intuitively, the WECSI of eight cities obtained by the PP and EW methods from a single city view are shown in Figure 4.
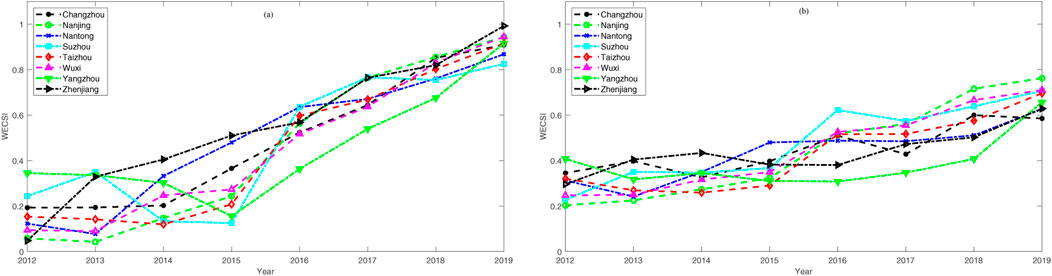
FIGURE 4. The variation of city water environment carrying status from city view based on projection pursuit method (A) and Entropy Weight method (B) respectively.
Figure 4A shows the evaluation results using the PP method, and Figure 4B shows the evaluation results by the EW method. In general, the water environment carrying status obtained from two different methods had the same yearly upward trend from 2012 to 2019. The WECS of Yangzhou was the lowest in the eight cities; Changzhou, Nantong, and Taizhou are in the middle class; Nanjing, Suzhou, and Wuxi had better carrying status, and the WECSIs calculated by the two methods for Zhenjiang are quite different.
As shown in Figure 4A, the WECSI values of these eight cities maintained a clear upward trend with the exception of Suzhou, whose WECSI fluctuated. Over the research period, the growth rate of WECSI was fairly similar in different cities. In 2012 and 2013, the WECS of Nanjing city had the lowest value among the eight cities, followed by Wuxi, Nantong, Taizhou, Changzhou, Suzhou and Yangzhou. Zhenjiang’s WECS improved the most; this city was the lowest among the eight cities in 2012, but had become the best by 2019.
Figure 4B shows that the WECS of Nanjing and Wuxi steadily improved year by year; in general, the WECS of Nantong, Suzhou, Taizhou and Zhenjiang showed the same trend as Nanjing and Wuxi, but there was a downward trend in some individual years such as 2013 and 2014; the WECS of Changhzou and Yangzhou fluctuated but hand an overall upward trend. Among the eight cities, the WECS in Nanjing, Wuxi, Suzhou and Taizhou had the greatest improvement.
The projection direction
Indicators whose projection direction value exceed 0.3 in Table 6 include water consumption per unit GDP (WCG), amount of fertilizer used in agriculture (AFA), inland waters aquaculture area (IWA), industrial sewage discharged (ISD), COD discharge in industrial sewage (CIS), ammonia nitrogen discharge in industrial sewage (AIS), domestic sewage discharge (DSD), COD discharge in domestic sewage (CDS), ammonia nitrogen discharge in domestic sewage (ADS), water quality success rate of surface water function areas (QWF), proportion of water quality above III in stream monitoring sections (PSW), greenery coverage rate of built up areas (GCB), and urban sewage treatment rate (STR). These 13 indicators had the greatest impact on the water environment carrying status in the eight cities.
The entropy weights of indicators exceeding 0.06 in Table 7 include water consumption per unit GDP (WCG), amount of fertilizer used in agriculture (AFA), inland waters aquaculture area (IWA), COD discharge in industrial sewage (CIS), ammonia nitrogen discharge in industrial sewage (AIS), domestic sewage discharge (DSD), ammonia nitrogen discharge in domestic sewage (ADS), water quality success rate of surface water function areas (QWF), proportion of water quality above III in stream monitoring sections (PSW), total water resources (TWR), forest coverage (FC), greenery coverage rate of built up area (GCB), urban sewage treatment rate (STR) and completed investment of industrial pollution prevention and control construction projects (CII).
In comparing the major influence indicators obtained by the two methods, 11 indicators are the same, while ISD and CDS indicators in the PP method, and the TWR, FC and CII indicators in the EW method do not appear simultaneously. The comparative analysis shows that the evaluation results of the two methods were generally the same, and suggesting that both of them can be used to evaluate the WECS.
4.3.3 Trend analysis of WECS from the horizontal year view
Figure 5 presents WECSI values calculated by the PP and EW methods from the horizontal year view. The WESCI scale obtained by the EW method was concentrated between 0.4209 and 0.6829, while the WECSI calculated by the PP method fluctuated. Except for Taizhou and Wuxi, the WECS rankings of each city obtained by the two methods were the same.
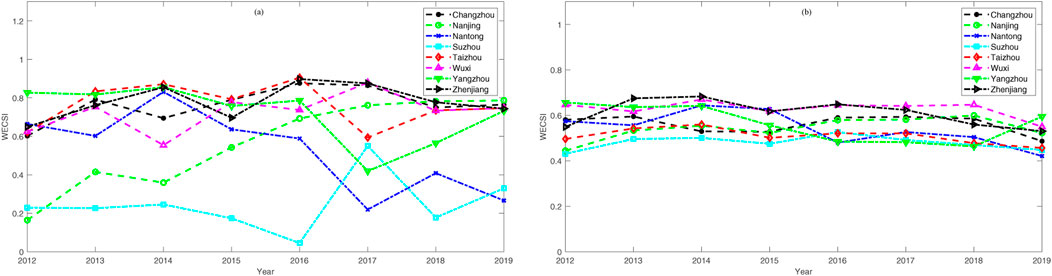
FIGURE 5. The variation of water environment carrying status from horizontal year view based on the projection pursuit (A) and EW method (B) respectively.
Figure 5A shows the variation of WECS calculated by the PP method. The WECSI values of the eight cities were ranked every year during the evaluation period. The WECS of Changzhou ranked second twice, third four times, and fifth and sixth once each. Nanjing ranked first twice, fourth, sixth and eighth once each, and seventh three times. Nantong ranked second and fourth once each, and sixth, seventh, and eighth twice each. Suzhou ranked sixth once, seventh twice, and eighth five times. Taizhou ranked first four times and fourth and fifth twice each. Wuxi ranked first, fourth, and sixth once each, third twice, and fifth three times. Yangzhou first and seventh once each, and second, fourth, and sixth twice each. Zhenjiang ranked second three times, third and fifth twice each, and fourth once. Overall, Suzhou city had the lowest WECS, followed by Nantong, Nanjing, Wuxi, Yangzhou, Changzhou, Zhenjiang, and Taizhou.
Figure 5B shows the variation of WECS obtained by the EW method. The WECSI values of the eight cities calculated by the EW method were ranked each year. Overall, Suzhou city had the lowest WECS, followed by Taizhou, Nantong, Nanjing, Yangzhou, Changzhou, Zhenjiang, and Wuxi. The WECS of Changzhou ranked third twice, fourth and seventh once each, and fifth twice. Nanjing ranked second once, fourth three times, sixth and seventh once each. Nantong ranked first, third, and fourth once each, fifth three times, and eighth twice. Suzhou ranked fifth once, seventh three times, and eighth four times. Taizhou ranked fifth and seventh once each and sixth six times. Wuxi ranked first two times, second five times and third once. Yangzhou ranked second and seventh once each, first, fourth, and eighth twice each, with clear downward trend and then an upward trend during the research period, similar to the results for the PP method. Zhenjiang ranked first three times, third twice, and second, fourth, and fifth once each.
The projection direction
Indicators whose projection direction value exceed 0.3 in Table 8 are urbanization rate (UR), GDP, daily domestic water consumption per capita (DDW), amount of fertilizer used in agriculture (AFA), industrial sewage discharged (ISD), COD discharge in industrial sewage (CIS), ammonia nitrogen discharge in industrial sewage (AIS), domestic sewage discharge (DSD), ammonia nitrogen discharge in domestic sewage (ADS), ecological index (EI), proportion of water quality above III in stream monitoring sections (PSW), forest coverage (FC), and urban sewage treatment rate (STR).
The entropy weights of indicators that exceed 0.06 in Table 9 are daily domestic water consumption per capita (DDW), amount of fertilizer used in agriculture (AFA), COD discharge in domestic sewage (CDS), water quality success rate of surface water function areas (QWF), proportion of water quality above III in stream monitoring sections (PSW), total water resources (TWR), forest coverage (FC), greenery coverage rate of built up area (GCB), urban sewage treatment rate (STR), and completed investment of industrial pollution prevention and control construction projects (CII).
4.4 Evaluation results analysis of WECS by the integrated method
4.4.1 Evaluation results of WECS by the integrated method
PP and EW are sample driven evaluation methods that are intended to reveal the classification and ranking structure of evaluation objects through the exploration of sample data.
From the analysis of part Section 4.3.2 and Section 4.3.3, the evaluation results obtained by PP and EW methods tended to be consistent; both methods can be used to evaluate the WECS of a region. The PP method is used to solve the high-dimensional non-linear problems; the method employs the projection value to reveal the structural characteristics of high-dimensional data, but it does have a certain difficulty in finding the global optimal value. The evaluation results of the EW method are determined by the discreteness of the sample data, so they are stable, but they are also very dependent. In order overcome the weaknesses of the two methods, the evaluation results of these two methods were integrated to obtain the final evaluation results for the WECS of the eight cities in Jiangsu Province. The final evaluation results of the WECSI from the single city view and horizontal year view are shown in Table 11 and Table 12; the indicator weights of different cities by the integrated method are shown in Table 10. These indicator weights are equal to the average of the square of the optimal projection direction a* and the entropy weight.
4.4.2 Analysis of the evaluation results of city WECS
In order to intuitively analyze the variation trend of WECS of eight cities from 2012 to 2019, the final results of WECSI using the integrated PP and EW method from single city and horizontal year views are shown in Figures 6A, B, respectively.
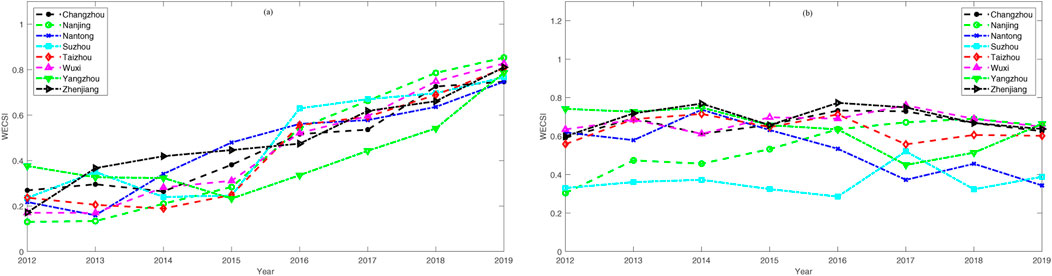
FIGURE 6. The variation of water environment carrying status index from city view (A) and horizontal year view (B) based on integrated method.
Table 11 and Figure 6A show the variation of WECS from the city view based on the integrated method. As shown, the WECSI of Nanjing and Zhenjiang city show a trend of continuous increase from 2012 to 2019. During the period from 2012 to 2019, the WECSI of Nantong, Wuxi, Yangzhou, and Taizhou all show a trend of decreasing first and then increasing. The WECSI of Nantong and Wuxi decreased slightly in 2013 and then showed annual increases. The WECSI of Yangzhou decreased annually from 2012 to 2015, then increased annually from 2015 to 2019, while the WECSI of Taizhou decreased from 2012 to 2014 annually, and then increased from 2014 to 2019 year by year. The WECSI of Changzhou and Suzhou displayed the greatest fluctuation; the values of 2013 were higher than in 2012, then decreased in 2014, and increased annually thereafter.
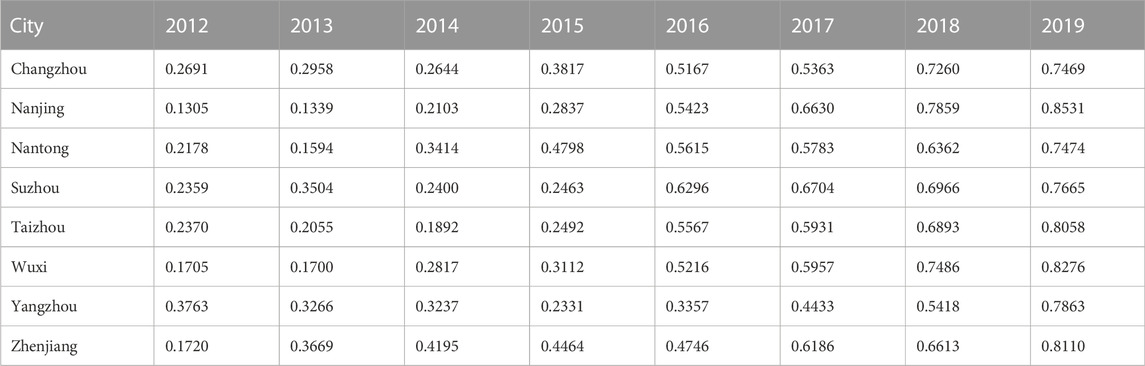
TABLE 11. Water environment carrying status index of eight cities along the Yangtze River in Jiangsu Province from the city view by the integrated method.
Table 12 and Figure 6B show the variation of WECSI in different years of the eight cities based on the integrated method. During the period 2012 to 2019, Suzhou city had the worst WECS, followed by Nantong, Nanjing, Taizhou, Changzhou, Wuxi, Yangzhou, and Zhenjiang. Combined with the analysis of temporal variation, although the WECS of Nanjing was least among the eight cities as a whole, there was significant improvement from 2012 to 2019; the WECS of Zhenjiang had been improving continuously; compared to the other seven cities, the WECS of Yangzhou changed from a relatively better status at the beginning to a relatively poor status. During the evaluation period, the WECS of Changzhou and Wuxi were always in the upper to middle ranking of the eight cities; Taizhou was in the lower-middle position, and Suzhou and Nantong ranked relatively low, although their WECS improved to some extent.
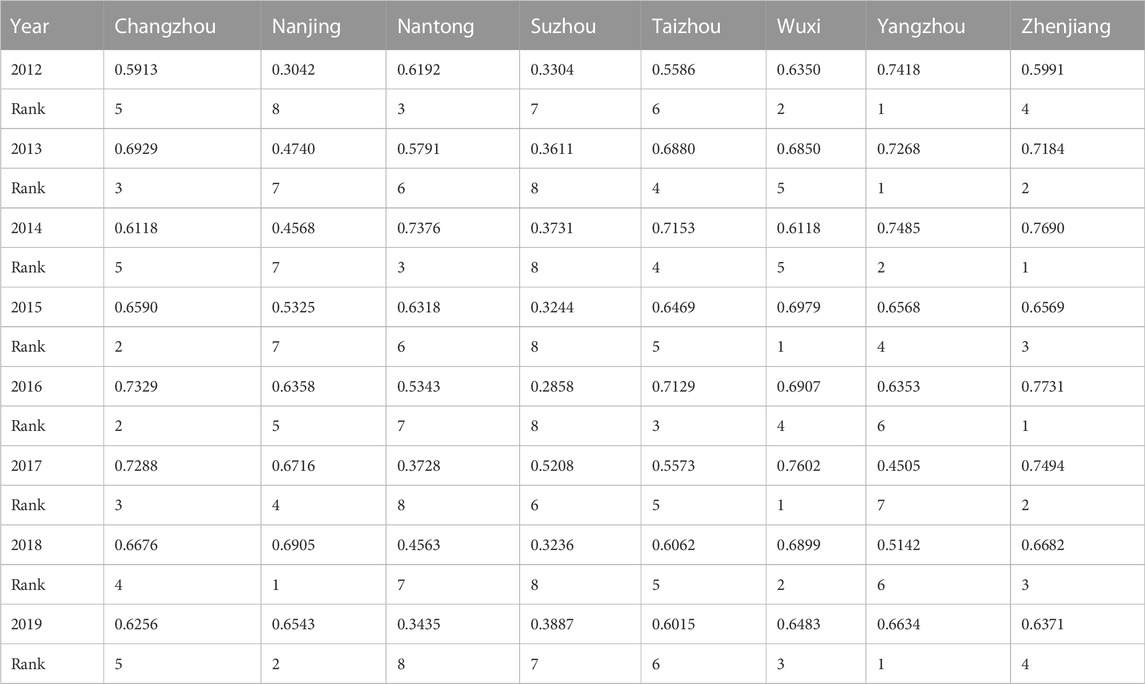
TABLE 12. Water environment carrying status index of eight cities along the Yangtze River in Jiangsu Province from the horizontal year view by integrated method.
4.4.3 Analysis of the major influencing factors of city WECS
The major influence indicators of each city are listed in Table 10. The major influence indicators of WECS in Changzhou are water consumption per unit GDP (WCG), amount of fertilizer used in agriculture (AFA), inland waters aquaculture area (IWA), domestic sewage discharge (DSD), water quality success rate of surface water function areas (QWF) and proportion of water quality above III in stream monitoring section (PSW). In Nanjing the major influence indicators are inland waters aquaculture area (IWA), COD discharge in industrial sewage (CIS), ammonia nitrogen discharge in industrial sewage (AIS), ammonia nitrogen discharge in domestic sewage (ADS), greenery coverage rate of built up area (GCB), and urban sewage treatment rate (STR). In Nantong, they are population density (PD), water consumption per unit GDP (WCG), amount of fertilizer used in agriculture (AFA), inland waters aquaculture area (IWA), COD discharge in domestic sewage (CDS) and greenery coverage rate of built up area (GCB). The amount of fertilizer used in agriculture (AFA), COD discharge in industrial sewage (CIS), domestic sewage discharge (DSD), ammonia nitrogen discharge in domestic sewage (ADS), water quality success rate of surface water function areas (QWF) and proportion of water quality above III in stream monitoring section (PSW) are the major influence indicators of WECS of Suzhou. They are inland waters aquaculture area (IWA), COD discharge in industrial sewage (CIS), ammonia nitrogen discharge in industrial sewage (AIS), domestic sewage discharge (DSD), ammonia nitrogen discharge in domestic sewage (ADS) and proportion of water quality above III in stream monitoring section (PSW) in Taizhou. In Wuxi they are inland water aquaculture area (IWA), industrial sewage discharged (ISD), COD discharge in industrial sewage (CIS), domestic sewage discharge (DSD), ammonia nitrogen discharge in domestic sewage (ADS) and water quality success rate of surface water function areas (QWF). In Yangzhou they are amount of fertilizer used in agriculture (AFA), COD discharge in industrial sewage (CIS), ammonia nitrogen discharge in industrial sewage (AIS), domestic sewage discharge (DSD), and greenery coverage rate of built up area (GCB). In Zhengjiang these are inland waters aquaculture area (IWA), industrial sewage discharged (ISD), water quality success rate of surface water function areas (QWF), proportion of water quality above III in stream monitoring section (PSW), greenery coverage rate of built up area (GCB), urban sewage treatment rate (STR), completed investment of industrial pollution prevention and control construction projects (CII).
4.5 Policy suggestions
Among the four subsystems, the pressure and response subsystems had the greatest influence on the WECS. The pressure subsystem dominates the changes in WECS; the second most important component is the response subsystem, followed by the state subsystem and the support subsystem. This indicates that the WECS of the eight cities still faces significant environmental pressure, even though the government has made substantial effort.
To improve the WECS of the eight cities based on our comprehensive analysis, we propose the following suggestions:
• The top five indicators affecting the WECS of the eight cities in Jiangsu Province are as follows: inland waters aquaculture area (Pressure), COD discharge in industrial sewage (Pressure), proportion of water quality above III in stream monitoring section (State), greenery coverage rate of built up areas (Support) and ammonia nitrogen discharge in domestic sewage (Pressure). Therefore, the department of Ecology and Environment of Jiangsu province should pay more attention to these indicators in the process of environmental control and governance. In order to improve the WECS of Jiangsu Province, the inland waters aquaculture area, COD discharge in industrial sewage and ammonia nitrogen discharge in domestic sewage should be reduced over entire range; the monitoring and supervision of water quality in stream monitoring section should be strengthened.
• For Changzhou, the government should focus on improving the water use efficiency, reducing the amount of fertilizer used in agriculture, and domestic sewage discharge; for Nanjing, COD and ammonia nitrogen discharge in industrial sewage should be reduced, and the urban sewage treatment rate should be improved; for Nantong, the government should focus on improving the water use efficiency, reducing the amount of fertilizer used in agriculture, and reducing COD discharge in domestic sewage; for Suzhou, the amount of fertilizer used in agriculture and domestic sewage discharge should be reduced, and the monitoring and supervision of water quality of surface water function areas should be strengthened; for Taizhou, the reducing of ammonia nitrogen discharge in industrial sewage and domestic sewage discharge is the governance a key point; for Wuxi, reduction of the industrial sewage discharged and domestic sewage discharge should be reduced, and strengthening the monitoring and supervision of water quality of surface water function areas are key points of government; for Yangzhou, the WECS is under great pressure, and the government should reduce the amount of fertilizer used in agriculture, ammonia nitrogen discharge in industrial sewage and domestic sewage discharge; for Zhenjiang, the government should focus on reducing industrial sewage discharged, strengthening the monitoring and supervision of water quality of surface water function areas, improving the urban sewage treatment rate, and investing in environmental protection.
5 Discussion
5.1 Main contributions
The main novel contributions of this paper are as follows.
• In this paper we proposed “water environment carrying status” concept to normalize the research on water environment carrying capacity. According to the literature, the WECC was defined as the maximum population size and economic scale that can be accommodated under the premise of meeting the requirement of water quality and quantity without destroying the ecological environment (Guo et al., 2018). However, the evaluation of WECC in most previous studies have not been consistent with WECC concept. To describe the research issues more accurately, we proposed the “water environment carrying status” concept. This status is determined by the pressure, response, support force, and their interactions with the social economy subsystem, natural water resource and environment condition, and habitat needs pressure.
• To consider the anthropogenic and natural influences on water environment status more appropriately, a novel conceptual framework, called PSRS, was proposed on the basis of the PSR model. As shown in Figure 1, the WECS of a region is influenced by the pressure, support force, response and the current state. So the PSRS framework can describe causal relationships of subsystems and major influencing factors of environmental status accurately, and it is more suitable than other frameworks proposed by previous researchers for the evaluation of WECS.
• Based on the novel framework, an evaluation index system reflecting the causal relationship between human social development and water environment was constructed. Taking eight cities along the Yangtze River in Jiangsu Province of China from 2012 to 2019 as a case study, the results of WECS evaluation were consistence with the characteristics reflected by the original data, suggesting the method is practical and feasible in theory and practice. We can expand the research scope to other regional WECS evaluation.
5.2 Limitation and future works
Due to the unavailability of data, some important indicators may have been overlooked in the construction of the evaluation index system; the WECSI introduced to describe the WECS is a relative value, and thus it cannot be compared with each other in different perspective. For these reasons, construction of scientific index system remains difficult, and this will be a focus of future research.
In order to conduct further research, we will try to use other statistical methods for evaluation of WECS and conduct multi-angle comparative analysis to find more effective evaluation method in the future research.
Data availability statements
The original contributions presented in the study are included in the article/Supplementary Material, further inquiries can be directed to the corresponding author.
Author contributions
All authors listed have made a substantial, direct, and intellectual contribution to the work and approved it for publication.
Funding
This paper is supported by Humanity and Social Science Youth Fund Project of Ministry of Education of China (20YJC630038).
Acknowledgments
The authors appreciate editor and reviewers for their insightful comments and suggestions to improve the manuscript substantially. In addition, we are very appreciating the Department of Ecology and Environment of Jiangsu Province, Jiangsu Forestry Bureau and Taizhou Water Conservancy Bureau for providing data support for this study. We thank LetPub (www.letpub.com) for its linguistic assistance during the preparation of this manuscript.
Conflict of interest
The authors declare that the research was conducted in the absence of any commercial or financial relationships that could be construed as a potential conflict of interest.
Publisher’s note
All claims expressed in this article are solely those of the authors and do not necessarily represent those of their affiliated organizations, or those of the publisher, the editors and the reviewers. Any product that may be evaluated in this article, or claim that may be made by its manufacturer, is not guaranteed or endorsed by the publisher.
References
Bu, J., Li, C., Wang, X., Zhang, Y., and Yang, Z. (2020). Assessment and prediction of the water ecological carrying capacity in Changzhou city, China. J. Clean. Prod. 277, 123988. doi:10.1016/j.jclepro.2020.123988
Carr, E. R., Wingard, P. M., Yorty, S. C., Thompson, M. C., Jensen, N. K., and Roberson, J. (2007). Applying DPSIR to sustainable development. Int. J. Sustain. Dev. World Ecol. 14, 543–555. doi:10.1080/13504500709469753
Ding, L., Chen, K.-l., Cheng, S.-g., and Wang, X. (2015). Water ecological carrying capacity of urban lakes in the context of rapid urbanization: A case study of east lake in wuhan. Phys. Chem. Earth, Parts A/B/C 89-90, 104–113. doi:10.1016/j.pce.2015.08.004
Feng, L.-H., Zhang, X.-C., and Luo, G.-Y. (2008). Application of system dynamics in analyzing the carrying capacity of water resources in Yiwu City, China. Math. Comput. Simul. 79, 269–278. doi:10.1016/j.matcom.2007.11.018
Gari, S. R., Newton, A., and Icely, J. D. (2015). A review of the application and evolution of the DPSIR framework with an emphasis on coastal social-ecological systems. Ocean Coast. Manag. 103, 63–77. doi:10.1016/j.ocecoaman.2014.11.013
Guo, Q., Wang, J., Yin, H., and Zhang, G. (2018). A comprehensive evaluation model of regional atmospheric environment carrying capacity: Model development and a case study in China. Ecol. Indic. 91, 259–267. doi:10.1016/j.ecolind.2018.03.059
Hu, A., and Xie, N. (2022). Research on dynamic evolution mechanism of watershed water environmental carrying capacity. Ecol. Econ. 38, 146–152. (Chinese).
Hu, A., Xie, N., and Zheng, M. (2019). Air quality evaluation based on grey clustering method: A case study of 74 cities in China. J. Grey Syst. 31, 1–27.
Hu, G., Zeng, W., Yao, R., Xie, Y., and Liang, S. (2021). An integrated assessment system for the carrying capacity of the water environment based on system dynamics. J. Environ. Manag. 295, 113045. doi:10.1016/j.jenvman.2021.113045
Huang, H.-F., Kuo, J., and Lo, S.-L. (2011). Review of PSR framework and development of a DPSIR model to assess greenhouse effect in Taiwan. Environ. Monit. Assess. 177, 623–635. doi:10.1007/s10661-010-1661-7
Lai, S., Sha, J., Eladawy, A., Li, X., Wang, J., Kurbanov, E., et al. (2022). Evaluation of ecological security and ecological maintenance based on pressure-state-response (PSR) model, case study: Fuzhou city, China. Hum. Ecol. Risk Assess. Int. J. 28, 734–761. doi:10.1080/10807039.2022.2081835
Liu, R., and Borthwick, A. G. (2011). Measurement and assessment of carrying capacity of the environment in Ningbo, China. J. Environ. Manag. 92, 2047–2053. doi:10.1016/j.jenvman.2011.03.033
Lou, W., Xiong, P., and Qiao, L. (2014). The mistaken in projection pursuit clustering modelling and improvement. Sci. Technol. Manag. Res. 34, 166–171. (Chinese) .
Magri, A., and Berezowska-Azzag, E. (2019). New tool for assessing urban water carrying capacity (WCC) in the planning of development programs in the region of Oran, Algeria. Sustain. Cities Soc. 48, 101316. doi:10.1016/j.scs.2018.10.040
Mosaffaie, J., Salehpour Jam, A., Tabatabaei, M. R., and Kousari, M. R. (2021). Trend assessment of the watershed health based on DPSIR framework. Land Use Policy 100, 104911. doi:10.1016/j.landusepol.2020.104911
Naimi Ait-Aoudia, M., and Berezowska-Azzag, E. (2016). Water resources carrying capacity assessment: The case of Algeria’s capital city. Habitat Int. 58, 51–58. doi:10.1016/j.habitatint.2016.09.006
Niemeijer, D., and de Groot, R. S. (2008a). A conceptual framework for selecting environmental indicator sets. Ecol. Indic. 8, 14–25. doi:10.1016/j.ecolind.2006.11.012
Niemeijer, D., and de Groot, R. S. (2008b). Framing environmental indicators: Moving from causal chains to causal networks. Environ. Dev. Sustain. 10, 89–106. doi:10.1007/s10668-006-9040-9
Pandey, V. P., and Shrestha, S. (2016). Groundwater environment in asian cities: Concepts, methods and case studies. Butterworth-Heinemann.
Peng, T., Deng, H., Lin, Y., and Jin, Z. (2021). Assessment on water resources carrying capacity in karst areas by using an innovative DPESBRM concept model and cloud model. Sci. Total Environ. 767, 144353. doi:10.1016/j.scitotenv.2020.144353
Pirrone, N., Trombino, G., Cinnirella, S., Algieri, A., Bendoricchio, G., and Palmeri, L. (2005). The driver-pressure-state-impact-response (DPSIR) approach for integrated catchment-coastal zone management: Preliminary application to the Po catchment-adriatic sea coastal zone system. Reg. Environ. Change 5, 111–137. doi:10.1007/s10113-004-0092-9
Ren, C., Guo, P., Li, M., and Li, R. (2016). An innovative method for water resources carrying capacity research – metabolic theory of regional water resources. J. Environ. Manag. 167, 139–146. doi:10.1016/j.jenvman.2015.11.033
Shen, L., Shu, T., Liao, X., Yang, N., Ren, Y., Zhu, M., et al. (2020). A new method to evaluate urban resources environment carrying capacity from the load-and-carrier perspective. Resour. Conservation Recycl. 154, 104616. doi:10.1016/j.resconrec.2019.104616
Wang, G., Xiao, C., Qi, Z., Meng, F., and Liang, X. (2021). Development tendency analysis for the water resource carrying capacity based on system dynamics model and the improved fuzzy comprehensive evaluation method in the Changchun city, China. Ecol. Indic. 122, 107232. doi:10.1016/j.ecolind.2020.107232
Wang, S., Xu, L., Yang, F., and Wang, H. (2014). Assessment of water ecological carrying capacity under the two policies in Tieling City on the basis of the integrated system dynamics model. Sci. Total Environ. 472, 1070–1081. doi:10.1016/j.scitotenv.2013.11.115
Wang, T., and Xu, S. (2015). Dynamic successive assessment method of water environment carrying capacity and its application. Ecol. Indic. 52, 134–146. doi:10.1016/j.ecolind.2014.12.002
Wang, Y., Zhou, X., and Engel, B. (2018). Water environment carrying capacity in Bosten Lake basin. J. Clean. Prod. 199, 574–583. doi:10.1016/j.jclepro.2018.07.202
Wei, X., Shen, L., Liu, Z., Luo, L., Wang, J., and Chen, Y. (2020). Comparative analysis on the evolution of ecological carrying capacity between provinces during urbanization process in China. Ecol. Indic. 112, 106179. doi:10.1016/j.ecolind.2020.106179
Wei, X., Wang, J., Wu, S., Xin, X., Wang, Z., and Liu, W. (2019). Comprehensive evaluation model for water environment carrying capacity based on VPOSRM framework: A case study in wuhan, China. Sustain. Cities Soc. 50, 101640. doi:10.1016/j.scs.2019.101640
Wu, M., Wu, J., and Zang, C. (2021a). A comprehensive evaluation of the eco-carrying capacity and green economy in the Guangdong-Hong Kong-Macao Greater Bay Area, China. J. Clean. Prod. 281, 124945. doi:10.1016/j.jclepro.2020.124945
Wu, X., and Hu, F. (2020). Analysis of ecological carrying capacity using a fuzzy comprehensive evaluation method. Ecol. Indic. 113, 106243. doi:10.1016/j.ecolind.2020.106243
Wu, Y., Ma, Z., Li, X., Sun, L., Sun, S., and Jia, R. (2021b). Assessment of water resources carrying capacity based on fuzzy comprehensive evaluation – case study of Jinan, China. Water Supply 21, 513–524. doi:10.2166/ws.2020.335
Yang, J., Lei, K., Khu, S., Meng, W., and Qiao, F. (2015). Assessment of water environmental carrying capacity for sustainable development using a coupled system dynamics approach applied to the Tieling of the Liao River Basin, China. Environ. Earth Sci. 73, 5173–5183. doi:10.1007/s12665-015-4230-0
Zhang, C., and Dong, S. (2009). A new water quality assessment model based on projection pursuit technique. J. Environ. Sci. 21, S154–S157. doi:10.1016/s1001-0742(09)60062-0
Zhang, J., Zhang, C., Shi, W., and Fu, Y. (2019). Quantitative evaluation and optimized utilization of water resources-water environment carrying capacity based on nature-based solutions. J. Hydrology 568, 96–107. doi:10.1016/j.jhydrol.2018.10.059
Zhou, D., Lin, Z., Liu, L., and Zimmermann, D. (2013). Assessing secondary soil salinization risk based on the PSR sustainability framework. J. Environ. Manag. 128, 642–654. doi:10.1016/j.jenvman.2013.06.025
Zhou, X.-Y., Lei, K., Meng, W., Khu, S.-T., Zhao, J., Wang, M., et al. (2017). Space–time approach to water environment carrying capacity calculation. J. Clean. Prod. 149, 302–312. doi:10.1016/j.jclepro.2017.02.110
Zhou, X., Luo, R., An, Q., Wang, S., and Lev, B. (2019). Water resource environmental carrying capacity-based reward and penalty mechanism: A dea benchmarking approach. J. Clean. Prod. 229, 1294–1306. doi:10.1016/j.jclepro.2019.05.004
Keywords: entropy method, PSRS framework, projection pursuit, water environment carrying status, water environmental status index
Citation: Hu A and Li Q (2022) A pressure-state-response-support framework for assessing regional water environmental carrying status. Front. Environ. Sci. 10:1023180. doi: 10.3389/fenvs.2022.1023180
Received: 19 August 2022; Accepted: 29 November 2022;
Published: 12 December 2022.
Edited by:
Xiaofei Tan, Hunan University, ChinaReviewed by:
Fengtai Zhang, Chongqing University of Technology, ChinaMuhammad Abrar Faiz, Institute of Geographic Sciences and Natural Resources Research (CAS), China
Copyright © 2022 Hu and Li. This is an open-access article distributed under the terms of the Creative Commons Attribution License (CC BY). The use, distribution or reproduction in other forums is permitted, provided the original author(s) and the copyright owner(s) are credited and that the original publication in this journal is cited, in accordance with accepted academic practice. No use, distribution or reproduction is permitted which does not comply with these terms.
*Correspondence: Aqin Hu, aqinhu@126.com